the Creative Commons Attribution 4.0 License.
the Creative Commons Attribution 4.0 License.
Sensitivity of woody carbon stocks to bark investment strategy in Neotropical savannas and forests
David Medvigy
William A. Hoffmann
Adam F. A. Pellegrini
Fire frequencies are changing in Neotropical savannas and forests as a result of forest fragmentation and increasing drought. Such changes in fire regime and climate are hypothesized to destabilize tropical carbon storage, but there has been little consideration of the widespread variability in tree fire tolerance strategies. To test how aboveground carbon stocks change with fire frequency and composition of plants with different fire tolerance strategies, we update the Ecosystem Demography model 2 (ED2) with (i) a fire survivorship module based on tree bark thickness (a key fire-tolerance trait across woody plants in savannas and forests), and (ii) plant functional types representative of trees in the region. With these updates, the model is better able to predict how fire frequency affects population demography and aboveground woody carbon. Simulations illustrate that the high survival rate of thick-barked, large trees reduces carbon losses with increasing fire frequency, with high investment in bark being particularly important in reducing losses in the wettest sites. Additionally, in landscapes that frequently burn, bark investment can broaden the range of climate and fire conditions under which savannas occur by reducing the range of conditions leading to either complete tree loss or complete grass loss. These results highlight that tropical vegetation dynamics depend not only on rainfall and changing fire frequencies but also on tree fire survival strategy. Further, our results indicate that fire survival strategy is fundamentally important in regulating tree size demography in ecosystems exposed to fire, which increases the preservation of aboveground carbon stocks and the coexistence of different plant functional groups.
- Article
(3239 KB) - Full-text XML
-
Supplement
(22898 KB) - BibTeX
- EndNote
Tropical savannas and forests are important components of the land carbon sink (Pan et al., 2011; Liu et al., 2015; Ahlström et al., 2015). However, their ability to continue sequestering carbon is uncertain (Malhi et al., 2008), due in part to the impact of projected increases in drought frequency and changes in fire regime on woody carbon stocks (Brando et al., 2014). Globally, tropical forests, savannas, and grasslands comprise ∼ 60 % of total terrestrial gross primary productivity (Beer et al., 2010) but are also responsible for over 65 % of global carbon emissions stemming from fire and deforestation (van der Werf et al., 2010, 2009). The balance between biological carbon uptake through photosynthesis and carbon emissions from respiration and biomass burning determines whether tropical savannas and forests are a net carbon sink or source.
Fire is critical in defining the vegetation structure and distribution of tropical savannas and forests (Bond et al., 2005; Hoffmann et al., 2012a; Staver et al., 2011b). A positive feedback between flammable grass presence and tree fire mortality in open canopies has been identified as an important mechanism in maintaining savanna regions (Archibald et al., 2009; Hoffmann et al., 2012b; Staver et al., 2011a). Some trees can persist, despite high fire frequencies in many savannas, because they invest heavily in building thick bark (Pellegrini et al., 2017a). Thick bark insulates the xylem and phloem from fire damage, increasing the probability of tree fire survival (Brando et al., 2012; Hoffmann et al., 2012a), and potentially decreasing ecosystem carbon vulnerability to increasing fire frequency with global climate change (Pellegrini et al., 2016b). However, this increase in bark investment may come at a growth cost, as thicker-barked savanna species grow more slowly than thinner-barked forest species under similar growing conditions (Hoffmann et al., 2012a; Rossatto et al., 2009). Though many savanna trees have growth strategies that employ thick bark as a fire survival mechanism, species vary greatly in their investment in bark (Pausas, 2015; Pellegrini et al., 2017a; Rosell, 2016).
Climate, particularly precipitation, has the potential to interact with fire frequency and tree growth strategy (Brando et al., 2014). In locations with low precipitation, tree growth rates are much slower than in locations with high precipitation (Baker et al., 2003). Slower growth rates result in a population of smaller trees with relatively thinner bark than their larger counterparts, making their stems more vulnerable to fire. In addition to climate, physiology can play a role because some trees grow more rapidly than others due to differences in maximum photosynthetic capacity or specific leaf area (Rossatto et al., 2009). Tree growth rate is critical for determining the ability of trees to recover from fire, and consequently the fire frequency necessary to maintain savanna.
Simulating savanna vegetation dynamics is notoriously difficult. However, progress has recently been made in identifying some key mechanisms needed to stabilize savannas in dynamic global vegetation models (DGVMs) (Scheiter and Higgins, 2009; Lehsten et al., 2016; Baudena et al., 2015; Higgins et al., 2000; Haverd et al., 2013; Lasslop et al., 2016). Despite recent progress, DVGMs are still unable to fully capture global savanna extent as emergent features, generally overpredicting the extent of either grasslands or tropical forests (Lasslop et al., 2016). This makes it difficult to quantify the impacts of projected climate change on carbon storage in the tropics because both carbon storage capacity and the resistance of ecosystem carbon to changes in precipitation and fire regime vary across tropical biomes. Additional mechanisms observed to be important for maintaining tree–grass coexistence in empirical studies, such as variability in tree fire survival strategy (Hoffmann et al., 2012a), are underrepresented in models (Haverd et al., 2013; Lehsten et al., 2016). As a result, the implications of including variability in tree fire survival strategy are relatively untested, providing one useful avenue to improve modeling of savanna–forest dynamics.
To better understand the sensitivity of tropical carbon storage to changes in rainfall regime and fire frequency, we updated the Ecosystem Demography model 2 (ED2) to include distinct tropical savanna and forest plant functional types (PFTs), each with a different bark investment strategy. We evaluated our model's predictions against observations of savanna and forest tree growth rates, tree inventories, and total aboveground woody carbon (AGB) for different fire frequencies using field data from savannas and forests in the Cerrado region of Brazil. We then used the model to test the following two hypotheses: (1) including bark investment as a tree fire survival strategy decreases simulated carbon losses to increasing fire frequency, regardless of precipitation regime, due to the higher probability of survival for thicker-barked, larger trees, and (2) including bark thickness as a fire survival strategy expands the environmental conditions under which trees and grasses coexist by allowing for increased tree survival in frequently burned savannas, but also comes at a growth-rate cost that limits trees' ability to shade out grasses. Finally, we considered the effects of changing fire frequency and fire survival strategy on ecosystem composition and aboveground carbon vulnerability along a rainfall gradient in the Neotropics.
2.1 Model description
Our model simulations were carried out in a cohort-based terrestrial biosphere model, ED2, using parameterizations from Xu et al. (2016) unless otherwise specified. ED2 explicitly scales up tree-level competition for light, water, and nutrients to the ecosystem level (Medvigy et al., 2009; Medvigy and Moorcroft, 2012). The effects of water limitation on photosynthesis have been previously identified to be important for simulating savanna–grass dynamics (Baudena et al., 2015; Lasslop et al., 2016). Correspondingly, a novel aspect of the version of ED2 used in this study is the mechanistic representation of water-limited photosynthesis, whereby leaf and stem water potential are tracked and used to solve for root zone water uptake, transport of water vertically through the sapwood, and transpiration of water into the atmosphere. Variability in hydraulic traits such as turgor loss point, xylem water conductivity, and marginal water use efficiency determine PFT-specific responses to changes in leaf and stem water potential. Importantly, this mechanistic water limitation scheme has been demonstrated to better resolve vegetation dynamics in water limited tropical ecosystems (Xu et al., 2016), such as tropical savanna and forest regions.
We have incorporated in ED2 the following new processes important to ecosystem fire resistance in the Neotropics: (1) a PFT-specific bark investment strategy in two updated tropical PFTs; (2) a carbon tradeoff between bark production and tree height, canopy area, sapwood area, rooting depth, and leaf carbon; (3) a fire survivorship function dependent on individual tree bark thickness; and (4) a dynamic feedback between tree size, survivorship probability, and grass biomass availability. Updated model codes are included as Supplement (ED2Model_Supplement_S1.tar.gz). The two new PFTs represent a generic tropical forest tree PFT and tropical savanna tree PFT. Both are based on the tropical brevideciduous PFT from Xu et al. (2016). Previous PFT versions do not include a bark thickness trait. The brevideciduous PFT was chosen because its intermediate wood density and specific leaf area represent a drought survival strategy incorporating both drought avoidance and resistance. This intermediate strategy is utilized by a broad array of tropical tree species (Xu et al., 2016). The savanna and forest PFTs differ only in their bark investment strategy and the associated tradeoffs. In the model, individual tree bark thickness is calculated according to the following equation (Thonicke et al., 2010):
In Eq. (1), bt is tree bark thickness in centimeters, β is the bark thickness slope coefficient that varies with PFT, and dbh is tree diameter at breast height in centimeters. In Neotropical savannas and forests, β ranges from ∼ 0.068 to 0.087 for trees of the forest functional guild and from ∼ 0.087 to 0.142 for trees of the savanna functional guild (Pellegrini et al., 2016a). We assigned β=0.077 and β=0.110 to our forest and savanna PFTs, respectively, based on the average of the observed ranges in bark thickness.
The carbon cost of a tree investing in bark is difficult to quantify. Here, we incorporated a cost through the tree allometric relations. Bark turnover rate in these systems is assumed to be negligible. Ordinarily in cohort- or individual-based models, dbh is allometrically related to woody biomass, leaf biomass, crown height, crown area, rooting depth, and sapwood area. The model also uses a standard allometric relationship between dbh and woody biomass. However, the new model relates the other derived properties to dbh with bark excluded (denoted dbh'). Thus, for a given dbh, a PFT with a large β will have a smaller dbh', fewer leaves, a shorter height, a shallower rooting depth, a smaller crown, and a smaller sapwood area for water transport than a PFT with a small β. A similar tradeoff has been used previously to quantify the costs and benefits associated with growing thick bark (Lawes et al., 2013) and accounts for the observed increased bark investment only in trees growing in fire-prone areas (Charles-Dominique et al., 2017; Pellegrini et al., 2017a). This approach probably exaggerates the cost of bark because the relative cost of a tree growing outer bark for the purpose of stem insulation may be substantially less than the cost of growing xylem. Thus we performed an additional set of simulations with no carbon cost associated with tree bark investment to capture the end members of the spectrum of carbon tradeoff associated with bark investment strategy.
The advantage of having thicker bark is incorporated through our fire survivorship function. This function prescribes that trees with thicker bark are more likely to survive a fire event than trees with thinner bark. Thus, large trees and trees with a large β at a given height are more likely to survive than small trees and trees with a small β. Survivorship is also dependent on the amount of grass biomass present. This grass dependence comports with observations that an abundance of grass biomass results in higher ecosystem flammability and hotter fires that cause increased tree mortality (Hoffmann et al., 2012b). These ideas are incorporated in the following equation based on Pellegrini et al. (2016a) derived from data in Hoffmann et al. (2009):
In Eq. (2), survivorship is the tree survivorship fraction of a given tree size class and functional guild, gb is grass biomass (in g C m−2), and 25 is the threshold for increased fire intensity based on grass biomass curves of savanna flammability from Hoffmann et al. (2012b). In the model, fire intensity is based only on grass biomass present and grass survivorship after fire is always zero. However, grass is able to reseed by continuous seed deposition from adjacent unburnt patches. We used the updated model to assess the joint effects of precipitation, fire disturbance, and bark investment strategy on (1) tree demography and AGB and (2) tree–grass coexistence.
2.2 Simulations
We conducted two classes of single-grid cell simulations. We first evaluated our model performance against observed datasets within the Cerrado region of Brazil at different fire frequencies. We then performed model experiments to assess the influence of bark investment strategy on vegetation carbon and tree–grass coexistence across a rainfall and fire gradient in the Cerrado. Our model experiments included two sets of simulations. First, we ran a control simulation that included C4 grass and our updated savanna and forest PFTs but no investment in bark or fire survival benefit (bt=0). Second, we ran simulations including the following three PFTs run simultaneously: C4 grass, our updated savanna PFT, and our updated forest tree PFT. Both savanna and forest tree PFTs included the bark fire survival strategy and allometric tradeoffs based on bark investment. This scenario assumes that bark and xylem have the same construction cost per unit volume. Given that bark construction cost may be lower than xylem construction costs, we also ran simulations including C4 grass and our updated savanna and forest tree PFTs with a fire survival strategy but no cost associated with bark production. Excluding this bark-growth tradeoff did not change the overall results. We include these simulations with fire survival strategy but no bark investment tradeoff for comparison in the Supplement.
In our simulations, ecosystems were spun up from tree seedlings initialized at a density of 1.0 seedling m−2. C4 grass was able to seed in at a rate of 0.001 kg C month−1 m−2. Simulations were forced with 0.5∘, 3 h meteorology from the Princeton Global Forcing dataset (Sheffield et al., 2006). To isolate the effects of precipitation and fire frequency, all simulations were forced with a constant 370 ppm atmospheric CO2 and meteorology looped over the same 20-year period (1980–2000). This 20-year period is long enough to ensure a majority of the years are not anomalous, but short enough to reduce the effects of trends in climate with global change. Median fire frequency was prescribed at the beginning of a simulation and fire interval varied stochastically within ±3 years of the user input value. Fire disturbance occurred at most once per year and the fraction of the landscape that burned was kept constant at 30 % during a given fire event. Thus, there were no climate feedbacks on fire, and grass biomass only affected fire intensity (i.e. tree fire survival probability), but not the frequency or burned area fraction. Windthrow disturbance affected 1 % of the landscape each year, resulting in 100 % mortality of trees taller than 5 m and 20 % mortality of trees smaller than 5 m.
2.2.1 Model evaluation
We evaluated performance of the updated model using ecosystem measurements from study sites located in IBGE and the adjacent JBB Ecological Reserves within the Cerrado region of Brazil at approximately 15.95∘ S and 47.85∘ W. In these reserves, mean annual temperature is 22.5 ∘C and mean annual precipitation (MAP) totals 1460 mm with a dry season during which precipitation is <50 mm month−1 from May to September. From the 1910s until reserve formation in the 1970s, the whole landscape was subject to frequent fire at ∼ 2-year intervals (Pellegrini et al., 2014). Since reserve formation, the initiation of fire management strategies has provided a landscape with diverse disturbance histories: fire return interval ranges from a few years in savannas to half a century in some adjacent forest patches (Pellegrini et al., 2014).
At the tree level, we compared annual diameter increments in the simulation with bark and without fire disturbance to annual diameter increments of twelve paired savanna and forest species measured over the years 2006–2007 (Rossatto et al., 2009). We performed a 35-year model spin-up from seedlings in accordance with the disturbance history and age structure of the site (Rossatto et al., 2009) and then examined the range of average annual diameter increments of our savanna and forest PFTs over a 20-year period to the range of observed annual diameter increments. In our simulations, the dbh size classes included in the calculation for the savanna PFT ranged from 5 to 9 cm with a mean of ∼ 7 cm and from 6 to 10 cm with a mean of ∼ 8 cm for the forest PFT in accordance with the Rossatto et al. (2009) observations.
Next, we assessed the ability of the model to predict observed tree size class distributions and measurements of AGB at sites with different fire frequencies to evaluate if the updated model with bark could more accurately capture ecosystem-level carbon dynamics and tree size abundance in response to fire compared to the control model without bark. We compared model simulated tree size distributions for trees with dbh >2 cm to inventories of trees with dbh >2 cm along 200–300 m2 transects made in July 2012 within the IBGE and JBB Ecological Reserves. Total AGB was calculated from diameter inventories using the allometric relations from Xu et al. (2016) and accounting for bark carbon investment assuming β=0.077. We performed a 35-year model spin-up in accordance with the disturbance history of the reserves after 1970 (Pellegrini et al., 2014) and then compared the range in simulated AGB over the next 10-year period in a simulation with bark fully included and a control simulation without bark investment to observed AGB estimates and tree size class distributions. In our simulations, our high fire frequency scenario was forced with a fire return interval that ranged from 2 to 8 years, our intermediate fire frequency scenario was forced with a return interval that ranged from 9 to 15 years, and our low fire frequency scenario was forced with a return interval that ranged from 57 to 63 years, in accordance with the disturbance regimes of Pellegrini et al. (2014) plots 3, 4–5, and 6, respectively. In these validations, the range in the model output represents the range in simulated AGB or tree size over the 10-year period. Variation in the observations is due to variation between plot biomass or in variation across individuals within plots sampled at a single point in time. Although the errors are not analogous, we intend to represent the range in model output resulting from growth and variable meteorological forcing over the 10-year time period following model spin-up.
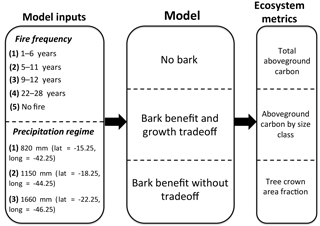
Figure 1Schematic of model experiments along a rainfall gradient. Varied model inputs include fire frequency and precipitation regime (associated with a particular study site locations in the Cerrado region of South America). Different model versions were used to understand the effect of including bark as a fire survival strategy on model outputs of various ecosystem metrics including aboveground woody carbon, tree size class distribution, and tree crown area fraction.
2.2.2 Model experiments
To assess the outcome of including bark investment as a fire survival strategy on carbon vulnerability and tree–grass coexistence, we conducted experiments along sites with a rainfall gradient within the Cerrado. We included locations with MAP within the first (MAP = 820 mm), central (MAP = 1150 mm), and fourth quartiles (MAP = 1660 mm) of the distribution from a network of sites. At each location we included simulations with bark investment and a growth tradeoff, and with no bark at four forced fire return intervals ranging from 1 to 6, 5 to 11, 9 to 15, and 22 to 28 years. Additionally, we included a simulation with no fire. For each simulation we performed a 100-year model spin-up from seedlings and then analyzed the subsequent 20-year average total AGB, AGB by tree size, and tree crown area fraction (Fig. 1). Tree crown area fraction ranged from 0, corresponding to open grassland, to 1, corresponding to closed canopy forest and was calculated by summing the crown area of each cohort (up to a maximum crown area of one). Savanna regions were defined as regions having a crown area fraction of 0.2–0.8.
3.1 Agreement between emergent tree growth in simulations and observations
Simulated growth rates for both the savanna and forest PFTs fell well within the observed range for Cerrado species when the allometric growth tradeoff with bark thickness was included (Fig. S1). The median growth rate for the savanna PFT was within <0.1 % of the observed median savanna species growth rate (Fig. S1a). Additionally, both observed and simulated savanna species had similar minimum diameter increments. However, the model did not capture the upper limit of faster growing savanna species, possibly due to additional differences in physiological traits such as allocation or the maximum rate of carboxylation. The model overestimated the median growth rate for the forest species by ∼ 23 %, but was able to capture a wide range of the variability in growth rates seen in the observations (Fig. S1b). Further, the interquartile range of observed and simulated diameter increments overlapped broadly.
Including a bark investment strategy in the model resulted in trees becoming more fire resistant with increasing size. As a result, for higher fire frequencies, simulations where bark was used to determine fire-driven mortality and growth tradeoffs predict a larger maximum tree size, which more closely reflects observed size class distribution and skew compared to the model without bark (Fig. S2). Further, at high fire frequencies, simulations with bark had the smallest percent difference in predictions of the median size class (23.0 % compared to 25.9 % for the simulation without bark) (Fig. S2a). At intermediate fire frequencies, simulations with bark also had a smaller percent difference in predictions of the median size class (27.0 % compared to 51.7 % for the simulation without bark) and better predict the skew in number count towards larger tree size classes (Fig. S2b). For low fire frequencies, including a bark investment strategy did not improve predictions of maximum tree size; however a better prediction for median tree size and skew towards larger trees was achieved (38.6 % compared to 44.1 % for the simulation without bark) (Fig. S2c).
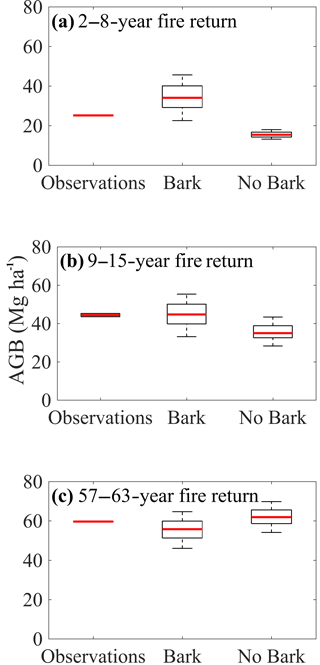
Figure 2Tree fire survival strategy impacts aboveground tree biomass with fire disturbance. Observed and model-simulated aboveground woody biomass (AGB) under (a) high-, (b) intermediate-, and (c) low-frequency fire regimes. Model simulations included a 35-year model spin-up in accordance with the disturbance history of the observations (Pellegrini et al., 2014). Simulated AGB was compared over the subsequent 10-year period for the model with bark and the model without bark. For model output, the red line denotes the predicted median AGB, the black box denotes the interquartile range, and the dotted error bars denote ±2.7 standard deviations over the subsequent 10-year period following the 35-year spin-up. For the observations, variation in AGB is due to variation in biomass across plots at the time of sampling. See Methods for additional details.
Size-specific survivorship affected predictions of ecosystem AGB under different fire frequencies. At high fire frequencies, simulations with a bark investment strategy captured the observed AGB within its predicted range and had the lower percent error between simulated and observed mean AGBs (an overestimation by 36.2 % compared to an underestimation by 38.8 % for the simulation without bark) (Fig. 2a). Under intermediate fire frequencies, simulations with a bark investment strategy overestimated mean AGB by 0.8 %, and the observed AGB was fully within the simulated interquartile range, whereas the simulation without bark underestimated mean AGB by 20.2 % and did not capture the observed AGB within the range of predicted values (Fig. 2b). At low-frequency fire, the simulation without bark predicts the observed AGB marginally more accurately than the model with a bark investment strategy (percent errors of + and −6.7%, respectively) (Fig. 2c).
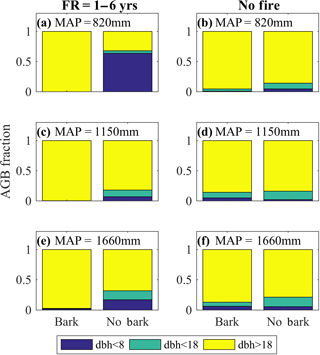
Figure 3Bark fire survival strategy increases the fraction of aboveground woody biomass in large trees, particularly with frequent fire. Model-simulated fraction of aboveground woody biomass (AGB) present in different tree diameter at breast height (dbh in cm) size classes for 2 < dbh <8 (blue), 8 < dbh <18 (cyan), and 18 < dbh (yellow) at low (a–b), intermediate (c–d), and high (e–f) MAP for a high-frequency (FR) fire regime (a, c, e), and a no-fire-disturbance simulation (b, d, f). Simulations were initialized with tropical tree and C4 grass plant functional types and included a 100-year model spin-up from seedlings. The subsequent 20-year average AGB fraction by tree size class is shown for the model with bark and the model without bark. See Fig. S2 for size class distributions associated with intermediate fire frequency.
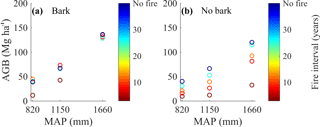
Figure 4Bark fire survival strategy buffers aboveground woody biomass loss with frequent fire, particularly at high MAP. Model-simulated total aboveground woody carbon (AGB) at different MAP and forced fire regimes for the model with bark (a) and the model with no bark (b). Simulations were initialized with tropical tree and C4 grass plant functional types and included a 100-year model spin-up from seedlings. The subsequent 20-year average AGB for each disturbance and precipitation regime is shown.
3.2 Bark investment decreases carbon losses at high fire frequencies
We found substantial differences in the fraction of AGB present in different tree size classes between the original model without bark and the updated model with a bark investment strategy, but these differences depended on fire frequency and precipitation. The impact of including bark thickness increased with MAP and fire frequency because the higher growth rates allowed for trees to grow larger faster, but the increased fire frequency restricted the growth of trees that did not invest in thick bark (Figs. 3, S3). When fire was frequent, the model without the bark investment strategy predicts a smaller absolute AGB and a large contribution of small- and intermediate-sized trees to total AGB, whereas the model version with bark investment strategy predicts a much higher absolute AGB with virtually all biomass allocated in the larger tree size classes (Figs. 3–4). These results were robust regardless of the cost of bark investment to tree growth (see Sect. 2.1) (Fig. S4). The greater proportion of biomass in large trees was due to the low probability of mortality during a fire because of the insulating capacity of bark and the relationship between tree size and bark thickness (Eq. 1). However, it should be noted that the biomass fraction of small trees is not illustrative of the frequency of small trees, as small tree biomass comprises a very small fraction of AGB when large trees are present. Thus, small trees grow between fires even in the simulations with a bark investment strategy, though they comprise very little of the absolute biomass. Indeed, the model with bark investment strategy predicts cumulative AGB mortality (resulting from fire, windthrow disturbance, age-related mortality, and carbon starvation) to occur almost exclusively in the small and large tree size classes, excluding midsized trees (Fig. S5). This absence of midsized trees mortality is indicative of an absence of midsized trees due to the high fire-driven mortality rate of small, low biomass trees. AGB mortality in large trees results from a low but persistent age-related mortality and windthrow disturbance. In contrast, trees of all size classes are present in the no bark simulation, and correspondingly the model predicts AGB mortality across all size classes, consistent with a large number of smaller trees, fewer intermediate- and large-sized trees, and an overall lower total AGB (Fig. 4, S5). Trends in AGB growth reflect almost identical biomass patterns. As would be expected, the difference in tree size distributions between the model without bark and the model with a bark investment strategy decreased substantially when fires were eliminated (Figs. 3b, d, f, S5b, d, f).
We also found that tree size distributions were largely unaffected by MAP in the presence of frequent fire in simulations with a bark investment strategy (Figs. 3a, c, e, S4); only minor impacts were found at intermediate fire frequency and high MAP (Fig. S3c). In contrast, the model without a bark investment strategy predicts that small size classes comprised a substantial fraction of AGB for high fire frequency simulations, particularly at low MAP (Fig. 3a, c, e).
Incorporating bark thickness decreased predicted carbon losses with increasing fire frequency (Fig. 4) because larger, thick-barked trees made up the majority of AGB (Fig. 3) and had a very low probability of mortality during a fire. When a bark investment strategy was included, fire caused almost no reduction in biomass at the wettest site (1660 mm yr−1 MAP) (Figs. 4a, S6a). In simulations with no bark investment strategy, trees were highly vulnerable to fire, so burning at ∼ 3-year intervals resulted in a 73 % reduction in biomass relative to when fire was excluded (Fig. 4b). However, the effect of including a bark investment strategy on reducing carbon losses decreased as MAP decreased (i.e., the difference in simulated carbon loss between scenarios without and with bark investment strategy was lowest in the driest site). For example, at an intermediate MAP (1150 mm yr−1), a 3-year fire return interval caused a 36 % reduction in AGB in the model with a bark investment strategy and an 81 % reduction in AGB using the control model without bark investment, relative to ecosystems without fire. At the driest site, a 3-year fire return interval caused a 70 % reduction in AGB in simulations with a bark investment strategy and a 76 % reduction in AGB for the simulations without bark, relative to ecosystems without fire.
There was a strong interaction between precipitation, fire, and bark investment strategy. When no bark investment strategy was included, both fire frequency and precipitation exerted an equivalently strong control on total AGB, and the range in AGB after 100 years of growth increased substantially with increasing precipitation, but strongly depended on fire frequency (Fig. 4b). However, when bark investment was included as a fire survival strategy, MAP exerted a much stronger control than fire on the total AGB (Figs. 4a, S6a), indicating the important role of water availability regulating growth when species become fire resistant.
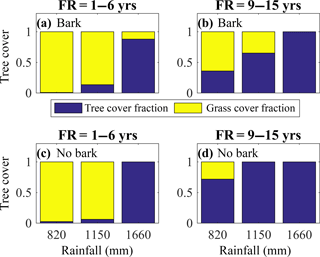
Figure 5Bark investment can broaden the range of climate and fire conditions under which savannas occur by reducing the range of conditions leading to either complete tree loss or complete grass loss. Model-simulated tree cover fraction present at different levels of mean annual precipitation (in mm) for the model with bark (a–b) and the model without bark (c–d) for fire frequencies (FR) of 1–6 years (a, c) and 9–15 years (b, d). Simulations were initialized with tropical tree and C4 grass plant functional types and included a 100-year model spin-up from seedlings. The subsequent 20-year average tree cover fraction is shown.
3.3 Environment and biological traits jointly affect simulated tree–grass coexistence
Both fire frequency and precipitation were important in maintaining tree–grass coexistence and thus in controlling the distribution of grasslands, savannas, and tropical forests (Fig. 5). At high fire frequencies and low precipitation, we simulated grasslands with minimal tree cover regardless of the model scheme (Figs. 5a, c, S7a, c). However, simulations with bark investment as a fire survival strategy expanded conditions under which tree crown area fraction ranged from 0.2 to 0.8. At fire return intervals of 1–6 years and intermediate MAP (1150 mm yr−1) and at less frequent fire return intervals (9–15 years) and intermediate MAP (1150 mm yr−1), bark investment mitigated either complete tree loss or complete grass loss (Figs. 5, S7). Thus, including bark investment as a fire survival strategy moderated the transition between grassland and forest at intermediate MAP under varying fire frequencies.
We documented that tree bark investment strategy interacts with precipitation and fire frequency to determine both (i) the stability of ecosystem carbon to fire and (ii) the coexistence of grasses and trees, illustrating that species traits, in addition to climate and fire, are critical for the stability of savanna and forest biomes. Bark investment strategy increased the stability of the carbon stock in large trees, which decreased ecosystem carbon losses with increased fire frequency under a range of precipitation conditions. Investment in bark was especially important in wetter savannas and forests, illustrating that the distribution of functional traits is fundamental to the resilience of wet forests to increased fire and changing rainfall regimes.
4.1 Implications for carbon losses
Our simulations illustrate that tree bark thickness as a fire survival trait substantially decreases fire-driven carbon losses, but the magnitude of the effect depends on precipitation regime (Fig. 4a–b). We found that bark investment was particularly important at reducing carbon losses at higher fire frequencies in locations with a high MAP (1660 mm). This is because trees had ample water availability, enabling smaller trees to grow rapidly and quickly become fire resistant. In contrast, the effect of bark investment offered little to no benefit in reducing carbon losses at the lowest MAP and highest fire frequency because small trees were not able to grow rapidly and accumulate thick enough bark to escape fire mortality. The decreasing utility of bark investment as a survival strategy at the lowest MAP and highest fire frequency likely occurred because bark investment slowed growth and prolonged the period during which trees were more susceptible to fire-driven mortality. Taken together, these results suggest that current models that do not account for bark investment strategies may underpredict Neotropical carbon resistance to fire in both savannas and forests. Such models would overpredict mortality of large thick-barked trees that make up a majority amount of aboveground woody biomass (Slik et al., 2013; Hanan et al., 2008). However, further work understanding the spatial distribution of tree species and their corresponding bark investment strategies is also critical (Pellegrini et al., 2017a; Rosell, 2016; Pausas, 2015; Dantas et al., 2013) because observations show that even large trees in rainforests have thin enough bark that they suffer substantial fire mortality (Uhl and Kauffman, 1990), although the forests with high mortality have precipitation values much higher than the maximum we consider (1660 mm).
4.2 Implications for tree–grass coexistence
Capturing savanna distributions globally has long been difficult for vegetation models, which overpredict the extent of either tropical forests or grasslands (Hickler et al., 2006; Cramer et al., 2001; Bonan et al., 2003; Hely et al., 2006; Schaphoff et al., 2006; Sato et al., 2007). A number of recent studies have focused on this issue: the adaptive dynamic global vegetation model (aDGVM) was able to capture savanna extent in Africa by including (i) trees with a higher fire mortality rate in small tree size classes, (ii) regenerative tree resprouting after fire events, and (iii) grass as super individuals (Scheiter and Higgins, 2009). The individual-based Populations-Order-Physiology model also included size-dependent tree mortality and was able to reproduce key vegetation structure and function along a rainfall and fire gradient in Australia (Haverd et al., 2013). Studies by Baudena et al. (2015) and Lasslop et al. (2016) have proposed several key mechanisms for capturing savannas in models: (1) water limitation on tree growth, (2) competition for water between grasses and trees, and (3) a grass–fire feedback.
The results from this study show a strong dependence of tree growth on precipitation and fire frequency, supporting observations (Pellegrini et al., 2017b; Higgins et al., 2007) and other modeling studies (Baudena et al., 2015). However, we found that including bark investment as a fire survival mechanism broadened the range of climate and fire conditions under which savannas occur by reducing the range of conditions leading to either complete tree loss or complete grass loss. This is due to the shift towards fewer, larger trees that store relatively more AGB per unit crown area than smaller trees, but are also resilient to frequent fire, resulting in ecosystems with intermediate crown cover in a wider range of precipitation and fire regimes. Thus, fire and precipitation as well as species-specific bark thickness traits have the potential to affect tree–grass coexistence, suggesting that inclusion of bark investment in models at broader spatial scales has the potential to substantially enhance our ability to accurately project changes in the tropical carbon sink with changes in fire and rainfall over the upcoming century.
4.3 Limitations and future work
Several avenues exist for future model improvement. Tree re-sprouting after fire has been shown to be essential in predicting the range of conditions for which tree–grass coexistence is possible (Higgins et al., 2000). Currently in our model, trees reproduce only through seedling recruitment. This has the potential to affect model benchmarking and simulation predictions because initial aboveground growth rates of re-sprouts are substantially higher compared to seedlings due to (a) access to belowground carbohydrate stores and (b) elimination of the need to allocate carbon for roots. Thus, re-sprouting may allow for better agreement between model predictions at higher growth rates and fire frequencies (Figs. 2, S1–2). Enabling tree re-sprouting may also stabilize savannas under frequent fire and low precipitation where all model versions currently simulate grassland (Fig. 5).
Additionally, the current fire model does not interact dynamically with climate or the nitrogen cycle. ED2 is capable of resolving nitrogen dynamics (Trugman et al., 2016) and we anticipate that coupling nitrogen and climate feedbacks to fire will be an important step in accurately modeling the carbon cycle. Despite these current limitations, the updated model accurately predicts growth, demographics, and AGB. We believe that these results can provide important insight into tree–grass coexistence and carbon resistance with changing fire frequency in the Neotropics with global change.
In conclusion, our results highlight that carbon storage in tropical savannas and forests depends not only on changing environmental drivers but also on tree fire survival strategy. Thus, we can improve projections of the tropical carbon sink with global climate change by increasing our understanding of the distribution of bark investment and incorporating this knowledge of bark investment into future vegetation models. Further, an increased understanding of the interaction between bark investment strategy and environmental drivers promises to increase our ability to project the distribution of savanna in regions previously simulated as grasslands or forests by reducing the range of conditions leading to either complete tree loss or complete grass loss.
Model codes are included in the Supplement.
Data presented here can be acquire from the corresponding author.
The supplement related to this article is available online at: https://doi.org/10.5194/bg-15-233-2018-supplement.
ATT, AFAP, DM, and WAH designed the research. ATT performed numerical experiments. ATT and AFAP analyzed the data. AFAP and WAH contributed field data. ATT drafted the paper and all authors contributed to writing of the manuscript.
The authors declare that they have no conflict of interest.
The authors gratefully acknowledge support from the Program in Latin
American Studies at Princeton University (Adam F. A. Pellegrini), the Princeton
Environmental Institute Walbridge Fund (Anna T. Trugman), the National Science
Foundation Graduate Research Fellowship (Anna T. Trugman and Adam F. A. Pellegrini), the National
Science Foundation Award 1151102. (David Medvigy) and DEB1354943 (William A. Hoffmann), and US
Department of Energy, Office of Science, Office of Biological and
Environmental Research, Terrestrial Ecosystem Science (TES) Program under
award number DE-SC0014363 (David Medvigy).
Edited by: Kirsten Thonicke
Reviewed by: two anonymous referees
Ahlström, A., Raupach, M. R., Schurgers, G., Smith, B., Arneth, A., Jung, M., Reichstein, M., Canadell, J. G., Friedlingstein, P., Jain, A. K., Kato, E., Poulter, B., Sitch, S., Stocker, B. D., Viovy, N., Wang, Y. P., Wiltshire, A., Zaehle, S., and Zeng, N.: The dominant role of semi-arid ecosystems in the trend and variability of the land CO2 sink, Science, 348, 895–899, https://doi.org/10.1126/science.aaa1668, 2015.
Archibald, S., Roy, D. P., van Wilgen, B. W., and Scholes, R. J.: What limits fire? An examination of drivers of burnt area in Southern Africa, Glob. Change Biol., 15, 613–630, https://doi.org/10.1111/j.1365-2486.2008.01754.x, 2009.
Baker, T. R., Swaine, M. D., and Burslem, D. F. R. P.: Variation in tropical forest growth rates: combined effects of functional group composition and resource availability, Perspectives in Plant Ecology, Evol. Syst., 6, 21–36, https://doi.org/10.1078/1433-8319-00040, 2003.
Baudena, M., Dekker, S. C., van Bodegom, P. M., Cuesta, B., Higgins, S. I., Lehsten, V., Reick, C. H., Rietkerk, M., Scheiter, S., Yin, Z., Zavala, M. A., and Brovkin, V.: Forests, savannas, and grasslands: bridging the knowledge gap between ecology and Dynamic Global Vegetation Models, Biogeosciences, 12, 1833–1848, https://doi.org/10.5194/bg-12-1833-2015, 2015.
Beer, C., Reichstein, M., Tomelleri, E., Ciais, P., Jung, M., Carvalhais, N., Rodenbeck, C., Arain, M. A., Baldocchi, D., Bonan, G. B., Bondeau, A., Cescatti, A., Lasslop, G., Lindroth, A., Lomas, M., Luyssaert, S., Margolis, H., Oleson, K. W., Roupsard, O., Veenendaal, E., Viovy, N., Williams, C., Woodward, F. I., and Papale, D.: Terrestrial gross carbon dioxide uptake: global distribution and covariation with climate, Science, 329, 834–838, https://doi.org/10.1126/science.1184984, 2010.
Bonan, G. B., Levis, S., Sitch, S., Verteinstein, M., and Oleson, K. W.: A dynamic global vegetation model for use with climate models: concepts and description of simulated vegetation dynamics, Glob. Change Biol., 9, 1543–1566, https://doi.org/10.1046/j.1529-8817.2003.00681.x, 2003.
Bond, W. J., Woodward, F. I., and Midgley, G. F.: The global distribution of ecosystems in a world without fire, The New Phytol., 165, 525–537, https://doi.org/10.1111/j.1469-8137.2004.01252.x, 2005.
Brando, P. M., Nepstad, D. C., Balch, J. K., Bolker, B., Christman, M. C., Coe, M., and Putz, F. E.: Fire-induced tree mortality in a neotropical forest: the roles of bark traits, tree size, wood density and fire behavior, Glob. Change Biol., 18, 630–641, https://doi.org/10.1111/j.1365-2486.2011.02533.x, 2012.
Brando, P. M., Balch, J. K., Nepstad, D. C., Morton, D. C., Putz, F. E., Coe, M. T., Silverio, D., Macedo, M. N., Davidson, E. A., Nobrega, C. C., Alencar, A., and Soares-Filho, B. S.: Abrupt increases in Amazonian tree mortality due to drought-fire interactions, P. Natl. Acad. Sci. USA, 111, 6347–6352, https://doi.org/10.1073/pnas.1305499111, 2014.
Charles-Dominique, T., Midgley, G. F., and Bond, W. J.: Fire frequency filters species by bark traits in a savanna–forest mosaic, J. Veg. Sci., 28, 728–735, doi:10.1111/jvs.12528, 2017.
Cramer, W., Bondeau, A., Woodward, F. I., Prentice, I. C., Betts, R. A., Brovkin, V., Cox, P. M., Fischer, V., Foley, J. A., Friend, A. D., Kucharik, C., Lomas, M. R., Ramanketty, N., Sitch, S., Smith, B., White, A., and Young-Molling, C.: Global response of terrestrial ecosystem structure and function to CO2 and climate change: results from six dynamic global vegetation models, Glob. Change Biol., 7, 357–373, 2001.
Dantas, V. L., Batalha, M. A., and Pausas, J. G.: Fire drives functional thresholds on the savanna–forest transition, Ecology, 94, 2454–2463, 2013.
Hanan, N. P., Sea, W. B., Dangelmayr, G., and Govender, N.: Do fires in savannas consume woody biomass? A comment on approaches to modeling savanna dynamics, Am. Nat., 171, 851–856, https://doi.org/10.1086/587527, 2008.
Haverd, V., Smith, B., Cook, G. D., Briggs, P. R., Nieradzik, L., Roxburgh, S. H., Liedloff, A., Meyer, C. P., and Canadell, J. G.: A stand-alone tree demography and landscape structure module for Earth system models, Geophys. Res. Lett., 40, 5234–5239, https://doi.org/10.1002/grl.50972, 2013.
Hely, C., Bremond, L., Alleaume, S., Smith, B., Sykes, M. T., and Guiot, J.: Sensitivity of African biomes to changes in the precipitation regime, Glob. Ecol. Biogeogr., 15, 258–270, https://doi.org/10.1111/j.1466-822X.2006.00235.x, 2006.
Hickler, T., Prentice, I. C., Smith, B., Sykes, M. T., and Zaehle, S.: Implementing plant hydraulic architecture within the LPJ Dynamic Global Vegetation Model, Glob. Ecol. Biogeogr., 15, 567–577, https://doi.org/10.1111/j.1466-8238.2006.00254.x, 2006.
Higgins, S. I., Bond, W. J., and Trollope, W. S. W.: Fire, resprouting and variability: a recipe for grass-tree coexistence in savanna, J. Ecol., 88, 213–229, 2000.
Higgins, S. I., Bond, W. J., February, E. C., Bronn, A., Euston-Brown, D. I. W., Enslin, B., Govender, N., Rademan, L., O'Regan, S., Potgieter, A. L. F., Scheiter, S., Sowry, R., Trollope, L., and Trollope, W. S. W.: Effects of four decades of fire manipulation on woody vegetation structure in savanna, Ecology, 88, 1119–1125, 2007.
Hoffmann, W. A., Adasme, R., Haridasan, M., T. de Carvalho, M., Geiger, E. L., Pereira, M. A. B., Gotsch, S. G., and Franco, A. C.: Tree topkill, not mortality, governs the dynamics of savanna-forest boundaries under frequent fire in central Brazil, Ecology, 90, 1326–1337, 2009.
Hoffmann, W. A., Geiger, E. L., Gotsch, S. G., Rossatto, D. R., Silva, L. C., Lau, O. L., Haridasan, M., and Franco, A. C.: Ecological thresholds at the savanna-forest boundary: how plant traits, resources and fire govern the distribution of tropical biomes, Ecol. Lett., 15, 759–768, https://doi.org/10.1111/j.1461-0248.2012.01789.x, 2012a.
Hoffmann, W. A., Jaconis, S. Y., McKinley, K. L., Geiger, E. L., Gotsch, S. G., and Franco, A. C.: Fuels or microclimate? Understanding the drivers of fire feedbacks at savanna-forest boundaries, Austral. Ecol., 37, 634–643, https://doi.org/10.1111/j.1442-9993.2011.02324.x, 2012b.
Lasslop, G., Brovkin, V., Reick, C. H., Bathiany, S., and Kloster, S.: Multiple stable states of tree cover in a global land surface model due to a fire-vegetation feedback, Geophys. Res. Lett., 43, 6324–6331, https://doi.org/10.1002/2016gl069365, 2016.
Lawes, M. J., Midgley, J. J., Clarke, P. J., and Jones, R.: Costs and benefits of relative bark thickness in relation to fire damage: a savanna/forest contrast, J. Ecol., 101, 517–524, https://doi.org/10.1111/1365-2745.12035, 2013.
Lehsten, V., Arneth, A., Spessa, A., Thonicke, K., and Moustakas, A.: The effect of fire on tree–grass coexistence in savannas: a simulation study, Int. J. Wild. Fire, 25, 137, https://doi.org/10.1071/wf14205, 2016.
Liu, Y. Y., van Dijk, A. I. J. M., de Jeu, R. A. M., Canadell, J. G., McCabe, M. F., Evans, J. P., and Wang, G.: Recent reversal in loss of global terrestrial biomass, Nat. Clim. Change, 5, 470–474, https://doi.org/10.1038/nclimate2581, 2015.
Malhi, Y., Roberts, J. T., Betts, R., Killeen, T. J., Li, W., and Nobre, C.: Climate Change, Deforestation, and the Fate of the Amazon, Science, 319, 169–172, 2008.
Medvigy, D., Wofsy, S. C., Munger, J. W., Hollinger, D. Y., and Moorcroft, P. R.: Mechanistic scaling of ecosystem function and dynamics in space and time: Ecosystem Demography model version 2, J. Geophys. Res., 114, https://doi.org/10.1029/2008jg000812, 2009.
Medvigy, D. and Moorcroft, P. R.: Predicting ecosystem dynamics at regional scales: an evaluation of a terrestrial biosphere model for the forests of northeastern North America, Philos. T. Roy. Soc. Lond., Biological Sciences, 367, 222–235, https://doi.org/10.1098/rstb.2011.0253, 2012.
Pan, Y., Birdsey, R. A., Fang, J., Houghton, R., Kauppi, P. E., Kurz, W. A., Phillipps, O. L., Shvidenko, A. Z., Lewis, S. L., Canadell, J. G., Ciais, P., Jackson, R. B., Pacala, S. W., McGuire, A. D., Piao, S., Rautiainen, A., Sitch, S., and Hayes, D.: A Large and Persistent Carbon Sink in the World's Forests, Science, 2011.
Pausas, J. G.: Bark thickness and fire regime, Funct. Ecol., 29, 317–327, 2015.
Pellegrini, A. F., Anderegg, W. R., Paine, C. E., Hoffmann, W. A., Kartzinel, T., Rabin, S. S., Sheil, D., Franco, A. C., and Pacala, S. W.: Convergence of bark investment according to fire and climate structures ecosystem vulnerability to future change, Ecol. Lett., https://doi.org/10.1111/ele.12725, 2017a.
Pellegrini, A. F. A., Hoffmann, W. A., and Franco, A. C.: Carbon accumulation and nitrogen pool recovery during transitions from savanna to forest in central Brazil, Ecol., 95, 342–352, 2014.
Pellegrini, A. F. A., Franco, A. C., and Hoffmann, W. A.: Shifts in functional traits elevate risk of fire-driven tree dieback in tropical savanna and forest biomes, Glob. Change Biol., 22, 1235–1243, https://doi.org/10.1111/gcb.13110, 2016a.
Pellegrini, A. F. A., Socolar, J. B., Elsen, P. R., and Giam, X.: Tradeoffs between savanna woody plant diversity and carbon storage in the Brazilian Cerrado, Glob. Change Biol., https://doi.org/10.1111/gcb.13259, 2016b.
Pellegrini, A. F. A., Pringle, R. M., Govender, N., Hedin, L. O., and Wardle, D.: Woody plant biomass and carbon exchange depend on elephant-fire interactions across a productivity gradient in African savanna, J. Ecol., 105, 111–121, https://doi.org/10.1111/1365-2745.12668, 2017b.
Rosell, J. A.: Bark thickness across the angiosperms: more than just fire, New Phytol., 211, 90–102, https://doi.org/10.1111/nph.13889, 2016.
Rossatto, D. R., Hoffmann, W. A., and Franco, A. C.: Differences in growth patterns between co-occurring forest and savanna trees affect the forest-savanna boundary, Funct. Ecol., 23, 689–698, https://doi.org/10.1111/j.1365-2435.2009.01568.x, 2009.
Sato, H., Itoh, A., and Kohyama, T.: SEIB–DGVM: A new Dynamic Global Vegetation Model using a spatially explicit individual-based approach, Ecol. Modell., 200, 279–307, https://doi.org/10.1016/j.ecolmodel.2006.09.006, 2007.
Schaphoff, S., Lucht, W., Gerten, D., Sitch, S., Cramer, W., and Prentice, I. C.: Terrestrial biosphere carbon storage under alternative climate projections, Climat. Change, 74, 97–122, https://doi.org/10.1007/s10584-005-9002-5, 2006.
Scheiter, S. and Higgins, S. I.: Impacts of climate change on the vegetation of Africa: an adaptive dynamic vegetation modelling approach, Glob. Change Biol., 15, 2224–2246, doi10.1111/j.1365-2486.2008.01838.x, 2009.
Sheffield, J., Goteti, G., and Wood, E. F.: Development of a 50-year high-resolution global dataset of meteorological forcings for land surface modeling, J. Clim., 19, 3088–3111, https://doi.org/10.1175/jcli3790.1, 2006.
Slik, J. W. F., Paoli, G., McGuire, K., Amaral, I., Barroso, J., Bastian, M., Blanc, L., Bongers, F., Boundja, P., Clark, C., Collins, M., Dauby, G., Ding, Y., Doucet, J.-L., Eler, E., Ferreira, L., Forshed, O., Fredriksson, G., Gillet, J.-F., Harris, D., Leal, M., Laumonier, Y., Malhi, Y., Mansor, A., Martin, E., Miyamoto, K., Araujo-Murakami, A., Nagamasu, H., Nilus, R., Nurtjahya, E., Oliveira, Á., Onrizal, O., Parada-Gutierrez, A., Permana, A., Poorter, L., Poulsen, J., Ramirez-Angulo, H., Reitsma, J., Rovero, F., Rozak, A., Sheil, D., Silva-Espejo, J., Silveira, M., Spironelo, W., ter Steege, H., Stevart, T., Navarro-Aguilar, G. E., Sunderland, T., Suzuki, E., Tang, J., Theilade, I., van der Heijden, G., van Valkenburg, J., Van Do, T., Vilanova, E., Vos, V., Wich, S., Wöll, H., Yoneda, T., Zang, R., Zhang, M.-G., and Zweifel, N.: Large trees drive forest aboveground biomass variation in moist lowland forests across the tropics, Glob. Ecol. Biogeogr., 22, 1261–1271, https://doi.org/10.1111/geb.12092, 2013.
Staver, A. C., Archibald, S., and Levin, S.: Tree cover in sub-Saharan Africa: rainfall and fire constrain forest and savanna as alternative stable states, Ecology, 92, 1063–1072, 2011a.
Staver, A. C., Archibald, S., and Levin, S. A.: The global extent and determinants of savanna and forest as alternative biome states, Science, 334, 230–232, 2011b.
Thonicke, K., Spessa, A., Prentice, I. C., Harrison, S. P., Dong, L., and Carmona-Moreno, C.: The influence of vegetation, fire spread and fire behaviour on biomass burning and trace gas emissions: results from a process-based model, Biogeosciences, 7, 1991–2011, https://doi.org/10.5194/bg-7-1991-2010, 2010.
Trugman, A. T., Fenton, N. J., Bergeron, Y., Xu, X., Welp, L. R., and Medvigy, D.: Climate, soil organic layer, and nitrogen jointly drive forest development after fire in the North American boreal zone, J. Adv. Model. Earth. Sy., 8, 1180–1209, https://doi.org/10.1002/2015MS000576, 2016.
Uhl, C. and Kauffman, J. B.: Deforestation, Fire Susceptibility, and Potential Tree Responses to Fire in the Eastern Amazon, Ecology, 71, 437–449, 1990.
van der Werf, G. R., Morton, D. C., DeFries, R. S., Olivier, J. G. J., Kasibhatla, P. S., Jackson, R. B., Collatz, G. J., and Randerson, J.: CO2 emissions from forest loss, Nat. Geosci., 2, 737–738, 2009.
van der Werf, G. R., Randerson, J. T., Giglio, L., Collatz, G. J., Mu, M., Kasibhatla, P. S., Morton, D. C., DeFries, R. S., Jin, Y., and van Leeuwen, T. T.: Global fire emissions and the contribution of deforestation, savanna, forest, agricultural, and peat fires (1997–2009), Atmos. Chem. Phys., 10, 11707–11735, https://doi.org/10.5194/acp-10-11707-2010, 2010.
Xu, X., Medvigy, D., Powers, J. S., Becknell, J. M., and Guan, K.: Diversity in plant hydraulic traits explains seasonal and inter-annual variations of vegetation dynamics in seasonally dry tropical forests, The New Phytol., 212, 80–95, https://doi.org/10.1111/nph.14009, 2016.