the Creative Commons Attribution 4.0 License.
the Creative Commons Attribution 4.0 License.
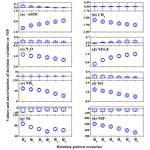
Using a modified DNDC biogeochemical model to optimize field management of a multi-crop (cotton, wheat, and maize) system: a site-scale case study in northern China
Wei Zhang
Chunyan Liu
Xunhua Zheng
Kai Wang
Feng Cui
Rui Wang
Siqi Li
Zhisheng Yao
Jiang Zhu
It is still a severe challenge to optimize the field management practices for a multi-crop system when simultaneously aiming at yield sustainability and minimum negative impacts on climate as well as atmosphere and water quality. This site-scale case study was devoted to developing a biogeochemical process model-based approach as a solution to this challenge. The best management practices (BMPs) of a three-crop system growing cotton and winter wheat–summer maize (W–M) in rotation, which is widely adopted in northern China, were identified. The BMPs referred to the management alternatives with the lowest negative impact potentials (NIPs) among the scenarios satisfying all given constraints. The independent variables used to determine the NIPs and those utilized as constrained criteria were simulated by the DeNitrification-DeComposition model, which was modified in this study. Due to the unsatisfactory performance of the model in daily simulations of nitric oxide (NO) emission and net ecosystem exchange of carbon dioxide (NEE), the model was modified to (i) newly parameterize the soil moisture effects on NO production during nitrification, and (ii) replace the original NEE calculation approach with an algorithm based on gross primary production. Validation of the modified model showed statistically meaningful agreements between the simulations and observations in the cotton and W–M fields. Three BMP alternatives with overlapping uncertainties of simulated NIPs were screened from 6000 management scenarios randomly generated by Latin hypercube sampling. All of these BMP alternatives adopted the baseline (currently applied) practices of crop rotation (3 consecutive years of cotton rotating with 3 years of W–M in each 6-year cycle), the fraction of crop residue incorporation (100 %), and deep tillage (30 cm) for cotton. At the same time, these BMP alternatives would use 18 % less fertilizer nitrogen and sprinkle or flood-irrigate ∼23 % less water than the baseline while adopting reduced tillage (5 cm) for W–M. Compared with the baseline practices, these BMP alternatives could simultaneously sustain crop yields, annually enlarge the soil organic carbon stock by 4 ‰ or more, mitigate the aggregate emission of greenhouse gases, NO release, ammonia volatilization, and nitrate leaching by ∼7 %, ∼25 %, ∼2 %, and ∼43 %, respectively, despite a ∼5 % increase in N2O emission. However, further study is still necessary for field confirmation of these BMP alternatives. Nevertheless, this case study proposed a practical approach to optimize multi-crop system management to simultaneously achieve multiple United Nations Sustainable Development Goals.
- Article
(2746 KB) - Full-text XML
-
Supplement
(750 KB) - BibTeX
- EndNote
Globally, fiber crops (i.e., cotton) and cereals such as wheat and maize have long played a relevant role in human society because they are primary sources of materials for the textile and food industries. In China, although cotton cultivation only covers 2.0 %–3.9 % of the annual crop harvest area (cotton lint production totalled 5.3 × 106–7.6 × 106 Mg from 2007 to 2016), the cultivation of cereals is quite large. Wheat and maize accounted for 39 % and 26 % of the harvest area and represented 1.29 × 108 and 2.2 × 108 Mg of grain, respectively, in 2016 (China Statistical Yearbook, 2017).
Northern China is both the second most important area of cotton production and the largest region of the winter wheat–summer maize double-cropping system (i.e., both crops are harvested within a year, and they are hereinafter referred to as W–M) in the country (e.g., Cui et al., 2014). Crop rotations of cotton and W–M have commonly been grown in this region, alternating every 3 to 5 years (e.g., Liu et al., 2010, 2014). Over the last few decades, the yields of cotton, wheat, and maize have been increased by employing intensified agricultural management practices, such as increased fertilizer inputs, advanced irrigation methods, and so on (e.g., Han, 2010). A recent study indicated that the cotton cropping system in northern China persistently functioned as an intensive carbon or net aggregate greenhouse gas (GHG) source compared with W–M (Liu et al., 2019). These previous studies have revealed that the change in the storage of soil organic carbon (ΔSOC), net ecosystem aggregate GHG emissions (NEGE), and other biogeochemical processes involving the multiple cropping system in northern China are likely closely related to the rotation pattern of cotton and W–M (e.g., Liu et al., 2010, 2014, 2019; Lv et al., 2014).
To maintain high productivity, the three-crop rotation system for cotton and W–M in northern China are characterized by large additions of synthetic nitrogen fertilizers and irrigation amount (e.g., Chen et al., 2014; Galloway et al., 2004), at 60–140 and 550–600 kg N ha−1 yr−1, and 140–200 and 90–690 mm yr−1 for cotton and W–M, respectively (e.g., Ju et al., 2009; Liu et al., 2014; Wang et al., 2008). High nitrogen and water inputs can result in high release potentials for nitrogenous pollutants, which can induce a series of environmental problems, such as water pollution due to increased nitrate () leaching (e.g., Collins et al., 2016). In addition, other field management practices, e.g., tillage and crop residue treatment, can also affect the emissions of reactive nitrogen and contribute to negative environmental effects (e.g., Zhang et al., 2017; Zhao et al., 2016). Therefore, the evaluation of the multiple-cropping system (e.g., rotations of cotton and W–M) is shifting from a single-goal method aimed at increasing crop yields to a multi-goal approach (e.g., Cui et al., 2014; Garnett et al., 2013; Zhang et al., 2018). This multi-goal strategy aims to simultaneously sustain/increase crop productivity to ensure food security, increase SOC content to improve soil fertility, mitigate NEGE to alleviate climate warming, reduce ammonia (NH3) volatilization and nitric oxide (NO) emission to secure air quality, and abate leaching to protect water quality.
According to the multi-goal approach, an objective method is applied to identify the best management practice (BMP), which evaluates each decision variable (using price-based proxies or other measures) and screens the best option with the minimal negative impact potential (NIP) under the given constraints at the annual scale (e.g., Cui et al., 2014; Xu et al., 2017). To screen the BMP, it is essential to quantify the biogeochemical effects of management practices at the annual scale. Field experiments are often only capable of focusing on the decision variables of very few management practices during short periods (e.g., Ding et al., 2007; Liu et al., 2010, 2015; Wang et al., 2013a, b). However, this limitation of field experiments can potentially be overcome by process-oriented biogeochemical models, such as DeNitrification-DeComposition (DNDC) (Li et al., 1992; Li, 2000, 2007, 2016), DAYCENT (Delgrosso et al., 2005), and LandscapeDNDC (Haas et al., 2012).
A three-crop (cotton, winter wheat, and summer maize) system in southern Shanxi Province was selected for this model-based site-scale case study. This study was undertaken to (i) diagnose problems with the DNDC95 model version that was validated in Cui et al. (2014) against the comprehensive field measurements of the selected W–M fields; (ii) make modifications and then validate the modified model for both cotton and W–M cropping systems, especially for the variables that determine NIPs and those involved in given constraints; and (iii) investigate the biogeochemical effects of various rotation patterns with different field management practices and identify the multi-goal BMP alternatives based on the modified model simulations. These efforts were undertaken to test two hypotheses: that a validated process-oriented biogeochemical model is capable of addressing a challenging issue – the optimization field management practices of a three-crop rotation system; and that the field management of an intensive three-crop rotation system can be optimized to simultaneously sustain the current crop yields and annually increase the SOC stock by 4 ‰ so as to implement the international “4 Per 1000” initiative (https://www.4p1000.org/, last access: 31 May 2019) – an action of the Paris Agreement aimed at combating climate change, mitigating aggregate greenhouse gas emissions, and reducing other negative impacts on the environment.
2.1 Brief introduction to DNDC95
The original DNDC95 model used by Cui et al. (2014) and in this study is one of the latest DNDC versions (http://www.dndc.sr.unh.edu/model/GuideDNDC95.pdf, last access: 15 September 2017). This model consists of two components with six modules in total. Driven by the given primary ecological factors, the first component simulates the field states of a soil–plant system, such as the soil chemical and physical status, vegetation growth, and organic matter decomposition. Driven by the soil-regulating variables yielded by the first component, the second component simulates the core biogeochemical processes of carbon and nitrogen transformations and the physical processes of liquid and gas transport; thus, the annual dynamics of net ecosystem exchanges of carbon dioxide (CO2)(NEE); emissions of methane (CH4), nitrous oxide (N2O), NH3, and NO; and leaching and the inter-annual dynamics of SOC and NEGE are simulated. These features enable the model to investigate the integrative biogeochemical effects of the rotation patterns of multiple crops and/or other management practices based on comprehensive validation. The minimum inputs used to facilitate the model simulation include (i) the meteorological variables of daily precipitation and maximum/minimum temperature; (ii) the soil (cultivated horizon) properties of the clay fraction, bulk density, SOC content, and pH; (iii) the crop parameters for the yield potential, thermal degree days (TDD) for maturity, and the mass fractions and carbon-to-nitrogen (C∕N) ratios of the grain, root, and leaf plus stem; (iv) the management practice variables of sowing and harvest (dates), fraction of incorporated/retained residue at harvest, tillage (date and depth), irrigation (date, method, and water amount), and fertilization (date, type, method, nitrogen amount, and C∕N ratio of organic manure); and (v) other variables (annual means of the NH3 concentration in the atmosphere and the ammonium plus concentration in rain water). For more details regarding the model, please see Li et al. (1992) and Li (2000, 2007, 2016).
2.2 Problem diagnosis and model modification
The daily simulations of the original DNDC95 showed poor model performance for NO emissions from the cotton field, e.g., with a very negative Nash–Sutcliffe efficiency index (NSI) of −1.03 for the 333 observations in 2009. Meanwhile, the original model often failed to capture the daily high NEE fluxes on rainy or cloudy days despite the agreement between the simulation and observation of the annual cumulative NEE (Cui et al., 2014). According to the program codes, a constant fraction (0.003) of a nitrification rate (Fn; in g N m−2 d−1) was adopted in the original model to calculate the daily NO production in the nitrification process. This was found to account for the former problem as the fraction could vary with soil moisture, which was mechanically similar to the N2O production in nitrification (shown by the model program codes). The latter problem was ascribed to the algorithm adopted to calculate daily NEE. In the original model, a daily NEE flux was calculated as the residue of daily CO2 release by soil heterotrophic respiration and daily CO2 uptake by the increase in cumulative net primary production (NPP). The daily cumulative NPP was calculated by portioning the input of potential crop yields to the day following a given NPP growth curve (shown by the model program codes; Li, 2016). Consequently, the insensitivity of a daily NPP increase to radiation intensity reduction resulted in a more negative daily NEE on a rainy or cloudy day. In order to solve these two problems, the model was modified in this study as follows.
In the model version modified in this study, the effect of the soil moisture (SM) in water-filled pore space (WFPS; dimensionless 0–1 fraction) on NO production was parameterized by referring to that for N2O production during nitrification and incorporated into the function by replacing the constant fraction mentioned above (Eq. 1). This modification was adopted to reflect that high soil moisture facilitates the production of NO as a by-product of nitrification (NOp; in g N m−2 d−1). The maximum fraction of NO production (Kn; dimensionless 0–1 fraction) during nitrification was calibrated as 0.03 using the observed daily NO fluxes from October 2007 to October 2008 in the cotton field. The other observations of daily and annual NO fluxes from both adjacent lands with different field treatments (Table S1 in the Supplement) were used to validate this modification.
In the model version modified in this study, a daily NEE flux (g C m−2 d−1) is calculated as the residue of the daily CO2 release from ecosystem respiration (ER; in g C m−2 d−1) and daily CO2 uptake due to gross primary production (GPP; in g C m−2 d−1). While the daily ER is simulated as it is in the original model, the daily GPP is calculated using Eq. (2), which is a widely applied hyperbola function of photosynthetically active radiation (PAR; in mmol m−2 d−1; e.g., Wang et al., 2013a; Zheng et al., 2008).
The photosynthetic parameters in Eq. (2), α (g C mmol−1) and GPPmax (g C m−2 d−1), denote apparent quantum yield and maximum GPP, respectively. Each parameter is quantified as the product of shoot standing biomass (Bs; in g C m−2) and biomass-specific apparent quantum yield (f1; in g C mmol−1 (g C m−2)−1) or specific GPPmax (f2; in g C m−2 d−1 (g C m−2)−1) corrected by an adaptation factor (a; dimensionless) reflecting inter-annual variations of a crop (Eqs. 3, 4). The variable Bs is simulated as it is in the original model. The seasonal dynamics of f1 and f2 is a function of the normalized plant developing stage (ds; dimensionless 0–1 fraction) (Eqs. 5, 6). The functions of f1 and f2 take the forms presented by Zheng et al. (2008) for winter wheat, whereas their empirical parameters of a, b, c, d1, d2, g, h, i, j, l, and m can be calibrated to adapt both functions to given conditions. Two daily NEE fluxes per week were randomly selected from the year-round eddy covariance observations in both cotton and W–M cropping systems (Cui et al., 2014; Liu et al., 2019; Wang et al., 2013a) to calibrate the values of these parameters specifically for cotton, winter wheat, and summer maize, while remaining daily data (independent NEE observations) were used to evaluate this modified NEE algorithm. For the calibrated values of these parameters, refer to Table S2.
2.3 Brief introduction to the selected field site and information on the data for model evaluation
The field site (34∘55.50′ N, 110∘42.59′ E; altitude of 348 m) selected for this modeling case study is located at Dongcun Farm, near Yongji County, Shanxi Province, in northern China. The site is subject to a temperate continental monsoon climate, and it had an annual precipitation of 580 mm and a mean air temperature of 14.4∘ from 1986 to 2010 (Cui et al., 2014). Cotton, winter wheat, and summer maize are the major crops grown at this farm and in the surrounding regions. Field experiments were performed in two adjacent fields (each of which was 100 m wide and 200 m long) for cotton and W–M between 2007 and 2010. The soil of the land cultivated with cotton and W–M was clay loam, with approximately 38% and 32 % clay (<0.002 mm), 57 % and 50 % silt (0.002–0.05 mm), 5 % and 18 % sand (0.05–2 mm), 10.0 and 11.3 g kg−1 SOC, 1.1 and 1.1 g kg−1 total nitrogen, a pH (in H2O) of 8.0 and 8.7 at a depth of 0–10 cm, and bulk densities (0–6 cm depth) of 1.20 and 1.17 g cm−3, respectively (Liu et al., 2010, 2011, 2012). A sprinkler system was applied to both fields. For more detailed information on the field experiments and observed data, please refer to Cui et al. (2014), Liu et al. (2010, 2011, 2014, 2015), and Wang et al. (2013a, b).
The modified model was validated with observations from both fields. The daily meteorological data from 2004 to 2010 were obtained directly from Cui et al. (2014). The measured data were used directly for the minimum required soil properties. The input data on the field capacity and wilting point in WFPS were 0.65 and 0.2, respectively (Cui et al., 2014). The crop parameters for cotton were directly determined by the field measurements, which were 1900 kg C ha−1 for potential grain yield (1.2 times the mean of the measured values), 0.41 and 25, 0.16 and 40, and 0.43 and 40 for the mass fractions and C∕N ratios of the grain, root, and leaf plus stem, respectively, and 3600 ∘C for the TDD. Detailed management practices (Table S3) were obtained from Li et al. (2009) and Liu et al. (2014). In contrast to the locally conventional fertilizer application rate of 110–140 kg N ha−1 yr−1 for cotton, the fertilizer doses for the experimental cotton field in 2007 and 2008 were reduced to 66–75 kg N ha−1 yr−1 to avoid overgrowth of the leaves in place of seeds or lint. The data from the cotton field available for the model calibration and validation included the daily observed soil temperature (5 cm depth), topsoil moisture (0–6 cm), daily NEE from eddy covariance measurement from 2008 to 2009, sub-weekly observed CH4 fluxes in the 2010 growing season (Liu et al., 2010, 2014, 2019; Wang et al., 2013b), daily N2O and NO fluxes from 2007 to 2008, annually measured grain yields from 2008 to 2010, and annual leaching from 2008 to 2009. All the data used by Cui et al. (2014) in the validation of the original model were used to revalidate the modified model performance for the selected W–M fields with different field management practices. In addition, two observations of the cumulative NH3 volatilization following urea topdressing to the winter wheat in the spring of 2008 (Tong et al., 2009) were also involved in the evaluation of the model performance (Yang et al., 2011). The information on all of the data used in the modified model calibration and validation is detailed in Table S1.
2.4 Scenario settings and simulations
For the three-crop system investigated, this study attempted to identify the BMP alternatives with the best rotation pattern between cotton and W–M and the optimized field management practices of this rotation pattern. For this purpose, six rotation pattern scenarios (hereinafter referred to as R0, R1, …, R5) were set for a 6-year cycle that was widely applied by the local farmers (Liu et al., 2010, 2011, 2014). The subscript number of each rotation pattern represents the number of the consecutive years of cotton cultivation. For instance, R0 denotes a 6-year monoculture of W–M, and R2 represents a rotation pattern consisting of 2 years of continuous cotton rotated with 4 years of continuous W–M. Because the local farmers typically did not adopt a cotton monoculture for longer than 5 years, the longest cotton monoculture only lasted for 5 years (R5). The transitions between cotton and W–M in the scenario rotations are detailed in Table S4.
As for the setting of the field management scenarios for the individual rotation patterns, four field management factors were considered, including (i) nitrogen fertilizer dose, (ii) water amount, (iii) method of irrigation, and (iv) depth of tillage. The values of these factors used for the baseline scenario were taken from the observations of the conventional management practices in the experimental region (Tables S3, S4, S5). The nitrogen doses of the baseline were 110 and 430 kg N ha−1 yr−1 for cotton and W–M, respectively. Over the last few decades, the fields in this region have been mostly flood-irrigated (Liu et al., 2010). Thus, flood-irrigation was chosen as the baseline method. The baseline timings and water amounts were established by referring to the 10–30 d cumulative precipitation prior to the individual irrigation events and the recorded timings and water amounts of the conventional management practices in the two adjacent fields. Thus, the irrigation frequencies and annual cumulative water amounts of the baseline varied from 1 to 3 times and 75 to 230 mm yr−1 for cotton and 4 to 6 times and 290 to 510 mm yr−1 for W–M (Table S5). The fraction of 100 % for residue incorporation and the conventional tillage to a depth of 20 cm for W–M and 30 cm for cotton were applied for the baseline practices. To screen the BMPs by fully taking the independent and interactive effects of rotation patterns and field management practices on the NIPs into account, a total of 6000 field management scenarios (each being a combination of the four management factors) for all the six rotation pattern scenarios (1000 for each) were randomly generated. The fertilizer doses and irrigation amounts were randomly selected, within their lower and upper bounds of continuous variations, using Latin hypercube sampling. The lower and upper bounds for nitrogen fertilizer doses (44–172 and 110–430 kg N ha−1 yr−1) and irrigation amount (40–100 mm per event) were set as 40 % and 100 % of the baseline, respectively. Only two irrigation methods, flooding (IF) – as the baseline – and sprinkling (IS), were included for the random sampling of this management factor. For cotton, the tillage depth was fixed at 30 cm. For W–M, four tillage depths (0 cm for no-till, 5 and 10 cm for reduced tillage, and 20 cm for the conventional practice) were included for random sampling of this factor. The BMPs for each rotation pattern scenario were first screened from 1000 field management scenarios. Then, the BMP alternatives were finally screened from the BMPs of the individual rotation pattern scenarios. These identified BMP alternatives would include the scenarios with overlapping NIP uncertainties, for which the random error at ±1 SD (instead of ±2 SD) for the total simulation error was adopted for the NIP of each scenario so as to achieve a relatively high screening precision. The decision variables and the NIP of baseline management scenarios for each rotation pattern scenario were used to particularly address the biogeochemical effects of rotation pattern.
An 18-year simulation was performed for each scenario. The annual averages for the simulated yields, decision variables, and NIPs were used to address the biogeochemical effects of rotation patterns or to screen the BMP alternatives. The simulations of all scenarios were driven by the meteorological data observed at Yuncheng station (approximately 60 km east of the experimental site) from 1996 to 2013 (http://data.cma.cn/data/cdcindex/cid/6d1b5efbdcbf9a58.html, last access: 10 October 2017). To stabilize the carbon and nitrogen dynamics and reduce the residual effects of the initial conditions (Palosuo et al., 2012; Zhang et al., 2015), a 12-year spin-up was performed for each scenario (i.e., a period of two consecutive 6-year rotation cycles) before the 18-year simulation. The spin-up for each scenario was driven by the same rotation pattern and field management practices as this scenario.
2.5 Method for identifying the best management practices
An objective method jointly relying on three constraints and NIPs was adopted in this study to screen the BMPs from given scenarios. These constraints included (i) stable or increased crop yields, (ii) an annual increase in the SOC stock by 4 ‰ or more, and (iii) reduced NEGE by 5 % or more. In the present study, the NEGE was determined by summing the emissions of CH4 and N2O as well as −ΔSOC, which was quantified as a CO2 equivalent (CO2eq) quantity. In the quantification of a NEGE, the global warming potentials at the 100-year time horizon, 34 for CH4 and 298 for N2O (IPCC, 2013), were used to convert the quantities of both gases into CO2 equivalents. A NIP was expressed as a price-based proxy quantity in USD ha−1 yr−1 and used to evaluate the potential for a climatically and environmentally integrative impact exerted by a set of field management practices for multi-crop system. The NIP was determined as a linear function of the individual decision variables, following Eq. (7), wherein the multi-goal decision variables, NEGE, NH3, NO, N2OODM, and NL, represent the annual net ecosystem aggregate GHG emission (Mg CO2eq ha−1 yr−1), NH3 volatilization, NO emission, release of N2O as an ozone layer depletion matter, and hydrological nitrogen loss (mainly by leaching), respectively (kg N ha−1 yr−1 for all the nitrogen-based variables). The coefficients k1, k2, k3, k4, and k5 are mass-scaled price-based proxies for the NEGE, NH3, NO, N2OODM, and NL, with values of 7.00 USD Mg−1 CO2eq and 5.02, 25.78, 1.33, and 1.92 USD kg−1 N, respectively (Cui et al., 2014). A lower NIP indicated a better set of management practices that can exert smaller negative impacts on the climate and environment. Accordingly, the BMP was identified as the scenario with the lowest NIP among the scenarios that could satisfy all three constraints.
2.6 Method for uncertainty quantification
The model simulation error (εs) of a NIP, a constraint variable (e.g., crop yield) or a decision variable involved in Eq. (7) represented the total simulation uncertainty. It was made of two components: the input-induced uncertainty (εinput) due to the uncertainties of input items; and the model uncertainty (εmodel) due to insufficiencies in scientific structure or process parameters.
The εinput of a simulated variable was a random error if the uncertainties of model input items were known as random errors. It was estimated using the Monte Carlo test with Latin hypercube sampling within the uncertainty ranges (95 % confidence interval – CI) of sensitive input items. In DNDC, the four basic soil properties (bulk density, pH, clay fraction, and SOC content) as input items were sensitive to the model outputs (e.g., Li, 2016). Accordingly, the uncertainties of these soil properties were regarded as the major sources of model output uncertainties, such as the constraint/decision variables, or NIP. The initial bulk density, pH, clay fraction, and SOC content ranged from 1.13 to 1.25 g cm−3, 8 to 8.7, 0.31 to 0.39, and 9 to 12 g kg−1 (at the 95 % CI), respectively, which were adapted from the means and two times SD of spatially replicated observations in the two adjacent fields (Liu et al., 2014, 2019). A uniform distribution for each of these soil properties was assumed in the Monte Carlo test, in which the sampling and simulation were iterated until the mean of simulated NIPs for all iterations converged to a certain level within a tolerance of 1 %. The NIP uncertainty due to the model input uncertainties was presented as the SD of these iterated simulations.
An εs was systematic error reflecting a model simulation bias diverging from the truth. In this regard, the εs of a constraint/decision variable could be estimated using the slope of a zero-intercept univariate linear regression of simulations against observations (ZIRs−o) or model relative biases (MRBs) resulting from model validation (Eq. 8). The MRBs were only used in case a significant ZIRs−o was not obtained in model validation. To ensure a relatively high BMP screening precision, the random uncertainty of the εs of a NIP was presented as one times SD, instead of two times SD to represent the 95 % CI, as the BMP alternatives referred to the management scenarios with overlapping NIP uncertainties. For a constraint/decision variable, the mean or SD of εs in an absolute magnitude was estimated as the product of (i) an adjustment factor, (ii) the simulated variable quantity, and (iii) an error factor. The adjustment factor was obtained from model validation, which was estimated as the mean of the ratios of individual observations to simulations. The error factor for a variable with a significant ZIRs−o was given as (mean-slope) ± SD-slopes−o, wherein mean-slopes−o and SD-slopes−o denote the mean and SD of the ZIRs−o slope, respectively. The item prior to and following the “±” was used to estimate the mean and SD of the εs. For a variable that failed to obtain a significant ZIRs−o in model validation, the mean and SD of the error factors were given as the mean and SD of the MRBs. The mean of the εs for a NIP was estimated by simply summing the weighted absolute εs of individual decision variables. This was because the decision variables involved in the additive items of Eq. (7) were independent of each other. Meanwhile, the SD of the εs for a NIP was mathematically propagated from the SDs of the absolute εs of the decision variables.
In this study, the constraint variables included crop yield, −ΔSOC, and NEGE, although NEGE was also one of the decision variables. While there was no direct measurement of −ΔSOC and NEGE, their observation-oriented estimates were involved in the model validation, which provided the basis for the εs estimation of either variable. For the experimental fields with NEE observations, there was no significant input quantity of organic matter in manure or any other form while crop residues were fully incorporated into the soil. In these cases, each annual/seasonal −ΔSOC could be estimated as the sum of annual/seasonal NEE and yields, according to the mass conservation law, and used to represent the observation, with its uncertainty propagated from the random errors of the annual/seasonal yield and NEE measurements. The random error of this observation-oriented −ΔSOC that represented the annual/seasonal net CO2 emission from the cropping system and those of the observed annual cumulative CH4 and N2O were propagated to estimate the observational error of the annual/seasonal NEGE. These observation-oriented estimates of −ΔSOC or NEGE were involved in model validation.
2.7 Statistics and analysis
Statistical criteria simultaneously used to evaluate the model validity included (i) the index of agreement (IA; Eq. 9), (ii) the NSI (Eq. 10; e.g., Moriasi et al., 2007; Nash and Sutcliffe, 1970), (iii) the determination coefficient (R2; Eq. 11) and the slope of a ZIR of observations against simulations (Jiang, 2010; Li et al., 2019), and (iv) the MRB (Eq. 8; e.g., Congreves et al., 2016; Willmott and Matsuura, 2005). In Eqs. (8)–(11), k and n (k=1, 2…, n) denote the kth pair and the total pair number of the values, respectively, represents the mean of the observations (o), and represents the predictions using the ZIR. The IA index fell between zero and one, with a value closer to one indicating a better simulation, and vice versa. An NSI value between zero and one indicated acceptable model performance. Better model performance was indicated by a slope and an R2 value that were closer to one, and vice versa. An absolute MRB () value smaller than the double coefficients of variation (CVs) of replicated observations implied a valid simulation (Dubache et al., 2019).
In this study, the statistical analysis and graphical comparison were performed using the SPSS Statistics client 19.0 (SPSS Inc., Chicago, USA) and Origin 8.0 (OriginLab, Northampton, MA, USA) software packages.
3.1 Validation of daily simulations for both cropping systems
The seasonal dynamics and magnitudes of the soil temperature (5 cm) and topsoil moisture (0–6 cm) were predicted well by the model simulations (Fig. 1a, b). The sound model performance was indicated by the IA, NSI, and ZIR slope and R2 values of 0.98 and 0.83, 0.93 and 0.15, 0.93 and 0.83, and 0.95 (n=677, p<0.001) and 0.42 (n=432, p<0.001) for the temperature and moisture, respectively.
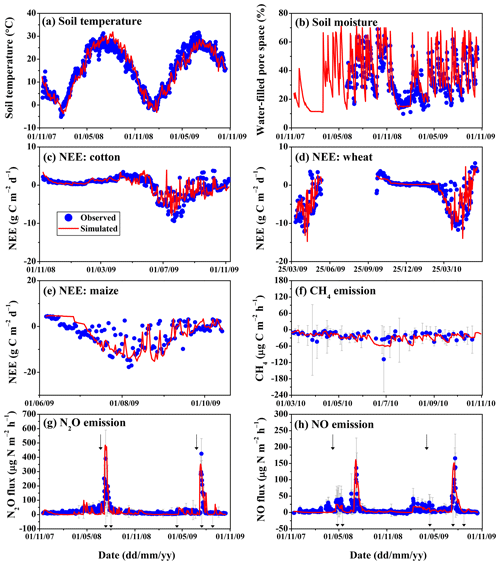
Figure 1Observed and simulated daily mean soil temperature (5 cm), soil moisture (0–6 cm), daily net ecosystem exchanges of carbon dioxide (NEE) in cotton field and winter wheat–summer maize fields, and daily fluxes of methane (CH4), nitrous oxide (N2O), and nitric oxide (NO) from the cotton field. The solid and dashed arrows indicate the dates of fertilization and irrigation, respectively. The measurement errors were not shown in panels (a)–(e) for figure clarity. The vertical bar for each observation in panels (f)–(h) indicates ±2 SD to represent the uncertainty at the 95 % confidence interval. The legend in (c) applies to all panels.
Compared with the original model, the modified model showed much better performance in simulating daily NEE fluxes from both cropping systems. It simulated the abrupt NEE fluxes on cloudy or rainy days in the growing season particularly well (Fig. 1c–e). In comparison with the original model for daily NEE simulations, the modified model enhanced the IA, NSI, and ZIR slope and R2 values from 0.74 to 0.81, 0.32 to 0.60, 0.60 to 0.96, and 0.27 to 0.60 (n=261, p<0.001), respectively, for the cotton field, and from 0.75 to 0.80, 0.30 to 0.51, 0.69 to 0.80, and 0.45 to 0.55 (n=311, p<0.001), respectively, for the W–M field. For the CH4 uptake, the observations and simulations showed similar seasonal variations (Fig. 1f), with IA, NSI, and ZIR slope and R2 values of 0.68, <0, 0.70, and 0.08 (n=69, p=0.018), respectively.
The simulated seasonal patterns and peak emissions of N2O and NO generally agreed with the observations (exemplified by Fig. 1g, h for the cotton field). Compared with the original model for daily N2O flux simulations, the modified model performed comparably well for the cotton field, with a NSI of around −0.45, and ZIR slope ∼0.49 and R2 values of ∼0.39 (n=592, p<0.001), although it showed better performance for the W–M fields, with IA, NSI, and ZIR slope and R2 values of 0.69 versus 0.52, 0.03 versus –0.26, 0.62 versus 0.46, and 0.16 (n=976, p<0.001) versus “not available”, respectively. Relative to original model, the modified model showed improved simulations for the daily NO fluxes from the cotton field, with increased IA, NSI, and ZIR slope and R2 values from 0.62 to 0.78, −1.03 to −0.04, 0.37 to 0.54, and 0.09 to 0.39 (n=333, p<0.001), respectively, while it performed comparably well for those from the W–M fields, with IA, NSI, and ZIR slope and R2 values of ∼0.82, ∼0.32, ∼0.74, and 0.35–0.40 (n=967, p<0.001), respectively.
In comparison with the observed daily NH3 fluxes (measured using micrometeorological technique) following a single fertilization event in the maize season in 2008, the modified model simulations showed IA, NSI, and ZIR slope and R2 values of 0.87, 0.12, 0.68, and 0.53 (n=11, p=0.07), respectively. However, the model failed to capture the immediate responses of daily NH3 fluxes to urea addition in the wheat fields, which were measured using a quasi-dynamic chamber method.
3.2 Validation of simulated variables in annual/seasonal cumulative quantities
For the cotton yields (seeds plus lint) over the three consecutive experimental years (2008–2010), the simulations were consistent with the observations in terms of the smaller (0.4 %–24 %) than the double CVs (39 %–56 %) of the spatially replicated measurements. For all of the experimental treatments of the cotton, wheat, and maize, the simulated yields of both the modified and original model strongly agreed with observations (Fig. 2a), with an IA of 0.93–0.95, a NSI of 0.75, and ZIR slope and R2 values of 0.96–1.00 and 0.75–0.78, respectively (n=35, p<0.001). This validation resulted in an adjustment factor of 0.96 and smaller error factors of 3.0±1.6 % for crop yields simulated by the modified model.
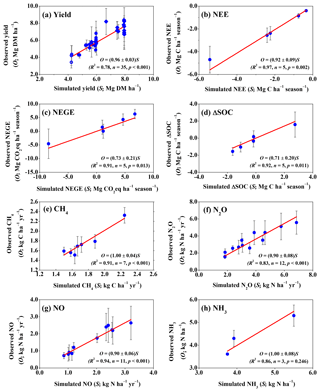
Figure 2Comparison between observations and simulations of crop yields, annual/seasonal cumulative NEE and NEGE, annual/seasonal ΔSOC, annual cumulative fluxes of methane (CH4) uptake, nitrous oxide (N2O) and nitric oxide (NO), and cumulative fluxes of ammonia (NH3). Yield refers to the seed yield of cotton (open circle) and the grain yield of winter wheat (solid circle) and summer maize (solid diamond). NEE refers to the net ecosystem exchanges of carbon dioxide. NEGE refers to the net ecosystem aggregate greenhouse gas emission. ΔSOC refers to the change in soil organic carbon stock. NEE, NEGE, and ΔSOC are annual for cotton and seasonal for wheat and maize. The observed ΔSOC was given as the opposite of NEE plus yield in carbon mass quantity for the cropping system with the incorporation of full residues, whereas each ΔSOC simulation was the sum of simulated changes in carbon stocks of soil humus, microbial biomass, and dissolvable organic compounds. Simulations resulted from the modified model. The slope errors of the zero-intercept linear regressions are ±2 SD to represent the 95 % confidence interval. Vertical bars indicate the standard deviation of three or four spatial replicates, with the exception of NEE. The errors of NEE were adapted from the coefficient of variation (on average 25 %) reported by Wang et al. (2013b). DM refers to dry matter. CO2eq refers to the carbon dioxide equivalent. The 100-year global warming potentials of 34 for CH4 and 298 for N2O (IPCC, 2013) were used to quantify NEGE in CO2eq quantity.
For the annual cumulative NEE of the cotton field during the two consecutive year-round periods and the seasonal cumulative NEE in two wheat seasons and one maize season, the simulations of both model versions showed comparably significant agreement with the observations (Fig. 2b), with an IA of 0.99–1.00, a NSI of 0.95–1.00, and ZIR slope and R2 values of 0.92–1.02 and 0.97–0.99, respectively (n=5, p≤0.000–0.002). The modified model simulations showed of 6 %–16 %, which were much less than the reported CV (25 %) of the eddy covariance observations.
Compared with the annual/seasonal NEGE quantities derived from the observations of crop yields, annual/seasonal cumulative NEE, and fluxes of CH4 and N2O, the simulations implicated good performance of the modified model (Fig. 2c), with an IA of 0.96, a NSI of 0.77, and ZIR slope and R2 values of 0.73 and 0.91 (n=5, p=0.013). Although the simulations showed an average overestimation of ∼25 %, their were only 17 %–72 % (33 % on average) of the observation-oriented CVs (27 %–170 %, with a mean of 91 %), implicating a statistically meaningful good performance of the modified model. This validation resulted in an adjustment factor of 0.73 and error factors of 25±19 % for the annual/seasonal NEGE simulated by the modified model.
In comparison with the annual/seasonal ΔSOC quantities estimated from the observed crop yields and annual/seasonal cumulative NEE, the simulations by the modified model (Fig. 2d) showed an IA of 0.96, a NSI of 0.75, and ZIR slope and R2 values of 0.71 and 0.92 (n=5, p=0.011). The ZIR slope indicated an average overestimation of the model by ∼30 %. Nevertheless, the of the individual simulations were only 4 %–79 % (30 % on average) of the observation-oriented CVs (30 %–210 %, with a mean of 97 %), still indicating a statistically meaningful consistency. This validation resulted in an adjustment factor of 0.71 and error factors of 30±19 % for the annual/seasonal ΔSOC simulated by the modified model.
The model simulations of the annual cumulative CH4 uptake in 2009 and 2010 showed significant agreement (Fig. 2e), with an IA of 0.98, a NSI of 0.91, and ZIR slope and R2 values of 1.00 and 0.91 (n=7, p<0.001). The were only 5 %–56 % (25 % on average) of the double CVs (10 %–24 %, with a mean of 17 %) for the spatially replicated observations. This validation resulted in an adjustment factor of 1.00 and error factors of % for the modified model simulations of cumulative CH4 uptake.
The modified model simulations of the annual cumulative N2O emissions from all of the field experimental treatments of the cotton and W–M fields were comparable with the observations (Fig. 2f), showing an IA of 0.94, a NSI of 0.72, and ZIR slope and R2 values of 0.90 and 0.83 (n=12, p<0.001). The of the individual simulations were only 6 %–93 % (36 % on average) of the double CVs (23 %–64 %, with a mean of 47 %) for the spatially replicated observations. For the annual cumulative N2O emissions simulated by the modified model, this validation resulted in an adjustment factor of 0.90 and error factors of 8.7±4.5 %.
Compared with annual cumulative N2O emissions, slightly better consistency with observations was obtained for the modified model simulations of the annual cumulative NO emissions from the cotton and W–M fields under different experimental conditions (Fig. 2f, g). The NO simulation showed an IA of 0.97, a NSI of 0.85, and ZIR slope and R2 values of 0.90 and 0.94 (n=11, p<0.001). The of the individual simulations were only 2 %–52 % (23 % on average) of the double CVs (30 %–99 %, with a mean of 66 %) for the spatially replicated observations. This validation provided an adjustment factor of 0.90 and error factors of 10.1±3.2 % for the cumulative NO emissions simulated by the modified model.
The simulations of cumulative NH3 volatilization during the 11 d following the three urea application events, with one in the maize field in summer and two in the winter wheat fields (with flood-irrigation and sprinkling, respectively) in spring (Fig. 2h), showed an IA of 0.97, a NSI of 0.86, and ZIR slope and R2 values of 1.00 and 0.86 (n=3, p=0.246). The simulations resulted in smaller MRBs of −8.8 % to 3.8 % (with a mean of −0.4 %), despite the model's failure to capture the quick responses of daily NH3 fluxes to the urea topdressing events. This validation resulted in an adjustment factor of 1.00 and error factors of % for the modified model simulations of cumulative NH3 volatilization following nitrogen applications.
The modified model simulations of the cumulative leaching from the cotton field in 2 consecutive years agreed with the observations, in terms of the smaller MRBs (−32 % to −27 %) than the two times CVs (109 %–115 %) for the spatially replicated observations. These MRBs represented the model underestimations by 3–4 and 13–21 kg N ha−1 yr−1 for the annual leaching rates in the cotton and W–M fields subject to the currently applied field management practices, respectively. This validation derived an adjustment factor of 1.42 and error factors of % for the modified model simulations.
The above results suggested that the modified DNDC95 model was especially applicable at this field site for investigating the biogeochemical effects of different rotation patterns between the cotton and W–M and those exerted by different management practices, and thus was capable of BMP identification.
3.3 Biogeochemical effects of different cotton and wheat–maize rotation patterns
Figure 3 illustrated the dynamics of the crop yields and each decision variable resulting from the consecutive simulations over 18 years for all of the rotation patterns subject to the field management practices of the baseline scenario. Figure 4 showed the relationship between the annual average of each decision variable and the number of consecutive years of cotton monoculture within the rotation patterns.
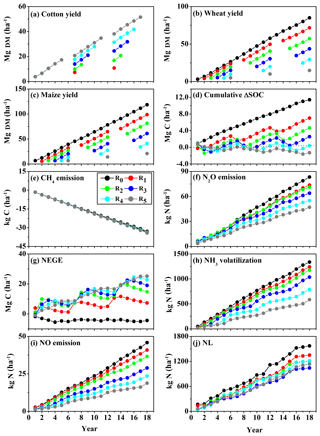
Figure 3Simulated cumulative crop yields, changes in soil organic carbon (ΔSOC), methane (CH4), nitrous oxide (N2O) releases, net ecosystem aggregate greenhouse gas emission (NEGE), ammonia (NH3) volatilization, nitric oxide (NO) emission, and nitrate leaching (NL) of individual rotation patterns (with a 6-year rotation cycle) over an 18-year period. R0, R1, …, R5 represent rotation patterns with the cotton cultivated consecutively for 0, 1, …, 5 year(s), respectively, within each 6-year rotation cycle. The legend in (e) applies to all panels. The simulations resulted from the modified model driven by the currently applied field management practices (i.e., the baseline field management scenario) and the observed means of input soil properties.
The average grain yields for the cotton, wheat, and maize were not significantly different among the various rotation patterns, with averages of 3.5, 4.8, and 6.7 kg dry matter ha−1 for cotton, wheat, and maize, respectively (Fig. 3a–c).
For the dynamic changes in the annual SOC stocks, the values were generally positive for W–M but negative for cotton, except for the first year after the transition to this fiber crop. As indicated by Fig. 3d, the simulated SOC contents over the 18-year period increased for R0, R1, R2, and R3 but decreased for R4 and R5. The annual average −ΔSOC increased significantly (p<0.001) with an increase in the consecutive years of cotton monoculture from 0 to 5 within the 6-year rotation cycle (Fig. 4a). The rotation patterns with the baseline management showed small variations in the CH4 uptake (Fig. 3e), with the uptake rates ranging from 1.6 to 2.1 kg C ha−1 yr−1. However, the annual averages for the CH4 uptake increased significantly (p<0.001) with the increased consecutive years of cotton monoculture (Fig. 4b). For N2O, the annual emissions showed large inter-annual variations (Fig. 3f), with CVs of 26 %–48 %. In addition, the average emissions of this gas decreased significantly from 4.6 to 2.6 kg N ha−1 yr−1 (Fig. 4c) after increasing consecutive years of cotton monoculture (p<0.001). As a result, the NEGE was significantly promoted (p=0.002) (Figs. 3g, 4d).
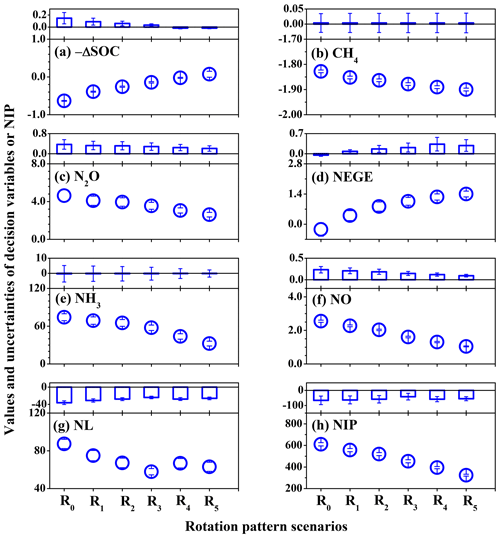
Figure 4Simulated effects of various rotation patterns between a cotton and winter wheat–summer maize cropping system with a 6-year cycle on decision variables and the negative impact potential (NIP). The subscript numbers in R0, R1, …, R5 refer to the number of consecutive years of cotton cultivation. The y axis units are megagrams of carbon per hectare per year (Mg C ha−1 yr−1) for the opposite of the mean annual increase in soil organic carbon stock (−ΔSOC), kilograms of carbon per hectare per year (kg C ha−1 yr−1) for methane (CH4) emissions, kilograms of nitrogen per hectare per year ( kg N ha−1 yr−1) for fluxes of nitrous oxide (N2O), ammonia (NH3), nitrous oxide (NO), and nitrate leaching (NL), megagrams of carbon dioxide equivalent per hectare per year (Mg CO2eq ha−1 yr−1) for net ecosystem aggregate greenhouse gas emission (NEGE), and US dollars per hectare per year (USD ha−1 yr−1) for NIP. The CO2eq was based on the 100-year global warming potentials, i.e., 34 for CH4 and 298 for N2O (IPCC, 2013). The NIP was calculated using Eq. (7), which is presented in the text. The vertical bar within the open circle of each datum point indicates the absolute uncertainty (1 SD) introduced by the input uncertainties of key soil properties. Each unfilled column indicates the absolute total uncertainty of the simulation, with its vertical bar representing its random uncertainty (1 SD).
Regarding the gaseous air pollutants NH3 and NO, the simulated emissions ranged from 17 to 103 and 0.5 to 3.3 kg N ha−1 yr−1, respectively (Fig. 3h, i). Figure 4e and f showed that the average annual emissions of both gases were significantly reduced after increasing the consecutive years of cotton monoculture (p≤0.001). The annual leaching of the different rotation patterns displayed significant inter-annual variations (Fig. 3j), with CVs of 41 %–69 %. Thus, the annual averages for leaching changed insignificantly in response to the consecutive years of cotton monoculture (p=0.056; Fig. 4g).
The NIP varied significantly among the various rotation patterns (p<0.001), declining from 610 to 324 USD ha−1 yr−1 with increased consecutive years of cotton monoculture (Fig. 4h). For the three constraints, the crop yields showed no obvious differences among the various rotation patterns. Both R0 and R5 represented the typical rotation patterns in the region. The simulations for the former indicated the greatest increase in SOC and the lowest NEGE but the highest NIP, whereas those for the latter showed the greatest SOC loss and the largest NEGE but the lowest NIP (Fig. 4a, d, h). These patterns indicated that neither typical rotation pattern is sustainable.
3.4 Identification of best management practices
Out of the 6000 field management scenarios, three under the R3 were finally identified as the BMP alternatives, which simultaneously satisfied the given constraints while yielding the comparably lowest NIPs (332–335 USD ha−1 yr−1) within the overlapping uncertainty ranges with εs of USD ha−1 yr−1 (Table 1). These BMP alternatives for the three-crop system recommended the following combination of field management practices: (i) the currently applied 6-year rotation cycle with 3-year cotton monoculture rotated with 3-year W–M; (ii) full incorporation of crop residues at harvest; (iii) the presently adopted crop cultivars and timing of sowing, fertilization (date, depth, and splits), irrigation (date and times), and harvest; (iv) the use of urea alone and 18 % lower rates (90 and 353 kg N ha−1 yr−1 for the cotton and W–M, respectively) than the conventional nitrogen fertilization; (v) sprinkling or flood-irrigation using ∼23 % less water (∼77 mm per event) than conventional flood-irrigation; and (vi) conventional plow tillage (30 cm depth) following final cotton harvest but reduced tillage (rotary 5 cm depth) for the W–M. In comparison with the simulations driven by the baseline scenario (R3 as the currently applied rotation pattern and its field management practices), the identified BMP alternatives could sustain the baseline crop yields while simultaneously enlarging the SOC stock by ≥4 ‰ and mitigating the NEGE, NH3 volatilization, NO emission, and leaching by ∼7 %, ∼25 %, ∼2 %, and ∼43 %, respectively, despite a slight increase (by ∼5 %) in the N2O emission (Table 1).
Table 1Simulated constraint and decision variables and negative impact potentials (NIPs) for the baseline (the conventionally applied practices) and the alternatives of the best management practices.

BAS: the baseline. BMP: different best management alternatives
denoted by subscript numbers. N: nitrogen fertilizer dose (kg N ha−1 yr−1) of cotton/wheat–maize (W–M). IrA: irrigation amount (mm per event). IM: irrigation method. IF: flood-irrigation.
IS: sprinkling irrigation. T: tillage depth (cm). R: rotation pattern.
R3: rotation pattern with 3 consecutive years of cotton rotated with 3
continuous years of W–M. Yield: seed (cotton) or grain (W–M) yield (Mg ha−1 in dry matter). Sim: annual quantity simulation.
εs: the absolute total simulation error (i.e., the
systematic error) of the annual quantity simulation, with its error (1 SD) representing the random uncertainty magnitude.
εinput: the random model simulation error (1 SD) due to input uncertainties of the key soil
properties including
clay fraction, bulk density, pH, and soil organic carbon content. ΔSOC: annual
change in soil organic carbon stock at a depth of 0–50 cm (Mg C ha−1 yr−1). NEGE: the net ecosystem aggregate greenhouse gas emissions in
carbon dioxide
equivalent (Mg CO2 eq ha−1 yr−1).
CH4: methane emissions (kg C ha−1 yr−1). N2O,
NH3, and NO: the emissions of nitrous oxide, ammonia, and nitric oxide, respectively (kg N ha−1 yr−1). NL: nitrate leaching (kg N ha−1 yr−1). The
CO2eq was based on
the 100-year global warming potentials of 34 for
CH4 and 298 for N2O (IPCC, 2013). NIP: negative impact potential
(USD ha−1 yr−1). The simulations are the averages
of 18 consecutive years.
4.1 Model performance
The DNDC model has been widely applied in agricultural systems around the world. The version modified in this study showed good performance in simulating the soil environmental factors (soil temperature and moisture), crop yields, NEE, NH3 volatilization, CH4 uptake, emissions of N2O and NO, and leaching for the investigated lands cultivated with cotton and W–M under different field management treatments. The satisfactory validations of both crop systems, especially for the constraint and decision variables at the annual scale, suggested that the modified DNDC95 could be applied to quantify the constraint and decision variables to determine the NIP for the cotton and W–M rotation system under various management practices.
The well-simulated soil environmental factors and crop yields provided a solid basis for further simulating the constraint and decision variables under any field management condition of the three-crop rotation system. This is because the soil environmental factors are the key factors regulating the biogeochemical processes, and crop yields are indicators of essential processes in plant nitrogen uptake (Chirinda et al., 2011; Kröbel et al., 2010). For the simulations of the N2O and NO emissions, discrepancies in daily emissions generally occur in the simulations of DNDC or other current biogeochemical models due to the interactions among soil environmental factors and complex carbon- and nitrogen-related processes (e.g., Bell et al., 2012; Chirinda et al., 2011; Cui et al., 2014; Lehuger et al., 2011), which may occasionally result in significant time lags between the observations and simulations (e.g., Zhang et al., 2015). For the cotton in this study, the significant underestimation of daily NO fluxes in spring was solved to some extent by modifying the model version used by Cui et al. (2014). However, this improvement did not significantly affect the annual cumulative emissions, which were not primarily contributed by the spring fluxes (Liu et al., 2015). In fact, occasional time lags of one to a few days for measured/simulated daily fluxes seldom lead to a significant modification for the seasonal/annual cumulative emissions of a nitrogenous gas. This is attributed to the control of the mass conservation law and the canceling effect of negative and positive daily errors. The modified algorithm improved the simulations of daily NEE fluxes, thereby providing a solid basis for yielding reliable annual/seasonal cumulative NEE quantities. According to the mass conservation law, the annual cumulative NEE can be included as one of the two additive items to estimate the annual ΔSOC of an annual crop system with retention/incorporation of full residues but without significant input/output of organic matter other than product removal at harvest. This approach may be used as an alternative algorithm in the modified model to simulate the annual ΔSOC of such a cropping system. In the present case study, the annual ΔSOC simulated by the modified model using this alternative approach was consistent with those simulated by the algorithm that quantifies the annual ΔSOC by summing the annual carbon pool changes in the humus, microbial biomass, and dissolvable organic compounds (Cui et al., 2014). The consistency was indicated by the of 20±50 % versus 37±117 % (95 % CI) in comparison with the three observation-oriented estimates of annual ΔSOC (two for cotton and one for the W–M). Due to the marginally small sample size (n=3), this preliminary result still requires confirmation through further study. The simulated NH3 volatilization from the cotton field accounted for 18 %–24 % of the applied fertilizer nitrogen during the two year-round periods involved in the model validation. These simulated nitrogen loss rates via NH3 volatilization were comparable with the reported field measurements of 10 %–23 % (Li et al., 2016).
The model validation in this study suggested that the satisfactory simulations of constraint and decision variables at the annual scale could provide a solid basis for BMP identification. Because of the limited annual observations of NH3 volatilization, leaching, and ΔSOC estimated from annually measured NEE, the insufficient validation still resulted in large uncertainties in the simulations of these three variables. Therefore, future studies are still required for further validation of the model performance using comprehensive observations covering these variables as well as the others, thus reducing the simulation errors of the constraint and decision variables so as to improve the screening precision of BMP alternatives.
4.2 Biogeochemical effects of the rotation pattern and other management practices
The scenario analysis relying on model simulations in this study showed that environmental nitrogen contamination could be reduced while (i) sustaining crop yields to protect food security, (ii) achieving the 4 ‰ goal in soil carbon sequestration, and (iii) decreasing the net ecosystem aggregate GHG emissions to mitigate climate change. The reductions in environmental nitrogen contamination could be attributed to the better synchronization of crop nitrogen requirements and soil nitrogen availability by optimizing field management practices.
For cotton, a period of 5 consecutive years is usually applied as the longest cotton monoculture to stabilize its yields. Within this period, balanced elemental nutrients are applied, and thus the negative effect of monoculture on cotton yields can be offset in practice (Han, 2010). In addition, the DNDC model assumes balanced nutrient supplies for any crops as well as optimum phytosanitary conditions; thus, the negative effects of monoculture are not taken into account (e.g., Li, 2016).
The simulated positive annual ΔSOC for the W–M cropping system were mainly attributed to the incorporation of the full aboveground residues (at rates of 5.1–7.0 Mg C ha−1 yr−1), which in turn favored carbon sequestration (Han et al., 2016). On the contrary, the simulations of annual ΔSOC for the cotton cropping system were negative. The SOC stock decreases resulted from (i) the more notable CO2 emissions over the longer fallow season and (ii) the lower rates of fully incorporated residues (at rates of 2.5–3.1 Mg C ha−1 yr−1) than those of W–M system (Liu et al., 2019). As a remarkable carbon sink, the W–M cropping system with the incorporation of the full crop residues could even completely compensate for the SOC lost during the first cotton-planting year following the transition. Thus, the simulated annual ΔSOC was generally positive during the first cotton cultivation year of a three-crop rotation cycle. As a result, the R0 (i.e., pure W–M continuously within each 6-year period) acted as a net GHG sink; this was due to the fact that the positive ΔSOC could exceed the N2O emission during the W–M cultivation, whereas all of the three-crop systems subject to R1–R5 rotation patterns would function as net GHG sources. The higher nitrogen application rate for the W–M system than for the cotton system resulted in more reactive nitrogen remaining in the soil (Chen et al., 2014; Ju et al., 2009), thereby stimulating higher N2O and nitrogenous air pollutant emissions in the trials with fewer cotton cultivation years. Therefore, the rotation patterns of cotton and W–M can be optimized to realize sustainable intensification in terms of sustaining crop yields at a relatively high level, maximizing SOC increase, and minimizing negative impacts on the climate and environment.
Northern China, as the most important agricultural region in the country, experienced an increase in crop yields by a factor of 2.8 from 1980 to 2008. During this period, the application of mineral fertilizers increased by a factor of 5.1. The rapid increase in fertilizer use has resulted in excessive nitrogen remaining in the soil, posing potential risks for the environment (Chen et al., 2011; Zhang et al., 2017). To solve this problem, a reduction in fertilizer application has been proposed in several previous studies (e.g., Chen et al., 2011, 2014; Liu et al., 2012). The results of the scenario analysis in this study indicated that further reducing the farmer-optimized nitrogen doses by 18 % could still sustain the crop yields while greatly decreasing the release of nitrogenous pollutants.
In addition to fertilization, over-irrigation has also been ubiquitous in northern China for a long time, and is threatening the water security of this region due to the sharply declining groundwater table and water pollution (Gao et al., 2015; Ju et al., 2009). For this reason, only management options that can reduce the amount of irrigation should be recommended due to the severe shortage of water resources in the region. In addition, adopting sprinkling irrigation instead of flood irrigation for an equal amount of water showed positive effects on the crop yields, indicating improved irrigation efficiency (Zhang et al., 2017). This result indicated that increasing the water-use efficiency via the application of alternative irrigation techniques coupled with reduced nitrogen doses could be a pathway to sustain crop yields.
Reduced tillage practices have been promoted in China in the recent years. To facilitate the decomposition of the woody cotton residues and avoid outbreaks of diseases and pests induced by continuous implementation of reduced tillage or no-till, the tillage practices were only adjusted in the W–M fields, whereas the currently applied deep tillage practices were maintained for the cotton when setting the tillage scenarios. The simulations showed that the reduced tillage or no-till practices could sustain the crop yields while also reducing NH3 volatilization and leaching; these results were consistent with the reports from other experimental studies (e.g., Zhao et al., 2016).
As shown above, the appropriate combinations of a rotation pattern and field management practices can satisfy the three given constraints while resulting in the lowest NIPs with overlapping uncertainties. However, direct observations in field experiments usually with very limited management treatments are far less sufficient for screening these appropriate combination alternatives. Nevertheless, identifying the appropriate combination alternatives is one of the purposes of biogeochemical models, such as DNDC. In principle, a biogeochemical model that is validated with limited observations from field experiments, such as the DNDC95 modified and used in this study, could be capable of fulfilling this task.
4.3 Evaluation of the best management practice
The scenario analysis in this study was effective for screening the BMP alternatives. The identified BMP alternatives could sustain the crop yields of the three-crop rotation system, increase the SOC stock annually at 4 ‰ or more, mitigate the NEGE, and reduce the NH3 and NO emissions as well as leaching due to the enhanced resource use efficiency in response to the reduced nitrogen-fertilizer doses, irrigation amounts, and tillage depth for the W–M system. Hence, the BMP alternatives could result in significantly reduced NIPs even compared to the currently applied field management practices that have been optimized by the local farmers. However, the BMP alternatives identified were based on the constraint and decision variables only validated against the observations at the single field site involved in this case study. In this regard, confirmation of these BMP alternatives at other sites in this region is still required in the future studies.
A biogeochemical model as an ideal tool for identifying the BMPs is reflected by near-zero εs for any constraint/decision variable or NIP. A small sample size of the observations used for validation of any constraint/decision variable would result in largely positive or negative εs (including large over- or under-estimations) for model simulations of the variable, likely account for the large εs of a NIP, and thus lead to a lower precision in screening the BMP alternatives. Therefore, the applicability of the approach proposed in this study for identifying the BMPs is highly dependent upon the validations using observations with appropriate sample sizes for individual constraint and decision variables. In this study, the εs and εinput for the simulated variables and NIPs of management scenarios were quantified. For the NIPs of the identified BMP alternatives, for instance, the εs and εinput at relative magnitudes were 6.5±4.9 % and ±3.3 %, respectively, which were similar to those (9.1±5.0 % and ±3.1 %, respectively) of the baseline scenario. According to these errors, the uncertainty ranges of the NIPs for the three alternatives almost fully overlapped with each other, and they were all beyond the uncertainty range of the NIP for the baseline scenario. This implied that the approach proposed in this study could be applicable for identifying the BMPs of the three-crop rotation system. Nevertheless, the εs uncertainty range of one times SD still fully diverged negatively from zero, due to the marginally small sample sizes of available ΔSOC, NH3 volatilization, and leaching observations that led to insufficient validations for these variables. Especially, the model underestimations of leaching (with an adjustment factor of 1.42 and error factors of %) overwhelmingly dominated the diverged εs of the NIPs, which were comparable to or larger than the εinput values. Relying on the few field observations, one was still not able to judge whether there were insufficiencies in the scientific structures or inappropriate parameters in the model to dominate the large εs for these variables. Therefore, multiple comprehensive field observations with appropriate sample sizes to fully cover all the relevant variables are necessary for use in an advanced biogeochemical model with multiple functions in order to address the best management issue of a multi-crop rotation system to achieve multiple benefits.
The DNDC model was established by following the mass conservation law. In other words, this model can accurately reflect the mass balance of the carbon or nitrogen budgets for the simulated soil layer (0–50 cm depth). This principle implies that only one nitrogen budget item could be omitted for model validation. This item is usually soil nitrogen loss via the production of dinitrogen gas (N2), mainly by denitrification, which is very difficult to measure in situ (e.g., Wang et al., 2013; Zhang et al., 2019). For both crop cropping systems, however, the nitrogen lost via this pathway could almost be fully inhibited in the topsoil, wherein the soil moisture contents were often lower than 60 % WFPS (Linn and Doran, 1984; Liu et al., 2011, 2014). For instance, the N2 emission likely accounts for approximately 1.6 % of the urea applied in a winter wheat season (Zhang et al., 2019), which is at a negligibly low level for the nitrogen balance.
Regarding the identification of the BMPs, the approach proposed and applied in this case study only includes the biogeochemical effects of management on the constraint/decision variables. This approach currently excludes other factors, such as those related to the costs of the management practices, thereby likely resulting in uncertainties in the screened BMP alternatives. Despite some deficiencies, this approach can be easily and automatically implemented as long as the simulations for all constraint and decision variables can be validated using comprehensive observations, implicating its potential applicability for more comprehensive situations. Adding the missing factors is one of the future research tasks to further improve this approach.
To address the challenging issue of optimizing multi-crop system management to simultaneously achieve multiple benefits, a biogeochemical model-based approach for identifying the best management practices (BMPs) was proposed and tested in this site-scale case study. A widely distributed three-crop system in northern China, which grew cotton in rotation with winter wheat and summer maize (W–M), was investigated. The BMPs referred to the management alternatives with the lowest negative impact potentials (NIPs), falling within overlapping uncertainty ranges, among the scenarios satisfying a set of constraints. The NIP of a scenario was defined as the linear function of five decision variables, including the net ecosystem aggregate greenhouse gas emission (NEGE), ammonia (NH3) volatilization, nitric oxide (NO) release, emissions of nitrous oxide (N2O) as an ozone layer depletion matter, and nitrate leaching. This study used three variables, i.e., crop yield, annual change in SOC stock (ΔSOC), and NEGE, to specify the applied constraints that were stable/increased crop yields, annual ΔSOC by 4 ‰ or more, and reduced annual NEGE by at least 5 % in comparison with those of the baseline scenario (as the currently applied practices in this study). The constraint and decision variables to determine the NIP of each scenario were provided by a simulation using the DeNitrification-DeComposition version 95 model (DNDC95), which was modified in this study. Due to the unsatisfactory performance of the model in daily simulations of NO emission and net ecosystem exchange of carbon dioxide (NEE), the model was modified to include a new parameterization of soil moisture effects on the NO production during nitrification and replacement of the original calculation approach for NEE with an algorithm based on gross primary production. For the variables with available measurements in two adjacent fields at the selected study site, the modified model showed statistically meaningful consistency between simulations and observations. Using the systematic errors obtained from the model validation to determine the simulation uncertainties of the concerned variables for each scenario and that of its NIP, the modified model simulations driven by 6000 management scenarios automatically identified three BMP alternatives. These BMP alternatives follow the current adopted rotation pattern (3 consecutive years of cotton rotated with 3 continuous years of W–M), with 18 % less fertilizer nitrogen, ∼23 % less irrigation via sprinkling or flood-irrigation, and reduced depth of tillage for the W–M in comparison with the current applied farmer-optimized management practices. This case study demonstrated the practicability of the model-based approach and outlined its potential applicability for optimizing the field management of a multi-crop system to simultaneously achieve multiple United Nations Sustainable Development Goals. It also emphasized the need to make comprehensive observations that fully cover the constraint and decision variables, other related factors, as well as all of the crops and management practices in question to facilitate effective BMP screening through virtual experiments using a biogeochemical model, such as DNDC. In future study to identify the BMPs, specifically for the three-crop rotation system at the regional scale, it is still necessary to use a 6-year model validation that includes a rotation of all three commodity crops as well as all of the studied management practices in question.
All of the model output used to produce the figures can be obtained from the Supplement, and all of the observed data sets used in this study can be made available from the co-authors upon request.
The supplement related to this article is available online at: https://doi.org/10.5194/bg-16-2905-2019-supplement.
XZ, CL, and JZ contributed to developing the idea and enhancing the science of this study. WZ proposed a new evaluation factor – the negative impact potential – designed and implemented the model simulations and virtual experiments, and prepared the paper with contributions from all co-authors. CL, KW, RW, and ZY contributed to obtaining the field measured data. FC and SL contributed to the model validation for the winter wheat–summer maize cropping system.
The authors declare that they have no conflict of interest.
This study was jointly supported by the National Key R&D Program of China (grant no. 2016YFA0602303) and the National Natural Science Foundation of China (grant nos. 41603075 and 41761144054).
This research has been supported by the National Key R&D Program of China (grant no. 2016YFA0602303) and the National Natural Science Foundation of China (grant nos. 41603075 and 41761144054).
This paper was edited by Paul Stoy and reviewed by three anonymous referees.
Bell, M. J., Jones, E., Smith, J., Smith, P., Yeluripati, J., Augustin, J., Juszczak, R., Olejnik, J., and Sommer, M.: Simulation of soil nitrogen, nitrous oxide emissions and mitigation scenarios at 3 European cropland sites using the ECOSSE model, Nutr. Cycl. Agroecosyst., 92, 161–181, 2012.
Chen, X., Cui, Z., Vitousek, P., Cassman, K., Matson, P., Bai, J., Meng, Q., Hou, P., Yue, S., Romheld, V., and Zhang, F.: Integrated soil-crop system management for food security, P. Natl. Acad. Sci. USA, 108, 6399–6404, 2011.
Chen, X., Cui, Z., Fan, M., Vitousek, P., Zhao, M., Ma, W., Wang, Z., Zhang, W., Yan, X., Yang, J., Deng, X., Gao, Q., Zhang, Q., Guo, S., Ren, J., Li, S., Ye, Y., Wang, Z., Huang, J., Tang, Q., Sun, Y., Peng, X., Zhang, J., He, M., Zhu, Y., Xue, J., Wang, G., Wu, L., An, N., Wu, L., Ma, L., Zhang, W., and Zhang, F.: Producing more grain with lower environmental costs, Nature, 514, 486–489, 2014.
China Statistical Yearbook: Agriculture, Complied by National Bureau of Statistics of China, China Statistics Press, Beijing, available at: http://www.stats.gov.cn/tjsj/ndsj/2017/indexch.htm (last access: 3 November 2017), 2017.
Chirinda, N., Kracher, D., Lægdsmand, M., Porter, J. R., Olesen, J. E., Petersen, B. M., Doltra, J., Kiese, R., and Butterbach-Bahl, K.: Simulating soil N2O emissions and heterotrophic CO2 respiration in arable systems using FASSET and MoBiLE-DNDC, Plant Soil, 343, 139–160, 2011.
Collins, A. L., Zhang, Y. S., Winter, M., Inman, A., Jones, J. I., Johnes, P. J., Cleasby, W., Vrain, E., Lovett, A., and Noble, L.: Tackling agricultural diffuse pollution: What might uptake of farmer-preferred measures deliver for emissions to water and air?, Sci. Total Environ., 547, 269–281, 2016.
Congreves, K. A., Grant, B. B., Dutta, B., Smith, W. N., Chantigny, M. H., Rochette, and Desjardins, R. L.: Prediction ammonia volatilization after field application of swine slurry: DNDC model development, Agr. Ecosyst. Environ., 219, 179–189, 2016.
Cui, F., Zheng, X., Liu, C., Wang, K., Zhou, Z., and Deng, J.: Assessing biogeochemical effects and best management practice for a wheat–maize cropping system using the DNDC model, Biogeosciences, 11, 91–107, https://doi.org/10.5194/bg-11-91-2014, 2014.
Delgrosso, S., Mosier, A., Parton, W., and Ojima, D.: DAYCENT model analysis of past and contemporary soil NO and net greenhouse gas flux for major crops in the USA, Soil Till. Res., 83, 9–24, 2005.
Ding, W., Meng, L., Yin, Y., Cai, Z., and Zheng, X.: CO2 emission in an intensively cultivated loam as affected by long-term application of organic manure and nitrogen fertilizer, Soil Biol. Biochem., 39, 669–679, 2007.
Dubache, G., Li, S., Zheng, X., Zhang, W., and Deng, J.: Modeling ammonia volatilization following urea application to winter cereal fields in the United Kingdom by improving a biogeochemical model, Sci. Total Environ., 660, 1403–1418, 2019.
Galloway, J. N., Dentenerd, F. J., Capone, D. G., Boyer, E. W., Howarth, R. W., Seitzinger, S. P., Asner, G. P., Cleveland, C. C., Green, P. A., Holland, E. A., Karl, D. M., Michaels, A. F., Porter, J. H., Townsend, A. R., and Vorosmarty, C. J.: Nitrogen Cycles: past, present, and future, Biogeochemistry, 70, 153–226, 2004.
Gao, B., Ju, X., Meng, Q., Cui, Z., Christie, P., Chen, X., and Zhang, F.: The impact of alternative cropping systems on global warming potential, grain yield and groundwater use, Agr. Ecosyst. Environ., 203, 46–54, 2015.
Garnett, T., Appleby, M. C., Balmford, A., Bateman, I. J., Benton, T. G., Bloomer, P., Burlingame, B., Dawkins, M., Dolan, L., and Fraser, D.: Sustainable intensification in agriculture: premises and policies, Science, 341, 33–34, 2013.
Haas, E., Klatt, S., Fröhlich, A., Kraft, P., Werner, C., Kiese, R., Grote, R., Breuer, L., and Butterbach-Bahl, K.: LandscapeDNDC: a process model for simulation of biosphere-atmosphere-hydrosphere exchange processes at site and regional scale, Landscape Ecol., 28, 615–636, 2012.
Han, C.: Temporal and spatial variation of soil nutrients of long-term monocultural cotton field and soil sustainable utilization in Xinjiang, Dissertation, Shihezi University, 2010.
Han, P., Zhang, W., Wang, G., Sun, W., and Huang, Y.: Changes in soil organic carbon in croplands subjected to fertilizer management: a global meta-analysis, Sci. Rep.-UK, 6, 27199, https://doi.org/10.1038/srep27199, 2016.
IPCC (Intergovernmental Panel on Climate Change): Climate Change 2013: The Physical Science Basis, Contribution of Working Group I to the Fifth Assessment Report of the Intergovernmental Panel on Climate Change, edited by: Stocker, T. F., Qin, D., Plattner, G.-K., Tignor, M. B., Allen, S. K., Boschung, J., Nauels, A., Xiao, Y., Bex, V., and Midgley, P. M., Cambridge University Press, Cambridge, UK and New York, NY, USA, 2013.
Jiang, Z.: Analysis on the establishment conditions of the square sum decomposition formular of regression model, J. Industr. Techn. Econ., 29, 116–119, 2010 (in Chinese).
Ju, X., Xing, G., Chen, X., Zhang, S., Zhang, L., Liu, X., Cui, Z., Yin, B., Christie, P., Zhu, Z., and Zhang, F.: Reducing environmental risk by improving N mannagement in intensive Chinese agricultural systems, P. Natl. Acad. Sci. USA, 106, 3041–3046, 2009.
Kröbel, R., Sun, Q., Ingwersen, J., Chen, X., Zhang, F., Müller, T., and Römheld, V.: Modelling water dynamics with DNDC and DAISY in a soil of the North China Plain: A comparative study, Environ. Modell. Softw., 25, 583–601, 2010.
Lehuger, S., Gabrielle, B., Laville, P., Lamboni, M., Loubet, B., and Cellier, P.: Predicting and mitigating the net greenhouse gas emissions of crop rotations in Western Europe, Agr. Forest Meteorol., 151, 1654–1671, 2011.
Li, C.: Modeling trace gas emissions from agricultural ecosystems, Nutr. Cycl. Agroecosyst., 58, 259–276, 2000.
Li, C.: Quantifying greenhouse gas emissions from soils: Scientific basis and modeling approach, J. Soil Sci. Plant Nutr., 53, 344–352, 2007.
Li, C.: Biogeochemistry: Scientific Fundamentals and Modelling Approach, Tsinghua University Press, Beijing, 2016 (in Chinese).
Li, C., Frolking, S., and Frolking, T. A.: A model of nitrous oxide evolution from soil driven by rainfall events: 1. Model Structure and Sensitivity, J. Geophys. Res., 97, 9759–9776, 1992.
Li, M., Liang, W., Zheng, X., Yang, Z., Zheng, P., Chen, Y., and Chen, D.: Characteristics of NO emission from typical saline soil of southern Shanxi cotton land, Climatic Environ. Res., 14, 318–328, 2009 (in Chinese).
Li, Q., Liao, N., Zhang, N., Zhou, G., Zhang, W., Wei, X., Ye, J., and Hou, Z.: Effects of cotton (Gossypium hirsutum L.) straw and its biochar application on NH3 volatilization and N use efficiency in a drip-irrigated cotton field, J. Soil Sci. Plant Nutr., 62, 534–544, 2016.
Li, S., Zheng, X., Zhang, W., Han, S., Deng, J., Wang, K., Wang, R., Yao, Z., and Liu, C.: Modeling ammonia volatilization folllowing the application of synthetic fertilizers to cultivated uplands with calcareous soils using an improved DNDC biogeochemstry model, Sci. Total Environ., 660, 931–946, 2019.
Linn, D. M. and Doran, J. W.: Effect of water-filled pore space on carbon dioxide and nitrous oxide production in tilled and nontilled soils, Soil Sci. Soc. Am. J., 48, 1267–1272, 1984.
Liu, C., Zheng, X., Zhou, Z., Han, S., Wang, Y., Wang, K., Liang, W., Li, M., Chen, D., and Yang, Z.: Nitrous oxide and nitric oxide emissions from an irrigated cotton field in Northern China, Plant Soil, 332, 123–134, 2010.
Liu, C., Wang, K., Meng, S., Zheng, X., Zhou, Z., Han, S., Chen, D., and Yang, Z.: Effects of irrigation, fertilization and crop straw management on nitrous oxide and nitric oxide emissions from a wheat-maize rotation field in northern China, Agr. Ecosyst. Environ., 140, 226–233, 2011.
Liu, C., Wang, K., and Zheng, X.: Responses of N2O and CH4 fluxes to fertilizer nitrogen addition rates in an irrigated wheat-maize cropping system in northern China, Biogeosciences, 9, 839–850, https://doi.org/10.5194/bg-9-839-2012, 2012.
Liu, C., Yao, Z., Wang, K., and Zheng, X.: Three-year measurements of nitrous oxide emissions from cotton and wheat-maize rotational cropping systems, Atmos. Environ., 96, 201–208, 2014.
Liu, C., Yao, Z., Wang, K., and Zheng, X.: Effects of increasing fertilization rates on nitric oxide emission and nitrogen use efficiency in low carbon calcareous soil, Agr. Ecosyst. Environ., 203, 83–92, 2015.
Liu, C., Yao, Z., Wang, K., Zheng, X., and Li, B.: Net ecosystem carbon and greenhouse gas budgets in fiber and cereal cropping systems, Sci. Total Environ., 647, 895–904, 2019.
Lv, J., Liu, X., Liu, H., Wang, X., Li, K., Tian, C., and Christie, P.: Greenhouse gas intensity and net annual global warming potential of cotton cropping systems in an extremely arid region, Nutr. Cycl. Agroecosyst., 98, 15–26, 2014.
Moriasi, D. N., Arnold, J. G., Van Liew, M. W., Bingner, R. L., Harmel, R. D., and Veith, T. L.: Model evaluation guidelines for systematic quantification of accuracy in watershed simulation, T. Am. Soc. Agr. Biol. Eng., 50, 885–900, 2007.
Nash, J. E. and Sutcliffe, J. V.: River flow forecasting through conceptual models: part I – a discussion of principles, J. Hydrol., 10, 282–290, 1970.
Palosuo, T., Foereid, B., Svensson, M., Shurpali, N., Lehtonen, A., Herbst, M., Linkosalo, T., Ortiz, C., Rampazzo Todorovic, G., Marcinkonis, S., Li, C., and Jandl, R.: A multi-model comparison of soil carbon assessment of a coniferous forest stand, Environ. Modell. Softw., 35, 38–49, 2012.
Tong, H., Zheng, X., Wang, R., Zhou, Z., Yue, J., Liu, C., Li, M., Liang, W., and Dong, H.: A preliminary study of measurement of NH3 volatilization from cropland using Qusi-dynamic chamber, Clim. Environ. Res., 14, 373–382, 2009 (in Chinese).
Wang, E., Yu, Q., Wu, D., and Xia, J.: Climate, agricultural production and hydrological balance in the North China Plain, Int. J. Climatol., 28, 1959–1970, 2008.
Wang, K., Liu, C., Zheng, X., Pihlatie, M., Li, B., Haapanala, S., Vesala, T., Liu, H., Wang, Y., Liu, G., and Hu, F.: Comparison between eddy covariance and automatic chamber techniques for measuring net ecosystem exchange of carbon dioxide in cotton and wheat fields, Biogeosciences, 10, 6865–6877, https://doi.org/10.5194/bg-10-6865-2013, 2013a.
Wang, K., Zheng, X., Pihlatie, M., Vesala, T., Liu, C., Haapanala, S., Mammarella, I., Rannik, Ü., and Liu, H.: Comparison between static chamber and tunable diode laser-based eddy covariance techniques for measuring nitrous oxide fluxes from a cotton field, Agr. Forest. Meteorl., 171–172, 9–19, 2013b.
Wang, R., Feng, Q., Liao, T., Zheng, X., Butterbach-Bahl, K., Zhang, W., and Jin, C.: Effects of nitrate concentration on the denitrification potential of a calcic cambisol and its fractions of N2, N2O and NO, Plant Soil, 363, 175–189, 2013.
Willmott, C. J. and Matsuurra, K.: Advantages of the mean aboslute error (MAE) over the root mean square error (RMSE) in assessing average model performance, Clim. Res., 30, 79–82, 2005.
Xu, Q., Li, Z., Hu, K., and Li, B.: Optimal management of water and nitrogen for farmland in North China Plain based on osculating value method and WHCNS model, Transaction of the Chinese Society of Agricultural Engineering, 33, 152–158, 2017 (in Chinese).
Yang, Z., Turner, D., Zhang, J., Wang, Y., Chen, M., Zhang, Q., Denmead, Q., Che, D., and Freney, J.: Loss of nitrogen by ammonia volatilzation and denitrification after application of urea to maize in Shanxi Province, China, Soil Res., 49, 462–469, 2011.
Zhang, W., Liu, C., Zheng, X., Zhou, Z., Cui, F., Zhu, B., Haas, E., Klatt, S., Butterbach-Bahl, K., and Kiese, R.: Comparison of the DNDC, LandscapeDNDC and IAP-N-GAS models for simulating nitrous oxide and nitric oxide emissions from the winter wheat-summer maize rotation system, Agr. Syst., 140, 1–10, 2015.
Zhang, W., Li, Y., Zhu, B., Zheng, X., Liu, C., Tang, J., Su, F., Zhang, C., Ju, X., and Deng, J.: A process-oriented hydro-biogeochemical model enabling simulation of gaseous carbon and nitrogen emissions and hydrologic nitrogen losses from a subtropical catchment, Sci. Total Environ., 616–617, 305–317, 2018.
Zhang, X., Bol, R., Rahn, C., Xiao, G., Meng, F., and Wu, W.: Agricultural sustainable intensification improved nitrogen use efficiency and maintained high crop yield during 1980–2014 in Northern China, Sci. Total Environ., 596–597, 61–68, 2017.
Zhang, Y., Wang, R., Pan, Z., Liu, Y., Zheng, X., Ju, X., Zhang, C., Butterbach-Bahl, K., and Huang, B.: Fertlizer nitrogen loss via N2 emission from calcareous soil following basal urea application of winter wheat, Atmos, Oceanic Sci. Lett., 12, 91–97, 2019.
Zhao, X., Liu, S. L., Pu, C., Zhang, X. Q., Xue, J. F., Zhang, R., Wang, Y. Q., Lal, R., Zhang, H. L., and Chen, F.: Methane and nitrous oxide emissions under no-till farming in China: a meta-analysis, Glob. Change Biol., 22, 1372–1384, 2016.
Zheng, X., Xie, B., Liu, C., Zhou, Z., Yao, Z., Wang, Y., Wang, Y.,Yang, L., Zhu, J., Huang, Y., and Butterbach-Bahl, K.: Quantifying net ecosystem carbon dioxide exchange of a short-plant cropland with intermittent chamber measurements, Global Biogeochem. Cy., 22, GB3031, https://doi.org/10.1029/2007GB003104, 2008.