the Creative Commons Attribution 4.0 License.
the Creative Commons Attribution 4.0 License.
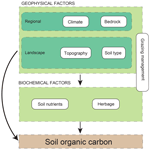
Interactions between biogeochemical and management factors explain soil organic carbon in Pyrenean grasslands
Antonio Rodríguez
Rosa Maria Canals
Josefina Plaixats
Elena Albanell
Haifa Debouk
Jordi Garcia-Pausas
Leticia San Emeterio
Àngela Ribas
Juan José Jimenez
M.-Teresa Sebastià
Grasslands are one of the major sinks of terrestrial soil organic carbon (SOC). Understanding how environmental and management factors drive SOC is challenging because they are scale-dependent, with large-scale drivers affecting SOC both directly and through drivers working at small scales. Here we addressed how regional, landscape and grazing management, soil properties and nutrients, and herbage quality factors affect 20 cm depth SOC stocks in mountain grasslands in the Pyrenees. Taking advantage of the high variety of environmental heterogeneity in the Pyrenees, we built a dataset (n=128) that comprises a wide range of environmental and management conditions. This was used to understand the relationship between SOC stocks and their drivers considering multiple environments. We found that temperature seasonality (difference between mean summer temperature and mean annual temperature; TSIS) was the most important geophysical driver of SOC in our study, depending on topography and management. TSIS effects on SOC increased in exposed hillsides, slopy areas, and relatively intensively grazed grasslands. Increased TSIS probably favours plant biomass production, particularly at high altitudes, but landscape and grazing management factors regulate the accumulation of this biomass into SOC. Concerning biochemical SOC drivers, we found unexpected interactive effects between grazer type, soil nutrients and herbage quality. Soil N was a crucial SOC driver as expected but modulated by livestock species and neutral detergent fibre contenting plant biomass; herbage recalcitrance effects varied depending on grazer species. These results highlight the gaps in knowledge about SOC drivers in grasslands under different environmental and management conditions. They may also serve to generate testable hypotheses in later/future studies directed to climate change mitigation policies.
- Article
(1393 KB) - Full-text XML
-
Supplement
(1279 KB) - BibTeX
- EndNote
Soil organic carbon (SOC) is crucial for the functioning of terrestrial ecosystems (Lal, 2004a). SOC enhances soil and water quality and biomass productivity and has an important role in relation to climate change (Lal, 2004b). Although grasslands have small aboveground biomass compared to other ecosystems, their SOC stocks can be comparable to those in forest ecosystems (Berninger et al., 2015). This is due to their high root biomass and residues, which are a substantial carbon source and can contribute to water retention in soil. This creates favourable conditions for the accumulation of organic matter (Von Haden and Dornbush, 2014; Yang et al., 2018). These attributes, together with the high extent of grassland global cover, make grasslands store around 34 % of the terrestrial carbon, mostly in their soils (White et al., 2000).
SOC accumulation results from a complex equilibrium between primary production and organic matter decomposition which depends on multiple environmental factors such as climate, soil texture and nutrients, or land management (Jenny, 1941; Schlesinger, 1977). Understanding how these scale-dependent environmental factors drive SOC is challenging because large-scale drivers also affect those working at fine spatial scales. This has been described as a hierarchy of controls over SOC (Fig. 1; Manning et al., 2015).
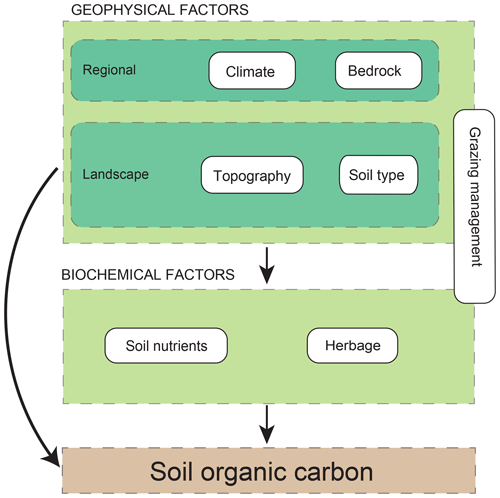
Figure 1Conceptual scheme used in this work to investigate potential environmental drivers with soil organic carbon (SOC). We assume that drivers may affect SOC both directly or hierarchically through another driver. Interactions between factors acting at different scales and belonging to different categories could also drive SOC. Grazing management has a special status because it may be acting at different scales, landscape and local.
Climate is known to be the main SOC driver at broad (global and regional) scales, mean annual precipitation (MAP) and mean temperature (MAT) being the most frequent climate indicators (Wiesmeier et al., 2019). However, climate annual variations represented by seasonality variables are commonly neglected when considering possible SOC drivers in broad-scale models, in spite of being important drivers of plant primary production and enzymatic activity of soil microorganisms (Fernández-Alonso et al., 2018; Garcia-Pausas et al., 2007; Puissant et al., 2018). Other regional and landscape factors like bedrock or topography are also considered to be at the top of the hierarchy because they influence multiple geophysical and biochemical factors affecting SOC, including soil texture and water flow paths (Gray et al., 2015; Hobley et al., 2015). Next in the hierarchy after regional and landscape factors are several soil geophysical properties, like pH and texture, which are controlled by climate and bedrock and which affect SOC through both plant primary production and microbial activity and the capacity to stabilize the SOC (Deng et al., 2016; Xu et al., 2016a).
Soil macro- and micro-nutrients are in the next level of the hierarchy, as their abundance is determined by multiple factors, including climate, soil pH, water content or clay content (Hook and Burke, 2000; de Vries et al., 2012). They play an essential role in primary production and herbage quality and act as resources for microbes to mineralize SOC (Aerts and Chapin, 1999; Vitousek and Howarth, 1991). However, these variables are commonly omitted as possible drivers of SOC in the broad-scale studies, especially in those studies focusing on predictive rather than explicative models (Gray et al., 2015; Manning et al., 2015; Zhang et al., 2018). This kind of variable is less frequently available and more difficult to measure than the other indicators used for SOC modelling (Manning et al., 2015). Furthermore, the use of soil nutrients as SOC drivers in linear models can be challenging, as they are often strongly linked to SOC dynamics. This may mask the effect of other drivers acting at larger spatial scales (Bing et al., 2016; Cleveland and Liptzin, 2007; Tipping et al., 2016).
Vegetation represents another group of SOC drivers affected by climate, topography and soil properties and nutrients (Fernández-Martínez et al., 2014; de Vries et al., 2012; Zhu et al., 2019). Plant biomass is the main input of organic carbon into the soil (Shipley and Parent, 1991). However, a not so frequently considered factor is plant litter quality, which can determine decomposition rates and patterns and hence soil carbon sequestration (Ottoy et al., 2017; Yan et al., 2018, 2019).
In addition to these factors, livestock management effects on grassland SOC are a noteworthy issue since they are poorly understood (Wiesmeier et al., 2019). It is known that herbivores can affect SOC through different paths, such as regulating the quantity and quality of organic matter returned to soil (Bardgett and Wardle, 2003) or affecting soil respiration and nutrients by animal trampling or soil microbiota alteration (Lu et al., 2017). Several studies confirmed the interaction between grazing and other SOC drivers at diverse scales (Abdalla et al., 2018; Eze et al., 2018; Lu et al., 2015, 2017; Zhou et al., 2017). Hence, grazing management may be considered a SOC driver with effects at multiple levels of the driver hierarchy (Fig. 1), both affecting other SOC drivers and interacting with them. However, most of the studies investigating grazing effects on SOC focus on grazing intensity in spite of evidence pointing to a greater role of grazer species in determining vegetation and SOC (Chang et al., 2018; Sebastià et al., 2008b).
In this study, our goal was to identify the main drivers of SOC stocks and their interactions in Pyrenean mountain grasslands. For this purpose, we considered a wide set of regional, landscape, soil geophysical and biochemical, and herbage quality factors, together with grazing management factors. Mountain grasslands comprise a wide range of all these conditions, which make carbon stocks highly variable (Garcia-Pausas et al., 2007, 2017). For this reason, data analysed here include a wide range of environmental conditions, comparable to studies on SOC developed at continental or even worldwide scales (Table 1). Additionally, we considered an exceptionally broad compilation of drivers (Table 1). To deal with correlations and interactions between SOC drivers, we developed an exhaustive modelling approach based on the controls over function hypothesis (de Vries et al., 2012). To facilitate the formulation of our specific questions to answer in this study, we classified SOC drivers into three main groups (Fig. 1): (i) geophysical factors, which include regional and landscape factors and are supposed to be the first sources of variation, (ii) biochemical factors, which include soil nutrients and herbage factors and could be conditioned by geophysical factors, and (iii) grazing management factors, which could affect SOC through multiple interactions with the rest of the variables at multiple scales. In particular, the specific questions of this study are the following. (1) What are the relative and interaction effects of the geophysical and biochemical SOC controls? (2) How does grazing management regulate the effects of other SOC drivers?
Table 1Considered factors affecting SOC stocks in some recent studies. V: the study considers this variable type; –: the study does not consider this variable type. In italics: present study.
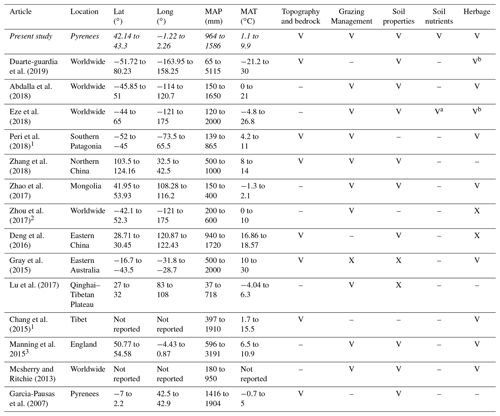
1 It considers SOC concentrations. 2 It considers total carbon stocks. 3 It considers total carbon stocks and its fractions. a Fertilizer effects. b Only aboveground and/or belowground biomass index.
2.1 Location and sampling design
The set of data used in this study has been extracted from the PASTUS Database (http://ecofun.ctfc.cat/?p=3538, last access: 12 January 2019), which was compiled by the Laboratory of Functional Ecology and Global Change (ECOFUN) of the Forest Sciences Centre of Catalonia (CTFC) and the University of Lleida (UdL). We sourced a wealth of data of 128 grassland patches distributed across the Pyrenees (Fig. S1 in the Supplement) and including topographical, climate, soil, herbage and management variables. The elaboration of the PASTUS Database concerning this study is summarized in Fig. S2. The sampled area encompasses a wide variety of temperate and cold–temperate climates with different precipitation conditions, depending on altitude and geographical location, from Mediterranean to continental and Boreo-Alpine environments (de Lamo and Sebastià, 2006; Rodríguez et al., 2018; Table 1). Almost all of the plant species in the grasslands from the PASTUS Database are perennial (Sebastià, 2004), and plant diversity is highly heterogeneous, as are the environmental conditions (Rodríguez et al., 2018).
Sampling in the PASTUS Database was designed according to a stratified random scheme, where samples were selected at random within strata. This process was done using the ArcMap 10 software (ESRI, Redlands, CA, USA). The basis for randomization was the map of habitats of Catalonia 1 : 50 000 (Carreras and Diego, 2006) for the eastern and central sectors of the Pyrenees, the map of habitats of Madres-Coronat 1 : 10 000 (Penin, 1997) for the north-eastern sector and the land use map of Navarra 1 : 25 000 (Gobierno de Navarra, 2003) for the western sectors. Four variables were initially considered for sampling stratification within each sector: altitude (< 1800; 1800–2300; >2300 m), slope (0–20; 20–30; > 30∘), macrotopography (mountain top/south-facing slope; valley bottom/north-facing slope) and grazer type (sheep; cattle; mixed). Accordingly, we determined a set of homogeneous grassland patches by crossing the stratification variable layers. Grassland patches were then listed by type and arranged within each list randomly to determine sampling priority. At least one to two replicates of each patch type defined by the stratification variables were sampled.
In each sampled grassland patch, a 10×10 m2 plot was systematically placed in the middle of each homogeneous grassland patch, including a particular plant community. We collected soil and vegetation samples and assessed environmental variables within each 100 m2 plot (see Rodríguez et al., 2018, for additional details about sampling design). Local variables were assessed inside the 100 m2 plots. Aboveground biomass was estimated from herbage cut at ground level in four 50×50 cm2 quadrats placed in a 2×2 m2 subplot inside the 100 m2 plot. Herbage from two of the four quadrats was dried and sent to the laboratory for duplicated chemico-bromatological analysis. In addition, in each quadrat, a 20 cm depth soil core was extracted with a 5×5 cm probe after herbage was removed. The soil sample in the probe was separated into two soil layers: 0–10 and 10–20 cm.
2.2 Regional and landscape environmental drivers
In order to investigate the relationship between SOC and potential environmental drivers, 30 independent environmental variables were initially considered (Table S1 in the Supplement). These variables were grouped into five sets: regional, landscape, livestock management, soil nutrient stocks, and herbage variables.
Regional variables included climate variables and bedrock. Climate variables were determined from Worldclim 2.0 (Fick and Hijmans, 2017). We selected mean annual temperature (MAT), mean summer temperature (MST), mean annual precipitation (MAP) and mean summer precipitation (MSP). The difference between mean summer and mean annual temperature emerged as a relevant explanatory factor of soil organic carbon stocks during previous modelling efforts by one of the co-authors (M.-Teresa Sebastià). Later attempts to improve models by substituting this variable with other temperature indices from climatic databases (Fick and Hijmans, 2017) showed that, for the PASTUS Database, this variable provided higher explanatory power than other temperature seasonality indices. Thus, we decided to keep it, and here we name it the Temperature Seasonality Index of Sebastià (TSIS from now on).
Bedrock type was determined in the field and confirmed by the geographical maps mentioned above. Bedrock was categorized into three categories: basic (marls and calcareous rocks), acidic (mostly sandstones and slates) and heterogeneous.
Landscape variables included topography and soil-type variables. Topography variables included slope, aspect, macrotopography and microtopography. Slope and aspect were determined in the field by clinometer and compass respectively. Macrotopography and microtopography were determined visually in the field. Preliminary modelling efforts suggested the reduction of the four macrotopographical positions initially identified in the field into two: mountain top and south-facing slopes were classified as exposed positions and valley bottoms and north-facing slopes as protected macrotopographical positions. Microtopography included three positions: convexities, concavities and smooth areas. Soil-type variables are described in the following.
2.3 Soil physicochemical analysis
To obtain bulk density, we air-dried and weighed the soil samples: we then sieved each sample to 2 mm to separate stones and gravels from the fine earth fraction. The fine fraction was sent to the laboratory for further physicochemical analysis. Standard physicochemical soil analyses were performed in the upper 0–10 cm soil layer of all grasslands. Some analyses were also performed on samples from the 10–20 cm soil layer, including soil organic carbon and total nitrogen. For those variables, we combined 0–10 and 10–20 cm values to obtain the whole top 20 cm soil layer.
All soil physicochemical analyses were performed on the fine earth, according to standard soil analysis methods. Textural classes were determined by the Bouyoucos method (Bouyoucos, 1936). Soil pH (measured in water), total organic carbon (TOC), total nitrogen (TN), calcium content (Ca), extractable phosphorus (P), magnesium (Mg) and potassium (K) were measured on air-dried samples (Schöning et al., 2013; Solly et al., 2014). Soil carbonates were determined using the Bernard calcimeter. Total carbon and nitrogen (N) contents of the fine earth were determined by an elemental auto-analyser. The organic C fraction was determined by subtracting inorganic C in the carbonates from the total C. Available phosphorus (P) was extracted by the Olsen method (Olsen, 1954). Ca, Mg and K were extracted by ammonium acetate (Simard, 1993) and measured by flame atomic absorption spectroscopy (AAS); David, 1960). Soil organic carbon (SOC) stocks in the upper 20 cm soil layer were then estimated by taking into account the organic C concentration in the sample and its bulk density and subtracting the coarse-particle (>2 mm) content, following García-Pausas et al. (2007). Despite recent studies suggesting that fixed mass SOC stocks estimates are preferable to fixed depth methods because they would be more robust to temporal and land use changes in bulk density (Ellert and Bettany 1995), we chose a fixed depth method for measuring SOC stocks. This decision was based on the fact that our work samples came from natural mountain grasslands, where grazing intensity is always low to moderate, and moreover, herbivore presence is seasonal. Therefore, we do not expect important changes in bulk density due to land use. Additionally fixed mass approaches presented the disadvantages of implying more technical difficulties than fixed depth measures, even in the most modern procedures (Haden et al., 2020), and could not deal well with differences in stoniness.
2.4 Herbage chemical and bromatological analysis and NIRS analysis
All four herbage samples per plot were oven-dried at 60 ∘C to constant weight to determine aboveground biomass and converted into g m−2. Two out of the four samples were sent to the laboratory for herbage quality analysis. Dried samples were ground to pass a 1 mm stainless steel screen (Cyclotec 1093 Sample mill, Tecator, Sweden) and stored at 4 ∘C until it was needed for use.
To develop NIRS prediction models, a random subset of 130 samples was used and analysed in duplicate according to the reference methods mentioned further. Procedures described by AOAC were used to determine dry matter (DM) and ash content or mineral matter (MM). Crude protein (CP) was determined by the Kjeldhal procedure (N × 6.25) using a Kjeltec Auto 1030 Analyser (Tecator, Sweden). Samples were analysed sequentially for neutral detergent fibre (NDF), acid-detergent fibre (ADF) and acid-detergent lignin (ADL) in accordance with the method described in Van Soest et al. (1991) using the Ankom 200 Fibre Analyser incubator (Ankom, USA). The fibre analysis was determined on an ash-free basis and without alpha amylase. We calculated two additional herbage quality indexes often used in the bibliography: NDF∕CP and ADL∕NH (Stockmann et al., 2013). For each subsample the C and N contents (CH and NH) were determined by the Dumas dry combustion method using an Elemental Analyzer EA1108 (Carlo Erba, Milan, Italy).
Afterwards, a total of 200 herbage samples were scanned as described below to collect their NIRS spectra. We estimated chemical and bromatological variables according to the equations derived from the previous calibrations on the initial 130 random samples.
NIRS data were recorded from 1100 to 2500 nm using a FOSS NIRSystem 5000 scanning monochromator (Hillerød, Denmark). Separate calibration equations were generated for grassland samples. Reflectance (R) data were collected in duplicate every 2 nm. WinISI III (v. 1.6) software (FOSS, Denmark) was employed for spectra data analysis and development of chemometric models. Prior to calibration, log 1∕R spectra were corrected for the effects of scatter using the standard normal variate (SNV), detrend (DT) and multiple scatter correction (MSC) and transformed into first or second derivatives using a different gap size (nm) and smoothing interval. For each sample, the mean of the spectra from the two lectures was used. Modified partial least squares (MPLS) was the regression method used for calibration development, and cross-validation was undertaken using the standard methodology in the NIRS software program. The performance of the model was determined by the following statistical tools: standard error of calibration (SEC); standard error of cross-validation (SECV); coefficient of determination for calibration (R2) and cross-validation (); the ratio of performance to deviation (RPD) described as the ratio of the standard deviation for the validation samples to the standard error of cross-validation (RPD = SD ∕ SECV) should ideally be at least 3, and the range error ratio (RER = Range ∕ SECV) described as the ratio of the range in the reference data to the SECV should be at least 10 (Williams and Sobering, 1996; Williams et al., 2014).
2.5 Livestock management variables
The management variables (grazer type) initially used for sampling stratification were determined from records available in the municipalities of the study area. Once the specific grassland patches to be sampled were determined, we carried out a detailed analysis of the management where the patches were located. To this effect, we carried out detailed surveys among farmers, shepherds and land managers. Sometimes the information collected was modified according to visual records in the field (e.g. cattle and/or cattle dung found in supposedly ungrazed areas). Information from municipalities was usually the most imprecise.
We considered two management variables: grazing intensity and grazer type. Grazing intensity was determined by estimating livestock stocking rates measured as livestock units ha−1 (LU ha−1) and treated as a semi-quantitative variable with three categories: low (1; lower than 0.2 LU ha−1), medium (2; between 0.2 and 0.4 LU ha−1) and high (3; above 0.4 LU ha−1). Grazer type was categorized into three main types: sheep, cattle and mixed. Mixed grazing included associations comprising small and big livestock species, mainly sheep and cattle, and more rarely horses. Sheep flocks always included some goats.
2.6 Statistical analyses
We applied two different modelling procedures: boosted regression trees (BRTs) and general linear models (GLMs). BRT is an automatic technique that combines insights from traditional statistical modelling and machine learning traditions (Elith et al., 2008). GLMs allowed us to design a hypothesis-based modelling procedure, ensuring that only effects with biological meaning were included, whereas BRT provided information about the data that could be neglected if only a GLM approach was followed.
All the statistical analyses were performed with the R ver. 3.4.3 software (R Core Team, 2016) at a 95 % significance level when appropriate.
2.6.1 Boosted regression trees global model
Including all SOC potential drivers, we fitted a model with BRT to identify the most important variables affecting SOC. BRT uses two algorithms: regression trees and boosting. Regression trees are from the decision tree group of models, and boosting builds and combines a collection of models (Elith et al., 2008). We chose this method because BRT can handle multiple variables better than other techniques such as GLMs and can detect automatically curvilinear relationships and interactions, ignoring non-informative ones. We used the gbm and dismo packages (Greenwell et al., 2019; Hijmans et al., 2017), which provide several functions to fit these models.
Firstly, we fitted a model with all the drivers (Table S1), configured with 15 folds, a Gaussian distribution of the error, a tree complexity of 5, a learning rate of 0.005, a bag fraction of 0.666, and five minimum observations by node. Secondly, we reduced the number of drivers by the method described in Elith et al. (2008). We estimated the change in the model's predictive deviance, dropping one by one each driver, and re-fitted the model with the set of variables which actually improved model performance (Fig. S3). We checked the relative importance of the drivers and the shape and size of the effects by partial effect plots.
2.6.2 General linear models
We designed and executed a modelling procedure based on general linear models (Legendre and Legendre, 1998) and a hierarchy of controls over function (Díaz et al., 2007; de Vries et al., 2012). We log-transformed SOC using the natural logarithm to prevent a breach of the normality assumption by the residuals of the models (Fig. S4). We built two models (Fig. S5), one model based only on geophysical drivers and grazing management (geophysical model) and another model including, in addition to the former drivers, the biochemical drivers: soil nutrients and herbage quality (combined model). With this approach we aimed to avoid ignoring significant effects of the geophysical variables, the original source of variation of SOC stocks according to the hierarchy of controls over function hypothesis (Manning et al., 2015), by masking them with the inclusion of biochemical drivers. We considered that the geophysical factors that potentially affect SOC were regional and landscape (topography and soil-type drivers), as they have been widely used in previous studies to model and predict SOC from landscape to continental scales (Manning et al., 2015; Wiesmeier et al., 2019). In addition to soil nutrients and herbage variables, we included again the livestock management variables in the combined model and looked for interactions involving these variables and biochemical drivers of SOC.
For model building (Fig. S5a), we added driver groups following a sequential order. To fit the geophysical model, we started adding regional, landscape and grazing management drivers and subsequently included soil properties. Afterwards, we sequentially included soil nutrients and herbage drivers to obtain the combined model. We added management variables from the beginning of the modelling process and re-included the discarded ones in each step to guarantee the detection of interactions between management variables and the rest of the drivers. Each time we added a set of drivers, we first considered their main effects and some quadratic terms which were found by preliminary analyses with the scatterplot.matrix function in R package car (Fox et al., 2018); afterwards we included possible level 2 interactions between all the selected drivers.
At every step we selected several candidate terms by a semi-automatic procedure (Fig. S5c) using a genetic algorithm included in R package glmulti (Calcagno, 2015). We used SOC as a response variable in the first step and the residuals of the previous model in the remaining steps (Fig. S5b). This semi-automatic process began by obtaining a best subset of models using the corrected Akaike information criterion (AICc), appropriate when n∕k is less than 40, n being the sample size and k the number of parameters in the most complex model (Symonds and Moussalli, 2011). We selected the best model and its equivalents obtained by the genetic algorithm, which were those within two Akaike information criterion-corrected (ΔAICc) values of the best model, as a ΔAICc < 2 indicates that the candidate model is almost as good as the best model (Burnham and Anderson, 2002).
For this set of models, we built averaged models using the MUMIn package (Barton, 2015). We calculated partial standardized coefficients, obtained by multiplying the unstandardized coefficient in the model by the partial standard deviation of the variable, which is a function of the standard deviation of the variable, the sample size, the number of variables in the model and the variance inflation factor of the variable (Barton, 2015). We selected all the variables with significant effects (alone or in interaction with each other) in the conditional average model, which was preferred over the full average model because we wanted to avoid excessive shrinkage effects at this moment of the modelling procedure (Grueber et al., 2011).
Then, we added these terms to the consolidated model and made a selection through a backward forward procedure. We used several methods to compare and determine the final model, including the AICc, the adjusted determination coefficient R2 () and model comparison techniques with the “anova()” function in R, using Chi-square tests to test whether the reduction in the residual sum of squares was statistically significant. Once we had the final model, we assessed the significance of each term by removing it and performing an F test. To estimate the significance of the main effects, we also removed the interaction terms in which they were involved to avoid transferring the effects of the main terms to the interaction terms (de Vries et al., 2012). We estimated the variance explained by the models through the adjusted determination coefficient R2 ().
Finally, we estimated the importance of the terms of each model by the lmg method in the relaimpo package (Grömping, 2006) and drew partial effect plots making predictions with R package emmeans (Lenth et al., 2019).
SOC stocks of the upper 20 cm layer ranged between 2.6 and 23 kg m−2, with a median and a mean value of 9.1 and 9.6 kg m−2 respectively. Standard deviation of the mean was 3.15 (n=125). Minimum, maximum, median and mean values of the continuous drivers are shown in Table S2.
3.1 Relative importance of SOC stock drivers
The final BRT global model achieved a good goodness of fit, with a cross-validated correlation value of 48 % and an explained deviance of 88 %. The most important variables explaining SOC stocks (Fig. 2) were soil N (18 %), soil C∕N (14 %) and clay (13 %), although other variables such as aboveground biomass (7 %), ADL (6 %) or silt (6 %) were also relevant for explaining SOC storage. Three important variables in the BRT model, aboveground biomass, silt and soil K, were not selected in the linear models (Tables 2 and 3). Although accounting for a lower importance value than the previous variables (5 %), TSIS was the most relevant among the climate drivers considered. TSIS was also noticeably important in both linear models (Fig. S6), especially in the geophysical model, not only as the main effect, but also in interaction with other variables (lmg: 4 %–10 %). According to the combined linear model, soil nutrient and herbage variables were other important SOC stock drivers (Fig. S7), but many of these effects occurred in interaction with grazer type.
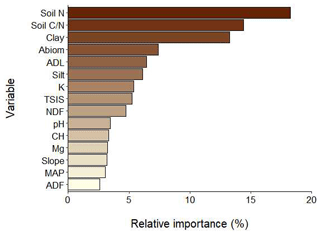
Figure 2Relative contributions (%) of driver variables in the final BRT model obtained. Soil N: soil nitrogen; soil C∕N: soil carbon to nitrogen ratio; clay: clay content; abiom: aboveground biomass; ADL: acid-detergent lignin; loam: loam content; K: soil potassium; TSIS: temperature seasonality; NDF: neutro-detergent fibre; pH: soil pH; CH: carbon in the herbage; Mg: soil magnesium; slope: terrain slope; MAP: mean annual precipitation; ADF: acid-detergent fibre. See Table S1 for more details about variables.
Table 2Results of the geophysical model for soil organic carbon (). MAP: mean annual precipitation; TSIS: temperature seasonality; slope: terrain slope; exposed: exposed position according to macrotopography; clay: clay content; low and medium intensity: low and medium grazing intensity.
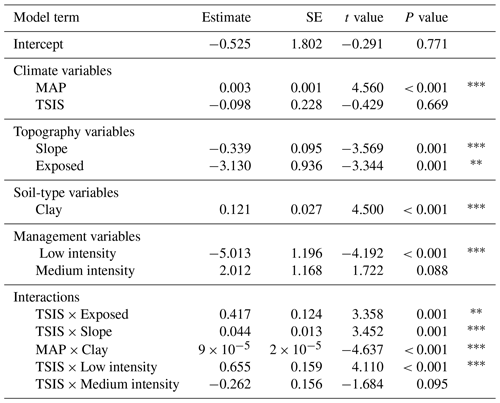
* P value < 0.05. P value < 0.01. P value < 0.001.
Table 3Results of the combined model for soil organic carbon (). MAP: mean annual precipitation; TSIS: mean summer temperature minus mean annual temperature; slope: terrain slope; cattle and mixed: cattle and mixed management according to grazing species; low and medium intensity: low and medium intensity according to grazing intensity; soil C∕N: soil carbon to nitrogen ratio; soil N: soil nitrogen; NDF: neutro-detergent fibre; ADL∕NH: acid-detergent lignin to nitrogen in the herbage ratio.
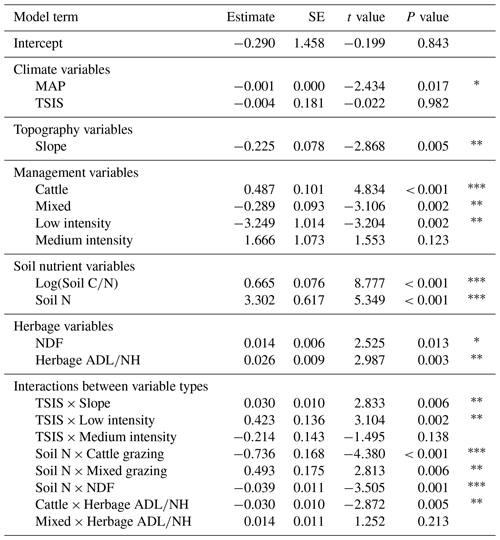
* P value < 0.05. P value < 0.01. P value < 0.001.
3.2 Geophysical, biochemical and grazing management effects on SOC stocks
The geophysical model (Table 2) explained 34 % of the total variance (). Overall, SOC stocks increased with TSIS under certain conditions: exposed hillsides, high slopes and low stocking rates (Fig. 3a, b and d). On the other hand, clay content had a positive relationship with SOC under low MAP values (Fig. 3c), which became negative at high MAP values.
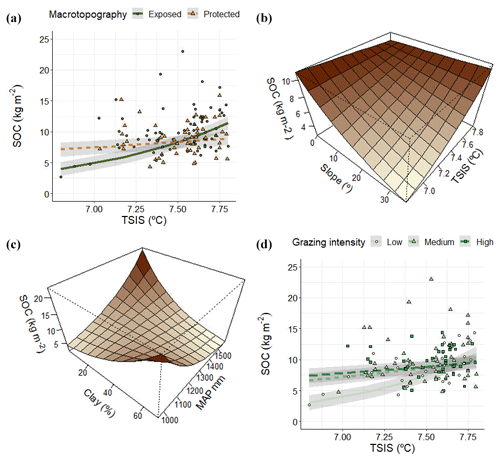
Figure 3Relationship between SOC and regional- and landscape-scale factors in the geophysical model. In (a) solid lines and circles represent exposed hillsides, and dotted lines and triangles indicate protected hillsides. In (d) solid lines and circles indicate low grazing intensity, dotted lines and triangles indicate medium grazing management intensity and dashed lines and squares indicate high grazing management intensity. In (a)–(d) line and plane values are predictions of the model across the corresponding predictors' range according to estimated marginal means. Grey areas around regression lines indicate standard errors. In (a) and (d) points indicate actual values.
Adding nutrient and herbage variables to the previous geophysical model to build the combined model (Table 3) increased the total variance () up to 61 %. Macrotopography and clay effects described by the geophysical model were removed by the new model terms included. SOC increased with C∕N (Fig. 4a). Soil nitrogen modulated the effects of livestock type and NDF on SOC. Cattle-grazed grasslands stored more SOC than mixed and sheep-grazed grasslands under low soil N conditions, whereas the reverse occurred at high soil N levels (Fig. 3b). NDF had negative effects on SOC stocks at high soil N values but had no effect under low soil N levels (Fig. 4c). Finally, herbage ADL∕NH had positive effects on SOC under mixed and sheep-grazing regimes, but there was no effect under cattle management (Fig. 4d).
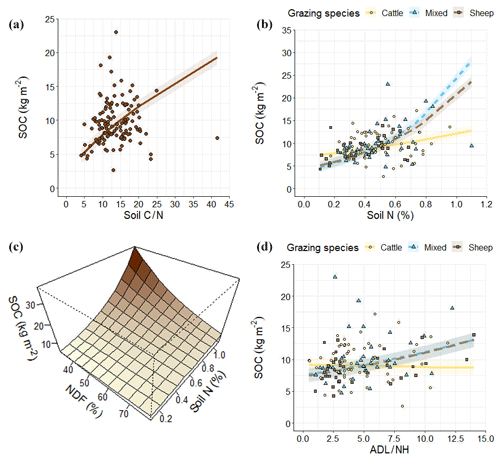
Figure 4The relationship between SOC and biochemical and herbage factors in the combined model. In (b) and (d) solid lines and circle points represent cattle grazing, dashed lines and square points indicate sheep grazing, and dotted lines and triangle points indicate mixed grazing. In (a)–(d) line and plane values are predictions of the model across the corresponding predictors' range according to estimated marginal means. In (a)–(d) line and plane values are predictions of the model across the corresponding predictors' range according to estimated marginal means. The grey spectrum indicates 95 % confidence intervals. In (a) and (d) points indicate actual values.
4.1 Considerations about the modelling procedure
Unsurprisingly, the SOC drivers selected and their main effects in both of the modelling approaches (BRTs and GLMs) were highly congruent (Figs. 2–4, S8). Consequently, we preferred to focus on the results from the linear models because this approximation allowed us to build models under a hierarchy of controls over function hypothesis (Manning et al., 2015). Hence, although it is not possible to unequivocally establish the causal links between SOC drivers (Grace, 2006; Grace and Bollen, 2005), with our GLM procedure we guarantee that the effects of the biochemical variables added in the combined model on SOC stocks have not been exclusively induced by geophysical drivers (de Vries et al., 2012). If this was the case, soil nutrient and herbage quality drivers would not have entered the combined model as significant terms. This happened with aboveground biomass, which is assumed to be a very important SOC driver, and indeed aboveground biomass was relevant in the BRT model, but in the GLM it was substituted by other, more meaningful, variables. In addition, our GLM modelling approach enabled us the selection of biologically meaningful interactions (Manning et al., 2015; de Vries et al., 2012), which cannot be done with a fully automatic approach like BRT. This GLM-sequenced modelling procedure, looking for the primary sources of variation, together with the stratified sampling design, is useful as it led us to select a set of lowly correlated drivers for our linear models (Table S5). Furthermore, the BRT model provided some valuable information, identifying some relevant SOC drivers which were discarded during the GML modelling, like aboveground biomass or soil silt and K (Figs. 2 and S8). The effects of those drivers were probably masked by the effects of other variables in our linear models (Yang et al., 2009), indicating that these factors were presumably pathways through which other variables drove SOC (de Vries et al., 2012). These variables, identified by BRT and discarded by GLMs, should be considered as potential SOC drivers in further studies, particularly when more detailed and difficult-to-obtain biochemical variables, present in our database, are not available.
4.2 Geophysical, biochemical and grazing management factors driving SOC stocks
Considering the difficulties of modelling SOC in a widely heterogeneous mountain environment (Garcia-Pausas et al., 2017), the geophysical model provided important information about broad-scale and topographic SOC drivers in the Pyrenees. This information could be useful not only for a better understanding of SOC patterns in mountain grasslands, but also for future modelling studies aiming to predict SOC, since geophysical variables are easier and less expensive to acquire and measure compared to biochemical variables (Manning et al., 2015).
Most studies on soil carbon usually pinpoint mean temperature and precipitation as the most important climate drivers of SOC (Hobley et al., 2015; Manning et al., 2015; Wiesmeier et al., 2019). Climate regulates large-scale patterns of aboveground net primary production (Chapin et al., 1987). In our study, temperature seasonality (TSIS) was a key driver of SOC, modulated by macrotopography, slope and grazing intensity (Table 2; Fig. 3). The highest variation of TSIS in our database, that is, the broadest temperature seasonality, occurred in cold environments, as compared to mild climates (Fig. S9). In mountain grasslands, cold climates imply a short phenological period of development for plants (Gómez, 2008). Hence, the positive effect of TSIS on SOC could be associated with a higher biomass accumulation in cold locations with more favourable temperatures during summer, this fact reducing geophysical stress for plants and broadening their growth period (Garcia-Pausas et al., 2007; Kikvidze et al., 2005). This increase in soil organic matter inputs during summer would overcome an eventual increase in soil organic matter decomposition caused by high temperatures (Sanderman et al., 2003).
The interactive effects of TSIS on SOC stocks with macrotopography and slope illustrate the capacity of landscape factors to modulate macroclimate effects on soil (Hook and Burke, 2000). Induced microclimate changes are often the explanation for the effects of topography in SOC (Lozano-García et al., 2016). In our case, SOC stocks increased with temperature seasonality, particularly in exposed locations, including south-facing hillsides and hillside tops (Fig. 3a; Table 2). In protected locations, including shady hillsides and valley bottoms, the hypothesized positive effect of increased TSIS values on plant productivity could be mitigated due to reduced solar radiation, long snow-covered periods and freezing episodes (Garcia-Pausas et al., 2007; López-Moreno et al., 2013). Additionally, differences in SOC between exposed and protected sites may also occur through other mechanisms, for instance the alteration of soil physicochemical properties (Zhang et al., 2018) or differences in vegetation (Sebastià, 2004). Since we used a hierarchy of controls approach (Manning et al., 2015), these indirect topographical effects on SOC stocks could be behind the exclusion in the linear models of some drivers selected in the BRT model, like silt or pH (Figs. 2 and 3). In addition, SOC stocks decreased with increase in slope, which may be attributed to reduced carbon inputs and increased carbon losses induced by steeper slopes (Yuan et al., 2019, and references therein). However, we found that increased temperature seasonality (TSIS) values partly compensated for negative slope effects on SOC.
The effect of temperature seasonality on SOC stocks was also modified by grazing management. At low TSIS values, SOC stocks increased under moderate to high grazing pressure; this effect disappeared as TSIS values increased (Fig. 3d). Recent meta-analyses concluded that intensive grazing commonly has decreasing effects on SOC (Abdalla et al., 2018; Eze et al., 2018; Mcsherry and Ritchie, 2013). However, these effects were strongly context-specific, depending on other factors, including climate and soil-type vegetation (Abdalla et al., 2018; Eze et al., 2018; Mcsherry and Ritchie, 2013). Moreover, moderate grazing intensities can increase SOC inputs by dung deposition and aboveground and root biomass production (Franzluebbers et al., 2000; Zeng et al., 2015). In our study, grazing intensity was relatively moderate (see methods); therefore, in our study increasing stocking rates may increase soil carbon inputs in moderate seasonality locations by enhancing aboveground and belowground productivity.
Soil texture also showed interactive effects on SOC stocks with climatic variables. In particular, clay effects on SOC stocks became negative as MAP values increased (Fig. 3c; Table 2). Both MAP and clay content are widely assumed to be positively correlated with SOC (Wiesmeier et al., 2019), but high soil water content caused by high MAP may inhibit decomposition if a shortage of oxygen supply occurs (Xu et al., 2016b). Furthermore, fine texture soils could be waterlogged frequently, leading to inhibition of root growth and soil C allocation belowground (Mcsherry and Ritchie, 2013).
4.3 Geophysical, biochemical and grazing management factors driving SOC stocks
Considering the difficulties of modelling SOC in a widely heterogeneous mountain environment (Garcia-Pausas et al., 2017), the geophysical model provided important information about SOC drivers in the Pyrenees. This information could be useful not only for a better understanding of SOC patterns in mountain grasslands, but also for future modelling studies aiming to predict SOC, since geophysical variables are easier and less expensive to acquire and measure compared to biochemical ones (Manning et al., 2015).
TSIS was a key driver of SOC with a varying effect depending on macrotopography, slope and grazing intensity (Table 2; Fig. 3). While most of the previous studies addressing soil carbon did not include any temperature seasonality variable as a potential SOC predictor, usually focusing on mean temperature and precipitation as the most important climate drivers of SOC (Hobley et al., 2015; Manning et al., 2015; Wiesmeier et al., 2019), our models suggest that TSIS and other temperature seasonality indexes should be included in further studies to provide more evidence of the extent of the effects of temperature seasonality on SOC stocks.
Climate regulates large-scale patterns of aboveground net primary production (Chapin et al., 1987). In the case of mountain grasslands, cold climates imply a short phenological period of development for plants (Gómez, 2008). Cold sites characterized by low mean temperatures presented a wider spectrum of TSIS values than warm sites, presenting both the lowest and highest TSIS values (Fig. S9). Hence, the positive effect of TSIS on SOC could be associated with a higher biomass accumulation in cold locations with more favourable temperatures during summer, this fact reducing geophysical stress for plants and broadening their growth period (Garcia-Pausas et al., 2007; Kikvidze et al., 2005). This rise in soil organic matter inputs during summer would overcome an eventual increase in soil organic matter decomposition rates due to high temperatures (Sanderman et al., 2003), which could even be diminished if microbial biomass decreases as a result of soil moisture reduction (Puissant et al., 2018).
The interactions of TSIS with macrotopography and slope illustrate the capacity of landscape factors to modulate macroclimate effects on soil (Hook and Burke, 2000). Induced microclimate changes are often the explanation for the effects of topography in SOC (Lozano-García et al., 2016). In our case, SOC stocks increased with temperature seasonality, particularly at mountain-exposed areas (Fig. 3a; Table 2). In protected sites, located on shady slopes and in valley bottoms, the hypothesized positive effect of high TSIS values on plant productivity could be mitigated due to lower solar radiation, longer snow-covered periods and freezing episodes (Garcia-Pausas et al., 2007; López-Moreno et al., 2013). Conversely, negative effects of low TSIS values on plant productivity could be compensated for thanks to the more humid conditions in protected sites compared to the exposed sites (Garcia-Pausas et al., 2007). Additionally, it is important to take into account that differences in SOC between exposed and protected sites may also occur through other mechanisms, for instance the alteration of soil physicochemical properties like pH, soil texture or stoniness (Zhang et al., 2018) or differences in vegetation (Sebastià, 2004). Since we used a hierarchy of controls approach (Manning et al., 2015), these topography indirect effects on SOC stocks could be behind the exclusion in the linear models of some drivers selected in the BRT model, like silt or pH (Figs. 2 and 3).
In addition, high TSIS values compensated for SOC stock decrease with a greater slope, which may be attributed to reduced carbon inputs and increased carbon losses induced by steeper slopes (Yuan et al., 2019, and references therein). Increases in grazing pressure elevated SOC stocks under low TSIS values (Fig. 3d). This was a surprising result according to recent meta-analyses, which concluded that grazing has commonly decreasing effects on SOC (Abdalla et al., 2018; Eze et al., 2018; Mcsherry and Ritchie, 2013). However, these effects were strongly context-specific, depending on other factors like climate and soil-type vegetation (Abdalla et al., 2018; Eze et al., 2018; Mcsherry and Ritchie, 2013). Moreover, light and medium grazing intensities can increase SOC inputs by dung deposition and promoting aboveground and root biomass production (Franzluebbers et al., 2000; Zeng et al., 2015). Considering that in our natural grasslands all grazing intensities are relatively low (see methods), our medium and high stock rates may increase soil carbon inputs in low-seasonality locations by enhancing aboveground and belowground productivity.
Interestingly, clay content and precipitation presented interacting effects on SOC (Fig. 3c; Table 2). Both MAP and clay content are widely assumed to be positively correlated with SOC (Wiesmeier et al., 2019). High MAP would increase SOC inputs by promoting plant productivity (Jobbágy and Jackson, 2000; Hobley et al., 2015). Clay positive effects are often attributed to a larger contact surface of soil particles (Kennedy et al., 2002), the absorption of negatively charged organic matter, high soil water retention and the exclusion of decomposer organisms due to their low pore size (Krull et al., 2001). In our study, high soil water contents caused by high MAP may inhibit decomposition if a shortage of oxygen supply occurs (Xu et al., 2016b). However, as MAP values increased, the clay effect on SOC became negative. To explain low SOC values at high MAP and high clay content, Mcsherry and Ritchie (2013) hypothesized that finer texture soils could be waterlogged more frequently, leading to inhibition of root growth and soil C allocation belowground.
The addition of soil nutrient and herbage variables to our geophysical model implied substitution of terms, including clay content and macrotopography, by newly added variables (Tables 2 and 3). This highlights the importance of indirect effects of these variables on SOC through other small-scale drivers (Leifeld et al., 2015; Xu et al., 2016b; Zhu et al., 2019). The combined model was complex and included infrequently tested effects involving interactions between grazer type, soil nutrients and herbage quality variables (Table 3, Fig. 4). Those results must be interpreted cautiously, because they are based on observational data but can contribute to generating testable hypotheses for later studies about some complex and untested relationships between SOC and its drivers. Interaction experiments concerning soil properties are expensive and rare in the literature (Rillig et al., 2019).
For this reason, SOC increased with the C∕N ratio (Fig. 4a), which may be explained by the difficulty of soil organic matter decomposition by soil microbes, decreasing decomposition rates of SOC with increasing soil C∕N (Wanyama et al., 2019; Xu et al., 2016b). A positive relationship between SOC and soil N was also expected, since most of the soil N is in combined form with organic matter (Cambardella and Elliott, 1994). However, in this case, due to the wide range of conditions and the randomized sampling design of the PASTUS Database, the raw correlation between soil N and SOC was somehow discrete (r=0.297; p value = 0.001; R2=0.088) in comparison to other studies (i.e. Yan et al., 2020). However, the novelty revealed by our model is that soil N could modulate the effects of certain SOC drivers, including livestock type and herbage NDF.
Cattle-grazed grasslands stored more SOC than mixed and sheep-grazed grasslands, but only under low soil N conditions (Fig. 4b). Chang et al. (2018) found that in a N poor semi-arid grassland, sheep decreased SOC content in comparison to cattle due to vegetation changes caused by their feeding preference for highly palatable forbs (Sebastià et al., 2008a), thus promoting less palatable grasses which supported less root biomass. In overall, under low soil N conditions, palatable plants are expected to contribute to SOC inputs through the stimulation of C allocation in forb roots (Ågren and Franklin, 2003; Warembourg et al., 2003) and the increase in the overall plant productivity due to legume atmospheric N fixation (Van Der Heijden et al., 2008).
However, these processes could decline under high soil N contents. For instance, legume atmospheric N fixation could be reduced since it requires additional energy in comparison to nitrogen acquisition from the soil (Ibañez et al., 2020; Minchin and Witty, 2005). Additionally, sheep selective feeding habits could shift plant leaf traits in the community towards nutrient-conservative leaf traits, which commonly induce fungal-based soil food webs with slow nutrient cycling and high SOC storage due to low decomposition rates (Orwin et al., 2010).
Additionally, grasslands with mixed grazed regimes stored even more SOC than sheep-grazed grasslands under high soil N conditions (Fig. 4b, Table 3). This result emphasizes that mixed livestock assemblages deserve particular attention, because mixed grazing can affect plant composition distinctly from single grazing species regimes and alter traveling and trampling behaviour of grazing animals (Aldezabal et al., 2019; Chang et al., 2018; Liu et al., 2015).
NDF was negatively related to SOC at high soil N values (Fig. 4c). NDF proportion represents the amount of structural compounds on litter and hence is inversely related to non-structural compound content (Goering and Van Soest, 1970). The latter are the main source of organic matter formation at the early stages of decomposition, and they are incorporated into microbial biomass in a highly efficient way (Cotrufo et al., 2013). However, if microbial necromass was recycled by microbes before its incorporation into mineral-associated organic matter (Córdova et al., 2018), it could be respired and mineralized instead of stored. Thus, our model suggests that incorporation of labile and fast metabolized non-organic compounds to soil organic matter could be a pathway of SOC allocation at high soil N in Pyrenean grasslands.
On the other hand, the ADL∕NH ratio was positively related to SOC in sheep and mixed grazed grasslands (Fig. 4d). The ADL∕NH ratio is a commonly used indicator for the resistance of litter to degradation, particularly at later stages of decomposition (Taylor et al., 1989). Hence, the increase in SOC stocks with ADL∕NH should be related to the physical pathway of soil organic matter incorporation, forming coarse particulate organic matter (Cotrufo et al., 2015). Moreover, our model suggests that this pathway would be inhibited under cattle grazing, presumably because of their higher digestive efficiency and thus less recalcitrant faeces (Wang et al., 2018) and their less selective diet compared to sheep, as the latter would avoid plants with a high lignin content, promoting recalcitrant litter (Rosenthal et al., 2012; Sebastià et al., 2008a).
Our results concerning interactions between grazer type and herbage quality provide some evidence of grazing effects not only through alterations of plant communities that were reported by previous studies in the region (Canals and Sebastià, 2000; Sebastià et al., 2008a), but also through interactions with them. Although grazing effects were not the most important factors affecting SOC stocks, this is by far the easiest component to manipulate in order to increase or maintain SOC in soils and face climate change (Komac et al., 2014). Considering our results, we suggest conducting more experiments to investigate grazer-type effects on SOC under different soil nutrient conditions and within plant communities with contrasting herbage quality parameters. Grazing management also has other advantages, such as preventing the accumulation of aboveground C and reducing the risk of forest fires (Nunes and Lourenço, 2017).
One key point of our results reinforces the idea that grazer type might be at least as important as grazing intensity in regulating grassland ecosystem dynamics (Tóth et al., 2018) and highlights the need for a more thorough research effort in disentangling not only grazing intensity, but also grazer-type effects on grassland soil organic carbon and nutrient cycling, under different environmental circumstances. The combined model provided some evidence that grazing may affect SOC not only through alterations of plant communities (Canals and Sebastià, 2000; Sebastià et al., 2008a), but also through interactions with them. Although grazing effects were not the most important factors affecting SOC stocks, this is by far the easiest component to manipulate in order to increase or maintain SOC in soils and face climate change (Komac et al., 2014). Despite the need for precise knowledge on the effects of different land uses on ecosystems for climate change mitigation (Lo et al., 2015), studies addressing grazer-type effects on SOC are scarce (i.e. Zhou et al., 2017; Chang et al., 2018). Considering our results, we suggest conducting more experiments which investigate grazer-type effects on SOC under different soil nutrient conditions and within plant communities with contrasting herbage quality parameters.
The models presented here show a series of novel broad-scale and local patterns concerning SOC stocks and their geophysical, biochemical and grazing management drivers. Factors driving SOC stocks often interacted in complex ways, within and between spatio-temporal scales. Temperature seasonality (TSIS) was the most critical geophysical factor, affecting SOC through interactions with topographical drivers and grazing intensity. This illustrates how not only climate mean annual conditions should be considered when modelling SOC drivers, but also seasonal patterns. Concerning biochemical factors, we found that the expected positive effect of soil N was modulated by livestock species and herbage NDF, and herbage recalcitrance effects on SOC varied depending on grazer type. Overall, we found a number of interactions highlighting the need to expand knowledge on grassland SOC drivers under different conditions, especially grazing. The latter is the most easily tractable factor affecting SOC. In conclusion, we provided valuable information for further studies dealing with SOC predictions at multiple broad scales and laid out the basis for generating new testable hypotheses for future studies, which may be useful for designing improved policies to palliate climate change.
Data are not public as the PASTUS Database is currently being used for other research projects. Please contact one of us by e-mail for queries concerning the data used in this study.
The supplement related to this article is available online at: https://doi.org/10.5194/bg-17-6033-2020-supplement.
AR designed the statistical procedure, carried out the statistical analyses and wrote the original draft. RMC was responsible for field monitoring, lab analyses and acquisition of information for the database implementation in the western Pyrenees (Navarra). She also reviewed the draft. EA designed the NIRS study and reviewed the draft. HD sampled and processed some of the data in the PASTUS Database and reviewed the draft. JGP processed some of the data in the PASTUS Database and reviewed the draft. JP carried out the chemical analyses of herbage samples for NIR calibration and validation equations and reviewed the draft. LSE designed the methodology and data collection and performed soil and vegetation sampling. She also reviewed the draft. ÀR sampled and processed some of the data in the PASTUS Database and reviewed the draft. JJJ collaborated in the fieldwork and reviewed the draft. MTS contributed to the conception, design and development of the PASTUS Database. In addition, she ensured funding and coordinated the projects whose data are included in PASTUS. Finally, she contributed to initial modelling, supervised the development of the paper, and read and reviewed the drafts.
The authors declare that they have no conflict of interest.
We would like to express our thanks to the many people who collaborated in fieldwork, sample processing and data analysis over time.
Research in this paper is based on the PASTUS Database, which was compiled from different funding sources over time, the most relevant being the EU Interreg III-A Programme (I3A-4-147-E) and the POCTEFA Programme/Interreg IV-A (FLUXPYR, EFA 34/08); the Spanish Science Foundation FECYT-MICINN (CARBOPAS: REN2002-04300-C02-01; CARBOAGROPAS: CGL2006-13555-C03-03 and CAPAS: CGL2010-22378-C03-01); and the Foundation Catalunya-La Pedrera and the Spanish Institute of Agronomical Research INIA (CARBOCLUS: SUM2006-00029-C02-0). Leticia San Emeterio was funded through a Talent Recruitment grant from Obra Social La Caixa – Fundación CAN. The ARAID Foundation provided support to Juan José Jiménez. This work was funded by the Spanish Science Foundation FECYT-MINECO (projects BIOGEI: GL2013-49142-C2-1-R and IMAGINE: CGL2017-85490-R) and the University of Lleida (PhD Fellowship to Antonio Rodríguez).
This paper was edited by Frank Hagedorn and reviewed by two anonymous referees.
Abdalla, M., Hastings, A., Chadwick, D. R., Jones, D. L., Evans, C. D., Jones, M. B., Rees, R. M., and Smith, P.: Critical review of the impacts of grazing intensity on soil organic carbon storage and other soil quality indicators in extensively managed grasslands, Agr. Ecosyst. Environ., 253, 62–81, https://doi.org/10.1016/j.agee.2017.10.023, 2018.
Aerts, R. and Chapin, F. S.: The Mineral Nutrition of Wild Plants Revisited: A Re-evaluation of Processes and Patterns, Adv. Ecol. Res., 30, 1–67, https://doi.org/10.1016/S0065-2504(08)60016-1, 1999.
Ågren, G. I. and Franklin, O.: Root : shoot ratios, optimization and nitrogen productivity, Ann. Bot., 92, 795–800, https://doi.org/10.1093/aob/mcg203, 2003.
Aldezabal, A., Pérez-López, U., Laskurain, N. A., and Odriozola, I.: El abandono del pastoreo afecta negativamente a la calidad del pasto en pastizales atlánticos ibéricos?; Grazing abandonment negatively affects forage quality in iberian atlantic grasslands, Rev. Ecol. Montaña, 174, 42, https://doi.org/10.3989/pirineos.2019.174002, 2019.
Bardgett, R. D. and Wardle, D. A.: Herbibore-mediated Linkages Between Abobeground and Belowground Communities, Ecology, 84, 2258–2268, https://doi.org/10.1890/02-0274, 2003.
Barton, K.: MuMIn: Multi-model inference, R package version 1.9.13, Version, 1.18, available at: https://cran.r-project.org/web/packages/MuMIn/ (last access: 20 May 2019), 2015.
Berninger, F., Susiluoto, S., Gianelle, D., Bahn, M., Wohlfahrt, G., Sutton, M., Garcia-Pausas, J., Gimeno, C., Sanz, M. J., Dore, S., Rogiers, N., Furger, M., Eugster, W., Balzarolo, M., Sebastià, M. T., Tenhunen, J., Staszewski, T., and Cernusca, A.: Management and site effects on carbon balances of european mountain meadows and rangelands, Boreal Environ. Res., 20, 748–760, 2015.
Bing, H., Wu, Y., Zhou, J., Sun, H., Luo, J., Wang, J., and Yu, D.: Stoichiometric variation of carbon, nitrogen, and phosphorus in soils and its implication for nutrient limitation in alpine ecosystem of Eastern Tibetan Plateau, J. Soils Sediments, 16, 405–416, https://doi.org/10.1007/s11368-015-1200-9, 2016.
Bouyoucos, G. J.: Directions for making mechanical analysis of soils by the hydrometer method, Soil Sci., 42, 225–2230, 1936.
Burnham, K. P. and Anderson, D. R.: Model Selection and Multimodel Inference: A Practical Information-Theoretic Approach, 2nd Edn., Springer, New York, 2002.
Calcagno, V.: Package glmulti 1.0.7, Model selection and multimodel inference made easy, Community Ecol. Packag., 1–20, available at: https://cran.rproject.org/web/packages/glmulti/index.html (last access: 2 May 2019), 2015.
Cambardella, C. A. and Elliott, E. T.: Carbon and Nitrogen Dynamics of Soil Organic Matter Fractions from Cultivated Grassland Soils, Soil Sci. Soc. Am. J., 58, 123–130, https://doi.org/10.2136/sssaj1994.03615995005800010017x, 1994.
Canals, R. M. and Sebastià, M. T.: Analyzing mechanisms regulating diversity in rangelands through comparative studies: A case in the southwestern Pyrennees, Biodivers. Conserv., 9, 965–984, https://doi.org/10.1023/A:1008967903169, 2000.
Carreras, J. and Diego, F. C.: Cartografia dels hàbitats de Catalunya 1 : 50 000, Generalitat de Catalunya, Barcelona, 2006.
Chang, Q., Wang, L., Ding, S., Xu, T., Li, Z., Song, X., Zhao, X., Wang, D., and Pan, D.: Grazer effects on soil carbon storage vary by herbivore assemblage in a semi-arid grassland, J. Appl. Ecol., 55, 2517–2526, https://doi.org/10.1111/1365-2664.13166, 2018.
Chang, R., Wang, G., Fei, R., Yang, Y., Luo, J., and Fan, J.: Altitudinal Change in Distribution of Soil Carbon and Nitrogen in Tibetan Montane Forests, Soil Sci. Soc. Am. J., 79, 1455–1469, https://doi.org/10.2136/sssaj2015.02.0055, 2015.
Chapin, F. S., Bloom, A. J., Field, C. B., and Waring, R. H.: Plant Responses to Multiple Environmental Factors, Bioscience, 37, 49–57, https://doi.org/10.2307/1310177, 1987.
Cleveland, C. C. and Liptzin, D.: C : N : P stoichiometry in soil: is there a “Redfield ratio” for the microbial biomass?, Biogeochemistry, 85, 235–252, https://doi.org/10.1007/s10533-007-9132-0, 2007.
Córdova, S. C., Olk, D. C., Dietzel, R. N., Mueller, K. E., Archontouilis, S. V., and Castellano, M. J.: Plant litter quality affects the accumulation rate, composition, and stability of mineral-associated soil organic matter, Soil Biol. Biochem., 125, 115–124, https://doi.org/10.1016/J.SOILBIO.2018.07.010, 2018.
Cotrufo, M. F., Wallenstein, M. D., Boot, C. M., Denef, K., and Paul, E.: The Microbial Efficiency-Matrix Stabilization (MEMS) framework integrates plant litter decomposition with soil organic matter stabilization: do labile plant inputs form stable soil organic matter?, Glob. Change Biol., 19, 988–995, https://doi.org/10.1111/gcb.12113, 2013.
Cotrufo, M. F. F., Soong, J. L. J. L., Horton, A. J. A. J., Campbell, E. E., Haddix, M. L. M. L. L., Wall, D. H. D. H., and Parton, W. J. W. J.: Formation of soil organic matter via biochemical and physical pathways of litter mass loss, Nat. Geosci., 8, 776–779, https://doi.org/10.1038/ngeo2520, 2015.
David, D. J.: The determination of exchangeable sodium, potassium, calcium and magnesium in soils by atomic-absorption spectrophotometry, Analyst, 85, 495–503, https://doi.org/10.1039/AN9608500495, 1960.
de Lamo, X. and Sebastià, M. T.: Role of biogeographical, ecological and management factors on plant diversity in grasslands, in: Sociedad Española para el Estudio de los Pastos (SEEP), Vol. 11, edited by: Lloveras, J., González-Rodríguez, A., Vázquez-Yañez, O., Piñeiro, J., Santamaría, O., Olea, L., and Poblaciones, M. J., 832–834, Sociedad Española para el Estudio de los Pastos (SEEP), Madrid, Spain, 2006.
Deng, X., Zhan, Y., Wang, F., Ma, W., Ren, Z., Chen, X., Qin, F., Long, W., Zhu, Z., and Lv, X.: Soil organic carbon of an intensively reclaimed region in China: Current status and carbon sequestration potential, Sci. Total Environ., 565, 539–546, https://doi.org/10.1016/j.scitotenv.2016.05.042, 2016.
de Vries, F. T., Manning, P., Tallowin, J. R. B., Mortimer, S. R., Pilgrim, E. S., Harrison, K. A., Hobbs, P. J., Quirk, H., Shipley, B., Cornelissen, J. H. C., Kattge, J., and Bardgett, R. D.: Abiotic drivers and plant traits explain landscape-scale patterns in soil microbial communities, Ecol. Lett., 15, 1230–1239, https://doi.org/10.1111/j.1461-0248.2012.01844.x, 2012.
Díaz, S., Lavorel, S., De Bello, F., Quétier, F., Grigulis, K., and Robson, T. M.: Incorporating plant functional diversity effects in ecosystem service assessments, P. Natl. Acad. Sci. USA, 104, 20684–20689, https://doi.org/10.1073/pnas.0704716104, 2007.
Duarte-guardia, S., Peri, P. L., Amelung, W., Sheil, D., Laffan, S. W., Borchard, N., Bird, M. I., and Peri, P. L.: Better estimates of soil carbon from geographical data?: a revised global approach, Mitig. Adapt. Strat. Gl., 24, 355–372, 2019.
Ellert, B. H. and Bettany, J. R.: Calculation of organic matter and nutrients stored in soils under contrasting management regimes, Can. J. Soil Sci., 75, 529–538, https://doi.org/10.4141/cjss95-075, 1995.
Elith, J., Leathwick, J. R., and Hastie, T.: A working guide to boosted regression trees, J. Anim. Ecol., 77, 802–813, https://doi.org/10.1111/j.1365-2656.2008.01390.x, 2008.
Eze, S., Palmer, S. M., and Chapman, P. J.: Soil organic carbon stock in grasslands: Effects of inorganic fertilizers, liming and grazing in different climate settings, J. Environ. Manage., 223, 74–84, https://doi.org/10.1016/j.jenvman.2018.06.013, 2018.
Fernández-Alonso, M. J., Díaz-Pinés, E., Ortiz, C., and Rubio, A.: Disentangling the effects of tree species and microclimate on heterotrophic and autotrophic soil respiration in a Mediterranean ecotone forest, Forest Ecol. Manage., 430, 533–544, https://doi.org/10.1016/j.foreco.2018.08.046, 2018.
Fernández-Martínez, M., Vicca, S., Janssens, I. A., Sardans, J., Luyssaert, S., Campioli, M., Chapin, F. S., Ciais, P., Malhi, Y., Obersteiner, M., Papale, D., Piao, S. L., Reichstein, M., Rodà, F., and Peñuelas, J.: Nutrient availability as the key regulator of global forest carbon balance, Nat. Clim. Chang., 4, 471–476, https://doi.org/10.1038/nclimate2177, 2014.
Fick, S. E. and Hijmans, R. J.: WorldClim 2: new 1-km spatial resolution climate surfaces for global land areas, Int. J. Climatol., 3, 4302–4315, https://doi.org/10.1002/joc.5086, 2017.
Fox, J., Weisberg, S., Price, B., Adler, D., Bates, D., and Baud-Bovy, G.: Package “car,” R Doc., 146, https://doi.org/10.1093/nar/gkq363, 2018.
Franzluebbers, A. J., Stuedemann, J. A., Schomberg, H. H., and Wilkinson, S. R.: Soil organic C and N pools under long-term pasture management in the Southern Piedmont USA, Soil Biol. Biochem., 32, 469–478, https://doi.org/10.1016/S0038-0717(99)00176-5, 2000.
Garcia-Pausas, J., Casals, P., Camarero, L., Huguet, C., Sebastià, M. T., Thompson, R., and Romanyà, J.: Soil organic carbon storage in mountain grasslands of the Pyrenees: Effects of climate and topography, Biogeochemistry, 82, 279–289, https://doi.org/10.1007/s10533-007-9071-9, 2007.
Garcia-Pausas, J., Romanyà, J., Montané, F., Rios, A. I., Taull, M., Rovira, P., and Casals, P.: Are Soil Carbon Stocks in Mountain Grasslands Compromised by Land-Use Changes?, in: High Mountain Conservation in a Changing World, edited by: Catalan, J., Ninot, J. M., and Aniz, M. M., 207–230, Springer International Publishing, Cham., 2017.
Gobierno de Navarra: Mapa de usos del suelo de Navarra 1 : 25 000, Gobierno de Navarra, Pamplona, Spain, 2003.
Goering, H. K. and Van Soest, P. J.: Forage fiber analyses (apparatus, reagents, prcedures, and some applications), USDA Agr Handb, available at: http://agris.fao.org/agris-search/search.do?recordID=US201301156229 (last access: 18 April 2019), 1970.
Gómez, D.: Aspectos ecológicos de los pastos, in: Pastos del Pirineo, edited by: Fillat, F., García-González, R., Gómez, D., and Reiné, R., 61–73, Consejo Superior de Investigaciones Científicas, CSIC, Madrid, Spain, 2008.
Grace, J. B.: Structural Equation Modeling and Natural Systems, Cambridge University Press, Cambridge, 2006.
Grace, J. B. and Bollen, K. A.: Interpreting the Results from Multiple Regression and Structural Equation Models, Bull. Ecol. Soc. Am., 86, 283–295, https://doi.org/10.1890/0012-9623(2005)86[283:itrfmr]2.0.co;2, 2005.
Gray, J. M., Bishop, T. F. A., and Wilson, B. R.: Factors Controlling Soil Organic Carbon Stocks with Depth in Eastern Australia, Soil Sci. Soc. Am. J., 79, 1741, https://doi.org/10.2136/sssaj2015.06.0224, 2015.
Greenwell, B., Boehmke, B., and Cunningham, J.: Package “gbm”, available at: https://cran.r-project.org/web/packages/gbm/index.html, last access: 15 February 2019.
Grömping, U.: R package relaimpo: relative importance for linear regression, J. Stat. Softw., 17, 139–147, https://doi.org/10.1016/j.foreco.2006.08.245, 2006.
Grueber, C. E., Nakagawa, S., Laws, R. J., and Jamieson, I. G.: Multimodel inference in ecology and evolution: Challenges and solutions, J. Evol. Biol., 24, 699–711, https://doi.org/10.1111/j.1420-9101.2010.02210.x, 2011.
Haden, A. C., Yang, W. H., and DeLucia, E. H.: Soils' dirty little secret: Depth‐based comparisons can be inadequate for quantifying changes in soil organic carbon and other mineral soil properties, Glob. Change Biol., 26, 3759–3770, https://doi.org/10.1111/gcb.15124, 2020.
Hijmans, R. J., Phillips, S., Leathwick, J., and Maintainer, J. E.: Package “dismo” Type Package, Species Distribution Modeling, available at: https://cran.r-project.org/web/packages/dismo/dismo.pdf (lsat access: 15 February 2019), 2017.
Hobley, E., Wilson, B., Wilkie, A., Gray, J., and Koen, T.: Drivers of soil organic carbon storage and vertical distribution in Eastern Australia, Plant Soil, 390, 111–127, https://doi.org/10.1007/s11104-015-2380-1, 2015.
Hook, P. B. and Burke, I. C.: Biogeochemistry in a shortgrass landscape: control by topography, soil texture, and microclimate, Ecology, 81, 2686–2703, https://doi.org/10.1890/0012-9658(2000)081[2686:BIASLC]2.0.CO;2, 2000.
Ibañez, M., Altimir, N., Ribas, A., Eugster, W., and Sebastià, M. T.: Phenology and plant functional type dominance drive CO2 exchange in seminatural grasslands in the Pyrenees, J. Agr. Sci., 158, 3–14, https://doi.org/10.1017/S0021859620000179, 2020.
Jenny, H.: Factors of soil formation: A System of Quantitative Pedology, Dover Publications, New York, USA, 1941.
Jobbágy, E. G. and Jackson, R. B.: The Vertical Distribution of Soil Organic Carbon and Its Relation to Climate and Vegetation, Source Ecol. Appl. Ecol. Appl., 10, 423–436, https://doi.org/10.1890/1051-0761(2000)010[0423:TVDOSO]2.0.CO;2, 2000.
Kennedy, M. J., Pevear, D. R., and Hill, R. J.: Mineral surface control of organic carbon in black shale, Science, 295, 657–60, https://doi.org/10.1126/science.1066611, 2002.
Kikvidze, Z., Pugnaire, F. I., Brooker, R. W., Choler, P., Lortie, C. J., Michalet, R., and Callaway, R. M.: Linking patterns and processes in alpine plant communities: A global study, Ecology, 86, 1395–1400, https://doi.org/10.1890/04-1926, 2005.
Komac, B., Domènech, M., and Fanlo, R.: Effects of grazing on plant species diversity and pasture quality in subalpine grasslands in the eastern Pyrenees (Andorra): Implications for conservation, J. Nat. Conserv., 22, 247–255, https://doi.org/10.1016/J.JNC.2014.01.005, 2014.
Krull, E., Baldock, J., and Skjemstad, J.: Soil Texture Effects on Decomposition and Soil Carbon Storage, in: Net Ecosystem Exchange Workshop, 103–110, available at: http://citeseerx.ist.psu.edu/viewdoc/summary?doi=10.1.1.121.3042 (last access: 13 May 2019), 2001.
Lal, R.: Soil Carbon Sequestration Impacts on Global Climate Change and Food Secruity, Science, 304, 1623–1627, 2004a.
Lal, R.: Soil carbon sequestration to mitigate climate change, Geoderma, 123, 1–22, https://doi.org/10.1016/J.GEODERMA.2004.01.032, 2004b.
Legendre, P. and Legendre, L.: Numerical Ecology, 2nd Edn., Elsevier, Amsterdam, 1998.
Leifeld, J., Meyer, S., Budge, K., Sebastia, M. T., Zimmermann, M., and Fuhrer, J.: Turnover of grassland roots in mountain ecosystems revealed by their radiocarbon signature: Role of temperature and management, PLoS One, 10, 1–13, https://doi.org/10.1371/journal.pone.0119184, 2015.
Lenth, R., Singmann, H., Love, J., Buerkne, P., and Herve, M.: Package “emmeans”, available at: https://cran.r-project.org/web/packages/emmeans/, last access: 18 March 2019.
Liu, J., Feng, C., Wang, D., Wang, L., Wilsey, B. J., and Zhong, Z.: Impacts of grazing by different large herbivores in grassland depend on plant species diversity, J. Appl. Ecol., 52, 1053–1062, https://doi.org/10.1111/1365-2664.12456, 2015.
Lo, Y. H., Blanco, J. A., Canals, R. M., González de Andrés, E., San Emeterio, L., Imbert, J. B., and Castillo, F. J.: Land use change effects on carbon and nitrogen stocks in the Pyrenees during the last 150 years: A modeling approach, Ecol. Modell., 312, 322–334, https://doi.org/10.1016/j.ecolmodel.2015.06.005, 2015.
López-Moreno, J. I., Revuelto, J., Gilaberte, M., Morán-Tejeda, E., Pons, M., Jover, E., Esteban, P., García, C., and Pomeroy, J. W.: The effect of slope aspect on the response of snowpack to climate warming in the Pyrenees, Theor. Appl. Climatol., 117, 207–219, https://doi.org/10.1007/s00704-013-0991-0, 2013.
Lozano-García, B., Parras-Alcántara, L., and Brevik, E. C.: Impact of topographic aspect and vegetation (native and reforested areas) on soil organic carbon and nitrogen budgets in Mediterranean natural areas, Sci. Total Environ., 544, 963–970, https://doi.org/10.1016/J.SCITOTENV.2015.12.022, 2016.
Lu, X., Yan, Y., Sun, J., Zhang, X., Chen, Y., Wang, X., and Cheng, G.: Carbon, nitrogen, and phosphorus storage in alpine grassland ecosystems of Tibet: effects of grazing exclusion, Ecol. Evol., 5, 4492–504, https://doi.org/10.1002/ece3.1732, 2015.
Lu, X., Kelsey, K. C., Yan, Y., Sun, J., Wang, X., Cheng, G., and Neff, J. C.: Effects of grazing on ecosystem structure and function of alpine grasslands in Qinghai-Tibetan Plateau: a synthesis, Ecosphere, 8, e01656, https://doi.org/10.1002/ecs2.1656, 2017.
Manning, P., de Vries, F. T., Tallowin, J. R. B., Smith, R., Mortimer, S. R., Pilgrim, E. S., Harrison, K. A., Wright, D. G., Quirk, H., Benson, J., Shipley, B., Cornelissen, J. H. C., Kattge, J., Bönisch, G., Wirth, C., and Bardgett, R. D.: Simple measures of climate, soil properties and plant traits predict national-scale grassland soil carbon stocks, J. Appl. Ecol., 52, 1188–1196, https://doi.org/10.1111/1365-2664.12478, 2015.
Mcsherry, M. E. and Ritchie, M. E.: Effects of grazing on grassland soil carbon: A global review, Glob. Change Biol., 19, 1347–1357, https://doi.org/10.1111/gcb.12144, 2013.
Minchin, F. R. and Witty, J. F.: Respiratory/carbon costs of symbiotic nitrogen fixation in legumes, in: Plant respiration, 195–205, Springer, Dordrecht, the Netherlands, 2005.
Nunes, A. N. and Lourenço, L.: Increased vulnerability to wildfires and post fire hydro-geomorphic processes in Portuguese mountain regions: What has changed?, Open Agric., 2, 70–82, https://doi.org/10.1515/opag-2017-0008, 2017.
Olsen, S. R.: Estimation of available phosphorus in soils by extraction with sodium bicarbonate, US Dept. of Agriculture, Washington D.C., USA, 1954.
Orwin, K. H., Buckland, S. M., Johnson, D., Turner, B. L., Smart, S., Oakley, S., and Bardgett, R. D.: Linkages of plant traits to soil properties and the functioning of temperate grassland, J. Ecol., 98, 1074–1083, https://doi.org/10.1111/j.1365-2745.2010.01679.x, 2010.
Ottoy, S., Van Meerbeek, K., Sindayihebura, A., Hermy, M., and Van Orshoven, J.: Assessing top- and subsoil organic carbon stocks of Low-Input High-Diversity systems using soil and vegetation characteristics, Sci. Total Environ., 589, 153–164, https://doi.org/10.1016/J.SCITOTENV.2017.02.116, 2017.
Penin, D.: Cartographie des habitats naturels (typologie Corine Biotope) du massif Madres-Coronat, 1st Edn., AGRNN/CPIE du Conflent, AGRNN/CPIE du Conflent Paris, France, 1997.
Peri, P. L., Rosas, Y. M., Ladd, B., Toledo, S., Lasagno, R. G., and Pastur, G. M.: Modelling soil carbon content in South Patagonia and evaluating changes according to climate, vegetation, desertification and grazing, Sustainability, 10, 438, https://doi.org/10.3390/su10020438, 2018.
Puissant, J., Jassey, V. E. J., Mills, R. T. E., Robroek, B. J. M., Gavazov, K., De Danieli, S., Spiegelberger, T., Griffiths, R., Buttler, A., Brun, J.-J. J., and Cécillon, L.: Seasonality alters drivers of soil enzyme activity in subalpine grassland soil undergoing climate change, Soil Biol. Biochem., 124, 266–274, https://doi.org/10.1016/j.soilbio.2018.06.023, 2018.
R Core Team: R: A Language and Environment for Statistical Computing, available at: https://www.r-project.org/, last access: 15 February 2016.
Rillig, M. C., Ryo, M., Lehmann, A., Aguilar-Trigueros, C. A., Buchert, S., Wulf, A., Iwasaki, A., Roy, J., and Yang, G.: The role of multiple global change factors in driving soil functions and microbial biodiversity, Science, 366, 886–890, https://doi.org/10.1126/science.aay2832, 2019.
Rodríguez, A., de Lamo, X., Sebastiá, M.-T., and Sebastià, M.-T.: Interactions between global change components drive plant species richness patterns within communities in mountain grasslands independetly of topography, J. Veg. Sci., 29, 1029–1039, https://doi.org/10.1111/jvs.12683, 2018.
Rosenthal, G., Schrautzer, J., and Eichberg, C.: Low-intensity grazing with domestic herbivores: A tool for maintaining and restoring plant diversity in temperate Europe, Tuexenia, 32, 167–205, 2012.
Sanderman, J., Amundson, R. G., and Baldocchi, D. D.: Application of eddy covariance measurements to the temperature dependence of soil organic matter mean residence time, Global Biogeochem. Cy., 17, 1061, https://doi.org/10.1029/2001GB001833, 2003.
Schlesinger, W. H.: Carbon Balance in Terrestrial Detritus, Annu. Rev. Ecol. Syst., 8, 51–81, https://doi.org/10.1146/annurev.es.08.110177.000411, 1977.
Schöning, I., Grüneberg, E., Sierra, C. A., Hessenmöller, D., Schrumpf, M., Weisser, W. W., and Schulze, E. D.: Causes of variation in mineral soil C content and turnover in differently managed beech dominated forests, Plant Soil, 370, 625–639, https://doi.org/10.1007/s11104-013-1654-8, 2013.
Sebastià, M.-T.: Role of topography and soils in grassland structuring at the landscape and community scales, Basic Appl. Ecol., 5, 331–346, https://doi.org/10.1016/j.baae.2003.10.001, 2004.
Sebastià, M.-T., Kirwan, L., and Connolly, J.: Strong shifts in plant diversity and vegetation composition in grassland shortly after climatic change, J. Veg. Sci., 19, 299–306, https://doi.org/10.3170/2008-8-18356, 2008a.
Sebastià, M.-T., de Bello, F., Puig, L., and Taull, M.: Grazing as a factor structuring grasslands in the Pyrenees, Appl. Veg. Sci., 11, 215–222, https://doi.org/10.3170/2008-7-18358, 2008b.
Shipley, B. and Parent, M.: Germination Responses of 64 Wetland Species in Relation to Seed Size, Minimum Time to Reproduction and Seedling Relative Growth Rate, Funct. Ecol., 5, 111, https://doi.org/10.2307/2389561, 1991.
Simard, R. R.: Ammonium acetate-extractable elements, in Soil sampling and methods of analysis, Vol. 1, 39–42, Lewis Publisher FL, USA, 1993.
Solly, E. F., Schöning, I., Boch, S., Kandeler, E., Marhan, S., Michalzik, B., Müller, J., Zscheischler, J., Trumbore, S. E., and Schrumpf, M.: Factors controlling decomposition rates of fine root litter in temperate forests and grasslands, Plant Soil, 382, 203–218, https://doi.org/10.1007/s11104-014-2151-4, 2014.
Stockmann, U., Adams, M. A., Crawford, J. W., Field, D. J., Henakaarchchi, N., Jenkins, M., Minasny, B., McBratney, A. B., Courcelles, V. de R. de, Singh, K., Wheeler, I., Abbott, L., Angers, D. A., Baldock, J., Bird, M., Brookes, P. C., Chenu, C., Jastrow, J. D., Lal, R., Lehmann, J., O'Donnell, A. G., Parton, W. J., Whitehead, D., and Zimmermann, M.: The knowns, known unknowns and unknowns of sequestration of soil organic carbon, Agr. Ecosyst. Environ., 164, 80–99, https://doi.org/10.1016/j.agee.2012.10.001, 2013.
Symonds, M. R. E. and Moussalli, A.: A brief guide to model selection, multimodel inference and model averaging in behavioural ecology using Akaike's information criterion, Behav. Ecol. Sociobiol., 65, 13–21, https://doi.org/10.1007/s00265-010-1037-6, 2011.
Taylor, B. R., Parkinson, D., and Parsons, W. F. J.: Nitrogen and Lignin Content as Predictors of Litter Decay Rates: A Microcosm Test, Ecology, 70, 97–104, https://doi.org/10.2307/1938416, 1989.
Tipping, E., Somerville, C. J., and Luster, J.: The C : N : P : S stoichiometry of soil organic matter, Biogeochemistry, 130, 117–131, https://doi.org/10.1007/s10533-016-0247-z, 2016.
Tóth, E., Deák, B., Valkó, O., Kelemen, A., Miglécz, T., Tóthmérész, B., and Török, P.: Livestock Type is More Crucial Than Grazing Intensity: Traditional Cattle and Sheep Grazing in Short-Grass Steppes, Land Degrad. Dev., 29, 231–239, https://doi.org/10.1002/ldr.2514, 2018.
Van Der Heijden, M. G. A., Bardgett, R. D., and Van Straalen, N. M.: The unseen majority: Soil microbes as drivers of plant diversity and productivity in terrestrial ecosystems, Ecol. Lett., 11, 296–310, https://doi.org/10.1111/j.1461-0248.2007.01139.x, 2008.
Vitousek, P. M. and Howarth, R. W.: Nitrogen Limitation on Land and in the Sea: How Can It Occur?, 1991.
Von Haden, A. C. and Dornbush, M. E.: Patterns of root decomposition in response to soil moisture best explain high soil organic carbon heterogeneity within a mesic, restored prairie, Agr. Ecosyst. Environ., 185, 188–196, https://doi.org/10.1016/j.agee.2013.12.027, 2014.
Van Soest, P. J., Robertson, J. B., and Lewis, B. A.: Methods for Dietary Fiber, Neutral Detergent Fiber, and Nonstarch Polysaccharides in Relation to Animal Nutrition, J Dairy Sci., 74, 3583–3597, https://doi.org/10.3168/jds.S0022-0302(91)78551-2, 1991.
Wang, J., Wang, D., Li, C., Seastedt, T. R., Liang, C., Wang, L., Sun, W., Liang, M., and Li, Y.: Feces nitrogen release induced by different large herbivores in a dry grassland, Ecol. Appl., 28, 201–211, https://doi.org/10.1002/eap.1640, 2018.
Wanyama, I., Pelster, D. E., Butterbach-Bahl, K., Verchot, L. V, Martius, C., and Rufino, M. C.: Soil carbon dioxide and methane fluxes from forests and other land use types in an African tropical montane region, Biogeochemistry, 143, 171–190, https://doi.org/10.1007/s10533-019-00555-8, 2019.
Warembourg, F. R., Roumet, C., and Lafont, F.: Differences in rhizosphere carbon-partitioning among plant species of different families, Plant Soil, 256, 347–357, https://doi.org/10.1023/A:1026147622800, 2003.
White, R., Murray, S., and Rohweder, M.: Pilot Analysis of Global Ecosystems: Grassland Ecosystems, 2000.
Wiesmeier, M., Urbanski, L., Hobley, E., Lang, B., von Lützow, M., Marin-Spiotta, E., van Wesemael, B., Rabot, E., Ließ, M., Garcia-Franco, N., Wollschläger, U., Vogel, H. J., and Kögel-Knabner, I.: Soil organic carbon storage as a key function of soils – A review of drivers and indicators at various scales, Geoderma, 333, 149–162, https://doi.org/10.1016/j.geoderma.2018.07.026, 2019.
Williams, P. C. and Sobering, D. C.: How do we do it: a brief summary of the methods we use in developing near infrared calibrations, in Near infrared spectroscopy: The future waves, edited by: Williams, P. C., Davies, A. M. C., and Williams, P., 185–188, NIR Publications, Chichester, UK, 1996.
Williams, T. E., Chang, C. M., Rosen, E. L., Garcia, G., Runnerstrom, E. L., Williams, B. L., Koo, B., Buonsanti, R., Milliron, D. J., and Helms, B. A.: NIR-Selective electrochromic heteromaterial frameworks: A platform to understand mesoscale transport phenomena in solid-state electrochemical devices, J. Mater. Chem. C, 2, 3328–3335, https://doi.org/10.1039/c3tc32247e, 2014.
Xu, X., Shi, Z., Li, D., Rey, A., Ruan, H., Craine, J. M., Liang, J., Zhou, J., and Luo, Y.: Soil properties control decomposition of soil organic carbon: Results from data-assimilation analysis, Geoderma, 262, 235–242, https://doi.org/10.1016/j.geoderma.2015.08.038, 2016a.
Xu, X., Shi, Z., Li, D., Rey, A., Ruan, H., Craine, J. M., Liang, J., Zhou, J., and Luo, Y.: Soil properties control decomposition of soil organic carbon: Results from data-assimilation analysis, Geoderma, 262, 235–242, https://doi.org/10.1016/J.GEODERMA.2015.08.038, 2016b.
Yan, J., Wang, L., Hu, Y., Tsang, Y. F., Zhang, Y., Wu, J., Fu, X., and Sun, Y.: Plant litter composition selects different soil microbial structures and in turn drives different litter decomposition pattern and soil carbon sequestration capability, Geoderma, 319, 194–203, https://doi.org/10.1016/J.GEODERMA.2018.01.009, 2018.
Yan, L., Li, Y., Wang, L., Zhang, X., Wang, J., Wu, H., Yan, Z., Zhang, K., and Kang, X.: Grazing significantly increases root shoot ratio but decreases soil organic carbon in Qinghai-Tibetan Plateau grasslands: A hierarchical meta-analysis, Land Degrad. Dev., 31, 2369–2378, https://doi.org/10.1002/ldr.3606, 2020.
Yan, Y., Tian, L., Du, Z., Chang, S. X., and Cai, Y.: Carbon, nitrogen and phosphorus stocks differ among vegetation patch types in a degraded alpine steppe, J. Soils Sediments, 19, 1809–1819, https://doi.org/10.1007/s11368-018-2191-0, 2019.
Yang, Y., Chen, Y., Li, Z., and Chen, Y.: Land-use/cover conversion affects soil organic-carbon stocks: A case study along the main channel of the Tarim River, China, PLoS One, 13, 1–14, https://doi.org/10.1371/journal.pone.0206903, 2018.
Yang, Y. H., Fang, J. Y., Pan, Y. D., and Ji, C. J.: Aboveground biomass in Tibetan grasslands, J. Arid Environ., 73, 91–95, https://doi.org/10.1016/J.JARIDENV.2008.09.027, 2009.
Yuan, Z.-Q., Fang, C., Zhang, R., Li, F.-M., Javaid, M. M., and Janssens, I. A.: Topographic influences on soil properties and aboveground biomass in lucerne-rich vegetation in a semi-arid environment, Geoderma, 344, 137–143, https://doi.org/10.1016/J.GEODERMA.2019.03.003, 2019.
Zeng, C., Wu, J., and Zhang, X.: Effects of grazing on above-vs. below-ground biomass allocation of alpine grasslands on the northern tibetan plateau, PLoS One, 10, 1–15, https://doi.org/10.1371/journal.pone.0135173, 2015.
Zhang, X., Liu, M., Zhao, X., Li, Y., Zhao, W., Li, A., Chen, S., Chen, S., Han, X., and Huang, J.: Topography and grazing effects on storage of soil organic carbon and nitrogen in the northern China grasslands, Ecol. Indic., 93, 45–53, https://doi.org/10.1016/J.ECOLIND.2018.04.068, 2018.
Zhao, Y., Ding, Y., Hou, X., Li, F. Y., Han, W., and Yun, X.: Effects of temperature and grazing on soil organic carbon storage in grasslands along the Eurasian steppe eastern transect, PLoS One, 12, 1–16, https://doi.org/10.1371/journal.pone.0186980, 2017.
Zhou, G., Zhou, X., He, Y., Shao, J., Hu, Z., Liu, R., Zhou, H., and Hosseinibai, S.: Grazing intensity significantly affects belowground carbon and nitrogen cycling in grassland ecosystems: a meta-analysis, Glob. Change Biol., 23, 1167–1179, https://doi.org/10.1111/gcb.13431, 2017.
Zhu, M., Feng, Q., Qin, Y., Cao, J., Zhang, M., Liu, W., Deo, R. C., Zhang, C., Li, R., and Li, B.: The role of topography in shaping the spatial patterns of soil organic carbon, Catena, 176, 296–305, https://doi.org/10.1016/J.CATENA.2019.01.029, 2019.