the Creative Commons Attribution 4.0 License.
the Creative Commons Attribution 4.0 License.
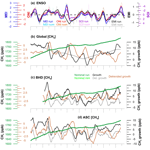
Limited impact of El Niño–Southern Oscillation on variability and growth rate of atmospheric methane
Dan Smale
Sylvia E. Nichol
Tony M. Bromley
Gordon W. Brailsford
Ross J. Martin
Rowena Moss
Sylvia Englund Michel
James W. C. White
The El Niño–Southern Oscillation (ENSO) has been suggested as a strong forcing in the methane cycle and as a driver of recent trends in global atmospheric methane mole fractions [CH4]. Such a sensitivity of the global CH4 budget to climate events would have important repercussions for climate change mitigation strategies and the accuracy of projections for future greenhouse forcing. Here, we test the impact of ENSO on atmospheric CH4 in a correlation analysis. We use local and global records of [CH4], as well as stable carbon isotopic records of atmospheric CH4 (δ13CH4), which are particularly sensitive to the combined ENSO effects on CH4 production from wetlands and biomass burning. We use a variety of nominal, smoothed, and detrended time series including growth rate records. We find that at most 36 % of the variability in [CH4] and δ13CH4 is attributable to ENSO, but only for detrended records in the southern tropics. Trend-bearing records from the southern tropics, as well as all studied hemispheric and global records, show a minor impact of ENSO, i.e. < 24 % of variability explained. Additional analyses using hydrogen cyanide (HCN) records show a detectable ENSO influence on biomass burning (up to 51 %–55 %), suggesting that it is wetland CH4 production that responds less to ENSO than previously suggested. Dynamics of the removal by hydroxyl likely counteract the variation in emissions, but the expected isotope signal is not evident. It is possible that other processes obscure the ENSO signal, which itself indicates a minor influence of the latter on global CH4 emissions. Trends like the recent rise in atmospheric [CH4] can therefore not be attributed to ENSO. This leaves anthropogenic methane sources as the likely driver, which must be mitigated to reduce anthropogenic climate change.
Attributing recent changes in the methane budget, and the associated impact on its growth rate, to specific natural or anthropogenic causes is essential for climate change mitigation. The impact of climatic variability on methane emissions is particularly important to assess the potential for CH4 release under future climate scenarios (e.g., from permafrost and wetland environments, as well as gas hydrates) in a reinforcing feedback. Atmospheric methane mole fractions [CH4] have increased by 140 % over pre-industrial levels (MacFarling Meure et al., 2006). The associated increase in radiative forcing makes CH4 the second most important anthropogenic greenhouse gas (Shindell et al., 2009). The long-term [CH4] increase until the late 1990s can be attributed to increasing emissions from fossil fuel production (Ferretti et al., 2005; Schaefer et al., 2016), as well as sources from agriculture (enteric fermentation in livestock, rice production), waste management, and anthropogenic burning (van Aardenne et al., 2001; Saunois et al., 2016). After a plateau in the early 2000s, [CH4] has been rising again since 2007. Considering recent reconstructions of methane's dominant atmospheric sink, i.e. the hydroxyl radical OH, we consider it likely that increasing emissions contribute to (Rigby et al., 2017), if not dominate (Naus et al., 2018), the [CH4] rise. If so, the methane source type that varied can be investigated with measurements of stable carbon isotope ratios in atmospheric methane (δ13CH4). The latter are influenced by the relative source contributions from 13C-depleted biogenic, 13C-rich pyrogenic, and thermogenic methane with intermediate δ13C. Isotope studies suggest that biogenic methane sources make either a dominant (Schaefer et al., 2016; Nisbet et al., 2016) or strong (Worden et al., 2017) contribution to the recent [CH4] rise. Biogenic methane comes predominantly from wetlands and agriculture. Schaefer et al. (2016) suggested agriculture as the more likely cause, primarily because satellite data place the increased emissions in Southeast Asia, India, and China (Houweling et al., 2014). However, this geographic footprint from an inversion of satellite data is also consistent with fluxes from one particular wetland emissions model (Houweling et al., 2014). Other studies also assume a stronger role of wetlands due to drier conditions during the plateau years (Bousquet et al., 2006) and higher wetland emissions afterwards, which are attributed to a switch to predominant La Niña conditions around 2007 (Bousquet et al., 2011; Nisbet et al., 2016). La Niña is the cold phase of El Niño–Southern Oscillation (ENSO) cycles, which have a strong impact on precipitation anomalies in tropical regions (Ropelewski and Halpert, 1987; Lyon and Barnston, 2005) (Fig. 1) that are key source areas for methane production from wetlands and biomass burning (Kirschke et al., 2013). ENSO impacts are strongest in the tropics, generally from December to February. During El Niño (La Niña) events in the December to February period, it tends to be drier (wetter) in the Indonesian region, north-east Brazil, and south-eastern Africa, whereas it tends to be wetter (drier) in the southern USA and Mexico, eastern China and Taiwan, and east-central Africa (Fig. 1). During El Niño (La Niña) events in the June to August period, it tends to be drier (wetter) in the Indonesian region, central America, and India.
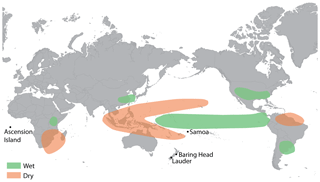
Figure 1Regions of ENSO impacts and monitoring stations used in this study. The map indicates the locations of the atmospheric monitoring stations on Ascension Island (ASC), Samoa (SMO), Baring Head (BHD), and Lauder (LAU). General precipitation anomalies during Northern Hemisphere El Niño conditions for December–February are taken from https://www.climate.gov/news-features/featured-images/global-impacts-el-nino-and-la-nina (last access: 5 December 2017). El Niño dry regions in June–August are similar for southern Asia and South America; during La Niña events opposite patterns for wet- and dryness develop in roughly the same regions.
The generally drier conditions during El Niños suppress global wetland emissions in models by up to 19 Tg yr−1 in the 1990s (Hodson et al., 2011). Several anthropogenic sources are subject to the same ENSO forcing and are expected to vary in concert with wetlands (e.g., rice agriculture, possibly livestock). At the same time, dry El Niño phases enhance CH4 emissions from both natural and anthropogenic biomass burning (van der Werf et al., 2006). Wet La Niña conditions have the opposite effect; summed across the globe they increase wetland emissions and lower biomass burning CH4. As tropical wetland fluxes are considerably larger than biomass burning emissions (Saunois et al., 2017), the expected net effect is a lower [CH4] growth rate caused by El Niño conditions and a higher one due to La Niñas. The ENSO impact on δ13CH4 should be more pronounced than the one on [CH4] because changes in wetland and biomass burning emissions combine to enrich atmospheric CH4 in 13C during El Niños and deplete it during La Niñas. Biogenic methanogenesis in wetlands discriminates strongly against 13C and creates methane that is 13C-depleted (δ13C = −58 ‰ for tropical wetlands) relative to the plant precursor material (δ13C of −12 ‰ to −28 ‰ ) and to the combined total of global emissions (δ13C 53.5 ‰). In contrast, during burning the isotope ratios of the precursor plant material are essentially conserved and lead to δ13C ‰ for CH4 emissions from fires (Schwietzke et al., 2016). The simultaneous suppression of 13C-depleted wetland CH4 and enhancement of very 13C-rich pyrogenic emissions (and vice versa) act in the same direction on the δ13CH4 of the combined source. The latter should be detectable in atmospheric δ13CH4 records if the impact of ENSO on the CH4 cycle is sufficiently large, as is predicted by the emission anomalies in wetland emission models (Hodson et al., 2011), reconstructed from satellite observations of burned area (van der Werf et al., 2010), and observed through variability in hydrogen cyanide (HCN) (Pumphrey et al., 2018), which is an indicator of biomass burning.
Varying contributions from wetlands dominated by C3 and C4 plants, which differ in the δ13CH4 of their emissions, may be part of the ENSO–CH4 signal or work to obscure it if controlled by other drivers. In general, we assume that δ13CH4 of the various emission sources has not changed over the ∼35-year period of our study. Although such changes, correlated to atmospheric CO2 mole fractions, have been reported to occur over centuries to millennia in ice core studies (Möller et al., 2013), they are likely negligible over the short duration and > 20 % CO2 change of our study period.
Changes in OH have also been suggested as partial or dominant drivers in recent CH4 trends, both for the onset of the 1999–2006 plateau (McNorton et al., 2016; Schaefer et al., 2016) and for the post-2007 [CH4] increase (Rigby et al., 2017; Turner et al., 2017). A chemistry–climate model suggests that ENSO modulates tropical OH (where hydroxyl levels are highest) via changes in NOx production through lightning, ozone availability, and specific humidity, as well as emissions of reactive carbon (Turner et al., 2018). Resulting changes in methane removal could create their own signal in atmospheric records of [CH4] and δ13CH4. They could also either reinforce or dampen the emission impacts discussed above.
We conduct correlation analyses between ENSO variability and [CH4], as well as δ13CH4 records, to quantify how much ENSO anomalies in emissions and sinks affect atmospheric CH4. Specifically, we explore how much of the year-to-year variability in atmospheric methane can be attributed to ENSO and how large the ENSO–CH4 signal is in dependence of latitude. We test if recent trends in methane growth rate can be attributed to wetland emissions controlled by ENSO dynamics or if agricultural sources are more likely drivers. ENSO is quantified by four different indices, which are based on ocean temperature, sea level pressure gradients, and a multivariate combination. [CH4] and δ13CH4 time series from four different locations were used, two from stations in the southern tropics (Samoa, SMO, and Ascension Island, ASC), the southern mid-latitudes (Baring Head, NZ; BHD), taken as representative of the Southern Hemisphere, and global average time series of [CH4] and δ13CH4 calculated from a network of global stations (Dlugokencky et al., 2011; Schaefer et al., 2016). We also investigate ENSO's impact on HCN data measured in Lauder, NZ (LAU), to quantify the biomass burning contribution separately. The aim is to detect the impact of ENSO on atmospheric CH4 on various spatial scales.
2.1 Data
For access to all data sets used in this study, see Sect. 10.
2.1.1 ENSO indices
We used four different indices in our analysis to cover various climatic effects of the ENSO cycle (Figs. 2a and 3a). The Southern Oscillation Index (SOI) is calculated from the gradient in mean sea-level pressure observations at Tahiti and Darwin, Australia (Troup, 1965). Further information on the SOI is given by Horel and Wallace (1981) and Trenberth (1976). The Ocean Niño Index (ONI) uses sea surface temperature (SST) anomalies in the eastern Pacific Niño 3.4. region (5∘ N–5∘ S, 120–170∘ W), which show smaller intra-seasonal variability than pressure and are further smoothed by using 3-month running means (Barnston et al., 1997; Kousky and Higgins, 2007).
The El Niño Modoki Index (EMI) is based on SST anomalies in the central Pacific (Ashok et al., 2007) rather than the eastern Pacific (the canonical El Niño). Events with the largest SST anomalies in the Modoki region show differences in the climate teleconnections to canonical El Niño events. The tropical precipitation differences are modest, but large differences in tropospheric circulation and wind anomalies (Yeh et al., 2009) can produce large extratropical differences in precipitation and temperature. The EMI has also been shown to be a significant predictor of tropical atmospheric ozone variations (Xie et al., 2014).
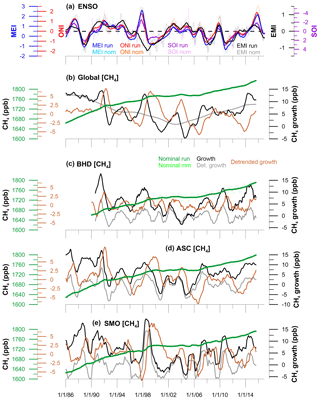
Figure 2Selected time series of ENSO indices and [CH4]. Panels from top to bottom: (a) Multivariate ENSO Index (MEI), Southern Oscillation Index (SOI), Ocean Niño Index (ONI), and El Modoki Index (EMI) shown for nominal literature data and their 12-month running means. (b) Global [CH4] records; monthly means, 12-month running mean, detrended 12-month running mean, as well as nominal and detrended growth rates. (c) [CH4] records from BHD (d) [CH4] records from ASC. (e) [CH4] records from SMO. BHD, ASC, and SMO display the same records as for global time series.
Variability in both atmospheric pressure and SST anomalies informs the Multivariate ENSO Index (MEI) (Wolter and Timlin, 1993, 1998). The various indices correlate highly with each other (r2=0.85 and higher), except the EMI (r2 between 0.33 and 0.52 for correlations with SOI, ONI, and MEI), which deviates from the others during the strong 1997–1998 El Niño event. Excluding the latter brings the correlation to r2 between 0.74 and 0.79.
An ENSO index based on precipitation data, the ESPI, (Curtis and Adler, 2000) correlates very highly with the MEI, the ONI, and the SOI (r2 of 0.902, 0.909, and 0.839, respectively). Therefore, we did not conduct separate calculations for the ESPI.
2.1.2 [CH4] time series
The [CH4] time series used in this study are from the Global Monitoring Division of the National Oceanic and Atmospheric Administration Earth System Research Laboratory (NOAA ESRL) Carbon Cycle Cooperative Global Air Sampling Network (Dlugokencky et al., 2017). These data include records from SMO (latitude 14.24∘ S, longitude 170.57∘ W) and ASC (7.92∘ S, 14.42∘ W), as well as global averages calculated by smoothing background data temporally and zonally, all with coverage from 1983 to 2017. In addition, we use data measured at the NZ National Institute of Water and Atmospheric Research (NIWA) from BHD in NZ (41.41∘ S, 174.87∘ E; 1992–2017) (Lowe et al., 1991). Both data sets are on the same international scale (Dlugokencky et al., 2005), although for the presented analysis, internal consistency of the time series is the relevant criterion; inter-laboratory offsets do not affect the findings. The individual time series (Fig. 2b–e) show seasonal cycles, inter-annual variability (IAV), and long-term trends. To investigate ENSO effects on these different timescales we derived the following seven records from the individual measurements at each station (Table 1): first, the nominal monthly mean values to capture the full variability in the data (“nom”), second, 12-month running means to represent IAV and trends (“run”), third, monthly resolved growth rate defined as the difference between the following 12 months and the preceding 12 months (“gro”), and fourth, a residual (“res”) as calculated by seasonal trend analysis by loess (STL; Cleveland et al., 1990). The seasonal window was set at 120 months, which forces a uniform seasonal cycle over the duration of the record. The residual therefore represents IAV in the expression of the seasonal cycle as well as other short-term anomalies. The fifth, sixth, and seventh records are the detrended time series for which the STL trend component is subtracted from the monthly means with subsequent determination of detrended monthly means, 12-month running means, and growth rate (“det-nom”, “det-run”, “det-gro”).
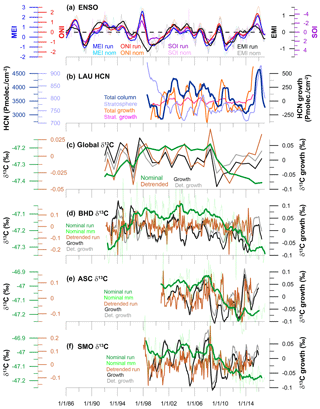
Figure 3Selected time series of ENSO indices, HCN, and δ13CH4. Panels from top to bottom: (a) multivariate ENSO Index (MEI), Southern Oscillation Index (SOI), Ocean Niño Index (ONI), and El Modoki Index (EMI) shown for nominal literature data and their 12-month running means. (b) HCN records as 12-month running means from LAU for total atmospheric column and stratosphere (12–100 km) and respective growth rates. (c) Global annually averaged δ13CH4 according to Schaefer et al. (2016) updated to end of 2016; nominal and detrended values and their respective growth rates. (d) δ13CH4 from BHD; monthly means, 12-month running mean, and detrended 12-month running mean, as well as nominal and detrended growth rates. (e) δ13CH4 from ASC. (f) δ13CH4 from SMO. ASC and SMO display same records as for BHD. Note scale differences between all δ13C axes to accentuate variability for comparison with ENSO.
2.1.3 δ13CH4 time series
The δ13CH4 time series used in this study were measured at three different laboratories, i.e., the Institute of Arctic and Alpine Research (INSTAAR), USA; the Institute for Environmental Physics (IUP) at Heidelberg University, Germany; and at NIWA. Details of the analytical methods are given by Schaefer et al. (2016) and references therein. All values are based on measured 13C ∕ 12C ratios and are reported in the standard δ notation δ13C = ( ‰ as per mil (‰) values, for which the reference standard is Vienna Pee Dee Belemnite. Records at SMO (1998–2016) and ASC (2000–2016) are measured at INSTAAR. The BHD record (1992–2016) is based on measurements at INSTAAR and NIWA. An annually averaged global δ13CH4 time series was established by Schaefer et al. (2016) based on data from INSTAAR, NIWA, and IUP. In this analysis, we use the measurements covering 1992–2016 (Fig. 3c). For the global δ13CH4 data set we conducted the analysis for the nominal annual means (nom) and growth rate, i.e. the difference between two subsequent yearly values (gro). We also detrended the time series by subtracting linear trends for the sub-periods 1992–1999 and 2007–2016 (“det”) and then calculating a detrended growth rate (det-gro). For the single-station δ13CH4 records of BHD, ASC, and SMO, we derived the same seven records as described for the [CH4] data (Fig. 3d–f).
2.1.4 HCN time series
HCN retrievals were computed from mid-infrared solar spectra measured at LAU (45.04∘ S, 169.68∘ E) as part of the Network for the Detection of Atmospheric Composition Change (NDACC). The time series has been described by Zeng et al. (2012), but the data used here are from updated retrievals using the improved SFIT4 algorithm (NDACC, 2014). The HCN data show strong seasonality that is even more pronounced in the updated retrievals. Zeng et al. (2012) found a significant negative trend for 1997–2009 and attributed it to variations in biomass burning. A similar deseasonalized trend is apparent in the updated record. HCN values are reported here as petamolecules cm−2. Measurements cover the period 1998–2017 when combined for two different instruments with a change-over point in 2000. We conducted our analyses for total column values (0–100 km). The latter signal is dominated by the tropospheric burden as measured in the 0–12 km height partial column; the correlation between total and tropospheric HCN is r2=0.997. In addition, we investigated whether the stratospheric HCN burden is differently impacted by ENSO. To that end, we used the 12–100 km partial column, which holds ∼22 % of the total HCN burden. This layer shows lower correlation with the total column record (r2=0.45).
Analogous to the monthly resolved methane records, we constructed monthly means, 12-month running means, growth rates, and STL residuals for the total column and stratospheric HCN data (Table 1, Fig. 3b). No detrended records other than STL residual were considered.
2.2 Analysis
We conducted correlation analyses between the time series of a chosen ENSO index and either a [CH4], δ13CH4, or HCN record as the dependent variable. The degree of correlation is quantified by the square (r2 value) of the Pearson correlation coefficient or, alternatively, of the Spearman ranking coefficient. The Pearson coefficient is more commonly used, but it assumes linear relationships between the variables and may underestimate non-linear correlations. We therefore also used the Spearman rank, which does not require linearity. Note that not all correlation combinations were tested using both coefficients.
A lag time between ENSO forcing and detection of resulting δ13CH4 or HCN variability at the measurement site, (or in the global average) is likely, due to a variety of factors that may lead to lags of unknown length and some of which may be cumulative, e.g., hydrology, plant growth and decay, microbial response, and seasonal triggers for methanogenesis or burning, as well as atmospheric chemistry, mixing, and transport between source regions and sampling sites. Therefore, it is difficult to define a cut-off for lags. Literature estimates of specific lags range from days (Chamberlain et al., 2016) to 7 months (Zhang et al., 2018; Zhu et al., 2017), not counting atmospheric transport. Given ENSO variability with a periodicity of 2–7 years (McPhaden et al., 2006), our analysis therefore allows for lag times of up to 5 years in monthly increments in the calculations and reports the maximum r2 and lag time (in months) for a given ENSO–[CH4] ∕ δ13CH4 ∕ HCN combination. We conducted the analysis for all permutations of the four ENSO indices as monthly means and their 12-month running means as well as the [CH4], δ13CH4, and HCN data products listed in Sect. 2.1 and Table 1. For all [CH4], δ13CH4, and HCN parameters we used the period 1998–2016, except for ASC, where data are only available from late 2000. Using the same period for all time series avoids differing correlation results due to varying data coverage. The period includes the strong El Niños of 1998 and 2015, as well as the strong La Niñas of 1999, 2007, and 2010. We also calculated correlations for the period 1983–2016 ([CH4] of SMO, ASC, and global) and 1992–2016 (δ13CH4 at BHD and global).
Most combinations have r2 values < 0.1 when comparing one dependent data set to the different ENSO time series (Tables 2–4). In the following, we only summarize results for the highest r2 for each dependent time series (across all the nominal, smoothed, and detrended records for a station). Given that Pearson coefficient and Spearman rank give comparable results (Tables 3 and 4), we quote the Spearman results, unless otherwise mentioned. The p values for the Spearman ranks indicate that all results for r2 > 0.1 are significant (p< 0.001), except for global δ13CH4 correlations, for which no p values below 0.05 occur. Although the analysis provides r2 values for lags up to 60 months (Tables 2–4), we consider it likely that lags of > 3 years indicate spurious correlations, given that individual ENSO events last 1–2 years and global atmospheric mixing times are on the order of 1 year. Therefore, we also report the highest r2 for lags < 3 years in the following sections. For other cases with lags > 3 years in Tables 2–4, the highest relevant r2 value is lower than the reported value, which places an upper limit on the influence of ENSO.
Table 2Spearman correlation of methane mole fraction with ENSO variability. Correlations (r2 values) for the Spearman ranking coefficient between [CH4] time series from various sites and ENSO indices with lag times (in months) for optimum results. Colour backgrounds indicate r2 values in 10 % classes. Grey background indicates correlations with p values > 0.001.
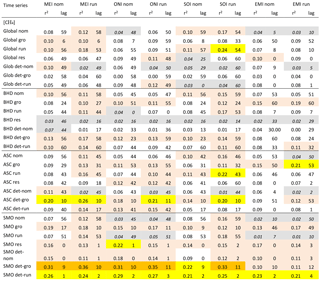
Methane mole fractions show correlations with ENSO of r2 values up to 0.36 at SMO, but only for detrended time series (Table 2). The highest values are from (detrended) growth rates, which can be more indicative of dynamics within an ENSO event, rather than its overall emissions impact (Zhang et al., 2018). For SMO detrended [CH4] series, lag times are fairly consistent across the various ENSO indices and generally shorter than 1 year. For other [CH4] records at SMO and ASC, the highest correlations are r2 < 0.23 and have lags of over 3 years (r2 < 0.19 for lags < 3 years). The global running mean [CH4] time series shows r2=0.24 (lag: 4.5 years; r2=0.04 for lag < 3 years) with the SOI running mean for the period 1998–2016. However, for the full length of available data, as well as all BHD records, all correlations are below r2=0.20, with lag times that are variable, extremely short (zero or 1 month), or over 3 years.
The highest correlations are between HCN total column running means, as well as stratospheric growth rates, and 12-month running mean ENSO records (Table 3). Here, ENSO accounts for 30 %–51 % of the observed variability, depending on the ENSO index. For both total and stratospheric HCN, lag times for maximum correlation are generally shorter than 1 year and are consistent (≤6 months difference) between the various ENSO indices, with exception of the EMI.
The δ13CH4 records from the stations SMO, ASC, and BHD all have r2 values below 0.24 (Table 3). Variability in lag times between different ENSO indices for the same dependent record is generally high.
None of the global δ13CH4 series produced statistically robust correlations with ENSO; all p values were higher than 0.05. The following findings are therefore not relevant for further interpretation. The highest correlation is between global detrended δ13CH4 and SOI monthly means with r2=0.37. Global δ13CH4, is the only parameter for which ENSO monthly means produce higher correlations than the smoothed (12-month running mean) record. Because the correlation calculation between annual δ13CH4 and ENSO monthly means is specific to the month of year, this indicates that global δ13CH4 is more sensitive to the seasonality of ENSO than its IAV. The actual ENSO influence on global δ13CH4 is shown through correlation with running ENSO indices, which is highest between nominal δ13CH4 values and SOI, with Pearson r2=0.25 for 1998–2016. For the period 1992–2016 this value drops to Pearson r2=0.20. The lack of statistical robustness for global δ13CH4-ENSO correlations may stem from the different resolution of the two sets of time series. In this case, the southern hemispheric record from BHD may represent the extratropical impact of ENSO on δ13CH4.
Table 3Spearman correlation of δ13CH4 and HCN with ENSO variability. Correlations (r2 values) for the Spearman ranking coefficient between dependent variables, i.e. δ13CH4 and HCN time series from various sites, and ENSO indices with lag times (in months) for optimum results. Colour backgrounds indicate r2 values in 10 % classes. Grey background indicates correlations with p values > 0.001.
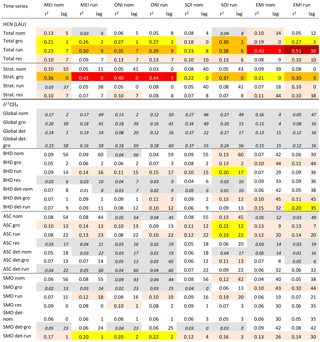
The full BHD record for 1992–2016 gives very similar results as the 1998–2016 subset used for comparison with the other stations (as discussed above). However, the shorter subset for 1998–2014 produces larger Pearson r2 values (0.26 for running means and SOI), and for 2001–2014 we find Pearson r2 values up to 0.38 (growth rate correlated to EMI). These shorter data sets omit the strong El Niño events of 1998 and/or 2015–2016, which could have been expected to have a strong influence on methane emissions and consequently δ13CH4.
For none of the stations (including global average) did the detrended δ13CH4 time series (incl. STL residuals) produce a markedly stronger correlation with ENSO than any of the other data series from that station. This is remarkable because ENSO can be expected to have more influence on IAV than on the long-term trends, which are quite pronounced.
Table 4Pearson correlation of δ13CH4 and HCN with ENSO variability. Correlations (r2 values) for the Pearson correlation coefficient between dependent variables, i.e. δ13CH4 and HCN time series from various sites, and ENSO indices with lag times (in months) for optimum results. Colour backgrounds indicate r2 values in 10 % classes. Results have not been screened for p values.
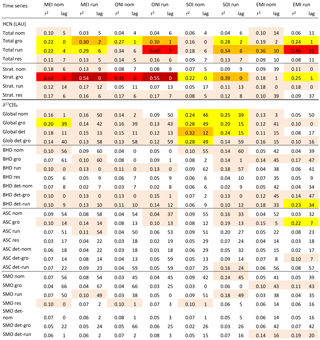
4.1 General causes and caveats for correlations of [CH4], δ13CH4, and HCN with ENSO
Detected correlations between ENSO indices and [CH4] ∕ δ13CH4 ∕ HCN by themselves do not prove a causal relationship. However, the underlying mechanisms for a potential forcing have been presented by van der Werf et al. (2006) for biomass burning and by Hodson et al. (2011) for wetland CH4 production. Accordingly, a correlation analysis is useful to quantify an upper limit of variability in the CH4 cycle attributable to ENSO. Because ENSO simultaneously suppresses wetland CH4 that is more 13C-depleted than the cumulative methane source and enhances pyrogenic CH4 that is more 13C-enriched (or vice versa), the two influences partly cancel out the combined emission rates, i.e. their impact on [CH4]. However, they reinforce each other's impact on total source δ13CH4. It is possible that biomass burning and wetland CH4 production have different response times to ENSO forcing, which would weaken their cumulative impact on δ13CH4. Similarly, a longer atmospheric residence time of CH4 (∼9 years, Prather et al., 2012) over HCN (∼3 months, Li et al., 2000) and a smaller relative portion of ENSO-sensitive emissions in the global methane source may lead to dampening effects in the [CH4] and δ13CH4 variability and hence lower correlation with ENSO indices compared to HCN. The available records for HCN and δ13CH4 from ASC and SMO only cover a small number of ENSO events, which could affect the results. However, when analysing sub-periods of global and BHD [CH4] and δ13CH4 records, we find larger correlations for shorter periods, particularly when strong ENSO events are excluded. This shows that the results are not biased against the detection of ENSO influences because records are too short. We also note that all stations measure background air; they are set up to detect broad spatial and temporal trends and not specific emission events such as an ENSO-triggered plume. However, if ENSO is invoked as a main cause of recent trends in [CH4] and δ13CH4 (Nisbet et al., 2016) this should be manifested in sizeable correlations.
4.2 Contrasting correlation patterns for [CH4] and δ13CH4 versus HCN
In all [CH4] and δ13CH4 records, ENSO cycles explain at most about one-third of the variability in detrended records and less than one-quarter in others. This is true even for the southern tropics, where ENSO has strong climatic impacts and where the majority of low-latitude wetland emissions and of biomass burning emissions originate (Kirschke et al., 2013). Correlations found for ASC and SMO, which represent this latitude band in our study, exceed those for the southern mid-latitudes or the global record only by a limited margin and only for detrended records. Further, inconsistent lag times, lags of more than 3 years, and higher correlation coefficients for the exclusion of major ENSO events point to spurious correlations.
In contrast, we find a prominent influence of ENSO on the biomass burning proxy HCN. ENSO impacts on HCN have been reported before, e.g., by Pumphrey et al. (2018), who observe suppression of HCN levels during La Niña events and enhancement during El Niños, particularly in equatorial Asia. That study found a rather confined geographical impact of El Niño events with strongly enhanced HCN emissions around Malaysia, Indonesia, and Papua New Guinea, as well as generally rapid transport eastward and to the stratosphere. We speculate that the fast, upward transport (although not observed for all El Niño events) explains why stratospheric growth rates are the most sensitive data set to ENSO. For the total column, the HCN burden is concentrated in lower tropospheric levels and may be subjected to more mixing of different air parcels. According to the results of Pumphrey et al. (2018), data from LAU in the southern mid-latitudes are outside the region of the strongest HCN signal. This is also evident in the zonal mean HCN climatologies of Sheese et al. (2017). However, ENSO accounts for up to 51 % of the variability in our biomass burning proxy record. One explanation for the lower combined wetland–pyrogenic δ13CH4 signal is low sensitivity of wetland CH4 production to ENSO events. This is consistent with r2 values of 0.12–0.26 between modelled wetland methane emissions (using different climate data sets as drivers) and MEI as reported by Zhang et al. (2018). Alternatively, other processes in the CH4 cycle obscure the ENSO impacts.
4.3 Impact of ENSO on methane emission rates
In a correlation analysis by Zhu et al. (2017), ENSO explained 49 % of IAV in modelled tropical wetland CH4 emissions. This is far higher than the combined effect with biomass burning on δ13CH4 in this study, and therefore seems to be an overestimate. Even so, the magnitude of the modelled emission changes is 6 Tg yr−1 at most. The modelling study of Hodson et al. (2011) finds slightly larger anomalies in global wetland emissions due to ENSO with mean reductions of Tg yr−1 and mean gains of Tg yr−1 for El Niño and La Niña events, respectively. Pandey et al. (2017) found in a comprehensive inversion study that the net effect of the strong 2011 La Niña on tropical and northern extratropical CH4 emissions was a global increase of +6.6 Tg yr−1. The wetland emission anomalies are expected to be partly compensated by changes in biomass burning that are of opposite sign. We are not aware of studies that quantify biomass burning anomalies for specific ENSO events. Assuming that ENSO is the main control of biomass burning emissions of CH4, the IAV in the Global Fire Emissions Database (GFED) data (van der Werf et al., 2010) may serve as an indication for possible ENSO impacts. In that case, the standard deviation of 2.4 Tg yr−1 for 1998–2014 would approximate the average impact, with maximum anomalies of up to 4 Tg yr−1. We use these numbers together with results from Hodson et al. (2011) in the following proof-of-concept discussions. The combined wetland–biomass burning anomalies are ∼6 Tg yr−1 for average ENSO events and ∼8 Tg yr−1 for extreme ones, restricted to 1–2-year-long individual events. This is well short of the sustained increase after 2007 when yearly emissions were ∼20 Tg higher than during the 1999–2006 plateau period and the 9 Tg yr−1 reduction during the 1990s (Schaefer et al., 2016). Previous findings that modelled tropical (Zhu et al., 2015) and global (Zhang et al., 2018) wetland CH4 emissions can explain at most 25 % and 14 %, respectively, of the variation in atmospheric methane growth rates therefore agree with our results that ENSO exerts only a minor control on atmospheric CH4.
4.4 Process-based understanding of ENSO impact on wetlands
A major contribution of ENSO to the recent [CH4] increase is inconsistent with independent assessments of wetland response, as shown above, but our findings do not detect any clear minor contribution of ENSO to [CH4] and δ13CH4 time series, either. Several reasons may explain the lack of correlation, whereby we assume that wetlands respond less than proposed. The main ENSO forcing on tropical wetland CH4 production is thought to be via wetland extent, which is driven by precipitation (Hodson et al., 2011, and Holmes et al., 2015, in contrast to Zhu et al., 2017, who find temperature to be dominant). However, a case study in the eastern Amazon finds that precipitation changes explain only 21 % of wetland CH4 emission variance during the wet season and 7 % over the whole year (Basso et al., 2016). The lack of a direct link between precipitation and wetland CH4 production is also evident in the large range in output from various wetland models even when forced with the same meteorological conditions (Melton et al., 2013), although the disagreement between models could also be due to an incomplete understanding of influences on the wetland cycle other than precipitation (Turetsky et al., 2014; Bridgham et al., 2013; Parker et al., 2018). Zhang et al. (2018) report an evolving response of wetland emissions to El Niños, where an initial reduction due to decreased wetland extend is counteracted by increased microbial activity under higher temperatures during the later stages of the event. A complex response of wetland CH4 production is not only seen in models, however. The inversion study of Pandey et al. (2017) found a global increase of +6.6 Tg yr−1 for the strong 2011 La Niña, but a reduction by −6.1 Tg yr−1 during the 2012 weak La Niña. Similarly, Liu et al. (2017) found that El Niño conditions produced opposing weather forcing and carbon cycle responses between various tropical regions, as well as differing ones between the 1998 and 2015 events. Another example of this is flooding in the Amazon region during La Niña events, while flooding in the wetlands of the Paraná region occurs during El Niños (Parker et al., 2018). Depending on the strength and geographical expression of the climate anomaly, ENSO may thus cause regional or global emission anomalies that are opposite to the expected pattern.
4.5 Evaluating the consistency of ENSO impacts throughout the record
The atmospheric [CH4] history shows global emission reductions in the 1990s and increases after 2007 (Schaefer et al., 2016). This would be consistent with ENSO forcing of the methane cycle, whereby the 1990s were dominated by drier El Niño periods, whereas the recent years of predominant La Niña conditions were wetter. Given that the magnitude of the low-latitude wetland CH4 source exceeds pyrogenic emissions rates, the expected emissions history would qualitatively match atmospheric trends. Also, for a short period between 2008 and 2011, Schaefer et al. (2016) observed the activation of CH4 emissions with an extremely 13C-depleted cumulative δ13CH4 ( ‰). Such a value on the global scale is hard to match by a single source type. The cumulative effect of wetland enhancement and fire suppression forced by the 2008 La Niña event would provide an excellent explanation. However, the isotopic signal of the emissions reductions in the 1990s should be similar if ENSO forcing was the cause. In contrast, Schaefer et al. (2016) found that the “lost emissions” during that period are quite 13C-rich and rather indicate a reduction in fossil fuel methane. An alternative interpretation of these isotope trends by Rice et al. (2016) requires simultaneous reductions of pyrogenic and biogenic emissions, which is also inconsistent with the expected ENSO forcing. A consistent match between ENSO phases and global δ13CH4 is therefore neither evident in the dominant δ13CH4 trends nor in the correlation analysis presented in this study.
4.6 Using isotopes to attribute emission changes
The impact of an ENSO emissions “perturbation” (i.e. the combined emissions anomaly of an event) on atmospheric δ13CH4 can be assessed in isotope mass balance calculations according to
where, for a given source, S and δ represent emission rate and δ13CH4, respectively (note that for scenarios discussed here, S may be negative, i.e., a reduction in emissions). Using generic isotope source signatures for biogenic, fossil fuel, and pyrogenic methane emissions from Schwietzke et al. (2016), we find that the average La Niña perturbations proposed in Sect. 4.3. have an effective δ13CH4 of −79 ‰, with −83 ‰ for extreme ones. As expected, the combined isotope leverage of wetland enhancement and fire reductions on the global source is strong, equalling the leverage of much larger source anomalies (20 Tg yr−1), with lower δ13CH4 of ‰ after 2007 as calculated by Schaefer et al. (2016). In addition to the assumed 6 Tg yr−1 ENSO perturbation, another ∼14 Tg yr−1 of emissions with δ13CH4 ‰ would be necessary to produce the observed [CH4] and δ13CH4 trends. The isotope mass balance then shows that the non-ENSO additional emissions are a roughly equal mix of fossil fuel and biogenic methane. Noting that the assumption that all years after 2007 experienced average La Niña conditions is unrealistic; these findings therefore show the following three points: (i) ENSO effects alone cannot explain the post-2007 [CH4] rise. (ii) There was an increase in biogenic sources in addition to ENSO-driven wetland anomalies. Other wetland variability may have contributed to the rise (Zhang et al., 2018); given the range in wetland model output (Melton et al., 2013) this stands to be confirmed by ensemble runs. In the absence of boreal emission increases (Sweeney et al., 2016), the only biogenic source large enough to accommodate the required changes is agriculture (Saunois et al., 2016). (iii) Any ENSO-driven reduction in biomass burning after 2007 allows for, or requires, growing fossil fuel emissions. The latter has recently been proposed by Worden et al. (2017), who reconstructed larger biomass burning reductions after 2007 than recorded by GFED, although without assigning the reductions to ENSO or other causes.
4.7 Role of other methane cycle processes
There is an alternative explanation for the lack of correlation between ENSO and the methane records. ENSO could affect CH4 emissions from tropical wetlands and biomass burning as predicted by Hodson et al. (2011) and van der Werf et al. (2006), respectively, but the resulting isotopic signal is overwhelmed by other components of the CH4 cycle. Such influences could be other sources (particularly anthropogenic ones), variability in atmospheric transport, or changes in CH4 sink processes. A stronger ENSO signal in southern tropical [CH4] and δ13CH4 compared to southern mid-latitudes and global average would be expected for several of these scenarios. This is because both biomass burning and wetland emissions show strong maxima in the southern tropics and should be the dominant sources in this latitudinal band (Kirschke et al., 2013). The detrended [CH4] records from SMO show such a signal, but one that explains only one-third of the IAV and does not seem to have significant impact on the trends. Further, we do not find higher ENSO forcing of the δ13CH4 variability, even in the core region of its climatic impact. Corbett et al. (2017) show that during La Niña events high surface temperatures over the western Pacific lead to upward transport over the Indonesian region (a CH4 source area from wetlands and rice paddies) and negative CH4 anomalies in the mid-troposphere (tropical surface air with relatively low [CH4] replaces air from the Northern Hemisphere with higher [CH4]). This mechanism would dampen the signal of higher La Niña emissions in surface records like SMO and ASC. However, the corresponding El Niño anomalies in mid-tropospheric CH4 over the central Pacific are smaller. This indicates that central Pacific surface air, where there are no CH4 sources, is closer in [CH4] to mid-tropospheric levels than surface air from the western Pacific. Unless there were strong longitudinal differences in mid-tropospheric [CH4], this is inconsistent with a scenario in which high concentrations of CH4 are generated over the western Pacific in La Niñas but transported upwards and away from the surface stations used in this study. On hemispheric or global scales, transport processes are unlikely to play a strong role, given the short mixing time of methane relative to its atmospheric turnover.
The low correlations of [CH4] and δ13CH4 with ENSO rule out a dominant role for ENSO-triggered sink changes in atmospheric methane records. Removal processes could lead to either amplification or dampening of source signals. Higher emissions of methane and carbon monoxide from biomass burning will draw down OH and weaken the sink. Emission factors from fires for CO are between 10- and 30-fold higher than for CH4 (van der Werf et al., 2017), so that the biomass burning dynamics dominate the source of reactive carbon, leaving less OH during El Niños and more during La Niñas to draw down CH4. This would provide a negative feedback for the emissions' [CH4] signal from ENSO forcing. In contrast, the feedback on the ENSO emissions' δ13CH4 signal would be positive due to varying enrichment of 13C–methane through sink fractionation (less removal leads to less 13C enrichment of relatively 13C-depleted wetland emissions during La Niñas; more removal increases the 13C enrichment from biomass burning emissions during El Niños further). In addition to the reactive carbon effect, Turner et al. (2018) found a further OH increase during La Niñas due to higher lightning rates with NOx production. Turner et al. (2018) could attribute 17 % of OH variability that is forced by climate cycles (rather than emissions of other atmospheric compounds) to ENSO. This is a minor part of the variability, but in consequence the dampening effect on [CH4] and the reinforcing feedback on δ13CH4 would add further to the reactive carbon feedbacks. In our correlation results these sink impacts are not apparent, as the [CH4] correlations for the tropical stations are higher than δ13CH4 correlations (Tables 2 and 3). Nevertheless, the OH dynamics provide a possible explanation for the limited ENSO impact on [CH4] variability and trends. They also make δ13CH4 a conservative proxy for the influence that ENSO exerts on tropical methane.
Whether ENSO has less influence on CH4 emissions than assumed or whether such an impact is overwhelmed by atmospheric removal or other CH4 cycle processes, our results suggest that global atmospheric trends in [CH4] and δ13CH4 are dominated by other components in the methane budget.
To study the impact of natural climate variability on recent trends in atmospheric methane concentration, we investigated the correlation between ENSO cycles and records of the mole fractions and stable carbon isotopes of methane, as well as HCN as a biomass burning indicator. As δ13CH4 is subject to a mutually reinforcing signal from ENSO suppression of wetland emissions and enhancement of biomass burning CH4 (or vice versa), as well as positive feedbacks from OH dynamics, it is particularly suited to study the role of ENSO in the CH4 cycle.
We find a sizeable effect of ENSO on biomass burning, as indicated by HCN variability in southern mid-latitudes. In contrast, ENSO explains a smaller fraction (≤37 %) of [CH4] IAV, even in the southern tropics, where the expected effect should be greatest. Trends in [CH4] and δ13CH4 in these latitudes are far less influenced by ENSO (≤23 %). On hemispheric and global scales the ENSO signal in the methane records is similarly weak. Our results do not rule out that ENSO influences CH4 emissions from wetlands and biomass burning through temperature, enhanced precipitation, or droughts in key regions, but any such impacts are overwhelmed by OH dynamics or other source and sink processes. We review literature estimates of ENSO-driven emissions and find them too small and sporadic to account for the post-2007 rise. Counteracting OH dynamics are expected to further dampen any influence ENSO may have on methane growth rates. Our findings suggest that ENSO is not an important driver for recent global trends in methane, including the [CH4] plateau and the increase in [CH4] since 2007. The latter must therefore have different causes. Our results do not rule out that wetland production is a contributor to the post-2007 [CH4] rise if driven by environmental controls other than ENSO. This is suggested by an increase in wetland CH4 production between the periods 2000–2006 and 2006–2014, although with the limited confidence of a single wetland emissions model (Zhang et al., 2018). The longer the atmospheric [CH4] and δ13CH4 trends persist, the less probable processes that impact IAV and short-lived cyclical events like ENSO as the driver are. Therefore, we consider increasing anthropogenic sources as the more likely cause of the [CH4] rise. Changes in removal rates via OH have been suggested as an additional (Rigby et al., 2017) or alternative (Turner et al., 2017) driver of the increase, but recent work suggests that sink impacts are not dominant (Naus et al., 2018). There is evidence for additional methane emissions from agriculture (Wolf et al., 2017) and from fossil fuel sources (Hausmann et al., 2016); both may contribute to the current rise in [CH4] (Worden et al., 2017). Further identification of these processes is necessary to inform climate change mitigation policies and climate projections.
Raw data for individual stations measured by INSTAAR and NIWA are available from the World Data Centre for Greenhouse Gases at https://gaw.kishou.go.jp/about_wdcgg/wdcgg (Japan Meteorological Agency, 2017); [CH4] and δ13CH4 data from NOAA_ESRL and INSTAAR are also available from ftp://aftp.cmdl.noaa.gov/data/trace_gases (National Oceanic and Atmospheric Administration, 2017). Data from Heidelberg University are available from http://www.iup.uni-heidelberg.de/institut/forschung/groups/kk/en/Data.html (Ruprecht-Karls-Universität Heidelberg, 2017). Lauder MIR-FTIR HCN is publicly available from the NDACC archive at ftp://ftp.cpc.ncep.noaa.gov/ndacc/station/lauder/hdf/ftir (National Oceanic and Atmospheric Administration, 2017). All data products and time series used in this study are available on request from the corresponding author.
HS designed the study, conducted the correlation analysis, and prepared the manuscript. TMB, RJM, RM, GWB, SEM, and JWCW supplied δ13CH4 data, DS supplied HCN data, and SEN conducted data processing and analysis.
The authors declare that they have no conflict of interest.
We thank Brittany Graham, Erik Behrens, Brett Mullan, and Sara Mikaloff Fletcher for
helpful discussions and advice. Edward Dlugokencky from NOAA ESRL supplied
[CH4] data. Dave Lowe started the BHD measurements that made this
analysis possible. This project was supported by NIWA funding under the Climate
and Atmosphere Research Programme CAAC1804 (2017/18 SCI).
Edited by: Fortunat Joos
Reviewed by: Joe
Melton and one anonymous referee
Ashok, K., Behera, S. K., Rao, S. A., Weng, H. Y., and Yamagata, T.: El Nino Modoki and its possible teleconnection, J. Geophys. Res., 112, C11007, https://doi.org/10.1029/2006JC003798, 2007.
Barnston, A. G., Chelliah, M., and Goldenberg, S. B.: Documentation of a highly ENSO-related SST region in the equatorial Pacific, Atmos. Ocean, 35, 367–383, 1997.
Basso, L. S., Gatti, L. V., Gloor, M., Miller, J. B., Domingues, L. G., Correia, C. S. C., and Borges, V. F.: Seasonality and interannual variability of CH4 fluxes from the eastern Amazon Basin inferred from atmospheric mole fraction profiles, J. Geophys. Res., 121, 168–184, 2016.
Bousquet, P., Ciais, P., Miller, J. B., Dlugokencky, E. J., Hauglustaine, D. A., Prigent, C., Van der Werf, G. R., Peylin, P., Brunke, E.-G., Carouge, C., Langenfelds, R. L., Lathiere, J., Papa, F., Ramonet, M., Schmidt, M., Steele, L. P., Tyler, S. C., and White, J.: Contribution of anthropogenic and natural sources to atmospheric methane variability, Nature, 443, 439–443, 2006.
Bousquet, P., Ringeval, B., Pison, I., Dlugokencky, E. J., Brunke, E.-G., Carouge, C., Chevallier, F., Fortems-Cheiney, A., Frankenberg, C., Hauglustaine, D. A., Krummel, P. B., Langenfelds, R. L., Ramonet, M., Schmidt, M., Steele, L. P., Szopa, S., Yver, C., Viovy, N., and Ciais, P.: Source attribution of the changes in atmospheric methane for 2006–2008, Atmos. Chem. Phys., 11, 3689–3700, https://doi.org/10.5194/acp-11-3689-2011, 2011.
Bridgham, S. D., Cadillo-Quiroz, H., Keller, J. K., and Zhuang, Q. L.: Methane emissions from wetlands: biogeochemical, microbial, and modeling perspectives from local to global scales, Glob. Change Biol., 19, 1325–1346, 2013.
Chamberlain, S. D., Gomez-Casanovas, N., Walter, M. T., Boughton, E. H., Bernacchi, C. J., DeLucia, E. H., Groffman, P. M., Keel, E. W., and Sparks, J. P.: Influence of transient flooding on methane fluxes from subtropical pastures, J. Geophys. Res., 121, 965–977, 2016.
Cleveland, R. B., Cleveland, W. S., McRae, J. E., and Terpenning, I.: STL: A seasonal-trend decomposition procedure based on Loess, J. Off. Stat., 6, 3–73, 1990.
Corbett, A., Jiang, X., Xiong, X. Z., Kao, A., and Li, L. M.: Modulation of midtropospheric methane by El Nino, Earth Space Sci., 4, 590–596, 2017.
Curtis, S. and Adler, R.: ENSO indices based on patterns of satellite-derived precipitation, J. Climate, 13, 2786–2793, 2000.
Dlugokencky, E. J., Myers, R. C., Lang, P. M., Masarie, K. A., Crotwell, A. M., Thoning, K. W., Hall, B. D., Elkins, J. W., and Steele, L. P.: Conversion of NOAA atmospheric dry air CH4 mole fractions to a gravimetrically prepared standard scale, J. Geophys. Res., 110, D18306, https://doi.org/10.1029/2005JD006035, 2005.
Dlugokencky, E. J., Nisbet, E. G., Fisher, R., and Lowry, D.: Global atmospheric methane: budget, changes and dangers, P. T. Roy Soc. A, 369, 2058–2072, 2011.
Dlugokencky, E. J., Lang, P. M., Crotwell, A. M., Mund, J. W., Crotwell, M. J., and Thoning, K. W.: Atmospheric Methane Dry Air Mole Fractions from the NOAA ESRL Carbon Cycle Cooperative Global Air Sampling Network, 1983–2016, in: Division, Version: 2018-08-01, available at: ftp://aftp.cmdl.noaa.gov/data/trace_gases/ch4/flask/surface/ (last access: 14 October 2018), 2017
Ferretti, D. F., Miller, J. B., White, J. W. C., Etheridge, D. M., Lassey, K. R., Lowe, D. C., MacFarling Meure, C. M., Dreier, M. F., Trudinger, C. M., van Ommen, T. D., and Langenfelds, R. L.: Unexpected changes to the global methane budget over the past 2000 years, Science, 309, 1714–1717, 2005.
Hausmann, P., Sussmann, R., and Smale, D.: Contribution of oil and natural gas production to renewed increase in atmospheric methane (2007–2014): top-down estimate from ethane and methane column observations, Atmos. Chem. Phys., 16, 3227–3244, https://doi.org/10.5194/acp-16-3227-2016, 2016.
Hodson, E. L., Poulter, B., Zimmermann, N. E., Prigent, C., and Kaplan, J. O.: The El Nino-Southern Oscillation and wetland methane interannual variability, Geophys. Res. Lett., 38, L08810, https://doi.org/10.1029/2011GL046861, 2011.
Holmes, M. E., Chanton, J. P., Tfaily, M. M., and Ogram, A.: CO2 and CH4 isotope compositions and production pathways in a tropical peatland, Global Biogeochem. Cy., 29, 1–18, 2015.
Horel, J. D. and Wallace, J. M.: Planetary-Scale Atmospheric Phenomena Associated with the Southern Oscillation, Mon. Weather Rev., 109, 813–829, 1981.
Houweling, S., Krol, M., Bergamaschi, P., Frankenberg, C., Dlugokencky, E. J., Morino, I., Notholt, J., Sherlock, V., Wunch, D., Beck, V., Gerbig, C., Chen, H., Kort, E. A., Röckmann, T., and Aben, I.: A multi-year methane inversion using SCIAMACHY, accounting for systematic errors using TCCON measurements, Atmos. Chem. Phys., 14, 3991–4012, https://doi.org/10.5194/acp-14-3991-2014, 2014.
Japan Meteorological Agency: World Data Centre for Greenhouse Gases (WDCGG), available at: https://gaw.kishou.go.jp/about_wdcgg/wdcgg, last access: 24 October 2017.
Kirschke, S., Bousquet, P., Ciais, P., Saunois, M., Canadell, J. G., Dlugokencky, E. J., Bergamaschi, P., Bergmann, D., Blake, D. R., Bruhwiler, L., Cameron-Smith, P., Castaldi, S., Chevallier, F., Feng, L., Fraser, A., Heimann, M., Hodson, E. L., Houweling, S., Josse, B., Fraser, P. J., Krummel, P. B., Lamarque, J. F., Langenfelds, R. L., Le Quere, C., Naik, V., O'Doherty, S., Palmer, P. I., Pison, I., Plummer, D., Poulter, B., Prinn, R. G., Rigby, M., Ringeval, B., Santini, M., Schmidt, M., Shindell, D. T., Simpson, I. J., Spahni, R., Steele, L. P., Strode, S. A., Sudo, K., Szopa, S., van der Werf, G. R., Voulgarakis, A., van Weele, M., Weiss, R. F., Williams, J. E., and Zeng, G.: Three decades of global methane sources and sinks, Nat. Geosci., 6, 813–823, 2013.
Kousky, V. E. and Higgins, R. W.: An alert classification system for monitoring and assessing the ENSO cycle, Weather Forecast, 22, 353–371, 2007.
Li, Q. B., Jacob, D. J., Bey, I., Yantosca, R. M., Zhao, Y. J., Kondo, Y., and Notholt, J.: Atmospheric hydrogen cyanide (HCN): Biomass burning source, ocean sink?, Geophys. Res. Lett., 27, 357–360, 2000.
Liu, J. J., Bowman, K. W., Schimel, D. S., Parazoo, N. C., Jiang, Z., Lee, M., Bloom, A. A., Wunch, D., Frankenberg, C., Sun, Y., O'Dell, C. W., Gurney, K. R., Menemenlis, D., Gierach, M., Crisp, D., and Eldering, A.: Contrasting carbon cycle responses of the tropical continents to the 2015–2016 El Nino, Science, 358, 191–193, 2017.
Lowe, D. C., Brenninkmeijer, C. A. M., Tyler, S. C., Lowe, D. C. Brenninkmeijer, C. A. M. Tyler, S. C., and Dlugokencky, E. J.: Determination of the isotopic composition of atmospheric methane and its application in the Antarctic, J. Geophys. Res., 96, 15455–15467, 1991.
Lyon, B. and Barnston, A. G.: ENSO and the spatial extent of interannual precipitation extremes in tropical land areas, J. Climate, 18, 5095–5109, 2005.
MacFarling Meure, C., Etheridge, D., Trudinger, C., Steele, P., Langenfelds, R., van Ommen, T., Smith, A., and Elkins, J.: Law Dome CO2, CH4 and N2O ice core records extended to 2000 years BP, Geophys. Res. Lett., 33, L14810, https://doi.org/10.1029/2006GL026152, 2006.
McNorton, J., Chipperfield, M. P., Gloor, M., Wilson, C., Feng, W., Hayman, G. D., Rigby, M., Krummel, P. B., O'Doherty, S., Prinn, R. G., Weiss, R. F., Young, D., Dlugokencky, E., and Montzka, S. A.: Role of OH variability in the stalling of the global atmospheric CH4 growth rate from 1999 to 2006, Atmos. Chem. Phys., 16, 7943–7956, https://doi.org/10.5194/acp-16-7943-2016, 2016.
McPhaden, M. J., Zebiak, S. E., and Glantz, M. H.: ENSO as an integrating concept in Earth science, Science, 314, 1740–1745, 2006.
Melton, J. R., Wania, R., Hodson, E. L., Poulter, B., Ringeval, B., Spahni, R., Bohn, T., Avis, C. A., Beerling, D. J., Chen, G., Eliseev, A. V., Denisov, S. N., Hopcroft, P. O., Lettenmaier, D. P., Riley, W. J., Singarayer, J. S., Subin, Z. M., Tian, H., Zürcher, S., Brovkin, V., van Bodegom, P. M., Kleinen, T., Yu, Z. C., and Kaplan, J. O.: Present state of global wetland extent and wetland methane modelling: conclusions from a model inter-comparison project (WETCHIMP), Biogeosciences, 10, 753–788, https://doi.org/10.5194/bg-10-753-2013, 2013.
Möller, L., Sowers, T., Bock, M., Spahni, R., Behrens, M., Schmitt, J., Miller, H., and Fischer, H.: Independent variations of CH4 emissions and isotopic composition over the past 160,000 years, Nat. Geosci., 6, 885–890, 2013.
National Oceanic and Atmospheric Administration: Earth System Research Laboratory, Global Monitoring Division, Data and Products, available at: ftp://aftp.cmdl.noaa.20 gov/data/trace_gases, last access: 1 December 2017.
National Oceanic and Atmospheric Administration: Network for the Detection of Atmospheric Composition Change, NDACC measurement stations, available at: ftp://ftp.cpc.ncep.noaa.gov/ ndacc/station/lauder/hdf/ftir, last access: 1 December 2017.
Naus, S., Montzka, S. A., Pandey, S., Basu, S., Dlugokencky, E. J., and Krol, M.: Constraints and biases in a tropospheric two-box model of OH, Atmos. Chem. Phys. Discuss., https://doi.org/10.5194/acp-2018-798, in review, 2018.
NDACC: Infrared Working Group: IRWG Uniform Retrieval Parameter Summary, available at: http://www.acom.ucar.edu/irwg/IRWG_Uniform_RP_Summary-3.pdf (last access: 3 March 2018), 2014
Nisbet, E. G., Dlugokencky, E. J., Manning, M. R., Lowry, D., Fisher, R. E., France, J. L., Michel, S. E., Miller, J. B., White, J. W. C., Vaughn, B., Bousquet, P., Pyle, J. A., Warwick, N. J., Cain, M., Brownlow, R., Zazzeri, G., Lanoiselle, M., Manning, A. C., Gloor, E., Worthy, D. E. J., Brunke, E. G., Labuschagne, C., Wolff, E. W., and Ganesan, A. L.: Rising atmospheric methane: 2007–2014 growth and isotopic shift, Global Biogeochem. Cy., 30, 1356–1370, 2016.
Pandey, S., Houweling, S., Krol, M., Aben, I., Monteil, G., Nechita-Banda, N., Dlugokencky, E. J., Detmers, R., Hasekamp, O., Xu, X. Y., Riley, W. J., Poulter, B., Zhang, Z., McDonald, K. C., White, J. W. C., Bousquet, P., and Rockmann, T.: Enhanced methane emissions from tropical wetlands during the 2011 La Nina, Sci. Rep., 7, 45759, https://doi.org/10.1038/srep45759, 2017.
Parker, R. J., Boesch, H., McNorton, J., Comyn-Platt, E., Gloor, M., Wilson, C., Chipperfield, M. P., Hayman, G. D., and Bloom, A. A.: Evaluating year-to-year anomalies in tropical wetland methane emissions using satellite CH4 observations, Remote Sens. Environ., 211, 261–275, 2018.
Prather, M. J., Holmes, C. D., and Hsu, J.: Reactive greenhouse gas scenarios: Systematic exploration of uncertainties and the role of atmospheric chemistry, Geophys. Res. Lett., 39, L09803, https://doi.org/10.1029/2012GL051440, 2012.
Pumphrey, H. C., Glatthor, N., Bernath, P. F., Boone, C. D., Hannigan, J. W., Ortega, I., Livesey, N. J., and Read, W. G.: MLS measurements of stratospheric hydrogen cyanide during the 2015–2016 El Niño event, Atmos. Chem. Phys., 18, 691–703, https://doi.org/10.5194/acp-18-691-2018, 2018.
Rice, A. L., Butenhoff, C. L., Teama, D. G., Roger, F. H., Khalil, M. A. K., and Rasmussen, R. A.: Atmospheric methane isotopic record favors fossil sources flat in 1980s and 1990s with recent increase, P. Natl. Acad. Sci. USA, 113, 10791–10796, 2016.
Rigby, M., Montzka, S. A., Prinn, R. G., White, J. W. C., Young, D., O'Doherty, S., Lunt, M. F., Ganesan, A. L., Manning, A. J., Simmonds, P. G., Salameh, P. K., Harth, C. M., Muhle, J., Weiss, R. F., Fraser, P. J., Steele, L. P., Krummel, P. B., McCulloch, A., and Park, S.: Role of atmospheric oxidation in recent methane growth, P. Natl. Acad. Sci. USA, 114, 5373–5377, 2017.
Ropelewski, C. F. and Halpert, M. S.: Global and Regional Scale Precipitation Patterns Associated with the El-Nino Southern Oscillation, Mon. Weather Rev., 115, 1606–1626, 1987.
Ruprecht-Karls-Universität Heidelberg: Institute for Environmental Physics, Data, available at: http://www.iup.uni-heidelberg.de/institut/forschung/groups/kk/en/Data.html, last access: 1 December 2017.
Saunois, M., Bousquet, P., Poulter, B., Peregon, A., Ciais, P., Canadell, J. G., Dlugokencky, E. J., Etiope, G., Bastviken, D., Houweling, S., Janssens-Maenhout, G., Tubiello, F. N., Castaldi, S., Jackson, R. B., Alexe, M., Arora, V. K., Beerling, D. J., Bergamaschi, P., Blake, D. R., Brailsford, G., Brovkin, V., Bruhwiler, L., Crevoisier, C., Crill, P., Covey, K., Curry, C., Frankenberg, C., Gedney, N., Höglund-Isaksson, L., Ishizawa, M., Ito, A., Joos, F., Kim, H.-S., Kleinen, T., Krummel, P., Lamarque, J.-F., Langenfelds, R., Locatelli, R., Machida, T., Maksyutov, S., McDonald, K. C., Marshall, J., Melton, J. R., Morino, I., Naik, V., O'Doherty, S., Parmentier, F.-J. W., Patra, P. K., Peng, C., Peng, S., Peters, G. P., Pison, I., Prigent, C., Prinn, R., Ramonet, M., Riley, W. J., Saito, M., Santini, M., Schroeder, R., Simpson, I. J., Spahni, R., Steele, P., Takizawa, A., Thornton, B. F., Tian, H., Tohjima, Y., Viovy, N., Voulgarakis, A., van Weele, M., van der Werf, G. R., Weiss, R., Wiedinmyer, C., Wilton, D. J., Wiltshire, A., Worthy, D., Wunch, D., Xu, X., Yoshida, Y., Zhang, B., Zhang, Z., and Zhu, Q.: The global methane budget 2000–2012, Earth Syst. Sci. Data, 8, 697–751, https://doi.org/10.5194/essd-8-697-2016, 2016.
Saunois, M., Bousquet, P., Poulter, B., Peregon, A., Ciais, P., Canadell, J. G., Dlugokencky, E. J., Etiope, G., Bastviken, D., Houweling, S., Janssens-Maenhout, G., Tubiello, F. N., Castaldi, S., Jackson, R. B., Alexe, M., Arora, V. K., Beerling, D. J., Bergamaschi, P., Blake, D. R., Brailsford, G., Bruhwiler, L., Crevoisier, C., Crill, P., Covey, K., Frankenberg, C., Gedney, N., Höglund-Isaksson, L., Ishizawa, M., Ito, A., Joos, F., Kim, H.-S., Kleinen, T., Krummel, P., Lamarque, J.-F., Langenfelds, R., Locatelli, R., Machida, T., Maksyutov, S., Melton, J. R., Morino, I., Naik, V., O'Doherty, S., Parmentier, F.-J. W., Patra, P. K., Peng, C., Peng, S., Peters, G. P., Pison, I., Prinn, R., Ramonet, M., Riley, W. J., Saito, M., Santini, M., Schroeder, R., Simpson, I. J., Spahni, R., Takizawa, A., Thornton, B. F., Tian, H., Tohjima, Y., Viovy, N., Voulgarakis, A., Weiss, R., Wilton, D. J., Wiltshire, A., Worthy, D., Wunch, D., Xu, X., Yoshida, Y., Zhang, B., Zhang, Z., and Zhu, Q.: Variability and quasi-decadal changes in the methane budget over the period 2000–2012, Atmos. Chem. Phys., 17, 11135–11161, https://doi.org/10.5194/acp-17-11135-2017, 2017.
Schaefer, H., Fletcher, S. E. M., Veidt, C., Lassey, K. R., Brailsford, G. W., Bromley, T. M., Dlugokencky, E. J., Michel, S. E., Miller, J. B., Levin, I., Lowe, D. C., Martin, R. J., Vaughn, B. H., and White, J. W. C.: A 21st-century shift from fossil-fuel to biogenic methane emissions indicated by (CH4)-C-13, Science, 352, 80–84, 2016.
Schwietzke, S., Sherwood, O. A., Ruhwiler, L. M. P. B., Miller, J. B., Etiope, G., Dlugokencky, E. J., Michel, S. E., Arling, V. A., Vaughn, B. H., White, J. W. C., and Tans, P. P.: Upward revision of global fossil fuel methane emissions based on isotope database, Nature, 538, 88–91, 2016.
Sheese, P. E., Walker, K. A., and Boone, C. D.: A global enhancement of hydrogen cyanide in the lower stratosphere throughout 2016, Geophys. Res. Lett., 44, 5791–5797, 2017.
Shindell, D. T., Faluvegi, G., Koch, D. M., Schmidt, G. A., Unger, N., and Bauer, S. E.: Improved Attribution of Climate Forcing to Emissions, Science, 326, 716–718, 2009.
Sweeney, C., Dlugokencky, E., Miller, C. E., Wofsy, S., Karion, A., Dinardo, S., Chang, R. Y. W., Miller, J. B., Bruhwiler, L., Crotwell, A. M., Newberger, T., McKain, K., Stone, R. S., Wolter, S. E., Lang, P. E., and Tans, P.: No significant increase in long-term CH4 emissions on North Slope of Alaska despite significant increase in air temperature, Geophys. Res. Lett., 43, 6604–6611, 2016.
Trenberth, K. E.: Spatial and Temporal Variations of Southern Oscillation, Q. J. Roy. Meteor. Soc., 102, 639–653, 1976.
Troup, A. J.: Southern Oscillation, Q. J. Roy. Meteor. Soc., 91, 490–506, 1965.
Turetsky, M. R., Kotowska, A., Bubier, J., Dise, N. B., Crill, P., Hornibrook, E. R. C., Minkkinen, K., Moore, T. R., Myers-Smith, I. H., Nykanen, H., Olefeldt, D., Rinne, J., Saarnio, S., Shurpali, N., Tuittila, E. S., Waddington, J. M., White, J. R., Wickland, K. P., and Wilmking, M.: A synthesis of methane emissions from 71 northern, temperate, and subtropical wetlands, Glob. Change Biol., 20, 2183–2197, 2014.
Turner, A. J., Frankenbergb, C., Wennberg, P. O., and Jacob, D. J.: Ambiguity in the causes for decadal trends in atmospheric methane and hydroxyl, P. Natl. Acad. Sci. USA, 114, 5367–5372, 2017.
Turner, A. J., Fung, I., Naik, V., Horowitzc, L. W., and Cohen, R. C.: Modulation of hydroxyl variability by ENSO in the absence of external forcing, P. Natl. Acad. Sci. USA, 115, 8931–8936, 2018.
van Aardenne, J. A., Dentener, F. J., Olivier, J. G. J., Goldewijk, C. G. M. K., and Lelieveld, J.: A 1 degrees × 1 degrees resolution data set of historical anthropogenic trace gas emissions for the period 1890–1990, Global Biogeochem. Cy., 15, 909–928, 2001.
van der Werf, G. R., Randerson, J. T., Giglio, L., Collatz, G. J., Kasibhatla, P. S., and Arellano Jr., A. F.: Interannual variability in global biomass burning emissions from 1997 to 2004, Atmos. Chem. Phys., 6, 3423–3441, https://doi.org/10.5194/acp-6-3423-2006, 2006.
van der Werf, G. R., Randerson, J. T., Giglio, L., Collatz, G. J., Mu, M., Kasibhatla, P. S., Morton, D. C., DeFries, R. S., Jin, Y., and van Leeuwen, T. T.: Global fire emissions and the contribution of deforestation, savanna, forest, agricultural, and peat fires (1997–2009), Atmos. Chem. Phys., 10, 11707–11735, https://doi.org/10.5194/acp-10-11707-2010, 2010.
van der Werf, G. R., Randerson, J. T., Giglio, L., van Leeuwen, T. T., Chen, Y., Rogers, B. M., Mu, M., van Marle, M. J. E., Morton, D. C., Collatz, G. J., Yokelson, R. J., and Kasibhatla, P. S.: Global fire emissions estimates during 1997–2016, Earth Syst. Sci. Data, 9, 697–720, https://doi.org/10.5194/essd-9-697-2017, 2017.
Wolf, J., Asrar, G. R., and West, T. O. and Revised methane emissions factors and spatially distributed annual carbon fluxes for global livestock, Carbon Balance Management, 12, 16 pp., 2017.
Wolter, K. and Timlin, M. S.: Monitoring ENSO in COADS with a seasonally adjusted principal component index, Proc. 17th Climate Diagnostics Workshop, 1993.
Wolter, K. and Timlin, M. S.: Measuring the strength of ENSO events: How does 1997/98 rank?, Weather, 53, 315–324, 1998.
Worden, J. R., Bloom, A. A., Pandey, S., Jiang, Z., Worden, H. M., Walker, T. W., Houweling, S., and Rockmann, T.: Reduced biomass burning emissions reconcile conflicting estimates of the post-2006 atmospheric methane budget, Nat. Commun., 8, 2227, https://doi.org/10.1038/s41467-017-02246-0, 2017.
Xie, F., Li, J. P., Tian, W. S., Zhang, J. K., and Sun, C.: The relative impacts of El Nino Modoki, canonical El Nino, and QBO on tropical ozone changes since the 1980s, Environ. Res. Lett., 9, 064020, https://doi.org/10.1088/1748-9326/9/6/064020, 2014.
Yeh, S. W., Kug, J. S., Dewitte, B., Kwon, M. H., Kirtman, B. P., and Jin, F. F.: El Nino in a changing climate, Nature, 462, 511–513, 2009.
Zeng, G., Wood, S. W., Morgenstern, O., Jones, N. B., Robinson, J., and Smale, D.: Trends and variations in CO, C2H6, and HCN in the Southern Hemisphere point to the declining anthropogenic emissions of CO and C2H6, Atmos. Chem. Phys., 12, 7543–7555, https://doi.org/10.5194/acp-12-7543-2012, 2012.
Zhang, Z., Zimmermann, N. E., Calle, L., Hurtt, G., Chatterjee, A., and Poulter, B.: Enhanced response of global wetland methane emissions to the 2015–2016 El Nino-Southern Oscillation event, Environ. Res. Lett., 13, 074009, https://doi.org/10.1088/1748-9326/aac939, 2018.
Zhu, Q., Peng, C., Chen, H., Fang, X., Liu, J., Jiang, H., Yang, Y., and Yang, G.: Estimating global natural wetland methane emissions using process modelling: spatio-temporal patterns and contributions to atmospheric methane fluctuations, Global Ecol. Biogeogr., 24, 959–972, 2015.
Zhu, Q., Peng, C., Ciais, P., Jiang, H., Liu, J., Bousquet, P., Li, S., Chang, J., Fang, X., and Zhou, X.: Inter-annual Variation in Methane Emissions from Tropical Wetlands Triggered by Repeated El Niño Southern Oscillation, Glob. Change Biol., 23, 4706–4716, 2017.