the Creative Commons Attribution 4.0 License.
the Creative Commons Attribution 4.0 License.
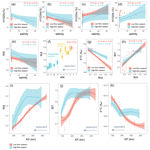
Environmental controls on the distribution of brGDGTs and brGMGTs across the Seine River basin (NW France): implications for bacterial tetraethers as a proxy for riverine runoff
Zhe-Xuan Zhang
Edith Parlanti
Christelle Anquetil
Jérôme Morelle
Anniet M. Laverman
Alexandre Thibault
Elisa Bou
Arnaud Huguet
Branched glycerol dialkyl glycerol tetraethers (brGDGTs) are bacterial lipids that have been widely used as environmental proxies in continental paleorecords. Another group of related lipids, branched glycerol monoalkyl glycerol tetraethers (brGMGTs), has recently been proposed as a potential paleotemperature proxy. Nevertheless, the sources and environmental dependencies of both brGDGTs and brGMGTs along the river–sea continuum are still poorly understood, complicating their application as paleoenvironmental proxies in some aquatic settings. In this study, the sources of brGDGTs and brGMGTs and the potential factors controlling their distributions are explored across the Seine River basin (NW France), which encompasses the freshwater-to-seawater continuum. BrGDGTs and brGMGTs were analyzed in soils, suspended particulate matter (SPM), and sediments (n=237) collected along the land–sea continuum of the Seine basin. Both types of compounds (i.e., brGDGTs and brGMGTs) are shown to be produced in situ, in freshwater and saltwater, based on their high concentrations and distinct distributions in aquatic settings (SPM and sediments) vs. soils. Redundancy analysis further shows that both salinity and nitrogen dominantly control the brGDGT distributions. Furthermore, the relative abundance of 6-methyl vs. that of 5-methyl brGDGTs (the IR6Me ratio), the total nitrogen (TN), the δ15N, and the chlorophyll a concentration co-vary in a specific geographical zone with low salinity, suggesting that 6-methyl brGDGTs are preferentially produced under low-salinity and high-productivity conditions. In contrast to brGDGTs, the brGMGT distribution appears to be primarily regulated by salinity, with a distinct influence on the individual homologues. Salinity is positively correlated with homologues H1020a and H1020b and negatively correlated with compounds H1020c and H1034b in SPM. This suggests that bacteria living in freshwater preferentially produce compounds H1020c and H1034b, whereas bacteria that primarily grow in saltwater appear to be predominantly responsible for the production of homologues H1020a and H1020b. Based on the abundance ratio of the freshwater-derived compounds (H1020c and H1034b) vs. their saltwater-derived homologues (H1020a and H1020b), a novel proxy, the Riverine IndeX (RIX), is proposed to trace riverine organic matter inputs, with high values (>0.5) indicating a higher riverine contribution. We successfully applied RIX to the Godavari River basin (India) and a paleorecord across the upper Paleocene and lower Eocene from the Arctic Coring Expedition at Lomonosov Ridge, showing its potential applicability to both modern samples and paleorecords.
- Article
(5541 KB) - Full-text XML
-
Supplement
(2680 KB) - BibTeX
- EndNote
Branched glycerol dialkyl glycerol tetraethers (brGDGTs) are membrane lipids produced by bacteria, with some of them belonging to the phylum Acidobacteria (Sinninghe Damsté et al., 2011; Chen et al., 2022; Halamka et al., 2023). These compounds were observed to occur ubiquitously in a wide range of terrestrial and aquatic environments (Schouten et al., 2013; Raberg et al., 2022). The distribution of brGDGTs (number of cyclopentane moieties and methyl groups; see the structures in Fig. S1 in the Supplement) has been linked with the pH and temperature in soils (Weijers et al., 2007; De Jonge et al., 2014; Véquaud et al., 2022), peats (Naafs et al., 2017; Véquaud et al., 2022), and lake sediments (Tierney et al., 2010; Russell et al., 2018; Martínez-Sosa et al., 2021; Raberg et al., 2021; Zhao et al., 2023). The brGDGT-based proxies (e.g., MBT) have been widely applied to reconstruct paleoclimate from sedimentary archives (Coffinet et al., 2018; Harning et al., 2020; Wang et al., 2020).
In aquatic settings, brGDGTs were initially suggested to be transported by erosion to the sediments (Hopmans et al., 2004). Based on this assumption, the branched and isoprenoid tetraethers (BIT) index was defined as the abundance ratio of the major brGDGTs to crenarchaeol (an isoprenoid GDGT mainly produced by marine Nitrososphaerota). The BIT index ranges between 0 and 1, with high BIT values (around 1) reflecting a higher contribution of terrestrial organic matter compared to marine organic matter (Hopmans et al., 2004).
In recent years, the BIT index has been broadly used to quantify the relative contribution of terrestrial organic matter in aquatic systems (Xu et al., 2020; Yedema et al., 2023) and to evaluate the reliability of the TEX86 paleothermometer (Cramwinckel et al., 2018). In addition to terrestrial sources, brGDGTs can also originate from aquatic settings, including rivers (e.g., De Jonge et al., 2015; Freymond et al., 2017; Kim et al., 2015; Zell et al., 2014, 2013), lakes (Tierney and Russell, 2009), and marine settings (Dearing Crampton-Flood et al., 2019; Zeng et al., 2023). This adds complexity to the identification of brGDGT sources in aquatic ecosystems and to the application of brGDGTs as (paleo)environmental proxies, including the BIT index.
Furthermore, BIT values must be carefully interpreted, especially considering the potential influence of the selective degradation of branched vs. isoprenoid GDGTs (Smith et al., 2012). Thus, complementary molecular proxies for quantifying the input of terrestrial organic matter to aquatic settings are still needed. These proxies may cross-validate other available terrestrial proxies, such as the δ13C of organic carbon (Lamb et al., 2006), heterocyst glycolipids (Kang et al., 2023), and long-chain diols (Lattaud et al., 2017). Recently, a machine-learning approach (the BIGMaC model) was proposed to infer the origins of environmental samples (e.g., soil, peat, marine, and lake settings) based on their GDGT distributions (Martínez-Sosa et al., 2023). While such an approach shows potential for differentiating distinct sources of GDGTs, its application to aquatic systems has not yet been extensively explored.
The improvement of chromatographic methods allowed the separation and quantification of 5-, 6-, and 7-methyl brGDGTs (methyl groups at the fifth, sixth, and seventh positions; Fig. S1), which previously co-eluted (De Jonge et al., 2013, 2014; Ding et al., 2016). This led to the development of new brGDGT-based proxies based on these specific brGDGT isomers (De Jonge et al., 2014). Compounds eluting later than 7-methyl brGDGTs are tentatively designated 1050d and 1036d, as their exact chemical structures are currently unknown (Wang et al., 2021). The fractional abundances of the individual brGDGT isomers were shown to be influenced by distinct environmental factors. For example, the relative abundance of 5-methyl brGDGTs was correlated with temperature, whereas that of 6-methyl brGDGTs was correlated with pH (De Jonge et al., 2014). In addition, recent studies in lakes observed an influence of salinity on the relative abundances of 6-methyl and 7-methyl brGDGTs and their late-eluting compounds (Wang et al., 2021; Kou et al., 2022). This suggests that salinity could also control the distribution of these compounds in other systems, like river–sea continuums, but this assumption has not yet been studied.
Compared with brGDGTs, the branched glycerol monoalkyl glycerol tetraethers (brGMGTs; also referred to as H-brGDGTs) are a much less studied group of lipids. Recent studies have revealed their presence in diverse environments, including peatlands (Naafs et al., 2018; Tang et al., 2021), marine settings (Liu et al., 2012; Xie et al., 2014), rivers (Kirkels et al., 2022a), soils (Baxter et al., 2021; Kirkels et al., 2022a), and lakes (Baxter et al., 2019, 2021). The brGMGTs are labeled H1020, H1034, and H1048 (see Fig. S1), with isomers indicated by the presence of a suffix letter (either “a”, “b”, or “c”, reflecting the order in which they elute according to Baxter et al., 2019). These compounds are structurally similar to brGDGTs but possess an additional covalent carbon–carbon bond between the alkyl chains, leading to an “H-shaped” structure. The bridge in brGMGTs was considered to be a primary adaptation to heat stress (Naafs et al., 2018; Baxter et al., 2019). Their presumed membrane stability under high-temperature conditions was inferred from the behavior of isoprenoid glycerol monoalkyl glycerol tetraethers (isoGMGTs), which were identified in a hyperthermophilic methanogen (Morii et al., 1998) and deep-sea hydrothermal vents (Schouten et al., 2008). Although a rigorous chemical characterization of brGMGTs is lacking and the source organisms of brGMGTs are unknown, correlations between the relative abundances of brGMGTs and mean annual temperature were observed in peat soils (Naafs et al., 2018) and lakes (Baxter et al., 2019), showing their potential as temperature proxies. In addition to temperature, anoxic conditions may also trigger brGMGT production in the anoxic zone of peats (Naafs et al., 2018; Tang et al., 2021), in the anoxic part of the water column and/or sediments in lakes (Baxter et al., 2021), in regularly inundated soils (Kirkels et al., 2022a), and in the oxygen minimum zone in marine environments (Xie et al., 2014). Furthermore, shifts in microbial community composition in response to other (unknown) environmental factors seem to control the relative abundances of brGMGTs in peats and lignites (Elling et al., 2023). Henceforth, in order to use the brGMGTs as environmental proxies in sedimentary records, it is still necessary to understand which factors control their distributions in riverine and marine water columns and sediments. This remains poorly understood (Sluijs et al., 2020; Bijl et al., 2021).
Based on previous studies of brGDGTs and brGMGTs in terrestrial and marine settings (Dearing Crampton-Flood et al., 2019; Wang et al., 2021; Kirkels et al., 2022a, b; Kou et al., 2022), we hypothesize (1) that both brGDGTs and brGMGTs can be produced in situ in aquatic systems and (2) that the brGDGT and brGMGT distributions are influenced by surrounding environmental factors and vary spatially along the land–sea continuum. These compounds have a potential to be used as proxies of riverine organic matter inputs along estuaries. These hypotheses were tested by examining and comparing the distributions of brGDGTs and brGMGTs in soils, suspended particulate matter (SPM), and sediments (n=237) collected all along the Seine River basin (NW France), covering its riverine and estuarine parts. The aim of the present study was (1) to investigate the sources of brGDGTs and brGMGTs along the Seine land–sea continuum, (2) to determine the predominant environmental controls affecting the distribution of these molecules, and (3) to assess the potential of brGMGTs as a riverine runoff proxy.
2.1 Study area
The Seine River basin (the Seine River and its estuary; Fig. 1a) is more than 760 km long and drains through the greater Paris region (over 12 million inhabitants) to the English Channel (Flipo et al., 2021). The Seine Estuary is a macrotidal estuary, given its large tidal range, small depth, and morphology. The maximum flows are generally observed in winter (over 700 m3 s−1; Fig. 1b), whereas the minimum flows are observed in summer (below 250 m3 s−1; Fig. 1b). The tide influences the estuary up to the city of Poses (site 5 at KP 202 in Fig. 1a; “KP” represents “kilometric point”, defined as the distance in kilometers from the city of Paris), where a dam constitutes the boundary between the river and the estuary. Based on spatiotemporal variations of salinity, the estuary can be divided into two major parts. The upstream estuary corresponds to the freshwater tidal sector (KP 202 to KP 298; from site 5 to site 12; Fig. 1a and Table 1), and the downstream estuary is influenced by a salinity gradient (starting at KP 298; from site 12 to the coastal area; Fig. 1a and Table 1) (Romero et al., 2016; Druine et al., 2018).
Table 1Locations of the sampling sites along the Seine basin, along with the types of samples collected.
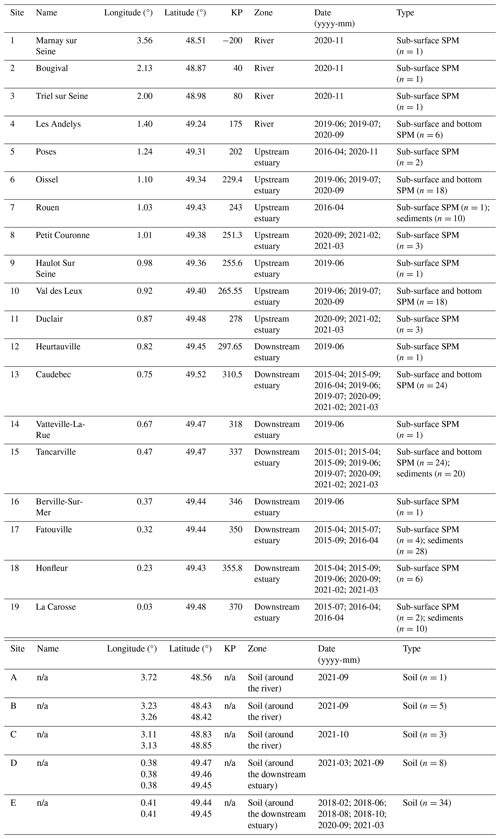
n/a – not applicable.
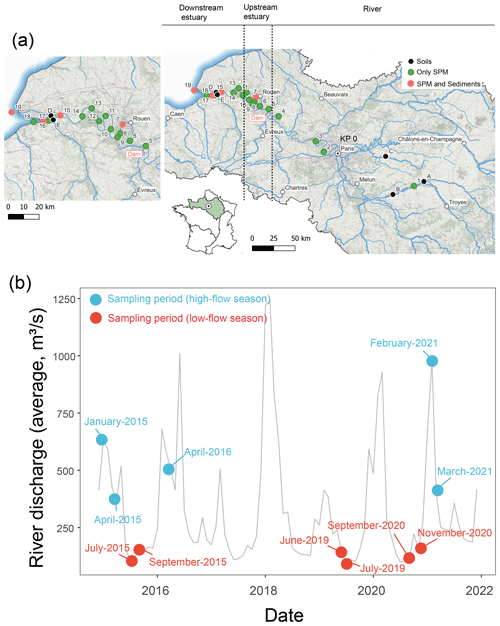
Figure 1(a) Geographical locations of sampling sites in the Seine River basin (KP: kilometric point, the distance in kilometers from the city of Paris (KP 0)). The sampling sites in the upstream estuary and downstream estuary are shown in the zoomed-in figure. Sub-surface SPM was collected at sites 1 to 18, while both sub-surface and bottom SPM were collected at sites 4, 6, 10, 13, and 15. The map was generated based on the layer from the Agence de l'Eau Seine-Normandie. (b) Mean monthly water discharge for the Seine River at the Paris Austerlitz station from 2015 to 2021 (data from https://www.hydro.eaufrance.fr/, last access: 12 October 2022). Circles indicate sampling periods in the high-flow (>250 m3 s−1: blue) and low-flow (<250 m3 s−1: red) seasons.
2.2 Sampling
From June 2019 to March 2021, water samples (n=102) were collected across the Seine River (Fig. 1a). Sub-surface water (ca. 1 m depth) samples were collected in high-flow (over 250 m3 s−1) and low-flow (below 250 m3 s−1) periods from the three zones (the river, upstream estuary, and downstream estuary) of the Seine River basin (Table 1). At five sites (sites 4, 6, 10, 13, and 15; Fig. 1a and Table 1), both sub-surface and bottom-water (2.2–16 m depth) samples were retrieved using a pump and placed into precleaned 20 L fluorinated high-density polyethylene (FLPE) Nalgene carboys. Estuarine water samples (at sites 6, 10, 13, and 15; Fig. 1a and Table 1) were collected at three tide periods (high tide, low tide, and mid-tide). For these sites, 0.25–43 L of water was immediately filtered using pre-combusted Whatman GF/F 0.7 µm glass fiber filters. After filtration, the filters were freeze-dried, scratched, and stored frozen at −20 °C prior to analysis.
Additional SPM samples (n=16; Table 1) used in this study for brGDGT and brGMGT analysis were collected from the upstream and downstream estuary (at sites 5, 7, 13, 15, 17, 18, and 19; Fig. 1a and Table 1) in 2015 and 2016, as detailed by Thibault et al. (2019). Sediments (n=68) from seven cores (10 cm depth) were collected using a UWITEC corer, as described by Thibault et al. (2019), in the river channel at the same sites as the SPM samples taken in 2015 and 2016 (Table 1). These sediments were further sliced (1 cm thickness) and freeze-dried. For each core, 10 samples were analyzed for brGDGTs and brGMGTs, except for the core collected at site 17 in April 2016, where no lipids were detected between 4–5 and 5–6 cm depth. Surficial soils (n=9) were collected in the lateral area of the upstream section of the Seine River in 2021 (sites A, B, and C; Fig. 1a and Table 1) and freeze-dried. Additional wetland soils and mudflat sediments (n=42) were collected in the downstream estuary in 2018, 2020, and 2021 (sites D and E; Fig. 1a and Table 1); these represented allochthonous material transported into the estuary by tidal effects. These samples were collected at low tide using a plexiglass® core (4.5 cm depth) and taken back to the laboratory, where they were homogenized, freeze-dried, and ground using a ball mill (model MM400, Retsch®).
2.3 Elemental and isotopic analyses
Elemental and isotopic analyses of the soils (surficial soils and mudflat sediments, n=51) and SPM (n=102) collected from 2018 to 2021 were performed following the method described in Thibault et al. (2019). The samples were split, and one aliquot was decarbonated. Briefly, 40 mg of SPM and 1 g of soil/sediment samples were firstly decarbonated by adding 10 mL of 3 M HCl for 2 h with magnetic stirring at room temperature. Subsequently, these samples were rinsed using ultrapure water and centrifuged until a neutral pH was reached. The obtained decarbonated samples were stored at −20 °C and freeze-dried. Both decarbonated and non-decarbonated samples (∼ 6 mg for SPM and ∼ 20 mg for soils) were enclosed in a tin capsule. The total organic carbon (TOC) content and stable carbon isotopic composition (δ13C) were measured in decarbonated samples using an elemental analyzer coupled with an isotope ratio mass spectrometer (Thermo Fisher Scientific Delta V Advantage) at the ALYSES platform (Sorbonne University/IRD, Bondy, France). The total nitrogen (TN) and nitrogen isotopic composition (δ15N) were measured in non-decarbonated samples, as acidification could impact the N contents (Ryba and Burgess, 2002). The isotopic composition (δ13C or δ15N) was expressed as the relative difference between isotopic ratios in samples and in standards (Vienna Pee Dee Belemnite for carbon and atmospheric N2 for nitrogen). Additional elemental and isotopic data based on SPM and sediments collected in 2015 and 2016 (n=84) were obtained from Thibault et al. (2019).
2.4 Lipid extraction and analyses
The lipids from surficial soils and mudflat sediments (4–20 g, n=51) and from SPM samples (∼ 150 mg, n=102) were extracted ultrasonically (3×) with 20 to 40 mL of dichloromethane (DCM) methanol (MeOH) (, ) per extraction. Lipids from the SPM and sediment samples (n=84) collected in 2015 and 2016 were previously extracted by Thibault (2018) following the same method. The total lipid extracts were then separated into fractions of increasing polarity on an activated silica gel column (ca. 10 mg) using (i) 30 mL of heptane, (ii) 30 mL of heptane DCM (, ), and (iii) 30 mL of DCM MeOH (, ) as eluents. An aliquot (30 %) of the third (polar) fraction containing GDGTs and GMGTs was dried, re-dissolved in heptane, and passed through a 0.2 µm polytetrafluoroethylene (PTFE) filter (Ultrafree-MC; Merck). C46 glycerol trialkyl glycerol tetraether (GTGT) was used as an internal standard (Huguet et al., 2006); 5 µL of this standard (0.01025 mg mL−1) was typically added to 45 µL of sample.
GDGTs and GMGTs were analyzed using a Shimadzu LCMS 2020 high-pressure liquid chromatograph coupled with a mass spectrometer with an atmospheric-pressure chemical ionization source (HPLC-APCI-MS) in selected ion monitoring mode, modified from Hopmans et al. (2016) and Huguet et al. (2019). Tetraether lipids were separated with two silica columns in tandem (BEH HILIC columns, 2.1 × 150 mm, 1.7 µm; Waters) thermostated at 30 °C. The injection volume was 30 µL. The flow rate was set at 0.2 mL min−1. GDGTs and GMGTs were eluted isocratically for 25 min with 82 % A 18 % B (A is hexane; B is hexane isopropanol , ), followed by a linear gradient to 65 % A 35 % B in 25 min, a linear gradient to 100 % B in 30 min, and back to 82 % A 18 % B in 4 min, maintained for 50 min. Identification of the different brGMGT isomers was achieved by comparison of the peak retention time with those of known brGMGTs in Baxter et al. (2019) and Kirkels et al. (2022a). Semi-quantification of GDGTs and brGMGTs was performed by comparing the integrated signal of the respective compound with the signal of a C46 synthesized internal standard (Huguet et al., 2006), assuming their response factors to be identical.
The detection limit was set at a signal-to-noise ratio (SNR) of 3. Peaks with a lower SNR (<3) are not distinguishable from the background noise and were considered to be below the limit of quantification.
2.5 Calculation of GDGT proxies
The index IR6Me represents the proportion of 6-methyl brGDGTs vs. 5-methyl brGDGTs and was calculated according to Eq. (1) (from De Jonge et al., 2015; the Roman numbers refer to the structures in Fig. S1):
The index IR7Me represents the proportion of 7-methyl brGDGTs and late-eluting isomers calculated according to Eq. (2) (from Wang et al., 2021):
The index IR6+7Me represents the average of IR6Me and IR7Me according to Eq. 3 (from Wang et al., 2021):
The Archaeol Caldarchaeol Ecometric (ACE) index was calculated according to Eq. (4) (from Turich and Freeman, 2011):
The BIT index, which includes the 6-methyl brGDGTs, was calculated according to Eq. (5) (from De Jonge et al., 2015):
Based on replicate injections of three different samples, the averaged standard deviations were 0.004 for IR6Me, 0.005 for IR7Me, 0.003 for IR6+7Me, 8.54 for ACE, and 0.032 for BIT.
2.6 Water quality measurements
Water turbidity was measured by a CTD probe from Sea-Bird Scientific. Water temperature, dissolved oxygen, salinity, and pH were measured using an automated YSI 6000 multi-parameter probe (YSI Inc., Yellow Springs, OH, USA). Chlorophyll a (Chl a) concentrations in water samples were measured after filtration through Whatman GF/F 0.7 µm glass fiber filters, which were stored frozen (−20 °C) before analysis. Chl a was extracted using filters with incubation in 10 mL of 90 % acetone for 12 h in the dark at 4 °C. After two centrifugations (1700 g, 5 min), Chl a concentrations were measured using a Turner Designs fluorometer according to the method of Strickland and Parsons (1972), as described in the reference protocol of SNO SOMLIT (Service d'observation du Milieu Littoral). Water quality measurements were performed at the Laboratoire Ecologie Fonctionnelle et Environnement (Université de Toulouse) as well as at UMR BOREA (Université de Caen Normandie).
2.7 Statistical analyses
All statistical analyses were performed using the R software (version 4.2.1). Non-parametric statistical tests were used due to the non-normal distribution of the dataset (tested by the Shapiro–Wilk normality test; p values < 0.05). Specifically, Spearman's correlation was used to investigate potential correlations among different features (environmental parameters, fractional abundances of brGDGTs and brGMGTs, and proxies derived from these compounds), and the unpaired two-sample Wilcoxon test (also known as the Mann–Whitney test or Wilcoxon rank-sum test) was used for two independent group comparisons. The significance level is indicated by asterisks: * indicates a p value < 0.05; indicates a p value < 0.01; indicates a p value < 0.001; indicates a p value < 0.0001; and ns (not significant) indicates a p value > 0.05.
A principal component analysis (PCA) was performed on the fractional abundances of brGDGTs and brGMGTs using the R packages factoextra and FactoMineR. The different groups of samples were highlighted by adding 95 % concentration ellipses. The proportion of the variance in brGDGT and brGMGT compositions that can be explained by different groups was evaluated by permutational multivariate analysis of variance using distance matrices (adonis) performed by the adonis2 function of the R package vegan using the Bray–Curtis distances and 999 permutations.
A redundancy analysis (RDA) was performed using the R package vegan to investigate the relationships between environmental parameters and the brGDGT or brGMGT distribution in SPM. The angles between brGDGTs or brGMGTs and environmental factors were used to identify the potential relationships. Right angles (90°) reflect a lack of linear correlations, whereas small or straight angles (close to 0 or 180°, respectively) imply positive or negative linear correlations, respectively. Compounds that were close to each other were assumed to be strongly linked, representing similar distribution patterns and comparable responses to the environmental conditions. To evaluate the relative importance of each explanatory variable (environmental parameter) to the brGDGT or brGMGT distribution, a hierarchical partitioning method implemented in the R package rdacca.hp was used. Briefly, this approach suggests that shared variance can be decomposed into equal components based on the number of predictors (environmental factors) involved, which allows the estimation of the relative importance of each predictor by adding its partial R2 to the sum of all allocated average shared R2. While most selection procedures, such as forward selection, use predictor ordering to assess variable importance, hierarchical partitioning calculates individual importance (the sum of unique and total average shared effects) from all subset models, generating an unordered assessment of variable importance (Lai et al., 2022).
Spatio-temporal variations in environmental factors and proxies derived from brGDGTs and brGMGTs were assessed after applying a locally estimated scatterplot smoothing (LOESS) method. This method identifies nonlinear data patterns and buffers the effect of aberrant data and outliers. LOESS was implemented by the geom_smooth function of the R package ggplot2.
2.8 Machine learning
The BigMac model, developed by Martínez-Sosa et al. (2023) based on the brGDGT and isoGDGT distribution, was applied. Subsequently, using the same algorithm (random forest), we developed our own model based on either brGDGTs or brGMGTs.
For independent models, our lipid dataset was split into a training set (75 %) and a test set (25 %). We then used a supervised machine-learning algorithm (random forest) to train models. This algorithm was applied to classify the downstream estuary and soil samples based on brGDGTs or brGMGTs as input and was implemented using the scikit-learn library (https://github.com/scikit-learn/, last access: 2 December 2023) (Pedregosa et al., 2011) in Python (version 3.10.12). Hyperparameter tuning was conducted using a randomized search approach implemented through the RandomizedSearchCV function in scikit-learn.
SHapley Additive exPlanations (SHAP) is a game-theoretical method used to interpret machine learning models (Lundberg et al., 2020). SHAP analysis was applied to identify which compounds were important for the classifications and was implemented by the SHAP library in Python. A higher SHAP value indicates a more substantial contribution of the feature (brGDGTs or brGMGTs) to the predicted outcome (downstream estuary or soils).
3.1 Distribution of bulk parameters from land to sea
The total organic carbon (TOC) content was significantly higher in the upstream estuary (4.64 ± 1.42 %, based on SPM and river channel sediments) than in the downstream estuary (3.30 ± 1.69 %, based on SPM and sediments), soils (3.03 ± 3.49 %, based on surficial soils and mudflat sediments), and the river (2.88 ± 1.14 %, based on SPM) (Table S1 in the Supplement). The total nitrogen (TN) content was higher in the upstream estuary (0.51 ± 0.17 %, based on SPM and sediments) than in the river (0.37 ± 0.15 %, based on SPM), downstream estuary (0.31 ± 0.14 %, based on SPM and river channel sediments), and soils (0.24 ± 0.17 %, based on surficial soils and mudflat sediments) (Table S1). Lower values were observed in the river (8.04 ± 4.31, based on SPM) and upstream estuary (9.42 ± 3.67, based on SPM and sediments) compared to the downstream estuary (10.73 ± 3.59, based on SPM and sediments) and soils (11.59 ± 4.79, based on surficial soils and mudflat sediments) (Fig. 2). Much lower values of δ13Corg were observed in the river (−31.30 ± 1.91 ‰, based on SPM) and upstream estuary (−30.62 ± 1.66 ‰, based on SPM and sediments) than in the downstream estuary (−26.45 ± 1.34 ‰, based on SPM and sediments) and soils (−26.55 ± 1.13 ‰, based on surficial soils and mudflat sediments) (Fig. 2). In addition, no significant differences in δ15N were observed along the river basin (Fig. 2).
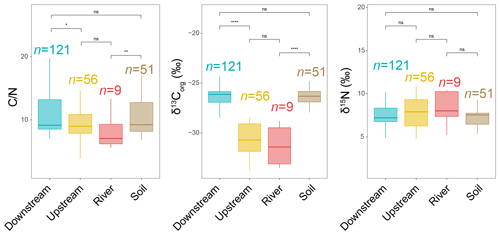
Figure 2Distribution of bulk parameters (, δ13Corg, and δ15N) from soils (surficial soils and mudflat sediments) as well as river, upstream-estuary, and downstream-estuary samples across the Seine River basin. Box plots of upstream- and downstream-estuary samples are based on SPM and sediments, whereas those of river samples are based only on SPM. Boxes show the upper and lower quartiles of the data, and whiskers show the range of the data. The boxes and whiskers are color coded based on the sample type (river in red, upstream estuary in yellow, and downstream estuary in blue). The center line in each box indicates the median value of the dataset. Statistical testing was performed using a Wilcoxon test (* p < 0.05; p < 0.01; p < 0.001; p < 0.0001; ns, not significant, p > 0.05).
3.2 Distribution of brGDGTs from land to sea
The brGDGTs (IIIa5, IIIb5, IIIc5, IIa5, IIb5, IIc5, IIIa6, IIIb6, IIIc6, IIa6, IIb6, IIc6, IIIa7, IIIb7, IIa7, Ia, Ib, Ic, 1050d, and 1036d) were all detected in all studied samples. The brGDGT chromatograms from upstream samples (SPM and river channel sediments) differed markedly from downstream-estuarine samples (SPM and sediments). For example, 6-methyl brGDGTs were much more abundant than 5-methyl brGDGTs in the river (SPM) and upstream estuary (SPM), whereas the strong predominance of 6-methyl vs. 5-methyl brGDGTs decreased in the downstream-estuarine SPM samples (Fig. S2). Furthermore, the peaks from the recently described 7-methyl brGDGTs and their late-eluting isomers (i.e., 1050d) were more pronounced in the downstream estuary than in the rest of the Seine basin (Fig. S2).
The relative abundances of the brGDGTs were determined all along the Seine River basin (Figs. 3 and S3, S4). The 6-methyl brGDGTs (IIIa6, IIa6, IIIb6, IIb6, and IIIc6) were significantly higher in the river (SPM) and upstream estuary (SPM and river channel sediments) than in soils (surficial soils and mudflat sediments) and the downstream estuary (SPM and river channel sediments). In addition, the relative abundances of 7-methyl brGDGTs (IIIa7 and IIa7) and their late-eluting compound (1050d) were significantly higher in the downstream estuary (SPM and river channel sediments) and soils (surficial soils and mudflat sediments) than in the river (SPM) and the upstream estuary (SPM and river channel sediments).
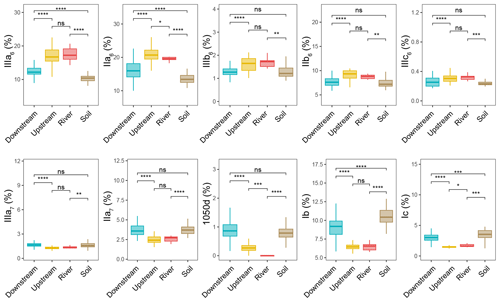
Figure 3Relative abundances of selected individual brGDGTs – cyclopentane-containing tetramethylated brGDGTs (Ib and Ic), 6-methyl brGDGTs (IIa6, IIIa6, IIb6, IIIb6, and IIIc6), 7-methyl brGDGTs (IIa7 and IIIa7), and brGDGT 1050d – with respect to 20 brGDGTs (IIIa5, IIIb5, IIIc5, IIa5, IIb5, IIc5, IIIa6, IIIb6, IIIc6, IIa6, IIb6, IIc6, IIIa7, IIIb7, IIa7, Ia, Ib, Ic, 1050d, and 1036d) from soil (surficial soils and mudflat soils or sediments, n=51), river (n=9), upstream-estuary (n=56), and downstream-estuary (n=121) samples across the Seine River basin. Box plots of upstream- and downstream-estuary samples are based on SPM and sediments, whereas those of river samples are based only on SPM. Boxes show the upper and lower quartiles of the data, and whiskers show the range of the data. The boxes and whiskers are color coded based on the sample type (river in red, upstream estuary in yellow, and downstream estuary in blue). The center line in each box indicates the median value of the dataset. Statistical testing was performed using a Wilcoxon test (* P < 0.05; P < 0.01; P < 0.001; P < 0.0001; ns, not significant, P > 0.05).
The concentration of total brGDGTs also showed differences along the land to sea continuum (Fig. S5a). The total brGDGT concentration decreased from the river (10.51 ± 5.91 µg g−1 organic carbon (Corg), based on SPM samples) to the upstream estuary (7.52 ± 5.09 µg g−1 Corg, based on SPM and sediments) and the downstream estuary (4.95 ± 4.09 µg g−1 Corg, based SPM and sediments). The concentration of total brGDGTs in soils from all around the Seine basin (1.55 ± 1.61 µg g−1 Corg, based on surficial soils and mudflat sediments) was significantly lower than that in SPM and sediments (Fig. S5a).
A principal component analysis (PCA) was performed to statistically compare the fractional abundances of brGDGTs (based on SPM and sediments collected in the river channel) from different locations (the river and the upstream and downstream estuary), which explained 54.1 % of the variance in the first two dimensions (Fig. 4a). The first axis (PC1) explained 40.9 % of the variance, with negative loadings for most of the 6-methyl brGDGTs and positive loadings for the remaining brGDGTs (Fig. 4a). Samples from the downstream estuary clustered apart from those from the river and upstream parts. Specifically, the brGDGT distribution was dominated by 6-methyl brGDGTs (IIIa6, IIIb6, IIIc6, IIa6, and IIb6) in river and upstream-estuarine samples, whereas in the downstream estuary, it was driven by 5-methyl brGDGTs (III5, IIa5, IIc5, IIb5 and IIIb5), tetramethylated brGDGTs (Ia, Ib, and Ic), 7-methyl brGDGTs (IIIa7, IIa7, and IIb7), and their late-eluting compounds (1050d and 1036d). The brGDGT distributions of soils (surficial soils and mudflat sediments) were included in the PCA biplot generated for SPM and river channel sediments. This revealed that the brGDGT distribution in soils mostly overlaps with those in downstream-estuarine SPM and river channel sediments (Fig. 4a).
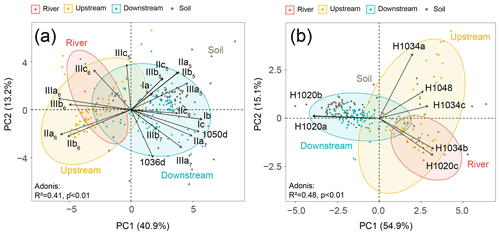
Figure 4PCA analysis of the fractional abundances of (a) brGDGTs and (b) brGMGTs. The coordinates of passive individuals (soils) were added passively as an overlay. They were predicted based on the information provided by the existing PCA performed on SPM and sediments (active individuals). Adonis analysis was used to evaluate how the variation can be explained by the variables (999 permutations).
A redundancy analysis (RDA) was performed to investigate the influence of the environmental factors (TOC, TN, temperature, water discharge, and salinity) on the brGDGT distributions in SPM samples (Fig. 5a and Table 2). It allowed 39.79 % of the variability to be explained through two dimensions. The RDA triplot (Fig. 5) showed how these factors correlate to the distributions of individual brGDGTs. The first axis of the RDA explained 33.16 % of the variability and was primarily correlated with salinity and TN, whereas the second axis explained 6.63 % of the variability and was associated with temperature, water discharge, and TOC (Fig. 5a and Table 2). Based on hierarchical partitioning, salinity and TN were the two most important variables in explaining the brGDGT variations (with an individual importance of 14.97 % for salinity and 13.47 % for TN; Fig. 5b and Table 2). Compared with the salinity and TN, other available parameters had much lower individual importance (3.68 % for water discharge, 3.6 % for temperature, and 2.12 % for TOC; Fig. 5b and Table 2).
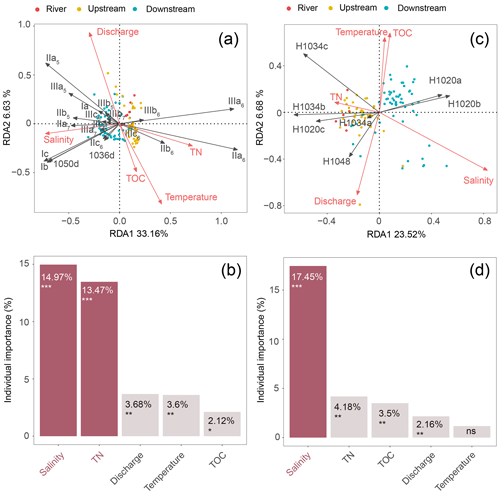
Figure 5RDA analysis showing relationships between environmental factors (TN, TOC, salinity, temperature, and discharge; red arrows) and the fractional abundances of (a) brGDGTs and (c) brGMGTs. The individual importance of each environmental factor (TN, TOC, salinity, temperature, and discharge) explaining the variation in the (b) brGDGT and (d) brGMGT distributions was determined by hierarchical partitioning analysis. The dataset used for RDA analysis is composed of SPM from the river (n=6; red), upstream estuary (n=42; yellow), and downstream estuary (n=59; blue). The significance level is indicated by asterisks: * p < 0.05; p < 0.01; p < 0.001; ns, not significant, p > 0.05. p values are derived from permutation tests (999 randomizations).
3.3 Distribution of brGMGTs from land to sea
The brGMGTs (H1020a, H1020b, H1020c, H1034a, H1034b, H1034c, and H1048) identified by Baxter et al. (2019) were detected in the samples collected across the Seine River basin. H1034a is the least-abundant isomer and is below the detection limit for most of the SPM and sediment samples in the Seine River basin (Figs. S6 and S7). The chromatograms revealed distinct distributions of brGMGTs in the different parts of the basin (SPM and sediments), with, e.g., a higher intensity for the homologue H1020c in the river samples (SPM) than in the samples from the upstream estuary (SPM) and downstream estuary (SPM) (Fig. S2). These spatial variations were apparent when calculating the fractional abundances of the individual brGMGTs (Figs. 6 and S5, S6). The relative abundances of H1020a and H1020b increased from the river to the downstream estuary, whereas those of 1020c and H1034b decreased (Fig. 6). In SPM and river channel sediments, the total brGMGT concentration was observed to be slightly (but not significantly) higher in the riverine part (0.26 ± 0.24 µg g−1 Corg) than in downstream-estuary (0.20 ± 0.13 µg g−1 Corg) and upstream-estuary (0.17 ± 0.18 µg g−1 Corg; Fig. S5b) samples. All over the basin, the total brGMGT concentrations were lowest in soils (surficial soils and mudflat sediments; 0.07 ± 0.09 µg g−1 Corg; Fig. S5b).
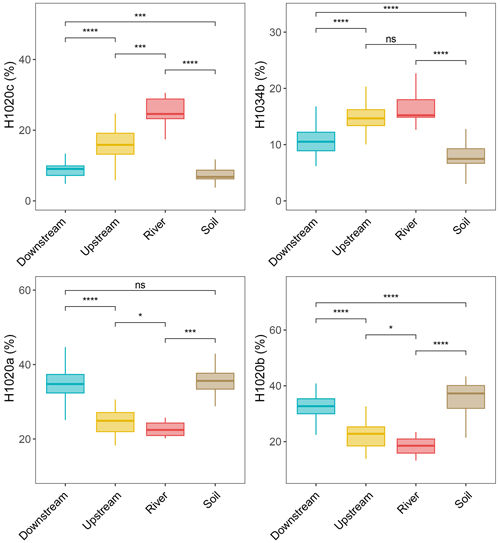
Figure 6Relative abundances of selected individual brGMGTs with respect to seven brGMGTs (H1020a, H1020b, H1020c, H1034a, H1034b, H1034c, and H1048) from soils (surficial soils and mudflat soils/sediments, n=51), the river (n=9), the upstream estuary (n=56), and the downstream estuary (n=121) across the Seine River basin. Box plots for the upstream and downstream estuaries are based on SPM and river channel sediments, whereas those for the river are based on SPM. Boxes show the upper and lower quartiles of the data, and whiskers show the range of the data. The boxes and whiskers are color coded based on the sample type (river in red, upstream estuary in yellow, and downstream estuary in blue). The center line of each box indicates the median value of the dataset. Statistical testing was performed using a Wilcoxon test (* p < 0.05; p < 0.01; p < 0.001; p < 0.0001; ns, not significant, p > 0.05).
The PCA analysis based on the brGMGT relative abundances (Fig. 4b) explained 70 % of the variance in the first two dimensions, which separated samples from different parts of the basin. The first axis explained 54.9 % of the variance, separating downstream-estuarine samples from riverine and upstream-estuarine samples, with negative loadings for two brGMGTs (H1020a and H1020b) and positive loadings for the remaining brGMGTs (H1020c, H1034a, H1034b, H1034c, and H1048). The second axis explained 15.1 % of the variance and mainly separated the riverine and upstream-estuarine samples, with higher relative abundances of the compounds H1020c and H1034b observed in riverine samples (Fig. 4b). The soil brGMGT distributions were passively added to the PCA biplot based on SPM and sediments, revealing that the soils largely overlap with the SPM and sediments collected in the downstream estuary (Fig. 4b).
An RDA was performed to investigate the factors that could explain the variability of the brGMGT distributions in the SPM samples (Fig. 5c and Table 2). This RDA explained 30.2 % of the variance in the first two axes. The RDA triplot showed that the first axis, accounting for 23.52 % of the variability, was associated mainly with salinity and to a lesser extent TN, while the second axis (6.68 %) was mainly driven by temperature, TOC, and water discharge (Fig. 5c and Table 2). Based on hierarchical partitioning, salinity had the highest individual importance (17.45 %) in explaining the variability of the brGMGT distribution, followed by TN (4.18 %), TOC (3.5 %), and water discharge (2.16 %) (Fig. 5d and Table 2).
4.1 Sources of brGDGTs and environmental controls on their distribution
4.1.1 Sources of brGDGTs
In order to determine the predominant origin of brGDGTs in the Seine River basin, the overall brGDGT concentrations and distributions in SPM and river channel sediments (n=186) were compared with those in soils (surficial soils and mudflat sediments, n=51). The brGDGT concentrations (normalized to Corg) and relative abundances of several brGDGTs (i.e., IIIa6, IIa6, IIIb6, IIb6, and IIIc6) in the SPM and sediments were significantly higher than those in soils (p < 0.05, Wilcoxon test; Figs. S5a and 3). Such differences in brGDGT concentrations and relative abundances between soils and aquatic settings (SPM and sediments) imply that a portion of the brGDGTs in the water column and sediments of the Seine River basin are produced in situ. This is in agreement with previous findings which suggested an in situ aquatic contribution to the brGDGT pool (Peterse et al., 2009; De Jonge et al., 2015; Dearing Crampton-Flood et al., 2021; Kirkels et al., 2022b).
More specifically, the fractional abundances of the two major 6-methyl brGDGTs (IIa6 and IIIa6) are significantly higher in the Seine River and upstream estuary than in soils (Fig. 3). This confirms that these brGDGTs are mostly produced within the river, adding to the growing body of evidence supporting riverine 6-methyl brGDGT production in the water column and/or sediment (De Jonge et al., 2015; Bertassoli et al., 2022; Kirkels et al., 2022b). A subsequent shift in the brGDGT distributions in the downstream estuary compared to the upstream areas is observed in the Seine River basin. The PCA analysis shows a separation of downstream-estuarine samples (influenced by seawater intrusion) from riverine and upstream estuary ones (without significant seawater intrusion) (Fig. 4a). This difference is predominantly driven by the higher abundances of 6-methyl brGDGTs in riverine and upstream-estuarine samples vs. the higher abundances of 5- and 7-methyl brGDGTs as well as compounds Ib and Ic and the late-eluting brGDGTs 1050d and 1036d in downstream-estuarine samples (Figs. 3, 4a, and S4). The decrease in the fractional abundance of 6-methyl brGDGTs from the upstream estuary to the downstream estuary cannot be explained by the dam located at Poses (Fig. 1a). This dam separates the riverine part of the Seine from the upstream-estuarine section. Even during the low-flow season (Fig. 1b), at least part of the water from the Seine River upstream of Poses flows into the estuary (Romero et al., 2019). Thus, the dam should not prevent (some of) the riverine brGDGTs associated with SPM from reaching the estuary. It cannot be excluded that part of the riverine sediment is trapped by this dam. Nevertheless, all our estuarine samples were collected downstream of the dam, implying that the observed changes in brGDGT abundance and distribution within the estuary are intrinsic to the biogeochemical functioning of the Seine Estuary and cannot be attributed to the dam.
Instead, a shift in brGDGTs along the land–sea continuum may reflect the fact that riverine 6-methyl brGDGTs are more easily degraded than soil-derived homologues and are only partially transferred downstream. This hypothesis is based on a previous study which showed a shift in the brGDGT distribution from the Yenisei River to the Kara Sea (De Jonge et al., 2015). They interpreted this as being due to a preferential degradation of labile (riverine) 6-methyl brGDGTs and an enrichment in less-labile (soil-derived) 5-methyl brGDGTs during transport (De Jonge et al., 2015). This suggests that only limited amounts of riverine 6-methyl brGDGTs are transferred to the ocean, as also shown in other recent studies (Cao et al., 2022; Kirkels et al., 2022b). Such preferential degradation of 6-methyl brGDGTs over other brGDGTs could be attributed to variations in how these molecules are attached to soil particles (Huguet et al., 2008). Indeed, the higher degradation of 6-methyl brGDGTs upstream could be attributed to a difference in their attachment to particles upstream compared to downstream. The median diameter of the SPM was monitored between February 2015 and June 2016 in both the upstream (sites 7 and 10) and downstream (sites 15 and 17) parts of the Seine Estuary (Druine, 2018). The particle size showed only slight dispersion (80–110 µm) under various hydrological conditions in the upstream-estuarine section. The homogeneity in particle size in the upstream estuary likely reflects its predominantly continental origin (i.e., from the Seine River before the dam at Poses). In contrast, a large variability in the size of SPM particles was observed in the downstream estuary (15–20 to 80–90 µm), which was attributed to the complex flocculation and defragmentation processes of particles in this part of the estuary (Druine, 2018). Hence, the variability in the size of SPM particles from upstream to downstream could influence the distribution of brGDGTs in the Seine Estuary.
In addition to this hypothesis, a shift in the brGDGT distribution during downstream transport could be explained by mixing with autochthonous (i.e., estuarine-produced) brGDGTs (Dearing Crampton-Flood et al., 2021). The relative abundances of several brGDGTs (i.e., Ib, Ic, IIIa7, IIa7, and 1050d) are indeed significantly higher in the downstream part of the Seine River basin than in the upstream part (p < 0.05, Wilcoxon test; Fig. 3), suggesting in situ brGDGT production in saltwater. Such a saltwater contribution can be visualized by the PCA based on the brGDGT distribution, which shows positive scores for the aforementioned compounds on the first axis (Fig. 4a). This axis is dominated by downstream samples influenced by seawater intrusion in the Seine Estuary (Fig. 4a).
It should be noted that the brGDGT distributions in soils were roughly similar to those observed in downstream-estuarine samples (SPM and river channel sediments) based on the PCA (Fig. 4a). Additionally, no significant differences were observed in the fractional abundances of several brGDGTs (IIIb6, IIb6, IIIc6, IIIa7, IIa7, 1050d, IIIa5, IIIb5, IIIb7, IIIc5, IIc6, and Ia) between soils and downstream samples (Figs. 3 and S4). This similarity in brGDGT distributions may be due to the influx of brGDGTs from the downstream soils into the downstream estuary, as 82 % of the soils were collected downstream (Fig. 1a and Table 1). Hence, it cannot be excluded that the brGDGTs detected in downstream-estuarine samples were at least partly derived from soils of the watershed. Nevertheless, the soil-derived brGDGT contribution to the downstream-estuarine samples is expected to be much lower than the autochthonous one, as the average brGDGT concentration in soils was ca. 3 times lower than the one in downstream-estuarine (i.e., SPM and river channel sediment) samples (Fig. S5a).
In order to further assess whether downstream-estuarine samples could be distinguished from soils, we applied the machine learning model (BigMac) developed by Martínez-Sosa et al. (2023) to our dataset, with isoGDGT and brGDGT data used as input. Most of our samples (SPM, sediments, and soils) were predicted to be lake type, with only one soil sample (soil6) collected at site B predicted to be soil type. This model suggests that, when considered altogether, the isoGDGT and brGDGT distributions are similar in aquatic and soil samples from the Seine Estuary and differ from the soil-type samples described by Martínez-Sosa et al. (2023). Since the BigMac model does not include a river-type or estuary-type category (Martínez-Sosa et al., 2023), further inclusion of both isoGDGT and brGDGT data from global riverine or estuarine samples in the BigMac model may help to enhance predictions for river-type or estuary-type SPM and sediment samples.
The BigMac model distinguishes the type of sample using IIa6 and crenarchaeol as the two most important predicting variables. When accounting for both isoGDGTs and brGDGTs in the Seine River basin, the fractional abundance of crenarchaeol vs. total GDGTs (i.e., isoGDGTs + brGDGTs) varies significantly, whereas that of IIa6 does not differ significantly between the downstream estuary and soils (Fig. S8). Hence, the inclusion of isoGDGTs in the model may greatly reduce the differences between sample types, as we observe significant differences in the fractional abundance of IIa6 when it is calculated vs. total brGDGTs only (Fig. 3). As the BigMac model relies on both the isoGDGT and brGDGT distributions, with no option to use brGDGTs alone, we chose to perform an independent analysis to assess the similarity in brGDGT relative abundance between downstream SPM and sediment samples on the one hand and soils from the Seine River basin on the other hand. This model was developed using the same algorithm (random forest) as that of Martínez-Sosa et al. (2023). Binary classification (downstream estuary vs. soils) was performed based on the fractional abundances of the brGDGTs. The trained model (Fig. S9) indicated distinguishable brGDGT distributions between the downstream-estuary (SPM and sediments) and soil samples, supporting the in situ production of brGDGTs in the downstream estuary. Although most of our soil samples were collected from the downstream estuary and showed similarity with the downstream SPM and sediment samples in PCA and the comparison of fractional abundances, we were able to distinguish their brGDGT compositions using machine learning.
4.1.2 Environmental controls on the brGDGT distribution
As several individual brGDGTs are suggested to be preferentially produced either in the riverine or the estuarine parts of the Seine basin, their distribution might be related to ambient environmental factors. The RDA (performed on SPM samples) highlights the relationships between the available environmental variables (salinity, TN, TOC, water discharge, and temperature) and the relative abundances of brGDGTs. Hierarchical partitioning indicates that salinity is the most important factor influencing the brGDGT distribution (14.97 %) in the Seine River basin (Fig. 5b and Table 2). Salinity is related to the relative abundances of compounds Ib and Ic, 7-methyl brGDGTs, and the late-eluting homologs 1050d and 1036d, which scored negatively on the first axis of the RDA (Fig. 5a). This is in line with the significant positive correlations between salinity and the relative abundances of these compounds (Fig. S10). This trend also supports the assumption about the aquatic production of ring-containing tetramethylated brGDGTs (Ib and Ic) in Svalbard fjords, which was thought to be linked to a salinity change (Dearing Crampton-Flood et al., 2019). The 7-methyl brGDGTs and their late-eluting isomers were also shown to be much more abundant in hypersaline lakes than in those of lower salinity (Wang et al., 2021). Such a salinity-dependent brGDGT composition has previously been interpreted as being due to membrane adaptation to salinity changes or a shift in bacterial community composition (Dearing Crampton-Flood et al., 2019; Wang et al., 2021). Hence, the significant positive correlations between salinity and these compounds in the Seine River basin suggest that brGDGT-producing bacteria have similar physiological mechanisms (i.e., membrane adaptation) to those reported in other aquatic settings (lakes and fjords) and/or that the diversity of these bacteria changes along the river–sea continuum. The salinity proxy (IR6+7me) proposed by Wang et al. (2021) does not show significant correlations with salinity in this study (p > 0.05, Wilcoxon test; Fig. S10). This suggests that the IR6+7me index is relatively insensitive in the Seine Estuary, potentially due to the preferential production of 6-methyl brGDGTs in specific estuarine regions (i.e., KP 255.6–337).
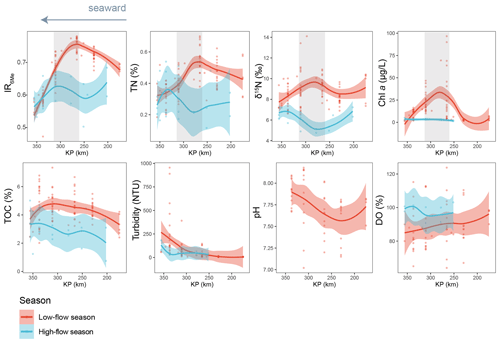
Figure 7Spatio-temporal variations of IR6Me and several environmental factors, including TN (%), δ15N (‰), Chl a (µg L−1), TOC (%), turbidity (NTU), pH, and dissolved oxygen saturation (DO, %). The trends showing variations are based on the locally estimated scatterplot smoothing (LOESS) method with a 95 % confidence interval. KP (kilometric point) represents the distance in kilometers from the city of Paris (KP 0). The dataset is based on SPM. The shaded area highlights a zone (KP 255.6–337) where IR6Me and several environmental parameters co-vary.
Indeed, a significant negative correlation between the salinity and the relative abundance of 6-methyl brGDGTs is observed in the Seine basin (Fig. S10), which suggests that the bacteria producing 6-methyl brGDGTs are preferentially present in the low-salinity area of the estuary. To explore this further, we investigated the spatio-temporal variations of the 6-methyl vs. 5-methyl brGDGT ratio: IR6Me (Fig. 7). High IR6Me values (0.69 ± 0.10) are associated with enhanced in situ production of 6-methyl brGDGTs within the Yenisei River (De Jonge et al., 2015). In the Seine River basin, seasonal variation in IR6me is observed. Specifically, much higher IR6Me values are observed in a specific zone of the estuary (260 < KP < 340) with a low salinity range (1.18 ± 2.71) during low-flow season (Fig. 7), suggesting that 6-methyl brGDGTs are preferentially produced in this zone when water discharge is low. Similarly, the preferential production of 6-methyl brGDGT at low discharge was previously observed in other river systems, including the Amazon River basin (Kirkels et al., 2020; Dearing Crampton-Flood et al., 2021; Bertassoli et al., 2022) as well as the Black and White rivers (Dai et al., 2019). It was suggested that the enhanced 6-methyl brGDGT production at low flows was due to a slow flow velocity and reduced soil mobilization. Although these hypotheses could account for the temporal variation in IR6Me in the Seine River basin, they are unlikely to explain the substantially high IR6Me values in this specific zone. Other environmental variables, such as the dissolved oxygen content (Wu et al., 2021) and pH (De Jonge et al., 2014, 2015), were previously suggested to have a potential influence on 6-methyl brGDGT distributions. Nevertheless, these two environmental factors do not co-vary with IR6Me in the present study and can be ruled out as causes of variation in the 6-methyl brGDGT distribution along the Seine River–sea continuum (Fig. 7). Hence, the production of 6-methyl brGDGTs in this zone of the Seine Estuary has to be triggered by other factors, such as the nutrient concentration.
High nutrient levels were shown to favor the production of 6-methyl versus 5-methyl brGDGTs in the water column in mesocosm experiments (Martínez-Sosa and Tierney, 2019). As the nutrient concentration is higher in the upstream part of the Seine Estuary (Wei et al., 2022), and this zone is characterized by high proportions of agricultural land use (Flipo et al., 2021), the substantial production of 6-methyl brGDGT observed in the aforementioned zone (260 < KP < 340; Fig. 7) during low flows could be attributed to elevated nutrient (particularly nitrogen) levels resulting from intensive agricultural activities. This is supported by the RDA triplot, which shows a strong correlation of TN with the brGDGT distribution in the Seine basin, with the major 6-methyl brGDGTs (i.e., IIa6 and IIIa6) plotting close to TN in the RDA triplot (Fig. 5a). In addition, TN and δ15N are observed to co-vary with IR6Me and to peak in the same zone (260 < KP < 340; Fig. 7) during the low-flow season. Nitrate from sewage effluents and manure are generally enriched in 15N compared to other sources, leading to strongly elevated δ15N values (10 ‰–25 ‰) (Leavitt et al., 2006; Andrisoa et al., 2019). Nutrients, in the form of nitrogen, can be concentrated at low discharge, thus triggering phytoplankton blooms (Romero et al., 2019). Hence, the elevated TN and δ15N signals in a specific zone of the estuary (260 < KP < 340) could be attributed to the increased nitrogen loading and 15N-enriched nitrate uptake by phytoplankton developing intensively during the low-flow season. The much higher chlorophyll a concentration in this zone under low-discharge conditions supports the hypothesis of phytoplankton blooms (Fig. 7). This high phytoplankton biomass might consequently create an environment that accelerates the growth and production of heterotrophic bacteria, which can in turn transform phytoplankton-derived organic matter (Buchan et al., 2014). As the brGDGT-producers were suggested to have a heterotrophic lifestyle (Weijers et al., 2010; Huguet et al., 2017; Blewett et al., 2022), they may transform phytoplankton-derived organic matter and thus participate in N cycling during blooms. Hence, the co-variation of all the parameters (IR6Me, TN, δ15N, and Chl a concentration), which peak in the low-salinity area during the low-flow season, suggests that a low salinity range and high phytoplankton productivity represent favorable conditions for 6-methyl brGDGT production.
4.2 Sources of brGMGTs and environmental controls on their distribution
4.2.1 Sources of brGMGTs
Similarly to the brGDGTs, the brGMGTs can also be produced in situ within the water column and/or sediments (Baxter et al., 2021; Kirkels et al., 2022a). In previous studies, brGMGTs were detected in only some of the soils surrounding the Godavari River basin (India; Kirkels et al., 2022a) and Lake Chala (East Africa; Baxter et al., 2021), suggesting limited brGMGT production in soils in comparison to aquatic settings. Consistently, in the Seine River basin, concentrations of brGMGTs in SPM and sediment samples are significantly higher than those in soils (p < 0.05, Wilcoxon test; Fig. S5), pointing to their predominant aquatic source.
A notable compositional shift in brGMGT distribution is observed along the Seine River basin, as revealed by the separation of riverine, upstream-estuarine, and downstream-estuarine samples in the PCA (Fig. 4b). The relative abundance of the two brGMGTs H1020c and H1034b gradually decreases across the basin (Fig. 6) and is significantly correlated with those of 6-methyl brGDGTs (Fig. S11). As 6-methyl brGDGTs are mainly produced in freshwater in the Seine basin, this suggests that the brGMGTs H1020c and H1034b and 6-methyl brGDGTs have a common freshwater origin and that the mixture of freshwater and marine water along the estuary leads to the dilution of these compounds during downstream transport. H1020c is the dominant brGMGT homologue in SPM from the riverine zone of the Seine and is one of the most abundant brGMGTs in the upstream part of the estuary (Fig. 6). Such a trend was also observed in SPM and riverbed sediments from the upper part of the Godavari River basin, which was attributed to the in situ riverine brGMGT production of this compound (Kirkels et al., 2022a).
The fractional abundances of the homologues H1020a and H1020b gradually increase along the Seine River basin. This is consistent with the higher abundances of H1020a and H1020b previously reported in marine sediments from the Bay of Bengal (Kirkels et al., 2022a). The predominance of these compounds in such samples was attributed to their in situ production in the marine realm. In line with this hypothesis, the relative abundances of brGMGTs H1020a and H1020b positively correlate with the brGDGTs Ib, Ic, IIIa7, IIa7 and 1050d (Fig. S11) in the Seine Estuary, suggesting a similar marine origin.
4.2.2 Environmental controls on the distribution of brGMGTs
The current knowledge on the parameters controlling the brGMGT distributions in the terrestrial and marine realms is still limited, as there is little literature available (Kirkels et al., 2022a). The correlations between the brGDGT and brGMGT relative abundances in the Seine River basin (Fig. S11) suggest that both types of compounds might be derived from overlapping source microorganisms, with common environmental factors controlling their membrane lipid compositions. In the Seine River basin, salinity is shown to be the main environmental parameter influencing the brGMGT distribution, as also observed for brGDGTs (Fig. 5). This is reflected in the significant (p < 0.05) increase in the relative abundances of homologues H1020a and H1020b with salinity and a concomitant significant negative correlation between this parameter and the relative abundances of homologues H1020c and H1034b (p < 0.05, Wilcoxon test; Fig. 8a–d). Nevertheless, the individual effect of TN on brGMGT relative abundances is observed to be much lower compared to that observed for brGDGTs (Fig. 5 and Table 2). This implies that, while they have common controlling factors, such as the salinity, they are also influenced by distinct parameters (i.e., TN), likely indicating distinct sources. This is consistent with a recent study showing that brGDGTs and brGMGTs likely originate from overlapping but not identical origins (Elling et al., 2023).
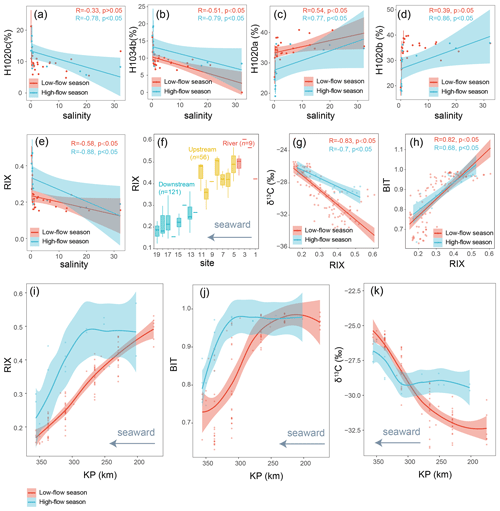
Figure 8(a–e) The correlations of salinity with the relative abundances of brGMGTs and RIX, analyzed through linear regression. The shaded areas represent 95 % confidence intervals. Each vertical error bar indicates the mean ± SD for samples with the same salinity. The dataset is based on SPM. (f) Distribution of RIX across the Seine River basin. Boxes show the upper and lower quartiles of the data, and whiskers show the range of the data. The boxes and whiskers are color coded based on the sample type (river in red, upstream estuary in yellow, and downstream estuary in blue). The center line in each box indicates the median value of the dataset. The dataset is based on SPM and sediments. (g–h) RIX plotted versus δ13C and BIT, with the results of linear regression shown. Shaded areas represent 95 % confidence intervals. (i–k) Spatio-temporal variations in RIX and several other terrestrial proxies, including BIT and δ13C (‰). The trends showing spatio-temporal variations were based on the locally estimated scatterplot smoothing (LOESS) method with a 95 % confidence interval. KP (kilometric point) represents the distance in kilometers from the city of Paris (KP 0). The dataset is based on SPM.
The shift in brGMGT distribution observed across the Seine River basin (Fig. 4) could be due to a change in the diversity of brGMGT-producing bacteria and/or an adaptation of these microorganisms to environmental changes occurring from upstream to downstream. The latter hypothesis seems unlikely, as a physiological adaptation of a given bacterial community would make it difficult to explain why the relative abundances of the three isomers of compound H1020, which share a similar structure, vary differently in response to salinity changes. Hence, a shift in brGMGT-producing bacterial communities across the basin is more likely. Compounds H1020c and H1034b could be predominantly produced by bacteria that preferentially grow in freshwater, and homologues H1020a and H1020b by bacteria that preferentially live in brackish or saltwater.
4.3 Potential implications for brGMGTs as a proxy for riverine runoff in modern systems
The distinct brGMGT distributions in freshwater and saltwater could be used to trace the organic matter (OM) produced upstream all along the Seine basin. To trace such a riverine runoff signal, we propose a new proxy, the Riverine IndeX (RIX), based on the fractional abundances of brGMGTs H1020c and H1034b versus H1020a and H1020b (Eq. 6):
The rationale for using RIX as a riverine runoff signal is that, in freshwater environments, the pool of brGMGTs is dominated by H1020c and H1034b, whereas H1020a and H1020b prevail in saltwater environments. This is further supported by the significant negative correlation between RIX and salinity (Fig. 8e).
Since the other salinity proxies (i.e., ACE and IR6+7Me) have shown positive correlations with salinity in previous studies (Turich and Freeman, 2011; Wang et al., 2021), they were expected to be positively correlated with salinity and negatively correlated with RIX in the Seine River basin. However, the ACE index (Turich and Freeman, 2011) and IR6+7Me (Wang et al., 2021) do not show significant correlations with salinity in the Seine River basin (p > 0.05, Wilcoxon test; Fig. S10), and they show weak but significant relations with RIX (Fig. S13). This could be attributed to the influence of factors other than salinity on these indices (i.e., ACE and IR6+7Me). Indeed, while ACE has been successfully applied in hypersaline systems (Turich and Freeman, 2011), it performs less effectively in certain saline settings due to the complex sources of archaeol and GDGTs (Huguet et al., 2015) and/or the distinct ionization efficiencies of these compounds (He et al., 2020; Wang et al., 2021). Similarly, IR6+7Me may be influenced by the preferential production of 6-methyl brGDGTs in a specific region of the estuary (KP 255.6–337), which is related to the nitrogen nutrient loadings there, as discussed in Sect. 4.1.2. Consequently, only RIX successfully tracks salinity variations in this basin, while ACE and IR6+7Me show a relative insensitivity to salinity. However, quantitatively reconstructing salinity with RIX is an important step forward that warrants further investigation. This requires comparing brGMGT distributions from various aquatic environments (e.g., estuaries and lakes) across salinity gradients.
RIX was calculated for the SPM and sediment samples from the Seine River basin and showed an obvious decreasing trend from upstream to downstream (Fig. 8f). RIX values in river (0.51 ± 0.06, SPM) and upstream-estuarine (0.40 ± 0.07, SPM and sediments) samples are significantly higher than those in soil (0.21 ± 0.13) and downstream-estuarine (0.23 ± 0.06, SPM and sediments) samples. RIX values of around 0.50 could therefore be considered to reflect the riverine endmember, while those below 0.30 could represent the saltwater endmember. A significant overlap in brGMGT distribution between soils and downstream samples was observed (Fig. 4b). This suggests that a portion of the brGMGT signal in the water masses of the Seine may be partially derived from the surrounding soils. In addition, a large variance in the soil brGMGT concentration was observed (Fig. S5b), suggesting that further investigation is needed to better understand the environmental controls on the brGMGT production in soils. As with brGDGTs, we applied a random forest algorithm to distinguish between the brGMGT distributions of downstream-estuary and soil samples. This trained model accurately distinguishes soils from downstream-estuarine samples (Fig. S12), indicating the in situ production of brGMGTs in the downstream estuary. Given the significantly low brGMGT concentrations in soils (p < 0.05, Wilcoxon test; Fig. S5b) and the distinct distributions of brGMGTs in soils and aquatic settings identified through PCA (Fig. 4) and machine learning (Fig. S12), it can be assumed that the impact of soil-derived brGMGTs on the observed RIX signal in the water column of the Seine basin is low.
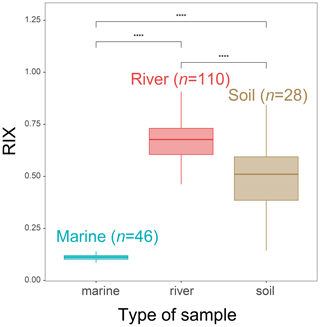
Figure 9RIX values in the soil, SPM and sediment samples from the Godavari River basin (India) and Bay of Bengal sediments (data from Kirkels et al., 2022a). Statistical testing was performed using a Wilcoxon test. Boxes show the upper and lower quartiles of the data, and whiskers show the range of the data. The boxes and whiskers are color coded based on the sample type (river in red, marine in blue, and soil in brown). The center line in each box indicates the median value of the dataset.
In order to test the general applicability of RIX, it was then applied to soil, riverine and marine samples (SPM and sediments) collected in the Godavari River basin and Bay of Bengal (Kirkels et al., 2022a). This site represents the only other river–sea continuum besides the Seine basin for which brGMGT data are presently available. Significant differences in RIX between the soil, SPM and sediment samples from the Godavari River basin are observed (p < 0.05, Wilcoxon test; Fig. 9). RIX values in soils (0.49 ± 0.16) around the Godavari River basin are significantly higher than those in the marine samples (p < 0.05, Wilcoxon test; Fig. 9). Therefore, the potential soil contribution would increase RIX in marine sediments. This is consistent with the observations in the Seine River basin. However, given the distinct distributions for soil and aquatic samples and the lower brGMGT concentration in soils (Kirkels et al., 2022a), the influence of soil-derived brGMGTs on riverine RIX values may be limited. In addition, 96 % of the RIX values in riverine SPM and riverbed sediments from the Godavari basin exceed 0.5, whereas all of the RIX values observed in marine sediments from the Bay of Bengal are below 0.3. This suggests that the RIX cutoff values defined using the samples from the Seine basin may be broadly applicable and valid for other river–sea continuums. This deserves further studies.
Further confirmation of the potential of RIX as a tracer of riverine OM comes from the significant correlations observed between this index and other proxies commonly used for tracing OM sources, i.e., BIT and δ13Corg (p < 0.05, Wilcoxon test; Fig. 8g–h). These proxies show roughly similar spatial and temporal variations in the Seine River basin. It is worth noting that another terrestrial proxy () was not included because it may be ineffective for tracing terrestrial OM in this anthropogenic estuary.
In the low-flow season, RIX and BIT gradually decrease while δ13Corg increases across the basin (Fig. 8i–k). Such trends during the low-discharge periods likely reflect the process of continuous riverine OM dilution caused by the mixing of freshwater and marine water masses (Thibault et al., 2019). The gradual dilution of the riverine OM signal along the Seine River basin could be due to the increase in seawater intrusion, and thus marine-derived OM, at low discharges (Ralston and Geyer, 2019; Kolb et al., 2022). In contrast, during the high-flow season, no such gradual dilution trend is observed. Instead, at high discharges, RIX, BIT, and δ13Corg remain roughly stable from KP 202 to 310.5 before BIT and RIX steeply decrease and δ13Corg increases. This trend can be explained by the fact that, at high flow rates, the limit of saltwater intrusion in the estuary shifts seaward rather than landward, allowing the riverine OM to be flushed further downstream than under low-discharge conditions. After this region, the riverine OM is diluted because of the mixing with marine water masses, as observed during the low-flow season. The trends observed in the Seine Estuary are consistent with previous studies in other regions, showing that terrestrial OM was only effectively transported downstream at high flow rates (Kirkels et al., 2020, 2022b).
Although BIT was successfully used in the Seine River basin as well as in previous studies to trace riverine (terrestrial) OM inputs (Hopmans et al., 2004; Xu et al., 2020), this index can be biased by the in situ production of brGDGTs in the water column and/or sediments (Sinninghe Damsté, 2016; Dearing Crampton-Flood et al., 2019) and the selective degradation of crenarchaeol vs. brGDGTs (Smith et al., 2012). Hence, high BIT values do not necessarily indicate higher contributions of terrestrial OM in some settings (Smith et al., 2012). Unlike the BIT index, which is based on two different families of compounds (isoGDGTs and brGDGTs), RIX is based on four compounds from the same family (brGMGTs), which likely have similar degradation rates and are therefore not influenced by selective degradation. Furthermore, RIX is based on the relative abundances of abundant brGMGTs which are all predominantly produced in aquatic settings, with two of them (H1020c and H1034b) being mainly produced in freshwater and two of them (H1020a and H1020b) mainly in saltwater. Therefore, RIX is based on compounds which are more specifically produced in the two endmembers (freshwater or saltwater), which could avoid the biases encountered with BIT. Overall, our work shows that, in addition to BIT and δ13Corg, RIX successfully captures the spatio-temporal dynamics of riverine OM in the Seine River basin, making this proxy a promising and complementary one for tracing riverine runoff in modern samples.
4.4 Application of RIX to a paleorecord across the upper Paleocene and lower Eocene
To further test the applicability of RIX as a riverine proxy in paleorecords, we calculated RIX and compared this proxy with BIT using the published brGMGT and GDGT data (Sluijs et al., 2020) from Integrated Ocean Drilling Program (IODP) Expedition 302 Hole 4A (see the location in Fig. S14) across the upper Paleocene and lower Eocene. This core is considered to record significant changes in terrestrial inputs (i.e., continental spores and pollen) due to sea level changes over time (Sluijs et al., 2009, 2006), making it a suitable paleorecord for testing runoff proxies.
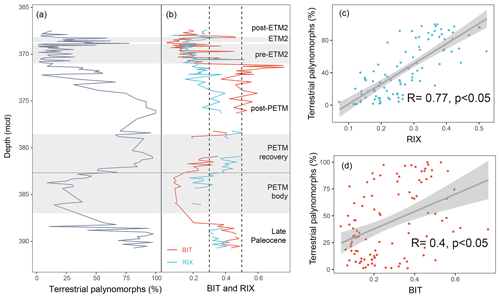
Figure 10Comparison between (a) terrestrial palynomorphs (%) and (b) BIT and RIX across the upper Paleocene and lower Eocene between 391 and 367 m composite depth below seafloor (mcd) from IODP Expedition 302 Hole 4A. Terrestrial palynomorph data are from Sluijs et al. (2006, 2009). RIX and BIT were calculated using data from Sluijs et al. (2020). Gray shading represents Eocene Thermal Maximum 2 (ETM2), the pre-ETM2 interval, and the Paleocene–Eocene Thermal Maximum (PETM). The dashed lines represent cutoff values of RIX (below 0.3 for the marine contribution and above 0.5 for the riverine contribution). Linear regression of RIX (c) and BIT (d) against the terrestrial palynomorphs. The shaded areas represent the 95 % confidence intervals.
In the late Paleocene, the relative abundance of terrestrial palynomorphs (spores and pollen) remains at high levels (Fig. 10a), indicating enhanced terrestrial inputs during this period. This is consistent with the presence of abundant amorphous organic matter during this interval, which is presumed to have originated from terrestrial sources (Sluijs et al., 2006). The enhanced freshwater contribution is also successfully captured by RIX and BIT (Fig. 10b), with values exceeding 0.3 for both proxies.
Palynomorph assemblages from the body of the Paleocene–Eocene Thermal Maximum (PETM) are characterized by substantially fewer terrestrial palynomorphs (Fig. 10a). This indicates a relative decrease in riverine runoff, which is also evidenced by a drop in both RIX and the BIT index (Fig. 10b). Such decreased runoff during the PETM body was previously attributed to a rise in sea level (Sluijs et al., 2006), which has been recorded in many other sites worldwide (Speijer and Morsi, 2002; Harding et al., 2011; Sluijs et al., 2014). During the recovery from the PETM, increased runoff is reflected by the gradual increase in the relative abundance of terrestrial palynomorphs (Fig. 10b), which was interpreted as being a consequence of a drop in sea level (Sluijs et al., 2006). A higher freshwater influx during this period is also indicated by increases in RIX and BIT (Fig. 10b).
The relative abundance of terrestrial palynomorphs decreases after the termination of the PETM (Fig. 10a). Similar decreasing trends are also evident for RIX during the post-PETM period (Fig. 10b). However, BIT levels remain high until the pre-Eocene Thermal Maximum 2 (ETM2) interval, showing distinct patterns compared to the terrestrial palynomorphs and RIX. One hypothesis for this distinction could be the sedimentary in situ production of brGDGTs (Peterse et al., 2009). Indeed, several studies have indicated that elevated BIT values may not accurately represent high levels of terrestrial inputs in marine environments (Smith et al., 2012). In contrast, the similar trends observed for RIX and terrestrial palynomorphs highlight the reliability of RIX as a valuable complementary runoff proxy (Fig. 10b).
At the onset of the pre-ETM2 interval, there is a significant decrease in terrestrial palynomorphs (Fig. 10a). Meanwhile, there is a sharp increase in the proportion of normal marine dinocysts, which was interpreted as a transgressive signal (Sluijs et al., 2008; Willard et al., 2019; Sluijs et al., 2009). Throughout the pre-ETM2 interval, the relative abundance of terrestrial palynomorphs remains consistently low (Fig. 10a). Additionally, the dinocyst assemblages suggest normal marine conditions for this period (Sluijs et al., 2009). These normal marine conditions are also well documented by RIX (Fig. 10b), as most of the samples demonstrate low values (below 0.3). In contrast, the BIT values exhibit some fluctuation, with several samples displaying high values (Fig. 10b). One potential hypothesis for the variability in BIT values could be that it is related to the in situ production of brGDGTs within sedimentary environments.
During the ETM2 interval, the increase in terrestrial palynomorphs suggests increased runoff from the continent to the site (Fig. 10a). The enhanced runoff signal during ETM2 is also supported by the dominance of low-salinity dinocyst taxa and the presence of massive amorphous organic matter (Sluijs et al., 2009; Willard et al., 2019). Both RIX and BIT show increasing trends for this interval (Fig. 10b), indicating that both indices reflect the runoff signal during this period.
Following ETM2, there is a decline in the relative abundance of terrestrial palynomorphs (Fig. 10a), which indicates a shift toward normal marine conditions. This shift is also supported by the dominance of normal marine dinocysts and low concentrations of massive amorphous organic matter (Sluijs et al., 2009; Willard et al., 2019). Additionally, this shift towards normal marine conditions is in line with the lower values (below 0.3) observed for both RIX and BIT (Fig. 10b).
Overall, RIX and BIT exhibit similar trends to terrestrial palynomorphs in the late Paleocene and the PETM, ETM2, and post-ETM2 periods. Both lipid proxies are likely reliable indicators of riverine runoff for these intervals. However, differences between RIX and BIT become more apparent in the post-PETM and pre-ETM2 periods in particular, when normal marine conditions prevail and in situ sedimentary production of brGDGTs may occur, resulting in high BIT values. RIX proves to be particularly valuable for these intervals, as it avoids the possible biases associated with BIT. This indicates that RIX performs better in this core compared with BIT, which is further supported by the observed higher correlation coefficient between RIX and terrestrial palynomorphs (0.77; Fig. 10c) compared with that between BIT and terrestrial palynomorphs (0.4; Fig. 10d).
In this study, the brGDGT and brGMGT concentrations and distributions in soils, SPM, and sediments (n=237) across the Seine River basin were investigated. Higher concentrations and distinct distributions of brGDGTs and brGMGTs in SPM and sediments compared with those in soils imply that both types of compounds can be produced in situ in aquatic settings. The distributions of brGDGTs and brGMGTs are strongly related to the salinity, but only the brGDGT distributions are significantly influenced by nitrogen nutrient loadings. In addition, the covariation of IR6Me, TN, δ15N, and the Chl a concentration within the low-salinity region suggests that riverine (6-methyl) brGDGT production is favored by low-salinity and high-productivity conditions.
In the Seine River basin, salinity correlates positively with H1020a and H1020b and negatively with H1020c and H1034b. This indicates that compounds H1020c and H1034b could be produced by bacteria that preferentially grow in freshwater, while homologues H1020a and H1020b could be produced by bacteria that mainly live in saltwater. Based on this, a novel proxy, the Riverine IndeX (RIX), was proposed to trace riverine OM input. The average value of RIX for the riverine samples is 0.51, which is much higher than that in downstream-estuarine samples (0.23 on average). This suggests that RIX values over 0.5 imply considerable riverine contributions, whereas RIX values below 0.3 indicate higher marine contributions. This cutoff value defined for the Seine River basin also works for the Godavari River basin (India), which implies that this novel proxy based on brGMGTs may be broadly applicable to modern samples. Finally, RIX was applied to a paleorecord across the upper Paleocene and lower Eocene and showed similar trends to terrestrial palynomorphs. Altogether, this showed the potential of RIX as a riverine runoff proxy in the modern period and deep time. Additional studies are needed to test its general applicability in modern samples and paleorecords.
The brGDGT and brGMGT data have been archived in PANGAEA (Zhang et al., 2024; https://doi.org/10.1594/PANGAEA.966245).
The supplement related to this article is available online at: https://doi.org/10.5194/bg-21-2227-2024-supplement.
ZXZ, EP, and AH conceptualized this study. CA conducted LC-MS analyses. JM and AML collected and analyzed wetland soils and mudflat sediments. ZXZ, AH, EP, AT, and EB participated in the fieldwork. ZXZ performed the laboratory work and data analyses and wrote the article in consultation with all the other authors.
The contact author has declared that none of the authors has any competing interests.
Publisher’s note: Copernicus Publications remains neutral with regard to jurisdictional claims made in the text, published maps, institutional affiliations, or any other geographical representation in this paper. While Copernicus Publications makes every effort to include appropriate place names, the final responsibility lies with the authors.
We would like to thank members of the SARTRE project for their assistance during the field campaigns. We appreciate the logistical support from Robert Lafite, Frédéric Azémar, Anaëlle Bernard, and Mahaut Sourzac. We are grateful to Pascal Claquin and Léo Chasselin for their support with water quality measurements and to Micky Tackx for the scientific coordination of the SARTRE project. We would like to thank Vincent Grossi for helpful discussion. We also thank Appy Sluijs for providing the palynological data for IODP Expedition 302 Hole 4A.
This study was funded by GIP Seine-Aval (the MOSAIC, PHARESEE, FEREE, and SARTRE projects) and CNRS/INSU and OFB (the EC2CO RUNTIME project). Zhe-Xuan Zhang's PhD scholarship is funded by the China Scholarship Council (no. 202004910406) and an ASDB from Sorbonne University. This work was supported by CESAM (UIDP/50017/2020, 65UIDB/50017/2020, LA/P/0094/2020).
This paper was edited by Cindy De Jonge and reviewed by three anonymous referees.
Andrisoa, A., Stieglitz, T. C., Rodellas, V., and Raimbault, P.: Primary production in coastal lagoons supported by groundwater discharge and porewater fluxes inferred from nitrogen and carbon isotope signatures, Mar. Chem., 210, 48–60, https://doi.org/10.1016/j.marchem.2019.03.003, 2019.
Baxter, A. J., Hopmans, E. C., Russell, J. M., and Sinninghe Damsté, J. S.: Bacterial GMGTs in East African lake sediments: Their potential as palaeotemperature indicators, Geochim. Cosmochim. Ac., 259, 155–169, https://doi.org/10.1016/j.gca.2019.05.039, 2019.
Baxter, A. J., Peterse, F., Verschuren, D., and Sinninghe Damsté, J. S.: Anoxic in situ production of bacterial GMGTs in the water column and surficial bottom sediments of a meromictic tropical crater lake: Implications for lake paleothermometry, Geochim. Cosmochim. Ac., 306, 171–188, https://doi.org/10.1016/j.gca.2021.05.015, 2021.
Bertassoli, D. J., Häggi, C., Chiessi, C. M., Schefuß, E., Hefter, J., Akabane, T. K., and Sawakuchi, A. O.: Controls on the distributions of GDGTs and n-alkane isotopic compositions in sediments of the Amazon River Basin, Chem. Geol., 594, 120777, https://doi.org/10.1016/j.chemgeo.2022.120777, 2022.
Bijl, P. K., Frieling, J., Cramwinckel, M. J., Boschman, C., Sluijs, A., and Peterse, F.: Maastrichtian–Rupelian paleoclimates in the southwest Pacific – a critical re-evaluation of biomarker paleothermometry and dinoflagellate cyst paleoecology at Ocean Drilling Program Site 1172, Clim. Past, 17, 2393–2425, https://doi.org/10.5194/cp-17-2393-2021, 2021.
Blewett, J., Elling, F. J., Naafs, B. D. A., Kattein, L., Evans, T. W., Lauretano, V., Gallego-Sala, A. V., Pancost, R. D., and Pearson, A.: Metabolic and ecological controls on the stable carbon isotopic composition of archaeal (isoGDGT and BDGT) and bacterial (brGDGT) lipids in wetlands and lignites, Geochim. Cosmochim. Ac., 320, 1–25, https://doi.org/10.1016/j.gca.2021.12.023, 2022.
Buchan, A., LeCleir, G. R., Gulvik, C. A., and González, J. M.: Master recyclers: features and functions of bacteria associated with phytoplankton blooms, Nat. Rev. Microbiol., 12, 686–698, https://doi.org/10.1038/nrmicro3326, 2014.
Cao, J., Lian, E., Yang, S., Ge, H., Jin, X., He, J., and Jia, G.: The distribution of intact polar lipid-derived branched tetraethers along a freshwater-seawater pH gradient in coastal East China Sea, Chem. Geol., 596, 120808, https://doi.org/10.1016/j.chemgeo.2022.120808, 2022.
Chen, Y., Zheng, F., Yang, H., Yang, W., Wu, R., Liu, X., Liang, H., Chen, H., Pei, H., Zhang, C., Pancost, R. D., and Zeng, Z.: The production of diverse brGDGTs by an Acidobacterium providing a physiological basis for paleoclimate proxies, Geochim. Cosmochim. Ac., 337, 155–165, https://doi.org/10.1016/j.gca.2022.08.033, 2022.
Coffinet, S., Huguet, A., Bergonzini, L., Pedentchouk, N., Williamson, D., Anquetil, C., Gałka, M., Kołaczek, P., Karpińska-Kołaczek, M., Majule, A., Laggoun-Défarge, F., Wagner, T., and Derenne, S.: Impact of climate change on the ecology of the Kyambangunguru crater marsh in southwestern Tanzania during the Late Holocene, Quaternary Sci. Rev., 196, 100–117, https://doi.org/10.1016/j.quascirev.2018.07.038, 2018.
Cramwinckel, M. J., Huber, M., Kocken, I. J., Agnini, C., Bijl, P. K., Bohaty, S. M., Frieling, J., Goldner, A., Hilgen, F. J., Kip, E. L., Peterse, F., van der Ploeg, R., Röhl, U., Schouten, S., and Sluijs, A.: Synchronous tropical and polar temperature evolution in the Eocene, Nature, 559, 382–386, https://doi.org/10.1038/s41586-018-0272-2, 2018.
Dai, G., Zhu, E., Liu, Z., Wang, Y., Zhu, S., Wang, S., Ma, T., Jia, J., Wang, X., Hou, S., Fu, P., Peterse, F., and Feng, X.: Compositional Characteristics of Fluvial Particulate Organic Matter Exported From the World's Largest Alpine Wetland, J. Geophys. Res.-Biogeo., 124, 2709–2727, https://doi.org/10.1029/2019JG005231, 2019.
Dearing Crampton-Flood, E., Peterse, F., and Sinninghe Damsté, J. S.: Production of branched tetraethers in the marine realm: Svalbard fjord sediments revisited, Org. Geochem., 138, 103907, https://doi.org/10.1016/j.orggeochem.2019.103907, 2019.
Dearing Crampton-Flood, E., van der Weijst, C. M., van der Molen, G., Bouquet, M., Yedema, Y., Donders, T. H., Sangiorgi, F., Sluijs, A., Damsté, J. S. S., and Peterse, F.: Identifying marine and freshwater overprints on soil-derived branched GDGT temperature signals in Pliocene Mississippi and Amazon River fan sediments, Org. Geochem., 154, 104200, https://doi.org/10.1016/j.orggeochem.2021.104200, 2021.
De Jonge, C., Hopmans, E. C., Stadnitskaia, A., Rijpstra, W. I. C., Hofland, R., Tegelaar, E., and Sinninghe Damsté, J. S.: Identification of novel penta- and hexamethylated branched glycerol dialkyl glycerol tetraethers in peat using HPLC–MS2, GC–MS and GC–SMB-MS, Org. Geochem., 54, 78–82, https://doi.org/10.1016/j.orggeochem.2012.10.004, 2013.
De Jonge, C., Hopmans, E. C., Zell, C. I., Kim, J.-H., Schouten, S., and Sinninghe Damsté, J. S.: Occurrence and abundance of 6-methyl branched glycerol dialkyl glycerol tetraethers in soils: Implications for palaeoclimate reconstruction, Geochim. Cosmochim. Ac., 141, 97–112, https://doi.org/10.1016/j.gca.2014.06.013, 2014.
De Jonge, C., Stadnitskaia, A., Hopmans, E. C., Cherkashov, G., Fedotov, A., Streletskaya, I. D., Vasiliev, A. A., and Sinninghe Damsté, J. S.: Drastic changes in the distribution of branched tetraether lipids in suspended matter and sediments from the Yenisei River and Kara Sea (Siberia): Implications for the use of brGDGT-based proxies in coastal marine sediments, Geochim. Cosmochim. Ac., 165, 200–225, https://doi.org/10.1016/j.gca.2015.05.044, 2015.
Ding, S., Schwab, V. F., Ueberschaar, N., Roth, V.-N., Lange, M., Xu, Y., Gleixner, G., and Pohnert, G.: Identification of novel 7-methyl and cyclopentanyl branched glycerol dialkyl glycerol tetraethers in lake sediments, Org. Geochem., 102, 52–58, https://doi.org/10.1016/j.orggeochem.2016.09.009, 2016.
Druine, F.: Flux sédimentaire en estuaire de Seine: quantification et variabilité multi-échelle sur la base de mesure de turbidité (réseau SYNAPSES), PhD thesis, Normandie Université, Institut français de recherche pour l'exploitation de la mer, 2018.
Druine, F., Verney, R., Deloffre, J., Lemoine, J.-P., Chapalain, M., Landemaine, V., and Lafite, R.: In situ high frequency long term measurements of suspended sediment concentration in turbid estuarine system (Seine Estuary, France): Optical turbidity sensors response to suspended sediment characteristics, Mar. Geol., 400, 24–37, https://doi.org/10.1016/j.margeo.2018.03.003, 2018.
Elling, F. J., Kattein, L., Naafs, B. D. A., Lauretano, V., and Pearson, A.: Heterotrophic origin and diverse sources of branched glycerol monoalkyl glycerol tetraethers (brGMGTs) in peats and lignites, Org. Geochem., 104558, https://doi.org/10.1016/j.orggeochem.2023.104558, 2023.
Flipo, N., Lestel, L., Labadie, P., Meybeck, M., and Garnier, J.: Trajectories of the Seine River Basin, in: The Seine River Basin, edited by: Flipo, N., Labadie, P., and Lestel, L., Springer International Publishing, Cham, 1–28, https://doi.org/10.1007/698_2019_437, 2021.
Freymond, C. V., Peterse, F., Fischer, L. V., Filip, F., Giosan, L., and Eglinton, T. I.: Branched GDGT signals in fluvial sediments of the Danube River basin: Method comparison and longitudinal evolution, Org. Geochem., 103, 88–96, https://doi.org/10.1016/j.orggeochem.2016.11.002, 2017.
Halamka, T. A., Raberg, J. H., McFarlin, J. M., Younkin, A. D., Mulligan, C., Liu, X.-L., and Kopf, S. H.: Production of diverse brGDGTs by Acidobacterium Solibacter usitatus in response to temperature, pH, and O2 provides a culturing perspective on brGDGT proxies and biosynthesis, Geobiology, 21, 102–118, https://doi.org/10.1111/gbi.12525, 2023.
Harding, I. C., Charles, A. J., Marshall, J. E. A., Pälike, H., Roberts, A. P., Wilson, P. A., Jarvis, E., Thorne, R., Morris, E., Moremon, R., Pearce, R. B., and Akbari, S.: Sea-level and salinity fluctuations during the Paleocene–Eocene thermal maximum in Arctic Spitsbergen, Earth Planet. Sc. Lett., 303, 97–107, https://doi.org/10.1016/j.epsl.2010.12.043, 2011.
Harning, D. J., Curtin, L., Geirsdóttir, Á., D'Andrea, W. J., Miller, G. H., and Sepúlveda, J.: Lipid Biomarkers Quantify Holocene Summer Temperature and Ice Cap Sensitivity in Icelandic Lakes, Geophys. Res. Lett., 47, e2019GL085728, https://doi.org/10.1029/2019GL085728, 2020.
He, Y., Wang, H., Meng, B., Liu, H., Zhou, A., Song, M., Kolpakova, M., Krivonogov, S., Liu, W., and Liu, Z.: Appraisal of alkenone- and archaeal ether-based salinity indicators in mid-latitude Asian lakes, Earth Planet. Sc. Lett., 538, 116236, https://doi.org/10.1016/j.epsl.2020.116236, 2020.
Hopmans, E. C., Weijers, J. W. H., Schefuß, E., Herfort, L., Sinninghe Damsté, J. S., and Schouten, S.: A novel proxy for terrestrial organic matter in sediments based on branched and isoprenoid tetraether lipids, Earth Planet. Sc. Lett., 224, 107–116, https://doi.org/10.1016/j.epsl.2004.05.012, 2004.
Hopmans, E. C., Schouten, S., and Sinninghe Damsté, J. S.: The effect of improved chromatography on GDGT-based palaeoproxies, Org. Geochem., 93, 1–6, https://doi.org/10.1016/j.orggeochem.2015.12.006, 2016.
Huguet, A., Grossi, V., Belmahdi, I., Fosse, C., and Derenne, S.: Archaeal and bacterial tetraether lipids in tropical ponds with contrasting salinity (Guadeloupe, French West Indies): Implications for tetraether-based environmental proxies, Org. Geochem., 83–84, 158–169, https://doi.org/10.1016/j.orggeochem.2015.02.010, 2015.
Huguet, A., Meador, T. B., Laggoun-Défarge, F., Könneke, M., Wu, W., Derenne, S., and Hinrichs, K.-U.: Production rates of bacterial tetraether lipids and fatty acids in peatland under varying oxygen concentrations, Geochim. Cosmochim. Ac., 203, 103–116, https://doi.org/10.1016/j.gca.2017.01.012, 2017.
Huguet, A., Coffinet, S., Roussel, A., Gayraud, F., Anquetil, C., Bergonzini, L., Bonanomi, G., Williamson, D., Majule, A., and Derenne, S.: Evaluation of 3-hydroxy fatty acids as a pH and temperature proxy in soils from temperate and tropical altitudinal gradients, Org. Geochem., 129, 1–13, https://doi.org/10.1016/j.orggeochem.2019.01.002, 2019.
Huguet, C., Hopmans, E. C., Febo-Ayala, W., Thompson, D. H., Sinninghe Damsté, J. S., and Schouten, S.: An improved method to determine the absolute abundance of glycerol dibiphytanyl glycerol tetraether lipids, Org. Geochem., 37, 1036–1041, https://doi.org/10.1016/j.orggeochem.2006.05.008, 2006.
Huguet, C., de Lange, G. J., Gustafsson, Ö., Middelburg, J. J., Sinninghe Damsté, J. S., and Schouten, S.: Selective preservation of soil organic matter in oxidized marine sediments (Madeira Abyssal Plain), Geochim. Cosmochim. Ac., 72, 6061–6068, https://doi.org/10.1016/j.gca.2008.09.021, 2008.
Kang, M., He, J., and Jia, G.: Evaluation of heterocyte glycolipids with a hexose sugar moiety for tracing terrestrial organic matter in the South China Sea, Chem. Geol., 635, 121604, https://doi.org/10.1016/j.chemgeo.2023.121604, 2023.
Kim, J.-H., Ludwig, W., Buscail, R., Dorhout, D., and Sinninghe Damsté, J. S.: Tracing tetraether lipids from source to sink in the Rhône River system (NW Mediterranean), Front. Earth Sci., 3, https://doi.org/10.3389/feart.2015.00022, 2015.
Kirkels, F. M. S. A., Ponton, C., Galy, V., West, A. J., Feakins, S. J., and Peterse, F.: From Andes to Amazon: Assessing Branched Tetraether Lipids as Tracers for Soil Organic Carbon in the Madre de Dios River System, J. Geophys. Res.-Biogeo., 125, e2019JG005270, https://doi.org/10.1029/2019JG005270, 2020.
Kirkels, F. M. S. A., Usman, M. O., and Peterse, F.: Distinct sources of bacterial branched GMGTs in the Godavari River basin (India) and Bay of Bengal sediments, Org. Geochem., 167, 104405, https://doi.org/10.1016/j.orggeochem.2022.104405, 2022a.
Kirkels, F. M. S. A., Zwart, H. M., Usman, M. O., Hou, S., Ponton, C., Giosan, L., Eglinton, T. I., and Peterse, F.: From soil to sea: sources and transport of organic carbon traced by tetraether lipids in the monsoonal Godavari River, India, Biogeosciences, 19, 3979–4010, https://doi.org/10.5194/bg-19-3979-2022, 2022b.
Kolb, P., Zorndt, A., Burchard, H., Gräwe, U., and Kösters, F.: Modelling the impact of anthropogenic measures on saltwater intrusion in the Weser estuary, Ocean Sci., 18, 1725–1739, https://doi.org/10.5194/os-18-1725-2022, 2022.
Kou, Q., Zhu, L., Ju, J., Wang, J., Xu, T., Li, C., and Ma, Q.: Influence of salinity on glycerol dialkyl glycerol tetraether-based indicators in Tibetan Plateau lakes: Implications for paleotemperature and paleosalinity reconstructions, Palaeogeogr. Palaeocl., 601, 111127, https://doi.org/10.1016/j.palaeo.2022.111127, 2022.
Lai, J., Zou, Y., Zhang, J., and Peres-Neto, P. R.: Generalizing hierarchical and variation partitioning in multiple regression and canonical analyses using the rdacca.hp R package, Methods Ecol. Evol., 13, 782–788, https://doi.org/10.1111/2041-210X.13800, 2022.
Lamb, A. L., Wilson, G. P., and Leng, M. J.: A review of coastal palaeoclimate and relative sea-level reconstructions using δ13C and ratios in organic material, Earth-Sci. Rev., 75, 29–57, https://doi.org/10.1016/j.earscirev.2005.10.003, 2006.
Lattaud, J., Kim, J.-H., De Jonge, C., Zell, C., Sinninghe Damsté, J. S., and Schouten, S.: The C32 alkane-1,15-diol as a tracer for riverine input in coastal seas, Geochim. Cosmochim. Ac., 202, 146–158, https://doi.org/10.1016/j.gca.2016.12.030, 2017.
Leavitt, P. R., Brock, C. S., Ebel, C., and Patoine, A.: Landscape-scale effects of urban nitrogen on a chain of freshwater lakes in central North America, Limnol. Oceanogr., 51, 2262–2277, https://doi.org/10.4319/lo.2006.51.5.2262, 2006.
Liu, X.-L., Summons, R. E., and Hinrichs, K.-U.: Extending the known range of glycerol ether lipids in the environment: structural assignments based on tandem mass spectral fragmentation patterns, Rapid Commun. Mass Sp., 26, 2295–2302, https://doi.org/10.1002/rcm.6355, 2012.
Lundberg, S. M., Erion, G., Chen, H., DeGrave, A., Prutkin, J. M., Nair, B., Katz, R., Himmelfarb, J., Bansal, N., and Lee, S.-I.: From local explanations to global understanding with explainable AI for trees, Nat. Mach. Intell., 2, 56–67, https://doi.org/10.1038/s42256-019-0138-9, 2020.
Martínez-Sosa, P. and Tierney, J. E.: Lacustrine brGDGT response to microcosm and mesocosm incubations, Org. Geochem., 127, 12–22, https://doi.org/10.1016/j.orggeochem.2018.10.011, 2019.
Martínez-Sosa, P., Tierney, J. E., Stefanescu, I. C., Dearing Crampton-Flood, E., Shuman, B. N., and Routson, C.: A global Bayesian temperature calibration for lacustrine brGDGTs, Geochim. Cosmochim. Ac., 305, 87–105, https://doi.org/10.1016/j.gca.2021.04.038, 2021.
Martínez-Sosa, P., Tierney, J. E., Pérez-Angel, L. C., Stefanescu, I. C., Guo, J., Kirkels, F., Sepúlveda, J., Peterse, F., Shuman, B. N., and Reyes, A. V.: Development and Application of the Branched and Isoprenoid GDGT Machine Learning Classification Algorithm (BIGMaC) for Paleoenvironmental Reconstruction, Paleoceanogr. Paleocl., 38, e2023PA004611, https://doi.org/10.1029/2023PA004611, 2023.
Morii, H., Eguchi, T., Nishihara, M., Kakinuma, K., König, H., and Koga, Y.: A novel ether core lipid with H-shaped C80-isoprenoid hydrocarbon chain from the hyperthermophilic methanogen Methanothermus fervidus, Biochim. Biophys. Acta, 1390, 339–345, https://doi.org/10.1016/S0005-2760(97)00183-5, 1998.
Naafs, B. D. A., Inglis, G. N., Zheng, Y., Amesbury, M. J., Biester, H., Bindler, R., Blewett, J., Burrows, M. A., del Castillo Torres, D., Chambers, F. M., Cohen, A. D., Evershed, R. P., Feakins, S. J., Gałka, M., Gallego-Sala, A., Gandois, L., Gray, D. M., Hatcher, P. G., Honorio Coronado, E. N., Hughes, P. D. M., Huguet, A., Könönen, M., Laggoun-Défarge, F., Lähteenoja, O., Lamentowicz, M., Marchant, R., McClymont, E., Pontevedra-Pombal, X., Ponton, C., Pourmand, A., Rizzuti, A. M., Rochefort, L., Schellekens, J., De Vleeschouwer, F., and Pancost, R. D.: Introducing global peat-specific temperature and pH calibrations based on brGDGT bacterial lipids, Geochim. Cosmochim. Ac., 208, 285–301, https://doi.org/10.1016/j.gca.2017.01.038, 2017.
Naafs, B. D. A., McCormick, D., Inglis, G. N., and Pancost, R. D.: Archaeal and bacterial H-GDGTs are abundant in peat and their relative abundance is positively correlated with temperature, Geochim. Cosmochim. Ac., 227, 156–170, https://doi.org/10.1016/j.gca.2018.02.025, 2018.
Pedregosa, F., Varoquaux, G., Gramfort, A., Michel, V., Thirion, B., Grisel, O., Blondel, M., Prettenhofer, P., Weiss, R., Dubourg, V., Vanderplas, J., Passos, A., Cournapeau, D., Brucher, M., Perrot, M., and Duchesnay, É.: Scikit-learn: Machine Learning in Python, J. Mach. Learn. Res., 12, 2825–2830, 2011.
Peterse, F., Kim, J.-H., Schouten, S., Kristensen, D. K., Koç, N., and Sinninghe Damsté, J. S.: Constraints on the application of the MBT/CBT palaeothermometer at high latitude environments (Svalbard, Norway), Org. Geochem., 40, 692–699, https://doi.org/10.1016/j.orggeochem.2009.03.004, 2009.
Raberg, J. H., Harning, D. J., Crump, S. E., de Wet, G., Blumm, A., Kopf, S., Geirsdóttir, Á., Miller, G. H., and Sepúlveda, J.: Revised fractional abundances and warm-season temperatures substantially improve brGDGT calibrations in lake sediments, Biogeosciences, 18, 3579–3603, https://doi.org/10.5194/bg-18-3579-2021, 2021.
Raberg, J. H., Miller, G. H., Geirsdóttir, Á., and Sepúlveda, J.: Near-universal trends in brGDGT lipid distributions in nature, Sci. Adv., 8, eabm7625, https://doi.org/10.1126/sciadv.abm7625, 2022.
Ralston, D. K. and Geyer, W. R.: Response to Channel Deepening of the Salinity Intrusion, Estuarine Circulation, and Stratification in an Urbanized Estuary, J. Geophys. Res.-Oceans, 124, 4784–4802, https://doi.org/10.1029/2019JC015006, 2019.
Romero, E., Le Gendre, R., Garnier, J., Billen, G., Fisson, C., Silvestre, M., and Riou, P.: Long-term water quality in the lower Seine: Lessons learned over 4 decades of monitoring, Environ. Sci. Policy, 58, 141–154, https://doi.org/10.1016/j.envsci.2016.01.016, 2016.
Romero, E., Garnier, J., Billen, G., Ramarson, A., Riou, P., and Le Gendre, R.: Modeling the biogeochemical functioning of the Seine estuary and its coastal zone: Export, retention, and transformations, Limnol. Oceanogr., 64, 895–912, https://doi.org/10.1002/lno.11082, 2019.
Russell, J. M., Hopmans, E. C., Loomis, S. E., Liang, J., and Sinninghe Damsté, J. S.: Distributions of 5- and 6-methyl branched glycerol dialkyl glycerol tetraethers (brGDGTs) in East African lake sediment: Effects of temperature, pH, and new lacustrine paleotemperature calibrations, Org. Geochem., 117, 56–69, https://doi.org/10.1016/j.orggeochem.2017.12.003, 2018.
Ryba, S. A. and Burgess, R. M.: Effects of sample preparation on the measurement of organic carbon, hydrogen, nitrogen, sulfur, and oxygen concentrations in marine sediments, Chemosphere, 48, 139–147, https://doi.org/10.1016/S0045-6535(02)00027-9, 2002.
Schouten, S., Baas, M., Hopmans, E. C., Reysenbach, A.-L., and Damsté, J. S. S.: Tetraether membrane lipids of Candidatus “Aciduliprofundum boonei”, a cultivated obligate thermoacidophilic euryarchaeote from deep-sea hydrothermal vents, Extremophiles, 12, 119–124, 2008.
Schouten, S., Hopmans, E. C., and Sinninghe Damsté, J. S.: The organic geochemistry of glycerol dialkyl glycerol tetraether lipids: A review, Org. Geochem., 54, 19–61, https://doi.org/10.1016/j.orggeochem.2012.09.006, 2013.
Sinninghe Damsté, J. S.: Spatial heterogeneity of sources of branched tetraethers in shelf systems: The geochemistry of tetraethers in the Berau River delta (Kalimantan, Indonesia), Geochim. Cosmochim. Ac., 186, 13–31, https://doi.org/10.1016/j.gca.2016.04.033, 2016.
Sinninghe Damsté, J. S., Rijpstra, W. I. C., Hopmans, E. C., Weijers, J. W. H., Foesel, B. U., Overmann, J., and Dedysh, S. N.: 13,16-Dimethyl octacosanedioic acid (iso-diabolic acid), a common membrane-spanning lipid of Acidobacteria subdivisions 1 and 3, Appl. Environ. Microbiol., 77, 4147–4154, https://doi.org/10.1128/AEM.00466-11, 2011.
Sluijs, A., Schouten, S., Pagani, M., Woltering, M., Brinkhuis, H., Damsté, J. S. S., Dickens, G. R., Huber, M., Reichart, G.-J., Stein, R., Matthiessen, J., Lourens, L. J., Pedentchouk, N., Backman, J., and Moran, K.: Subtropical Arctic Ocean temperatures during the Palaeocene/Eocene thermal maximum, Nature, 441, 610–613, https://doi.org/10.1038/nature04668, 2006.
Sluijs, A., Brinkhuis, H., Crouch, E. M., John, C. M., Handley, L., Munsterman, D., Bohaty, S. M., Zachos, J. C., Reichart, G.-J., Schouten, S., Pancost, R. D., Damsté, J. S. S., Welters, N. L. D., Lotter, A. F., and Dickens, G. R.: Eustatic variations during the Paleocene-Eocene greenhouse world, Paleoceanography, 23, PA4216, https://doi.org/10.1029/2008PA001615, 2008.
Sluijs, A., Schouten, S., Donders, T. H., Schoon, P. L., Röhl, U., Reichart, G.-J., Sangiorgi, F., Kim, J.-H., Sinninghe Damsté, J. S., and Brinkhuis, H.: Warm and wet conditions in the Arctic region during Eocene Thermal Maximum 2, Nat. Geosci., 2, 777–780, https://doi.org/10.1038/ngeo668, 2009.
Sluijs, A., van Roij, L., Harrington, G. J., Schouten, S., Sessa, J. A., LeVay, L. J., Reichart, G.-J., and Slomp, C. P.: Warming, euxinia and sea level rise during the Paleocene–Eocene Thermal Maximum on the Gulf Coastal Plain: implications for ocean oxygenation and nutrient cycling, Clim. Past, 10, 1421–1439, https://doi.org/10.5194/cp-10-1421-2014, 2014.
Sluijs, A., Frieling, J., Inglis, G. N., Nierop, K. G. J., Peterse, F., Sangiorgi, F., and Schouten, S.: Late Paleocene–early Eocene Arctic Ocean sea surface temperatures: reassessing biomarker paleothermometry at Lomonosov Ridge, Clim. Past, 16, 2381–2400, https://doi.org/10.5194/cp-16-2381-2020, 2020.
Smith, R. W., Bianchi, T. S., and Li, X.: A re-evaluation of the use of branched GDGTs as terrestrial biomarkers: Implications for the BIT Index, Geochim. Cosmochim. Ac., 80, 14–29, https://doi.org/10.1016/j.gca.2011.11.025, 2012.
Speijer, R. P. and Morsi, A.-M. M.: Ostracode turnover and sea-level changes associated with the Paleocene-Eocene thermal maximum, Geology, 30, 23–26, https://doi.org/10.1130/0091-7613(2002)030<0023:OTASLC>2.0.CO;2, 2002.
Strickland, J. D. H. and Parsons, T. R.: A Practical Handbook of Seawater Analysis, edited by: Stevenson, J. C., 2nd Edn., Fisheries Research Board of Canada, https://doi.org/10.25607/OBP-1791, 1972.
Tang, X., Naafs, B. D. A., Pancost, R. D., Liu, Z., Fan, T., and Zheng, Y.: Exploring the Influences of Temperature on “H-Shaped” Glycerol Dialkyl Glycerol Tetraethers in a Stratigraphic Context: Evidence From Two Peat Cores Across the Late Quaternary, Front. Earth Sci., 8, https://doi.org/10.3389/feart.2020.541685, 2021.
Thibault, A.: Dynamique de la matière organique dans la Seine: approche globale et moléculaire, PhD thesis, Sorbonne Université, https://theses.hal.science/tel-02503219 (last access: 23 April 2024), 2018.
Thibault, A., Derenne, S., Parlanti, E., Anquetil, C., Sourzac, M., Budzinski, H., Fuster, L., Laverman, A., Roose-Amsaleg, C., Viollier, E., and Huguet, A.: Dynamics of organic matter in the Seine Estuary (France): Bulk and structural approaches, Mar. Chem., 212, 108–119, https://doi.org/10.1016/j.marchem.2019.04.007, 2019.
Tierney, J. E. and Russell, J. M.: Distributions of branched GDGTs in a tropical lake system: Implications for lacustrine application of the MBT/CBT paleoproxy, Org. Geochem., 40, 1032–1036, https://doi.org/10.1016/j.orggeochem.2009.04.014, 2009.
Tierney, J. E., Russell, J. M., Eggermont, H., Hopmans, E. C., Verschuren, D., and Sinninghe Damsté, J. S.: Environmental controls on branched tetraether lipid distributions in tropical East African lake sediments, Geochim. Cosmochim. Ac., 74, 4902–4918, https://doi.org/10.1016/j.gca.2010.06.002, 2010.
Turich, C. and Freeman, K. H.: Archaeal lipids record paleosalinity in hypersaline systems, Org. Geochem., 42, 1147–1157, https://doi.org/10.1016/j.orggeochem.2011.06.002, 2011.
Véquaud, P., Thibault, A., Derenne, S., Anquetil, C., Collin, S., Contreras, S., Nottingham, A. T., Sabatier, P., Werne, J. P., and Huguet, A.: FROG: A global machine-learning temperature calibration for branched GDGTs in soils and peats, Geochim. Cosmochim. Ac., 318, 468–494, https://doi.org/10.1016/j.gca.2021.12.007, 2022.
Wang, H., An, Z., Lu, H., Zhao, Z., and Liu, W.: Calibrating bacterial tetraether distributions towards in situ soil temperature and application to a loess-paleosol sequence, Quaternary Sci. Rev., 231, 106172, https://doi.org/10.1016/j.quascirev.2020.106172, 2020.
Wang, H., Liu, W., He, Y., Zhou, A., Zhao, H., Liu, H., Cao, Y., Hu, J., Meng, B., Jiang, J., Kolpakova, M., Krivonogov, S., and Liu, Z.: Salinity-controlled isomerization of lacustrine brGDGTs impacts the associated MBT5ME' terrestrial temperature index, Geochim. Cosmochim. Ac., 305, 33–48, https://doi.org/10.1016/j.gca.2021.05.004, 2021.
Wei, X., Garnier, J., Thieu, V., Passy, P., Le Gendre, R., Billen, G., Akopian, M., and Laruelle, G. G.: Nutrient transport and transformation in macrotidal estuaries of the French Atlantic coast: a modeling approach using the Carbon-Generic Estuarine Model, Biogeosciences, 19, 931–955, https://doi.org/10.5194/bg-19-931-2022, 2022.
Weijers, J. W. H., Schouten, S., van den Donker, J. C., Hopmans, E. C., and Sinninghe Damsté, J. S.: Environmental controls on bacterial tetraether membrane lipid distribution in soils, Geochim. Cosmochim. Ac., 71, 703–713, https://doi.org/10.1016/j.gca.2006.10.003, 2007.
Weijers, J. W. H., Wiesenberg, G. L. B., Bol, R., Hopmans, E. C., and Pancost, R. D.: Carbon isotopic composition of branched tetraether membrane lipids in soils suggest a rapid turnover and a heterotrophic life style of their source organism(s), Biogeosciences, 7, 2959–2973, https://doi.org/10.5194/bg-7-2959-2010, 2010.
Willard, D. A., Donders, T. H., Reichgelt, T., Greenwood, D. R., Sangiorgi, F., Peterse, F., Nierop, K. G. J., Frieling, J., Schouten, S., and Sluijs, A.: Arctic vegetation, temperature, and hydrology during Early Eocene transient global warming events, Global Planet. Change, 178, 139–152, https://doi.org/10.1016/j.gloplacha.2019.04.012, 2019.
Wu, J., Yang, H., Pancost, R. D., Naafs, B. D. A., Qian, S., Dang, X., Sun, H., Pei, H., Wang, R., Zhao, S., and Xie, S.: Variations in dissolved O2 in a Chinese lake drive changes in microbial communities and impact sedimentary GDGT distributions, Chem. Geol., 579, 120348, https://doi.org/10.1016/j.chemgeo.2021.120348, 2021.
Xie, S., Liu, X.-L., Schubotz, F., Wakeham, S. G., and Hinrichs, K.-U.: Distribution of glycerol ether lipids in the oxygen minimum zone of the Eastern Tropical North Pacific Ocean, Org. Geochem., 71, 60–71, https://doi.org/10.1016/j.orggeochem.2014.04.006, 2014.
Xu, S., Zhang, Z., Jia, G., Yu, K., Lei, F., and Zhu, X.: Controlling factors and environmental significance of BIT and δ13C of sedimentary GDGTs from the Pearl River Estuary, China over recent decades, Estuar. Coast. Shelf S., 233, 106534, https://doi.org/10.1016/j.ecss.2019.106534, 2020.
Yedema, Y. W., Sangiorgi, F., Sluijs, A., Sinninghe Damsté, J. S., and Peterse, F.: The dispersal of fluvially discharged and marine, shelf-produced particulate organic matter in the northern Gulf of Mexico, Biogeosciences, 20, 663–686, https://doi.org/10.5194/bg-20-663-2023, 2023.
Zell, C., Kim, J.-H., Moreira-Turcq, P., Abril, G., Hopmans, E. C., Bonnet, M.-P., Sobrinho, R. L., and Damsté, J. S. S.: Disentangling the origins of branched tetraether lipids and crenarchaeol in the lower Amazon River: Implications for GDGT-based proxies, Limnol. Oceanogr., 58, 343–353, https://doi.org/10.4319/lo.2013.58.1.0343, 2013.
Zell, C., Kim, J.-H., Balsinha, M., Dorhout, D., Fernandes, C., Baas, M., and Sinninghe Damsté, J. S.: Transport of branched tetraether lipids from the Tagus River basin to the coastal ocean of the Portuguese margin: consequences for the interpretation of the MBT′/CBT paleothermometer, Biogeosciences, 11, 5637–5655, https://doi.org/10.5194/bg-11-5637-2014, 2014.
Zeng, Z., Xiao, W., Zheng, F., Chen, Y., Zhu, Y., Tian, J., and Zhang, C.: Enhanced production of highly methylated brGDGTs linked to anaerobic bacteria from sediments of the Mariana Trench, Front. Mar. Sci., 10, 1233560, https://doi.org/10.3389/fmars.2023.1233560, 2023.
Zhao, B., Russell, J. M., Tsai, V. C., Blaus, A., Parish, M. C., Liang, J., Wilk, A., Du, X., and Bush, M. B.: Evaluating global temperature calibrations for lacustrine branched GDGTs: Seasonal variability, paleoclimate implications, and future directions, Quaternary Sci. Rev., 310, 108124, https://doi.org/10.1016/j.quascirev.2023.108124, 2023.
Zhang, Z.-X., Parlanti, E., Anquetil, C., Morelle, J., Laverman, A., Thibault, A., Bou, E., and Huguet, A.: Bulk geochemical data and fractional abundances of brGDGTs and brGMGTs in soils, SPM and sediments in the Seine River basin (NW France), PANGAEA [data set], https://doi.org/10.1594/PANGAEA.966245, 2024.