the Creative Commons Attribution 4.0 License.
the Creative Commons Attribution 4.0 License.
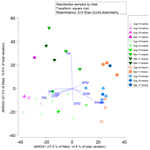
Biogeographical distribution of microbial communities along the Rajang River–South China Sea continuum
Edwin Sien Aun Sia
Zhuoyi Zhu
Jing Zhang
Wee Cheah
Shan Jiang
Faddrine Holt Jang
Aazani Mujahid
Fuh-Kwo Shiah
The Rajang River is the main drainage system for central Sarawak in Malaysian Borneo and passes through peat domes through which peat-rich material is being fed into the system and eventually into the southern South China Sea. Microbial communities found within peat-rich systems are important biogeochemical cyclers in terms of methane and carbon dioxide sequestration. To address the critical lack of knowledge about microbial communities in tropical (peat-draining) rivers, this study represents the first seasonal assessment targeted at establishing a foundational understanding of the microbial communities of the Rajang River–South China Sea continuum. This was carried out utilising 16S rRNA gene amplicon sequencing via Illumina MiSeq in size-fractionated samples (0.2 and 3.0 µm GF/C filter membranes) covering different biogeographical features and sources from headwaters to coastal waters. The microbial communities found along the Rajang River exhibited taxa common to rivers (i.e. predominance of β-Proteobacteria) while estuarine and marine regions exhibited taxa that were common to the aforementioned regions as well (i.e. predominance of α− and γ-Proteobacteria). This is in agreement with studies from other rivers which observed similar changes along salinity gradients. In terms of particulate versus free-living bacteria, nonmetric multi-dimensional scaling (NMDS) results showed similarly distributed microbial communities with varying separation between seasons. Distinct patterns were observed based on linear models as a result of the changes in salinity along with variation of other biogeochemical parameters. Alpha diversity indices indicated that microbial communities were higher in diversity upstream compared to the marine and estuarine regions, whereas anthropogenic perturbations led to increased richness but less diversity. Despite the observed changes in bacterial community composition and diversity that occur along the continuum of the Rajang River to the sea, the PICRUSt predictions showed minor variations. The results provide essential context for future studies such as further analyses on the ecosystem response to anthropogenic land-use practices and probable development of biomarkers to improve the monitoring of water quality in this region.
- Article
(6545 KB) - Full-text XML
-
Supplement
(1429 KB) - BibTeX
- EndNote
Biogeochemical transformations are primarily governed by microbial communities (Konopka, 2009), and it is crucial to understand their dynamics in order to predict biosphere modulations in response to a changing climate. Despite the importance of freshwater to society and despite hosting the highest microbial diversity (Besemer et al., 2013), microbial community composition and diversity in freshwater habitats, especially in lotic environments, are much less studied compared to marine and soil communities (Kan, 2018).
Lotic environments are the interface between soil and aquatic environments and aquatic environments as terrestrial environments seed microbes into the adjacent water column due to surface runoff (Crump et al., 2012). Until recently, rivers were thought to be passive channels in the carbon (C) cycling and weathering products until it became clear that rivers regulate for example the transfer of nutrients from land to coastal areas (Smith and Hollibaugh, 1993). Several studies have shown that bacteria are key players in nutrient processing in freshwater systems (Cotner and Biddanda, 2002; Findlay, 2010; Madsen, 2011). Zhang et al. (2018a) stated that the organic matter composition is strongly modified by bacteria as well as its resistance to degradation. Bacteria strongly influence the fluvial organic matter, hence playing a role in carbon cycle (Dittmar et al., 2001) and recent studies in the Rajang River have demonstrated that, as indicated by high concentrations of D-form amino acids (Zhu et al., 2019). Moreover, it was demonstrated by Jiang et al. (2019) that dissolved organic nitrogen was mineralised to , again highlighting the biogeochemical activity and the importance of microbes in the Rajang River. Until now, there has, however, been no study on their diversity, a gap that this study aims to fill. Thus, it is essential to understand the dynamics and structure of microbial communities in them to assess their contribution towards biogeochemical fluxes such as carbon and nitrogen (Battin et al., 2008; Raymond et al., 2013), as well as phosphorus cycling (Hall et al., 2013). In addition, the fluxes as well as transformations of organic matter as well as nutrients in aquatic systems are environmentally driven by parameters such as temperature or the availability of nutrients in these ecosystems (Welti et al., 2017). In turn, various gradients (i.e physical, chemical, hydrological or even biological) contribute to the changes in the microbial diversity and distribution living within the lotic environments (Zeglin, 2015).
Next-generation sequencing technologies have enabled a better understanding of the rare or unculturable biosphere which traditional culture methods would not have been able to elucidate (Boughner and Singh, 2016; Cao et al., 2017). Only a few studies assessing bacterial community composition have been undertaken in lotic or riverine environments (Fortunato et al., 2012; Ladau et al., 2013; Zwart et al., 2002), with even fewer focusing on the diversity of surface-attached biofilms in lotic environments, particularly in comparison to biofilm studies in benthic habitats (Zeglin, 2015). Furthermore, bacterial assemblages on suspended particles were shown to differ from free-living bacterioplankton in a number of studies (Bidle and Fletcher, 1995; Crump et al., 1999) in which the ratios between both fractions are often influenced by the quality of suspended particulate matter (Doxaran et al., 2012). Even fewer studies attempt to map bacterial community composition in a river-to-sea continuum across multiple seasons and habitats (Fortunato et al., 2012), and it was only recently reported that the most abundant riverine bacterioplankton resemble lake bacteria and can be regarded as “typical” freshwater bacteria (Lozupone and Knight, 2007; Zwart et al., 2002). Metagenomics studies substantiated the dominance of Proteobacteria and Actinobacteria while Bacteroidetes, Cyanobacteria and Verrucomicrobia were also found to be abundant in rivers (Cottrell et al., 2005; Kolmakova et al., 2014; Lemke et al., 2009; Newton et al., 2011; Read et al., 2015; Staley et al., 2013). While there are studies related to the freshwater–marine gradients of rivers such as studies by Crump and Hobbie (2005) and Fortunato et al. (2013) and tropical peatlands (Kanokratana et al., 2011; Mishra et al., 2014; Yule et al., 2016; Too et al., 2018), to the authors' knowledge, this is the first study which links both freshwater–marine gradients as well as tropical peatlands as a cohesive component (i.e. tropical peat-draining river to coastal ecosystem). Due to their high diversity and fast generation time, microbial communities (Hunt and Ward, 2015) are the first responders to environmental changes (both natural and anthropogenic events such as storms, upwelling and pollutants). Liao et al. (2019) showed that extensive agricultural land-use in the inter-tidal region of a watershed resulted in the prevalence of bacteria pathogen-like sequences, whereas Bruland et al. (2008) stated that the assemblages of microbes also vary temporally as a function of oceanographic conditions, river discharge, tidal phase and season.
This study focuses on the Rajang River, which is the longest river in Malaysia and one of the most socio-economically important peat-draining rivers in South East Asia. It transports large amounts of terrestrial material (Müller-Dum et al., 2019), experiences two monsoonal seasons (Sa'adi et al., 2017) and is subject to anthropogenic disturbances (Gaveau et al., 2016; Miettinen et al., 2016). Thus, it is fundamental to take into consideration both seasonal and anthropogenic influences on the microbial communities of the Rajang River. Given the rapid development in Sarawak and the importance of microbes in several biogeochemical processes in the Rajang River (Jiang et al., 2019; Martin et al., 2018; Müller-Dum et al., 2019; Zhu et al., 2019), it is imperative to study the microbial communities to enable future predictions and management responses. The Rajang River offers the opportunity to study the microbial diversity along a river-to-sea continuum and at the same time assess the influence of natural conditions such as seasons (dry vs. wet), different soil types (peat vs. mineral soil) and anthropogenic disturbances (e.g human settlements and plantations) on microbial succession. This study aims to investigate (1) the microbial community structure, diversity and probable function across wet and dry seasons in order to (2) understand the underlying factors that may influence the spatial and seasonal distribution of the prokaryotic communities and the nutrient dynamics involved in the Rajang River.
2.1 Study area and sampling strategy
This study was conducted along ∼300 km of the Rajang River in Sarawak, Malaysia (Fig. 1a). The region has an equatorial climate characterised by constant temperatures, high extensive rainfall and high humidity (Wang et al., 2009, 2005; see also Supplement Fig. S1). The Rajang delta system consists of an alluvial valley, an associated coastal plain and a delta plain (Staub and Esterle, 1993). The coastal plain is dissected into several small tributaries, namely Igan, Lassa, Paloh and Rajang (Fig. 1a). The shoreline experiences tides and seasonally strong waves ranging from 3–6 m with intensity increasing from the east to the west. According to Wetlands International (2015), the land surrounding the study sites is characterised by land use change (Fig. 1b) and a range of anthropogenic activities, such as oil palm and sago plantations (Fig. 1c), human settlements, and transportation and sand dredge.
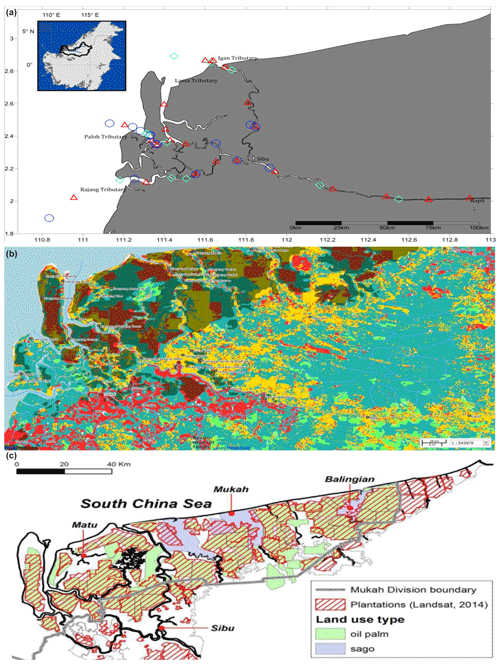
Figure 1Location of Rajang River within Sarawak, Malaysia (inset). Panel (a) shows the stations sampled during three different cruises: August 2016 (red triangles), March 2017 (blue circles) and September 2017 (cyan diamonds). (b) GIS data from 2010 (Sarawak Geoportal, 2018) indicating various forest types. Red colour represents non-forest areas (2010), yellow represents non-forest areas (2013), light green represents primary forests, teal represents secondary forests, and dark green represents potential peat swamp forests. (c) Digitised NREB map obtained from Wetlands International (2015). The map shows the plantation cover as determined from Landsat showing licensed oil palm and sago plantations (licensed).
A total of 59 water samples were collected along salinity gradients during three cruises (Fig. 1a), covering both wet and dry seasons as well as different source types (i.e. mineral or peat soils). Source types sampled were grouped as follows: (1) marine, (2) brackish peat, (3) freshwater peat and (4) mineral soils. From Sibu towards Kapit (upriver), the riparian zone is mineral soil, whereas from Sibu downwards to the coast it consists of peat which was then further divided into freshwater (salinity 0 to ∼1 PSU) and brackish water (salinity 2–28 PSU). The August 2016 cruise (coloured red) is classified as the dry season based on the lower mean rainfall value as compared to the other two (March and September 2017), in which both are classified as the wet season (refer to Fig. S1). The cruise in August 2016 represented the highest sampling frequency in order to obtain complete coverage of representative regions, while the cruises in March and September 2017 were aimed to obtain seasonal representatives for each region. Approximately 250–500 mL of water was filtered through 3.0 µm pore size track-etched membranes (Nucleopore™, Whatman, Germany) via vacuum filtration. This was referred to as the “Particulate-attached” fraction. The filtrate from the 3.0 µm portion was collected in a sterile glass bottle and subsequently filtered through 0.2 µm pore size track-etched membranes (Nucleopore™, Whatman, Germany). The smaller fraction was referred to as “free-living” fraction. A total of 117 filters were recovered (1×3.0 µm was discarded due to contamination) and immediately stored at −20 ∘C and sent to the Australian Centre for Ecogenomics (ACE), Brisbane, for DNA extraction, library preparation and processing utilising the Illumina platform (Bentley et al., 2008).
2.2 Illumina sequencing and bioinformatics analyses
Initial upstream processes were carried out by the Australian Centre for Ecogenomics utilising the ACE mitag pipeline (ACE, 2016). The primers utilised were based on the V3–V4 hypervariable regions of the 16S rRNA gene. Briefly, fastq files generated from the Illumina platform were quality-trimmed with fastqc, primer sequences were trimmed with Trimmomatic, and poor quality sequences were removed using a sliding window of 4 bases with an average base quality of more than 15. High-quality sequences were subsequently processed using the mothur (Schloss et al., 2009) pipeline. Sequences were aligned against the SILVA database (Quast et al., 2013; Yilmaz et al., 2014), a “pre.cluster” command was executed for de-noising, and chimeric sequences were removed using the “chimera.vsearch” function. Chimera-free 16S rRNA bacterial gene sequences were taxonomically assigned against the EzTaxon database (Kim et al., 2012) using the naïve Bayesian classifier with a threshold of 80 %. The quality-filtered sequences were then clustered into operational taxonomic units (OTUs) at 97 % similarity cutoff, with singleton OTUs being omitted. In order to reduce bias caused by variations in sample size, high-quality reads were randomly subsampled to 923 reads per sample. Apart from the results and discussion shown for free-living and particle-attached bacteria, the remaining discussion is based on the pooled results of both components. The alpha diversity was calculated using the estimate_richness function embedded within the plot_richness function found within the phyloseq package utilising R (v.3.5.3). For the analyses of potential functional genes, Phylogenetic Investigation of Communities by Reconstruction of Unobserved States (PICRUSt, Langille et al., 2013) was utilised. The metagenomics prediction table produced from PICRUSt was utilised to produce pathway abundance profiles using HUMAnN2 (Franzosa et al., 2018). It should be noted that the reconstructed functional genes were based on the GreenGenes (DeSantis et al., 2006) database and not the EzTaxon database used for the phylogeny.
2.3 Physico-chemical data and geochemical analyses
Monthly precipitation data for the period in between the cruises (August 2016 to September 2017) were obtained from the Tropical Rainfall Measuring Mission website (NASA, 2019) in order to gauge the seasonality (wet or dry; see Fig. S1). In the laboratory, nutrients (nitrate, ; nitrite, ; ammonium, ; Phosphate, ; and silicate, ) were photometrically determined utilising a SKALAR Sanplus continuous flow analyser in the State Key Laboratory of Estuarine and Coastal Research (SKLEC), Shanghai (details described in Sia et al., 2019). and were determined manually following Grasshoff et al. (1999). The total dissolved nitrogen (TDN) and total dissolved phosphate (TDP) were determined indirectly by obtaining the values for and via oxidation with an alkaline-persulfate solution (Ebina et al., 1983). The concentrations of dissolved organic nitrogen (DON) and dissolved organic phosphorus (DOP) were estimated by subtraction of DIN from TDN and from TDP, respectively. Belawai samples (2∘13′47.16′′ N, 111∘12′19.04′′ E) were used in an incubation experiment to study the net primary productivity and respiration rate of the Rajang River. Technical triplicates were incubated in both light and dark set-ups (refer to Supplement Table S1 for details).
2.4 Statistical analyses and distLM model
Ordination visualisation, non-metric multidimensional scaling (NMDS, Kruskal–Wallis: Kruskal stress formula: 1; minimum stress: 0.01), similarity analyses (ANOSIM) and coherence plots were executed using PRIMER 7 (Clarke and Gorley, 2015) to determine if the various terrestrial source types or different land use impacted the bacterial community. Permutational multivariate analysis of variance (PERMANOVA) was used based on the Bray–Curtis dissimilarity of the Hellinger transformed resemblance matrix to infer the impact of anthropogenic activities (land use) on the microbial communities. By partitioning the community variation (using a Bray–Curtis dissimilarity matrix resemblance), distance-based linear models (DistLM) were used to determine the extent to which the bacterial community structure can be explained by environmental variables (Legendre and Anderson, 1999). Normalising transformations of the environmental variables were carried out prior to execution of DistLM analyses using the “Normalise Variables” function in the PRIMER 7 software. A Hellinger transformed OTU abundance table was used as the response variable for the variation partition analysis. The authors would like to note that the distLM models are based on only the August 2016 and March 2017 cruise as there was a lack of physico-chemical data from the September 2017 cruise due to malfunctioning equipment. Multi-collinearity between variables was tested utilising the “Draftsman Plot” function in Primer 7 (Clarke and Gorley, 2006; Fig. S1). However, it is sufficient to draw linkages between the major drivers of microbial communities between seasons as March 2017 and September 2017 were considered wet seasons based on the average precipitation (see Fig. S1).
3.1 Clustering of samples according to ANOSIM global test scores
A total of 74 690 high-quality bacterial sequences were obtained from a total of 117 samples, with 200 to 2615 sequence reads per sample. The sequences were clustered into 2087 OTUs at the 97 % confidence interval. Instead of displaying bacterial diversity by station, bacterial communities were grouped together according to the R scores obtained from the ANOSIM global test, with the parameters “cruise”, “source type” and “land use” showing the highest scores (ANOSIM global R=0.737, P<0.001, Table 1). Furthermore, multi-variate analysis showed that the microbial community composition differed among the different land uses as well as sites nested with land use and source type (Table 2).
3.2 Shifts in bacterial community structure
The NMDS graph (2-D stress score: 0.18, Fig. 2) supported ANOSIM results by clustering samples according to (i) source type and land use as well as (ii) cruises. The x axis (MDS1 scores) clearly reflects changes in terms of salinity (river–sea continuum) while the y axis (MDS2 scores) emulates the different cruises. It is apparent that there were seasonal variations as shown from the lighter shade points, representing the August 2016 (dry season) samples, compared to those with darker shades representing both March and September 2017 (wet season) samples (Fig. 2). There were clear overlaps of samples from mineral soil and freshwater peat origin. We also observed a gradual shift of samples from mineral soils and freshwater peat towards brackish and then marine samples.
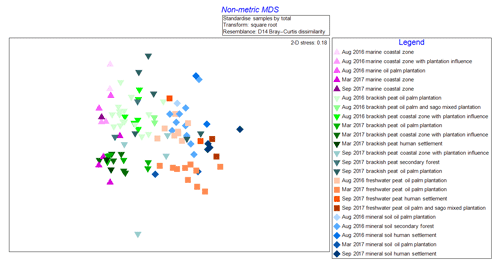
Figure 2Non-metric multi-dimensional scaling (NMDS) graph of samples according to cruise, source type and land use.
3.3 Bacterial distribution according to source type and cruise
To further support that the four different source types support distinct bacterial communities, the relative abundance was mapped into a percentage plot (Fig. 3).
The core microbial communities along the Rajang River–South China Sea continuum consist of Proteobacteria, Firmicutes, Actinobacteria, Bacteroidetes, Deinococcus–Thermus and Cyanobacteria in varying abundances (Figs. 3, S4), indicating high variation within the system. The phylum Deinococcus–Thermus was abundant in freshwater peat and in mineral soils, albeit at a lesser extent compared to freshwater peat (Fig. 3). Taking seasonality into consideration, the relative abundance (%) of Deinococcus–Thermus drastically decreased in September 2017. Contrary, the abundance of Cyanobacteria was greater within marine as well as brackish peat for the cruises of March and September 2017 but not for August 2016. For the August 2016 cruise, Cyanobacteria were found throughout all source types albeit at lower counts compared to the other cruises. Similar changes in bacterial community were observed during different cruises but at different sections of the river. For the freshwater peat and mineral soils, the cruises of August 2016 and March 2017 had greater resemblance towards each other. Furthermore, there was a distinct split in terms of the bacterial community composition for the four source types across all sampling cruises i.e. marine and brackish peat had similar composition and freshwater peat and mineral soils had similar composition. In terms of a river–sea continuum, the most apparent changes in the community composition were observed during March 2017, which presented an almost step-wise change in bacterial community composition.
3.4 Alpha diversity indices
Based on the observed indices (Fig. 4), mineral soils generally had the highest counts of unique OTUs. However, during the September 2017 cruise, the freshwater region had the highest values. Based on the Chao1 indices, there was a significant effect of the source type on the observed richness (p<0.001), with increasing values from marine to mineral soils. In the March and September 2017 cruise, the Chao1 indices were found to have greater variability as compared to the August 2017 cruise. For the September 17 cruise, we observed increased values of Chao1 across the brackish peat, freshwater peat and mineral soils. According to the Shannon indices, the diversity of the microbial communities varied significantly along the different source types (p<0.001). In the dry season the Shannon indices were found to be higher than those of March and September 2017 samples, except for the Brackish peat September 2017 samples. In terms of the Simpson diversity indices, the August 2016 season was found to have the higher values as compared to the March 2017 and September 2017 season.
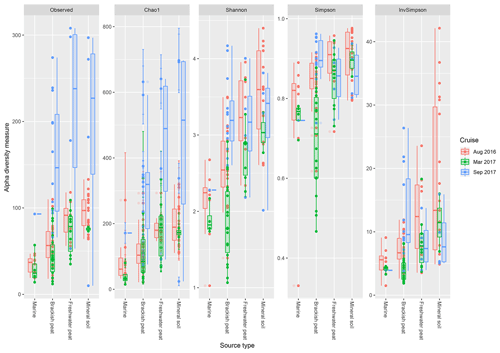
Figure 4The calculated α-diversity indices (observed, Chao1, Shannon, Simpson and inverse Simpson) of the four different source type along the salinity gradient.
Based on the effects of land use on the diversity indices (Fig. 5), the sites which are surrounded by human settlements had higher observed indices (regardless of the cruise), with the exception of the Shannon indices in August 2016. Samples surrounded by secondary forest had the second-highest values, with samples from August 2016 repeatedly higher than the other two cruises. There were significant differences (p<0.001) between samples from the coastal region with generally lower indices compared to upstream samples (i.e. human settlement, oil palm and sago plantation, oil palm plantation and secondary forest).
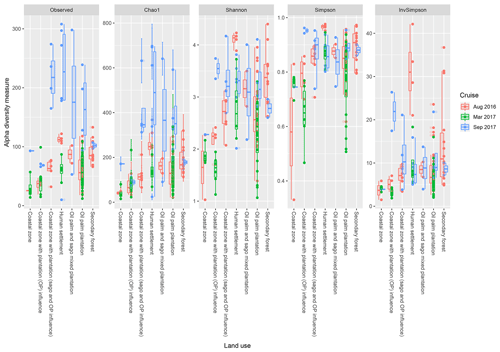
Figure 5The calculated α-diversity indices (observed, Chao1, Shannon, Simpson and inverse Simpson) of the land use types, which include coastal zone, coastal zone with plantation (OP) influence, coastal zone with plantation (sago and oil palm influence), human settlement, oil palm and sago mixed plantation, oil palm plantation, and secondary forest.
3.5 Functional profile of bacterial communities
Based on the potential KEGG pathways (Fig. 6), the functional profiles of the microbial communities were predicted for the August 2016 and March 2017 samples. The main functions found were oxidative phosphorylation (20.09 %), carbon fixation pathways in prokaryotes (19.00 %) and methane metabolism (18.36 %), respectively. This was then followed by nitrogen metabolism (11.50 %), carbon fixation in photosynthetic organisms (7.67 %), and inorganic ion transport and metabolism (5.68 %). The remaining functional groups were photosynthesis, sulfur metabolism, inositol phosphate metabolism, phosphotransferase system (PTS), carbohydrate metabolism, phosphonate and phosphinate metabolism, and lastly mineral absorption (4.92 %, 4.31 %, 2.96 %, 2.34 %, 1.83 %, 1.11 % and 0.23 %, respectively). Clear differences were observed between source types and seasons and potential KEGG pathways displayed similar composition among samples originating from either (i) marine and brackish peat, or (ii) freshwater peat and mineral soil. In terms of gene abundances, the March 2017 samples (wet season) were found to have higher gene abundances, with the highest counts in brackish peat followed by marine samples. However, marine samples in August 2016 displayed slightly higher gene counts compared to the brackish peat.
3.6 Distance-based linear model of bacterial communities and environmental parameters
Marginal DistLM was performed in order to gauge the extent of physicochemical parameters or environmental variables accounting for a compelling proportion of variation in the bacterial communities. Significant vectors of environmental variables (R2>0.3892, P<0.001) were calculated based on a linear model (DistLM) and plotted against the bacterial community composition (Fig. 7). Salinity was the single best predictor variable explaining bacterial community variation (15.27 %), followed by DIP (10.57 %). The remaining physico-chemical parameters were dissolved oxygen (DO, 9.64 %) and suspended particulate matter (SPM, 6.55 %), whereas for the biogeochemical parameters, silicate (9.27 %), DOP (8.04 %), DON (6.37 %), dissolved organic carbon (DOC, 5.27 %) and dissolved inorganic nitrogen (DIN, 4.29 %) respectively made up the remaining variables (all variables P=0.001, except for DIN, P=0.002).
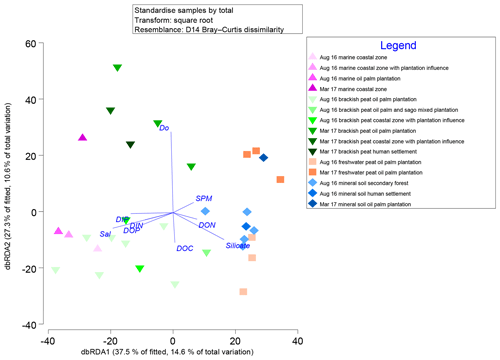
Figure 7Distance-based redundancy analysis (dbRDA) plot of cruise, source type and land use on a linear model (DistLM) of normalised predictor variables.
The distLM model clustered samples from the August 2016 cruise separately from the March 2017 samples. Brackish peat, as well as marine samples from August 2016, correlated more strongly with salinity, irrespective of land use. On the contrary, the March 2017 samples were found to cluster separately with DO. In addition, the August 2016 mineral soil samples correlated with silicate.
This study presents seasonal and spatial distribution of particulate-attached and free-living bacteria in the longest river in Malaysia in an attempt to map the bacterial community composition of the water column across several habitats with relation to the riparian zones and anthropogenic activities in a river-to-sea continuum. Our dataset develops a comparison of the microbial community across two dimensions: spatial biogeography from headwaters to the coastal zone as well as through time (seasonally). The rich supporting dataset also allows us to assess underlying nutrient dynamics influencing the microbial communities.
4.1 General diversity of core bacterial communities along the Rajang River–South China Sea continuum in comparison with global systems
The majority of bacterial taxa were restricted to a relatively small number of assemblages. Dominant phyla typically found in Malaysian peat swamps such as Proteobacteria (Kanokratana et al., 2011; Too et al., 2018; Tripathi et al., 2016) are found throughout the Rajang River, whereas Acidobacteria is not a major phylum in the Rajang River. However, due to the heterogeneity of the Rajang River, substantial shifts in OTU diversity were shown, while exhibiting successional changes in community composition downstream. We observed abrupt shifts in terms of richness and diversity as well as bacterial distribution, which were structured according to macro-scale source types. Staley et al. (2015) proposed that variability in microbial communities was lower due to the presence or absence of OTUs but likely due to shifts in their relative abundance. While there were shifts in the community composition, overlap between the core microbiome (i.e. free-living and particle-attached portions) of samples were evident (Figs. S2, S8). The similar bacterial community structure in terms of particle association was in line with studies by Noble et al. (1997) and Hollibough et al. (2000) in the Chesapeake Bay (winter season) and San Francisco Bay, respectively. Hollibough et al. (2000) demonstrated that the difference or similarity of the particle association of bacterial community was due to the origin as well as composition of the particles, particularly in marine snow or estuarine particles. In the aforementioned study, there was limited metabolic divergence and similar communities between the estuarine turbidity maxima and the river samples. Due to the short residence time, the rapid exchange of organisms likely reduced the divergence of phylogenetic composition. The short residence time in the Rajang River likely reflected a similar scenario to San Francisco Bay (Müller-Dum et al., 2019). When comparing with other rivers, the predominance of the Proteobacteria phylum, especially within the brackish peat region (Figs. 3, S4), was similar to a recent study on the Pearl River Delta (Chen et al., 2019). In another study by Doherty et al. (2017) on the main stem of the Amazon River (a blackwater-influenced river, similar to the Rajang River), Actinobacteria were much more abundant (25.8 %) compared to the Rajang River (11.95 %).
4.2 Factors determining bacterial community composition
4.2.1 Spatial and environmental drivers
As shown in Fig. 2, it can be observed that there was a continual shift in microbial communities, suggesting mixing of the microbial communities from the headwaters to the coast (Fortunato et al., 2012), which has also been observed along the Upper Mississippi River (Staley et al., 2015) and along the Danube River (Savio et al., 2015). The decrease in richness and evenness was similar to a study conducted by Savio et al. (2015) in which the bacterial evenness and richness declined downriver, which was in line with the river continuum concept (Vannote et al., 1980). The presence of peat did not affect the alpha diversity indices, which was reflected in the shift in taxa occurring from freshwater (which includes freshwater peat) towards the saline region (which includes brackish peat).
Salinity, DIP and DO are major environmental drivers of species distribution (Peter et al., 2011; Wilhelm et al., 2015). In this study, marine and brackish peat samples correlated well with salinity. This was neatly supported by the distribution of samples on the distLM fitted dbRDA graph (Fig. 7), in which the affinity for each of the samples correlates to the physical environment (e.g. the samples which group along the salinity vector were the samples which correlate with the marine as well as brackish peat region. The predominance of β-Proteobacteria in the freshwater region and the predominance of α- and γ-Proteobacteria (Fig. S3) in the estuarine region is typically the main group in seawaters (Nogales et al., 2011) and similar to findings by Silveira et al. (2011) on the bacterioplankton community along the river-to-ocean continuum from the Parnaioca River towards the Atlantic Ocean. This shows that salinity exhibited a strong influence on the abundances of Proteobacteria and Firmicutes. Furthermore, based on the linear model (Fig. 7), salinity was an important factor in driving the shift in microbial communities (Table 3), similar to findings by Herlemann et al. (2011) along a 200 km salinity gradient in the Baltic Sea. The dispersal of taxa of microbial communities from fresh to marine waters faces a strong barrier due to salinity (Fortunato and Crump, 2015), likely explaining the reduced relative abundances of some taxa (Fig. 3). For example, Chloroflexi has a higher relative abundance upstream while Deinococcus–Thermus shows lower relative abundance downstream. Such dispersals are further influenced by transitional waters such as estuaries and plumes, in which the microbial communities are exposed to rapidly changing physico-chemical conditions such as nutrients, temperature and sporadic anthropogenic inputs (Crump et al., 2004).
Table 3Proportion of combined community variation based on marginal DistLM test that is explained by each predictor variable using two cruises (August and March 2017).
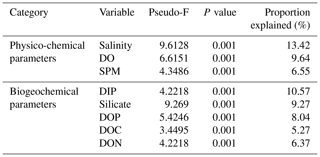
While the distribution of the core microbial communities are indicative of the river–sea continuum, it is noteworthy that several phyla were distinctly associated with specific source types. The distinct shift in bacterial taxa for example from freshwater to brackish waters (and lack thereof between freshwater peat and brackish peat; Fig. 3) indicates that peat did not have a significant effect on the distribution of bacterial taxa. This was further supported by the fact that DOC (as a proxy for organic matter of peat origin) only accounts for 5.27 % of the community variation (Table 3). A study on blackwater rivers in the Orinoco Basin, Venezuela (Castillo et al., 2004), showed that increased DOC resulted in higher bacterial production; however, the change in bacterial production was not a reflection of its influence on the community composition. This was supported based on a simple respiration experiment conducted in August 2016 (Supplement Table S1), in which the respiration rate (0.44±016 g DO L−1 d−1) was higher than that of the primary production rate (0.39±0.08 g DO L−1 d−1).
Samples influenced by DO (Fig. 7) are from the estuarine region which showed an almost anoxic zone (refer to Fig. S6). The low availability of oxygen was mirrored in higher counts (samples belonging to the brackish peat category showed the highest counts regardless of phyla as well as season; Fig. S4). However, higher counts (particularly the phylum Chloroflexi and Cyanobacteria which are normally associated with production of oxygen via primary productivity) do not reflect higher primary production within this zone. Zones of coastal estuaries are usually deemed to have higher primary productivity; however, it can be inferred that the depletion in oxygen and higher pCO2 emissions (Müller-Dum et al., 2019) within the brackish peat region of the August 2016 campaign was a result of high bacterial productivity. This can be further supported by the high SPM as a proxy of turbidity of the brackish peat (Fig. S6), which may have resulted in the reduced primary productivity, which in turn can explain the lower DO values. As aforementioned earlier, the respiration rate (0.44±016 g DO L−1 d−1) was higher than that of the primary production rate (0.39±0.08 DO L−1 d−1). This was similar to a study in the Scheldt River in which the higher bacterial production occurred in the turbidity maxima together with the depletion of oxygen (Goosen et al., 1995).
Functional potential of major taxa according to source types
In the Rajang River, the relative abundance of bacterial OTUs were higher in the estuary as well as marine region, reflecting that while the microbial communities are structured by salinity, the abundance was more a reflection of the nutrients available, especially in estuaries which exhibit circulation patterns which can result in localised nutrient-rich conditions (They et al., 2019). This was further supported by the higher relative abundance of oxidative phosphorylation genes as well as the nitrogen metabolism within the brackish peat and further supported by Jiang et al. (2019) demonstrated through incubation studies in which N transformations in the Rajang River estuary mixing zone were higher than those in the Rajang River and coastal region. In a study done by Yang et al. (2013), the dominance of Proteobacteria influenced the nitrogen cycle via the processes of nitrification and denitrification, in which aeration would increase its abundance and result in higher mortality of Cyanobacteria. Hence, lower Proteobacteria abundance resulted in the higher abundance of Cyanobacteria which occur as evidently shown in Fig. 3. Furthermore, the higher presence of Chloroflexi (Ward et al., 2018) and Cyanobacteria (Guida et al., 2017) within the marine and brackish peat region indicated its probable role in carbon fixation, as reflected by the higher gene counts (carbon fixation pathways in prokaryotes) in the marine and brackish peat regions as compared to the freshwater peat and mineral soil (Fig. 6). Furthermore, the presence of the genus Sphingomonas, a purple-sulfur bacterium, allowed the utilisation of carbon dioxide (carbon fixation pathways in prokaryotes) and oxidation of hydrogen sulfide (sulfur metabolism) (Pfennig, 1975) (Fig. 6). In the case of Firmicutes, the higher abundance of Firmicutes in the brackish region was reflective of the overall production as opposed to selective growth of the particular source type, as Firmicutes were found throughout all four source types. The highest level of Deinococcus–Thermus (Fig. 3) was found in freshwater peat environments, indicating its preference for this environment. It was interesting to note that most studies on bacterial community composition show that the phylum Deinococcus–Thermus occurs in a higher abundance in extreme environments such as in hot springs (Zhang et al., 2018b) or in studies that are analogous for Mars (Joseph et al., 2019). In most of these studies, Deinococcus–Thermus was found in low abundance (e.g. 1 % in Antarctic marine environments, 1.5 % in hypersaline soils; Giudice and Azzaro, 2019; Vera-Gargallo et al., 2019) when compared to the Rajang River.
4.2.2 Seasonality as a driver of microbial community composition
While the development of unique community structures was strongly influenced by spatial factors, seasonality also played a role. When taking into consideration the major genera, there was a fundamental shift in bacterial community composition along the continuum (Figs. 3, 4). The second-most abundant taxon, Proteobacteria (β-Proteobacteria) peaked during seasons of high discharge. The same pattern of peaking during high discharge can be observed in the Rajang River, with considerably higher relative abundance in the wet season (Fig. 3) which could be a result of the intense rainfall that led to the large input of freshwater (Silveira et al., 2011), and ultimately resulting in a “trickling” over microbial patterns from the freshwater to the brackish region. Furthermore, there was a distinct difference in terms of bacterial richness and diversity indices between the dry season (August 2016) and both wet seasons, with September 2017 having higher observed indices, while March 2017, despite being a wet season as well, had lower or variable observed indices. This difference in the two wet seasons could be the due to different stages of phytoplankton bloom as mentioned earlier, as an algal bloom occurred in September 2017 while March 2017 was after an algal bloom event. This was reflected in the Simpson index as well as the indices for September 2017 being lower than those of the August 2016 or March 2017 samples. Similarly, Zhou et al. (2018) demonstrated that the Simpson Indices for bacteria increased after the onset of an algal bloom (brackish peat, September 2017), whereas the Shannon indices was at the lowest (brackish peat, March 2017) (when assuming that the region in which phytoplankton blooms occur was the brackish peat region). Overall, there was greater diversity (based on Shannon indices) in the dry season (August 2016) than the wet seasons (March and September 2017), whereas there were greater OTUs in the wet season (observed index).
Seasonal variability was also observed between the source types, particle association and down to the genus level (Figs. 2, S2 and S5). Based on the precipitation as an indicator of the seasonality, a probable “transitioning” phase was observed in the dry season (August 2016), with the microbial communities being more alike with the March 2017 samples (Fig. 8) when comparing both wet seasons (March and September 2017). Within the phylum rank (Fig. 3), the presence of Cyanobacteria during the March and September 2017 cruises indicates the influence of seasonality. However, while March and September 2017 were both considered to be wet seasons based on the precipitation, in terms of the relative abundance, there are considerable differences between the two cruises. The greater abundance of Bacteroidetes in March 2017 may be indicative of the community composition adjusting due to the processing of organic material caused by the higher cyanobacterial abundance in the September 2017 cruise. This was similar to a study by Pinhassi et al. (2004), in which the higher abundance of Bacteroidetes follows after an algal bloom. Moreover, the shifts in community composition from August 2016 to March 2017 and from March to September 2017 are indicative of the influence of seasonality. While March and September 2017 were similar in terms of climate, September 2017 had higher precipitation during that month, which led to higher run-off from the riparian region as compared with the March 2017 wet season. This could have led to the increase in cyanobacteria, which was also reflected in the increase of picoplankton size class during the wet season, as it was hypothesised that the September 2017 might be more optimal for picoplankton proliferation (Fig. S7). Furthermore, in comparison, August 2016 and March 2017 were similar in terms of the proportion of the relative abundance of the community composition (Fig. 3).
4.2.3 Land-use change and anthropogenic drivers
There has been little to no literature regarding the changes in microbial community composition as a result of land-use changes that occur within this region, particularly throughout the catchment area of the Rajang River. The results obtained from this study suggest that the run-off from anthropogenic activities alters the microbial community composition. The Cytophaga–Flavobacterium–Bacteroidetes group, known as the CFB group, is commonly associated with humans (Weller et al., 2000), reflecting anthropogenic influences on the samples, especially within the brackish areas which have several human settlements and plantations. This was shown in the coherence plots in Figs. S10 and S11, in which the CFB group in the Bacteroidetes phylum is shown to be more pronounced in areas with influence on oil palm plantations. Lee-Cruz et al. (2013) demonstrated that conversions of tropical forests to oil palm plantations are much more severe as compared to logged-over forests in terms of bacterial community composition, as logged-over forests were shown to exhibit some resilience and resistance (to a certain extent). This was shown in the clustering of bacterial taxa adjacent to the oil palm plantation regardless of the source type (Fig. S12), as the vector of Flavobacteriia decreases in the samples of oil palm plantation in the brackish peat, as does, to a certain extent, the vector of Bacteroidia in the oil palm plantation samples in the freshwater peat. Furthermore, among the identified possible pathogenic bacteria, according to Reza et al. (2018), the taxa Flavobacterium is a potential fish pathogen and is commonly found in freshwater habitats (Lee and Eom, 2017) as well as coastal pelagic zones (Eilers et al., 2001). In the Rajang River, it was the sixth most abundant class (Fig. S4). This is cause for concern as it was found to be high in the coastal regions as well as brackish regions where fisheries and fishing activities are concentrated.
Anthropogenic disturbances, in particular settlements and logging (secondary forest), led to higher diversity indices (Fig. 6). On the contrary, sites surrounded by oil palm plantations displayed the lowest diversity indices, supporting results by Mishra et al. (2014) who found similar results in peatlands. Furthermore, the OTU overlapping of major anthropogenic activities (i.e. settlements and oil palm plantations) in Fig. S9 reflected the possibility of higher abundance of generalists as compared to sensitive species (Jordaan et al., 2019), as microbial communities generally adapt to permanent stress events such as increased concentrations of inorganic or organic nutrients. In another study conducted by Fernandes et al. (2014), anthropogenically influenced mangroves had 2 times more γ-Proteobacteria compared to pristine mangroves. This was similar to the March 2017 cruise along the Rajang River, in which γ-Proteobacteria was the predominant class in the marine and brackish peat region along with the significant increase in the aforementioned Bacteroidetes, which can be associated to anthropogenic activities. On the other hand, during the dry season, the diversity of the “less-disturbed” region was higher than the disturbed regions. However, it should be noted that the coastal zone generally has the lowest richness and diversity amongst the other regions regardless of the presence or absence of anthropogenic activities. Hence, the extent of salinity intrusion may also result in the loss of diversity and richness of the microbial communities (Shen et al., 2018) in the Rajang River.
This study represents the first assessment of the microbial communities of the Rajang River, the longest river in Malaysia, expanding our knowledge of microbial ecology in tropical regions. The predominant taxa are Proteobacteria (50.29 %), followed by Firmicutes (22.35 %) and Actinobacteria (11.95 %). The microbial communities were found to change according to the source type, as distinct patterns were observed as a result of the changes in salinity along with variation of other biogeochemical parameters. Alpha diversity indices indicate that the microbial diversity was higher upstream as compared to the marine and estuarine regions, whereas anthropogenic perturbations (regions with oil palm plantations and human settlements) led to increased richness but less diversity compared to those that were less affected by anthropogenic perturbations (coastal zone and secondary forest). The PICRUSt results showed differences between source types. Areas surrounded by oil palm plantations showed the lowest diversity and other signs of anthropogenic impacts included the presence of the CFB group as well as probable algal blooms. In order to further gauge and substantiate the functional and metabolic capacity of the microbial communities within each specific source type, metaproteomics as well as metabolomics should be carried out along with mixing experiments. This approach will contribute towards a better understanding of the response of microbial communities to anthropogenic perturbations, as well as their role in degrading peat-related run-off from the surrounding riparian regions.
Raw sequences have been deposited with the NCBI BioSample database under BioProject ID PRJNA565954.
The supplement related to this article is available online at: https://doi.org/10.5194/bg-16-4243-2019-supplement.
ESAS, MM, JZ, and AM designed the study. ESAS, FHJ, and SJ performed the sample preparation during the campaigns. SJ and ZZ performed the nutrient measurements. WH and FKS performed the picoplankton measurements. ESAS and MM prepared the paper with contributions from all co-authors.
The authors declare that they have no conflict of interest.
This article is part of the special issue “Biogeochemical processes in highly dynamic peat-draining rivers and estuaries in Borneo”. It is not associated with a conference.
The authors would like to thank the Sarawak Forestry Department and Sarawak Biodiversity Centre for permission to conduct collaborative research in Sarawak waters under permit numbers NPW.907.4.4(Jld.14)-161, Park Permit no. WL83/2017 and SBC-RA-0097-MM. Special mention to the boatmen who helped us to collect samples, in particular Lukas Chin and his crew during the Rajang River cruises. Also, the authors are very grateful to Kim Mincheol of KOPRI for providing the mothur codes and supercomputer for processing the sequences. We would also like to thank Patrick Martin for providing DOC measurements and Denise Müller-Dum for providing SPM measurements. Gonzalo Carassco and Nagur Cherukuru as well as student helpers from UNIMAS, Swinburne Sarawak, SKLEC and NOCS greatly aided with the logistics and fieldwork.
This research has been supported by the MOHE FRGS 15 Grant (grant no. FRGS/1/2015/WAB08/SWIN/02/1), the SKLEC Open Research Fund (grant no. SKLEC-KF201610), and the Newton-Ungku Omar Fund (grant no. NE/P020283/1).
This paper was edited by Palanisamy Shanmugam and reviewed by two anonymous referees.
ACE: ACE pipeline, available at: https://wiki.ecogenomic.org/doku.php?id=amplicon_pipeline_readme (last access: 1 September 2018), 2016.
Battin, T. J., Kaplan, L. A., Findlay, S., Hopkinson, C. S., Marti, E., Packman, A. I., Newbold, J. D., and Sabater, F.: Biophysical controls on organic carbon fluxes in fluvial networks, Nat. Geosci., 1, 95–100, 2008.
Bentley, D. R., Balasubramanian, S., Swerdlow, H. P., Smith, G. P., Milton, J., Brown, C. G., Hall, K. P., Evers, D. J., Barnes, C. L., Bignell, H. R., Boutell, J. M., Bryant, J., Carter, R. J., Keira Cheetham, R., Cox, A. J., Ellis, D. J., Flatbush, M. R., Gormley, N. A., Humphray, S. J., Irving, L. J., Karbelashvili, M. S., Kirk, S. M., Li, H., Liu, X., Maisinger, K. S., Murray, L. J., Obradovic, B., Ost, T., Parkinson, M. L., Pratt, M. R., Rasolonjatovo, I. M. J., Reed, M. T., Rigatti, R., Rodighiero, C., Ross, M. T., Sabot, A., Sankar, S. V, Scally, A., Schroth, G. P., Smith, M. E., Smith, V. P., Spiridou, A., Torrance, P. E., Tzonev, S. S., Vermaas, E. H., Walter, K., Wu, X., Zhang, L., Alam, M. D., Anastasi, C., Aniebo, I. C., Bailey, D. M. D., Bancarz, I. R., Banerjee, S., Barbour, S. G., Baybayan, P. A., Benoit, V. A., Benson, K. F., Bevis, C., Black, P. J., Boodhun, A., Brennan, J. S., Bridgham, J. A., Brown, R. C., Brown, A. A., Buermann, D. H., Bundu, A. A., Burrows, J. C., Carter, N. P., Castillo, N., Chiara E. Catenazzi, M., Chang, S., Neil Cooley, R., Crake, N. R., Dada, O. O., Diakoumakos, K. D., Dominguez-Fernandez, B., Earnshaw, D. J., Egbujor, U. C., Elmore, D. W., Etchin, S. S., Ewan, M. R., Fedurco, M., Fraser, L. J., Fuentes Fajardo, K. V, Scott Furey, W., George, D., Gietzen, K. J., Goddard, C. P., Golda, G. S., Granieri, P. A., Green, D. E., Gustafson, D. L., Hansen, N. F., Harnish, K., Haudenschild, C. D., Heyer, N. I., Hims, M. M., Ho, J. T., et al.: Accurate whole human genome sequencing using reversible terminator chemistry, Nature, 456, 53–59, 2008.
Besemer, K., Singer, G., Quince, C., Bertuzzo, E., Sloan, W., and Battin, T. J.: Headwaters are critical reservoirs of microbial diversity for fluvial networks, Proc. R. Soc. B, 280, 20131760, https://doi.org/10.1098/rspb.2013.1760, 2013.
Bidle, K. D. and Fletcher, M.: Comparison of free-living and particle-associated bacterial communities in the chesapeake bay by stable low-molecular-weight RNA analysis, Appl. Environ. Microbiol., 61, 944–952, 1995.
Boughner, L. A. and Singh, P.: Microbial Ecology: Where are we now?, Postdoc J., 4, 3–17, https://doi.org/10.14304/surya.jpr.v4n11.2, 2016.
Bruland, K. W., Lohan, M. C., Aguilar-Islas, A. M., Smith, G. J., Sohst, B., and Baptista, A.: Factors influencing the chemistry of the near-field Columbia River plume: Nitrate, silicic acid, dissolved Fe, and dissolved Mn, J. Geophys. Res.-Ocean., 113, C00B02, https://doi.org/10.1029/2007JC004702, 2008.
Cao, Y., Fanning, S., Proos, S., Jordan, K., and Srikumar, S.: A review on the applications of next generation sequencing technologies as applied to food-related microbiome studies, Front. Microbiol., 8, 1–16, https://doi.org/10.3389/fmicb.2017.01829, 2017.
Castillo, M. M., Allan, J. D., Sinsabaugh, R. L., and Kling, G. W.: Seasonal and interannual variation of bacterial production in lowland rivers of the Orinoco basin, Freshw. Biol., 49, 1400–1414, https://doi.org/10.1111/j.1365-2427.2004.01277.x, 2004.
Chen, L., Tsui, M. M. P., Lam, J. C. W., Hu, C., Wang, Q., Zhou, B., and Lam, P. K. S.: Variation in microbial community structure in surface seawater from Pearl River Delta: Discerning the influencing factors, Sci. Total Environ., 660, 136–144, https://doi.org/10.1016/j.scitotenv.2018.12.480, 2019.
Clarke, K. and Gorley, R.: PRIMER version 7: User manual/tutorial, 2015.
Clarke, K. R. and Gorley, R.: PRIMER v6: User Manual/Tutorial (Plymouth Routines in Multivariate Ecological Research), PRIMER-E, Plymouth, 190 pp., 2006.
Cotner, J. B. and Biddanda, B. A.: Small Players, Large Role: Microbial Influence on Biogeochemical Processes in Pelagic Aquatic Ecosystems, Ecosystems, 5, 105–121, https://doi.org/10.1007/s10021-001-0059-3, 2002.
Cottrell, M. T., Waidner, L. A., Yu, L., and Kirchman, D. L.: Bacterial diversity of metagenomic and PCR libraries from the Delaware River, Environ. Microbiol., 7, 1883–1895, https://doi.org/10.1111/j.1462-2920.2005.00762.x, 2005.
Crump, B. C., Hopkinson, C. S., Sogin, M. L., and Hobbie, J. E.: Microbial Biogeography along an Estuarine Salinity Gradient: Combined Influences of Bacterial Growth and Residence Time, Appl. Environ. Microbiol., 70, 1494–1505, https://doi.org/10.1128/AEM.70.3.1494-1505.2004, 2004.
Crump, B. C., Amaral-Zettler, L. A., and Kling, G. W.: Microbial diversity in arctic freshwaters is structured by inoculation of microbes from soils, ISME J., 6, 1629–1639, https://doi.org/10.1038/ismej.2012.9, 2012.
DeSantis, T. Z., Hugenholtz, P., Larsen, N., Rojas, M., Brodie, E. L., Keller, K., Huber, T., Dalevi, D., Hu, P., and Andersen, G. L.: Greengenes, a Chimera-Checked 16S rRNA Gene Database and Workbench Compatible with ARB, Appl. Environ. Microbiol., 72, 5069–5072, https://doi.org/10.1128/AEM.03006-05, 2006.
Dittmar, T., Fitznar, H. P., and Kattner, G.: Origin and biogeochemical cycling of organic nitrogen in the eastern Arctic Ocean as evident from D- and L-amino acids, Geochim. Cosmochim. Ac., 65, 4103–4114, https://doi.org/10.1016/S0016-7037(01)00688-3, 2001.
Doherty, M., Yager, P. L., Moran, M. A., Coles, V. J., Fortunato, C. S., Krusche, A. V., Medeiros, P. M., Payet, J. P., Richey, J. E., Satinsky, B. M., Sawakuchi, H. O., Ward, N. D., and Crump, B. C.: Bacterial biogeography across the Amazon River-ocean continuum, Front. Microbiol., 8, 1–17, https://doi.org/10.3389/fmicb.2017.00882, 2017.
Doxaran, D., Ehn, J., Bélanger, S., Matsuoka, A., Hooker, S., and Babin, M.: Optical characterisation of suspended particles in the Mackenzie River plume (Canadian Arctic Ocean) and implications for ocean colour remote sensing, Biogeosciences, 9, 3213–3229, https://doi.org/10.5194/bg-9-3213-2012, 2012.
Ebina, J., Tsutsui, T., and Shirai, T.: Simultaneous determination of total nitrogen and total phosphorus in water using peroxodisulfate oxidation, Water Res., 17, 1721–1726, https://doi.org/10.1016/0043-1354(83)90192-6, 1983.
Eilers, H., Pernthaler, J., Peplies, J., Glöckner, F. O., Gerdts, G., and Amann, R.: Isolation of Novel Pelagic Bacteria from the German Bight and Their Seasonal Contributions to Surface Picoplankton, Appl. Environ. Microbiol., 67, 5134–5142, https://doi.org/10.1128/AEM.67.11.5134-5142.2001, 2001.
Fernandes, S. O., Kirchman, D. L., Michotey, V. D., Bonin, P. C., and Lokabharathi, P. A.: Bacterial diversity in relatively pristine and anthropogenically-influenced mangrove ecosystems (Goa, India), Brazilian J. Microbiol., 45, 1161–1171, https://doi.org/10.1590/S1517-83822014000400006, 2014.
Findlay, S.: Stream microbial ecology, J. North Am. Benthol. Soc., 29, 170–181, 2010.
Fortunato, C. S. and Crump, B. C.: Microbial gene abundance and expression patterns across a river to ocean salinity gradient, PLoS One, 10, 1–22, https://doi.org/10.1371/journal.pone.0140578, 2015.
Fortunato, C. S., Herfort, L., Zuber, P., Baptista, A. M., and Crump, B. C.: Spatial variability overwhelms seasonal patterns in bacterioplankton communities across a river to ocean gradient, Isme J., 6, 554–563, 2012.
Fortunato, C. S., Eiler, A., Herfort, L., Needoba, J. A., Peterson, T. D., and Crump, B. C.: Determining indicator taxa across spatial and seasonal gradients in the Columbia River coastal margin, Isme J., 7, 1899–1911, 2013.
Franzosa, E. A., McIver, L. J., Rahnavard, G., Thompson, L. R., Schirmer, M., Weingart, G., Lipson, K. S., Knight, R., Caporaso, J. G., Segata, N., and Huttenhower, C.: Species-level functional profiling of metagenomes and metatranscriptomes, Nat. Methods, 15, 962–968, https://doi.org/10.1038/s41592-018-0176-y, 2018.
Gaveau, D. L. A., Salim, M., and Arjasakusuma, S.: Deforestation and industrial plantations development in Borneo, https://doi.org/10.17528/CIFOR/DATA.00049, 2016.
Giudice, A. and Azzaro, M.: The Ecological Role of Micro-organisms in the Antarctic Environment, edited by: Castro-Sowinski, S., Springer Polar, 298 pp., 2019.
Goosen, N. K., van Rijswijk, P., and Brockmann, U.: Comparison of heterotrophic bacterial production rates in early spring in the turbid estuaries of the Scheldt and the Elbe, Hydrobiologia, 311, 31–42, https://doi.org/10.1007/BF00008569, 1995.
Grasshoff, K., Kremling, K., and Ehrhardt, M.: Methods of Seawater Analysis, 3rd Edn., Wiley-VCH, Weinheim, 600 pp., 1999.
Guida, B. S., Bose, M., and Garcia-Pichel, F.: Carbon fixation from mineral carbonates, Nat. Commun., 8, 1–6, https://doi.org/10.1038/s41467-017-00703-4, 2017.
Hall, R. O., Baker, M. A., Rosi-Marshall, E. J., Tank, J. L., and Newbold, J. D.: Solute-specific scaling of inorganic nitrogen and phosphorus uptake in streams, Biogeosciences, 10, 7323–7331, https://doi.org/10.5194/bg-10-7323-2013, 2013.
Herlemann, D. P. R., Labrenz, M., Jürgens, K., Bertilsson, S., Waniek, J. J., and Andersson, A. F.: Transitions in bacterial communities along the 2000 km salinity gradient of the Baltic Sea, ISME J., 5, 1571–1579, https://doi.org/10.1038/ismej.2011.41, 2011.
Hollibough, J., S. Wong, P., and Murrell, M.: Similarity of particle-associated and free-living bacterial communities in northern San Francisco Bay, California, Aquat. Microb. Ecol., 21, 103–114, 2000.
Hunt, D. E. and Ward, C. S.: A network-based approach to disturbance transmission through microbial interactions , Front. Microbiol. , 6, 1182, https://doi.org/10.3389/fmicb.2015.01182, 2015.
Jiang, S., Müller, M., Jin, J., Wu, Y., Zhu, K., Zhang, G., Mujahid, A., Rixen, T., Muhamad, M. F., Sia, E. S. A., Jang, F. H. A., and Zhang, J.: Dissolved inorganic nitrogen in a tropical estuary in Malaysia: transport and transformation, Biogeosciences, 16, 2821–2836, https://doi.org/10.5194/bg-16-2821-2019, 2019.
Jordaan, K., Comeau, A. M., Khasa, D. P., and Bezuidenhout, C. C.: An integrated insight into the response of bacterial communities to anthropogenic contaminants in a river: A case study of the Wonderfonteinspruit catchment area, South Africa, PLoS One, 14, e0216758, https://doi.org/10.1371/journal.pone.0216758, 2019.
Joseph, R. G., Dass, R. S., Rizzo, V., Cantasano, N., and Bianciardi, G.: Evidence of Life on Mars?, 1, 40–81, 2019.
Kan, J.: Storm Events Restructured Bacterial Community and Their Biogeochemical Potentials, J. Geophys. Res.-Biogeo., 123, 2257–2269, https://doi.org/10.1029/2017JG004289, 2018.
Kanokratana, P., Uengwetwanit, T., Rattanachomsri, U., Bunterngsook, B., Nimchua, T., Tangphatsornruang, S., Plengvidhya, V., Champreda, V., and Eurwilaichitr, L.: Insights into the Phylogeny and Metabolic Potential of a Primary Tropical Peat Swamp Forest Microbial Community by Metagenomic Analysis, Microb. Ecol., 61, 518–528, https://doi.org/10.1007/s00248-010-9766-7, 2011.
Kim, O.-S., Cho, Y.-J., Lee, K., Yoon, S.-H., Kim, M., Na, H., Park, S.-C., Jeon, Y. S., Lee, J.-H., Yi, H., Won, S., and Chun, J.: Introducing EzTaxon-e: a prokaryotic 16S rRNA gene sequence database with phylotypes that represent uncultured species, Int. J. Syst. Evol. Microbiol., 62, 716–21, https://doi.org/10.1099/ijs.0.038075-0, 2012.
Kolmakova, O. V., Gladyshev, M. I., Rozanov, A. S., Peltek, S. E., and Trusova, M. Y.: Spatial biodiversity of bacteria along the largest Arctic river determined by next-generation sequencing, FEMS Microbiol. Ecol., 89, 442–450, https://doi.org/10.1111/1574-6941.12355, 2014.
Konopka, A.: What is microbial community ecology, ISME J., 3, 1223–1230, https://doi.org/10.1038/ismej.2009.88, 2009.
Ladau, J., Sharpton, T. J., Finucane, M. M., Jospin, G., Kembel, S. W., O'Dwyer, J., Koeppel, A. F., Green, J. L., and Pollard, K. S.: Global marine bacterial diversity peaks at high latitudes in winter, ISME J., 7, 1669–1677, https://doi.org/10.1038/ismej.2013.37, 2013.
Langille, M. G. I., Zaneveld, J., Caporaso, J. G., McDonald, D., Knights, D., Reyes, J. A., Clemente, J. C., Burkepile, D. E., Vega Thurber, R. L., Knight, R., Beiko, R. G., and Huttenhower, C.: Predictive functional profiling of microbial communities using 16S rRNA marker gene sequences, Nat. Biotechnol., 31, 814–821, https://doi.org/10.1038/nbt.2676, 2013.
Lee, S.-Y. and Eom, Y.-B.: Analysis of Microbial Composition Associated with Freshwater and Seawater, Biomed. Sci. Lett., 22, 150–159, https://doi.org/10.15616/bsl.2016.22.4.150, 2017.
Lee-Cruz, L., Edwards, D. P., Tripathi, B. M., and Adams, J. M.: Impact of Logging and Forest Conversion to Oil Palm Plantations on Soil Bacterial Communities in Borneo, Appl. Environ. Microbiol., 79, 7290–7297, https://doi.org/10.1128/aem.02541-13, 2013.
Legendre, P. and Anderson, M. J.: Distance-Based Redundancy Analysis: Testing Multispecies Responses In Multifactorial Ecological Experiments, Ecol. Monogr., 69, 1–24, https://doi.org/10.1890/0012-9615(1999)069[0001:DBRATM]2.0.CO;2, 1999.
Lemke, M. J., Lienau, E. K., Rothe, J., Pagioro, T. A., Rosenfeld, J., and Desalle, R.: Description of freshwater bacterial assemblages from the upper Paraná river floodpulse system, Brazil, Microb. Ecol., 57, 94–103, https://doi.org/10.1007/s00248-008-9398-3, 2009.
Liao, H., Yu, K., Duan, Y., Ning, Z., Li, B., He, L., and Liu, C.: Profiling microbial communities in a watershed undergoing intensive anthropogenic activities, Sci. Total Environ., 647, 1137–1147, https://doi.org/10.1016/j.scitotenv.2018.08.103, 2019.
Lozupone, C. A. and Knight, R.: Global patterns in bacterial diversity, P. Natl. Acad. Sci. USA, 104, 11436–11440, https://doi.org/10.1073/pnas.0611525104, 2007.
Madsen, E. L.: Microorganisms and their roles in fundamental biogeochemical cycles, Curr. Opin. Biotechnol., 22, 456–464, https://doi.org/10.1016/j.copbio.2011.01.008, 2011.
Martin, P., Cherukuru, N., Tan, A. S. Y., Sanwlani, N., Mujahid, A., and Müller, M.: Distribution and cycling of terrigenous dissolved organic carbon in peatland-draining rivers and coastal waters of Sarawak, Borneo, Biogeosciences, 15, 6847–6865, https://doi.org/10.5194/bg-15-6847-2018, 2018.
Miettinen, J., Shi, C., and Liew, S. C.: Land cover distribution in the peatlands of Peninsular Malaysia, Sumatra and Borneo in 2015 with changes since 1990, Glob. Ecol. Conserv., 6, 67–78, https://doi.org/10.1016/j.gecco.2016.02.004, 2016.
Mishra, S., Lee, W. A., Hooijer, A., Reuben, S., Sudiana, I. M., Idris, A., and Swarup, S.: Microbial and metabolic profiling reveal strong influence of water table and land-use patterns on classification of degraded tropical peatlands, Biogeosciences, 11, 1727–1741, https://doi.org/10.5194/bg-11-1727-2014, 2014.
Müller-Dum, D., Warneke, T., Rixen, T., Müller, M., Baum, A., Christodoulou, A., Oakes, J., Eyre, B. D., and Notholt, J.: Impact of peatlands on carbon dioxide (CO2) emissions from the Rajang River and Estuary, Malaysia, Biogeosciences, 16, 17–32, https://doi.org/10.5194/bg-16-17-2019, 2019.
NASA: Tropical Rainfall Measuring Mission, available at: https://pmm.nasa.gov/TRMM, last access: 12 February 2019.
Newton, R. J., Jones, S. E., Eiler, A., McMahon, K. D., and Bertilsson, S.: A guide to the natural history of freshwater lake bacteria, Microbiol. Mol. Biol. Rev., 75, 14–49, https://doi.org/10.1128/MMBR.00028-10, 2011.
Noble, P. A., Bidle, K. D., and Fletcher, M.: Natural Microbial Community Compositions Compared by a Back-Propagating Neural Network and Cluster Analysis of 5S rRNA, Appl. Environ. Microbiol., 63, 1762–1770, 1997.
Nogales, B., Lanfranconi, M. P., Piña-Villalonga, J. M., and Bosch, R.: Anthropogenic perturbations in marine microbial communities, FEMS Microbiol. Rev., 35, 275–298, https://doi.org/10.1111/j.1574-6976.2010.00248.x, 2011.
Peter, H., Ylla, I., Gudasz, C., Romaní, A. M., Sabater, S., and Tranvik, L. J.: Multifunctionality and Diversity in Bacterial Biofilms, PLoS One, 6, e23225, https://doi.org/10.1371/journal.pone.0023225, 2011.
Pfennig, N.: The phototrophic bacteria and their role in the sulfur cycle, Plant Soil, 43, 1–16, https://doi.org/10.1007/BF01928472, 1975.
Pinhassi, J., Sala, M. M., Havskum, H., Peters, F., Guadayol, Ò., Malits, A., and Marrasé, C.: Changes in Bacterioplankton Composition under Different Phytoplankton Regimens, Appl. Environ. Microbiol., 70, 6753–6766, https://doi.org/10.1128/AEM.70.11.6753-6766.2004, 2004.
Quast, C., Pruesse, E., Yilmaz, P., Gerken, J., Schweer, T., Yarza, P., Peplies, J., and Glöckner, F. O.: The SILVA ribosomal RNA gene database project: Improved data processing and web-based tools, Nucleic Acids Res., 41, 590–596, https://doi.org/10.1093/nar/gks1219, 2013.
Raymond, P. A., Hartmann, J., Lauerwald, R., Sobek, S., McDonald, C., Hoover, M., Butman, D., Striegl, R., Mayorga, E., Humborg, C., Kortelainen, P., Dürr, H., Meybeck, M., Ciais, P., and Guth, P.: Global carbon dioxide emissions from inland waters, Nature, 503, 355–359, https://doi.org/10.1038/nature12760, 2013.
Read, D. S., Gweon, H. S., Bowes, M. J., Newbold, L. K., Field, D., Bailey, M. J., and Griffiths, R. I.: Catchment-scale biogeography of riverine bacterioplankton, ISME J., 9, 516–526, https://doi.org/10.1038/ismej.2014.166, 2015.
Reza, M. S., Mizusawa, N., Kumano, A., Oikawa, C., Ouchi, D., Kobiyama, A., Yamada, Y., Ikeda, Y., Ikeda, D., Ikeo, K., Sato, S., Ogata, T., Kudo, T., Jimbo, M., Yasumoto, K., Yoshitake, K., and Watabe, S.: Metagenomic analysis using 16S ribosomal RNA genes of a bacterial community in an urban stream, the Tama River, Tokyo, Fish. Sci., 84, 563–577, https://doi.org/10.1007/s12562-018-1193-6, 2018.
Sa'adi, Z., Shahid, S., Ismail, T., Chung, E. S., and Wang, X. J.: Distributional changes in rainfall and river flow in Sarawak, Malaysia, Asia-Pacific, J. Atmos. Sci., 53, 489–500, https://doi.org/10.1007/s13143-017-0051-2, 2017.
Savio, D., Sinclair, L., Ijaz, U. Z., Parajka, J., Reischer, G. H., Stadler, P., Blaschke, A. P., Blöschl, G., Mach, R. L., Kirschner, A. K. T., Farnleitner, A. H., and Eiler, A.: Bacterial diversity along a 2600 km river continuum, Environ. Microbiol., 17, 4994–5007, https://doi.org/10.1111/1462-2920.12886, 2015.
Schloss, P. D., Westcott, S. L., Ryabin, T., Hall, J. R., Hartmann, M., Hollister, E. B., Lesniewski, R. A., Oakley, B. B., Parks, D. H., Robinson, C. J., Sahl, J. W., Stres, B., Thallinger, G. G., Van Horn, D. J., and Weber, C. F.: Introducing mothur: Open-Source, Platform-Independent, Community-Supported Software for Describing and Comparing Microbial Communities, Appl. Environ. Microbiol., 75, 7537–7541, https://doi.org/10.1128/AEM.01541-09, 2009.
Shen, D., Langenheder, S., and Jürgens, K.: Dispersal modifies the diversity and composition of active bacterial communities in response to a salinity disturbance, Front. Microbiol., 9, 1–13, https://doi.org/10.3389/fmicb.2018.02188, 2018.
Sia, E. S. A., Zhang, J., Jiang, S., Zhu, Z., Carrasco, G., Holt Jang, F., Mujahid, A., and Müller, M.: Behaviour of Dissolved Phosphorus with the associated nutrients in relation to phytoplankton biomass of the Rajang River-South China Sea continuum, Biogeosciences Discuss., https://doi.org/10.5194/bg-2019-219, in review, 2019.
Silveira, C. B., Vieira, R. P., Cardoso, A. M., Paranhos, R., Albano, R. M., and Martins, O. B.: Influence of salinity on bacterioplankton communities from the Brazilian rain forest to the coastal Atlantic Ocean, PLoS One, 6, 1–9, https://doi.org/10.1371/journal.pone.0017789, 2011.
Smith, S. V. and Hollibaugh, J. T.: Coastal metabolism and the oceanic organic carbon balance, Rev. Geophys., 31, 75–89, https://doi.org/10.1029/92RG02584, 1993.
Staley, C., Unno, T., Gould, T. J., Jarvis, B., Phillips, J., Cotner, J. B., and Sadowsky, M. J.: Application of Illumina next-generation sequencing to characterize the bacterial community of the Upper Mississippi River, J. Appl. Microbiol., 115, 1147–1158, https://doi.org/10.1111/jam.12323, 2013.
Staley, C., Gould, T. J., Wang, P., Phillips, J., Cotner, J. B., and Sadowsky, M. J.: Species sorting and seasonal dynamics primarily shape bacterial communities in the Upper Mississippi River, Sci. Total Environ., 505, 435–445, https://doi.org/10.1016/j.scitotenv.2014.10.012, 2015.
Staub, J. R. and Esterle, J. S.: Provenance and sediment dispersal in the Rajang River delta/coastal plain system, Sarawak, East Malaysia, Sediment. Geol., 85, 191–201, https://doi.org/10.1016/0037-0738(93)90083-H, 1993.
They, N. H., Marins, L. F., Möller, O. O., and Abreu, P. C.: High bacterial activity in nutrient rich saltwater: Evidence from the uncoupling between salinity and nutrients in the Patos Lagoon estuary, Estuar. Coast. Shelf Sci., 216, 148–156, https://doi.org/10.1016/j.ecss.2018.09.001, 2019.
Too, C. C., Keller, A., Sickel, W., Lee, S. M., and Yule, C. M.: Microbial Community Structure in a Malaysian Tropical Peat Swamp Forest: The Influence of Tree Species and Depth, Front. Microbiol., 9, 1–13, https://doi.org/10.3389/fmicb.2018.02859, 2018.
Tripathi, B. M., Song, W., Slik, J. W. F., Sukri, R. S., Jaafar, S., Dong, K., and Adams, J. M.: Distinctive Tropical Forest Variants Have Unique Soil Microbial Communities, But Not Always Low Microbial Diversity, Front. Microbiol., 7, 376, 1–11, https://doi.org/10.3389/fmicb.2016.00376, 2016.
Vannote, R. L., Minshall, G. W., Cummins, K. W., Sedell, J. R., and Cushing, C. E.: The River Continuum Concept, Can. J. Fish. Aquat. Sci., 37, 130–137, https://doi.org/10.1139/f80-017, 1980.
Vera-Gargallo, B., Chowdhury, T. R., Brown, J., Fansler, S. J., Durán-Viseras, A., Sánchez-Porro, C., Bailey, V. L., Jansson, J. K., and Ventosa, A.: Spatial distribution of prokaryotic communities in hypersaline soils, Sci. Rep., 9, 1–12, https://doi.org/10.1038/s41598-018-38339-z, 2019.
Wang, B., Huang, F., Wu, Z., Yang, J., Fu, X., and Kikuchi, K.: Multi-scale climate variability of the South China Sea monsoon: A review, Dyn. Atmos. Ocean., 47, 15–37, https://doi.org/10.1016/j.dynatmoce.2008.09.004, 2009.
Wang, P., Clemens, S., Beaufort, L., Braconnot, P., Ganssen, G., Jian, Z., Kershaw, P., and Sarnthein, M.: Evolution and variability of the Asian monsoon system: State of the art and outstanding issues, Quaternary Sci. Rev., 24, 595–629, https://doi.org/10.1016/j.quascirev.2004.10.002, 2005.
Ward, L. M., Hemp, J., Shih, P. M., McGlynn, S. E., and Fischer, W. W.: Evolution of phototrophy in the Chloroflexi phylum driven by horizontal gene transfer, Front. Microbiol., 9, 1–16, https://doi.org/10.3389/fmicb.2018.00260, 2018.
Weller, R., Glöckner, F., and Amann, R.: 16S rRNA-Targeted Oligonucleotide Probes for the in situ Detection of Members of the Phylum Cytophaga-Flavobacterium-Bacteroides, Syst. Appl. Microbiol., 23, 107–114, 2000.
Welti, N., Striebel, M., Ulseth, A. J., Cross, W. F., DeVilbiss, S., Glibert, P. M., Guo, L., Hirst, A. G., Hood, J., Kominoski, J. S., MacNeill, K. L., Mehring, A. S., Welter, J. R., and Hillebrand, H.: Bridging food webs, ecosystem metabolism, and biogeochemistry using ecological stoichiometry theory, Front. Microbiol., 8, 1–14, https://doi.org/10.3389/fmicb.2017.01298, 2017.
Wetlands International: Flooding projections from elevation and subsidence models for oil palm plantations in Rajang Delta peatlands, Sarawak, Malaysia, Deltares, available at: https://www.deltares.nl/app/uploads/2015/06/Rajang-Delta-Peatland-Subsidence-Flooding-Deltares-2015.pdf (last access: 15 May 2019), 2015.
Wilhelm, L., Besemer, K., Fragner, L., Peter, H., Weckwerth, W., and Battin, T. J.: Altitudinal patterns of diversity and functional traits of metabolically active microorganisms in stream biofilms, ISME J., 9, 2454–2464, https://doi.org/10.1038/ismej.2015.56, 2015.
Yang, X., Xie, P., Ma, Z., Wang, Q., Fan, H., and Shen, H.: Decrease of NH4+-N by bacterioplankton accelerated the removal of cyanobacterial blooms in aerated aquatic ecosystem, J. Environ. Sci., 25, 2223–2228, https://doi.org/10.1016/S1001-0742(12)60282-4, 2013.
Yilmaz, P., Parfrey, L. W., Yarza, P., Gerken, J., Pruesse, E., Quast, C., Schweer, T., Peplies, J., Ludwig, W., and Glöckner, F. O.: The SILVA and “all-species Living Tree Project (LTP)” taxonomic frameworks, Nucleic Acids Res., 42, 643–648, https://doi.org/10.1093/nar/gkt1209, 2014.
Yule, C. M., Lim, Y. Y., and Lim, T. Y.: Degradation of Tropical Malaysian Peatlands Decreases Levels of Phenolics in Soil and in Leaves of Macaranga pruinosa, Front. Earth Sci., 4, 1–9, 2016.
Zeglin, L. H.: Stream microbial diversity in response to environmental changes: Review and synthesis of existing research, Front. Microbiol., 6, 1–15, https://doi.org/10.3389/fmicb.2015.00454, 2015.
Zhang, C., Dang, H., Azam, F., Benner, R., Legendre, L., Passow, U., Polimene, L., Robinson, C., Suttle, C. A., and Jiao, N.: Evolving paradigms in biological carbon cycling in the ocean, Natl. Sci. Rev., 5, 481–499, https://doi.org/10.1093/nsr/nwy074, 2018a.
Zhang, Y., Huang, L., Jiang, H., and Wu, G.: Hyperthermophilic Anaerobic Nitrate-Dependent Fe(II) Oxidization by Tibetan Hot Spring Microbiota and the Formation of Fe Minerals, Geomicrobiol. J., 36, 30–41, https://doi.org/10.1080/01490451.2018.1492047, 2018b.
Zhou, J., Richlen, M. L., Sehein, T. R., Kulis, D. M., Anderson, D. M., and Cai, Z.: Microbial community structure and associations during a marine dinoflagellate bloom, Front. Microbiol., 9, 1–21, https://doi.org/10.3389/fmicb.2018.01201, 2018.
Zhu, Z., Oakes, J., Eyre, B., Hao, Y., Sia, E. S. A., Jiang, S., Müller, M., and Zhang, J.: The non-conservative distribution pattern of organic matter in Rajang, a tropical river with peatland in its estuary, Biogeosciences Discuss., https://doi.org/10.5194/bg-2019-157, in review, 2019.
Zwart, G., Crump, B. C., Agterveld, M. P. K., and Hagen, F.: Typical freshwater bacteria: an analysis of available 16S rRNA gene sequences from plankton of lakes and rivers , Aquat. Microb. Ecol., 28, 141–155, 2002.