the Creative Commons Attribution 4.0 License.
the Creative Commons Attribution 4.0 License.
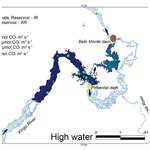
Carbon dioxide (CO2) concentrations and emission in the newly constructed Belo Monte hydropower complex in the Xingu River, Amazonia
Kleiton R. de Araújo
Henrique O. Sawakuchi
Dailson J. Bertassoli Jr.
André O. Sawakuchi
Karina D. da Silva
Thiago B. Vieira
Nicholas D. Ward
Tatiana S. Pereira
The Belo Monte hydropower complex located in the Xingu River is the largest run-of-the-river (ROR) hydroelectric system in the world and has one of the highest energy production capacities among dams. Its construction received significant media attention due to its potential social and environmental impacts. It is composed of two ROR reservoirs: the Xingu Reservoir (XR) in the Xingu's main branch and the Intermediate Reservoir (IR), an artificial reservoir fed by waters diverted from the Xingu River with longer water residence time compared to XR. We aimed to evaluate spatiotemporal variations in CO2 partial pressure (pCO2) and CO2 fluxes (FCO2) during the first 2 years after the Xingu River impoundment under the hypothesis that each reservoir has contrasting FCO2 and pCO2 as vegetation clearing reduces flooded area emissions. Time of the year had a significant influence on pCO2 with the highest average values observed during the high-water season. Spatial heterogeneity throughout the entire study area was observed for pCO2 during both low- and high-water seasons. FCO2, on the other hand, only showed significant spatial heterogeneity during the high-water period. FCO2 (0.90±0.47 and 1.08±0.62 µmol m2 d−1 for XR and IR, respectively) and pCO2 (1647±698 and 1676±323 µatm for XR and IR, respectively) measured during the high-water season were on the same order of magnitude as previous observations in other Amazonian clearwater rivers unaffected by impoundment during the same season. In contrast, during the low-water season FCO2 (0.69±0.28 and 7.32±4.07 µmol m2 d−1 for XR and IR, respectively) and pCO2 (839±646 and 1797±354 µatm for XR and IR, respectively) in IR were an order of magnitude higher than literature FCO2 observations in clearwater rivers with naturally flowing waters. When CO2 emissions are compared between reservoirs, IR emissions were 90 % higher than values from the XR during low-water season, reinforcing the clear influence of reservoir characteristics on CO2 emissions. Based on our observations in the Belo Monte hydropower complex, CO2 emissions from ROR reservoirs to the atmosphere are in the range of natural Amazonian rivers. However, the associated reservoir (IR) may exceed natural river emission rates due to the preimpounding vegetation influence. Since many reservoirs are still planned to be constructed in the Amazon and throughout the world, it is critical to evaluate the implications of reservoir traits on FCO2 over their entire life cycle in order to improve estimates of CO2 emissions per kilowatt for hydropower projects planned for tropical rivers.
- Article
(4861 KB) -
Supplement
(343 KB) - BibTeX
- EndNote
Rivers and streams are no longer considered passive pipes where terrestrial organic matter (OM) travels unchanged from land to sea (Cole et al., 2007). The OM transported by inland waters may be converted to carbon dioxide (CO2) or methane (CH4) and escape to the atmosphere as gaseous emissions (Battin et al., 2009; Ward et al., 2013). Inland waters cover an approximate area of 4.6 to 5 ×106 km2 or about 3 % of Earth's land surface (Downing et al., 2006; Verpoorter et al., 2014). Roughly 5.1 Pg C yr−1 is mobilized into inland waters from the terrestrial biosphere (Drake et al., 2017), of which about 2.1 Pg C yr−1 is emitted to the atmosphere as CO2 (Raymond et al., 2013). Despite the relatively small area covered by inland waters, their carbon emissions offset the ocean's carbon sink (1.42±0.53 Pg C yr−1) (Landchützer et al., 2014).
Channel impoundment promotes several changes in river properties such as surface wind shear, water temperature, discharge and turbulence, and organic and inorganic sediment input (St. Louis et al., 2000). These changes alter the microbial community structure and biogeochemical processes in the water column and riverbed sediments, with consequent impacts on the dissolved carbon load, production, and eventual release to the atmosphere as CO2 (Battin et al., 2008). The intense decomposition of OM contained in flooded soils, in addition to the consumption of allochthonous OM deposited in the reservoir, may lead to an increase in the CO2 production, and outgassing, particularly during the first years of channel impoundment (Guérin et al., 2006). Longer water residence time and reduction in water flow velocity, on the other hand, may increase light penetration depth due to the deposition of suspended sediments, possibly counterbalancing those emissions due to higher CO2 uptake by primary producers (Duarte and Prairie, 2005). Alternatively, this condition may stimulate OM decomposition via photooxidation that is favored by increased light absorbance (Miller and Zepp, 1995) and microbial priming effects driven by interactions between allochthonous and autochthonous carbon sources (Ward et al., 2016).
Some of the hydropower dam impacts may be minimized according to the dam design. Run-of-the-river (ROR) hydropower systems maintain a similar flow to a natural river (Csiki and Rhoads, 2010), which generates smaller reservoirs that operate according to seasonal variations in water levels (Egré and Milewski, 2002). The Belo Monte hydropower complex in the lower Xingu River operates as a ROR dam, and it is the largest hydropower plant in the Amazon. It ranks third in the world in terms of installed capacity (11 233 MW), but with high variation in energy production throughout the year due to the high seasonality of the water discharge of the Xingu River (EPE, 2009). Significant debate has surrounded the Belo Monte hydropower project since its initial survey in the 1980s due to the magnitude of the environmental impact and threat to local indigenous people (Fearnside, 2006). These discussions lasted at least 20 years and resulted in a series of changes and revisions to the initial project (Fearnside, 2006). Nevertheless, the Belo Monte hydropower complex had its reservoirs filled in 2015 (MME, 2011), amid strong environmental controversies (Fearnside, 2017) including uncertainties in estimates of greenhouse gas (GHG) emissions (Fearnside, 2002). As such, alterations in the natural carbon cycling in the aquatic environments under direct and indirect influence of the Belo Monte hydropower facilities may result in significant impacts on the regional carbon budget. This is a critical question to evaluate the GHG emissions related to hydroelectricity produced from impoundment of large tropical rivers.
Hundreds of new hydropower reservoirs are currently under construction or planning stages in tropical South America, Africa, and Asia (Winemiller et al., 2016), and many of them may be ROR reservoirs. However, to our knowledge, estimates of GHG emissions from ROR reservoirs only include measurements performed several decades after the construction of a small temperate reservoir in Switzerland or obtained through modeling for tropical reservoirs in Brazil (DelSontro et al., 2010; Faria et al., 2015). Therefore, most of the GHG emissions estimates available in the literature are for storage reservoirs but also with measurements representative of several years (>10 years) after the construction of the hydropower dams (Kemenes et al., 2011; Lima et al., 2002). Exceptions are a tropical storage reservoir (Abril et al., 2005) and a boreal storage reservoir (Teodoru et al., 2011) studied since impoundment. These studies showed that CO2 emissions were higher during the first years of impoundment. Thus, estimates of GHG emissions immediately after river impoundment are critical for determining the overall carbon balance of the hydroelectricity system lifetime.
The Belo Monte hydropower plant has two reservoirs operating under ROR conditions. The Xingu Reservoir (XR) was formed by the impoundment of the Xingu River channel, which has waters diverted to feed the Intermediate Reservoir (IR), created by the impoundment of a valley artificially connected to the left margin of the Xingu River. Although both reservoirs are considered to be ROR, they differ in water residence time and type of flooded vegetation and substrates. Flooded areas in the XR correspond mainly to seasonally flooded forest, but upland forest in marginal areas was also flooded locally. Vegetation was removed from most of the flooded areas, but a part of the flooded forest islands in the XR was not cleared. On the other hand, the IR flooded large swaths of upland forest, and pasture areas and its water residence time is higher than in the XR.
The aim of this study is to evaluate CO2 emissions from the Belo Monte hydropower complex during the first 2 years post-impoundment by assessing the spatial and temporal variability in CO2 partial pressure (pCO2) and CO2 fluxes (FCO2) in the XR and IR. This evaluation is crucial to understand GHG emissions from reservoirs in the eastern Amazon, a tropical region poised to add 153 more hydropower facilities in the coming decades (Aneel, 2019). Considering the physiographic and hydraulic differences in the XR and IR, we hypothesize that (1) the two Belo Monte reservoirs have contrasting pCO2 and FCO2 and (2) the clearing of forest vegetation significantly reduces the emissions from areas flooded by the reservoirs during the first 2 years after channel impoundment.
2.1 Study area
The Xingu River is the second largest clearwater tributary of the Amazon River. It drains an area of 504 000 km2 and flows from central Brazil (15∘ S) to the lower Amazon River in eastern Amazon (3∘ S) (Latrubesse et al., 2005; Eletrobrás, 2009a). Clearwater rivers are characterized by neutral to slightly alkaline pH, low concentration of suspended sediment, and high light penetration (Sioli, 1984). The climate of the region has high seasonality with the rainy period usually starting in December, extending until May, and rainfall peaking in March and April (Inmet, 2017). The dry season occurs from June to November with the driest months occurring in September and October (Fig. 1). The average monthly rainfall and temperature were 188±145 mm and 27.5±1.0 ∘C, respectively (10-year average from 2004 to 2014) (Inmet, 2017). In accordance with the rainfall regime, river discharge is marked by strong seasonality with the low-water season occurring from September to November and the high-water season from March to May. The historic average discharge of the Xingu River in the sector of the Belo Monte hydropower complex for the period from 2004 to 2014 was 1408±513 m3 s−1 during the low-water season and 18 983 ± 9228 m3 s−1 in the high-water season (Fig. 1) (ANA, 2017). The dominant land cover in the middle and lower Xingu watershed is tropical rainforest, although agriculture and deforested areas occur mainly in the southern and southwestern areas of the basin and close to Altamira, the largest city near the Belo Monte hydropower complex (Eletrobrás, 2009a). The studied area ranges from the lower Iriri River, the largest tributary of the Xingu River, to downstream of the sector known as Volta Grande do Xingu (Xingu Great Bend), near the municipality of Vitória do Xingu (Fig. 2).
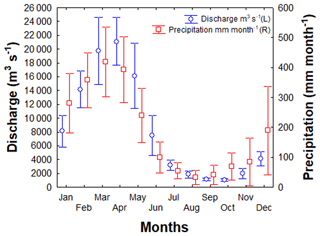
Figure 1Average river discharge (m3 s−1) of the Xingu River (left y axis) and precipitation (millimeters per month, right y axis) at Altamira from 2004 to 2014. Bars indicate monthly standard deviation. Data are from ANA (2017) and Inmet (2017).
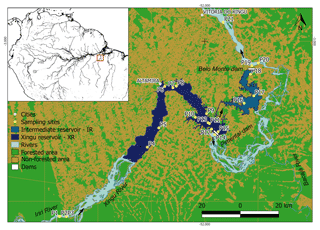
Figure 2Sampling sites upstream (Iriri River), within, and downstream of the reservoirs and the location of the two dams (white bars) in the Xingu River. Black arrows indicate flow direction. Land cover data are based on the vegetation characterization from Almeida et al. (2016), where nonforested area groups are pasture, deforested, secondary vegetation, and urban areas.
The construction of Belo Monte started in 2011 and reservoirs (Fig. 2) were flooded in 2015 (EPE, 2011). The studied reservoirs have maximum depths reaching 20.5 m in the XR and 58.3 m in the IR, although both dams have similar intake depths of about 15–20 m. The Pimental dam in the Xingu River channel hosts six turbines and floodgates that regulate the water flow from the XR through a 28 km channel to feed the IR formed by the Belo Monte dam. The latter contains the main power station with 18 turbines summing 11 000 MW of potential energy production, equivalent to 97 % of the total installed power capacity of 11 233 MW (Eletrobrás, 2009b; EPE, 2009).
Together the reservoirs occupy an area of 516 km2. The XR extends over an area of 382 km2 (Eletrobrás, 2009a) from which 94 km2 corresponds to land permanently or seasonally flooded, similar to the natural water level condition during the high-water season (Fig. 2). It is estimated that 52 % of the total area flooded by the XR was not cleared of vegetation (Norte Energia, 2015). Differently, the IR occupies an area of 134 km2 and large flooded areas of pasture and upland nonflooded forest (locally called “terra firme forest”). Contrary to the XR, the IR flooded area was totally cleared of vegetation before reservoir filling (Norte Energia, 2015). Waters diverted from the XR return to the Xingu River channel after flowing around 34 km over flooded lands in the IR (Fig. 2) (Eletrobrás, 2009b; EPE, 2009). The sector of the Xingu River between the outflows of the XR and IR, including part of the Xingu Great Bend, has reduced water discharge and flow controlled by operational conditions of the Belo Monte hydropower complex.
The residence times (RTs) of the XR and IR were calculated based on the maximum potential discharge established for each dam (Eletrobrás, 2009a). We assumed that the sum of both discharges is the total discharge in an extreme scenario, and therefore equivalent to the fraction of the total river discharge passing through each dam. The fraction of discharge was combined with the historical average annual discharge of the Xingu River (ANA, 2017), similarly to Faria et al. (2015), using the following Eq. (1):
where RT is the water residence time given in seconds, and later converted into days; V is the reservoir volume in cubic meters (m3); and Q is the volumetric discharge in cubic meters per second (m3 s−1). The XR has an RT of 3.4 d, while IR has an RT of 20.2 d. This difference was used to test if the RT plays a significant role in the CO2 emissions in ROR reservoirs.
2.2 pCO2 and FCO2 to the atmosphere
In order to cover zones with different flooded substrates and hydrologic characteristics, the sampling sites included the original river channel within the XR, flooded lands (forest and pasture) of both reservoirs, and upstream and downstream river channel sections outside the influence of the reservoirs (Fig. 2). Four classes were considered to evaluate the spatial heterogeneity of FCO2:
- i.
Unaffected river channel include sites located in the channels of the Xingu and Iriri rivers outside reservoir areas, in sectors upstream and further downstream of the reservoirs;
- ii.
Main channel includes the Xingu River main branch within the reservoir area (XR);
- iii.
Flooded areas include lands of pasture and upland forest formerly nonflooded during the high-water-level season and seasonally flooded forested islands that were permanently inundated by both reservoirs;
- iv.
Downstream of the dams includes sites immediately downstream of the dams that receive the water discharge from turbines of the XR and IR dams.
Sampling sites near the confluence of the Xingu and Iriri rivers (sites P1 and P3, Table 1) were used as reference sites for areas without direct influence of the reservoirs. The sites further downstream of the dams (P20 and P21) were characterized to investigate the influence of the reservoirs on the downstream FCO2 (Table 1).
Table 1Locations of sampling sites in the Xingu and Iriri rivers and reservoirs (XR and IR) of the Belo Monte hydropower complex. Sites were classified according to pre- and post-flooded vegetation types, water depth, and sampling season (H1: high-water season of 2016, H2: high-water season of 2017, and L: low-water season of 2017).
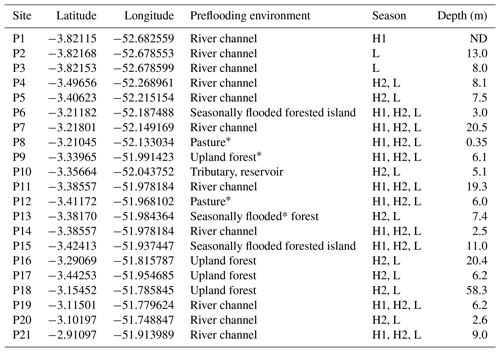
ND – no data collected. * Vegetation not removed prior to reservoir filling.
During the year of 2017 (high-water-level and low-water-level seasons), values of pCO2 in the water column were obtained using the headspace equilibration method according to Hesslein et al. (1991). The pCO2 was measured following three depth classes (Table 1): (i) near bottom (0.5–1.0 m above the river or reservoir bottom), (ii) 60 % (at 60 % of total water depth), and (iii) surface (up to 0.3 m of water depth). Sites shallower than 7.5 m were sampled only at 60 % of the total depth. Polycarbonate bottles of 1 L were overflowed 3 times their volume with water drawn by a submersible pump. The bottle was closed with rubber stopper adapted with tubes and Luer-lock valves, allowing the simultaneous injection of 60 mL of atmospheric air and withdrawal of the same volume of water using syringes, creating the headspace. The bottles were shaken for 3 min to equilibrate the gas in the water and headspace air. Water was then reinjected simultaneously to the collection of the headspace air. Atmospheric air samples were also collected using 60 mL syringes for corrections related to atmospheric CO2. All gas samples were transferred from syringes to glass vials that were precapped with butyl rubber stoppers and evacuated with a vacuum pump. pCO2 was measured using a Picarro® G2201-i cavity ring-down spectrometer (CRDS), and concentration calculations were based on Wiesenburg and Guinasso Jr. (1979).
Diffusive CO2 emission was measured with floating chambers during 2016 and 2017 high-water seasons using an infrared gas analyzer (IRGA) LI-COR® LI-820 coupled to a 7.7 L opaque (covered with reflexive aluminum tape) floating chamber with 0.08 m2 of area and 11.7 cm of height. The analyzer captures the change in CO2 concentration inside the chamber by constant recirculation driven by a micropump with an air flow of 150 mL min−1. For each site, three consecutive deployments were made for 5 min each from a drifting boat to avoid extra turbulence. During the 2017 low-water season CO2 miniloggers (Bastviken et al., 2015) placed inside 6 L opaque (covered with reflexive aluminum tape) floating chambers with 0.07 m2 of area and 10.5 cm of height were used to measure FCO2. Sensors were placed inside the two chambers and deployed simultaneously during 20–30 min with a logging frequency of 30 s. FCO2 fluxes from water to the atmosphere were calculated according to Frankignoulle et al. (1998):
The FCO2 (mol CO2 m−2 s−1) is given by the changes in pCO2 inside the chamber during the deployment time (δpCO2∕δt, µatm s−1), taking into account the chamber volume (V, m3), the universal gas constant (R, atm m3 mol−1 K−1), water temperature (T, K) and the area covered by the chamber (A, m2). Measurements were discarded when the R2 of the linear relation between pCO2 and time (δpCO2∕δt) was lower than 0.90 (R2<0.90) or had negative FCO2 values with surface pCO2 higher than atmospheric pCO2 measured on site. The gas sampling survey (Fig. 2 and Table 1) occurred during the high-water-level seasons in April 2016 and May 2017 and during the low-water-level season in September 2017. Due to technical difficulties, pCO2 data were only collected during 2017 and FCO2 samplings of 2017 were made with different equipment.
2.3 Gas transfer velocity (k600)
The air–water gas transfer coefficient k (cm h−1) of CO2 was estimated based on the surface water CO2 concentration inside the floating chamber by Eq. (3):
where V and A are the chamber volume (cm3) and area (cm2), respectively; α is the Ostwald solubility coefficient (dimensionless); t is the time (h); and the subscripts w, i, and f refer to the partial pressure in the surface water and initial and final times inside the chamber, respectively. Ostwald solubility coefficient was calculated from K0 as described by Wanninkhof et al. (2009). Finally, k values were normalized to k600 following the Eqs. (4) and (5) (Alin et al., 2011; Jähne et al., 1987; Wanninkhof, 1992):
where kT is the measured k value at in situ temperature (T), ScT is the Schmidt number calculated from temperature, and 600 is the Schmidt number for temperature of 20 ∘C. The Schmidt number is calculated as a temperature (T) function:
2.4 Physicochemical characteristics
Depth profiles with a measurement interval of 1 m were done for water temperature, pH, dissolved oxygen (DO), and conductivity using a multiparameter probe (EXO2®, YSI). During the high-water-season sampling campaigns in 2016 and 2017, technical challenges prevented measurement of pH, dissolved oxygen (DO), and conductivity during the 2017 low-water sampling. For statistical analysis these measurements were selected following the same water depth classes applied to pCO2 measurements (surface, 60 %, and near the bottom). Additionally, air temperature and wind speed were measured at the same time as chamber deployments with a handheld meteorological meter (Kestrel® 5500) positioned at 2 m above the water surface.
2.5 Statistical analysis
Statistical analyses were performed to check the correlation among CO2 variables (FCO2 and pCO2) and water column characteristics (pH, dissolved oxygen (DO), and water temperature) and to evaluate the spatial and seasonal variation in FCO2, pCO2, and k600. Normality and heterogeneity of variance were not achieved by Shapiro–Wilk and Bartlett tests, respectively. Thus, nonparametric and multivariate statistical tests were used. The seasonal and spatial variability in FCO2, pCO2, k600, and wind velocity were tested by PERMANOVA (permutational multivariate analysis of variance; Anderson, 2001), a multivariate test that compares group variance (within and between) through a distance matrix using permutation to achieve p value. The Euclidian index was used as distance method and 9999 permutations to run the analysis. The FCO2 statistics were assessed separately by season due to the different sampling methods. The Spearman correlation test (Zar, 2010) was performed to evaluate the correlation between FCO2 versus pCO2, FCO2 versus wind speed, k600 versus wind speed, and pCO2 versus physicochemical variables (pH, DO, and water temperature). All statistical analyses were performed in R (R Core Team, 2016) using the Vegan package (Oksanen et al., 2017) and Statistica (StatSoft 8.0) using 5 % (0.05) as critical alpha for significance.
3.1 Temporal and spatial variability in pCO2 and FCO2
Mean pCO2 from areas upstream and downstream of the dams was 1163±660 µatm. Based on 2017 data, pCO2 values differed significantly between seasons (, R2=0.09, p=0.0045) with higher pCO2 in the high-water season (1391±630 µatm) compared to the low-water period (976±633 µatm) (Fig. 3a). The type of environment also had a significant role in pCO2 distribution throughout the area affected by the reservoirs (, R2=0.37, p=0.0002). During the high-water season the highest average pCO2 was observed downstream of the dams. In contrast, during the low-water season the highest average pCO2 values were observed in the reservoirs over the flooded areas. Unaffected river channel categorized areas had the lowest pCO2 in both seasons (Fig. 3).
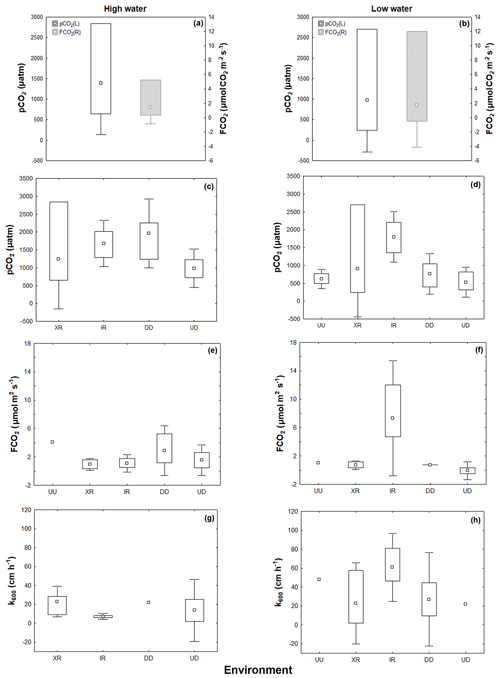
Figure 3Boxplots showing the spatial and temporal variability in pCO2 and FCO2. Whiskers indicate standard deviation, boxes are maximum and minimum values, and the middle points are mean values. High-water FCO2 (2016 and 2017 campaigns) and pCO2 from all depth values were averaged to characterize the environmental category. Sampling sites were categorized according to river flow in unimpounded upstream (UU) to sites located upstream of reservoirs (Xingu Reservoir, XR, and Intermediate Reservoir, IR) that grouped sites within reservoir areas; downstream of the dams (DD) that corresponded to sites directly receiving turbine outflow; and unimpounded downstream (UD) related to sites further downstream with no or low reservoir influence. Temporal variation may be observed by the overall seasonal variation in pCO2 and FCO2 during high (a) and low water (b); likewise, the spatial distribution to pCO2 on high (c) and low water (d) is shown. Also, FCO2 (e, f) and k600 (g, h) by season are displayed for high- and low-water seasons, respectively.
On average, across all seasons bottom water had higher pCO2 (1269±689 µatm) compared to surface water (998±613 µatm) (, R2=0.07, p=0.0261) (Table 2). Surface pCO2 was positively correlated with FCO2 both during the high-water (r=0.80; p=0.0009) and low-water (r=0.71; p=0.012) seasons (Fig. 3). Bottom water pCO2 showed correlation with FCO2 only during the high-water season (r=0.68; p=0.042), while data from the low-water season have a nonsignificant correlation (r=0.45; p=0.16) (Table 3). Average FCO2 for all sites sampled during 2016 and 2017 high-water seasons was 1.38±1.12 µmol CO2 m−2 s−1 with similarity between years (, R2=0.01, p=0.7790). Therefore, FCO2 data from the high-water seasons of 2016 and 2017 were treated as a single data set for further calculations.
Table 2Summary of FCO2 (µmol CO2 m2 s−1), pCO2 (µatm), gas transfer velocity (k600, cm h−1) averages and literature values. High-water season averages for FCO2 correspond to 2016 and 2017 high-water seasons since no significant variation was detected. Env represents environment, Res represents reservoirs, Camp represents sampling campaign, Season represents sampling season, and n represents number of sites averaged to each variable.
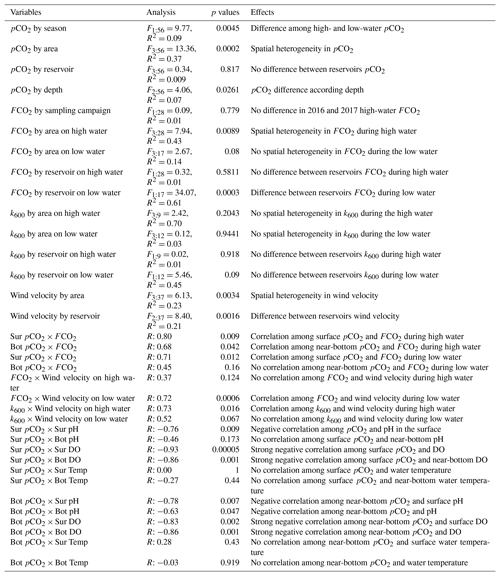
IR – Intermediate Reservoir. ND – no data available. UR – unaffected river channel. XR – Xingu Reservoir.
Table 3Statistical analysis results grouped by variable. The pseudo-F (F) and R2 on the analysis column are related to the PERMANOVA test and R (rho) values are related to the Spearman correlation. Prefixes Sur and Bot represent surface and near-bottom depths, respectively; DO is dissolved oxygen; and Temp is water temperature. Temporal, spatial, and correlation implications of statistics are described as Effects.
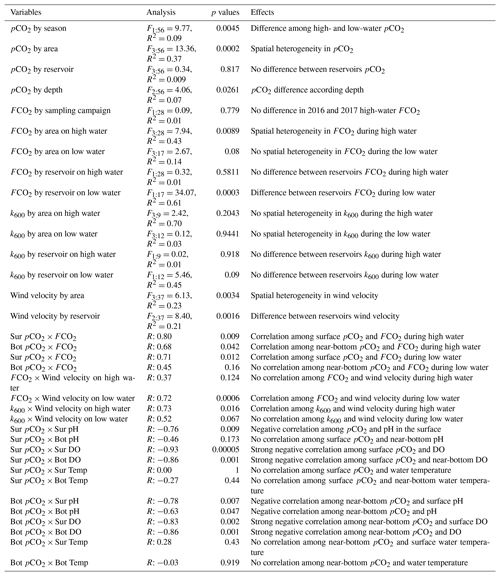
The highest (12.00±3.21 µmol CO2 m−2 s−1) and lowest (−0.52 µmol CO2 m−2 s−1) FCO2 values were observed during the low-water season (Fig. 3). Significant difference in FCO2 was observed among environments sampled during high-water season (, R2=0.43, p=0.0089), while the low-water season was not statistically different (, R2=0.14, p=0.08) (Fig. 4 and Table 3) when considering the whole study area. The highest (2.89±1.74 µmol CO2 m−2 s−1) and lowest (0.84±0.42 µmol CO2 m−2 s−1) average FCO2 occurred in sectors downstream of the dams and in flooded areas sampled during the high-water season, respectively. Negative FCO2 values were exclusively observed during the low-water season in the river channel (Table 2 and Fig. 4).
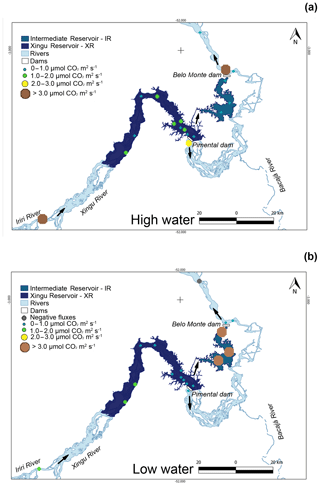
Figure 4Spatial and temporal variation in the FCO2 values (µmol CO2 m−2 d−1) in the reservoirs (XR and IR) of the Belo Monte hydropower complex during high water includes 2 years of data (2016 and 2017) while (a) low water only has 1 year (2017) (b). Black arrows indicate flow direction; colors and circle sizes indicate the type and intensity of CO2 fluxes.
In addition to the spatial heterogeneity, preexisting vegetation cover influenced pCO2 and FCO2 in the XR. Areas previously covered by pasture, upland forest, and seasonally flooded forest had significantly different CO2 concentrations. Sites that were 90 and 25 km downstream of the Pimental (XR) and Belo Monte (IR) dams, respectively, had lower pCO2 and FCO2 values compared to areas within the reservoirs.
3.2 pCO2 and FCO2 in the reservoirs
The spatial variability in pCO2, FCO2, and k600 was assessed within and between reservoirs. We evaluated the total CO2 emissions from reservoirs by grouping flooded areas and the river channel of the XR for comparison with flooded areas from the IR. FCO2 and pCO2 presented higher values in the XR during the high-water season, while the opposite pattern occurred in the IR (Table 2).
XR and IR seasonal variation was not significant even when high-water (, R2=0.03, p=0.1536) and low-water (, R2=0.03, p=0.4684) seasons were evaluated separately (Table 3). pCO2 also showed no significant difference between XR and IR (, R2=0.009, p=0.8170). As observed for pCO2, there was no effect of reservoir type on FCO2 variability during high-water conditions (, R2=0.01, p=0.5811). In contrast, FCO2 during low-water conditions differed significantly between XR and IR (, R2=0.61, p=0.0003). The IR had the highest average FCO2 (7.32±4.06 µmol CO2 m−2 s−1) during the low-water season, while the XR presented low FCO2 (0.69±0.28 µmol CO2 m−2 s−1). Despite variations in FCO2 and pCO2, no difference in k600 was observed between reservoirs during the high-water (, R2=0.01, p=0.9180) or low-water seasons (, R2=0.45, p=0.0900) (Table 3).
3.3 Gas transfer velocity (k600)
The average k600 was 17.8±10.2 and 34.1±24.0 cm h−1 for high- and low-water seasons, respectively, without significant spatial heterogeneity across environments (, R2=0.70, p=0.2043 and , R2=0.03, p=0.9441, respectively). Values of k600 are correlated with wind speed (r=0.73; p=0.016) during the high-water season, although this observation was not significant during the low-water season (r=0.53; p=0.067).
Wind speeds ranged from 0.7 to 4.8 m s−1, when considering measurements for all sites and sampling periods. Highest average wind speed was observed on the river channel environment, while downstream of the dams had the lowest (3.21±0.89 and 1.66±0.88 m s−1, respectively) (Table 4). In contrast to k600, wind speed varied significantly across environments (, R2=0.23, p=0.0034), including variation between the XR and IR (, R2=0.21, p=0.0016).
Table 4Overall physicochemical characterization comprising the three depth classes (surface, 60 %, and near the bottom) sampled during the high-water seasons of 2016 and 2017, except Temp (water temperature) and WS (wind speed), which correspond to both high and low water. The variables pH, DO (dissolved oxygen), Cond (conductivity), Temp, and WS (wind speed) are presented according to the environment.

3.4 Physicochemical characteristics
The air temperatures at the studied sites varied between 27.5 and 33.8 ∘C during sampling in both seasons, with the maximum temperatures registered during the low-water period. The surface water temperature ranged from 29.2 to 32.7 ∘C, with maximum temperature registered during the high-water period. The lowest (6.60±0.26) and highest (6.81±0.21) average pH values were in waters of flooded areas and the river channel, respectively (Table 4). The water column was relatively well oxygenated in all studied environments, reaching average DO concentration up to 7.28±0.73 mg L−1 in the unaffected river channel and lowest concentration in flooded areas (5.44±2.00 mg L−1) (Table 4). Water conductivity varied from 20.60 to 38.30 µS cm−1 in the studied environments with the highest average value (31.60±8.63 µS cm−1) recorded in flooded areas and lowest value (29.30±4.85 µS cm−1) in areas downstream of the dams (Table 4). In the study sites, pCO2 is negatively and strongly correlated with pH and DO (Table 3). Correlation between pCO2 and water temperature was absent while FCO2 was positively correlated with wind speed (Table 3).
4.1 Temporal and spatial variability in pCO2 and FCO2
Although pCO2 and FCO2 are typically correlated (Rasera et al., 2013), in this study we observed several examples where variability in gas transfer velocities drive variable fluxes even when pCO2 was fairly constant. It has been shown that the amount of CO2 in the water column and CO2 emissions from Amazon rivers to the atmosphere vary significantly among seasons with higher fluxes generally observed during the high-water season (Alin et al., 2011; Rasera et al., 2013; Richey et al., 2002; Sawakuchi et al., 2017). We observed significant variability in pCO2 between high- and low-water seasons, as well as in terms of physiographic–hydrologic environment, which influenced FCO2 values. High pCO2 production during the high-water season can be related to increased input of terrestrial organic and inorganic carbon into the rivers by surface runoff and subsurface flow of water (Raymond and Saiers, 2010; Ward et al., 2017). Remaining vegetation and soils are the major sources of OM in areas flooded by hydropower reservoirs that sustain high rates of CO2 production during the initial years of impoundment (Guérin et al., 2008). In addition, the seasonal input of autochthonous and allochthonous organic material deposited in the reservoirs with higher water RT may result in seasonal pCO2 and FCO2 variability.
The oversaturation in CO2 observed for XR and IR during high-water conditions was spatially heterogeneous (Table 2). In the river channel environment of the XR, pCO2 decreased as FCO2 increased and the contrary occurred in flooded areas. This is perhaps due to the main OM source to the XR being standing vegetation associated with remnant flooded forests and pasture, which agrees with higher pCO2 from flooded areas. Flooded vegetation is recognized to be the main source of OM in reservoirs, playing an important role in the CO2 production and creating gradients of reservoir CO2 emissions (Roland et al., 2010; Teodoru et al., 2011). The different characteristics including vegetation clearing, variation on hydrodynamic conditions, water depth (Teodoru et al., 2011; Roland et al., 2010), and OM availability (Cardoso et al., 2013) may explain the difference in the observed FCO2 and pCO2 values.
About 59 % of the XR area is the original channel of the Xingu River. However, the water velocity under reservoir conditions is slower than in channel sectors outside the effect of dams and regulated by spillways of the Pimental dam. FCO2 measured upstream of the XR during the high-water season in a sector where the channel is flowing under natural conditions (Iriri River sites) was significantly higher than in the XR sector (Table 2). CO2 concentrations in the water column may decrease, especially in upper water layers, in response to the increased photosynthetic uptake of CO2 during lower rainfall periods (Amaral et al., 2018). During the low-water season, pCO2 and FCO2 decreased resulting in homogeneous FCO2 likely due to photosynthetic activity in all environments, with the exception of the IR (Table 2). In addition, CO2 undersaturation relative to the atmosphere and observed CO2 uptake may be attributed to elevated primary productivity, which is facilitated by the high light penetration and has been similarly observed in previous studies in Amazonian floodplain lakes and other clearwater rivers during the low-water season (Amaral et al., 2018; Rasera et al., 2013; Gagne-Maynard et al., 2017). The occurrence of negative FCO2 was observed only in the unaffected river channel at the furthest downstream site. This pattern can be related to the downstream decrease in suspended sediments due to increased sediment deposition in the reservoirs. FCO2 in the XR and IR may also be favored by wind activity due to larger fetch for wave formation within the reservoirs. Wave action could favor degassing as well as the increase in suspended sediments that reduce light penetration and photosynthetic activity. These processes may also result in the observed decrease in pCO2 and FCO2 downstream of the dams. The site downstream of IR (P21) is within the river extent (<30 km) that could still be affected by the reservoir similar to observations downstream of the Amazonian Balbina reservoir (Kemenes et al., 2016). However, the XR should only have a minor effect on the downstream site due to its longer distance from the dam outflow (90 km) and the presence of many large rapids and waterfalls in the Volta Grande region, quickly degassing the dissolved CO2 coming from the upstream reservoir. The decrease in pCO2 and FCO2 persisted in areas downstream of the Belo Monte reservoirs as indicated by measurements performed in this study during the high-water and low-water seasons. The river reaches downstream of the Belo Monte dams have CO2 emissions similar to observations from previous studies with emissions also decreasing downstream (Abril et al., 2005; Kemenes et al., 2011).
River reaches downstream of tropical storage reservoirs FCO2 measured in the Sinnamary River downstream of the Petit-Saut reservoir in French Guiana was 10.49±3.94 µmol CO2 m−2 s−1 (Guérin et al., 2006), which is more than 3 times our average downstream FCO2 (2.89±1.74 µmol CO2 m−2 s−1) during high-water season (Table 2). Although the Petit-Saut dam has a smaller reservoir, its turbine intake is hypolimnetic (Abril et al., 2005), capturing CO2-rich bottom waters that increase downstream emissions through turbine passage (Guérin et al., 2006; Kemenes et al., 2011, 2016). Alternatively, the Belo Monte hydropower facility operates as a ROR dam and has waters mixed without stratification and lower CO2 oversaturation than in the Petit-Saut reservoir likely due to vegetation clearing.
4.2 pCO2 and FCO2 on Belo Monte reservoirs
The IR presented an average FCO2 about 90 % higher than values observed in the XR during low-water season. Although the XR has a larger surface area than the IR (excluding the water diversion channel), most of it corresponds to the natural river channel under a hydraulic condition similar to the high-water season with less flooded areas, restricted to narrow upland margins, but including large flooded forested islands. On the other hand, the higher flooded area extension of the IR was previously covered by upland forest and pasture resulting in higher organic matter availability. CO2 emissions from the IR during the low-water season were even above the range of emissions observed in storage reservoirs in the Amazon such as the Tucuruí hydropower complex, built in 1984 on the clearwater Tocantins River (Lima et al., 2002). After more than 30 years, the Tucuruí reservoir still contributes 3.61±1.62 µmol CO2 m−2 s−1 to the atmosphere (Lima et al., 2002). In comparison to the XR ( µmol CO2 m−2 s−1) the Tucuruí reservoir has higher FCO2. However, this is 3 times lower than FCO2 (7.32±4.06 µmol CO2 m−2 s−1) measured in the IR during the low-water season.
Some characteristics of the Tucuruí reservoir such as the lack of vegetation clearing prior to flooding and large reservoir area contribute to its relatively high GHG emissions (Fearnside, 2002). It must be considered that XR had partial vegetation removal in some areas, while IR had its entire landscape cleared. FCO2 and pCO2 measured during high-water conditions in the Belo Monte reservoirs area (Table 2) were of the same order of magnitude as emissions measured in Amazon clearwater rivers unaffected by impoundment including the Tapajós River, which has hydrologic conditions similar to the Xingu River (Table 5) (Alin et al., 2011; Rasera et al., 2013; Sawakuchi et al., 2017). The vegetation clearing possibly maintained the low CO2 emissions on both reservoirs during high water. However, the CO2 emission from the IR is higher during low water, exceeding the fluxes of the Amazon River (Tables 2 and 5). When analyzed separately, average FCO2 values observed for XR and IR overcome these natural emissions. Based on the Belo Monte case, ROR dams are a CO2 source to the atmosphere similar to natural rivers during high-water season. However, the associated reservoir may promote increased CO2 emission during the low-water season compared to natural emissions from river channels.
Table 5Average literature values and standard deviation of FCO2, pCO2, and k600 to Amazonian clearwater rivers according to season. Referential values were averaged from the Amazonian clear water rivers Tapajós (Alin et al., 2011; Sawakuchi et al., 2017), Araguaia, Javaés, and Teles Pires (Rasera et al., 2013) in the correspondent season when available.
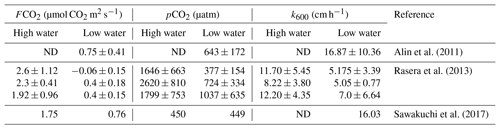
Our highest FCO2 values were observed in the IR during the low-water season, which is in contrast to previous observations in other tropical and subtropical reservoirs in China and French Guiana (Abril et al., 2005; Wang et al., 2015). In the aforementioned reservoirs, lower pCO2 was observed during the low-water season, which was attributed to high photosynthetic rates in the epilimnion. pCO2 in the XR and other sites outside the reservoirs in the Xingu River also showed lower pCO2 during the low-water season, indicating that higher fluxes may have been mitigated by enhanced primary productivity caused by reduced turbidity. Residence time can also play an important role in pCO2. For example, the Three Gorges reservoir has a peak in pCO2 and low chlorophyll a concentrations during summer and spring seasons when RT is the lowest (Li et al., 2017). In this case the reservoir type (river type) directly influences water mixing and consequently the RT, similar to the differences observed here between the IR and XR. In low RT reservoirs, nitrogen and phosphorous may not be the limiting factor to phytoplankton growth and it may be restricted by the high flow (Xu et al., 2011). The deficit in CO2 consumption related to an underperforming phytoplankton community may point to a imbalanced sink in the reservoir carbon balance that remains poorly understood.
CO2 emissions may be correlated with prior vegetation flooding with higher FCO2 occurring in areas with the highest carbon stocks such as forests and wetlands (Teodoru et al., 2011). Although vegetation was cleared in the IR before flooding, the upper soil layer may have kept a high concentration of plant-derived material fueling emissions. This condition explains the higher average pCO2 in IR compared to XR with the former area also having higher average FCO2 values. The XR has substrates with relatively reduced carbon storage because almost half of the area represents the original river channel dominated by bedrock or sandy substrates and islands formed by sand and mud deposition, which would not store as much carbon (Sawakuchi et al., 2015).
4.3 Gas transfer velocity (k600)
Although no significant difference in k600 was observed between the reservoirs of the Belo Monte hydropower complex, the observed gas transfer velocities vary among different environment types. The XR had gas transfer velocities in the range of the Furnas reservoir in the Grande River draining the Cerrado biome (savanna), which has a k600 of 19.6±2.5 cm h−1 (Paranaíba et al., 2017). This value is similar to k600 values obtained in this study for the XR (23.0±8.0 and 22.9±21.4 cm h−1 during high- and low-water seasons, respectively). In contrast, the IR had a k600 of 7.1±1.5 cm h−1 (high water), which resembles gas transfer velocities of the Lago Grande de Curuai (6.0 cm h−1, following Cole and Caraco wind-based model) (Rudorff et al., 2011) in the floodplain of the Amazon River. We observed that in the XR reservoir area, FCO2 values were higher in the main channel environment. In addition, the relatively stable water flow due to the ROR-type reservoir also had a large fetch area for wave formation in comparison with the sheltered flooded areas in bays and small tributaries. This is consistent with the positive correlation observed between wind speed and FCO2 here and in other large rivers where a vast water surface interacts with wind along its fetch, promoting the formation of waves that enhance water turbulence, k600, and FCO2 (Abril et al., 2005; Paranaíba et al., 2017; Rasera et al., 2013; Raymond and Cole, 2001; Vachon et al., 2013). In addition, in the low-water season the elevated gas transfer coefficients coupled with the short water residence time suggests that the system has a strong influence of water turbulence on k600.
In this study, we observed significant variability in FCO2 related to the type of fluvial environment and land use of areas flooded by the reservoirs of the Belo Monte hydropower complex. The observed CO2 emissions were 90 % higher for the IR compared to XR during low-water season indicating that flooded land and higher residence time may play important roles in CO2 emissions to the atmosphere even in ROR reservoirs. Our measurements comprise the first 2 years after reservoir filling, which is a critical period to assess GHG emissions from reservoirs. During the high-water season, the XR had average CO2 emissions similar to Amazonian clearwater rivers without impounding and considerably lower emissions than several other tropical reservoirs that have been studied. However, CO2 emissions during the low-water season were higher than natural emissions and the IR FCO2 exceeded emissions measured in storage reservoirs of other tropical rivers. ROR reservoirs alter CO2 emissions compared to naturally flowing Amazonian clearwater rivers, except when installed on the main river channel. On upland forested areas, ROR reservoirs can experience significantly increased CO2 production rates due to preimpoundment vegetation and soil organic matter. Despite vegetation removal the, IR had the highest FCO2 observed in this study. Although vegetation removal is considered an effective approach for reducing GHG emissions from hydropower reservoirs we show that tropical reservoirs can still have significant emissions even after vegetation suppression. A long-term monitoring of GHG emissions at Belo Monte working at full capacity, and including a more detailed assessment of the downstream sections of the reservoirs, is needed to obtain a robust estimate of carbon emissions related to the energy produced by the Belo Monte hydropower complex over its entire life cycle.
All data are available in the figures and tables of the article and its Supplement. Meteorological and hydrological data were obtained from government agency databases:
ANA: Agência Nacional Das Águas, available at: https://www.snirh.gov.br/hidroweb/publico/medicoes_historicas_abas.jsf, last access: 27 August 2017.
Inmet: Instituto Nacional De Meteorologia, available at: http://www.inmet.gov.br/projetos/rede/pesquisa/, last access: 12 July 2017.
The supplement related to this article is available online at: https://doi.org/10.5194/bg-16-3527-2019-supplement.
KRdA collected and analyzed the data and prepared the article with the contribution of all co-authors. HOS designed the study, cooperated in the field sampling, and supported with guidance on data analysis. DJB Jr. also collected the data and conducted the laboratory analysis. AOS attained the grant award, contributed to setting up the field equipment and measuring infrastructure, and designed the field sampling. KRdA, KDS, and TBV conducted the statistical analysis. NDW and TSP contributed with technical advice and guidance throughout the project implementation and paper-writing stages.
The authors declare that they have no conflict of interest.
This study has been funded by Fundação de Amparo à Pesquisa do Estado de São Paulo (FAPESP) and from Coordenação de Aperfeiçoamento de Pessoal de Nível Superior (CAPES) as a master's scholarship for Kleiton R. de Araújo. We are grateful to Marcelo G. P. de Camargo, Hildegard de H. Silva, Victor A. T. Alem, Agna L. B. Figueiredo, and Thomas K. Akabame for the field sampling and laboratorial support. We are thankful to Oliver Lucanus for final text contributions and to FAPESP for a doctoral scholarship for Dailson J. Bertassoli Jr. André O. Sawakuchi is supported by Conselho Nacional de Desenvolvimento Científico e Tecnológico (CNPq). This publication was supported by Pró-Reitoria de Pesquisa e Pós-Graduação/ UFPA (PROPESP/ UFPA).
This research has been supported by the FAPESP (grant nos. 16/02656-9 and 2016/11141-2), the CNPq (grant no. 304727/2017-2), and the CAPES (grant no. PPGBC-2017).
This paper was edited by Ji-Hyung Park and reviewed by two anonymous referees.
Abril, G., Guérin, F., Richard, S., Delmas, R., Galy-Lacaux, C., Gosse, P., Tremblay, A., Varfalvy, L., Dos Santos, M. A., and Matvienko, B.: Carbon dioxide and methane emissions and the carbon budget of a 10-year old tropical reservoir (Petit Saut, French Guiana), Global Biogeochem. Cy., 19, 1–16, https://doi.org/10.1029/2005GB002457, 2005.
Alin, S. R., Rasera, M. D. F. F. L., Salimon, C. I., Richey, J. E., Holtgrieve, G. W., Krusche, A. V., and Snidvongs, A.: Physical controls on carbon dioxide transfer velocity and flux in low-gradient river systems and implications for regional carbon budgets, J. Geophys. Res.-Biogeo., 116, G01009, https://doi.org/10.1029/2010JG001398, 2011.
Almeida, C. A., Coutinho, A. C., Esquerdo, J. C. D. M., Adami, M., Venturieri, A., Diniz, C. G., Dessay, N., Durieux, L., and Gomes, A. R.: High spatial resolution land use and land cover mapping of the Brazilian Legal Amazon in 2008 using Landsat-5/TM and MODIS data, Acta Amazon., 46, 291–302, https://doi.org/10.1590/1809-4392201505504, 2016.
Amaral, J. H. F., Borges, A. V., Melack, J. M., Sarmento, H., Barbosa, P. M., Kasper, D., de Melo, M. L., De Fex-Wolf, D., da Silva, J. S., and Forsberg, B. R.: Influence of plankton metabolism and mixing depth on CO2 dynamics in an Amazon floodplain lake, Sci. Total Environ., 630, 1381–1393, https://doi.org/10.1016/j.scitotenv.2018.02.331, 2018.
ANA: Agência Nacional Das Águas, available at: https://http://www.snirh.gov.br/hidroweb/publico/medicoes_historicas_abas.jsf, last access: 27 August 2017.
Anderson, M. J.: A new method for non-parametric multivariate analysis of variance, Austral Ecol., 26, 32–46, 2001.
Aneel: Agência Nacional de Energia Elétrica, available at: http://www.aneel.gov.br/, last acess: 30 May 2019.
Bastviken, D., Sundgren, I., Natchimuthu, S., Reyier, H., and Gålfalk, M.: Technical Note: Cost-efficient approaches to measure carbon dioxide (CO2) fluxes and concentrations in terrestrial and aquatic environments using mini loggers, Biogeosciences, 12, 3849–3859, https://doi.org/10.5194/bg-12-3849-2015, 2015.
Battin, T. J., Kaplan, L. A., Findlay, S., Hopkinson, C. S., Marti, E., Packman, A. I., Newbold, J. D., and Sabater, F.: Biophysical controls on organic carbon fluxes in fluvial networks, Nat. Geosci., 2, 595–595, https://doi.org/10.1038/ngeo602, 2008.
Battin, T. J., Luyssaert, S., Kaplan, L. A., Aufdenkampe, A. K., Richter, A., and Tranvik, L. J.: The boundless carbon cycle, Nat. Geosci., 2, 598–600, https://doi.org/10.1038/ngeo618, 2009.
Cardoso, S. J., Vidal, L. O., Mendonça, R. F., Tranvik, L. J., Sobek, S., and Roland F.: Spatial variation of sediment mineralization supports differential CO2 emissions from a tropical hydroelectric reservoir, Front. Microbiol., 4, 101, https://doi.org/10.3389/fmicb.2013.00101, 2013.
Cole, J. J., Prairie, Y. T., Caraco, N. F., McDowell, W. H., Tranvik, L. J., Striegl, R. G., Duarte, C. M., Kortelainen, P., Downing, J. A., Middelburg, J. J., and Melack J.: Plumbing the global carbon cycle: Integrating inland waters into the terrestrial carbon budget, Ecosystems, 10, 171–184, https://doi.org/10.1007/s10021-006-9013-8, 2007.
Csiki, S. and Rhoads, B. L.: Hydraulic and geomorphologival effects of run-of-the-river dams, Prog. Phys. Geogr., 34, 755–780, https://doi.org/10.1177/0309133310369435, 2010.
DelSontro, T., McGinnis, D. F., Sobek, S., Ostrovsky, I., and Wehrli, B.: Extreme methane emissions from a Swiss hydropower reservoir: contribution from bubbling sediments, Environ. Sci. Technol., 44, 2419–2425, https://doi.org/10.1021/es9031369, 2010.
Downing, J. A., Prairie, Y. T., Cole, J. J., Duarte, C. M., Tranvik, L. J., Striegl, R. G., McDowell, W. H., Kortelainen, P., Caraco, N. F., Melack, J., and Middelburg, J. J.: The global abundance and size distribution of lakes, ponds, and impoundments, Limnol. Oceanogr., 51, 2388–2397, https://doi.org/10.4319/lo.2006.51.5.2388, 2006.
Drake, T. W., Raymond, P. A., and Spencer, R. G. M.: Terrestrial carbon inputs to inland waters: A current synthesis of estimates and uncertainty, Limnol. Oceanogr. Lett., 3, 132–142, https://doi.org/10.1002/lol2.10055, 2017.
Duarte, C. M. and Prairie, Y. T.: Prevalence of heterotrophy and atmospheric CO2 emissions from aquatic ecosystems, Ecosystems, 8, 862–870, https://doi.org/10.1007/s10021-005-0177-4, 2005.
Egré, D. and Milewski, J. C.: The diversity of hydropower projects, Energ. Policy, 30, 1225–1230, https://doi.org/10.1016/S0301-4215(02)00083-6, 2002.
Eletrobrás: Hydroelectric utilization of the Xingu river basin, AAI – Integrated Environmental Assessment of the Xingu River Basin, São Paulo, 204 pp., 2009a (in Portuguese).
Eletrobrás: Belo Monte hydroelectric power, Environmental Impact Study, Rio de Janeiro, 426 pp., 2009b (in Portuguese).
EPE: Energy Research Company – Generation epanxion bidding studies AHE Belo Monte, Technical Evaluation, Mines and Energy Ministry, Rio de Janeiro, 87 pp., 2009 (in Portuguese).
EPE: Energy Research Company – Ten Year Expansion Plan 2020, Final Report, Mines and Energy Ministry, Brasília, 319 pp., 2011 (in Portuguese).
Faria, F. A. M., Jaramillo, P., Sawakuchi, H. O., Richey, J. E., and Barros, N.: Estimating greenhouse gas emissions from future Amazonian hydroelectric reservoirs, Environ. Res. Lett., 10, 124019, https://doi.org/10.1088/1748-9326/10/12/124019, 2015.
Fearnside, P. M.: Greenhouse Gas Emissions from a Hydroelectric Reservoir (Brazil's Tucurui Dam) and the Energy Policy Impactions, Water Air Soil Poll., 133, 69–96, https://doi.org/10.1023/A:1012971715668, 2002.
Fearnside, P. M.: Dams in the Amazon: Belo Monte and Brazil's hydroelectric development of the Xingu River Basin, Environ. Manage., 38, 16–27, https://doi.org/10.1007/s00267-005-0113-6, 2006.
Fearnside, P. M.: Brazil's Belo Monte Dam: lessons of an Amazonian resource struggle, DIE ERDE–Journal of the Geographical Society of Berlin, 148, 167–184, https://doi.org/10.12854/erde-148-46, 2017.
Frankignoulle, M., Abril, G., Borges, A., Bourge, I., Canon, C., Delille, B. E. L., and Théare, J.: Carbon Dioxide Emission from European Estuaries, Science, 282, 434–436, https://doi.org/10.1126/science.282.5388.434, 1998.
Gagne-Maynard, W. C., Ward, N. D., Keil, R. G., Sawakuchi, H. O., Da Cunha, A. C., Neu, V., Brito, D. C., Less, D. F. S., Diniz, J. E. M., Valerio, A. M., Kampel, M., Krusche, A. V., and Richey, J. E.: Evaluation of primary production in the lower Amazon River based on a dissolved oxygen stable isotopic mass balance, Front. Mar. Sci., 4, 26, https://doi.org/10.3389/fmars.2017.00026, 2017.
Guérin, F., Abril, G., Richard, S., Burban, B., Reynouard, C., Seyler, P., and Delmas, R.: Methane and carbon dioxide emissions from tropical reservoirs: Significance of downstream rivers, Geophys. Res. Lett., 33, 1–6, https://doi.org/10.1029/2006GL027929, 2006.
Guérin, F., Abril, G., de Junet, A., and Bonnet, M. P.: Anaerobic decomposition of tropical soils and plant material: Implication for the CO2 and CH4 budget of the Petit Saut Reservoir, Appl. Geochem., 23, 2272–2283, https://doi.org/10.1016/j.apgeochem.2008.04.001, 2008.
Hesslein, R. H., Rudd, J. W. M., Kelly, C., Ramlal, P., and Hallard, K.: Carbon dioxide partial pressure in the surface waters of lakes in Northwestern, Ontario and the MacKenzie Delta region, Canada, in: Second International Symposium on Gas Transfer at Water Surfaces, August 1990, Vicksburg, USA, 413–431, 1991.
Inmet: Instituto Nacional De Meteorologia, available at: http://www.inmet.gov.br/projetos/rede/pesquisa/, last access in: 12 July 2017.
Jähne, B. J., Münnich, K. O. M., Bösinger, R., Dutzi, A., Huber, W., and Libner, P.: On the Parameters Influencing Air-Water Gas Exchange, J. Geophys. Res., 92, 1937–1949, https://doi.org/10.1029/JC092iC02p01937, 1987.
Kemenes, A., Forsberg, B. R., and Melack, J. M.: CO2 emissions from a tropical hydroelectric reservoir (Balbina, Brazil), J. Geophys. Res.-Biogeo., 116, 1–11, https://doi.org/10.1029/2010JG001465, 2011.
Kemenes, A., Forsberg, B. R., and Melack, J. M.: Downstream emissions of CH4 and CO2 from hydroelectric reservoirs (Tucurui, Samuel, and Curua-Una) in the Amazon basin, Inland Waters, 6, 295–302, https://doi.org/10.1080/IW-6.3.980, 2016.
Landchützer, P., Gruber, N., Bakker, D. C. E., and Schuster, U.: Recent variability of glolbal ocean carbon sink, Global Biogeochem. Cy., 28, 927–949, https://doi.org/10.1002/2014GB004853, 2014.
Latrubesse, E. M., Stevaux, J. C., and Sinha, R.: Tropical rivers, Geomorphology, 70, 187–206, https://doi.org/10.1016/j.geomorph.2005.02.005, 2005.
Li, S., Wang, F., Luo W., Wang, Y., and Deng, B.: Carbon dioxide emissions from the Three Gorges Reservoir, China, Acta Geochim., 36, 645–657, https://doi.org/10.1007/s11631-017-0154-6, 2017.
Lima, I. B. T., Victoria, R. L., Novo, E. M. L. M., Feigl, B. J., Ballester, B. J., and Ometto, J. P.: Methane, carbon dioxide and nitrous oxide emissions from two Amazonian Reservoirs during high water table, Verhandlungen, 28, 438–442, https://doi.org/10.1080/03680770.2001.11902620, 2002.
Miller, W. L. and Zepp, R. G.: Photochemical production of dissolved inorganic carbon from terrestrial organic matter: Significance to the oceanic organic carbon cycle, Geophys. Res. Lett., 22, 417–420, https://doi.org/10.1029/94GL03344, 1995.
MME: Ministério de Minas e Energia, available at: http://www.mme.gov.br/web/guest/destaques-do-setor-de-energia/belo-monte (last access: 16 June 2019), 2011.
Norte Energia: Supressão vegetal – situação de execução, Technical Note, Superintendência dos Meios Físico e Biótico, Diretoria Socioambiental, Brasília – DF, 24 pp., 2015.
Oksanen, J., Blanchet, F. G., Friendly, M., Kindt, R., Legendre, P., McGlinn, D., Michin, P. R., O'Hara, R. B., Simpson, G. L., Solymos, P., Stevens, M. H. H., Szoecs, E., and Wagner, H.: vegan: Community Ecology Package, R package version 2.4-3, CRAN network, available at: https://CRAN.R-project.org/package=vegan, last access: 17 March 2017.
Paranaíba, J. R., Barros, N., Mendonça, R., Linkhorst, A., Isidorova, A., Roland, F., Almeida, R. M., and Sobek, S.: Spatially resolved measurements of CO2 and CH4 concentration and gas-exchange velocity highly influence carbon-emission estimates of reservoirs, Environ. Sci. Technol., 52, 607–615, https://doi.org/10.1021/acs.est.7b05138, 2017.
Rasera, M. de F. F. L., Krusche, A. V., Richey, J. E., Ballester, M. V. R., and Victória, R. L.: Spatial and temporal variability of pCO2 and CO2 efflux in seven Amazonian Rivers, Biogeochemistry, 116, 241–259, https://doi.org/10.1007/s10533-013-9854-0, 2013.
Raymond, P. A. and Cole, J. J.: Gas Exchange in Rivers and Estuaries: Choosing a Gas Transfer Velocity, Estuaries, 24, 312, https://doi.org/10.2307/1352954, 2001.
Raymond, P. A. and Saiers, J. E.: Event controlled DOC export from forested watersheds, Biogeochemistry, 100, 197–209, https://doi.org/10.1007/s10533-010-9416-7, 2010.
Raymond, P. A., Hartmann, J., Lauerwald, R., Sobek, S., McDonald, C., Hoover, M., Butman, D., Striegl, R., Mayorga, E., Humborg, C., Kortelainen, P., Dürr, H., Meybeck, M., Ciais, P., and Guth, P.: Global carbon dioxide emissions from inland waters, Nature, 503, 355–359, https://doi.org/10.1038/nature12760, 2013.
R Core Team: R: A Language and Environment for Statistical Computing. R Foundation for Statistical Computing, Vienna, Austria, 2016.
Richey, J. E., Melack, J. M., Aufdenkampe, A. K., Ballester, V. M., and Hess, L. L.: Outgassing from Amazonian rivers and wetlands as a large tropical source of atmospheric CO2, Nature, 416, 617–620, https://doi.org/10.1038/416617a, 2002.
Roland, F., Vidal, L. O., Pacheco, F. S., Barros, N. O., Assireu, A., Ometto, J. P. H. B., Cimbleris, A. C. P., and Cole, J. J.: Variability of carbon dioxide flux from tropical (Cerrado) hydroelectric reservoirs, Aquat. Sci., 72, 283–293, https://doi.org/10.1007/s00027-010-0140-0, 2010.
Rudorff, C. M., Melack, J. M., MacIntyre, S., Barbosa, C. C., and Novo, E. M.: Seasonal and spatial variability of CO2 emission from a large floodplain lake in the lower Amazon, J. Geophys. Res., 116, G04007, https://doi.org/10.1029/2011JG001699, 2011.
Sawakuchi, A. O., Hartmann, G. A., Sawakuchi, H. O., Pupim, F. N., Bertassoli, D. J., Parra, M., Antinao, J. L., Sousa, L. M., Sabaj Pérez, M. H., Oliveira, P. E., Santos, R. A., Savian, J. F., Grohmann, C. H., Medeiros, V. B., McGlue, M. M., Bicudo, D. C., and Faustino, S. B.: The Volta Grande do Xingu: reconstruction of past environments and forecasting of future scenarios of a unique Amazonian fluvial landscape, Sci. Dril., 20, 21–32, https://doi.org/10.5194/sd-20-21-2015, 2015.
Sawakuchi, H. O., Neu, V., Ward, N. D., Barros, M. de L. C., Valerio, A. M., Gagne-Maynard, W., Cunha, A. C., Less, D. F. S., Diniz, J. E. M., Brito, D. C., Krusche, A. V., and Richey, J. E.: Carbon Dioxide Emissions along the Lower Amazon River, Front. Mar. Sci., 4, 1–12, https://doi.org/10.3389/fmars.2017.00076, 2017.
Sioli, H.: The Amazon and its main affluents: Hidrology, morpholy of the river courses and river types, in: The Amazon: limnology and landscape ecology of a mighty tropical river and its basin, edited by: Sioli, H. and Dumont, H. J., Dr W. Junk Publishers, the Netherlands, 127–165, https://doi.org/10.1007/978-94-009-6542-3, 1984.
St. Louis, V. L., Kelly, C. A., Duchemin, E., Rudd, J. W. M., and Rosenberg D. M.: Reservoir surfaces as sources of greenhouse gases to the atmosphere: a global estimate, BioScience, 5, 766–775, https://doi.org/10.1641/0006-3568(2000)050[0766:RSASOG]2.0.CO;2, 2000.
Teodoru, C. R., Prairie, Y. T., and Del Giorgio, P. A.: Spatial Heterogeneity of Surface CO2 Fluxes in a Newly Created Eastmain-1 Reservoir in Northern Quebec, Canada, Ecosystems, 14, 28–46, https://doi.org/10.1007/s10021-010-9393-7, 2011.
Vachon, D., Prairie, Y. T., and Smith, R.: The ecosystem size and shape dependence of gas transfer velocity versus wind speed relationships in lakes, Can. J. Fish. Aquat. Sci., 70, 1757–1764, https://doi.org/10.1139/cjfas-2013-0241, 2013.
Verpoorter, C., Kutser, T., Seekell, D. A., and Tranvik, L. J.: A global inventory of lakes based on high-resolution satellite imagery, Geophys. Res. Lett., 41, 6396–6402, https://doi.org/10.1002/2014GL060641, 2014.
Wang, F., Cao, M., Wang, B., Fu, J., Luo, W., and Ma, J. Seasonal variation of CO2 diffusion flux from a large subtropical reservoir in East China, Atmos. Environ., 103, 129–137, https://doi.org/10.1016/j.atmosenv.2014.12.042, 2015.
Wanninkhof, R. H.: Relationship between wind speed and gas exchange, J. Geophys. Res., 97, 7373–7382, https://doi.org/10.1029/92JC00188, 1992.
Wanninkhof, R., Asher, W. E., Ho, D. T., Sweeney, C., and McGillis, W. R.: Advances in Quantifying Air-Sea Gas Exchange and Environmental Forcing, Annu. Rev. Mar. Sci., 1, 213–244, https://doi.org/10.1146/annurev.marine.010908.163742, 2009.
Ward, N. D., Keil, R. G., Medeiros, P. M., Brito, D. C., Cunha, A. C., Dittmar, T., Yager, P. L., Krusche, A. V., and Richey, J. E.: Degradation of terrestrially derived macromolecules in the Amazon River, Nat. Geosci., 6, 530–533, https://doi.org/10.1038/ngeo1817, 2013.
Ward, N. D., Bianchi, T. S., Sawakuchi, H. O., Gagne-Maynard, W., Cunha, A. C., Brito, D. C., Neu, V., Matos Valerio, A., Silva, R., Krusche, A. V., and Richey, J. E.: The reactivity of plant-derived organic matter and the potential importance of priming effects along the lower Amazon River. J. Geophys. Res.-Biogeo., 121, 1522–1539, https://doi.org/10.1002/2016JG003342, 2016.
Ward, N. D., Bianchi, T. S., Medeiros, P. M., Seidel, M., Richey, J. E., Keil, R. G., and Sawakuchi, H. O.: Where carbon goes when water flows: carbon cycling across the aquatic continuum, Front. Mar. Sci., 4, 7, https://doi.org/10.3389/fmars.2017.00007, 2017.
Wiesenburg, D. A. and Guinasso Jr., N. L.: Equilibrium solubilities of methane, carbon monoxide, and hydrogen in water and sea water, J. Chem. Eng. Data, 24, 356–360, https://doi.org/10.1021/je60083a006, 1979.
Winemiller, K. O., McIntyre, P. B., Castello, L., Fluet-Chouinard, E., Giarrizzo, T., Nam, S., Baird, I. G., Darwall, W., Lujan, N. K., Harrison, I., Stiassny, M. L. J., Silvano, R. A. M., Fitzgerald, D. B., Pelicice, F. M., Agostinho, A. A., Gomes, L. C., Albert, J. S., Baran, E., Petrere, M., Zarfl, C., Mulligan, M., Sullivan, J. P., Arantes, C. C., Sousa, L. M., Koning, A. A., Hoeinghaus, D. J., Sabaj, M., Lundberg, J. G., Armbruster, J., Thieme, M. L., Petry, P., Zuanon, J., Vilara, G. T., Snoeks, J., Ou, C., Rainboth, W., Pavanelli, C. S., Akama, A., van Soesbergen, A., and Saenz, L.: Balancing hydropower and biodiversity in the Amazon, Congo, and Mekong, Science, 351, 128–129, https://doi.org/10.1126/science.aac7082, 2016.
Xu, Y., Zhang, M., Wang, L., Kong, L., and Cai, Q.: Changes in water types under the regulated mode of water level in Three Gorges Reservoir, China, Quaternary Int., 244, 272–279, https://doi.org/10.1016/j.quaint.2011.01.019, 2011.
Zar, J. H.: Biostatistical Analysis, 5th Edn., Pearson, New Jersey, 931 pp., 2010.