the Creative Commons Attribution 4.0 License.
the Creative Commons Attribution 4.0 License.
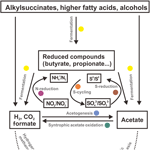
Identifying the core bacterial microbiome of hydrocarbon degradation and a shift of dominant methanogenesis pathways in the oil and aqueous phases of petroleum reservoirs of different temperatures from China
Zhichao Zhou
Bo Liang
Li-Ying Wang
Jin-Feng Liu
Hojae Shim
Ji-Dong Gu
Microorganisms in petroleum reservoirs play significant roles in hydrocarbon degradation, and through the terminal electron-accepting process of methanogenesis, they also contribute to microbially enhanced oil recovery (MEOR) worldwide, with great economic and environmental benefits. Here, a molecular investigation, using the 16S rRNA and mcrA gene profiles based on MiSeq sequencing and clone library construction methods, was conducted on oil and water (aqueous) phases of samples of high (82–88 ∘C), moderate (45–63 ∘C), and low temperatures (21–32 ∘C) from seven petroleum reservoirs in China. A core bacterial microbiome with a small proportion of shared operational taxonomic unit (OTU) values, but a high proportion of sequences among all reservoirs was discovered, including aerobic degraders, sulfate- and nitrate-reducing bacteria, fermentative bacteria, and sulfur-oxidizing bacteria distributed mainly in Proteobacteria, Bacteroidetes, Deferribacteres, Deinococcus–Thermus, Firmicutes, Spirochaetes, and Thermotogae. Their prevalence in the previously reported petroleum reservoirs and successive enrichment cultures suggests their common roles and functions involved in aliphatic and aromatic hydrocarbon degradation. The methanogenic process generally shifts from the dominant hydrogenotrophic pathway in the aqueous phase to the acetoclastic pathway in the oil phase in high-temperature reservoirs, but the opposite was true for low-temperature reservoirs. No difference was detected between the two phases in moderate temperature reservoirs. Physicochemical factors, including pH; temperature; phase conditions; and nitrate, Mn2+, and Mg2+ concentrations were the main factors correlated to the microbial compositional and functional profiles significantly. Linear discriminant analysis (LDA) effect size (LEfSe) analysis shows distribution differences of microbial groups towards pH, temperature, and the oil and aqueous phases. Using the software Tax4Fun for functional profiling indicated functional metabolism differences between the two phases, including amino acids, hydrocarbons in the oil phase, and carbohydrates in the aqueous phase.
- Article
(3254 KB) - Full-text XML
-
Supplement
(2075 KB) - BibTeX
- EndNote
Microbially enhanced oil recovery (MEOR) is one of the most feasible and profitable technologies for extracting residual oil from low-productivity reservoirs, by utilizing microorganisms as the major functional players for hydrocarbon transformation via fermentation and methanogenesis in MEOR applications (Magot et al., 2000; Mbadinga et al., 2011; Mesle et al., 2013). Implementation of MEOR could also attenuate side effects by injection of nitrate into oil wells to stimulate the propagation of nitrate-reducing bacteria (NRB) and inhibit the growth of sulfate-reducing bacteria (SRB), subsequently reducing the negative effects by sulfidogenic activity on corrosion and oil quality deterioration (Gao et al., 2013). Currently, investigations of compositional and functional profiles of petroleum reservoir microbiomes have attracted attention from both scientists and engineers because their information will improve our understanding of microbial diversity and function in oil reservoir systems and implement MEOR through manipulating microbial community composition and activities (Magot et al., 2000; Mesle et al., 2013; Youssef et al., 2009).
Petroleum reservoirs are often characterized as high-temperature and high-pressure subterranean ecosystems, but low-temperature reservoirs also exist around the world (Grabowski et al., 2005; Li et al., 2013; Voordouw et al., 1996; Li et al., 2014). The physicochemical and geographic differences among petroleum reservoirs, together with exogenous injection of microorganisms and nutrients during MEOR operation, could affect the composition and functional groups of the indigenous microbial community. Many previously discovered bacterial and archaeal groups have important functional roles, e.g., sulfate-reducing, nitrate- or nitrite-reducing, and fermentative bacteria; thermophilic archaeal hydrocarbon degraders; and methanogens, as well as exogenous aerobic hydrocarbon degraders, in the microbial community (Gao et al., 2015a, b; Lenchi et al., 2013; Li et al., 2012a; Orphan et al., 2003; Wang et al., 2012; Grabowski et al., 2005; Pham et al., 2009). The common and specific roles and functions of these microorganisms in petroleum reservoirs are of great value and should be comprehensively examined.
Methanogens cooperate with hydrocarbon degraders and fermentative bacteria synergistically to make the overall degradation processes thermodynamically favorable under largely anaerobic conditions (Mbadinga et al., 2011). Fermentative products from hydrocarbon degradation provide the essential growth substrates for methanogens with two major pathways, namely hydrogenotrophic and acetoclastic methanogenesis (Magot et al., 2000). In many cases the syntrophic acetate oxidization pathway coupling with hydrogenotrophic methanogenesis is prevalent in both in situ and enrichment incubation ex situ (Mesle et al., 2013; Liang et al., 2015; Wang et al., 2012; Lee et al., 2015; Mayumi et al., 2011). However, very little is known of the factors that shape the methanogenic pathways and the structure of methanogenic communities.
Petroleum reservoir is a complex system consisting of porous sandstones with oil, water, and air. Microorganisms attached to the oil phase of petroleum fluids are largely neglected in majority of the previous investigations due to technical difficulties in DNA extraction and sequencing (Kryachko et al., 2012). Oil-attached microorganisms influence the oil–water interface properties via the production of biosurfactants and metabolites or the formation of adsorbed colloidal particles to enhance oil recovery performance (Kobayashi et al., 2012; Kryachko et al., 2012; Wang et al., 2014). The distribution, function, and contribution of these microorganisms to MEOR success could be very different from those of the aqueous phase (Kobayashi et al., 2012). Oil-degrading microorganisms, including thermophilic hydrocarbon-degrading archaea and methanogens, play different roles in the MEOR process (Mbadinga et al., 2011). Their compositional patterns and functional profiles in terms of temperature and the oil and aqueous phases are of great value for the understanding of the mechanism of MEOR. Knowledge of the major microbial drivers, their potential functions, distribution characteristics, and changing patterns towards environmental parameters should be one of the research directions taken for a better understanding of the MEOR process. In this study, the research objectives were to reveal the compositional and functional differences of petroleum-reservoir-inhabiting microorganisms under different temperatures (high: 82–88 ∘C; moderate: 45–63 ∘C; and low: 21–32 ∘C), the methanogenesis pathways in the oil and aqueous phases of these samples, and the influence of physicochemical factors on microbial community composition.
2.1 Characterization of geographic properties of sampling reservoirs
Petroleum production fluid samples were collected from seven oilfields across China, covering oil wells of different geographical locations and temperatures. The reservoirs and crude oil properties, together with the the aqueous-phase chemical characteristics of this study, are listed in Table 1. Detailed reservoir properties are described in the Supplement.
2.2 Sample collection, ion concentration measuring, and DNA extraction
Each sample, containing a mixture of crude oil and water, was collected on site in a sterilized container after flushing each wellhead for at least 3–5 min. The containers were screw capped to avoid air invasion and transported back to the nearby laboratory immediately for further processing. The oil and water mixture was gently heated to 50 ∘C to make it semifluid and then separated into oil and aqueous phases in a separatory funnel. Heating was operated as quickly as possible according to the solidification degree of individual petroleum fluid samples, in order to reduce the lytic effect of microbial cells within them. Ion concentrations of the aqueous-phase samples were measured using Dionex 600 ion chromatography (Triad Scientific, Inc., Manasquan, NJ, USA) following the manufacturer's instructions.
To obtain aqueous-phase DNA, the aqueous phase of each sample after oil and water separation was first filtered through a 0.22 µm pore size polycarbonate membrane filter and a portion of the membrane filter was used to extract DNA with a AxyPrep™ Bacterial Genomic DNA Miniprep Kit according to the manufacturer's instructions (Axygen Biosciences, USA). For the oil phase, three volumes of iso-octane (2, 2, 4-trimethylpentane) were used to dissolve the crude oil and then centrifuged at 5000 rpm for 30 min to concentrate non-dissolved particulates and microbial cells at least three times to obtain enough materials. Repetitive DNA extractions were then conducted on these materials and combined to meet the quantity requirement for the downstream quality control. Finally, all DNA samples from the aqueous and oil phases were measured by the Nanodrop Model 2000 for concentration and checked for DNA integrity by electrophoresis.
2.3 Clone library construction and analysis
The methyl-coenzyme-M reductase-encoding gene (mcrA) primer pair, ME3MF and ME3MF-e–ME2r′ (Narihiro and Sekiguchi, 2011), and the 16S rRNA gene primer pair targeting methanogens, MetA86f–ARC915r (Wright and Pimm, 2003; Narihiro and Sekiguchi, 2011; Yu et al., 2008), were used to generate gene clone libraries for each sample (aqueous and oil phases separately) according to the method established previously (Zhou et al., 2015) (Table 2).
A FunGene pipeline chimera check was applied to check mcrA gene sequences using the UCHIME de novo mode (Edgar et al., 2011). USEARCH software was used to check chimeras of methanogenic 16S rRNA gene sequences using a QIIME-compatible SILVA 119 release SSURef database (“rdp_gold fasta”) file as a reference. Then, for mcrA gene sequences, a de novo operational taxonomic unit (OTU) picking method was applied by QIIME at a cutoff value of 0.05 (Caporaso et al., 2010). Representative OTU sequences were aligned and inserted into the mcrA gene ARB project database through the maximum parsimony method without changing the initial tree topology (Angel et al., 2012; Ludwig et al., 2004). The phylogenetic affiliation was assigned, and taxonomic composition results were processed by QIIME accordingly (Fig. S1 in the Supplement). For methanogenic 16S rRNA gene sequence clustering and diversity analysis, the same method was applied via QIIME as described in the followings.
2.4 MiSeq sequencing and QIIME-based analysis
The prokaryotic universal primer pair 515F–909R (Caporaso et al., 2012; Wang and Qian, 2009) and archaeal universal primer pair Arch347F–Arch806R (Takai and Horikoshi, 2000) were used to amplify samples of this study (both with barcodes attached to the forward primers, Table 2). Two independent polymerase chain reaction (PCR) reactions were conducted and then combined to yield enough PCR products to compromise variations between different batches. Then, pooled PCR products of each sample with approximately 100 or 200 ng DNA were subjected to one MiSeq run. Sequencing samples were prepared using TruSeq DNA Kit according to the manufacturer's instruction. The library was uploaded to an Illumina MiSeq platform for sequencing with reagent kit v2 (2× 250 bp) or v3 (2× 300 bp), as described in the manufacturer's manual.
After merging paired-end reads from raw sequencing data with FLASH-1.2.8, the fastx toolkit was applied to split merged reads from one run into individual samples according to the primer barcodes (Magoc and Salzberg, 2011). Then, all sequences were split into each library with the name of each sample attached according to the barcode map using the QIIME command “split_libraries” (Caporaso et al., 2010). The criterion for filtering out underqualified sequences was “-s 15 -k -a 6 -r -l 150 -b 12 -M 5 -e 0”. Chimera checking was conducted by USEARCH software using the QIIME-compatible SILVA 119 release SSURef database (“rdp_gold” fasta) file as the reference (Edgar, 2010). Clustering; picking OTU; taxonomy assignment, aligning, filtering alignments, and phylogenetic tree construction; taxonomic composition summarizing; and alpha and beta diversity analyses were conducted step-by-step by the QIIME pipeline with QIIME-compatible SILVA 119 SSURef database as the reference (Caporaso et al., 2010). In clustering, the “pick_open_reference_otus.py” command was used to conduct OTU dividing, and the BLAST method was used to assign taxonomy to input sequences. This subsampled open-reference OTU picking method was the performed optimized and optimal strategy suggested by the developers (Rideout et al., 2014). After OTU table “biom” files were generated, in order to get the bacterial community composition information from prokaryotic 16S rRNA gene primer amplified libraries, the “filter_taxa_from_otu_table.py” command was introduced to only retain bacterial OTUs in the “biom” file. Similarly, exclusive archaeal and methanogenic OTU table files could also be processed from archaeal 16S rRNA gene primer amplified libraries. Summary information of each sample OTU abundance could be calculated by the “biom summarize-table” command, and then the lowest number among all samples was chosen as the subsampling size to make each library acquire an even size using the “multiple_rarefaction_even_depth.py” command. The taxonomic compositional table was drawn according to the subsampled biom file. Since there was no lane mask file available in this SILVA-compatible 119 release SSURef database, an alignment filtering method was performed independently with an entropy threshold of 0.1 and gap filter threshold of 0.9 after obtaining aligned sequences via the PyNAST method. Diversity parameters of each library could be generated by alpha diversity calling, with the rarefaction curve; Good's coverage value; and Shannon, Chao1, Simpson, and phylogenetic diversity (PD) whole tree indices calculated. Beta diversity, which delineates the dissimilarity relationship among samples, was generated and visualized through unweighted and weighted UniFrac matrix and non-phylogenetic Bray–Curtis matrix method. The pairwise-shared OTU numbers were calculated from “biom” by the command “shared_phylotypes.py”. Core microbiome (shared OTU table in a specific sample category) was identified by the “compute_core_microbiome.py” command.
2.5 Diversity and statistical analysis
The statistical significance of the community composition of samples among different categories was valued by the anosim and adonis methods, implemented in “compare_categories” command in QIIME. A Mantel test was used to compare the distance matrix of physicochemical parameters and UniFrac and Bray–Cutis distance matrix from beta diversity analysis by QIIME. For aqueous-phase samples, both of the in situ physicochemical parameters and ion concentrations were used in the analysis, while only in situ physicochemical parameters were included for oil-phase samples. The compositional bar chart and bubble chart were modified and illustrated from taxonomic summary results. Tax4Fun was used to predict the functional capabilities based on abundance profiles of microbial 16S rRNA gene datasets (Asshauer et al., 2015). Linear discriminant analysis (LDA) effect size (LEfSe) analysis was applied to illustrate the biomarker species with high statistical significance in different sample categories and the functional profiles statistically distributed in different sample categories (Segata et al., 2011).
2.6 Quantitative PCR on mcrA gene abundance
The quantitative PCR measurement was conducted using the iTaq™ Universal SYBR® Green Supermix Kit (BIO-RAD). The qPCR mixture contained in 15 µL : 7.5 µL of Supermix, 16 µg of bovine serum albumin (BSA) (Roche), and 1 µM final concentration of the primer pair, i.e., ME3MF and ME3MF-e (250 : 1) and ME2r′. Annealing temperature was set to be the same as the clone library PCR setting, and the rest thermocycling settings were according to the manufacturer's instructions. A single randomly picked pMD18-T plasmid with the mcrA gene inserted was used to make the standard curve. The DNA template concentration was adjusted to 0–40 ng µL−1. Results that deviated significantly from values in the replicate groups were omitted, and undetermined results (under the detection limit) were also deleted. The property of the final adjusted standard curve is r2=0.995 and Eff% = 83.32.
2.7 Sequencing result deposition
For clone library sequences, KT314862–KT315353 were assigned to methanogenic 16S rRNA gene sequences and KT314340–KT314835 were assigned to mcrA gene sequences. For high-throughput sequencing data based on Illumina MiSeq platform, PRJEB9855 was assigned to prokaryotic 16S rRNA gene sequencing data and PRJEB10996 was assigned to archaeal 16S rRNA gene sequencing data.
3.1 Common OTU among different categories and core bacterial microbiome
Community composition results showed that 21 bacterial phyla were obtained with an average abundance of more than 0.1 % (Fig. 1a) and that the three major archaeal phyla were Thaumarchaeota, Euryarchaeota, and Crenarchaeota (Fig. 1b). Pairwise-shared OTU numbers of all samples indicated that, irrespective of combinations between aqueous- and oil-phase samples, the average numbers of shared bacterial OTUs ranged from 199.9 to 292.4, accounting for 26.6 %–36.2 % of the total OTU numbers within individual samples; average numbers of shared archaeal OTUs ranged from 1.8 to 11.9, accounting for 8.5 %–23.4 % of total OTU numbers within individual samples (Table S4 in the Supplement). Core bacterial OTU numbers among aqueous, oil, and all samples were 73, 57, and 46, which accounted for 7.1 %–10.1 %, 5.9 %–10.3 %, and 4.5 %–8.3 % of OTU numbers in individual samples. The core archaeal OTU number among aqueous samples was only 3, accounting for 3.9 %–8.1 % of OTU numbers in individual samples, and no archaeal OTU was shared among oil-phase samples.
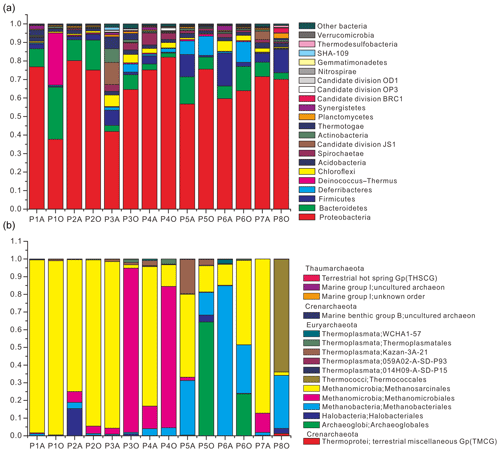
Figure 1Relative abundance of bacteria (a) and archaea (b) from 14 aqueous- and oil-phase samples. Bacterial community was taxonomically assigned at the level of phylum. Those phyla with an average abundance of all samples below 0.1 % were combined into the “other bacteria” category. The archaeal community was taxonomically assigned at the level of class.
However, by investigating taxonomic profiles of core bacterial OTUs, the shared OTUs were 49, 41, and 34 genera in aqueous, oil, and all samples, corresponding to 65.5 %, 59.9 %, and 58.8 % of average sequences in the total bacterial community, respectively (Tables S5 and S6). Most of the core bacterial OTUs belonged to the most abundant 36 genera, of which the numbers of shared genera among aqueous, oil, and all samples were 28, 23, and 23, respectively (Fig. 2, and Tables S5 and S6).
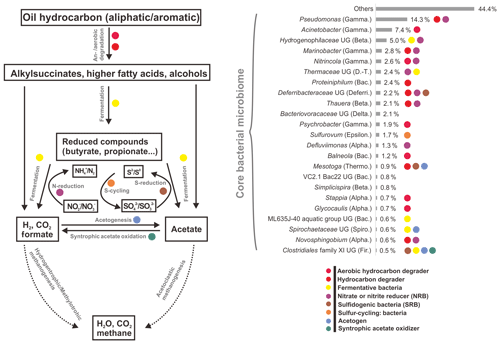
Figure 2Average abundances and functional roles of core bacterial microbiome in all petroleum samples (including aqueous- and oil-phase samples). Core microbial OTUs (23 OTUs) were affiliated to the most abundant genera. The functional roles were assigned according to reference publications (Supplement). Degradation pathway was modified from Mbadinga et al. (2011). Abbreviations: Alpha., Beta., Gamma., Delta., Epsilon. (Alpha-, Beta-, Gamma-, Delta-, Epsilonproteobacteria), D.-T. (Deinococcus–Thermus), Bac. (Bacteroidetes), Deferri. (Deferribacteres), Thermo. (Thermotogae), Spiro. (Spirochaetes), and Fir. (Firmicutes). UG stands for uncultured genus.
There was no significant difference of shared bacterial OTU numbers within and between aqueous- and oil-phase samples, suggesting a core microbiome was shared among all components. The core OTUs covered around two-thirds of the total bacterial sequences, even though the percentages of core or total OTU number for individual samples were 4.5 % to 10.3 %. The core microbiome shared among all petroleum reservoirs could be the key participants mediating critical microbial processes, such as activation, degradation, fermentation, oil emulsification, and methane generation (Yamane et al., 2008; Wang et al., 2014; Pham et al., 2009; Orphan et al., 2000; Magot et al., 2000). This spectrum of core microbiome shares common functional roles in facilitating MEOR and is modified by the in situ physicochemical conditions of different reservoirs (Fig. 2 and Table S5). It is important to connect the major microbial players, including their community compositions and specific functional capacities, to the interpretation of MEOR processes in the petroleum reservoirs. Meanwhile, the core microbiome serves as a good basis for simplifying microbial participants and, primarily, their roles in the petroleum reservoirs and is useful for modeling and monitoring the MEOR processes of petroleum reservoirs from different locations. Moreover, substantial portions of the aerobic bacteria being discovered in the core bacterial microbiome across different reservoirs imply that exogenous bacteria introduced into subsurface reservoirs by water flushing can be also represented in the core composition and it plays important roles in enhancing oil recovery (Gao et al., 2015b; Youssef et al., 2009).
Bacterial community distribution in the aqueous phase showed correlation with temperature via the Mantel test using unweighted UniFrac matrix method (Table S8). Additionally, temperature also significantly affected the dissimilar distance matrix of PCoA coordinates of the bacterial community via both adonis and anosim statistical analyses (Tables S3 and S7). However, temperature changed the proportions of the taxa of the core microbiome significantly but did not change their presence or absence (Tables S3, S7 and S8). Consequently, the common genera discovered from this study show that, despite geographic and physicochemical differences, there is a core microbiome with small OTU numbers but large sequence proportions in these petroleum reservoirs across China that are possibly responsible for mediating hydrocarbon degradation processes. Prevalent core genera discovered in this study also appear to be the most abundant components, as previously reported in petroleum reservoirs within and beyond China (Gao et al., 2015a, b; Li et al., 2012a; Orphan et al., 2003, 2000; Tang et al., 2012; Yamane et al., 2008; Dahle et al., 2008; Li et al., 2015, 2014; Liang et al., 2015) and enrichment cultures from petroleum reservoirs worldwide (Gray et al., 2011; Li et al., 2012b; Liang et al., 2015; Wang et al., 2011).
3.2 Shift of major methanogenesis pathways between the oil and aqueous phases
The methanogenic process generally shifts from the dominant hydrogenotrophic pathway in the aqueous phase to the acetoclastic pathway in the oil phase of high-temperature reservoirs. The opposite is true for low-temperature samples, but no difference was detected between the two phases in moderate temperature reservoirs (Fig. 3). The different patterns of shifting of the major methanogenesis mechanisms in the aqueous and oil phases were evident in different temperature petroleum reservoirs of this study. Quantitative measurements of mcrA gene in both the aqueous and oil phases of all samples are summarized in the Supplement (Fig. S1).
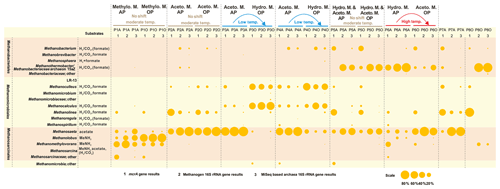
Figure 3Bubble chart of the proportional composition of methanogens based on MiSeq sequencing results of archaeal 16S rRNA genes and clone sequencing results of the mcrA gene and methanogenic 16S rRNA gene. The major substrate utilization properties originated in Liu and Whitman (2008). MeNH2 is methylamine and substrates in parentheses refer to being utilized by some but not all species. Methanothermobacter thermautotrophicus strain Delta H is the phylogenetically closest cultured clone to Methanobacteriaceae archaeon 15aZ. Thus, the combination of Methanothermobacter and the Methanobacteriaceae archaeon 15aZ was used. Methanogenesis shift was based on the transition of major methanogenesis pathway. Abbreviations: Aceto. M., Hydro. M., and Methylo. M. are Acetoclastic methanogenesis, Hydrogenotrophic methanogenesis, and Methylotrophic methanogenesis, respectively.
The underlying methanogenesis mechanism could change substantially in reservoirs with distinctive geochemical properties (Magot et al., 2000). The dominance of methylotrophic methanogenesis is rarely observed in petroleum reservoirs. It is claimed that there is a very low possibility that methyl-compounds could be generated during the degradation of kerogen (Mesle et al., 2013). However, the dominance of obligate methylotrophic Methanolobus was found in all water, rock and coal samples in a coal bed methane reservoir within the eastern Ordos Basin of China (Guo et al., 2012). It could be deduced that the prevalence of methylotrophic methanogenesis under certain conditions is directly fueled by the existence and availability of methyl-containing substances. Since the relative abundances of bacteria in petroleum reservoir samples are always higher than those of methanogenic community, the methanogenesis process might not be the dominant process among all microbial processes. For instance, in P1 and P5 aqueous samples, sulfate concentrations are considerably high (Table 1) and geochemical conditions are more favorable for sulfate reduction than methanogenesis. A large proportion of Firmicutes and Deferribacteres in P5A were potentially responsible for the activities of sulfate reduction, according to their relative abundance (Fig. 1) and functional capacities (Fig. 2). It is suggested the methylated compounds could be produced by the degradation of glycine betaine (an osmoprotective agent) that are accumulated or generated by halophiles in saline petroleum (Ollivier and Alazard, 2010). Subsequently, it fuels the growth of methylotrophic methanogens in certain petroleum environments. Although no molecular or chemical evidence was reported for this process in petroleum environments, a pioneer study on hydraulic fracturing in shales has detected glycine betaine as the major known osmoprotectant in the produced fluids, and the combined metagenomic and metabolite findings suggest the similar glycine betaine utilization pathways in fractured shales (Daly et al., 2016).
Numerous studies based on in situ or in enrichment incubation microcosms on the aqueous phase of reservoir fluids indicate that syntrophic acetate oxidation associated with hydrogenotrophic methanogenesis is the major hydrocarbon degradation pathway (Wang et al., 2012; Mayumi et al., 2011; Lee et al., 2015; Mbadinga et al., 2012; Gray et al., 2011). The dominance of hydrogenotrophic methanogenesis in subsurface ecosystems could result from the external hydrogen originating from maturation of organic matter and/or mineral hydrolysis (Head et al., 2003) and the synergistic effect, in association with acetate oxidizers, whereby acetate was firstly oxidized to H2 and CO2 is then utilized by methanogenesis (Liu and Whitman, 2008). Additionally, a stable isotope labeling experiment on oil-degrading microcosms showed that despite the coexistence of acetoclastic methanogenesis and acetate syntrophic oxidization in the initial state, the latter process prevailed over the former one when introducing a low acetate concentration initially (Gray et al., 2011). The above evidence suggests that acetate syntrophic oxidization could exceed acetoclastic methanogenesis and contribute substrates H2 and CO2 to potentially favor hydrogenotrophic methanogenesis process.
It is still difficult to determine whether the temperature has directly or indirectly been involved in the alteration of the methanogenesis pathways. Reservoir fluid constituents may affect methanogenic degradation because crude oil and creosote inhibit acetoclastic methanogenesis (Warren et al., 2004) and volatile hydrocarbons (nC5–nC10) inhibit methanogenic degradation rates without changing the abundances of both hydrogenotrophic and acetoclastic methanogens (Sherry et al., 2014). Since most of the currently available community data are based on the microbial assemblages within injection or production water, a new understanding of the local microbiome distribution and changes should focus on the oil or hydrophobic fraction of the reservoir fluids (Kobayashi et al., 2012; Tang et al., 2012; Lenchi et al., 2013). Consequently, the alteration pattern of major methanogenesis in the aqueous and oil phases under different temperature conditions could be further delineated. A combination of methods, including synthesis and quantification of the degradation intermediate (Bian et al., 2015), stable isotope labeling on tracing substrate transformation (Gray et al., 2011), and molecular analysis of the metabolically active microorganisms can advance the information of anaerobic degradation and methanogenesis processes in reservoir systems.
3.3 Physicochemical influence and taxa and function profiles
Temperature is an important physical factor shaping the community structure of bacterial (anosim and adonis p<0.01) and methanogenic communities (anosim p<0.05 and adonis p<0.01) of the samples in this study (Fig. 4 and Table S7). Furthermore, a significant difference of taxa abundance among the three temperature categories for both bacterial and archaeal communities was evident by LEfSe analysis (Fig. 5). For the bacterial community, sample group (aqueous or oil phase from the same sample group), temperature (anosim and adonis p<0.01), and pH (adonis p<0.05) showed significant effects on separating samples into different categories. For the archaeal community, significant differences among sample categories were detected with sample group and temperature (both adonis p<0.05) and pH and phase (anosim and adonis p<0.05). For the methanogenic community, significant differences among sample categories were detected with sample group and temperature (sample group: anosim and adonis p<0.05; temperature: anosim p<0.05 and adonis p<0.01) (Table S7).
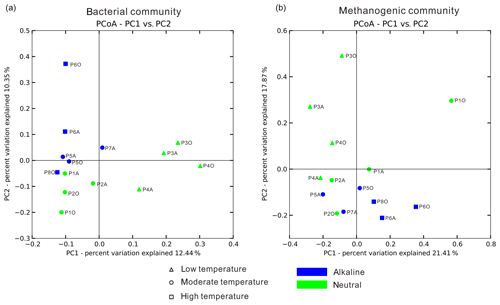
Figure 4Principal coordinate analysis plot figures based on unweighted UniFrac matrices. Bacterial (a) and methanogenic (b) communities of 14 samples were separately analyzed to delineate the dissimilarity distances between each sample based on phylogenetic classification. The sample dots were categorized in terms of temperature (dot shape) and pH condition (color).
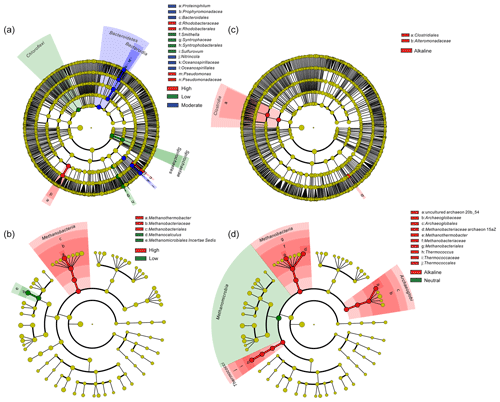
Figure 5Cladogram based on LEfSe analysis results of bacteria (a, c) and archaea (b, d) in terms of temperature (a, b) and pH (c, d) categories. The taxonomic trees were generated from phylum to genus (inside to outside) in the hierarchical structure. Biomarker taxonomic levels were labeled as the color that had a logarithmic LDA score of at least 3.5. Pre-sample normalization was used to format the relative abundance data. All-against-all strategy was used in the multi-class analysis step.
The nitrate concentration dissimilarity matrix was significantly associated with all unweighted and weighted UniFrac and Bray–Cutis matrices (all p<0.05) for aqueous bacterial community (Table S8). Meanwhile, methanogenic community in the oil phase was significantly affected by reservoir depth, temperature, pH, and water flooding operation years based on association analysis of the weighted UniFrac matrix but not the unweighted UniFrac matrix (all p<0.05) (Table S8), indicating that it was the abundance difference of certain taxa affecting the compositional pattern. More detailed relationships between physicochemical factors and bacterial, archaeal, and methanogenic communities are summarized in the Supplement (Tables S7 and S8).
The nitrate dissimilarity matrix was significantly correlated with dissimilarity matrices of all aqueous-phase bacterial communities using both unweighted and weighted UniFrac and Bray–Curtis matrices (all p<0.05) (Table S8). Nitrate is an important chemical used in injection water to inhibit corrosion and maintain crude oil quality (Gao et al., 2013). Nitrate stimulates the growth of nitrate-reducing bacteria and inhibits the growth of SRB (Nemati et al., 2001; Gao et al., 2013). Consequently, nitrate injection shapes the microbial communities in petroleum reservoirs. Mn2+ and Mg2+ were shown to be strongly associated with the bacterial community of the aqueous phase based on the Bray–Curtis matrix (both p<0.05) (Table S8). Metal ions can be electron acceptors for direct or indirect hydrocarbon degradation under anaerobic conditions (Mbadinga et al., 2011). Metal reducers could also utilize electrons from syntrophic partners to further facilitate direct aromatic hydrocarbon degradation (Kunapuli et al., 2007).
Differences in taxa and function profiles in the oil and aqueous phases were analyzed based on LEfSe and Tax4Fun (Figs. S2 and S3, and Table S9). For bacterial communities, amino-acid metabolism and xenobiotics biodegradation and metabolism were distributed more in the oil phase, while carbohydrate metabolism was distributed more in the aqueous phase. For archaeal communities, ubiquinone and other terpenoid quinone biosynthesis and butanoate and tryptophan metabolism, together with other glycan degradation pathways were distributed more in the oil phase. Since FTU (fraction of taxa that could be mapped to existing KEGG pathway) values of archaeal communities were unevenly distributed from sample to sample, the reliability of these functional predictions is in question (Table S9). Meanwhile, the database of Tax4Fun is far from completeness, due to the enormous amount of uncultured microorganism and their unknown genomes. To date, there are still very limited studies investigating the inhabiting preference of microbiomes in petroleum reservoirs. Meanwhile, their functional contributions to hydrocarbon degradation and methanogenesis in both the aqueous and oil phases remain elusive, which calls for further efforts towards this point (Kryachko et al., 2012; Wang et al., 2014; Kobayashi et al., 2012).
A core bacterial microbiome containing a small proportion of OTUs but a relatively large proportion of sequences mediating hydrocarbon degradation and fermentation was revealed by analysis of oil reservoirs of different temperatures. The core and common bacterial microbiome of major biodegrading functions were shared among geographically and physicochemically different reservoirs. The different and shifting patterns of the dominant methanogenesis pathway in the aqueous and oil phases within samples of different temperatures were evident. Factors of pH; temperature; phase conditions; and nitrate, Mn2+, and Mg2+ concentrations shaped the microbial compositional and functional profiles significantly. Moreover, biomarker groups of bacteria and archaea associated with different pH, temperature, and phase conditions indicate major differences in the biochemical function of amino acid metabolism, xenobiotics metabolism enriched in the oil phase, and carbohydrate metabolism enriched in the aqueous phase.
The petroleum reservoir MiSeq 16S rRNA gene sequencing original datasets are available on the following GitHub page: https://github.com/ChaoLab/PetroleumMicrobiome (last access: 6 November 2019).
The supplement related to this article is available online at: https://doi.org/10.5194/bg-16-4229-2019-supplement.
ZZ, BL, LYW, BZM, HS, and JDG conceived the project and designed the experiments. ZZ, BL, and LYW conducted the sampling, chemical, and molecular experiments. JFL, BZM, and JDG managed sample collection and supervised data interpretation. ZZ performed the original data analysis and drafted the original manuscript. All members contributed to refining the manuscript and approved the final version.
The authors declare that they have no conflict of interest.
Xiangzhen Li's group at the Chengdu Institute of Biology, Chinese Academy of Sciences, is thanked for their MiSeq sequencing efforts and related technical support. Kelly Lau is thanked for her supportive technician work. We are grateful for the support from local administrative and technical staff at the Shengli, Daqing, Huabei, Xinjiang Karamay, and Jiangsu oilfields.
This research has been supported by the NSFC/RGC (grant no. N HKU718/11), the NSFC (grant nos. 41373070 and 41530318) and a postgraduate scholarship from HKU.
This paper was edited by Tina Treude and reviewed by two anonymous referees.
Angel, R., Claus, P., and Conrad, R.: Methanogenic archaea are globally ubiquitous in aerated soils and become active under wet anoxic conditions, ISME J., 6, 847–862, https://doi.org/10.1038/ismej.2011.141, 2012.
Asshauer, K. P., Wemheuer, B., Daniel, R., and Meinicke, P.: Tax4Fun: predicting functional profiles from metagenomic 16S rRNA data, Bioinformatics, 31, 2882–2884, https://doi.org/10.1093/bioinformatics/btv287, 2015.
Bian, X.-Y., Mbadinga, S. M., Liu, Y.-F., Yang, S.-Z., Liu, J.-F., Ye, R.-Q., Gu, J.-D., and Mu, B.-Z.: Insights into the Anaerobic Biodegradation Pathway of n-Alkanes in Oil Reservoirs by Detection of Signature Metabolites, Sci. Rep.-UK, 5, 9801, https://doi.org/10.1038/srep09801, 2015.
Caporaso, J. G., Kuczynski, J., Stombaugh, J., Bittinger, K., Bushman, F. D., Costello, E. K., Fierer, N., Pena, A. G., Goodrich, J. K., Gordon, J. I., Huttley, G. A., Kelley, S. T., Knights, D., Koenig, J. E., Ley, R. E., Lozupone, C. A., McDonald, D., Muegge, B. D., Pirrung, M., Reeder, J., Sevinsky, J. R., Tumbaugh, P. J., Walters, W. A., Widmann, J., Yatsunenko, T., Zaneveld, J., and Knight, R.: QIIME allows analysis of high-throughput community sequencing data, Nat. Methods, 7, 335–336, https://doi.org/10.1038/nmeth.f.303, 2010.
Caporaso, J. G., Lauber, C. L., Walters, W. A., Berg-Lyons, D., Huntley, J., Fierer, N., Owens, S. M., Betley, J., Fraser, L., Bauer, M., Gormley, N., Gilbert, J. A., Smith, G., and Knight, R.: Ultra-high-throughput microbial community analysis on the Illumina HiSeq and MiSeq platforms, ISME J., 6, 1621–1624, https://doi.org/10.1038/ismej.2012.8, 2012.
Dahle, H., Garshol, F., Madsen, M., and Birkeland, N.-K.: Microbial community structure analysis of produced water from a high-temperature North Sea oil-field, Anton. Van Lee., 93, 37–49, https://doi.org/10.1007/s10482-007-9177-z, 2008.
Daly, R. A., Borton, M. A., Wilkins, M. J., Hoyt, D. W., Kountz, D. J., Wolfe, R. A., Welch, S. A., Marcus, D. N., Trexler, R. V., and MacRae, J. D.: Microbial metabolisms in a 2.5-km-deep ecosystem created by hydraulic fracturing in shales, Nat. Microbiol., 1, 16146, https://doi.org/10.1038/nmicrobiol.2016.146, 2016.
Edgar, R. C.: Search and clustering orders of magnitude faster than BLAST, Bioinformatics, 26, 2460–2461, https://doi.org/10.1093/bioinformatics/btq461, 2010.
Edgar, R. C., Haas, B. J., Clemente, J. C., Quince, C., and Knight, R.: UCHIME improves sensitivity and speed of chimera detection, Bioinformatics, 27, 2194–2200, https://doi.org/10.1093/bioinformatics/btr381, 2011.
Gao, P., Li, G., Dai, X., Dai, L., Wang, H., Zhao, L., Chen, Y., and Ma, T.: Nutrients and oxygen alter reservoir biochemical characters and enhance oil recovery during biostimulation, World J. Microb. Biot., 29, 2045–2054, https://doi.org/10.1007/s11274-013-1367-4, 2013.
Gao, P., Tian, H., Li, G., Sun, H., and Ma, T.: Microbial diversity and abundance in the Xinjiang Luliang long-term water-flooding petroleum reservoir, MicrobiologyOpen, 4, 332–342, https://doi.org/10.1002/mbo3.241, 2015a.
Gao, P. K., Li, G. Q., Tian, H. M., Wang, Y. S., Sun, H. W., and Ma, T.: Differences in microbial community composition between injection and production water samples of water flooding petroleum reservoirs, Biogeosciences, 12, 3403–3414, https://doi.org/10.5194/bg-12-3403-2015, 2015b.
Grabowski, A., Nercessian, O., Fayolle, F., Blanchet, D., and Jeanthon, C.: Microbial diversity in production waters of a low-temperature biodegraded oil reservoir, FEMS Microbiol. Ecol., 54, 427–443, https://doi.org/10.1016/j.femsec.2005.05.007, 2005.
Gray, N. D., Sherry, A., Grant, R. J., Rowan, A. K., Hubert, C. R. J., Callbeck, C. M., Aitken, C. M., Jones, D. M., Adams, J. J., Larter, S. R., and Head, I. M.: The quantitative significance of Syntrophaceae and syntrophic partnerships in methanogenic degradation of crude oil alkanes, Environ. Microbiol., 13, 2957–2975, https://doi.org/10.1111/j.1462-2920.2011.02570.x, 2011.
Guo, H., Liu, R., Yu, Z., Zhang, H., Yun, J., Li, Y., Liu, X., and Pan, J.: Pyrosequencing reveals the dominance of methylotrophic methanogenesis in a coal bed methane reservoir associated with Eastern Ordos Basin in China, Int. J. Coal Geol., 93, 56–61, https://doi.org/10.1016/j.coal.2012.01.014, 2012.
Head, I. M., Jones, D. M., and Larter, S. R.: Biological activity in the deep subsurface and the origin of heavy oil, Nature, 426, 344–352, https://doi.org/10.1038/nature02134, 2003.
Kobayashi, H., Endo, K., Sakata, S., Mayumi, D., Kawaguchi, H., Ikarashi, M., Miyagawa, Y., Maeda, H., and Sato, K.: Phylogenetic diversity of microbial communities associated with the crude-oil, large-insoluble-particle and formation-water components of the reservoir fluid from a non-flooded high-temperature petroleum reservoir, J. Biosci. Bioeng., 113, 204–210, https://doi.org/10.1016/j.jbiosc.2011.09.015, 2012.
Kryachko, Y., Dong, X., Sensen, C. W., and Voordouw, G.: Compositions of microbial communities associated with oil and water in a mesothermic oil field, Anton. Van Lee., 101, 493–506, https://doi.org/10.1007/s10482-011-9658-y, 2012.
Kunapuli, U., Lueders, T., and Meckenstock, R. U.: The use of stable isotope probing to identify key iron-reducing microorganisms involved in anaerobic benzene degradation, ISME J., 1, 643–653, https://doi.org/10.1038/ismej.2007.73, 2007.
Lee, S.-H., Park, J.-H., Kim, S.-H., Yu, B. J., Yoon, J.-J., and Park, H.-D.: Evidence of syntrophic acetate oxidation by Spirochaetes during anaerobic methane production, Bioresource Technol., 190, 543–549, https://doi.org/10.1016/j.biortech.2015.02.066, 2015.
Lenchi, N., Inceoglu, O., Kebbouche-Gana, S., Gana, M. L., Lliros, M., Servais, P., and Garcia-Armisen, T.: Diversity of Microbial Communities in Production and Injection Waters of Algerian Oilfields Revealed by 16S rRNA Gene Amplicon 454 Pyrosequencing, PLoS One, 8, e66588, https://doi.org/10.1371/journal.pone.0066588, 2013.
Li, C.-Y., Zhang, D., Li, X.-X., Mbadinga, S. M., Yang, S.-Z., Liu, J.-F., Gu, J.-D., and Mu, B.-Z.: The biofilm property and its correlationship with high-molecular-weight polyacrylamide degradation in a water injection pipeline of Daqing oilfield, J. Hazard. Mater., 304, 388–399, https://doi.org/10.1016/j.jhazmat.2015.10.067, 2015.
Li, D., Midgley, D., Ross, J., Oytam, Y., Abell, G. J., Volk, H., Daud, W., and Hendry, P.: Microbial biodiversity in a Malaysian oil field and a systematic comparison with oil reservoirs worldwide, Arch. Microbiol., 194, 513–523, https://doi.org/10.1007/s00203-012-0788-z, 2012a.
Li, G., Gao, P., Wu, Y., Tian, H., Dai, X., Wang, Y., Cui, Q., Zhang, H., Pan, X., Dong, H., and Ma, T.: Microbial Abundance and Community Composition Influence Production Performance in a Low-Temperature Petroleum Reservoir, Environ. Sci. Technol., 48, 5336–5344, https://doi.org/10.1021/es500239w, 2014.
Li, H., Wang, X.-L., Mu, B.-Z., Gu, J.-D., Liu, Y.-D., Lin, K.-F., Lu, S.-G., Lu, Q., Li, B.-Z., Li, Y.-Y., and Du, X.-M.: Molecular detection, quantification and distribution of alkane-degrading bacteria in production water from low temperature oilfields, Int. Biodeterior. Biodegrad., 76, 49–57, https://doi.org/10.1016/j.ibiod.2012.06.007, 2013.
Li, W., Wang, L.-Y., Duan, R.-Y., Liu, J.-F., Gu, J.-D., and Mu, B.-Z.: Microbial community characteristics of petroleum reservoir production water amended with n-alkanes and incubated under nitrate-, sulfate-reducing and methanogenic conditions, Int. Biodeterior. Biodegrad., 69, 87–96, https://doi.org/10.1016/j.ibiod.2012.01.005, 2012b.
Liang, B., Wang, L.-Y., Mbadinga, S. M., Liu, J.-F., Yang, S.-Z., Gu, J.-D., and Mu, B.-Z.: Anaerolineaceae and Methanosaeta turned to be the dominant microorganisms in alkanes-dependent methanogenic culture after long-term of incubation, AMB Express, 5, 37, https://doi.org/10.1186/s13568-015-0117-4, 2015.
Liu, Y. and Whitman, W. B.: Metabolic, phylogenetic, and ecological diversity of the methanogenic archaea, Ann. NY Acad. Sci., 1125, 171–189, https://doi.org/10.1196/annals.1419.019, 2008.
Ludwig, W., Strunk, O., Westram, R., Richter, L., Meier, H., Yadhukumar, Buchner, A., Lai, T., Steppi, S., Jobb, G., Forster, W., Brettske, I., Gerber, S., Ginhart, A. W., Gross, O., Grumann, S., Hermann, S., Jost, R., Konig, A., Liss, T., Lussmann, R., May, M., Nonhoff, B., Reichel, B., Strehlow, R., Stamatakis, A., Stuckmann, N., Vilbig, A., Lenke, M., Ludwig, T., Bode, A., and Schleifer, K. H.: ARB: a software environment for sequence data, Nucleic Acids Res., 32, 1363–1371, https://doi.org/10.1093/nar/gkh293, 2004.
Magoc, T. and Salzberg, S. L.: FLASH: fast length adjustment of short reads to improve genome assemblies, Bioinformatics, 27, 2957–2963, https://doi.org/10.1093/bioinformatics/btr507, 2011.
Magot, M., Ollivier, B., and Patel, B. K. C.: Microbiology of petroleum reservoirs, Anton. Van Lee., 77, 103–116, https://doi.org/10.1023/a:1002434330514, 2000.
Mayumi, D., Mochimaru, H., Yoshioka, H., Sakata, S., Maeda, H., Miyagawa, Y., Ikarashi, M., Takeuchi, M., and Kamagata, Y.: Evidence for syntrophic acetate oxidation coupled to hydrogenotrophic methanogenesis in the high-temperature petroleum reservoir of Yabase oil field (Japan), Environ. Microbiol., 13, 1995–2006, https://doi.org/10.1111/j.1462-2920.2010.02338.x, 2011.
Mbadinga, S. M., Wang, L.-Y., Zhou, L., Liu, J.-F., Gu, J.-D., and Mu, B.-Z.: Microbial communities involved in anaerobic degradation of alkanes, Int. Biodeter. Biodegr., 65, 1–13, https://doi.org/10.1016/j.ibiod.2010.11.009, 2011.
Mbadinga, S. M., Li, K.-P., Zhou, L., Wang, L.-Y., Yang, S.-Z., Liu, J.-F., Gu, J.-D., and Mu, B.-Z.: Analysis of alkane-dependent methanogenic community derived from production water of a high-temperature petroleum reservoir, Appl. Microbiol. Biotechnol., 96, 531–542, https://doi.org/10.1007/s00253-011-3828-8, 2012.
Mesle, M., Dromart, G., and Oger, P.: Microbial methanogenesis in subsurface oil and coal, Res. Microbiol., 164, 959–972, https://doi.org/10.1016/j.resmic.2013.07.004, 2013.
Narihiro, T. and Sekiguchi, Y.: Oligonucleotide primers, probes and molecular methods for the environmental monitoring of methanogenic archaea, Microb. Biotechnol., 4, 585–602, https://doi.org/10.1111/j.1751-7915.2010.00239.x, 2011.
Nemati, M., Jenneman, G. E., and Voordouw, G.: Mechanistic study of microbial control of hydrogen sulfide production in oil reservoirs, Biotechnol. Bioeng., 74, 424–434, https://doi.org/10.1002/bit.1133, 2001.
Ollivier, B. and Alazard, D.: The oil reservoir ecosystem, in: Handbook of hydrocarbon and lipid microbiology, Springer-Verlag, Berlin, Heidelberg, Germany, 2259–2269, 2010.
Orphan, V. J., Taylor, L. T., Hafenbradl, D., and Delong, E. F.: Culture-dependent and culture-independent characterization of microbial assemblages associated with high-temperature petroleum reservoirs, Appl. Environ. Microb., 66, 700–711, https://doi.org/10.1128/aem.66.2.700-711.2000, 2000.
Orphan, V. J., Goffredi, S. K., Delong, E. F., and Boles, J. R.: Geochemical influence on diversity and microbial processes in high temperature oil reservoirs, Geomicrobiol. J., 20, 295–311, https://doi.org/10.1080/01490450303898, 2003.
Pham, V. D., Hnatow, L. L., Zhang, S., Fallon, R. D., Jackson, S. C., Tomb, J.-F., DeLong, E. F., and Keeler, S. J.: Characterizing microbial diversity in production water from an Alaskan mesothermic petroleum reservoir with two independent molecular methods, Environ. Microbiol., 11, 176–187, https://doi.org/10.1111/j.1462-2920.2008.01751.x, 2009.
Rideout, J. R., He, Y., Navas-Molina, J. A., Walters, W. A., Ursell, L. K., Gibbons, S. M., Chase, J., McDonald, D., Gonzalez, A., Robbins-Pianka, A., Clemente, J. C., Gilbert, J. A., Huse, S. M., Zhou, H.-W., Knight, R., and Caporaso, J. G.: Subsampled open-reference clustering creates consistent, comprehensive OTU definitions and scales to billions of sequences, PeerJ, 2, e545, https://doi.org/10.7717/peerj.545, 2014.
Segata, N., Izard, J., Waldron, L., Gevers, D., Miropolsky, L., Garrett, W. S., and Huttenhower, C.: Metagenomic biomarker discovery and explanation, Genome Biol., 12, R60, https://doi.org/10.1186/gb-2011-12-6-r60, 2011.
Sherry, A., Grant, R. J., Aitken, C. M., Jones, D. M., Head, I. M., and Gray, N. D.: Volatile hydrocarbons inhibit methanogenic crude oil degradation, Front. Microbiol., 5, 131, https://doi.org/10.3389/fmicb.2014.00131, 2014.
Takai, K. and Horikoshi, K.: Rapid detection and quantification of members of the archaeal community by quantitative PCR using fluorogenic probes, Appl. Environ. Microb., 66, 5066, https://doi.org/10.1128/aem.66.11.5066-5072.2000, 2000.
Tang, Y.-Q., Li, Y., Zhao, J.-Y., Chi, C.-Q., Huang, L.-X., Dong, H.-P., and Wu, X.-L.: Microbial Communities in Long-Term, Water-Flooded Petroleum Reservoirs with Different in situ Temperatures in the Huabei Oilfield, China, PLoS One, 7, e33535, https://doi.org/10.1371/journal.pone.0033535, 2012.
Voordouw, G., Armstrong, S. M., Reimer, M. F., Fouts, B., Telang, A. J., Shen, Y., and Gevertz, D.: Characterization of 16S rRNA genes from oil field microbial communities indicates the presence of a variety of sulfate-reducing, fermentative, and sulfide-oxidizing bacteria, Appl. Environ. Microb., 62, 1623–1629, 1996.
Wang, L.-Y., Gao, C.-X., Mbadinga, S. M., Zhou, L., Liu, J.-F., Gu, J.-D., and Mu, B.-Z.: Characterization of an alkane-degrading methanogenic enrichment culture from production water of an oil reservoir after 274 days of incubation, Int. Biodeter. Biodegr., 65, 444–450, https://doi.org/10.1016/j.ibiod.2010.12.010, 2011.
Wang, L.-Y., Duan, R.-Y., Liu, J.-F., Yang, S.-Z., Gu, J.-D., and Mu, B.-Z.: Molecular analysis of the microbial community structures in water-flooding petroleum reservoirs with different temperatures, Biogeosciences, 9, 4645–4659, https://doi.org/10.5194/bg-9-4645-2012, 2012.
Wang, L.-Y., Ke, W.-J., Sun, X.-B., Liu, J.-F., Gu, J.-D., and Mu, B.-Z.: Comparison of bacterial community in aqueous and oil phases of water-flooded petroleum reservoirs using pyrosequencing and clone library approaches, Appl. Microbiol. Biot., 98, 4209–4221, https://doi.org/10.1007/s00253-013-5472-y, 2014.
Wang, Y. and Qian, P.-Y.: Conservative fragments in bacterial 16S rRNA genes and primer design for 16S ribosomal DNA amplicons in metagenomic studies, PLoS One, 4, e7401, https://doi.org/10.1371/journal.pone.0007401, 2009.
Warren, E., Bekins, B. A., Godsy, E. M., and Smith, V. K.: Inhibition of acetoclastic methanogenesis in crude oil- and creosote-contaminated groundwater, Biorem. J., 8, 1–11, https://doi.org/10.1080/10889860490465840, 2004.
Wright, A. D. G. and Pimm, C.: Improved strategy for presumptive identification of methanogens using 16S riboprinting, J. Microbiol. Meth., 55, 337–349, https://doi.org/10.1016/s0167-7012(03)00169-6, 2003.
Yamane, K., Maki, H., Nakayama, T., Nakajima, T., Nomura, N., Uchiyama, H., and Kitaoka, M.: Diversity and Similarity of Microbial Communities in Petroleum Crude Oils Produced in Asia, Biosci. Biotech. Bioch., 72, 2831–2839, https://doi.org/10.1271/bbb.80227, 2008.
Youssef, N., Elshahed, M. S., and McInerney, M. J.: Microbial Processes in Oil Fields: Culprits, Problems, and Opportunities, Adv. Appl. Microbiol., 66, 141–251, 2009.
Yu, Z., Garcia-Gonzalez, R., Schanbacher, F. L., and Morrison, M.: Evaluations of different hypervariable regions of archaeal 16S rRNA genes in profiling of methanogens denaturing by Archaea-specific PCR and gradient gel electrophoresis, Appl. Environ. Microb., 74, 889–893, https://doi.org/10.1128/aem.00684-07, 2008.
Zhou, Z., Chen, J., Cao, H., Han, P., and Gu, J.-D.: Analysis of methane-producing and metabolizing archaeal and bacterial communities in sediments of the northern South China Sea and coastal Mai Po Nature Reserve revealed by PCR amplification of mcrA and pmoA genes, Front. Microbiol., 5, 789, https://doi.org/10.3389/fmicb.2014.00789, 2015.