the Creative Commons Attribution 4.0 License.
the Creative Commons Attribution 4.0 License.
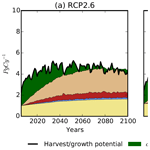
Simulating growth-based harvest adaptive to future climate change
Rasoul Yousefpour
Julia E. M. S. Nabel
Julia Pongratz
Forests are the main source of biomass production from solar energy and take up around 2.4±0.4 PgC per year globally. Future changes in climate may affect forest growth and productivity. Currently, state-of-the-art Earth system models use prescribed wood harvest rates in future climate projections. These rates are defined by integrated assessment models (IAMs), only accounting for regional wood demand and largely ignoring the supply side from forests. Therefore, we assess how global growth and harvest potentials of forests change when they are allowed to respond to changes in environmental conditions. For this, we simulate wood harvest rates oriented towards the actual rate of forest growth. Applying this growth-based harvest rule (GB) in JSBACH, the land component of the Max Planck Institute's Earth system model, forced by several future climate scenarios, we realized a growth potential 2 to 4 times (3–9 PgC yr−1) the harvest rates prescribed by IAMs (1–3 PgC yr−1). Limiting GB to managed forest areas (MF), we simulated a harvest potential of 3–7 PgC yr−1, 2 to 3 times higher than IAMs. This highlights the need to account for the dependence of forest growth on climate. To account for the long-term effects of wood harvest as integrated in IAMs, we added a life cycle analysis, showing that the higher supply with MF as an adaptive forest harvesting rule may improve the net mitigation effects of forest harvest during the 21st century by sequestering carbon in anthropogenic wood products.
- Article
(727 KB) - Full-text XML
-
Supplement
(337 KB) - BibTeX
- EndNote
Forest ecosystems play a major role in taking up global CO2 emissions and affect global climate conditions through a range of complex biophysical and biogeochemical processes. Forests are the main source of biomass production from solar energy through photosynthesis and are estimated to take up globally around 2.4±0.4 PgC yr−1 (Pan et al., 2011). A large part of this uptake can be attributed to direct and indirect human interference: direct human impact by forest management creates young forests that sequester carbon during regrowth (Houghton et al., 2012) and provides material for fossil-fuel substitution (Nabuurs et al., 2013). However, forest utilization and interaction of management with large-scale natural disturbances, such as forest fires, may immediately emit tonnes of CO2 to the atmosphere and act as a source of CO2 emissions (Bonan, 2008). Indirect human impact alters environmental conditions, in particular climate and atmospheric CO2 concentrations, which historically has caused carbon uptake by the terrestrial vegetation (Le Quéré et al., 2018). Any change in environmental conditions affects forest growth, risks of hazards, and productivity and, consequently, the amount of wood that can be harvested (Temperli et al., 2012; Sohngen and Tian, 2016).
The effects of changes in environmental conditions on the state of the biosphere are represented in state-of-the-art Earth system models (ESMs). However, the description of forest management in these models is largely independent of environmental changes: so far, ESMs have employed prescribed wood harvest amounts. These are derived from national statistics for the historical period and from global integrated assessment models (IAMs) for future scenarios, such as Representative Concentration Pathways (RCPs). IAMs determine the wood harvest rates based on the supply of woody materials from vegetation and the demands of regional industries and population (van Vuuren et al., 2011). However, changes in the supply via forest growth and changed structural conditions, especially under climate change and increasing CO2 concentrations, are ignored. The main drivers of these models are economic, i.e., market price, and population growth scenarios and forest harvest decisions are only reactive to the assumed socioeconomic scenarios and do not take forest ecosystem dynamics and growth into account.
In this study we investigate the relevance of changes in environmental conditions for the growth potential of forests and subsequently their harvest potentials. Moreover, we explore the ecological potential of world forest resources for wood production and the implications for carbon mitigation. To assess growth and harvest potentials, we investigate forest growth under various future climate scenarios. We allow forests to be harvested and to regrow in response to the respective changes in environmental conditions, in all scenarios such that the growth increment is removed each year; i.e., the biomass stocks are neither reduced nor increased. We call this “growth-based” harvesting (GB). Removing the annual increment mirrors the forest management concept of “sustained yield”. Sustained yield management is a strong sustainability policy applied in sustainable forest management which aims to maintain forest stocks as natural capital and controls wood extraction (Luckert and Williamson, 2005). According to the sustained yield concept, the maximum wood harvest rate to utilize forest resources equals the actual rate of forest growth. Exceeding regrowth rates would result in the exploitation of forest ecosystems and would decrease forest yield and productivity. On the other hand, minimalistic usage, i.e., falling below regrowth rates, would not be an optimal allocation of forest resources from the perspective of production. However, the traditional concept of sustained yield management, as defined above, does not account for changes in the growth rates (Luckert und Williamson, 2005), although forest growth rates are highly dependent on the environmental conditions (Collins et al., 2018). It has been noted before that any decision about forest management should take into account the effects of changes in climate and CO2 concentrations on forest growth (Yousefpour et al., 2012; Hickler et al., 2015; Sohngen and Tian, 2016; Sohngen et al., 2016) and consequently on the harvest rate (Temperli et al., 2012; Jönsson et al., 2015). Here we demonstrate how altered growth potentials translate into higher harvest potentials under an adaptive growth-based harvest. We first idealize the concept for this study in that GB is applied worldwide, irrespective of the accessibility of the forest for forest management activities, but allowing for the dependence of wood harvest on altered climate conditions and CO2 concentrations. In a second, post-processing, step we link these results to actual harvest potentials by overlaying information on the accessibility of forest areas, where accessibility refers to any hindrance to use the forest, be it due to conservation or biodiversity aspects, or restricted accessibility due to the distance from transport ways or topographical obstacles. For this step we overlay the managed forest area map by Kraxner et al. (2017). Kraxner et al. (2017) used the FAO definition for primary forest and for naturally regenerated forest of native species, when there are no clearly visible indications of human activities and the ecological processes are not significantly disturbed. Using a map of today's managed forest as a proxy for accessibility in the future must be seen as a conservative approach, as technological means to access forests are generally increasing over time.
Keeping in mind the above-mentioned problem of not accounting for changing environmental conditions in global forest utilization modeling, the goal of this study is to establish a modeling framework that allows harvesting rates to respond interactively to environmental changes. We further assess the maximum potential of global forest resources for wood production and the long-term CO2 mitigation effects of wood harvest, which are implicitly (defining future wood harvest rate based on the storyline of RCPs for mitigation) or explicitly (e.g., using wood for bioenergy production) the drivers of forest utilization in IAMs. We compare the outcome of the growth-based harvest with the outcome when applying prescribed wood harvest amounts from three different RCPs realized by IAMs and commonly used by ESMs as external forcing (Hurtt et al., 2011). Since harvested material is used in the IAMs to estimate the amount of bioenergy wood, which in turn is needed in the IAMs to analyze energy and carbon mitigation policies, we perform a first-order assessment of the CO2 consequences of altering the harvest rates in response to climate. Similarly, and to determine the mitigation potential of wood products, we allocate the harvested material to products of different lifetimes according to FAO country-specific statistics (FAOSTAT, 2016). The change in atmospheric carbon content resulting from the release of CO2 by the decay of these products is quantified, accounting for compensating fluxes by the ocean and terrestrial vegetation (Maier-Reimer and Hasselmann, 1987). This impulse response function approach approximates the uptake of emissions by natural sinks in land and ocean, independent of the source, and is a common tool to estimate the fraction of emissions held by the atmosphere over time after the emission occurred (e.g., Pongratz et al., 2011a; O'Halloran et al., 2012). The net mitigation effect of wood harvest is then defined as the difference between the total amount of harvested material and the change in atmospheric carbon content.
2.1 Dynamic global vegetation model JSBACH
We implemented the GB harvesting rule in JSBACH, the land component of the MPI-ESM (Reick et al., 2013). In the applied version of JSBACH, vegetation is represented by 12 plant functional types (PFTs), including 6 woody PFTs. Each PFT is globally endowed with properties in relation to integrated processes in JSBACH and PFT-specific phenology, albedo, morphology, and photosynthetic parameters (Pongratz et al., 2009). Organic carbon is stored in three vegetation pools: living tissue as “green”, woody material as “wood”, sugar and starches as the “reserve pool”, and two soil pools with a fast (about 1 year) and a slow (about 100 years) turnover time (Raddatz et al., 2007). Wood harvest activities do not change the area or characteristics of different PFTs, but affect the carbon pools of woody PFTs (forests and shrubs) by removing carbon from the wood pool, resembling trees' stems' and branches' removal via harvesting (Reick et al., 2013). Harvest thus affects the vegetation carbon stocks, but the model does not represent a feedback of the harvest activity on the forest productivity.
We applied JSBACH in offline mode; i.e., it was not coupled to the atmosphere, but it was driven by the CMIP5 output of the MPI-ESM (Giorgetta et al., 2013) from experiments with CO2 forcing according to three different RCP scenarios (RCP2.6, RCP4.5, and RCP8.5) for the year 2006–2100. We used a T63/1.9∘ horizontal resolution and conducted our simulations with disturbances due to fire and wind. The simulations were conducted without dynamic vegetation and without land-use transitions to prevent changes in the areas occupied by the different PFTs and to be thus able to isolate the effects of forest management activities. Further details on the model version and the simulation setup are given in the Supplement (file S1).
2.2 RCP wood harvest
The current standard module for anthropogenic land cover and land-use change in JSBACH is based on the harmonized land-use protocol (Reick et al., 2013), which provides land-use scenarios for the period 1500–2100 (Hurtt et al., 2011). As part of this protocol, a set of globally gridded harvest maps from the IAM implementations of the RCPs is provided (Hurtt et al., 2011). The prescribed wood harvest rate maps are defined to meet regional timber and bioenergy demand driven by the increasing population (as the model MESSAGE simulates for RCP8.5; Riahi et al., 2011), assumptions about population and labor productivity (GCAM for RCP4.5; Brenkert et al., 2003), or demand, trade, and supply of agricultural products and wood-based bio-energy (IMAGE for RCP2.6; van Vuuren et al., 2011). The RCP wood harvest rates are all based on the demand for wood and bioenergy as the main driver of decisions by IAMs on forest harvest, neglecting changing availability of forest resources under environmental changes. For example, RCP8.5 applies the forest sector model DIMA (Riahi et al., 2011), which is a spatial model for simulating forestry processes to meet specific regional demand on wood and bioenergy. RCP4.5 bases wood harvest rates solely on the price of carbon affected by emissions and mitigation potentials of forestry and agricultural activities (Hurtt et al., 2011). Finally, RCP2.6 relies on the forecasted timber and fuelwood demand from forest resources and applies a series of forest management rules (plantation, clear cutting, selective logging) to meet this demand as the only driver of wood harvest rate in the IMAGE model (Stehfest et al., 2014). In JSBACH simulations, the harvest prescribed in these maps is fulfilled, taking above-ground carbon of all vegetation pools and all PFTs proportionally to the different pool sizes. In this study, however, we concentrate on the carbon harvested from the wood pool of the woody PFTs, which by far contributes most of the harvested volume.
2.3 Growth-based (GB) harvesting rule to estimate growth potentials
As an alternative for the prescribed harvest maps, we implemented the GB harvesting rule, which allows for adaptive wood harvesting reacting to changes in wood increments, and is accordingly dependent on climate and CO2 conditions. We define the GB rule as the allowance to harvest specific volumes of wood to the extent of the average increment (i.e., the average annual growth). Applying GB, we aim to stabilize the wood carbon pool in the woody PFTs at the level of a selected reference period. In the current paper we selected the maximum level for the present period (1996–2005) simulated with JSBACH (see file S1 in the Supplement). Using a reference level determined from the last 10 years of the historical simulation allows us to keep the standing wood at the present level and to account for the dependence of forest growing stocks (carbon pools) on disturbances, silvicultural interventions, and varying environmental conditions. Under the GB harvesting rule, the wood harvest is only allowed to reduce the wood carbon pool down to the reference level. Aside from environmentally driven decreases, the wood carbon pool thus nearly remains constant over the whole simulation time.
2.4 Simulation runs with JSBACH
We conducted six simulations (Table 1) from 2006 to 2100, all starting from the same initial state (see file S1 in the Supplement). The simulations differ in the applied harvest rule and in their climate and CO2 forcing. While the different RCP harvest maps were applied in simulations with the corresponding MPI-ESM RCP forcing, each MPI-ESM RCP forcing was additionally run applying the GB harvesting rule.
2.5 Growth-based harvesting restricted to managed forests (MF)
To infer how much biomass could potentially be harvested (harvest potentials) from the growth potentials simulated under GB, we conduct a post-processing step overlaying a map that masks out forest areas that are subject to conservation and infrastructural limits, or that have not been influenced by human activities so far due to other reasons (Kraxner et al., 2017). Applying nearest neighbor interpolation on the 1 km2 spatially explicit map of primary forest intensity (0 %–100 %; Fig. 6 in Kraxner et al., 2017), we derived a T63 map of primary forest area. This static map was used to filter the growth-based harvest determined in the GB simulations for 2006 to 2100, to only account for managed forests (MF) in the mitigation assessment.
2.6 Analysis of wood harvest impacts on forest disturbances and natural mortality
To analyze the mechanisms driving differences in GB and RCP wood harvest amounts, we can formulate changes in above-ground wood carbon stocks over time (dCw∕dt) as carbon gains from net primary production allocated to the wood pools (NPPw) minus losses due to natural disturbances and anthropogenic management (i.e., wood harvest, h):
In this conceptual formulation, the loss due to natural disturbances depends on the size of the carbon stock and a time constant (τ). As net primary production in our model does not depend on harvest, GB growth potentials (pGB) and RCP harvest can be related as
The amount of growth potential under GB can thus be split into several terms: the first term is the reference harvest rate of the RCPs. The second term accounts for the difference in loss due to natural disturbances in the RCP and the GB simulation. In JSBACH this can further be split into differences in losses due to background mortality, such as self-thinning of forests, due to fire, and due to windbreak. JSBACH explicitly integrates two modules for the simulation of fire and wind disturbances depending on climate and carbon pools. The third term accounts for the changes in the above-ground wood pool realized over time in the simulations. As shown below, the RCP harvest results in an increase of above-ground woody biomass over the 21st century for all three scenarios. For GB, on the other hand, dCwGB∕dt should theoretically be close to zero over time as GB aims to sustain the above-ground carbon pools of woody PFTs; however, reductions in NPP due to less favorable climatic conditions or increased disturbances can entail negative dCwGB∕dt. To summarize, GB includes the RCP wood harvest and, moreover, makes use of additionally accumulated carbon and eventually reduced mortalities to adapt harvest decisions to the novel climate and forest growing conditions.
2.7 Accounting for the mitigation potential of forest management in the Earth system
We account for the long-term effects of wood harvest, as in IAMs, by approaching a life cycle analysis. Many wood products have lifetimes of decades to centuries. Here, we assess the effect on atmospheric carbon content when harvested carbon is transferred, at least in part, to longer lived product pools, instead of entering the atmosphere immediately. We compare this mitigation effect achievable by the wood products harvested under the GB concept after the map of managed forest area is overlaid (MF) to those achievable according to the three RCP harvest maps. To this end, we distinguish three anthropogenic wood product pools – bioenergy, paper, and construction – with 1-, 10-, and 100-year lifetimes, respectively, as are typically assumed in global modeling studies (Houghton et al., 1983; McGuire et al., 2001).
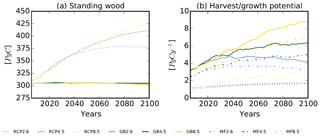
Figure 1Development of global standing wood carbon pools forced by three different RCP scenarios and subject to the harvesting rules of the Representative Concentration Pathways (RCP2.6, RCP4.5, and RCP8.5) or subject to growth-based harvesting (GB2.6, GB4.5, and GB8.5) (a). Development of RCP wood harvest rates, of the growth potential of forests under GB, and of the harvest potential under GB limited to global managed forest area (MF2.6, MF4.5, and MF8.5) (b). All lines are smoothed over 10 years.
To allocate the wood biomass harvested in our JSBACH simulations to different product pools, we made use of FAO country-specific statistics reporting wood production in 14 different categories (FAOSTAT, 2016). For our analysis, we assume that the production technology and allocated percentage of each country's total wood production to these 14 categories remains constant at 2005 levels over the 21st century and used these percentages to allocate wood biomass harvest from JSBACH (remapped to countries – see a calculus example in file S2 in the Supplement). The 14 categories are then assigned to the three distinct anthropogenic wood product pools. We assume that the harvested material entering one of these three product pools in a year decays at a rate of 1 per lifetime, i.e., that all material used for bioenergy is respired to the atmosphere within the same year it is harvested, while the material entering the paper and construction pool is emitted at a constant rate over the following 10 or 100 years, respectively. The emissions at a given year for paper and construction pools are therefore composed of a fraction of that year's harvest, but also of the legacy of material harvested earlier, yielding annual emissions E from all three product pools as follows:
Here, f for bioenergy (b), paper (p), and construction wood (c) are the fractions with which the harvested biomass is assigned to the product pools (see file S2 in the Supplement). We call E “emissions from product decay” in the following.
To account for the fact that the emissions from product decay leave the atmosphere over time to be taken up by the terrestrial biosphere and the ocean, we apply the response function (Eq. 4) by Maier-Reimer and Hasselmann (1987). Convolution of this response function with the time series of annual emissions from product decay until year t results in the change in atmospheric carbon content in that year, C(t) (Eq. 5).
Emissions are present in the atmosphere as they occur and, therefore, G(0)=1 and . The constants Ap and the time constants τp are fitted for p>0 using one of the best fits found by Maier-Reimer und Hasselmann (1987): the sum of four exponential terms with time constants τ1, τ2, τ3, and τ4 of approximately 1.9, 17.3, 73.6, and 362.9 years, and constants a1, a2, a3, and a4 of 0.098, 0.249, 0.321, and 0.201. Accordingly, Eq. (4) is an exponential function that accounts for the uptake of CO2 by ocean and land over time and Eq. (5) integrates the accumulated amount of total CO2 concentrations in the atmosphere at each time step regarding past and present emissions. The mitigation effect of wood products is then determined as the difference between the harvested material and the change in atmospheric carbon content.
3.1 Comparison of GB and RCP harvesting
Above-ground woody biomass is simulated to increase by the end of the 21st century for the RCP wood harvest (Fig. 1a), despite an increase of the amounts of wood harvest (Fig. 1b). This implies that the changes in environmental conditions lead to a larger accumulation of woody biomass than is removed by the increased harvest. Depending on the RCP, the simulated increase in above-ground woody biomass may reach 133 % (425 PgC in 2100) of the initial level in 2005 (320 PgC) for RCP8.5 and substantially higher levels of 128 % and 117 % for RCP4.5 and RCP2.6, respectively (Fig. 1a). The temporal pattern of this increase, with a strong increase only in the first half of the century for RCP2.6 or throughout the century for RCP8.5, reflects the projected evolution of changes in CO2 and climate (Collins et al., 2018).
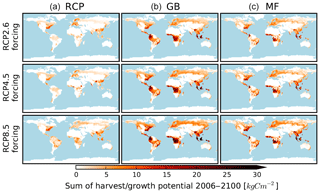
Figure 2Spatial distribution of the harvest realized in JSBACH when harvest rates are prescribed from the Representative Concentration Pathways (a), for the growth-based harvest potential (b), and for the growth-based harvest restricted to the managed forest area (c). All values are summed over the entire simulated period (2006–2100).
For the GB rule, woody biomass remains more or less constant over time (Fig. 1a), as the average annual increment is removed by harvest by definition of the GB rule (see Methods). Consequently, the growth potential of global forest resources under GB is simulated to be as high as 9 PgC yr−1 at the end of the century subject to the realization of RCP8.5 climatic conditions, or about 4 to 6 PgC yr−1 for the other two scenarios (Fig. 1b). About two-thirds of the growth potential lie in managed forest areas and are thus potentially harvestable (Fig. 1b, MF harvest curves). The MF harvest potentials are thus 2 to 3 times (3–7 PgC yr−1) as high as those of prescribed wood harvest simulated by IAMs for the RCPs. Note that, as described in the methods, managed forest areas refer to the present-day state and may expand in the future, which would further increase the harvest potential. These figures are for harvestable wood biomass amount and differ from commercially useable timber including bioenergy, paper, and construction woody biomass (see Sects. 2.7 and 3.3).
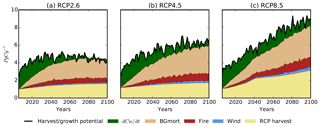
Figure 3Composition of growth-based harvest (GB) forced by different climate change scenarios (RCP2.6, RCP4.5, and RCP8.5 in panels a, b, and c, respectively). dCw∕dt refers to the difference in changes in above-ground woody biomass between Representative Concentration Pathways' and GB harvest (where changes in biomass in GB are by construction of the harvest rule close to 0), BGmort refers to the difference in woody carbon losses between RCP and GB harvest due to background mortality, fire refers to that due to fire disturbance, and wind refers to that due to wind disturbance. GB and RCP harvest are as in Fig. 1b.
We map the geographical distribution of RCP harvest as well as growth and harvest potential under the GB harvesting rule applied to all global forest (GB) and managed forest areas (MF) to recognize regional hotspots (Fig. 2). Central Latin America including the accessible parts of the Amazon forest, large parts of North America, the accessible parts of central Africa, eastern Asia, and Europe including Russia can be recognized under all climate scenarios as hotspots for allocation of simulated harvest activities. The large harvest potentials of the supply-based harvest in the tropics contrast with the patterns of the demand-based RCP harvest; in particular in RCP2.6 and RCP4.5 much of the global harvest is provided from eastern North America, central Europe, and eastern Asia. A reasonable proportion of GB harvest amount in the tropics is masked out in MF as inaccessible forest area; nevertheless the tropics contribute a large harvest potential from the wood supply side in both GB and MF.
3.2 Separation of the processes underlying the growth potentials under future climate scenarios
The harvest potential under the GB harvesting rule in JSBACH exceeds RCPs' wood harvest defined by IAMs, not only because of taking into account changes in growth rates caused by changed environmental conditions, but also due to avoided mortality and disturbances (see Methods section). Figure 3 shows the separation of the growth potential underlying the GB harvest into changes in standing wood as compared to RCP harvest, avoided background mortality, natural fire, and wind disturbances, and the amount prescribed originally by RCPs. The largest contribution to the growth potential under the GB harvesting rules exceeding the RCP harvest is the lower background mortality, which is directly related to lower accumulation of woody biomass (see Fig. 1a). This lower accumulation also leads to the decreased carbon losses from fire and wind disturbances. Depending on the climate scenario (RCPs), the simulated reduction of mortality and disturbances add up to 2–5 PgC yr−1 at the end of the century. Under the RCP harvest, woody biomass is simulated to mostly increase beyond what is required by the increasing harvest rates (see Fig. 1). Harvesting this “surplus”, i.e., the increase of standing biomass over time, by applying RCP harvest rates and harvesting less biomass than the annual increment provides, also contributes to the larger growth potentials under the GB harvesting rule. The temporal evolution is different from that of avoided mortality and disturbances, reflecting the projected changes in CO2 and climate. Greater fluctuation of the growth potential compared to the RCPs' annual wood harvest amounts is because of the direct dependency of the forest's productivity on climate fluctuations.
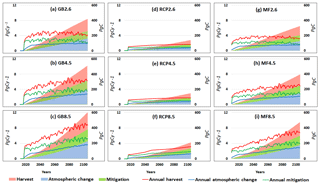
Figure 4Net mitigation potentials from the growth potential under the growth-based harvesting rule (GB) (a, b, c), Representative Concentration Pathways' (RCP) harvest (d, e, f), and GB harvest limited to managed forest area (MF) (g, h, i). Left axes show the annual carbon fluxes due to harvested material and product decay changing atmospheric CO2 concentration, and the mitigation potential of wood products as the difference of both. Right axes accumulate the annual figures over time.
3.3 Mitigation potential of GB versus RCP wood harvest
We show the mitigation potential of forest resources in the 21st century under growth-based harvesting of global forest (GB) and managed forest (MF) areas versus the RCP wood harvest prescribed from IAMs in Fig. 4. Due to the larger harvested amounts, the mitigation potential is higher for GB and MF compared to RCP harvest and the magnitude depends on the underlying climate scenario. The advantage of growth-based harvesting lies in storing a larger amount of carbon in wood products whilst keeping above-ground woody carbon pools constant. These aspects are largely ignored by IAMs. Table 2 below shows the net mitigation potentials of world forest resources (GB and MF against RCP harvest) by wood harvest at the middle and end of the 21st century (2050 and 2100). The highest mitigation effect is achieved in the GB8.5 scenario, with 140.6 and 379.1 PgC up to 2050 and 2100, respectively. These figures account for 278 % and 287 % more global carbon storage than in the RCP8.5 scenario with prescribed RCP wood harvest, with 50.6 and 132.1 PgC mitigation up to 2050 and 2100, respectively. Only considering current managed forests, the mitigation effect realized for MF8.5 still reaches a maximum mitigation potential of 109.3 and 295.8 PgC up to 2050 and 2100, respectively.
RCPs define wood harvest in each region according to scenarios realized by IAMs about social and economic developments in the 21st century, but independent of ecological capacities of forest ecosystems (Hurtt et al., 2011; Riahi et al., 2011; van Vuuren et al., 2011; Stehfest et al., 2014). Although the growth-based harvesting rule realizes a potentially larger wood harvest amount than the RCPs, it remains as per definition a sustained-yield forest harvesting approach and guarantees sustainability of the current ecological conditions at each region with respect to standing biomass. However, as a consequence, regions with low standing biomass, for example due to extensive historical harvest, will maintain these low biomass levels. Below we discuss the effectivity of GB in adapting to new environmental conditions and the mitigation potential and highlight the missing issues in our simulation analysis, especially about the provisioning of multiple goods and services (e.g., biodiversity, forest health), and future research themes about the integration of diversified management strategies in ESMs.
Table 2Net mitigation potentials of GB (growth-based), MF (managed forest), and RCP (Representative Concentration Pathway) harvest at the middle and end of the 21st century.
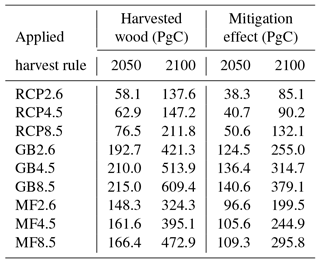
Accounting for the climate state in simulating future forest harvest is crucial (Temperli et al., 2012; Sohngen and Tian, 2016). Accordingly, the novelty of the GB applied in this study is the dynamic nature of this management approach based on the ecology of forest ecosystems and climatic and atmospheric conditions. According to Schelhaas et al. (2010), an accelerated level of wood harvest to reduce the vulnerability of European old forests to wind and fire disturbances is needed to stop the current buildup of growing stock. Applying GB in this study realized an increased wood harvest rate for European forests (see Fig. 2) showing first signs of carbon sink saturation and high vulnerability to natural disturbances (Nabuurs and Maseraet, 2013). Global studies of this nature are largely missing due to the lack of data and forest ecosystems' complexity on the global scale. Our idealized simulations suggest that GB does not only effectively safeguard sustainability of the current forest biomass on the global scale, but also positively affects the resistance of forest resources against natural disturbances and efficiently utilizes forest growth and productivity potentials (see Fig. 3). Our estimates are, of course, sensitive to the choice of reference level: in this study, we applied the maximum current (1996–2005) above-ground wood biomass as the reference level. Any changes in this reference may affect the realized harvest potentials and should be carefully defined regarding ecological potentials and economic implications.
In our simulations, future environmental changes are mostly beneficial for accumulation of forest biomass, apparent from increasing standing biomass in the RCP harvest scenarios or the increase of GB and MF harvest rates over the 21st century. This is in line with other studies projecting above-ground forest carbon storage to increase in the future (e.g., Tian et al., 2016). These effects of environmental changes on forest growth are largely missing in the IAMs providing the wood harvest scenarios to dynamic global vegetation models (DGVMs) and ESMs (see our description of IAMs in Sect. 2.2). IAMs do not account for the fact that the demand side may also be influenced by the availability of the resource, and, accordingly, the increased biomass stocks projected for the future would likely lead to larger wood harvest rates than IAMs simulate by assuming present-day growth conditions. The extent to which accounting for environmental changes may influence estimates of harvestable material (e.g., apparent from comparisons of GB and MF harvest potentials under RCP2.6 as compared to RCP8.5; see Fig. 1) highlights the need to include these effects in models, such as IAMs, that estimate future wood harvest. Our study is limited to considering biomass growth, albeit in interaction with soil conditions also responding to the altered climate. In reality, harvest decisions would consider further variables that depend on environmental conditions, such as the maximum soil expectation value, which are not explicitly simulated neither in our model nor in IAMs.
Note that the estimates of GB wood harvest as provided by our model are not meant as plausible estimates of actual future harvest, which as described before depends not just on resource availability and accessibility of areas, but is also demand-driven by other economic and political considerations. Limiting GB to available managed forest area, MF realized less harvest potential than GB, however, still a larger amount than RCP and with a higher mitigation potential (see Table 2). Also, actual future harvest will interact with other land-use decisions such as changes in forest cover due to agricultural expansion, but also afforestation. We have further not accounted for the effects of wood harvest on biodiversity, forest health, and other ecosystem services. Chaudhary et al. (2015) state that the effect of forest management on species richness, for example, highly depends on the management regime applied. They refer to literature reporting a positive effect of logging activities on species richness as a result of establishing early successional colonizers. Additionally, applying selective logging approaches (e.g., future crop trees of targeted species) for forest management may enhance forest recovery and reduce unintended changes in species composition (Luciana de Avila et al., 2017). Instead of actual forest harvest that considers all these aspects in its decision-making, our study provides an estimate of the ecological potentials for wood harvest. However, the change in resource potentials with climate change forms the ecological basis for realistic decision-making.
There is uncertainty in simulating ecosystem response to environmental changes. Regional forest inventories show an increase in biomass due to historical environmental changes (excluding effects of land-use change) (Pan et al., 2011). The largest sinks are found by these studies to be in the tropical regions, coinciding with our simulated regions of largest potentials for additional wood harvest. Also the other regions showing larger potential for wood harvest under GB and MF than RCP, such as North America, Europe, Russia, and eastern Asia, currently exhibit carbon uptake due to historical environmental changes. This gives some confidence in the robustness of our results, in particular since most models project the carbon sink in vegetation to continue in the future; however, its magnitude is uncertain (Sitch et al., 2008). A large source of uncertainty is the strength of the CO2 fertilization effect (Kauwe et al., 2013; Hickler et al., 2015), which is reflected in a large spread across models in estimates of global total (vegetation plus soil) terrestrial carbon stocks (Arora et al., 2013) and of vegetation productivity (Zaehle et al., 2014). To better assess these effects, we additionally simulated the future GB and MF harvest potentials under present-day climate and CO2 conditions (see simulations of GBpd and MFpd in file S1 in the Supplement). These simulations led to a wood harvest potential larger than that with RCPs' harvest rates and rather constant harvest over time (∼3.2 and 2.7 PgC annually for GBpd and MFpd, respectively; see Fig. S1 in the Supplement). The harvest amount of GBpd is equal to RCP8.5 harvest amount at the end of the century in our simulations. Differences between GBpd and MFpd and the simulations forced by the different RCPs as well as differences among the latter illustrate the effects of changes in climate and CO2 concentration on forest growth and resulting harvest potentials. The differences in wood harvest amounts between the harvest simulations based on GB and MF and those with prescribed RCP wood harvest rates in the first simulation year show differences of applying the supply-based harvest rule (GB and MF) versus the demand-based RCPs under current environmental conditions. The geographic allocation of growth and harvest potentials for GBpd and MFpd (see Fig. S2) resembles those under RCPs, however, with higher global values. The fact that the GBpd and MFpd harvest potential are higher than the RCP harvest implies that the larger potentials as compared to RCP harvest are partly attributable to the harvest simulated by IAMs not using the full sustained, ecological potential (e.g., due to real-world demand). However, the harvest potentials under RCP climate all grow substantially larger than the harvest potential under present-day climate. This depicts the isolated effect of environmental changes, particularly CO2 fertilization, on the simulated potential harvest.
A further uncertainty in the model we used is that our model did not explicitly account for a nitrogen cycle. Nitrogen may become a limiting factor for the additional uptake of carbon in vegetation, although future climate change might also lead to higher nutrient availability due to faster decomposition rates (Friedlingstein and Prentice, 2010). Further, nitrogen deposition may reduce nitrogen limitation (Churkina et al., 2010), and it is not predictable whether artificial fertilization of managed forests may find widespread application in the future. Overall, therefore, quantifications of effects of future climate change on global carbon stocks derived from individual models have to be treated with care. Our model includes present-day nitrogen limitation implicitly by choice of photosynthetic parameters and includes structural limits prohibiting the development of wood densities beyond observational values. Tests with a similar model version to ours but representing an explicit nitrogen cycle suggest a rather small sensitivity of the land carbon cycle to nitrogen limitation under CO2 increases and climate changes in the range of the RCP scenarios investigated here (Goll et al., 2017). The increase in gross primary production (GPP) over the industrial era of our model (or similar versions) lies at the high end, but is within the range of other models (Anav et al., 2013); recent evidence from long-term atmospheric carbonyl sulfide (COS) records shows that models with high GPP growth are most consistent with observations (Campbell et al., 2017). The location of the largest potentials of GB and partly MF harvest simulated in our study being in the tropical forests is consistent with the large carbon sinks derived from inventories for past environmental change (Pan et al., 2011).
GB harvest was simulated to mitigate 255–380 PgC, depending on the realized RCP, through wood product usage for the period 2006–2100 from global forest resources. Moreover, it accounted for sustaining the above-ground wood carbon pool at the reference level of 1996–2005. A comprehensive mitigation study, however, should take into account the total carbon balance of forest ecosystems including soil plus litter carbon. Growth enhanced by environmental changes, as simulated to lead to accumulation of woody biomass in the RCP harvest simulations (Fig. 1a), may lead to larger input to the soil (if not removed by wood harvest). However, soil carbon pools respond differently to environmental changes than forest biomass. In particular, soil carbon models generally assume enhanced soil respiration under higher temperatures (Friedlingstein et al., 2006), which may substantially offset the additional carbon uptake by the vegetation (Ciais et al., 2013). As these processes act the same in our simulations of GB, MF, and RCP harvesting rules (as they share the same climate scenarios), effects of environmental changes on soil carbon will likely not substantially affect our comparison of GB, MF, and RCP harvest in relative terms, but may alter the net carbon balance in each of them. Further, the usage of wood products implies removal of carbon off-field. This can lead to depletion of soil plus litter carbon stocks. Observational data generally found small decreases of soil carbon, but a substantial reduction of deadwood material (Erb et al., 2017). Such effects must be expected to be stronger for GB and MF harvest with its larger harvested biomass than for RCP harvest, reducing on-site carbon stocks, but consequently also soil respiration. Estimating a mitigation potential based on the net carbon balance of vegetation, soil plus litter and product pools therefore would depend on the actual size of soil and vegetation carbon pools and the lifetimes of products relative to the lifetimes of the on-site carbon, which are further subject to a changing climate. There is not a unique lifetime for anthropogenic wood products pools in the literature. Lifetimes of construction wood, for example, spanning from 67 years in Härtl et al. (2017) up to 160–200 years in van Kooten et al. (2007) are applied in recent studies. Regarding global variation of carbon turnover rate, Carvalhais et al. (2013) find mean turnover times of 15 and 255 years for carbon residing in vegetation and soil near to the Equator and higher latitudes over 75∘, respectively. Regarding the uncertainty about the lifetime of anthropogenic wood pools, we stay consistent with the applied figures in FAO statistics (FAOSTAT, 2016) and other land carbon budget studies (Houghton et al., 1983; McGuire et al., 2001).
Despite carbon fluxes being the focus of land-use change as a mitigation tool (e.g., UNFCC, 2012), forest management may enhance or mitigate climate change by a range of other mechanisms such as a change in surface albedo (e.g., Rautiainen et al., 2011; Otto et al., 2014) or turbulent heat fluxes (e.g., Miller et al., 2011). Such biogeophysical effects need to be accounted for in a complete assessment of the mitigation potentials, as has been done for global land cover change (Pongratz et al., 2011b) or for forest management on the local (Bright et al., 2011) or regional scale (Naudts et al., 2016). These biogeophysical effects are particularly important for the local climate (Winckler et al., 2017). In our study, we restrict estimates of mitigation potential to carbon fluxes only and thus focus on the perspective of mitigating global greenhouse gas concentrations. This further allows for a direct comparison of the wood harvest scenarios provided as part of the RCPs.
Different from economic models, ESMs do not consider costs associated with early mitigation measures and thereby implicitly assume a zero social discount rate, meaning that there is no preference for immediate mitigation. However, the discount rate plays a major role in finding the most efficient mitigation action economically (Stern, 2007). Van Kooten et al. (1999) analyzed the sensitivity of investments for carbon sequestration to discount rate in western Canada and found that applying zero discount may not provide enough incentive for increasing carbon storage. However, most forest carbon cost studies are inconsistent in using terms, geographic scope, assumptions, program definitions, and methods (Richards and Stokes, 2004) and may not truly assess carbon sequestration potentials of forest ecosystems. Therefore, if there were a social preference for prompt climate change mitigation, carbon sinks later in the century should be discounted. Regarding the discussion on discount rate, Johnston and van Kooten (2015) argue that applying sufficiently high discount rates in substituting biomass for fossil fuels never leads to carbon neutrality.
We recommend that future research on integration of management strategies in DGVMs and ESMs should regard ecological sustainability as well as socioeconomic challenges. In reality and today, forest management is more of a gamble than a scientific debate (Bellassen and Luyssaert, 2014), and there is no consensus in applying a certain forest harvest rule (e.g., GB) among forest owners, decision-makers, and local users. The rationale for managing forest resources sustainably and efficiently is generally recognized and implemented (Luckert and Williamson, 2005; Elbakidze et al., 2013). However, the process of forest management decision-making is based on past experiences with a business-as-usual strategy. Adaptation to future environmental change and the minimization of the risks associated with climate change impacts have recently been fully integrated in forest research (Lindner et al., 2014); however, this remains at an experimental level in implementation (Yousefpour and Hanewinkel, 2015). Mitigation, in turn, is of public interest and there are some attempts internationally to account for mitigation effects of forest management in carbon policy. International programs such as the Kyoto Protocol encourage forest managers to store carbon in the forest stocks on the ground, applying financial instruments such as tax reduction and direct purchase of carbon offsets. Therefore, inclusion of financial aspects in global forest management modeling and decision-making may help to put scientific results into practice (Hanewinkel et al., 2013). This suggestion is in line with van Vuuren et al. (2011) about the necessity of strengthening the cooperation between integrated assessment models (IAMs) and Earth system modeling communities to improve the understanding of interactions and joint development of environmental and human systems. Our study is the first implementation to account for the climate dependence of forest growth on the global scale for harvest potentials. It suggests the importance of considering this dependence: the growth-based harvest approach (GB) as applied in this study may realize wood harvest potentials 2 to 4 times as high as those of prescribed wood harvest simulated by IAMs for the RCPs and would almost triple the net mitigation effects of wood products. By limiting GB to managed forests (MF), we simulated a lower harvest potential than GB, still 2 to 3 times more than in the IAMs, which could double the net mitigation effect of wood harvest potential in the 21st century. To move from estimates of potentials to actual harvest rates, climate-dependent forest growth needs to be integrated with socioeconomic factors to fully incorporate economic aspects of forestry practices within a dynamic forest growth and yield modeling system.
Scripts used in the analysis and other supplementary information that may be useful in reproducing the authors' work are archived by the Max Planck Institute for Meteorology and can be obtained by contacting publications@mpimet.mpg.de.
Primary data are archived by the Max Planck Institute for Meteorology and can be obtained by contacting publications@mpimet.mpg.de.
The Supplement includes two main files: a pdf document (S1) on the “details on JSBACH, the model version, the simulation setup, and the additional simulation with present-day forcing” and a zipped Excel file (S2) as an example of how “mitigation potentials of woody products in their lifetime” are calculated. The supplement related to this article is available online at: https://doi.org/10.5194/bg-16-241-2019-supplement.
RY and JP initiated the study. RY performed the model simulations. JEMSN implemented the code changes. All authors contributed to analysis of the simulations and the writing of the manuscript.
The authors declare that they have no conflict of interest.
This work was supported by the German Research Foundation's Emmy Noether
Program (PO 1751/1-1). Computational resources were made available by the
German Climate Computing Center (DKRZ) through support from the German
Federal Ministry of Education and Research (BMBF).
Edited by: Anja Rammig
Reviewed by: three anonymous referees
Anav, A., Friendlingstein, P., Kidston, M., Bopp, L., Ciais, P., Cox, P., Jones, C., Jung, M., Myneni, R., and Zhu, Z.: Evaluating the Land and Ocean Components of the Global Carbon Cycle in the CMIP5 Earth System Models, J. Climate, 26, 6801–6843, 2013.
Arora, V. K., Boer, G. J., Friedlingstein, P., Eby, M., Jones, C. D., Christian, J. R., Bonan, G., Bopp, L., Brovkin, V., Cadule, P., Hajima, T., Ilyinam, T., Lindsay, K., Tjiputra, J. F., and Wu, T.: Carbon-concentration and carbon-climate feedbacks in CMIP5 Earth system models, J. Climate, 26, 5289–5314, 2013.
Bellassen, V. and Luyssaert, S.: Carbon sequestration: Managing forests in uncertain times, Nature, 506, 153–155, https://doi.org/10.1038/506153a, 2014.
Bonan, G. B.: Forests and climate change: forcings, feedbacks, and the climate benefits of forests, Science, 320, 1444–1449, https://doi.org/10.1126/science.1155121, 2008.
Brenkert, A., Smith, S., Kim, S., and Pitcher, H.: Model Documentation for the MiniCAM, PNNL-14337, Pacific Northwest National Laboratory, Richland, Washington, USA, 2003.
Bright, R. M., Stromman, A. H., and Peters, G. P.: Radiative Forcing Impacts of Boreal Forest Biofuels: A Scenario Study for Norway in Light of Albedo, Environ. Sci. Technol., 45, 7570–7580, https://doi.org/10.1021/es201746b, 2011.
Campbell, J. E., Berry, J. A., Seibt, U., Smith, S. J., Montzka, S. A., Launois, T., Belviso, S., Bopp, L., and Laine, M.: Large historical growth in global terrestrial gross primary production, Nature, 544, 84–87, 2017.
Churkina, G., Zaehle, S., Hughes, J., Viovy, N., Chen, Y., Jung, M., Heumann, B. W., Ramankutty, N., Heimann, M., and Jones, C.: Interactions between nitrogen deposition, land cover conversion, and climate change determine the contemporary carbon balance of Europe, Biogeosciences, 7, 2749–2764, https://doi.org/10.5194/bg-7-2749-2010, 2010.
Ciais, P., Sabine, C., Bala, G., Bopp, L., Brovkin, V., Canadell, J., Chhabra, A., DeFries, A., Galloway, J., Heimann, M., Jones, C., Le Quéré, C., Myneni, R. B., Piao S., and Thornton, P.: Carbon and Other Biogeochemical Cycles, in: Climate Change 2013: The Physical Science Basis. Contribution of Working Group I to the Fifth Assessment Report of the Intergovernmental Panel on Climate Change, 465–570, 2013.
Elbakidze, M., Andersson, K., Angelstam, P., Armstrong, G. W., Axelsson, R., Doyon, F., Hermansson, M., Jacobsson, J., and Pautov, Y.: Sustained yield forestry in Sweden and Russia: how does it correspond to sustainable forest management policy?, Ambio, 42, 160–173, https://doi.org/10.1007/s13280-012-0370-6, 2013.
Erb, K.-H., Luyssaert, S., Meyfroidt, P., Pongratz, J., Don, A., Kloster, S., Kuemmerle, T., Fetzel, T., Fuchs, R., Herold, M., Haberl, H., Jones, C. D., Marin-Spiotta, E., McCallum, I., Robertson, E., Seufert, V., Fritz, S., Valade, A., Wiltshire, A., and Dolman, A. J.: Land management: data availability and process understanding for global change studies, Glob. Change Biol., 23, 512–533, https://doi.org/10.1111/gcb.13443, 2017.
FAOSTAT: FAOSTAT–Forestry, available at: http://faostat.fao.org/site/626/default.aspx#ancor (last access: September 2018), 2016.
Friedlingstein, P. and Prentice, I. C.: Carbon-climate feedbacks: a review of model and observation based estimates, Curr. Opin. Env. Sust., 2, 251–257, https://doi.org/10.1016/j.cosust.2010.06.002, 2010.
Friedlingstein, P., Cox, P., Betts, R., Bopp, L., Bloh, W. von, Brovkin, V., Cadule, P., Doney, S., Eby, M., Fung, I., Bala, G., John, J., Jones, C., Joos, F., Kato, T., Kawamiya, M., Knorr, W., Lindsay, K., Matthews, H. D., Raddatz, T., Rayner, P., Reick, C., Roeckner, E., Schnitzler, K.-G., Schnur, R., Strassmann, K., Weaver, A. J., Yoshikawa, C., and Zeng, N.: Climate-carbon cycle feedback analysis: Results from the (CMIP)-M-4 model intercomparison, J. Climate, 19, 3337–3353, 2006.
Giorgetta, M. A., Jungclaus, J., Reick, C. H., Legutke, S., Bader, J., Böttinger, M., Brovkin, V., Crueger, T., Esch, M., Fieg, K., Glushak, K., Gayler, V., Haak, H., Hollweg, H.-D., Ilyina, T., Kinne, S., Kornblueh, L., Matei, D., Mauritsen, T., Mikolajewicz, U., Mueller, W., Notz, D., Pithan, F., Raddatz, T., Rast, S., Redler, R., Roeckner, E., Schmidt, H., Schnur, R., Segschneider, J., Six, K. D., Stockhause, M., Timmreck, C., Wegner, J., Widmann, H., Wieners, K.-H., Claussen, M., Marotzke, J., and Stevens, B.: Climate and carbon cycle changes from 1850 to 2100 in MPI-ESM simulations for the Coupled Model Intercomparison Project phase 5, J. Adv. Model. Earth Sy., 5, 572–597, https://doi.org/10.1002/jame.20038, 2013.
Goll, D. S., Winkler, A. J., Raddatz, T., Dong, N., Prentice, I. C., Ciais, P., and Brovkin, V.: Carbon–nitrogen interactions in idealized simulations with JSBACH (version 3.10), Geosci. Model Dev., 10, 2009–2030, https://doi.org/10.5194/gmd-10-2009-2017, 2017.
Hanewinkel, M., Cullmann, D. A., Schelhaas, M.-J., Nabuurs, G.-J., and Zimmermann, N. E.: Climate change may cause severe loss in the economic value of European forest land, Nat. Clim Change, 3, 203–207, https://doi.org/10.1038/NCLIMATE1687, 2013.
Hickler, T., Rammig, A., and Werner, C.: Modelling CO2 Impacts on Forest Productivity, Current Forestry Reports, 1, 69–80, https://doi.org/10.1007/s40725-015-0014-8, 2015.
Houghton, R. A., Hobbie, J. E., Melillo, J. M., Moore, B., Peterson, B. J., Shaver, G. R., Woodwell, G. M.: Changes in the Carbon Content of Terrestrial Biota and Soils between 1860 and 1980: A Net Release of CO2 to the Atmosphere, Ecol. Monogr., 53, 235–262, https://doi.org/10.2307/1942531, 1983.
Houghton, R. A., House, J. I., Pongratz, J., van der Werf, G. R., DeFries, R. S., Hansen, M. C., Le Quéré, C., and Ramankutty, N.: Carbon emissions from land use and land-cover change, Biogeosciences, 9, 5125–5142, https://doi.org/10.5194/bg-9-5125-2012, 2012.
Hurtt, G. C., Chini, L. P., Frolking, S., Betts, R. A., Feddema, J., Fischer, G., Fisk, J. P., Hibbard, K., Houghton, R. A., Janetos, A., Jones, C. D., Kindermann, G., Kinoshita, T., Klein Goldewijk, K., Riahi, K., Shevliakova, E., Smith, S., Stehfest, E., Thomson, A., Thornton, P., van Vuuren, D. P., and Wang, Y. P.: Harmonization of land-use scenarios for the period 1500–2100: 600 years of global gridded annual land-use transitions, wood harvest, and resulting secondary lands, Climatic Change, 109, 117–161, https://doi.org/10.1007/s10584-011-0153-2, 2011.
Jönsson, A. M., Lagergren, F., and Smith, B.: Forest management facing climate change – an ecosystem model analysis of adaptation strategies, Mitig. Adapt. Strat. Gl., 20, 201–220, https://doi.org/10.1007/s11027-013-9487-6, 2015.
Kauwe, M. G. d., Medlyn, B. E., Zaehle, S., Walker, A. P., Dietze, M. C., Hickler, T., Jain, A. K., Luo, Y. Q., Parton, W. J., Prentice, I. C., Smith, B., Thornton, P. E., Wang ShuSen, Wang YingPing, Warlind, D., Weng, E. S., Crous, K. Y., Ellsworth, D. S., Hanson, P. J., Kim HyunSeok, Warren, J. M., Oren, R., and Norby, R. J.: Forest water use and water use efficiency at elevated CO2: a model-data intercomparison at two contrasting temperate forest FACE sites, Glob. Change Biol., 19, 1759–1779, 2013.
Kraxner, F., Schepaschenkoa, D., Fussb, S., Lunnanc, A., Kindermanna, G., Aokie, K., Dürauera, M., Shvidenkoa, A., and Seea, L.: Mapping certified forests for sustainable management – A global tool for information improvement through participatory and collaborative mapping, Forest Policy Econ., 83, 10–18, 2017.
Le Quéré, C., Andrew, R. M., Friedlingstein, P., Sitch, S., Pongratz, J., Manning, A. C., Korsbakken, J. I., Peters, G. P., Canadell, J. G., Jackson, R. B., Boden, T. A., Tans, P. P., Andrews, O. D., Arora, V. K., Bakker, D. C. E., Barbero, L., Becker, M., Betts, R. A., Bopp, L., Chevallier, F., Chini, L. P., Ciais, P., Cosca, C. E., Cross, J., Currie, K., Gasser, T., Harris, I., Hauck, J., Haverd, V., Houghton, R. A., Hunt, C. W., Hurtt, G., Ilyina, T., Jain, A. K., Kato, E., Kautz, M., Keeling, R. F., Klein Goldewijk, K., Körtzinger, A., Landschützer, P., Lefèvre, N., Lenton, A., Lienert, S., Lima, I., Lombardozzi, D., Metzl, N., Millero, F., Monteiro, P. M. S., Munro, D. R., Nabel, J. E. M. S., Nakaoka, S.-I., Nojiri, Y., Padin, X. A., Peregon, A., Pfeil, B., Pierrot, D., Poulter, B., Rehder, G., Reimer, J., Rödenbeck, C., Schwinger, J., Séférian, R., Skjelvan, I., Stocker, B. D., Tian, H., Tilbrook, B., Tubiello, F. N., van der Laan-Luijkx, I. T., van der Werf, G. R., van Heuven, S., Viovy, N., Vuichard, N., Walker, A. P., Watson, A. J., Wiltshire, A. J., Zaehle, S., and Zhu, D.: Global Carbon Budget 2017, Earth Syst. Sci. Data, 10, 405–448, https://doi.org/10.5194/essd-10-405-2018, 2018.
Lindner, M., Fitzgerald, J. B., Zimmermann, N. E., Reyer, C., Delzon, S., van der Maaten, E., Schelhaas, M.-J., Lasch, P., Eggers, J., van der Maaten-Theunissen, M., Suckow, F., Psomas, A., Poulter, B., and Hanewinkel, M.: Climate change and European forests: What do we know, what are the uncertainties, and what are the implications for forest management?, J. Environ. Manage., 146, 69–83, https://doi.org/10.1016/j.jenvman.2014.07.030, 2014.
Luckert, M. K. and Williamson, T.: Should sustained yield be part of sustainable forest management?, Can. J. Forest. Res., 35, 356–364, https://doi.org/10.1139/x04-172, 2005.
Maier-Reimer, E. and Hasselmann, K.: Transport and storage of CO2 in the ocean – an inorganic ocean-circulation carbon cycle model, Clim. Dynam., 2, 63–90, https://doi.org/10.1007/BF01054491, 1987.
McGuire, A. D., Sitch, S., Clein, J. S., Dargaville, R., Esser, G., Foley, J., and Meier, R. A.: Carbon balance of the terrestrial biosphere in the twentieth century: analyses of CO2, climate and land use effects with four process–based ecosystem models, Global Biogeochem. Cy., 15, 183–206, https://doi.org/10.1029/2000GB001298, 2001/
Miller, S. D., Goulden, M. L., Hutyra, L. R., Keller, M., Saleska, S. R., Wofsy, S. C., Silva Figueira, A. M., da Rocha, H. R., and de Camargo, P. B.: Reduced impact logging minimally alters tropical rainforest carbon and energy exchange, P. Natl. Acad. Sci. USA, 108, 19431–19435, https://doi.org/10.1073/pnas.1105068108, 2011.
Nabuurs, G.-J. and Maseraet, O.: Forestry, in: Climate Change 2007: Mitigation, Contribution of Working Group III to the Fourth Assessment Report of the Intergovernmental Panel on Climate Change, edited by: Metz, B., Davidson, O. R., Bosch, P. R., Dave, R., and Meyer, L. A., 2013.
Nabuurs, G.-J., Lindner, M., Verkerk, P. J., Gunia, K., Deda, P., Michalak, R., and Grassi, G.: First signs of carbon sink saturation in European forest biomass, Nat. Clim. Change, 3, 792–796, https://doi.org/10.1038/nclimate1853, 2013.
Naudts, K., Chen, Y., McGrath, M. J., Ryder, J., Valade, A., Otto, J., and Luyssaert, S.: Europe's forest management did not mitigate climate warming, Science, 351, 597–600, 2016.
O'Halloran, T.-L., Law, B. E., Goulden, M. L., Wang, Z., Barr, G. J., Schaaf, C., Brown, M., Fuentes, J. D., Göckede, M., Black, A., and Engel, V.: Radiative forcing of natural forest disturbances, Glob. Change Biol., 18, 555–565, 2012.
Otto, J., Berveiller, D., Bréon, F.-M., Delpierre, N., Geppert, G., Granier, A., Jans, W., Knohl, A., Kuusk, A., Longdoz, B., Moors, E., Mund, M., Pinty, B., Schelhaas, M.-J., and Luyssaert, S.: Forest summer albedo is sensitive to species and thinning: how should we account for this in Earth system models?, Biogeosciences, 11, 2411–2427, https://doi.org/10.5194/bg-11-2411-2014, 2014.
Pan, Y., Birdsey, R. A., Fang, J., Houghton, R., Kauppi, P. E., Kurz, W. A., Phillips, O. L., Shvidenko, A., Lewis, S. L., Canadell, J. G., Ciais, P., Jackson, R. B., Pacala, S. W., McGuire, A. D., Piao, S., Rautiainen, A., Sitch, S., and Hayes, D.: A Large and Persistent Carbon Sink in the World's Forests, Science, 333, 988–993, https://doi.org/10.1126/science.1201609, 2011.
Pongratz, J., Reick, C. H., Raddatz, T., and Claussen, M.: Effects of anthropogenic land cover change on the carbon cycle of the last millennium, Glob. Biogeochem. Cy., 23, https://doi.org/10.1029/2009GB003488, 2009.
Pongratz, J., Caldeira, K., Reick, C. H., and Claussen, M.: Coupled climate-carbon simulations indicate minor global effects of wars and epidemics on atmospheric CO2 between AD 800 and 1850, Holocene, 21, https://doi.org/10.1177/0959683610386981, 2011a.
Pongratz, J., Reick, C. H., Raddatz, T., Caldeira, K., and Claussen, M.: Past land use decisions have increased mitigation potential of reforestation, Geophys. Res. Lett., 38, L15701, https://doi.org/10.1029/2011GL047848, 2011b.
Raddatz, T. J., Reick, C. H., Knorr, W., Kattge, J., Roeckner, E., Schnur, R., Schnitzler, K.-G., Wetzel, P., and Jungclaus, J.: Will the tropical land biosphere dominate the climate-carbon cycle feedback during the twenty-first century?, Clim. Dynam., 29, 565–574, https://doi.org/10.1007/s00382-007-0247-8, 2007.
Rautiainen, M., Stenberg, P., Mottus, M., and Manninen, T.: Radiative transfer simulations link boreal forest structure and shortwave albedo, Boreal. Environ. Res., 16, 91–100, 2011.
Reick, C. H., Raddatz, T., Brovkin, V., and Gayler, V.: Representation of natural and anthropogenic land cover change in MPI-ESM, J. Adv. Model. Earth Sy., 5, 459–482, https://doi.org/10.1002/jame.20022, 2013.
Riahi, K., Rao, S., Krey, V., Cho, C., Chirkov, V., Fischer, G., Kindermann, G., Nakicenovic, N., and Rafaj, P.: RCP 8.5 – A scenario of comparatively high greenhouse gas emissions, Clim. Change, 109, 33–57, https://doi.org/10.1007/s10584-011-0149-y, 2011.
Richards, K. R. and Stokes, C.: A review of forest carbon sequestration cost studies: a dozen years of research, Clim. Change, 63, 1–48, 2004.
Schelhaas, M.-J., Hengeveld, G., Moriondo, M., Reinds, G. J., Kundzewicz, Z. W., ter Maat, H., and Bindi, M.: Assessing risk and adaptation options to fires and windstorms in European forestry, Mitig. Adapt. Strat. Gl., 15, 681–701, https://doi.org/10.1007/s11027-010-9243-0, 2010.
Sitch, S., Huntingford, C., Gedney, N., Levy, P. E., Lomas, M., Piao, S. L., Betts, R., Ciais, P., Cox, P., Friedlingstein, P., Jones, C. D., Prentice, I. C., and Woodward, F. I.: Evaluation of the terrestrial carbon cycle, future plant geography and climate-carbon cycle feedbacks using five Dynamic Global Vegetation Models (DGVMs), Glob. Change Biol., 14, 2015–2039, https://doi.org/10.1111/j.1365-2486.2008.01626.x, 2008.
Sohngen, B. and Tian, X.: Global climate change impacts on forests and markets, Forest Policy Econ., 72, 18–26, https://doi.org/10.1016/j.forpol.2016.06.011, 2016.
Sohngen, B., Tian, X., and Kim, J.: Global Climate Change Impacts on Forests and Markets, Forest Policy Econ.,72, 18–26, 2016.
Stehfest, E., van Vuuren, D., Kram, T., and Bouwman, L.: Integrated Assessment of Global Environmental Change with IMAGE 3.0, PBL Netherlands Environmental Assessment Agency, The Hague, the Nethermands, 2014.
Stern, N.: The Economics of Climate Change: The Stern Review, Cambridge University Press, Cambridge, UK, 2007.
Temperli, C., Bugmann, H., and Elkin, C.: Adaptive management for competing forest goods and services under climate change, Ecol. Appl., 22, 2065–2077, 2012.
Tian, X., Sohngen, B., Kim, J. B., Ohrel, S., and Cole, J.: Global climate change impacts on forests and markets, Environ. Res. Lett., 11, 035011, https://doi.org/10.1088/1748-9326/11/3/035011, 2016.
UNFCC: Report of the Conference of the Parties serving as the meeting of the Parties to the Kyoto Protocol on its seventh session, held in Durban from 28 November to 11 December 2011: Addendum decisions adopted by the Conference of the Parties serving as the meeting of the Parties to the Kyoto Protocol, United Nations, Geneva, Nairobi, New York, Vienna, 49 pp., 2012.
van Kooten, G. C., Krcmar-Nozic, E., Stennes, B., and van Gorkom, R.: Economics of fossil fuel substitution and wood product sinks when trees are planted to sequester carbon on agricultural lands in western Canada, Can. J. Forest. Res., 29, 1669–1678, https://doi.org/10.1139/cjfr-29-11-1669, 1999.
van Vuuren, D. P., Stehfest, E., den Elzen, Michel G. J., Kram, T., van Vliet, J., Deetman, S., Isaac, M., Klein Goldewijk, K., Hof, A., Mendoza Beltran, A., Oostenrijk, R., and van Ruijven, B.: RCP2.6: Exploring the possibility to keep global mean temperature increase below 2 ∘C, Clim. Change, 109, 95–116, https://doi.org/10.1007/s10584-011-0152-3, 2011.
van Kooten, G. C. and Sohngen, B.: Economics of Forest Carbon Sinks: A Review, International Review of Environmental & Resource Economics, 1, 237–269, 2007.
Winckler, J., Reick, C., and Pongratz, J.: Robust identification of local biogeophysical effects of land-cover change in a global climate model, J. Climate, 30, 1159–1176, https://doi.org/10.1175/JCLI-D-16-0067.1, 2017.
Yousefpour, R. and Hanewinkel, M.: Forestry professionals' perceptions of climate change, impacts and adaptation strategies for forests in south-west Germany, Clima. Change, 130, 273–286, https://doi.org/10.1007/s10584-015-1330-5, 2015.
Yousefpour, R., Jacobsen, J. B., Thorsen, B. J., Meilby, H., Hanewinkel, M., and Oehler, K.: A review of decision-making approaches to handle uncertainty and risk in adaptive forest management under climate change, Ann. For. Sci., 69, 1–15, https://doi.org/10.1007/s13595-012-0192-5, 2012.
Zaehle, S., Medlyn, B. E., Kauwe, M. G. de, Walker, A. P., Dietze, M. C., Hickler, T., Luo, Y., Wang, Y.-P., El-Masri, B., Thornton, P., Jain, A., Wang, S., Warlind, D., Weng, E., Parton, W., Iversen, C. M., Gallet-Budynek, A., McCarthy, H., Finzi, A. C., Hanson, P. J., Prentice, I. C., Oren, R., and Norby, R. J.: Evaluation of 11 terrestrial carbon-nitrogen cycle models against observations from two temperate Free-Air CO2 Enrichment studies, New Phythol., 202, 803–822, https://doi.org/10.1111/nph.12697, 2014.