the Creative Commons Attribution 4.0 License.
the Creative Commons Attribution 4.0 License.
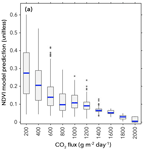
Ecosystem responses to elevated CO2 using airborne remote sensing at Mammoth Mountain, California
Kerry Cawse-Nicholson
Joshua B. Fisher
Caroline A. Famiglietti
Amy Braverman
Florian M. Schwandner
Jennifer L. Lewicki
Philip A. Townsend
David S. Schimel
Ryan Pavlick
Kathryn J. Bormann
Antonio Ferraz
Emily L. Kang
Pulong Ma
Robert R. Bogue
Thomas Youmans
David C. Pieri
We present an exploratory study examining the use of airborne remote-sensing observations to detect ecological responses to elevated CO2 emissions from active volcanic systems. To evaluate these ecosystem responses, existing spectroscopic, thermal, and lidar data acquired over forest ecosystems on Mammoth Mountain volcano, California, were exploited, along with in situ measurements of persistent volcanic soil CO2 fluxes. The elevated CO2 response was used to statistically model ecosystem structure, composition, and function, evaluated via data products including biomass, plant foliar traits and vegetation indices, and evapotranspiration (ET). Using regression ensemble models, we found that soil CO2 flux was a significant predictor for ecological variables, including canopy greenness (normalized vegetation difference index, NDVI), canopy nitrogen, ET, and biomass. With increasing CO2, we found a decrease in ET and an increase in canopy nitrogen, both consistent with theory, suggesting more water- and nutrient-use-efficient canopies. However, we also observed a decrease in NDVI with increasing CO2 (a mean NDVI of 0.27 at 200 g m−2 d−1 CO2 reduced to a mean NDVI of 0.10 at 800 g m−2 d−1 CO2). This is inconsistent with theory though consistent with increased efficiency of fewer leaves. We found a decrease in above-ground biomass with increasing CO2, also inconsistent with theory, but we did also find a decrease in biomass variance, pointing to a long-term homogenization of structure with elevated CO2. Additionally, the relationships between ecological variables changed with elevated CO2, suggesting a shift in coupling/decoupling among ecosystem structure, composition, and function synergies. For example, ET and biomass were significantly correlated for areas without elevated CO2 flux but decoupled with elevated CO2 flux. This study demonstrates that (a) volcanic systems show great potential as a means to study the properties of ecosystems and their responses to elevated CO2 emissions and (b) these ecosystem responses are measurable using a suite of airborne remotely sensed data.
- Article
(9531 KB) - Full-text XML
- BibTeX
- EndNote
The author's copyright for this publication is transferred to California Institute of Technology.
Terrestrial ecosystems have consistently taken up carbon over the past century, in excess or balancing losses due to deforestation and land use change, and this sink has grown with time (Le Quéré et al., 2016; Schimel et al., 2015). Much debate, however, has centred on the drivers of this uptake. Suggested mechanisms include nitrogen deposition (Peterson and Melillo, 1985), land use (Schimel, 1995), and the direct effects of carbon dioxide on plant growth (Norby et al., 2016). The last, which proposes that increased atmospheric CO2 yields increased photosynthetic rates, is both the most probable and the most controversial. Although a multitude of experiments have shown positive photosynthetic responses to increased CO2 consistent with the observed growth in the terrestrial sink (Drake et al., 1997), many ecologists have argued that plant growth in intact ecosystems is limited by water, light, or nutrients rather than CO2 (Körner, 2006; McGuire et al., 1995).
The Free-Air Carbon dioxide Enrichment (FACE) experiments, introduced in the 1990s, allow for CO2 fertilization of intact ecosystems by creating controlled fumigation conditions without the use of a growth chamber (Lewin et al., 1994). The FACE studies have been invaluable to our understanding of the CO2 effect, which contributes to the largest uncertainties in projections of Earth's climate. These studies have shown some consistent responses indicative of enhanced growth (Norby et al., 2016), as well as other physiological, morphological, and ecosystem consequences, but also suffer from several structural limitations. Perhaps most notably, only short-term study periods are feasible, and it is difficult to measure slower processes like plant acclimation, shifts in species dominance induced by CO2, or other long-term mechanisms mediated by changes to soil organic matter and nutrients. This is where the long-term localized emissions of volcanic CO2 can play a game-changing role in how to assess the long-term CO2 effect on ecosystems.
As a result of limited empirical evidence for the strength of CO2 fertilization effects, global carbon cycle models disagree about the significance of their associated impacts. Some models show very large CO2 effects, while others indicate a smaller or saturating effect (Kolby Smith et al., 2015). Because future predicted fossil carbon uptake is highly dependent on the strength of the simulated CO2 fertilization, any constraints on the long-term effect of elevated CO2 on ecosystems would be valuable in reducing uncertainty in coupled carbon–climate models (Friedlingstein et al., 2014).
Persistent diffuse volcanic CO2 emissions through soils result from the degassing of magma beneath volcanoes and offer a continuous natural experiment to study vegetation responses to elevated CO2 that is expansive in both space and time. These surface discharges yield broad atmospheric enhancements that transport CO2 downwind (Kerrick, 2001), resulting in swaths of variably affected plants whose periods of exposure can be over hundreds of years (Cook et al., 2001). Because volcanic CO2 emissions are a vital part of the global carbon cycle (Mason et al., 2017; Schwandner et al., 2017) and have been monitored worldwide for decades (Boudoire et al., 2017; Camarda et al., 2012; Perez et al., 2011; Gerlach, 1991), the rate and spatial distribution of these fluxes are well-understood due to an abundance of field surveys in many volcanic systems (e.g. Lewicki et al., 2014; Giammanco et al., 2007; Cardellini et al., 2003; Werner and Brantley, 2003; Hernández et al., 1998). The “kill zone” is the exact location where CO2 is emitted through the soil – a property of the soil being altered by the emission. Although the spatial distributions of CO2 emissions within tree-kill areas have been well mapped (List et al., 2005; Werner and Brantley, 2003; Pickles et al., 2001, and others), linking CO2 measurements to vegetation responses along a spatially diffuse CO2 degassing continuum (outside of the tree-kill zone) is a natural yet underutilized opportunity for studying the effects of elevated CO2 on plants (Schwandner et al., 2004). Furthermore, many CO2 emissions in volcanic systems have been ongoing for decades or centuries, thus allowing for the observation of equilibrium, long-term ecosystem responses after transient and acclimational responses have passed.
While FACE experiments may demonstrate ecological responses to increased CO2 at the outset of elevation, studies in volcanic areas can do the same on super-century scales. However, because volcanic emissions can affect entire landscapes differentially depending on the flow dynamics of the gas, they require new and innovative techniques for analysis. Remote-sensing observations allow for detailed measurements across a wide spatial extent that can be used to analyse ecological indicators of CO2 effects.
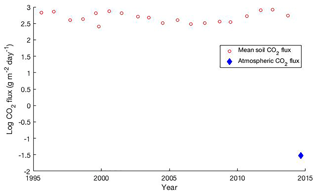
Figure 1The USGS has measured elevated soil CO2 flux at Horseshoe Lake for the past 2 decades (Werner et al., 2014). These values are consistently higher than the atmospheric CO2 measured by USGS California Volcano Observatory eddy covariance station at Horseshoe Lake at the time of the AVIRIS overpass on 21 October 2014.
Here, we present an exploratory study examining the use of airborne remote-sensing data to detect ecological responses to elevated volcanic CO2 emissions. It is a fundamental principal of volcanology that all active volcanoes emit CO2 continuously during their entire life cycle. We leveraged existing data over Mammoth Mountain, California – a much-studied volcano that has been passively emitting CO2 at high concentrations through faults and fissures on its flanks. The emissions have been measured systematically since a large earthquake swarm in 1989, and their variability has been well documented by repeated CO2 efflux mapping (Lewicki et al., 2014b; Werner et al., 2014; Farrar et al., 1995). Figure 1 shows that the elevated soil CO2 fluxes, measured by the USGS over a span of 2 decades, far exceed the atmospheric CO2 measured by a flux tower at the same site.
We developed a statistical framework for examining the relationships between field measurements of soil CO2 emissions into the air below the forest canopy and a suite of remotely sensed ecological variables. In this investigation, we aim to (i) evaluate the viability of using a passively degassing volcanic system to study the properties of ecosystems, (ii) assess the detectability of ecological responses to elevated soil CO2 emissions via airborne data alone, and (iii) present key lessons enabling future studies to extend our framework to other biomes. This methodology can be applied to any site that is exposed to elevated CO2.
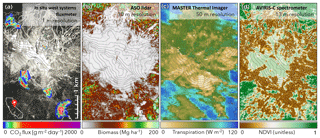
Figure 2A wealth of remotely sensed imagery has been acquired over Mammoth Mountain. Some data products used in this study include (a) maps of soil CO2 flux simulated based on accumulation chamber measurements, shown overlaid on aerial RGB image, (b) above-ground biomass derived from the Airborne Snow Observatory (ASO) lidar, (c) evapotranspiration derived from the MODIS/ASTER (MASTER) airborne simulator, and (d) normalized difference vegetation index (NDVI) derived from the Airborne Visible/Infrared Imaging Spectrometer (AVIRIS image).
2.1 Data
Airborne remote-sensing data from multiple sources have been acquired over Mammoth Mountain, California, USA, providing a substantial means to assess ecosystem structure (products derived from lidar, such as canopy height and biomass), composition (products derived from spectral data, such as vegetation indices and plant foliar traits), and function (data products derived from thermal data, such as evapotranspiration). Figure 2 illustrates several of the different products used in this study, highlighting the diversity of data sources and spatial resolutions.
Mammoth Mountain is an upper montane forest ecosystem, characterized by abundant Pinus contorta (lodgepole pine) and also by mature stands of Abies magnifica (red fir), Pinus jeffreyi (Jeffrey pine), Pinus albicaulis (whitebark pine), and Juniperus occidentalis (western juniper) (Potter, 1998). The elevation of our study areas ranged from 2700 to 2950 m. Tree-kill soils are immature High Sierra soils formed from granite, pumice, rhyolite, and obsidian parent materials (McGee and Gerlach, 1998).
2.1.1 Ground measurements
We investigated soil CO2 fluxes within five actively degassing areas on Mammoth Mountain documented by Werner et al. (2014) in 2011 and 2012, which represents a period of relatively high emissions (up to 2000 g m−2 d−1 of CO2). As described by Werner et al. (2014), fluxes were measured along fixed grid points using the accumulation chamber method (Rahn et al., 1996). In situ measurements were obtained using a West Systems® (Florence, Italy) portable flux meter equipped with a LI-COR820 infrared gas analyser. Based on statistical analysis, Werner et al. (2014) found soil CO2 fluxes measured within areas of volcanic CO2 emissions to be significantly elevated over background areas that were dominated by soil respiration of CO2. Maps of soil CO2 flux were simulated from in situ measurements at 1 m resolution using a sequential Gaussian simulation algorithm by these authors, and we resampled their data to the Airborne Visible/Infrared Imaging Spectrometer (AVIRIS) resolution (13 m) using nearest neighbour resampling. Conventionally, studies of diffuse soil degassing of CO2 on volcanoes have emphasized an understanding of the modes, locations, geometries, and changes in volcanic flank degassing for purposes of volcanological research, hazard assessment, and monitoring. In many cases, volcanologists have focused on areas associated with sufficient emissions of heat and CO2 for vegetation to have been killed off. In this study however, we focused on vegetated areas where somewhat more mildly enhanced levels of volcanic CO2 emissions into the forest ecosystems might be beneficial for plant growth rather than the reverse. As such, we investigated zones and gradients around tree-kill areas, excluding areas that were barren or contained dead trees by filtering by fractional vegetation cover, where appropriate. The tree-kill areas have local soil conditions that are not representative of the larger ecosystem. In addition, because tree-kill areas on Mammoth Mountain are largely associated with “cold” CO2 emissions, we completely avoided confounding influences of hydrothermal heat or acidic vapour emission on ecosystem response. Indeed, there is no significant H2S nor any SO2 present at soil levels at this site, and CO2 makes up ∼ 99 % of the gas by volume; see, for example, data in Werner et al. (2014) and Sorey et al. (1998), and a number of papers on volcanic degassing at Mammoth Mountain (Lewicki 2014, 2012, 2008, 2007, 2006). The remaining 1 % is made up of N2 and O2.
The use of a high spatial-resolution time-averaged (to limit the influence of varying meteorological conditions) map of canopy-level atmospheric CO2 concentration would be most applicable to assess ecosystem response to elevated atmospheric CO2 concentrations. However, such maps are unavailable. We therefore took advantage of the extensive record of soil CO2 fluxes available for Mammoth Mountain. Although the effects of elevated CO2 in the soil may be difficult to de-convolve from elevated CO2 in the atmosphere, we treat their effects uniformly. Implications of this are discussed below.
Although the airborne datasets cover a wider region, only points with associated soil CO2 flux measurements were used to derive our models. The CO2 flux measurements were spatially resampled to match the resolution of the other datasets, which resulted in small estimations with low confidence along the edges. To avoid spurious model fits, edge points with CO2 < 5 g m−2 d−1 were excluded, where the CO2 range is [0,2000] g m−2 d−1. In the remainder of this paper, analysed points with elevated CO2 flux will be referred to as eCO2.
2.1.2 AVIRIS
The AVIRIS Classic instrument acquires data from 400 to 2500 nm in 224 contiguous spectral channels. AVIRIS imagery was acquired over Mammoth in October 2014; this flight was chosen from a number of possible surveys of the area to minimize snow cover and also because of its temporal proximity to the eCO2 ground measurements. The standard level 2 (L2) atmospherically corrected reflectance data (Thompson et al., 2015) were used (available from https://aviris.jpl.nasa.gov/, last access: 19 November 2018), and the data had a spatial resolution of 13 m. These data were collected as part of the NASA HyspIRI Preparatory Airborne Campaign.
Vegetation indices
Vegetation indices are commonly used as an indicator of vegetation health and/or greenness. While many vegetation indices are related, they differ enough to be considered independent variables. For instance, some account for soil moisture, and others weight plant greenness more heavily. This was an exploratory effort in investigating the effects of CO2 on any measure of plant function, composition, and structure, and so we attempted to cover all avenues of investigation. The following indices were derived from the AVIRIS spectral data:
-
the normalized difference vegetation index (NDVI)
-
simple ratio index
-
enhanced vegetation index
-
red edge normalized difference vegetation index
-
modified red edge simple ratio index
-
modified red edge normalized difference vegetation index
-
Vogelmann red edge index 1.
Each uses a ratio between narrow bands to represent vegetation health as a single index, and all are described more fully in Thenkabail et al. (2016).
Foliar traits
The chemical composition of plants affects light interactions, especially in the short-wave infrared (Singh et al., 2015). Therefore, imaging spectroscopy can be used to map key vegetation properties, especially those affecting carbon and nutrient interactions. Spectral features, derived from data such as AVIRIS, have been shown to correlate significantly with certain chemicals and plant properties, such as carbon, nitrogen, nitrogen isotope 15, leaf mass per area (LMA), cellulose, and acid-digestible lignin (Singh et al., 2015). These properties are associated with photosynthesis, light-harvesting ability, and nutrient fluxes and can be used to characterize vegetation responses to disturbances or climate trends (Townsend et al., 2008).
The data were first corrected for their bi-directional reflectance distribution function (BRDF), using the Ross-Thick BRDF model with a quadratic volumetric scattering term (Lucht et al., 2000; Roujean et al., 1992). In situ vegetation chemical measurements, along with propagated uncertainties, were used to derive partial least squares regression models for each trait. Since these equations were derived in the nearby area of the Sierra Nevada, these equations were applied to the BRDF-corrected AVIRIS data used in this study.
Infeasible negative numbers were removed for the modelling.
2.1.3 MASTER
The MODIS/ASTER (MASTER) airborne simulator acquires data in 50 channels between 0.4 and 13 µm. We utilized the five thermal channels (10–13 µm), which had been processed to Level 2 (available from https://master.jpl.nasa.gov/, last access: 19 November 2018). MASTER data were acquired in November 2013, with a 50 m spatial resolution.
Land surface temperature
The five thermal bands from MASTER were used to calculate land surface temperature (LST) in a standard Level 2 product. The acquired data were processed to radiance using MODTRAN 5.2 for the atmospheric correction, along with a water vapour scaling method (Tonooka, 2005). The temperature emissivity separation (TES) algorithm was then used to derive LST and spectral emissivity (Gillespie et al., 1998).
The MASTER data are at coarser spatial resolution (50 m) compared to the other datasets (e.g. the working resolution for reprojection is the AVIRIS resolution of 13 m). An ideal dataset would have MASTER acquired at 13 m or similar (∼ 10 m; i.e. the scale of an individual tree canopy), but in order to build a comparable dataset for this analysis, we used two resampling methods: the standard nearest neighbour resampling and a statistically principled method proposed in Ma et al. (2018). The statistical model proposed by Ma et al. (2018) represented LST as a combination of low-dimensional random effects linked with basis functions and a Gaussian graphical model (also called Gaussian Markov random field). As demonstrated by Ma et al. (2018), this model provides a flexible and computationally efficient way to characterize potentially complex and non-stationary spatial variability. The parameters of the underlying statistical model were fitted to MASTER LST and evapotranspiration (ET) data at 50 m resolution, using maximum likelihood estimation via an expectation-maximization (EM) algorithm. The resampled data at 13 m spatial resolution were then generated via conditional statistical simulation in which we required that when aggregated back to the original coarse resolution, the resampled data matched the original MASTER data exactly.
Evapotranspiration
ET is the key water variable in ecosystem functioning, indicating plant water use and loss (Fisher et al., 2017). In this study, ET was calculated using the Priestley–Taylor model used by the Jet Propulsion Laboratory (PT-JPL) retrieval (Fisher et al., 2008), which partitions ET into canopy transpiration, soil evaporation, and interception evaporation by transforming potential ET (Priestley and Taylor, 1972) into actual ET using ecophysiological constraints. The ECOSTRESS ET retrieval system was used to incorporate MASTER LST as the thermal input (Fisher et al., 2015); additional ancillary data were incorporated from MODIS and Landsat to constrain meteorological and phenological controls on ET (Famiglietti et al., 2018; Verma et al., 2016; Ryu et al., 2011; Kobayashi et al., 2008). The final ET product used here was only the canopy transpiration component (referred to as ET throughout), as our analytical interest lies only in the vegetation response to eCO2.
2.1.4 ASO
The Airborne Snow Observatory (ASO, http://aso.jpl.nasa.gov, last access: 19 November 2018) is a coupled lidar (Riegl Q1560) and spectrometer (CASI-1500) mounted on a King Air A90 aircraft and was originally developed to monitor snow in the mountains for water resource management (Painter et al., 2016). The Riegl Q1560 is a dual-scanning lidar with two 1064 nm laser sources; each scanner is tilted in the along-track direction by and the cross-track direction by for enhanced retrieval of vertical surfaces. On 27 June 2017 ASO surveyed Mammoth Mountain, retrieving comprehensive lidar point cloud data at a mean of 7.8 pt m−2 (max. value ∼ 60 pt m−2). Riegl RiPROCESS software was then used to (a) extract point cloud data from raw waveforms (RiANALYZE) using the RiMTA Multiple Time Around algorithm and the RLMS Simple Classification Procedure for classification (SCP1), (b) georeference the point cloud (RiWORLD), and c) export the point cloud to LAS 1.2 in UTM projection (RiWORLD).
Digital terrain model
The ASO lidar point cloud data were filtered to remove outliers by applying an elevation filter to eliminate points that exceed ±100 m from a baseline digital terrain model (DTM) that was obtained from the USGS (United States Geological Survey). The ASO data processing chain includes the identification of ground and off-ground points using the multiscale curvature classification algorithm (Evans and Hudak, 2007) and the calculation of a DTM (3 m × 3 m) that corresponds to the bare soil surface as interpolated from the lidar points classified as ground. Any data voids were then filled-in using search windows that were centred on each void pixel.
Slope and aspect
The slope (steepness) and aspect (direction) were derived directly from the DTM with the terrain analysis processing tool provided by QGIS (https://qgis.org/en/site/, last access: 3 December 2018). These geo-algorithms use a first-order derivative estimation to calculate the slope angle for each pixel in degrees relative to the horizontal plane and the slope exposition in degrees anticlockwise from north.
Aspect was processed to account for circular angles, by considering
where α is the aspect derived from the DTM as described above and d is the prevailing wind direction. In the absence of local data, we assumed the prevailing wind direction to be 270∘ (e.g. Lewicki and Hilley, 2014; Lewicki et al., 2008; Anderson and Farrar, 2001). (Note that the results presented below were not sensitive to this assumption.)
Canopy height and biomass
The above-ground biomass (AGB) map (30 m × 30 m) was calculated by integrating ASO lidar measurements on forest structure and field inventory data into an allometric equation developed by Garcia et al., (2017):
where MCH and FC are lidar-derived maps of mean canopy height and fractional cover, respectively. Equation (3) was calibrated using AGB reference values derived from 69 field inventory plots located in the Stanislaus National Forest and Yosemite National Park, Sierra Nevada, California. To compute the lidar-derived maps, we first normalized the ASO lidar point cloud to calculate the effective height of vegetation by removing the effect of topography using the DTM described here above. Then, we used the normalized point cloud to calculate a canopy height model (CHM; 1 m × 1 m) by selecting the highest lidar point within each grid cell. Finally, the MCH was calculated by averaging the CHM within each 30 m cell, whereas the FC was computed as the ratio of grid cells covered by vegetation (i.e. MCH > 2 m) to the total number of cells. Note that we defined both MCH and FC with a grid cell size of 30 m in order to agree with the size of the field samples (Garcia et al., 2017). We assumed that Eq. (3) was transferable to our study site because the calibration plots are located only 80 km apart and they are both populated by vegetation of the upper montane and subalpine biotic zones.
2.1.5 Compiling the dataset
The data were first processed to create derived products, and then geolocated to the AVIRIS native resolution of 13 m. That is, for each AVIRIS pixel, the other datasets were resampled and reprojected so that every pixel is associated with a vector of remotely sensed and derived values. Datasets with finer resolution (soil CO2 flux and lidar) were averaged using the nearest neighbour principle. Derived products with coarser resolution (fractional cover, biomass, and evapotranspiration) were resampled using nearest neighbour resampling (e.g. the same biomass value may cover multiple AVIRIS pixels). Because its pixels were the largest, ET was also resampled using a statistically based method, described above in Sect. 2.1.3. We note that although the downscaling approach is robust and statistically sound, we acknowledge that our statistical estimates involving ET will include some uncertainty due to spatial resolution.
Once all pixels had been resampled, we had a total of 5520 data points. For certain experiments we found it necessary to threshold by fractional vegetation cover (FC > 0.7; n=55), although the full dataset was used wherever possible.
The dates of acquisition also differed across datasets. The soil CO2 flux datasets used in this study were measured during a peak in CO2 emissions (Werner et al., 2014), and this peak in emissions is thought to affect future plant growth. However, we are observing a snapshot of vegetation function within a zone small enough to be influenced by the same meteorological inputs, and our models have accounted for confounding factors such as slope, elevation, and aspect. Therefore, we considered measurements to be relative on a spatial scale, by comparing neighbouring pixels. The topographic confounders and the fractional cover are derived from the lidar data acquired 4 years after the MASTER data; however, we do not expect changes in the terrain during that time period, and tree presence is unlikely to have changed significantly.
2.2 Statistical modelling
The variables assessed included vegetation indices, plant foliar traits, evapotranspiration, canopy height, and biomass. Given this combination of variables, we tested whether changes in eCO2 were associated with significant changes in vegetation. We performed a series of multiple linear regressions using eCO2 as a predictor of various vegetation variables; in particular, regression ensembles build collections of linear regression models, utilizing different predictor combinations, including multiplication of predictor variables. To control for confounding variables including elevation, slope, and aspect (which are topographic proxies for temperature, moisture, and light, respectively), we included them as predictors in the model. Then, the regression coefficient estimate for eCO2 is an estimate of the change in the response variable due to a change in eCO2, holding all other variables in the model (the confounders) constant. Random forests were investigated and found to produce similar results. For ease of interpretation, we present here the results of the linear regression ensembles.
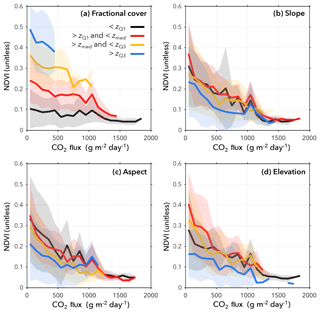
Figure 3Relationships between many ecological variables, including NDVI, and eCO2 depend highly on confounding factors. The NDVI data are partitioned into quartiles and coloured such that, if z is the confounding variable (fractional cover, slope, aspect, or elevation), then zQ1 is the first quartile of the confounding data; zmed is the median of the confounding data; and zQ3 is the third quartile. Partitioning by fractional cover yields clear separations in the response variable (a) fractional cover, as expected, since rising eCO2 will have a less measurable effect on sparse vegetation within the pixel. The impact of (b) slope, (c) elevation, and (d) aspect is less clear visually, but their contribution to the model is statistically significant.
Fractional vegetation cover (FC; derived from the lidar) was considered a proxy for vegetation presence. The geometric variables elevation, slope, and aspect were also derived from the lidar point cloud, as described above. Figure 3 illustrates the stratified behaviour of NDVI as coloured by the four confounding variables. There is a particularly clear separation for fractional cover, which reinforces an expected result: eCO2 had a negligible effect on vegetation indices and other variables over bare ground but showed higher impacts on fully vegetated pixels. Therefore, we model each vegetation variable, V, as
where C is the elevated soil CO2 flux, F is the fractional vegetation cover, S is the slope, A is the aspect, E is the elevation, and is random error. The function f(⋅) describes relationships between the predictor variables, which for this model is limited to the first-order interactions:
Our hypothesis is ; that is, that the effect of eCO2 on vegetation variable V is different from zero. Our null hypothesis is then .
Certain other confounding variables may affect the modelled relationships. The following scenarios and/or variables were also tested as confounders but did not affect the model outcome: pixel position, site number, and species (plant species were estimated by performing an unsupervised classification on the AVIRIS data). The eCO2 dataset was also shifted to simulate winds and atmospheric pressure (Ogretim et al., 2013). This did not have an impact on the results.
Additionally, diurnal patterns of mountain slope air flows may dilute and enrich the bulk air mass the trees are exposed to with respect to CO2 concentrations (Pypker et al., 2007). If these air flow patterns are strong, they may drain the local CO2 enhancement during morning and evening hours, when these flow events are usually strongest. However, due to the constant nature of these localized enhanced emissions, the gradient, if it was diluted by such effects, re-establishes itself during calmer daytime and night-time hours, as is evident by the volcanically diffuse CO2 emission signal being detectable from airborne in situ measurements above the investigated sites as well (Gerlach et al., 1999).
When evaluating the dynamics between different variables, it is assumed that the study from which our ground measurements were derived (Werner et al., 2014), covered most of the known CO2 diffuse emission areas, and so the remainder of the scene exists as a control. The control pixels were also thresholded according to the range of the confounding variables found for the eCO2 points. Therefore, we considered only control points with elevation, slope, and aspect values, respectively, between 2700 and 2950 m, less than 30∘, and less than 350∘.
Although the models were run for 42 explanatory variables (including additional vegetation indices, foliar traits, and other vegetation descriptors), for the sake of brevity we only present the best-performing variables (traits with significant p values are shown, and for all other variables, those with significant p values and R2>0.5). For the variables shown in Table 2, the p value of the eCO2 term, b1, was, for each model, <0.05 and in most cases ≪0.01. The most significant predictor was determined by ordering terms by p values.
Table 2The best-performing vegetation indices (VIs) and traits are shown with the predictive significance of eCO2 in the model and with their correlation with a regression ensemble that included elevation, slope, aspect, and fractional cover as confounding variables (n=5520). The most significant predictor was determined by ordering terms by p values.
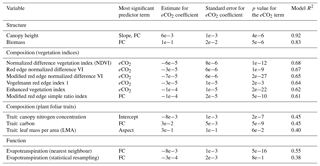
Table 3The best-performing vegetation indices (VIs) and traits from Table 2 are shown with the ordered significance of every input into the model. Since the model allows multiplication between terms, each input is ordered by first occurrence when ranking p values from smallest (most significant) to largest (least significant).
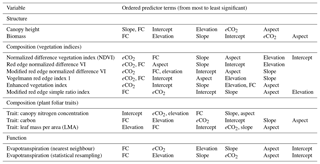
As the confounding variables are expected to drive the behaviour of ecosystem properties, a reduced eCO2 “rank” (in terms of p value significance) does not negate the impact of eCO2 in the models; in fact, each ecosystem variable was strongly influenced by increasing eCO2, given the low p values for the eCO2 coefficient in each model. The rank of each predictor variable is given in Table 3. Since multiplicative terms are allowed, two terms in a single ranking column (say, slope and fractional cover) means that the multiplication between the two terms is the term with the lowest p value. To reduce the complexity of the table, each variable is listed only once, in order of first appearance, whether singly or as a product.
4.1 Structure: canopy height and biomass
Canopy height and biomass were accurately modelled with high R2, as seen in Table 2 and Fig. 4, although eCO2 was the least significant predictor. In each case, eCO2 was still regarded as statistically significant but had lower predictive power than the topographic variables.
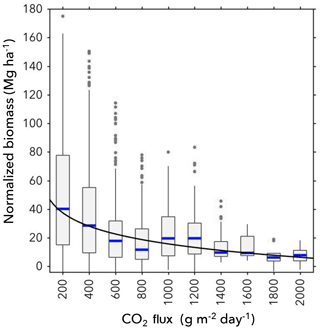
Figure 5The biomass model prediction is shown for increasing eCO2. There is high variability at low eCO2 values, but overall there is a small, but apparent, decrease in biomass with increasing eCO2.
Figure 5 shows the predictor variable eCO2 against the predicted biomass. There is variability at low eCO2 levels but overall a small decrease in biomass with increasing eCO2. This decrease appears to saturate and is better fit by a logarithmic function; however, given that interactions between terms in the model are allowed, we do not necessarily expect a linear fit, since the eCO2 contribution to the model may be multiplied by other confounding variables. There is also a decrease in biomass variance. In other words, trees exposed to higher eCO2 are more similar.
4.2 Composition: vegetation indices and foliar traits
The performance of different vegetation indices and foliar traits varied. NDVI was best modelled (R2=0.68), with eCO2 as the most significant predictor (p value of ). In general, the indices were better modelled than the traits.
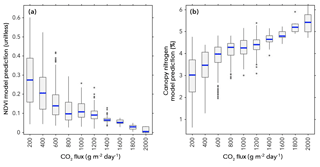
Figure 6(a) The modelled NDVI prediction is shown for predictor variable eCO2. There is a decrease in NDVI for increasing eCO2, despite larger variance at low eCO2 values. (b) The modelled canopy nitrogen concentration trait prediction is shown for predictor variable eCO2. There is a clear increase in canopy nitrogen concentration with increasing eCO2.
Figure 6 shows the predicted model for NDVI (a) and the canopy nitrogen concentration trait (b) against the eCO2 predictor variable. Modelled NDVI decreases with increasing eCO2, and there is a decrease in variance with increasing eCO2. The modelled canopy nitrogen concentration trait increases with increasing eCO2.
4.3 Function: evapotranspiration
Canopy transpiration was relatively well represented by the eCO2 model with an R2=0.55. For comparison, total ET was not well represented by the eCO2 model (R2=0.23), which is sensible, as eCO2 is expected to affect only plant transpiration and not soil evaporation. eCO2 was the second most significant predictor, with fractional vegetation cover the most significant. Given that MASTER data were originally acquired at a much coarser resolution (50 m) than the eCO2 ground data (1 m) and that both were resampled to 13 m resolution for a spatially consistent analysis, there may have been error introduced due to the resampling. This effect is seen by the much lower model fit with the statistical resampling, although the predicted models follow the same trend. In the remainder of the paper, references to ET refer to the data resampled using nearest neighbour resampling.
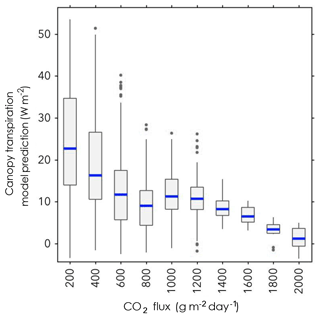
Figure 7The normalized canopy transpiration prediction is shown against predictor variable eCO2, for training data with nearest neighbour resampling. There is a clear decrease in ET for increasing eCO2, with larger variance at low eCO2 values.
Figure 7 shows the ET predicted by the model for the predictor variable eCO2. There is a decrease in ET for increasing eCO2, along with a decrease in variance.
4.4 Ecosystem synergies
Given that many of the vegetation indices and traits are only appropriate in the presence of vegetation, a fractional cover threshold of 0.7 was used for the eCO2 sample, for the sake of evaluating the dynamics between modelled variables. With this threshold, only 55 data points remained, and so the sample size is too small to make claims of statistical significance. Therefore, we present the following results as interesting observations that may inform future data acquisition.
Figure 8 shows the dynamics between variables in the entire scene (i.e. non-elevated, background soil CO2) versus the points with eCO2 measurements. It is important to note that in each sub-figure, independent data sources are used to avoid showing intrinsically correlated datasets. Fractional cover and biomass are derived from the ASO lidar data; the vegetation trait data and foliar traits are derived from AVIRIS imagery; and ET is derived from MASTER data. In this case, the variables shown are directly as observed (or derived directly from the data source).
We observed interesting dynamics between ecosystem variables, suggesting great potential for future research. In the eCO2 subset, NDVI was, on average, lower than that observed for the same fractional cover in the control dataset (Fig. 8a). This is consistent with the model illustration of decreased greenness for increasing eCO2. Similarly, ET was lower in the eCO2 subset for pixels with the same NDVI observed in the control, showing a greater degree of stress even when plants have the same greenness (Fig. 8b). In addition, the strong linear relationship between ET and NDVI appears to break down for the points affected by eCO2.
Canopy nitrogen in the eCO2 subset increased with fractional cover, unlike the control, which remained flat, which again mimics the modelled data findings (Fig. 8c). ET was lower in the eCO2 subset for the same biomass, which implies that plants are doubly affected by the enhanced CO2 – the biomass decreases with increasing eCO2, and the ET decreases further with decreasing biomass (Fig. 8d). Again, the strong linear relationship between ET and biomass breaks down for those points affected by eCO2. These findings suggest complex relationships between ecosystem parameters in their response to increasing eCO2.
Using airborne remotely sensed ecosystem properties against a ground-measured database of eCO2 (volcanic excess CO2 emanating into the forest canopy through the soil), we evaluated the effects of increasing eCO2 on plant structure, function, and composition. Our aims were to (i) evaluate whether a passively degassing volcanic system is a viable means to study properties of ecosystems, (ii) determine if ecosystem variables are adequately detected using airborne data, and (iii) present key lessons learnt that can enable similar studies over different biomes.
This study has provided initial observations of ecological responses to eCO2 that are measurable from airborne data. We found that (a) eCO2 was a significant predictor in regression ensemble models of ecosystem variables and (b) there were visual differences between the sites of increased eCO2 and the background image. This work also demonstrates that an active volcanic system is a viable way in which to study the CO2 effect on ecosystems.
The regression ensemble model showed that eCO2 was a significant predictor for two structural variables (canopy height and biomass), nine composition variables (six vegetation indices, three foliar traits), and a function variable (ET). Therefore, as hypothesized, eCO2 affects ecosystems in structure, composition, and function, all of which are detectable both with airborne observations as well as within a volcanically derived eCO2 system. Further evaluation of the model showed that both canopy height and biomass decreased with increasing eCO2; the vegetation indices decrease with increasing eCO2; canopy nitrogen concentration increases; LMA decreases; carbon decreases; and ET decreases.
Some of these observations contrasted with results found in other published studies, while others agreed. For instance, our study found a decrease in NDVI with increasing eCO2, which correlates to the multispectral satellite findings of Rouse et al. (2010) and Cholathat et al. (2011). In some cases, the decrease others have found can be explained by the tree-kill effect, where vegetation is removed. However, by accounting for fractional cover in our models, we have shown that NDVI decreases independently of fractional cover (see in Fig. 8). This shows that, regardless of whether the number of trees changes, the greenness of individual trees is reduced. This finding is in direct contrast with the CO2 fertilization hypothesis, which states that rising CO2 has a positive effect on plant growth and productivity due to increased availability of carbon, which has been shown using field data (Zhu et al., 2016; Huang et al., 2007). However, this decrease in NDVI could also be explained by a reduction in leaves, rather than a reduction in leaf health, due to more efficient leaves (e.g. higher nutrient concentration, more efficient in water use).
The decrease in canopy height and biomass agrees with the tree-ring study done by Biondi and Fessenden (1999), which also found slower lodgepole pine growth rates in high CO2 emission areas on Mammoth Mountain. However, a study by Smith et al. (2013) found an increase in biomass in the mixed-species temperate forest FACE experiment. In that experiment, there was large variation between and within species, and the experiment was limited to 4 years. Perhaps a long-term species composition shift due to eCO2 was the cause of the change in biomass in our study, but we do not have individual tree species-level data to support this hypothesis.
Our model showed an increase in canopy nitrogen, which could indicate species selection or individual plant optimization, given the decrease in NDVI, biomass, and ET. Canopy nitrogen is associated with a plant's investment in photosynthesis (Singh et al., 2015). We also found an increase in canopy nitrogen relative to fractional cover, showing that the change in nitrogen was not impacted by an increase in overall vegetation for those sites (Fig. 8). Tercek et al. (2008) noted that Dichanthelium lanuginosum (hot springs panic grass) in Yellowstone had made physiological adjustments to photosynthetic enzymes in response to long-term exposure to CO2, and a study of ice cores showed a 40 % decrease in stomatal density over the last 200 years, which parallelled an increase in global CO2 (Woodward, 1987). However, Sharma and Williams (2009) evaluated vegetation naturally exposed to CO2 in Yellowstone National Park and found reduced nitrogen at a leaf level in Pinus contorta (lodgepole pine) and increased nitrogen at a leaf level for Linaria dalmatica (Dalmation toadflax; an invasive, non-native herb). Once again, the species-level differences highlight the need for remote-sensing analysis over areas that encompass wide species variation, in order to understand overall trends.
Kimball et al. (1998) found a slight increase in ET in a FACE experiment over cotton fields, but that increase was within the error of the ET estimation and so was not deemed statistically significant. In contrast, Nendel et al. (2009) found a decrease in ET and an increase in dry above-ground biomass over a FACE crop rotation experiment. In this study, we found a decrease in ET. In addition, we found a decrease in ET relative to both NDVI and biomass, when comparing the points affected by eCO2 to those unaffected points in the surrounding area. The unaffected sites showed a positive linear relationship between ET and both NDVI and biomass, which appeared to break down for points affected by eCO2 in both relationships.
The combination of lower NDVI, higher canopy nitrogen, and higher ET suggests a canopy that uses less water with rising CO2 resulting in higher water use efficiency, with a nutrient-rich canopy. Since leaves are stronger and more efficient, fewer are required for photosynthesis. While biomass increased slightly, the more obvious change was the decrease in the variance of biomass, which points to alignment to more similar trees with elevated CO2.
High fluxes of CO2 through soils in kill zones on Mammoth Mountain have likely impacted forest ecosystems through oxygen deprivation in soil pore space, inhibition of root respiration, and soil acidification (McGee and Gerlach, 1998; Farrar et al., 1995; Qi et al., 1994). Since we used soil CO2 flux as the predictor variable in the model, some of the observed ecosystem responses may therefore be due to the effects of high concentrations of CO2 on the soil environment or some combination of soil and atmospheric effects. However, by using fractional cover as an input to the model and excluding the kill zones altogether to derive Figure 8, we are focusing on the CO2 gradient over vegetated areas around these zones, that are unlikely to be affected by soil acidification. The Mammoth Mountain soil CO2 flux dataset does, however, provide a record of CO2 emissions that is more stable in space and time than measurements of atmospheric CO2 concentrations. In particular, forest canopies will through time be exposed to eCO2 at highly variable levels because the originally mostly invariant eCO2 once emitted through the soil into the sub-canopy atmosphere is subject to highly variable dispersion from thermal and wind disturbances at minute, diurnal, and seasonal scales (Staebler and Fitzjarrald, 2004). In-canopy concentration measurements of eCO2 will therefore be highly variable, and, especially if conducted instantaneously, may not be representative of the long-term relative exposure strength in the canopy.
Vegetation at this site is also responding to the partial pressure of CO2 in the atmosphere, among other gases. A response above the asymptote of the net photosynthetic rate versus internal CO2 partial pressure (A-Ci curve) would result in very little vegetation response to the partial pressures (Tissue et al., 1999). However, the partial pressures even at elevated molar concentration at Mammoth are about 60 % of those at sea level. The fact that we see systematic ecosystem effects suggests that elevation is not on the flat part of the A-Ci curve. In other words, even if elevation were to reduce the CO2 effect, we still are seeing strong CO2 effects regardless, highlighting just how important and strong of a response we are able to detect.
We will clarify that the effects should not necessarily be given a subjective description of “negative”; rather, it is important to note that the CO2 fertilization effect is unlikely to continue indefinitely, particularly at the same rates that FACE studies have shown only in the short term. All other experiments have been unable to show long-term effects. Our study suggests that over the scale of decades, some of these hypothesized greening or biomass increases may not be sustainable. Other results, such as an increase in canopy nitrogen with increasing CO2, do seem to remain consistent with our study, however.
This exploratory study leveraged existing data acquired over Mammoth Mountain. We used ASO lidar, AVIRIS, and MASTER data to derive products that describe ecosystem structure, composition, and function and used field eCO2 measurements to show that elevated CO2 was a significant predictor of ecosystem variables, including vegetation indices, plant foliar traits, biomass, and evapotranspiration. While our study has shown the promise of airborne remote sensing in detecting measurable ecosystem changes in forest ecosystems on and around a CO2-emitting volcanic system, it was also completed using an existing ad hoc collection of data. The nature of the collection of data sources enabled us to understand the details of the data characteristics necessary for future studies.
While this study is useful for showing the benefit of both a passively emitting volcanic system and airborne data for evaluating the ecosystem response to eCO2, we anticipate that more meaningful results would be obtained with all datasets acquired simultaneously, at the same resolution. ET in particular varies over short time periods due to the influence of meteorological inputs, and so multi-temporal acquisitions would provide a better overview of the ecosystem function. Other possible factors could affect this very complex system, some of which have been discussed previously, including pH, oxidative stress, water/nutrient availability, extreme climate events, and plant vigour. More fieldwork and test sites would be needed to rule out many of these factors. Other data, such as photosynthesis, may also add to future analysis. We note that this study was exploratory and that this study was intended to identify both potential signals as well as design elements for further study.
This exploratory study used airborne remote-sensing data, coupled with ground measurements of volcanic soil CO2 flux on a forested volcano, to derive relationships between rising CO2 emissions and ecosystem structure, function, and composition metrics. We have shown that passively emitting volcanic systems are viable environments in which to study CO2 impacts on ecosystems, with eCO2 the most significant predictor in regression ensemble models of several ecological variables, including NDVI, canopy nitrogen concentration, ET, and biomass. When comparing differences between vegetation parameters affected by eCO2 and those estimated over the background scene, we found contrasting patterns and dynamics between ecological variables, showing that a combination of different remote-sensing platforms is capable of providing a comprehensive view of ecosystem responses to long-term elevated volcanic CO2.
Key lessons learnt from this study include the following:
-
Future campaigns should acquire all data at the same or similar resolution at individual tree scale.
-
Significantly more than 55 vegetated tree points are necessary in order to draw meaningful conclusions regarding the dynamics between variables at Mammoth Mountain (which has one dominant tree species). The number of required points in other environments will vary according to ecosystem complexity and will likely far exceed this number.
-
Combining lidar and spectral data across a range of wavelengths yielded a more complete view than using any one data source alone.
Soil CO2 data are the property of the USGS and may be requested through Cynthia Werner at the USGS. AVIRIS data are available by searching for flight line f141021t01p00r05 at https://aviris.jpl.nasa.gov/alt_locator/ (AVIRIS Data Portal, 2018). MASTER data are available via https://masterprojects.jpl.nasa.gov/L2_Products (MASTER Data Products, 2018). The flight ID is 14-903-00; line 02. Lidar data used in this study are the property of the Airborne Snow Observatory and may be obtained through Kat Bormann at JPL.
The authors declare that they have no conflict of interest.
We thank Cynthia Werner for providing soil CO2 flux data over our study
area; Gregory Halverson for processing ET data; Zhiwei Ye for processing
functional trait data; and Anthony Bloom, Stuart Wilkinson, Christoph Kern,
and Deborah Bergfeld for discussions during earlier stages of this project.
The research described in this paper was carried out at the Jet Propulsion
Laboratory, California Institute of Technology, under contract with the
National Aeronautics and Space Administration.
Government sponsorship acknowledged.
Edited by: Jochen Schöngart
Reviewed by: two anonymous referees
Anderson, D. E. and Farrar, C. D.: Eddy covariance measurement of CO2 flux to the atmosphere from an area of high volcanogenic emissions, Mammoth Mountain, California, Chem. Geol., 177, 31–42, 2001.
AVIRIS Data Portal: Jet Propulsion Laboratory, California Institute of Technology, available at: https://aviris.jpl.nasa.gov/alt_locator/, last access: 13 December 2018.
Biondi, F. and Fessenden, J. E.: Response of Lodgepole Pine growth to CO2 degassing at Mammoth Mountain, California, Ecology, 80, 2420–2426, 1999.
Boudoire, G., Di Muro, A., Liuzzo, M., Ferrazzini, V., Peltier, A., Gurrieri, S., Michon, L., Giudice, G., Kowalski, P., and Boissier, P.: New perspectives on volcano monitoring in a tropical environment: Continuous measurements of soil CO2 flux at Piton de la Fournaise (La Réunion Island, France), Geophys. Res. Lett., 44, 8244–8253, 2017.
Camarda, M., De Gregorio, S., and Gurrieri, S.: Magma-ascent processes during 2005–2009 at Mt. Etna inferred by soil CO2 emissions in peripheral areas of the volcano, Chem. Geol., 330, 218–227, 2012.
Cardellini, C., Chiodini, G., and Frondini, F.: Application of stochastic simulation to CO2 flux from soil: mapping and quantification of gas release, J. Geophys. Res., 108, 2425, https://doi.org/10.1029/2002JB002165, 2013.
Cholathat, R., Li, X., and Ge, L.: Monitoring natural analog of geologic carbon sequestration using multi-temporal Landsat TM images in Mammoth Mountain, Long Valley Cadera, California, Int. Geosci. Remote Se., Vancouver, 24–29 July 2011, 2011.
Cook, A. C., Hainsworth, L. J., Sorey, M. L., Evans, W. C., and Southon, J. R.: Radiocarbon studies of plant leaves and tree rings from Mammoth Mountain, CA: a long-term record of magmatic CO2 release, Chem. Geol., 177, 117–131, 2001.
Drake, B. G., Gonzàlez-Meler, M. A., and Long, S. P.: More Efficient Plants: A Consequence of Rising Atmospheric CO2?, Annu. Rev. Plant Phys., 48, 609–639, https://doi.org/10.1146/annurev.arplant.48.1.609, 1997.
Evans, J. S. and Hudak, A. T.: A multiscale curvature algorithm for classifying discrete return LiDAR in forested environments, IEEE T. Geosci. Remote, 45, 1029–1038, https://doi.org/10.1109/TGRS.2006.890412, 2007.
Farrar, C. D., Sorey, M. L., Evans, W. C., Howle, J. F., Kerr, B. D., Kennedy, B. M., and Southon, J. R.: Forest-killing diffuse CO2 emission at Mammoth Mountain as a sign of magmatic unrest, Nature, 376, 675–678, https://doi.org/10.1038/376675a0, 1995.
Fisher, J. B., and ECOSTRESS Algorithm Development Team: ECOsystem Spaceborne Thermal Radiometer Experiment on Space Station (ECOSTRESS): Level-3 Evapotranspiration Algorithm Theoretical Basis Document, 24 pp, Jet Propulsion Laboratory, Pasadena, 2015.
Fisher, J. B., Melton, F., Middleton, E., Hain, C., Anderson, M., Allen, R., McCabe, M. F., Hook, Baldocchi, D., Townsend, P. A., Kilic, A., Tu, K., Miralles, D. D., Perret, J., Lagouarde, J.-P., Waliser, D., Purdy, A. J., French, A., Schimel, D., Famiglietti, J. S., Stephens, G., and Wood, E. F.: The Future of Evapotranspiration: Global requirements for ecosystem functioning, carbon and climate feedbacks, agricultural management, and water resources, Water Resour. Res., 53, 2618–2626, 2017.
Fisher, J. B., Tu, K., Baldocchi, D. D.: Global estimates of the land-atmosphere water flux based on monthly AVHRR and ISLSCP-II data, validated at 16 FLUXNET sites, Remote Sens. Environ., 112, 901–919, 2008.
Friedlingstein, P., Meinshausen, M., Arora, V. K., Jones, C. D., Anav, A., Liddicoat, S. K., and Knutti, R.: Uncertainties in CMIP5 Climate Projections due to Carbon Cycle Feedbacks, J. Climate, 27, 512–526, 2014.
Garcia, M., Saatchi, S., Ferraz, A., Silva, C.A., Ustin, S., Koltunov, A., and Balzter, H.: Impact of data model and point density on aboveground forest biomass estimation from airborne LiDAR, Carbon Balance and Management, 12, 4, https://doi.org/10.1186/s13021-017-0073-1, 2017.
Gerlach, T. M.: Present-day CO2 emissions from volcanos, Eos Trans. AGU, 72, 249–255, https://doi.org/10.1029/90EO10192, 1991.
Gerlach, T. M., Doukas, M. P., McGee, K. A., and Kessler, R.: Airborne detection of diffuse carbon dioxide emissions at Mammoth Mountain, California, Geophys. Res. Lett., 26, 3661–3664, 1999.
Giammanco, S., Sims, K. W. W., and Neri, M.: Measurements of 220Rn and 222Rn and CO2 emissions in soil and fumarole gases on Mt. Etna volcano (Italy): Implications for gas transport and shallow ground fracture, Geochem. Geophy. Geosy., 8, 10, https://doi.org/10.1029/2007GC001644, 2007.
Gillespie, A., Rokugawa, S., Matsunaga, T., Cothern, J. S., Hook, S. J., and Kahle, A. B.: A temperature and emissivity separation algorithm for Advanced Spaceborne Thermal Emission and Relection Radiometer (ASTER) images, IEEE T. Geosci. Remote Sens., 36, 1113–1126, 1998.
Hernández, P. A., Pérez, N. M., Salazar, J. M., Nakai, S., Notsu, K., and Wakita, H.: Diffuse emission of carbon dioxide, methane, and helium-3 from Teide Volcano, Tenerife, Canary Islands, Geophys. Res. Lett., 25, 3311–3314, https://doi.org/10.1029/98GL02561, 1998.
Huang, J.-G., Bergeron, Y., Denneler, B., Berninger, F., and Tardif, J.: Response of forest trees to increased atmospheric CO2, Crit. Rev. Plant Sci., 26, 265–283, https://doi.org/10.1080/07352680701626978, 2007.
Kerrick, D. M.: Present and past nonanthropogenic CO2 degassing from the solid earth, Rev. Geophys., 39, 565–585. https://doi.org/10.1029/2001RG000105, 2001.
Kimball, B. A, LaMorte, R. L., Seay, R. S., Pinter Jr, P. J., Rokey, R. R., Hunsaker, D. J., Dugas, W. A., Heuer, M. L., Mauney, J. R., Hendrey, G. R., Lewin, K. F., and Nagy, J.: Effects of free-air CO2 enrichment on energy balance and evapotranspiration of cotton, Agr. Forest Meteorol., 70, 259–278, 1998.
Kobayashi, H. and Iwabuchi, H.: A coupled 1-D atmosphere and 3-D canopy radiative transfer model for canopy reflectance, light environment, and photosynthesis simulation in a heterogeneous landscape, Remote Sens. Environ., 112, 173–185, 2008.
Kolby Smith, W., Reed, S. C., Cleveland, C. C., Ballantyne, A. P., Anderegg, W. R. L., Wieder, W. R., and Running, S. W.: Large divergence of satellite and Earth system model estimates of global terrestrial CO2 fertilization, Nat. Clim. Change, 6, 306–310, https://doi.org/10.1038/nclimate2879, 2015.
Körner, C.: Plant CO2 responses: an issue of definition, time and resource supply, New Phytol., 172, 393–411, https://doi.org/10.1111/j.1469-8137.2006.01886.x, 2006.
Le Quéré, C., Andrew, R. M., Canadell, J. G., Sitch, S., Korsbakken, J. I., Peters, G. P., Manning, A. C., Boden, T. A., Tans, P. P., Houghton, R. A., Keeling, R. F., Alin, S., Andrews, O. D., Anthoni, P., Barbero, L., Bopp, L., Chevallier, F., Chini, L. P., Ciais, P., Currie, K., Delire, C., Doney, S. C., Friedlingstein, P., Gkritzalis, T., Harris, I., Hauck, J., Haverd, V., Hoppema, M., Klein Goldewijk, K., Jain, A. K., Kato, E., Körtzinger, A., Landschützer, P., Lefèvre, N., Lenton, A., Lienert, S., Lombardozzi, D., Melton, J. R., Metzl, N., Millero, F., Monteiro, P. M. S., Munro, D. R., Nabel, J. E. M. S., Nakaoka, S.-I., O'Brien, K., Olsen, A., Omar, A. M., Ono, T., Pierrot, D., Poulter, B., Rödenbeck, C., Salisbury, J., Schuster, U., Schwinger, J., Séférian, R., Skjelvan, I., Stocker, B. D., Sutton, A. J., Takahashi, T., Tian, H., Tilbrook, B., van der Laan-Luijkx, I. T., van der Werf, G. R., Viovy, N., Walker, A. P., Wiltshire, A. J., and Zaehle, S.: Global Carbon Budget 2016, Earth Syst. Sci. Data, 8, 605–649, https://doi.org/10.5194/essd-8-605-2016, 2016.
Lewicki, J. L., Hilley, G. E., Tosha, T., Aoyagi, R., Yamamoto, K., and Benson, S. M.: Dynamic coupling of volcanic CO2 flow and wind at the HorseshoeLake tree kill, Mammoth Mountain, CA, Geophys. Res. Lett., 34, L03401, https://doi.org/10.1029/2006GL028848, 2006.
Lewicki, J. L., Hilley, G. E., Tosha, T., Aoyagi, R., Yamamoto, K., and Benson, S. M.: Dynamic coupling of volcanic CO2 flow and wind at the Horseshoe Lake tree kill, Mammoth Mountain, California, Geophys. Res. Lett., 34, L03401, https://doi.org/10.1029/2006GL028848, 2007.
Lewicki, J. L., Fischer, M. L., and Hilley, G. E.: Six-week time series of eddy covariance CO2 flux at Mammoth Mountain, California: performance evaluation and role of meteorological forcing, J. Volcanol. Geoth. Res., 171, 178–190, https://doi.org/10.1016/j.jvolgeores.2007.11.029, 2008.
Lewicki, J. L., Hilley, G. E., Dobeck, L., and Marino, B. D.: Eddy covariance imaging of diffuse volcanic CO2 emissions at Mammoth Mountain, CA, USA, Bull. Volcanol., 74, 135–141, 2012.
Lewicki, J. L. and Hilley, G. E.: Multi-scale observations of the variability of magmatic CO2 emissions, Mammoth Mountain, CA, USA, J. Volcanol. Geoth. Res., 284, 1–15, https://doi.org/10.1016/j.jvolgeores.2014.07.011, 2014.
Lewicki, J. L., Hilley, G. E., Shelly, D. R., King, J. C., McGeehin, J. P., and Mangan, M.: Crustal migration of CO2-rich magmatic fluids recorded by tree-ring radiocarbon and seismicity at Mammoth Mountain, CA, USA, Earth Planet. Sci. Lett., 390, 52–58, 2014.
Lewin, K. F., Hendrey, G. R., Nagy, J., and LaMorte, R. L.: Design and application of a free-air carbon dioxide enrichment facility, Agric. Forest Meteorol., 70, 15–29, https://doi.org/10.1016/0168-1923(94)90045-0, 1994.
List, M. R.: Geologic remote sensing and GIS evaluation of volcanogenic CO2-induced tree kills: Mammoth Mountain, California, 1991–2004, M.S. Thesis, CSUS, 2005.
Ma, P., Kang, E. L., Braverman, A., and Nguyen, H.: Spatial statistical downscaling for constructing high-resolution nature runs in global observing system simulation experiments, in review, 2018.
Mason, E., Edmonds, M., and Turchyn, A. V.: Remobilization of crustal carbon may dominate volcanic arc emissions, Science, 357, 290–294, 2017.
MASTER Data Products: Jet Propulsion Laboratory, California Institute of Technology, available at: https://masterprojects.jpl.nasa.gov/L2_Products/, last access: 13 December 2018.
McGee, K. A. and Gerlach, T. M.: Annual cycle of magmatic CO2 in a tree-kill soil at Mammoth Mountain, California: Implications for soil acidification, Geology, 26, 463–466, https://doi.org/10.1130/0091-7613(1998)026<0463:ACOMCI>2.3.CO;2, 1998.
McGuire, A. D., Melillo, J. M., and Joyce, L. A.: The Role of Nitrogen in the Response of Forest Net Primary Production to Elevated Atmospheric Carbon Dioxide, Annu. Rev. Ecol. Syst., 26, 473–503, 1995.
Nendel, C., Kersebaum, K. C., Mirschel, W., Manderscheid, R., Weigel, H.-J., and Wenkel, K.-O.: Testing different CO2 response algorithms against a FACE crop rotation experiment, NJAS – Wageningen, J. Life Sci., 57, 17–25, 2009.
Norby, R. J., De Kauwe, M. G., Domingues, T. F., Duursma, R. A., Ellsworth, D. S., Goll, D. S., and Zaehle, S.: Model–data synthesis for the next generation of forest free-air CO2 enrichment (FACE) experiments, New Phytol., 209, 17–28, https://doi.org/10.1111/nph.13593, 2016.
Ogretim, E., Crandall, D., Gray, D. D., and Bromhai, G. S.: Effects of Atmospheric Dynamics on CO2 Seepage at Mammoth Mountain, California USA, Journal of Computational Multiphase Flows, 5, 283–294, 2013.
Painter, T. H., Berisford, D. F., Boardman, J. W., Bormann, K. J., Deems, J. S., Gehrke, F., Hedrick, A., Joyce, M., Laidlaw, R., Marks, D., Mattmann, C., McGurk, B., Ramirez, P., Richardson, M., Skiles, S. M., Seidel, F. C., and Winstral, A.: The Airborne Snow Observatory: Fusion of scanning lidar, imaging spectrometer, and physically-based modeling for mapping snow water equivalent and snow albedo, Remote Sens. Environ., 184, 139–152. https://doi.org/10.1016/j.rse.2016.06.018, 2016.
Perez, N. M., Hernandez, P. A., Padilla, G., Nolasco, D., Barrancos, J., Melian, G., and Ibarra, M.: Global CO2 emission from volcanic lakes, Geology, 39, 235–238, 2011.
Peterson, B. J. and Melillo, J. M.: The potential storage of carbon caused by eutrophication of the biosphere, Tellus B, 37, 117–127, https://doi.org/10.1111/j.1600-0889.1985.tb00060.x, 1985.
Pickles, W. L., Kasameyer, P. W., Martini, B. A., Potts, D. C., and Silver, E. A.: Geobotanical Remote Sensing for Geothermal Exploration, Geothermal Resources Council 2001 Annual Meeting, San Diego, California, 26–29 August, 2001.
Potter, D. A.: Forested Communities of the Upper Montane in the Central and Southern Sierra Nevada, United States Department of Agriculture General Technical Report PSW-GTR-169, 1998.
Pypker, T. G., Unsworth, M. H., Mix, A. C., Rugh, W., Ocheltree, T., Alstad, K., and Bond, B. J.: Using Nocturnal Cold Air Drainage Flow to Monitor Ecosystem Processes in Complex Terrain, Ecol. Appl., 17, 702–714, 2007.
Qi, J., Marshall, J. D., and Mattson, K. G.: High soil carbon dioxide concentrations inhibit root respiration of Douglas fir, New Phytol., 128, 435–442, 1994.
Rahn, T. A., Fessenden, J. E., and Wahlen, M.: Flux chamber measurements of anomalous CO2 emission from the flanks of Mammoth Mountain, California, Geophys. Res. Lett., 23, 1861–1864, 1996.
RiANALYZE: available at: http://www.riegl.com/products/software-packages/rianalyze/, last access: 8 July 2017.
Rouse, J. H., Shaw, J. A., Lawrence, R. L., Lewicki, J. L., Dobeck, L. M., Repasky, K. S., and Spangler, L. H.: Multi-spectral imaging of vegetation for detecting CO2 leaking from underground, Environ. Earth Sci., 60, 313–323, 2010.
Ryu, Y., Baldocchi, D. D, Kobayashi, H., van Ingen, C., Li, J., Black, T. A., Beringer, J., van Gorsel, E., Knohl, A., Law, B. E., and Roupsard, O.: Integration of MODIS land and atmosphere products with a coupled-process model to estimate gross primary productivity and evapotranspiration from 1 km to global scales, Global Biogeochem. Cy., 25, GB4017, https://doi.org/10.1029/2011GB004053, 2011.
Schimel, D. S.: Terrestrial ecosystems and the carbon cycle, Glob. Change Biol., 1, 77–91, https://doi.org/10.1111/j.1365-2486.1995.tb00008.x, 1995.
Schimel, D., Stephens, B. B., and Fisher, J. B.: Effect of increasing CO2 on the terrestrial carbon cycle, P. Natl. Acad. Sci. USA, 112, 436–441. https://doi.org/10.1073/pnas.1407302112, 2015.
Schwandner, F. M., Gunson, M. R., Miller, C. E., Carn, S. A., Eldering, A., Krings, T., and Podolske, J. R.: Spaceborne detection of localized carbon dioxide sources, Science, 358, eaam5782, https://doi.org/10.1126/science.aam5782, 2017.
Schwandner, F. M., Seward, T. M., Gize, A. P., Hall, P. A., and Dietrich, V. J.: Diffuse emission of organic trace gases from the flank and crater of a quiescent active volcano (Vulcano, Aeolian Islands, Italy), J. Geophys. Res.-Atmos., 109, D04301, https://doi.org/10.1029/2003JD003890, 2004.
Sharma, S. and Williams, D. G.: Carbon and oxygen isotope analysis of leaf biomass reveals contrasting photosynthetic responses to elevated CO2 near geologic vents in Yellowstone National Park, Biogeosciences, 6, 25–31, https://doi.org/10.5194/bg-6-25-2009, 2009.
Singh, A., Serbin, S. P., McNeil, B. E., Kingdon, C. C., and Townsend, P. A.: Imaging spectroscopy algorithms for mapping canopy foliar chemical and morphological traits and their uncertainties, Ecol. Appl., 25, 2180–2197, 2015.
Smith, A. R., Lukac, M., Hood, R., Healey, J. R., Miglietta, F., and Godbold, D. L.: Elevated CO2 enrichment induces a differential biomass response in a mixed species temperate forest plantation, New Phytol., 198, 156–168, 2013.
Sorey, M. L., Evans, W. C., Kennedy, B. M., Farrar, C. D., Hainsworth, L. J., and Hausback, B.: Carbon dioxide and helium emissions from a reservoir of magmatic gas beneath Mammoth Mountain, California, J. Geophys. Res.-Sol. Ea, 103, 15303–15323, 1998.
Staebler, R. M. and Fitzjarrald, D. R.: Observing subcanopy CO2 advection, Agr. Forest Meteorol., 112, 139–156, 2004.
Tercek, M. T., Al-Niemi, T. S., and Stout, R. G.: High Levels of Carbon Dioxide in YellowStone National Park: A Glimpse into the Future?, Yellowstone Science, 16, 12–19, 2008.
Thenkabail, P. S., Lyon, J. G., and Huete, A.: Hyperspectral Remote Sensing of Vegetation, CRC Press, Taylor and Francis Group, 405–406, 2016.
Thompson, D. R., Gao, B.-C., Green, R. O., Roberts, D. A., Dennison, P. E., and Lundeen, S. R.: Atmospheric correction for global mapping spectroscopy: ATREM advances for the HyspIRI preparatory campaign, Remote Sens. Environ., 167, 64–77, 2015.
Tissue, D. T., Griffin, K. L., and Ball, J. T.: “Photosynthetic Adjustment in Field-Grown Ponderosa Pine Trees after Six Years of Exposure to Elevated CO2”, Tree Physiol., 19, Oxford University Press, 221–228, 1999.
Tonooka, H.: Accurate Atmospheric Correction of ASTER Thermal Infrared Imagery Using the WVS Method, IEEE Trans. Geos. Remote Sens., 43, 2778–2792, 2005.
Verma, M., Fisher, J. B., Mallick, K., Ryu, Y., Kobayashi, H., Guillaume, A., Moore, G., Ramakrishnan, L., Hendrix, V., Wolf, S., Sikka, M., Kiely, G., Wohlfahrt, G., Gielen, B., Roupsard, O., Toscano, P., Arain, M. A., and Cescatti, A.: Global surface net-radiation at 5 km from MODIS Terra, Remote Sens., 8, 1–20, 2016.
Werner, C. and Brantley, S.: CO2 emissions from the Yellowstone volcanic system, Geochem. Geophy. Geosy., 4, 1061, https://doi.org/10.1029/2002GC000473, 2003.
Werner, C., Bergfeld, D., Farrar, C. D., Doukas, M. P., Kelly, P. J., and Kern, C.: Decadal-scale variability of diffuse CO2 emissions and seismicity revealed from long-term monitoring (1995–2013) at Mammoth Mountain, California, USA, J. Volcanol. Geotherm. Res., 289, 51–63, https://doi.org/10.1016/j.jvolgeores.2014.10.020, 2014.
Woodward, F. I.: Stomatal numbers are sensitive to increases in CO2 from pre-industrial levels, Nature, 327, 617–618, 1987.
Zhu, Z., Piao, S., Myneni, R. B., Huang, M., Zeng, Z., Canadell, J. G., Ciais, P., Sitch, P., Friedlingstein, P., Arneth, A., Cao, C., Cheng, L., Kato, E., Koven, C., Li, Y., Lian, X., Liu, Y., Liu, R., Mao, J., Pan, Y., Peng, S., Peñuelas, J., Poulter, B., Pugh, T. A. M., Stocker, B. D., Viovy, N., Wang, X., Wang, Y., Xiao, Z., Yang, H., Zaehle, S., and Zeng, N.: Greening of the Earth and its drivers, Nat. Clim. Change, 6, 791–795, 2016.