the Creative Commons Attribution 4.0 License.
the Creative Commons Attribution 4.0 License.
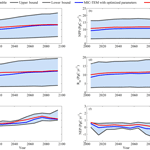
Microbial decomposition processes and vulnerable arctic soil organic carbon in the 21st century
Junrong Zha
Various levels of representations of biogeochemical processes in current biogeochemistry models contribute to a large uncertainty in carbon budget quantification. Here, we present an uncertainty analysis with a process-based biogeochemistry model, the Terrestrial Ecosystem Model (TEM), into which detailed microbial mechanisms were incorporated. Ensemble regional simulations with the new model (MIC-TEM) estimated that the carbon budget of the arctic ecosystems is 76.0±114.8 Pg C during the 20th century, i.e., Pg C under the RCP 2.6 scenario and 94.7±46 Pg C under the RCP 8.5 scenario during the 21st century. Positive values indicate the regional carbon sink while negative values are a source to the atmosphere. Compared to the estimates using a simpler soil decomposition algorithm in TEM, the new model estimated that the arctic terrestrial ecosystems stored 12 Pg less carbon over the 20th century, i.e., 19 and 30 Pg C less under the RCP 8.5 and RCP 2.6 scenarios, respectively, during the 21st century. When soil carbon within depths of 30, 100, and 300 cm was considered as initial carbon in the 21st century simulations, the region was estimated to accumulate 65.4, 88.6, and 109.8 Pg C, respectively, under the RCP 8.5 scenario. In contrast, under the RCP 2.6 scenario, the region lost 0.7, 2.2, and 3 Pg C, respectively, to the atmosphere. We conclude that the future regional carbon budget evaluation largely depends on whether or not adequate microbial activities are represented in earth system models and on the sizes of soil carbon considered in model simulations.
- Article
(2709 KB) - Full-text XML
- Companion paper 2
- Companion paper 3
-
Supplement
(736 KB) - BibTeX
- EndNote
Northern high-latitude soils and permafrost contain more than 1600 Pg carbon (Tarnocai et al., 2009). Climate over this region has warmed in recent decades (Serreze and Francis, 2006) and the increase is 1.5 to 4.5 times the global mean (Holland and Bitz, 2003). Warming-induced changes in carbon cycling are expected to exert large feedbacks on the global climate system (Davidson and Janssens, 2006; Christensen and Christensen, 2007; Oechel et al., 2000).
Warming is expected to accelerate soil C loss by increasing soil respiration but increasing nutrient mineralization, thereby stimulating plant net primary production (NPP) (Mack et al., 2004). Thus, the variation of climate may switch the role of the arctic system between a C sink and a source if soil C loss overtakes NPP (Davidson et al., 2000; Esteban G. Jobbágy and Jackson, 2000). Process-based biogeochemical models such as the Terrestrial Ecosystem Model (TEM; Hayes et al., 2014; Raich and Schlesinger, 1992; McGuire et al., 1992; Zhuang et al., 2001, 2002, 2003, 2010, 2013), Biome-BGC (Running and Coughlan, 1988), CASA (Potter et al., 1993), CENTURY (Parton et al., 1994), and the Biosphere Energy Transfer Hydrology scheme (BETHY) (Knorr, 2000) have been widely used to quantify the response of carbon dynamics to climatic changes (Todd-Brown et al., 2011). An ensemble of process-based model simulations suggests that arctic ecosystems acted as a sink of atmospheric CO2 in recent decades (McGuire et al., 2012; Schimel, 2013). However, the response of this sink to increasing levels of atmospheric CO2 and climate change is still uncertain (Todd-Brown et al., 2013). The IPCC fifth report also shows that land carbon storage is the largest source of uncertainty in the global carbon budget quantification (Ciais et al., 2013).
Much of the uncertainty is also due to the relatively lower levels of representation of ecosystem processes that determine the exchanges of water, energy, and C between land ecosystems and the atmosphere (Wieder et al., 2013) and ignorance of some key biogeochemical mechanisms (Schmidt et al., 2011). For example, heterotrophic respiration (RH) is the primary loss pathway for soil organic carbon (Hanson et al., 2000; Bond-Lamberty and Thomson, 2010), and it generally increases with increasing temperature (Davidson and Janssens, 2006) and moisture levels in well-drained soils (Cook and Orchard, 2008). Moreover, this process is closely related to soil nitrogen mineralization that determines soil N availability and affects gross primary production (Hao et al., 2015). To date, most models treated soil decomposition as a first-order decay process, i.e., CO2 respiration is directly proportional to soil organic carbon. However, it is not clear if these models are robust under changing environmental conditions (Lawrence et al., 2011; Schimel and Weintraub, 2003; Barichivich et al., 2013) since they often ignored the effects of changes in biomass and composition of decomposers, while recent empirical studies have shown that microbial abundance and community play a significant role in soil carbon decomposition (Allison and Martiny, 2008). The control that microbial activity and enzymatic kinetics imposed on soil respiration suggests the need for an explicit representation of microbial physiology, and enzymatic activity, in addition to the direct effects of soil temperature and soil moisture on heterotrophic respiration (Schimel and Weintraub, 2003). Recent mechanistically based models which explicitly incorporated the microbial dynamics and enzyme kinetics that catalyze soil C decomposition have produced notably different results and a closer match to contemporary observations (Wieder et al., 2013; Allison et al., 2010) indicating the need to incorporate these microbial mechanisms into large-scale earth system models to quantify carbon dynamics under future climatic conditions (Wieder et al., 2013; Allison et al., 2010).
This study advanced a microbe-based biogeochemistry model (MIC-TEM) based on the extant TEM (Raich and Schlesinger, 1992; McGuire et al., 1992; Zhuang et al., 2001, 2002, 2003, 2010, 2013; Hao et al., 2015). In MIC-TEM, the heterotrophic respiration is not only a function of soil temperature, soil organic matter (SOM), and soil moisture but also considers the effects of dynamics of microbial biomass and enzyme kinetics (Allison et al., 2010). The verified MIC-TEM was used to quantify the regional carbon dynamics in northern high latitudes (north of 45∘ N) during the 20th and 21st centuries.
2.1 Overview
Below we first briefly describe how we advanced the MIC-TEM by modifying the soil respiration process in TEM (Zhuang et al., 2003) to better represent carbon dynamics in terrestrial ecosystems. Second, we describe how we parameterized and verified the new model using observed net ecosystem exchange data at representative sites and how simulated NPP was evaluated with Moderate Resolution Imaging Spectroradiometer (MODIS) data to demonstrate the reliability of a new model at regional scales. Third, we present how we applied the model to the northern high latitudes for the 20th and 21st centuries. Finally, we introduce how we conducted the sensitivity analysis on initial soil carbon input, using gridded observation-based soil carbon data of three soil depths during the 21st century.
2.2 Model description
TEM is a highly aggregated, large-scale biogeochemical model that estimates the dynamics of carbon and nitrogen fluxes and pool sizes of plants and soils using spatially explicit information on climate, elevation, soils, and vegetation (McGuire et al., 1992; Zhuang et al., 2003, 2010; Melillo et al., 1993). To explicitly consider the effects of microbial dynamics and enzyme kinetics on large-scale carbon dynamics of northern terrestrial ecosystems, we developed MIC-TEM by coupling version 5.0 of TEM (Zhuang et al., 2003, 2010) with a microbial-enzyme module (Hao et al., 2015; Allison et al., 2010). Our modification of the TEM improved the representation of the heterotrophic respiration (RH) from a first-order structure to a more detailed structure (Fig. S1 in the Supplement).
In TEM, heterotrophic respiration RH is calculated as a function of soil organic carbon (SOC), temperature sensitivity of heterotrophic soil respiration (Q10), soil moisture (f(MOIST)), and the gram-specific decomposition constant Kd:
where temp is soil temperature in the top 20 cm (unit: ∘C). CO2 production from the SOC pool is directly proportional to the pool size, and the activity of decomposers only depends on the built-in relationships with soil temperature and moisture (Todd-Brown et al., 2012). Therefore, the changes in microbial community composition or adaption of microbial physiology to new conditions were not represented in TEM. However, current studies indicate that soil C decomposition depends on the activity of biological communities dominated by microbes (Schimel and Weintraub, 2003), implying that the biomass and composition of the decomposer community cannot be ignored (Todd-Brown et al., 2012).
We thus revised the first-order soil C structure in TEM to a second-order structure considering microbial dynamics and enzyme kinetics according to Allison et al. (2010). In MIC-TEM, heterotrophic respiration (RH) is calculated as
where ASSIM and CUE represent microbial assimilation and carbon use efficiency, respectively. ASSIM is modeled with a Michaelis–Menten function:
where DOC is dissolved organic carbon and Vmaxuptake is the maximum velocity of the reaction and calculated using the Arrhenius equation:
where is the pre-exponential coefficient, Eauptake is the activation energy for the reaction (J mol−1), R is the gas constant (8.314 J mol−1 K−1), and temp is the temperature in Celsius under the reaction occurs. Here we used soil temperature in the top 20 cm.
Moreover, Kmuptake is calculated as a linear function of temperature:
Microbial biomass MIC is modeled as
where microbial biomass death (DEATH) and enzyme production (EPROD) are modeled as proportional to microbial biomass with rate constants rdeath and rEnzProd:
where rdeath and rEnzProd are the rate constants of microbial death and enzyme production, respectively.
DOC is part of soil organic carbon:
where MICtoSOC is carbon input ratio as dead microbial biomass to SOC, representing the fraction of microbial death that flows into SOC, and is set as a constant value according to Allison et al. (2010). SOC dynamics are modeled as follows:
where litterfall is estimated as a function of vegetation carbon (Zhuang et al., 2010). The enzymatic decay of SOC is calculated as
where Vmax is the maximum velocity of the reaction and calculated using the Arrhenius equation:
The parameters Km and carbon use efficiency (CUE) are temperature sensitive and calculated as a linear function of temperature between 0 and 50 ∘C:
where CUEslope and CUE0 are parameters for calculating CUE and Kmslope and Km0 are parameters for calculating Km. The values of CUEslope, CUE0, Kmslope, and Km0 were derived from Allison et al. (2010).
ELOSS is also a first-order process, representing the loss of enzymes:
where renzloss is the rate constant of enzyme loss. The enzyme pool (ENZ) is modeled as follows:
Heterotrophic respiration (RH) is an indispensable component of soil respiration (Bond-Lamberty and Thomson, 2010) and is closely coupled with soil nitrogen (N) mineralization, which determines soil N availability, affecting gross primary production (GPP).
2.3 Model parameterization and validation
The variables and parameters of these microbial dynamics and their impacts on soil C decomposition were detailed in Allison et al. (2010) (Table 1). Here we parameterized MIC-TEM for representative ecosystem types in northern high latitudes based on monthly net ecosystem production (NEP, gCm−2 mon−1) measurements from the AmeriFlux network (Davidson et al., 2000) (Table S1 in the Supplement). The results for model parameterization are presented in Fig. S2. Another set of level-4 gap-filled NEP data were used for model validation at site level (Table S2). The site-level monthly climate data of air temperature (∘C), precipitation (mm), and cloudiness (%) were used to drive the model. Gridded MODIS NPP data from 2001 to 2010 were used to evaluate regional NPP simulations. The MODIS NPP data were developed by the MOD17 MODIS project. The product name is Net Primary Production Yearly L4 Global 1 km. The critical parameter used in the MOD17 algorithm is conversion efficiency parameter ε. More information about the MODIS NPP product can be found at https://neo.sci.gsfc.nasa.gov/view.php?datasetId=MOD17A2_M_PSN (last access: January 2017).
In TEM, NPP is calculated as
where GPP is gross primary production and RA is autotrophic respiration. GPP is defined as
where Cmax is the maximum rate of carbon assimilation, PAR is photosynthetically active radiation, and f(phenology) represents the effects of leaf area (Raich and Schlesinger, 1992). The function f(foliage) represents the ratio of canopy leaf biomass relative to maximum leaf biomass (Zhuang et al., 2002). T is monthly air temperature, and f(CO2) represents the effects of elevated atmospheric CO2 (McGuire et al., 1992; Pan et al., 1998). The function f(NA) models the limiting effects of plant nitrogen status on GPP (McGuire et al., 1992; Pan et al., 1998). The function f(FT) represents the effects of freeze–thaw (Zhuang et al., 2003). For detailed GPP and RA calculations, see Zhuang et al. (2003).
The parameterization was conducted with a global optimization algorithm SCE-UA (Shuffled Complex Evolution) (Duan et al., 1994) to minimize the difference between the monthly simulated and measured net ecosystem exchange (NEE) at these sites (Fig. S2). The cost function of the minimization is
where NEPobs,i and NEPsim,i are the observed and simulated NEP, respectively. k is the number of data pairs for comparison. Other parameters used in MIC-TEM were default values from TEM 5.0 (Zhuang et al., 2003, 2010). The optimized parameters were used for model validation and regional extrapolations.
2.4 Regional simulations
Two sets of regional simulations for the 20th century using MIC-TEM and TEM at a spatial resolution of 0.5∘ latitude × 0.5∘ longitude were conducted. Gridded forcing data of monthly air temperature, precipitation, and cloudiness were used, along with other ancillary inputs including historical atmospheric CO2 concentrations, soil texture, elevation, and potential natural vegetation. Climatic inputs vary over time and space, whereas soil texture, elevation, and land cover data are assumed to remain unchanged throughout the 20th century and only vary spatially. The transient climate data during the 20th century were obtained from the Climatic Research Unit (CRU TS3.1) of the University of East Anglia (Harris et al., 2014). The spatially explicit data include potential natural vegetation (Melillo et al., 1993), soil texture (Zhuang et al., 2003), and elevation (Zhuang et al., 2015).
Similarly, two sets of simulations were conducted driven with two contrasting climate change scenarios (RCP 2.6 and RCP 8.5) over the 21st century. The future climate change scenarios were derived from the HadGEM2-ES model, which is a member of the CMIP5 project (https://esgf-node.llnl.gov/search/cmip5/, January 2017). The future atmospheric CO2 concentrations and climate forcing from each of the two climate change scenarios were used. The simulated NPP, RH, and NEP by both models (TEM 5.0 and MIC-TEM) were analyzed. The positive NEP represents a CO2 sink from the atmosphere to terrestrial ecosystems, while a negative value represents a source of CO2 from terrestrial ecosystems to the atmosphere.
Moreover, in order to test the parameter uncertainty in our model, we conducted the regional simulations with 50 sets of parameters for both historical and future studies. The 50 sets of parameters were obtained according to the method in Tang and Zhuang (2008). The upper and lower bounds of the regional estimations were generated based on these simulations.
2.5 Sensitivity to initial soil carbon input
Future carbon dynamics can be affected by varying initial soil carbon amount. In the standard simulation of TEM, the initial soil carbon amount for transient simulations was obtained from equilibrium and spin-up periods directly for each grid cell in the region. To test the sensitivity to the initial soil carbon amount in transient simulations for the 21st century, we used empirical soil organic carbon data extracted from the Northern Circumpolar Soil Carbon Database (NCSCD) (Tarnocai et al., 2009), as the initial soil carbon amount. The soil carbon data products for three different depths of 30, 100, and 300 cm were used. The sensitivity test was conducted for transient simulations under the RCP 2.6 and RCP 8.5 scenarios. To avoid the instability of the C : N ratio caused by replacing the initial soil carbon pool with observed data at the beginning of transient period, initial soil nitrogen values were also generated based on the soil carbon data and corresponding C : N ratio map for transient simulations (Zhuang et al., 2003; Raich and Schlesinger, 1992).
3.1 Model verification at site and regional levels
With the optimized parameters, MIC-TEM reproduces the carbon dynamics well for alpine tundra, boreal forest, temperate coniferous forest, temperate deciduous forest, grasslands, and wet tundra with R2 ranging from 0.70 for Ivotuk to 0.94 for the Bartlett Experimental Forest (Fig. S3, Table S3). In general, the model performs better for forest ecosystems than for tundra ecosystems. The temporal NPP from 2001 to 2010 simulated by MIC-TEM and TEM was compared with MODIS NPP data (Fig. S4). Pearson correlation coefficients are 0.52 (MIC-TEM and MODIS) and 0.34 (TEM and MODIS). NPP simulated by MIC-TEM showed higher spatial correlation coefficients with MODIS data than TEM (Fig. S5). By considering more detailed microbial activities, the heterotrophic respiration is more adequately simulated using the MIC-TEM. The simulated differences in soil decomposition result in different levels of soil-available nitrogen, which influences the nitrogen uptake by plants, the rate of photosynthesis, and NPP. The spatial correlation coefficient between NPP simulated by MIC-TEM and MODIS is close to 1 in most study areas, suggesting the reliability of MIC-TEM at the regional scale.
3.2 Regional carbon dynamics during the 20th century
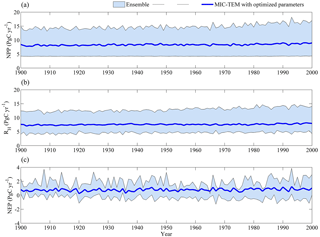
Figure 1Simulated annual net primary production (NPP, a), heterotrophic respiration (RH, b), and net ecosystem production (NEP, c) by MIC-TEM with an ensemble of parameters.
The equifinality of the parameters in MIC-TEM was considered in our ensemble regional simulations to measure the parameter uncertainty (Tang and Zhuang, 2008). Here and below, the ensemble means and the inter-simulation standard deviations are shown to measure the uncertainty unless otherwise specified. These ensemble simulations indicated that the northern high latitudes go from acting as a carbon source of 38.9 Pg C to being a carbon sink of 190.8 Pg C by different ensemble members, with a mean of 64.2±21.4 Pg at the end of the 20th century, while the simulation with the optimized parameters estimates a regional carbon sink of 77.6 Pg with an interannual standard deviation of 0.21 Pg C yr−1 during the 20th century (Fig. 1). Simulated regional NEP with optimized parameters using TEM and MIC-TEM showed an increasing trend throughout the 20th century except for a slight decrease during the 1960s (Fig. 2). The spatial distributions of NEP simulated by MIC-TEM for different periods in the 20th century also show an increasing trend (Fig. 3). Positive values of NEP represent sinks of CO2 into terrestrial ecosystems, while negative values represent sources of CO2 to the atmosphere. From 1900 onwards, both models estimated a regional carbon sink during the 20th century. With optimized parameters, TEM estimated higher NPP and RH at 0.6 and 0.3 Pg C yr−1 than MIC-TEM, respectively, at the end of the 20th century (Fig. 2). MIC-TEM estimated a carbon sink increase from 0.64 to 0.83 Pg C yr−1 during the century while the estimated increase by TEM was much higher (0.28 Pg C yr−1) (Fig. 2). At the end of the century, MIC-TEM-estimated NEP reached 1.0 Pg C yr−1 in comparison with TEM estimates of 0.3 Pg C yr−1. TEM-estimated NPP and RH are 0.5 and 0.3 Pg C yr−1 higher, respectively. As a result, TEM estimated that the region accumulated 11.4 Pg more carbon than MIC-TEM. Boreal forests are a major carbon sink at 0.55 and 0.63 Pg C yr−1 estimated by MIC-TEM and TEM, respectively. Alpine tundra contributes the least sink. Overall, TEM overestimated the sink by 12.5 % in comparison to MIC-TEM for forest ecosystems and 16.7 % for grasslands. For wet tundra and alpine tundra, TEM overestimated about 20 % and 33 %, respectively, in comparison with MIC-TEM (Table 2).
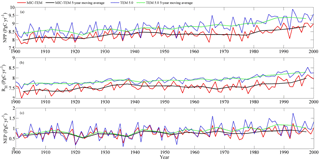
Figure 2Simulated annual net primary production (NPP, a), heterotrophic respiration (RH, b), and net ecosystem production (NEP, c) by MIC-TEM and TEM.
3.3 Regional carbon dynamics during the 21st century
Simulated regional annual NPP and RH increase under the RCP 8.5 scenario with both models (Fig. 4). With optimized parameters, MIC-TEM-estimated NPP increases from 9.2 in the 2000s to 13.2 Pg C yr−1 in the 2090s, while TEM-predicted NPP is 2.0 Pg C yr−1 higher in the 2000s and 0.3 Pg C yr−1 higher in the 2090s (Fig. 4). Similarly, TEM also overestimated RH by 1.7 Pg C yr−1 in the 2000s and by 0.25 Pg C yr−1 in the 2090s, respectively (Fig. 4). As a result, the regional sink increases from 0.53 Pg C yr−1 in the 2000s to 1.4 Pg C yr−1 in the 2070s and then decreases to 1.1 Pg C yr−1 in the 2090s as estimated by MIC-TEM (Fig. 4). Given the uncertainty in parameters, MIC-TEM predicted that the region acts as a carbon sink of 48.7 to 140.7 Pg, with a mean of 71.7±26.6 Pg at the end of 21st century, while the simulation with optimized parameters estimates a regional carbon source of 79.5 Pg with an interannual standard deviation of 0.37 Pg C yr−1 during the 21st century (Fig. 4). TEM predicted a similar trend for NEP, which overestimated the carbon sink with a magnitude of 19.2 Pg compared to the simulation by MIC-TEM with optimized parameters. Under the RCP 2.6 scenario (Fig. 4), the increase in NPP and RH is smaller from 2000 to 2100 compared to the simulation under the RCP 8.5. MIC-TEM predicted that NPP increases from 9.1 to 10.9 Pg C yr−1, and TEM estimated it to be 1.6 Pg C yr−1 higher at the beginning and 0.9 Pg C yr−1 higher in the end of the 21st century (Fig. 4). Consequently, MIC-TEM-predicted NEP fluctuates between sinks and sources during the century, being neutral before 2070 and a source of between −0.2 and −0.3 Pg C yr−1 after the 2070s. As a result, the region acts as a carbon source of 1.6 Pg C with an interannual standard deviation of 0.24 Pg C yr−1 estimated with MIC-TEM and a sink of 27.6 Pg C with an interannual standard deviation of 0.2 Pg C yr−1 estimated with TEM during the century (Fig. 4). When considering the uncertainty source of parameters, MIC-TEM predicted that the region goes from acting as a carbon source of 64.8 Pg C to being a carbon sink of 58.6 Pg C during the century with a mean of Pg at the end of 21st century (Fig. 4).
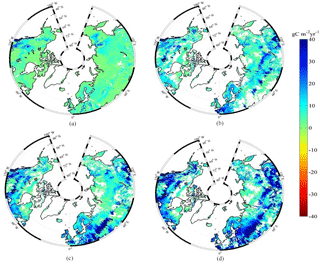
Figure 3Spatial distribution of NEP simulated by MIC-TEM for the periods (a) 1900–1930, (b) 1931–1960, (c) 1961–1990, and (d) 1991–2000. Positive values of NEP represent sinks of CO2 into terrestrial ecosystems, while negative values represent sources of CO2 to the atmosphere.
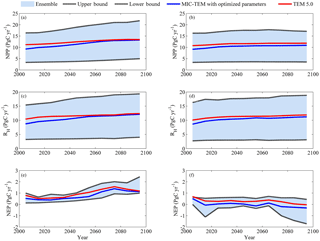
Figure 4Predicted changes in carbon fluxes: (a, b) NPP, (c, d) RH, and (e, f) NEP for all land areas north of 45∘ N in response to transient climate change under (a, c, e) the RCP 8.5 scenario and (b, d, f) the RCP 2.6 scenario with MIC-TEM and TEM 5.0. The decadal running mean is applied. The blue area represents the upper and lower bounds of simulations.
3.4 Model sensitivity to initial soil carbon
Under the RCP 2.6, without replacing the initial soil carbon with inventory-based estimates (Tarnocai et al., 2009) in model simulations, TEM estimated that the regional SOC is 604.2 Pg C and accumulates an additional 12.1 Pg C during the 21st century. When using estimated soil carbon (Tarnocai et al., 2009) within depths of 30, 100, and 300 cm as initial pools in simulations, TEM predicted that regional SOC is 429.5, 689.3, and 1003.4 Pg C in 2000 and increases by 9.9, 16.0, and 22.8 Pg C at the end of the 21st century, and the regional cumulative carbon sink is 20.4, 34.0, and 48.1 Pg C, respectively, during the century. In contrast, using the same inventory-based SOC estimates, MIC-TEM projected that the region goes from acting as a cumulative carbon sink to being a source at 0.7, 2.2, and 3.0 Pg C. Under the RCP 8.5, both models predicted that the region acts as a carbon sink, regardless of the magnitudes of initial soil carbon pools used, with a TEM-projected sink of 71.7, 120, and 155.6 Pg C and a much smaller cumulative sink of 65.4, 88.6, and 109.8 Pg C estimated with MIC-TEM (Table 3).
During the last few decades, a greening accompanying warming and rising atmospheric CO2 in the northern high latitudes (>45∘ N) has been documented (McGuire et al., 1995; McGuire and Hobbie, 1997; Chapin and Starfield, 1997; Stow et al., 2004; Callaghan et al., 2005; Tape et al., 2006). The large stocks of carbon contained in the region (Tarnocai et al., 2009) are particularly vulnerable to climate change (Schuur et al., 2008; McGuire et al., 2009). To date, the degree to which the ecosystems may serve as a source or a sink of C in the future is still uncertain (McGuire et al., 2009; Wieder et al., 2013). Therefore, accurate models are essential for predicting carbon–climate feedbacks in the future (Todd-Brown et al., 2013). Our regional simulations indicate that the region is currently a carbon sink, which is consistent with many previous studies (White et al., 2000; Houghton, 2007), and this sink will grow under the RCP 8.5 scenario but shift to a carbon source under the RCP 2.6 scenario by 2100. MIC-TEM shows a higher correlation between NPP and soil temperature (R=0.91) than TEM (R=0.82), suggesting that MIC-TEM is more sensitive to environmental changes (Table S4).
Our regional estimates of carbon fluxes by MIC-TEM are within the uncertainty range from other existing studies. For instance, Zhuang et al. (2003) estimated the region to be a sink of 0.9 Pg C yr−1 in extratropical ecosystems for the 1990s, which is similar to our estimation of 0.83 Pg C yr−1 by MIC-TEM. White et al. (2000) estimated that, during the 1990s, regional NEP above 50∘ N is 0.46 Pg C yr−1 while Qian et al. (2010) estimated that NEP increased from 0 to 0.3 Pg C yr−1 for the high-latitude region above 60∘ N during last century and reached 0.25 Pg C yr−1 during the 1990s. White et al. (2000) predicted that, from 1850 to 2100, the region accumulated 134 Pg C in terrestrial ecosystems, in comparison with our estimates of 77.6 Pg C with MIC-TEM and 89 Pg C with TEM. Our projection of a weakening sink during the second half of the 21st century is consistent with previous model studies (Schaphoff et al., 2013). Our predicted trend of NEP is very similar to the finding of White et al. (2000), indicating that NEP increases from 0.46 Pg C yr−1 in the 2000s and reaches 1.5 Pg C yr−1 in the 2070s, then decreases to 0.6 Pg C yr−1 in the 2090s.
The MIC-TEM-simulated NEP generally agrees with the observations. However, model simulations still deviate from the observed data, especially for tundra ecosystems. The deviation may be due to the uncertainty or errors in the observed data, which do not constrain the model parameters well. Uncertain driving data such as temperature and precipitation are also a source of uncertainty for transient simulations. In addition, we assumed that vegetation will not change during the transient simulation. However, over the past few decades in the northern high latitudes, temperature increases have led to vegetation changes (Hansen et al., 2006), including latitudinal tree line advance (Lloyd, 2005) and increasing shrub density (Sturm et al., 2001). Vegetation can shift from one type to another because of competition for light, N, and water (White et al., 2000). For example, needleleaf trees tend to replace tundra gradually in response to warming. In some areas, forests even moved several hundreds of kilometers within 100 years (Gear and Huntley, 1991). The vegetation changes will affect carbon cycling in these ecosystems. In addition, we have not yet considered the effects of management of agricultural lands (Cole et al., 1997), but Zhuang et al. (2003) showed that the changes in agricultural land use in northern high latitudes have been small.
The largest limitation on this study is that we have not explicitly considered the fire effects. Warming in the northern high latitudes could favor fire in its frequency, intensity, seasonality, and extent (Kasischke and Turetsky, 2006; Johnstone and Kasischke, 2005; Soja et al., 2007; Randerson et al., 2006; Bond-Lamberty et al., 2007). Fire has profound effects on northern forest ecosystems, altering the N cycle and water and energy exchanges between the atmosphere and ecosystems. An increase in wildfires will destroy most of aboveground biomass and consume organic soils, resulting in less carbon uptake by vegetation (Harden et al., 2000), leading to a net release of carbon in the short term. However, a suite of biophysical mechanisms of ecosystems, including post-fire increase in the surface albedo and rates of biomass accumulation, may, in turn, exert a negative feedback on climate warming (Amiro et al., 2006; Goetz et al., 2007), further influencing the carbon exchanges between ecosystems and the atmosphere.
Moreover, carbon uptake in land ecosystems depends on new plant growth, which connects closely with the availability of nutrients such as mineral nitrogen. Recent studies have shown that when soil nitrogen is in short supply, most terrestrial plants form symbiotic relationships with fungi; hyphae provide nitrogen to plants, and in return, plants provide sugar to fungi (Hobbie and Hobbie, 2008, 2006; Schimel and Hättenschwiler, 2007). This symbiotic relationship has not been considered in our current modeling, which may lead to a large uncertainty in our quantification of carbon and nitrogen dynamics.
A shift in microbial community structure was not considered in our model, which could affect the temperature sensitivity of heterotrophic respiration (Stone et al., 2012). The Michaelis–Menten constant (Km) could also adapt to climate warming, and it may increase more significantly with increasing temperature in cold-adapted enzymes than in warm-adapted enzymes (German et al., 2012; Somero, 2004; Dong and Somero, 2009). Carbon use efficiency (CUE) is also a controversial parameter in our model. Empirical studies in soils suggest that microbial CUE declines by at least 0.009 ∘C−1 (Steinweg et al., 2008), while other studies find that CUE is invariant with temperature (López-Urrutia and Morán, 2007). Another key microbial trait lacking in our modeling is microbial dormancy (He et al., 2015). Dormancy is a common, bet-hedging strategy used by microorganisms when environmental conditions limit their growth and reproduction (Lennon and Jones, 2011). Microorganisms in dormancy are not able to drive biogeochemical processes such as soil CO2 production, and, therefore, only active microorganisms should be involved in utilizing substrates in soils (Blagodatskaya and Kuzyakov, 2013). Many studies have indicated that soil respiration responses to environmental conditions are more closely associated with the active portion of microbial biomass than total microbial biomass (Hagerty et al., 2014; Schimel and Schaeffer, 2012; Steinweg et al., 2013). Thus, the ignorance of microbial dormancy could fail to distinguish between microbes with different physiological states, introducing uncertainties into our carbon estimation.
This study used a more detailed microbial biogeochemistry model to investigate the carbon dynamics in the region for the past and this century. Regional simulations using MIC-TEM indicated that, over the 20th century, the region is a sink of 77.6 Pg C. This sink could reach 79.5 Pg C under the RCP 8.5 scenario or shift to a carbon source of 1.6 Pg under the RCP 2.6 scenario during the 21st century. On the other hand, traditional TEM overestimated the carbon sink under the RCP 8.5 scenario with a magnitude of 19.2 Pg than MIC-TEM and predicted that this region would act as a carbon sink with a magnitude of 27.6 Pg under the RCP 2.6 scenario during the 21st century. Using recent soil carbon stock data as initial soil carbon in model simulations, the region was estimated to shift from a carbon sink to a source, with total carbon release at 0.7–3 Pg by 2100 depending on initial soil carbon pools at different soil depths under the RCP 2.6 scenario. In contrast, the region acts as a carbon sink at 55.4–99.8 Pg in the 21st century under the RCP 8.5 scenario. Without considering more detailed microbial processes, models estimated that the region acts as a carbon sink under both scenarios. Under the RCP 2.6 scenario, the cumulative sink ranges from 9.9 to 22.8 Pg C. Under the RCP 8.5 scenario, the cumulative sink is even larger at 71.7–155.6 Pg C. This study indicated that more detailed microbial physiology-based biogeochemistry models estimate carbon dynamics very differently from using a relatively simple microbial decomposition-based model. The comparison with satellite products or other estimates for the 20th century suggests that the more detailed microbial decomposition should be considered to adequately quantify C dynamics in northern high latitudes.
All data used in this study are available from the authors upon request.
The supplement related to this article is available online at: https://doi.org/10.5194/bg-15-5621-2018-supplement.
QZ designed the study. JZ conducted model development, simulation, and analysis. JZ and QZ wrote the paper.
The authors declare that they have no conflict of interest.
This research was supported by an NSF project (IIS-1027955), a DOE project
(DE-SC0008092), and a NASA LCLUC project (NNX09AI26G) to Qianlai Zhuang. We
acknowledge the Rosen High Performance Computing Center at Purdue for
computing support. We thank the National Snow and Ice Data center for
providing Global Monthly EASE-Grid Snow Water Equivalent data and the
National Oceanic and Atmospheric Administration for North American Regional
Reanalysis (NARR). We also acknowledge the World Climate Research Programme's
Working Group on Coupled Modeling Intercomparison Project CMIP5, and we thank
the climate modeling groups for producing and making available their model
output.
Edited by: Alexey V. Eliseev
Reviewed by: two anonymous referees
Allison, S. D. and Martiny, J. B.: Colloquium paper: resistance, resilience, and redundancy in microbial communities, P. Natl. Acad. Sci. USA, 105, 11512–11519, https://doi.org/10.1073/pnas.0801925105, 2008.
Allison, S. D., Wallenstein, M. D., and Bradford, M. A.: Soil-carbon response to warming dependent on microbial physiology, Nat. Geosci., 3, 336–340, https://doi.org/10.1038/ngeo846, 2010.
Amiro, B. D., Orchansky, A. L., Barr, A. G., Black, T. A., Chambers, S. D., Chapin Iii, F. S., Goulden, M. L., Litvak, M., Liu, H. P., McCaughey, J. H., McMillan, A., and Randerson, J. T.: The effect of post-fire stand age on the boreal forest energy balance, Agr. Forest Meteorol., 140, 41–50, https://doi.org/10.1016/j.agrformet.2006.02.014, 2006.
Barichivich, J., Briffa, K. R., Myneni, R. B., Osborn, T. J., Melvin, T. M., Ciais, P., Piao, S., and Tucker, C.: Large-scale variations in the vegetation growing season and annual cycle of atmospheric CO2 at high northern latitudes from 1950 to 2011, Glob. Change Biol., 19, 3167–3183, https://doi.org/10.1111/gcb.12283, 2013.
Blagodatskaya, E. and Kuzyakov, Y.: Active microorganisms in soil: Critical review of estimation criteria and approaches, Soil Biol. Biochem., 67, 192–211, https://doi.org/10.1016/j.soilbio.2013.08.024, 2013.
Bond-Lamberty, B. and Thomson, A.: Temperature-associated increases in the global soil respiration record, Nature, 464, 579–582, https://doi.org/10.1038/nature08930, 2010.
Bond-Lamberty, B., Peckham, S. D., Ahl, D. E., and Gower, S. T.: Fire as the dominant driver of central Canadian boreal forest carbon balance, Nature, 450, 89–92, https://doi.org/10.1038/nature06272, 2007.
Callaghan, T., Björn, L. O., Chernov, Y., Chapin, T., Christensen, T. R., Huntley, B., Ims, R., Jolly, D., Jonasson, S., Matveyeva, N., Panikov, N., Oechel, W., and Shaver, G.: Chapter 7: Arctic tundra and polar desert ecosystems, Arctic climate impact assessment, 243–352, ACIA Overview report. Cambridge University Press, 1020 pp., 2005.
Chapin, F. S. and Starfield, A. M.: Time lags and novel ecosystems in response to transient climatic change in arctic Alaska, Climatic Change, 35, 449–461, 1997.
Christensen, J. H. and Christensen, O. B.: A summary of the PRUDENCE model projections of changes in European climate by the end of this century, Climatic Change, 81, 7–30, https://doi.org/10.1007/s10584-006-9210-7, 2007.
Ciais, P., Sabine, C., Bala, G., Bopp, L., Brovkin, V., Canadell, J., Chhabra, A., DeFries, R., Galloway, J., Heimann, M., Jones, C., Quéré, C. L., Myneni, R. B., Piao, S., and Thornton, P.: Carbon and other biogeochemical cycles, Climate change 2013: the physical science basis. Contribution of Working Group I to the Fifth Assessment Report of the Intergovernmental Panel on Climate Change, 465–570, 2013.
Cole, C. V., Duxbury, J., Freney, J., Heinemeyer, O., Minami, K., Mosier, A., Paustian, K., Rosenberg, N., Sampson, N., Sauerbeck, D., and Zhao, Q.: Global estimates of potential mitigation of greenhouse gas emissions by agriculture, Nutr. Cycl. Agroecosys., 49, 221–228, 1997.
Cook, F. J. and Orchard, V. A.: Relationships between soil respiration and soil moisture, Soil Biol. Biochem., 40, 1013–1018, https://doi.org/10.1016/j.soilbio.2007.12.012, 2008.
Davidson, E. A. and Janssens, I. A.: Temperature sensitivity of soil carbon decomposition and feedbacks to climate change, Nature, 440, 165–173, https://doi.org/10.1038/nature04514, 2006.
Davidson, E. A., Trumbore, S. E., and Amundson, R.: Biogeochemistry: soil warming and organic carbon content, Nature, 408, 789–790, https://doi.org/10.1038/35048672, 2000.
Dong, Y. and Somero, G. N.: Temperature adaptation of cytosolic malate dehydrogenases of limpets (genus Lottia): differences in stability and function due to minor changes in sequence correlate with biogeographic and vertical distributions, J. Exp. Biol., 212, 169–177, https://doi.org/10.1242/jeb.024505, 2009.
Duan, Q., Sorooshian, S., and Gupta, V. K.: Optimal use of the SCE-UA global optimization method for calibrating watershed models, J. Hydrol., 158, 265–284, 1994.
Esteban G. Jobbágy, and Jackson, R. B.: The vertical distribution of soil organic carbon and its relation to climate and vegetation, Ecol. Appl., 10, 423–436, 2000.
Gear, A. J. and Huntley, B.: Rapid changes in the range limits of Scots pine 4000 years ago, Science, 251, 544–547, 1991.
German, D. P., Marcelo, K. R. B., Stone, M. M., and Allison, S. D.: The Michaelis-Menten kinetics of soil extracellular enzymes in response to temperature: a cross-latitudinal study, Glob. Change Biol., 18, 1468–1479, https://doi.org/10.1111/j.1365-2486.2011.02615.x, 2012.
Goetz, S. J., Mack, M. C., Gurney, K. R., Randerson, J. T., and Houghton, R. A.: Ecosystem responses to recent climate change and fire disturbance at northern high latitudes: observations and model results contrasting northern Eurasia and North America, Environ. Res. Lett., 2, 045031, https://doi.org/10.1088/1748-9326/2/4/045031, 2007.
Hagerty, S. B., van Groenigen, K. J., Allison, S. D., Hungate, B. A., Schwartz, E., Koch, G. W., Kolka, R. K., and Dijkstra, P.: Accelerated microbial turnover but constant growth efficiency with warming in soil, Nat. Clim. Change, 4, 903–906, https://doi.org/10.1038/nclimate2361, 2014.
Hansen, J., Sato, M., Ruedy, R., Lo, K., Lea, D. W., and Medina-Elizade, M.: Global temperature change, P. Natl. Acad. Sci. USA, 103, 14288–14293, https://doi.org/10.1073/pnas.0606291103, 2006.
Hanson, P. J., Edwards, N. T., Garten, C. T., and Andrews, J. A.: Separating root and soil microbial contributions to soil respiration: A review of methods and observations, Biogeochemistry, 48, 115–146, 2000.
Hao, G., Zhuang, Q., Zhu, Q., He, Y., Jin, Z., and Shen, W.: Quantifying microbial ecophysiological effects on the carbon fluxes of forest ecosystems over the conterminous United States, Climatic Change, 133, 695–708, https://doi.org/10.1007/s10584-015-1490-3, 2015.
Harden, J. W., Trumbore, S. E., Stocks, B. J., Hirsch, A., Gower, S. T., O'neill, K. P., and Kasischke, E. S.: The role of fire in the boreal carbon budget, Glob. Change Biol., 6, 174–184, 2000.
Harris, I., Jones, P. D., Osborn, T. J., and Lister, D. H.: Updated high-resolution grids of monthly climatic observations – the CRU TS3.10 Dataset, Int. J. Climatol., 34, 623–642, https://doi.org/10.1002/joc.3711, 2014.
Hayes, D. J., Kicklighter, D. W., McGuire, A. D., Chen, M., Zhuang, Q., Yuan, F., Melillo, J. M., and Wullschleger, S. D.: The impacts of recent permafrost thaw on land–atmosphere greenhouse gas exchange, Environ. Res. Lett., 9, 045005, https://doi.org/10.1088/1748-9326/9/4/045005, 2014.
He, Y., Yang, J., Zhuang, Q., Harden, J. W., McGuire, A. D., Liu, Y., Wang, G., and Gu, L.: Incorporating microbial dormancy dynamics into soil decomposition models to improve quantification of soil carbon dynamics of northern temperate forests, J. Geophys. Res.-Biogeo., 120, 2596–2611, https://doi.org/10.1002/2015jg003130, 2015.
Hobbie, J. E. and Hobbie, E. A.: 15N in symbiotic fungi and plants estimates nitrogen and carbon flux rates in Arctic tundra, Ecology, 87, 816–822, 2006.
Hobbie, E. A. and Hobbie, J. E.: Natural Abundance of 15N in Nitrogen-Limited Forests and Tundra Can Estimate Nitrogen Cycling Through Mycorrhizal Fungi: A Review, Ecosystems, 11, 815–830, https://doi.org/10.1007/s10021-008-9159-7, 2008.
Holland, M. M. and Bitz, C. M.: Polar amplification of climate change in coupled models, Clim. Dynam., 21, 221–232, https://doi.org/10.1007/s00382-003-0332-6, 2003.
Houghton, R. A.: Balancing the Global Carbon Budget, Annu. Rev. Earth Pl. Sc., 35, 313–347, https://doi.org/10.1146/annurev.earth.35.031306.140057, 2007.
Johnstone, J. F. and Kasischke, E. S.: Stand-level effects of soil burn severity on postfire regeneration in a recently burned black spruce forest, Can. J. Forest Res., 35, 2151–2163, https://doi.org/10.1139/x05-087, 2005.
Kasischke, E. S. and Turetsky, M. R.: Recent changes in the fire regime across the North American boreal region – Spatial and temporal patterns of burning across Canada and Alaska, Geophys. Res. Lett., 33, L09703, https://doi.org/10.1029/2006gl025677, 2006.
Knorr, W.: Annual and interannual CO2 exchanges of the terrestrial biosphere: process-based simulations and uncertainties, Global Ecol. Biogeogr., 9, 225–252, 2000.
Lawrence, D. M., Oleson, K. W., Flanner, M. G., Thornton, P. E., Swenson, S. C., Lawrence, P. J., Zeng, X., Yang, Z.-L., Levis, S., Sakaguchi, K., Bonan, G. B., and Slater, A. G.: Parameterization improvements and functional and structural advances in Version 4 of the Community Land Model, J. Adv. Model. Earth Sy., 3, M03001, https://doi.org/10.1029/2011ms000045, 2011.
Lennon, J. T. and Jones, S. E.: Microbial seed banks: the ecological and evolutionary implications of dormancy, Nature reviews, Microbiology, 9, 119–130, https://doi.org/10.1038/nrmicro2504, 2011.
Lloyd, A. H.: Ecological histories from Alaskan tree lines provide insight into future change, Ecology, 86, 1687–1695, 2005.
López-Urrutia, A., and Morán, X. A. G.: Resource limitation of bacterial production distorts the temperature dependence of oceanic carbon cycling, Ecology, 88, 817–822, 2007.
Mack, M. C., Schuur, E. A. G., Bret-Harte, M. S., Shaver, G. R., and Chapin III, F. S. C.: Ecosystem carbon storage in arctic tundra reduced by long-term nutrient fertilization, Nature, 431, 440–443, 2004.
McGuire, A. D. and Hobbie, J. E.: Global climate change and the equilibrium responses of carbon storage in arctic and subarctic regions, in: Modeling the Arctic system: A workshop report on the state of modeling in the Arctic System Science program, 53–54, 1997.
McGuire, A. D., Melillo, J. M., Joyce, L. A., Kicklighter, D. W., Grace, A. L., Moore III, B. M., and Vorosmarty, C. J.: Interactions between carbon and nitrogen dynamics in estimating net primary productivity for potential vegetation in North America, Global Biogeochem. Cy., 6, 101–124, 1992.
McGuire, A. D., Melillo, J. M., Kicklighter, D. W., and Joyce, L. A.: Equilibrium responses of soil carbon to climate change: Empirical and process-based estimates, J. Biogeogr., 22, 785–796, 1995.
McGuire, A. D., Anderson, L. G., Christensen, T. R., Dallimore, S., Guo, L., Hayes, D. J., Heimann, M., Lorenson, T. D., Macdonald, R. W., and Roulet, N.: Sensitivity of the carbon cycle in the Arctic to climate change, Ecol. Monogr., 79, 523–555, 2009.
McGuire, A. D., Christensen, T. R., Hayes, D., Heroult, A., Euskirchen, E., Kimball, J. S., Koven, C., Lafleur, P., Miller, P. A., Oechel, W., Peylin, P., Williams, M., and Yi, Y.: An assessment of the carbon balance of Arctic tundra: comparisons among observations, process models, and atmospheric inversions, Biogeosciences, 9, 3185–3204, https://doi.org/10.5194/bg-9-3185-2012, 2012.
Melillo, J. M., McGuire, A. D., Kicklighter, D. W., Moore III, B., Vorosmarty, C. J., and Schloss, A. L.: Global climate change and terrestrial net primary production, Nature, 363, 234–240, https://doi.org/10.1038/363234a0, 1993.
Oechel, W. C., Vourlitis, G. L., Hastings, S. J., Zulueta, R. C., Hinzman, L., and Kane, D.: Acclimation of ecosystem CO2 exchange in the Alaskan Arctic in response to decadal climate warming, Nature, 406, 978–981, https://doi.org/10.1038/35023137, 2000.
Pan, Y., McGuire, A. D., Melillo, J. M., Kicklighter, D. W., Sitch, S., and Prentice, I. C.: A biogeochemistry-based dynamic vegetation model and its application along a moisture gradient in the continental United States, J. Vegetation Sci., 13, 369–382, 2002.
Parton, W. J., Ojima, D. S., Cole, C. V., and Schimel, D. S.: A general model for soil organic matter dynamics: sensitivity to litter chemistry, texture and management, SSSA Spec. Publ., 39, 147–167, 1994.
Potter, C. S., Randerson, J. T., Field, C. B., Matson, P. A., Vitousek, P. M., Mooney, H. A., and Klooster, S. A.: Terrestrial ecosystem production: a process model based on global satellite and surface data, Global Biogeochem. Cy., 7, 811–841, 1993.
Qian, H., Joseph, R., and Zeng, N.: Enhanced terrestrial carbon uptake in the Northern High Latitudes in the 21st century from the Coupled Carbon Cycle Climate Model Intercomparison Project model projections, Glob. Change Biol., 16, 641–656, https://doi.org/10.1111/j.1365-2486.2009.01989.x, 2010.
Raich, J. W. and Schlesinger, W. H.: The global carbon dioxide flux in soil respiration and its relationship to vegetation and climate, Tellus B, 44, 81–99, 1992.
Randerson, J. T., Liu, H., Flanner, M. G., Chambers, S. D., Jin, Y., Hess, P. G., Pfister, G., Mack, M. C., Treseder, K. K., Welp, L. R., Chapin, F. S., Harden, J. W., Goulden, M. L., Lyons, E., Neff, J. C., Schuur, E. A. G., and Zender, C. S.: The impact of boreal forest fire on climate warming, Science, 314, 1130–1132, 2006.
Running, S. W. and Coughlan, J. C.: A general model of forest ecosystem processes for regional applications I. Hydrologic balance, canopy gas exchange and primary production processes, Ecol. Model., 42, 125–154, 1988.
Schaphoff, S., Heyder, U., Ostberg, S., Gerten, D., Heinke, J., and Lucht, W.: Contribution of permafrost soils to the global carbon budget, Environ. Res. Lett., 8, 014026, https://doi.org/10.1088/1748-9326/8/1/014026, 2013.
Schimel, J. P. and Weintraub, M. N.: The implications of exoenzyme activity on microbial carbon and nitrogen limitation in soil: a theoretical model, Soil Biol. Biochem., 35, 549–563, https://doi.org/10.1016/s0038-0717(03)00015-4, 2003.
Schimel, J. P. and Hättenschwiler, S.: Nitrogen transfer between decomposing leaves of different N status, Soil Biol. Biochem., 39, 1428–1436, https://doi.org/10.1016/j.soilbio.2006.12.037, 2007.
Schimel, J. P. and Schaeffer, S. M.: Microbial control over carbon cycling in soil, Front. Microbiol., 3, 348, https://doi.org/10.3389/fmicb.2012.00348, 2012.
Schmidt, M. W., Torn, M. S., Abiven, S., Dittmar, T., Guggenberger, G., Janssens, I. A., Kleber, M., Kogel-Knabner, I., Lehmann, J., Manning, D. A., Nannipieri, P., Rasse, D. P., Weiner, S., and Trumbore, S. E.: Persistence of soil organic matter as an ecosystem property, Nature, 478, 49–56, https://doi.org/10.1038/nature10386, 2011.
Schuur, E. A. G., Bockheim, J., Canadell, J. G., Euskirchen, E., Field, C. B., Goryachkin, S. V., Hagemann, S., Kuhry, P., Lafleur, P. M., Lee, H., and Mazhitova, G.: Vulnerability of permafrost carbon to climate change: Implications for the global carbon cycle, BioScience, 58, 701–714, 2008.
Serreze, M. C. and Francis, J. A.: The Arctic on the fast track of change, Weather, 61, 65–69, 2006.
Soja, A. J., Tchebakova, N. M., French, N. H. F., Flannigan, M. D., Shugart, H. H., Stocks, B. J., Sukhinin, A. I., Parfenova, E. I., Chapin, F. S., and Stackhouse, P. W.: Climate-induced boreal forest change: Predictions versus current observations, Global Planet. Change, 56, 274–296, https://doi.org/10.1016/j.gloplacha.2006.07.028, 2007.
Somero, G. N.: Adaptation of enzymes to temperature: searching for basic “strategies”, Comp. Biochem. Phys. B, 139, 321–333, https://doi.org/10.1016/j.cbpc.2004.05.003, 2004.
Steinweg, J. M., Plante, A. F., Conant, R. T., Paul, E. A., and Tanaka, D. L.: Patterns of substrate utilization during long-term incubations at different temperatures, Soil Biol. Biochem., 40, 2722–2728, https://doi.org/10.1016/j.soilbio.2008.07.002, 2008.
Steinweg, J. M., Dukes, J. S., Paul, E. A., and Wallenstein, M. D.: Microbial responses to multi-factor climate change: effects on soil enzymes, Front. Microbiol., 4, 146, https://doi.org/10.3389/fmicb.2013.00146, 2013.
Stone, M. M., Weiss, M. S., Goodale, C. L., Adams, M. B., Fernandez, I. J., German, D. P., and Allison, S. D.: Temperature sensitivity of soil enzyme kinetics under N-fertilization in two temperate forests, Glob. Change Biol., 18, 1173–1184, https://doi.org/10.1111/j.1365-2486.2011.02545.x, 2012.
Stow, D. A., Hope, A., McGuire, D., Verbyla, D., Gamon, J., Huemmrich, F., Houston, S., Racine, C., Sturm, M., Tape, K., Hinzman, L., Yoshikawa, K., Tweedie, C., Noyle, B., Silapaswan, C., Douglas, D., Griffith, B., Jia, G., Epstein, H., Walker, D., Daeschner, S., Petersen, A., Zhou, L., and Myneni, R.: Remote sensing of vegetation and land-cover change in Arctic Tundra Ecosystems, Remote Sens. Environ., 89, 281–308, https://doi.org/10.1016/j.rse.2003.10.018, 2004.
Sturm, M., Racine, C., and Tape, K.: Climate change: increasing shrub abundance in the Arctic, Nature, 411, 546–547, https://doi.org/10.1038/35079180, 2001.
Tang, J. and Zhuang, Q.: Equifinality in parameterization of process-based biogeochemistry models: A significant uncertainty source to the estimation of regional carbon dynamics, J. Geophys. Res.-Biogeo., 113, G04010, https://doi.org/10.1029/2008jg000757, 2008.
Tape, K. E. N., Sturm, M., and Racine, C.: The evidence for shrub expansion in Northern Alaska and the Pan-Arctic, Glob. Change Biol., 12, 686–702, https://doi.org/10.1111/j.1365-2486.2006.01128.x, 2006.
Tarnocai, C., Canadell, J. G., Schuur, E. A. G., Kuhry, P., Mazhitova, G., and Zimov, S.: Soil organic carbon pools in the northern circumpolar permafrost region, Global Biogeochem. Cy., 23, GB2023, https://doi.org/10.1029/2008gb003327, 2009.
Todd-Brown, K. E. O., Hopkins, F. M., Kivlin, S. N., Talbot, J. M., and Allison, S. D.: A framework for representing microbial decomposition in coupled climate models, Biogeochemistry, 109, 19–33, https://doi.org/10.1007/s10533-011-9635-6, 2011.
Todd-Brown, K. E. O., Randerson, J. T., Post, W. M., Hoffman, F. M., Tarnocai, C., Schuur, E. A. G., and Allison, S. D.: Causes of variation in soil carbon simulations from CMIP5 Earth system models and comparison with observations, Biogeosciences, 10, 1717–1736, https://doi.org/10.5194/bg-10-1717-2013, 2013.
White, A., Cannell, M. G. R., and Friend, A. D.: The high-latitude terrestrial carbon sink: a model analysis, Glob. Change Biol., 6, 227–245, 2000.
Wieder, W. R., Bonan, G. B., and Allison, S. D.: Global soil carbon projections are improved by modelling microbial processes, Nat. Clim. Change, 3, 909–912, https://doi.org/10.1038/nclimate1951, 2013.
Zhuang, Q., Romanovsky, V. E., and McGuire, A. D.: Incorporation of a permafrost model into a large-scale ecosystem model: Evaluation of temporal and spatial scaling issues in simulating soil thermal dynamics, J. Geophys. Res.-Atmos., 106, 33649–33670, https://doi.org/10.1029/2001jd900151, 2001.
Zhuang, Q., McGuire, A. D., O'Neill, K. P., Harden, J. W., Romanovsky, V. E., and Yarie, J.: Modeling soil thermal and carbon dynamics of a fire chronosequence in interior Alaska, J. Geophys. Res., 108, FFR 3-1-FFR-3-26, https://doi.org/10.1029/2001jd001244, 2002.
Zhuang, Q., He, J., Lu, Y., Ji, L., Xiao, J., and Luo, T.: Carbon dynamics of terrestrial ecosystems on the Tibetan Plateau during the 20th century: an analysis with a process-based biogeochemical model, Global Ecol. Biogeogr., 19, 649–662, https://doi.org/10.1111/j.1466-8238.2010.00559.x, 2010.
Zhuang, Q., Chen, M., Xu, K., Tang, J., Saikawa, E., Lu, Y., Melillo, J. M., Prinn, R. G., and McGuire, A. D.: Response of global soil consumption of atmospheric methane to changes in atmospheric climate and nitrogen deposition, Global Biogeochem. Cy., 27, 650–663, https://doi.org/10.1002/gbc.20057, 2013.
Zhuang, Q., Zhu, X., He, Y., Prigent, C., Melillo, J. M., McGuire, D. A., Prinn, R. G., and Kicklighter, D. W.: Influence of changes in wetland inundation extent on net fluxes of carbon dioxide and methane in northern high latitudes from 1993 to 2004, Environ. Res. Lett., 10, 095009, https://doi.org/10.1088/1748-9326/10/9/095009, 2015.
Zhuang, Q., McGuire, A. D., Melillo, J. M., Clein, J. S., Dargaville, R. J., Kicklighter, D. W., Myneni, R. B., Dong, J., Romanovsky, V. E., Harden, J., and Hobbie, J. E.: Carbon cycling in extratropical terrestrial ecosystems of the Northern Hemisphere during the 20th century: a modeling analysis of the influences of soil thermal dynamics, Tellus B, 55, 751–776, https://doi.org/10.3402/tellusb.v55i3.16368, 2003.
- Article
(2709 KB) - Full-text XML