the Creative Commons Attribution 4.0 License.
the Creative Commons Attribution 4.0 License.
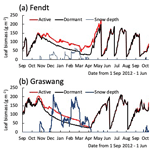
Wintertime grassland dynamics may influence belowground biomass under climate change: a model analysis
Genki Katata
Rüdiger Grote
Matthias Mauder
Matthias J. Zeeman
Masakazu Ota
Rising temperatures and changes in snow cover, as can be expected under a warmer global climate, may have large impacts on mountain grassland productivity limited by cold and long winters. Here, we combined two existing models, the multi-layer atmosphere-SOiL-VEGetation model (SOLVEG) and the BASic GRAssland model (BASGRA), which accounts for snow, freeze–thaw events, grass growth, and soil carbon balance. The model was applied to simulate the responses of managed grasslands to anomalously warm winter conditions. The grass growth module considered key ecological processes under a cold environment, such as leaf formation, elongation and death, tillering, carbon allocation, and cold acclimation, in terms of photosynthetic activity. Input parameters were derived for two pre-Alpine grassland sites in Germany, for which the model was run using 3 years of data that included a winter with an exceptionally small amount of snow. The model reproduced the temporal variability of observed daily mean heat fluxes, soil temperatures, and snow depth throughout the study period. High physiological activity levels during the extremely warm winter led to a simulated CO2 uptake of 100 gC m−2, which was mainly allocated into the belowground biomass and only to a minor extent used for additional plant growth during early spring. If these temporary dynamics are representative of long-term changes, this process, which is so far largely unaccounted for in scenario analysis using global terrestrial biosphere models, may lead to carbon accumulation in the soil and/or carbon loss from the soil as a response to global warming.
- Article
(4304 KB) -
Supplement
(327 KB) - BibTeX
- EndNote
Grasslands are important for food production as a means of fodder for livestock. There productivity strongly depends on climatic conditions and is thus expected to be highly influenced by climate change (Jing et al., 2014; Tubiello et al., 2007). Particular large changes regarding temperature and snow cover alterations are predicted to occur in high latitudes and mountainous regions (Pepin et al., 2015), leading to the expectation that mountain grassland ecosystems will be particularly affected (Xie et al., 2017). Therefore, understanding the response of mountain grassland ecosystems to snow cover conditions is crucial for estimating grassland productivity and respective impacts on carbon and energy balances under climate change.
Although forage production from grasslands is known to be limited by cold and long winters in mountainous regions, there are still uncertainties regarding the impacts of winter conditions on grassland ecology (i.e., grasses, clover, other herbaceous species, flowers, and mosses; Rapacz et al., 2014). During winter seasons, low temperatures interact with the presence or absence of snow cover in controlling the acclimation status of grassland vegetation (Ergon et al., 2018). For example, low temperature limits the productivity of grassland vegetation either directly due to its effects on photosynthesis or cell growth, or indirectly by inducing senescence and dormancy. In addition, photosynthesis and growth are prevented even under relatively mild temperatures as long as the vegetation is covered by snow. In turn, mild temperatures combined with only short snow periods enable photosynthetic activity throughout the whole winter leading to drastically increasing gross primary production (GPP), which has been observed at low-elevation grasslands (Zeeman et al., 2017). The resulting increase in carbon availability may be used for increased aboveground growth during spring, be released or put into belowground organs for storage or resource acquisition, with largely different impacts on the overall carbon cycle.
In order to assess the impacts of a changing environment, the general responses of photosynthesis and phenology to temperature and radiation have been explicitly described in common process-based grassland models (e.g., Cannell and Thornley, 1998; Soussana et al., 2012, as reviewed in Van Oijen et al., 2018), although the consideration of species-specific sensitivities may not always be adequate (Höglind et al., 2011; Tuba et al., 2008). Three major uncertainties can be depicted: the first is the representation of growth phases in phenological sub-models since photosynthesis gain and storage activation is often restricted to empirically determined periods that may considerably shift under climate change (e.g., Desai et al., 2015). The second uncertainty is related to the allocation process that distributes acquired carbon according to certain rules that may depend on growth stages but generally do not consider an environmental change of sink strength such as the limitation of cell expansion by low temperatures (Körner, 2008; Rabenhorst, 2005). Finally, acclimation processes that may lead to a relatively high photosynthesis in colder environments (Sage and Kubien, 2007) are not accounted for. Carbon production in the cold might thus lead to enhanced carbon storage rather than grass growth (Hoch and Körner, 2003; Körner, 2008). In addition, models need to have a close link to soil processes in order to quantify carbon releases from soil respiration that might also be enhanced by warmer winter temperatures (e.g., Scholz et al., 2018).
In order to improve the representation of wintertime grassland dynamics, this study focuses on the relationship of productivity responses under varying temperature and snow cover duration in mountainous areas. In contrast to most existing studies with grassland models for temperate climate conditions that focus on spring and summer seasons (Höglind et al., 2016), we investigate the effect of changing winter conditions. In particular, we differentiate between environmental limitations on photosynthesis and growth (e.g., temperature, water, and nutrient controls; Boisvenue and Running, 2006) and also consider plant internal drivers (e.g., accumulation and depletion of accumulate nonstructural carbohydrates; Kozlowski, 1992; Fatichi et al., 2014). Therefore, we apply a process-based land surface model that can simulate both physical (snow and freeze–thaw) and biological processes (carbon allocation under cold stresses) and which includes sink limitations. This integrated model is based on a multi-layer atmosphere–soil–vegetation model (SOLVEG; Katata et al., 2014), and is run at two managed grassland sites in the German pre-Alpine region. The simulation period covers a number of years that include normal (2011–2012 and 2012–2013) and extremely warm (2013–2014) winters. The results are evaluated with measurements and are discussed based on sensitivity analysis.
2.1 SOLVEG
The one-dimensional multi-layer model SOLVEG consists of four sub-models: atmosphere, soil, vegetation, and radiation within the vegetation canopy as shown in Fig. S1 in the Supplement. The general description is available in Katata (2009), Katata and Ota (2017), Nagai (2004), and Ota et al. (2013). Details of the processes of snow accumulation and melting, freeze–thaw in soil, and grassland vegetation growth and development are described in the Supplement.
In the atmosphere sub-model, one-dimensional diffusion equations are solved between atmospheric layers for horizontal wind speeds, potential temperature, specific humidity, liquid water content of fog, turbulent kinetic energy and length scale (Katata, 2009), and gas and aerosol concentrations (Katata and Ota, 2017). In upper boundary conditions, the variables of horizontal wind speeds, potential temperature, and specific humidity (and liquid water content of the fog, gas and aerosol concentrations, if available) are typically obtained from hourly or half-hourly observational data. For further explanation, see Sect. 2.3. Bulk transfer equations are applied at the lowest layer using the soil surface temperature and specific humidity calculated in the soil sub-model. In the soil sub-model, the soil temperature, volumetric soil water content, and specific humidity in the soil pores are predicted based on heat conduction, mass balance in liquid water, and water vapor diffusion equations, respectively (Katata, 2009). Root water uptake is calculated from the transpiration rate in the vegetation sub-model. For CO2 concentration in soil, mass conservation equations for liquid and gas phases are solved (Nagai, 2004). Organic matter dynamics are also considered (Ota et al., 2013), such as microbial decomposition and dissolved organic carbon (DOC) leaching in the aboveground litter layer, belowground input of carbon from roots (root litter), and soil organic carbon (SOC) turnover and DOC transport along water flows throughout the soil profile for three SOC pools (active, slow, and passive) with different turnover times.
In the vegetation sub-model, profiles of the leaf temperature, leaf surface water, and the vertical liquid water flux are predicted (Nagai, 2004). The heat budget equation at the leaf surface is solved to predict the leaf temperature using key variables from the atmosphere sub-model combined with the radiation scheme. At the upper boundary of the sub-model, the given precipitation intensity is used for calculating vertical liquid water flux within the canopy based on the surface water budget equation. The CO2 assimilation rate due to photosynthesis is predicted using Farquhar's formulations (Farquhar et al., 1980) and stomatal resistance. In the radiation sub-model, direct and diffuse downward and upward fluxes of solar and longwave radiation are calculated to obtain the radiation energy input at the canopy layers. Fractions of sunlit and shaded leaves at each canopy layer are computed for the stomatal resistance and energy budget calculations.
A multi-layer snow module is mainly developed based on the Community Land Model (CLM; Oleson et al., 2010) and SNTHERM (Jordan, 1991), while the model is unique in including the gravitational and capillary liquid water flows in the unsaturated snow layer based on van Genuchten's concept of water flow in the unsaturated zone (see Hirashima et al., 2010). In the soil module, freeze–thaw processes in soil based on the freezing-point depression equation (Zhang et al., 2007) are considered in heat conduction and liquid water flow equations.
To simulate the winter-related processes for grassland phenology such as leaf development and senescence due to cold stresses, the relevant scheme in the grass growth model named BASic GRAssland model (BASGRA; Höglind et al., 2016) is coupled with the vegetation sub-model of SOLVEG to simulate vegetation growth. The three main features that characterize plant growth in BASGRA are (1) simulation of source–sink relations where the source consists of both current photosynthesis and remobilization of reserves; (2) simulation of leaf area dynamics and tillering for vegetative and generative tillers; and (3) cold hardening and the effect of physical winter stress factors on tiller survival and plant growth. BASGRA has been well validated by using several experimental datasets of harvestable dry matter of perennial rye grass collected in Europe (Schapendonk et al., 1998) and from five locations in Norway, covering a wide range of agroclimatic regions, day lengths, and soil conditions (Höglind et al., 2016). BASGRA consists of the LINGRA grassland model (Van Oijen et al., 2005) with models for cold hardening and soil physical winter processes, while diurnal CO2 assimilation is calculated as accumulation of the net assimilation for each time step within the vegetation sub-model (Nagai, 2004) instead of the original scheme of photosynthetic processes in BASGRA. When snow covers grass, no photosynthesis is assumed to occur due to low light availability and only soil respiration is considered. BASGRA uses a so-called “big-leaf” approach (Monteith, 1981), thus predicting the total leaf area index (LAI) of the whole grassland vegetation canopies. Since SOLVEG uses a multi-layer structure of canopies, the profile of leaf area density is obtained from simply dividing total LAI by canopy height (h) by assuming vertically uniformity for all canopy layers. Canopy height, which is not simulated in BASGRA, is calculated by the function of LAI with fitting parameters.
The carbon gain from photosynthesis and remobilized reserves is allocated among sinks based on changing sink priorities and strengths. Sink strengths are calculated based on the dynamics of leaves and stems and the acclimation to low temperature. The following five sinks are considered: the processes of cold hardening, replenishment of the reserve pool, leaf growth, stem growth, and root growth. Sink strengths are defined as the rate at which these processes would proceed with no source limitation. The hardening process has top priority, so its demand is met in full if source strength is large enough, irrespective of the four other sinks. Root growth has lowest priority and depends on carbon unused by other sinks. The strength priority between reserves on the one hand, and leaves and stems on the other hand changes with day length. When day lengths are shorter than a cultivar-specific threshold, reserves have higher priority than stems and leaves, with the opposite during the rest of the year. Leaves and stems have equal priority so they receive carbon according to their sink strengths. The removal of tillers and leaves by cutting can be simulated during the growing season, with subsequent regrowth of the sward. The regrowth rate after cutting is calculated at each phenological stage. Natural turnover of leaves and roots is modeled using typical life spans in years (Arora and Boer, 2005), while BASGRA does not simulate the senescence of elongating tillers or roots. The fraction of roots in soil layers and rooting depth are modeled as a function of root biomass (Arora and Boer, 2003), which may be required to be tested at multiple biomes. Daily amounts of the dead root biomass (root litter) are used as inputs to SOC in the soil sub-model of SOLVEG.
2.2 Empirical parameterizations for cold acclimation
Although the relation between the maximum catalytic capacity of Rubisco (Vcmax) and air temperature is quite well established (e.g., Bernacchi et al., 2001; Leuning, 2002; Smith and Dukes, 2013), parameters related to photosynthesis are still uncertain (Kattge and Knorr, 2007) also for low temperature (Höglind et al., 2011). Thus, in the vegetation sub-model, we introduced the empirical factor for cold stress of grasslands, fcold, to empirically simulate the reduction of photosynthesis under low air temperature as per the following equations (see also Supplement):
where An (µmol m−2 s−1) is the net CO2 assimilation rate at each canopy layer, which is calculated by subtracting the leaf respiration rate Rd (µmol m−2 s−1) from the assimilation rate, wc (µmol m−2 s−1) is the limitation by efficiency of the photosynthetic enzyme system (Rubisco), we (µmol m−2 s−1) is the limitation by the absorbed photosynthetically active radiation (PAR), ws (µmol m−2 s−1) is the limitation by the capacity of leaves to export the products of photosynthesis, Ta (∘C) is the daily and vertical mean air temperature for all canopy layers, and Tph (∘C) is the threshold air temperature above which grasslands are photosynthetically active. Determination of the value of this threshold temperature is important to avoid the overestimation (mainly from fall to winter) of photosynthesis at a low temperature (Höglind et al., 2011). In the original BASGRA, Tph is set to 1 ∘C; that is, Vcmax starts decreasing linearly when Ta drops below 1 ∘C until it becomes zero at −4 ∘C. However, in the SOLVEG simulation, since the values of Tph may change depending on environmental conditions, the value of Tph is calibrated for each site so that the model reproduces the observed CO2 flux during the extremely warm winter period.
2.3 Study sites and observational data
The model is applied to two sites of managed grassland named Graswang (47.5708∘ N, 11.0326∘ E, 864 m a.s.l.) and Fendt (47.8329∘ N, 11.0607∘ E, 595 m a.s.l.) belonging to the TERestrial ENvironmental Observatories (TERENO) network in Germany. General information on the climate and management of the sites is available in Table 1. Both sites are located in the Bavarian Alpine Foreland, in the south of Germany and north of the Alps (Mauder et al., 2013; Zeeman et al., 2017, 2019). The grasses are harvested several times during the growing season defined as the period from April to October.
Half-hourly data of precipitation, atmospheric pressure, horizontal wind speed, air temperature, and humidity, and incoming long- and shortwave radiation were used at the top atmospheric layer as a height of 3.5 m. Data of friction velocity (u*), sensible (H) and latent heat (λE), and CO2 fluxes () observed over the grassland based on the open-path eddy covariance method using a three-dimensional sonic anemometer (CSAT3; Campbell Scientific, USA) and an open-path CO2∕H2O gas analyzer (LI-7500; Li-Cor, USA) were used for validation of the simulation results. The net radiation (Rnet) over the canopies, soil temperature at 0.05 m in depth, and snow depth were also used to evaluate the simulated surface energy and water balances. Details of the site characteristics and micrometeorological observations are described by Zeeman et al. (2017).
2.4 Calibration and validation procedure
Direct comparisons between the results using the original (SOLVEG only) and integrated models (SOLVEG coupled with BASGRA) are difficult because the vegetation dynamics had been prescribed in the original model, requiring time series of total LAI or leaf biomass data, which is used for evaluation in this study. Thus, we simply focus on the calibration of the integrated model only to investigate the impact of wintertime carbon uptake on grassland dynamics. Parameters used for SOLVEG simulations are summarized in Table 2. The study period was approximately 3 years long from 1 December 2011 to 1 November 2014, and included both normal (2011–2012 and 2012–2013) and extremely warm (2013–2014) winters. Typical values of soil hydrological parameters (e.g., saturated hydraulic conductivity) in the study area are given to SOLVEG runs from the past model study (Hingerl et al., 2016). The set of parameters of BASGRA for typical perennial grass species of timothy in the Nordic region (Höglind et al., 2016) is applied. Grass-cutting events are determined from clear reductions in the CO2 flux, surface albedo, and phenology camera observations according to Zeeman et al. (2017). The threshold temperature for cold stresses (Tph in Eq. 2) is manually determined in the simulation for each site to obtain the best agreement between simulated and measured CO2 flux over the canopy during winter. By changing the Tph value from the range between 1 and 11 ∘C with an increment of 2 ∘C (not shown in the figure), we obtained the best results as Tph=1 ∘C and 11 ∘C for Graswang and Fendt, respectively. The calibration results of daily mean surface fluxes (Rnet, H, λE, and ) are statistically evaluated using the mean error (ME), the root-mean-square error (RMSE), intercept and slope of linear regression lines, and Pearson's correlation coefficient (R).
2.5 Scenario determination for sensitivity analysis
To investigate the impact of cold acclimation of grassland vegetation on the CO2 balance and carbon allocation at mountain grassland ecosystems, two scenarios using the SOLVEG model are defined based on the experimental results of Höglind et al. (2011): “active scenario” (Tph=1 ∘C) and “dormant scenario” (Tph=11 ∘C). The former indicates that photosynthesis is active during most of the wintertime and photosynthesis takes place even at the low temperature of 1 ∘C. In contrast, the latter represents a situation where grass physiology is more or less shut down and photosynthesis ceases under the conditions of the relatively high temperature of 11 ∘C to protect from cold stresses. Both scenarios are adopted for both Graswang and Fendt, covering the same period.
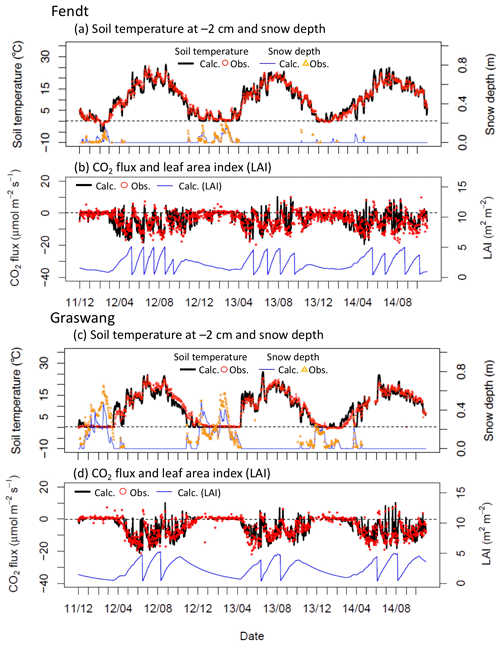
Figure 2Time series for calculated (solid lines) and observed (open symbols) (a, c) daily mean soil temperature at a depth of 0.02 m and snow depth and (b, d) CO2 flux () and leaf area index (LAI) at (a–d) Fendt and (e–h) Graswang throughout the study period. Sudden decreases in calculated LAI in (b, d) represent grass-cutting events.
3.1 Model calibration and validation
Figure 1 shows the temporal changes in simulated and observed daily surface heat fluxes over the grassland at Fendt and Graswang throughout the 3-year study period. The model generally reproduced the typical seasonal changes measured at both sites, for example, low values of the Bowen ratio (H ∕ λE) at Fendt during the growing season (from April to October) and negative sensible heat flux (H) at Fendt in December 2013, as suggested by Zeeman et al. (2017).
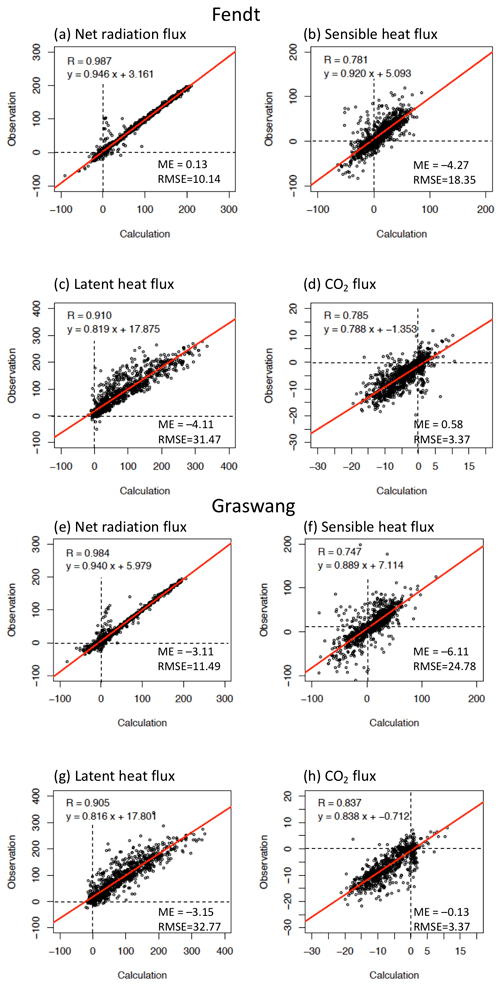
Figure 3Scatter diagrams of calculated and observed (a, e) daily mean net radiation (Rnet), (b, f) sensible (H) and (c, g) latent (λE) heat, and (d, h) CO2 fluxes () at (a–d) Fendt and (e–h) Graswang for the study period. ME (mean error) = calculations − observations; RMSE: root-mean-square error.
Figure 2 illustrates the time series of modeled and observed daily soil temperature and snow depth at the two sites. Observed changes in soil temperature and snow depth (Fig. 2a, c) were also generally reproduced by the model; for example, when the grassland was covered by the snow at Graswang from December 2012 to February 2013, soil temperature at a depth of 0.02 m remained at almost 0 ∘C in both observations and calculations (Fig. 2c). Sudden increases in soil temperature during periodical snow-free conditions were also reproduced by the model; this was particularly evident at Fendt during the extremely warm winter of 2013–2014 (Fig. 2a).
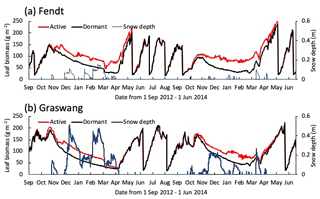
Figure 4Time series for calculated leaf biomass and snow depth (blue lines) at (a) Fendt and (b) Graswang from 1 September 2012 until 1 June 2014, in active (red lines) and dormant cases (black lines).
Simulated and observed daily CO2 fluxes () over the canopies and simulated LAI at both sites are presented in Fig. 2b. The model simulation match the observed increase of CO2 uptake due to regrowth of grassland vegetation as well as the sudden decline after harvests (Fig. 2b and d). No drought stress was apparent in the simulations at both sites during the study period (not shown). During the extremely warm winter from December 2013 to February 2014, a net release of CO2 at Fendt was also well reproduced by the model (Fig. 2b) using the calibrated value of Tph=1 ∘C (Table 2). At Graswang, both observed and simulated CO2 fluxes were very small and close to zero (Fig. 3d), which was achieved in the model by setting a high threshold temperature for cold acclimation (calibrated as Tph=11 ∘C; Table 2).
Scatter diagrams and statistical comparisons of daily energy and CO2 fluxes at the two sites throughout the study period are presented in Fig. 3. At both sites, the slopes of the regression lines were overall close to unity and values of the intercepts were sufficiently small for Rnet, H, and λE. High correlations were also observed between measured and simulated CO2 fluxes at both sites.
3.2 Sensitivity analysis
Figure 4 illustrates temporal changes in simulated snow depth and leaf biomass obtained for the active and dormant scenarios at both sites for the normal winter (2012–2013) and extremely warm winter (2013–2014). It is obvious that leaf biomass during winter is higher in the active scenarios, mostly because of a higher leaf growth at the end of the vegetation period. In addition, some minor leaf growth also occurs in the snow-free winter periods. Nevertheless, leaf biomasses converge during spring and are similar again at the first cutting event in May/June (Fig. 4b).
Figures 5 and 6 depict the cumulative GPP and ecosystem respiration (RE), mean leaf and root biomass, carbon reserve content (total stock of carbon that can be allocated to any of the plant elements such as leaves, stems, and roots), and LAI, simulated for Fendt and Graswang during winter and spring in 2014, respectively. In the following, we focus on Fendt for illustration of the scenario differences (Fig. 5) but would like to emphasize that the responses are similar at both sites. The differences in absolute values, especially a smaller LAI and less biomass are due to the generally cooler conditions at the Graswang site. It should be noted that such differences in environmental conditions between the sites required the calibration of the value of Tph for each site (Sect. 2.2). Both GPP and RE were higher in the active scenario than in the dormant one as expected by the model construction (Fig. 5a and b); this was particularly apparent as cumulative GPP differed by a factor of 3 or by approximately 100 gC m−2 yr−1 (Fig. 5a). Changes in leaf biomass and LAI during the subsequent spring in the active scenario were slightly lower than in the dormant scenario, indicating that a small part of the additional GPP has been used for foliage growth (Fig. 5c and f). In contrast, changes in root biomass (below ground) both during winter and spring in the active scenario were approximately 3 times higher than in the dormant scenario (Fig. 5d). Simulated carbon reserve contents in both winter and spring were similar in the two simulation scenarios (Fig. 5e), showing that the carbon fixed by photosynthesis was immediately allocated to the above- or belowground biomass.
The results demonstrate that the modified SOLVEG model that considers the major physical (snow and freeze–thaw) and biological processes (carbon allocation under cold stresses) can reasonably simulate heat and carbon transfer processes in managed grassland ecosystems (Figs. 1–3). In particular, the model with calibrated Tph values reproduced the low CO2 uptake during the normal winter period at Graswang as a response to low soil temperatures that limit photosynthesis even throughout the snow-free conditions (Fig. 2d). On the other hand, it was also possible to represent the observed high uptake of CO2 at Fendt in the extremely warm winter with the model (Fig. 2b). The key parameter that determined the above CO2 uptake processes was the threshold air temperature of Tph in Eq. (2) for the photosynthetic activity level of grassland ecosystems. Tuning of the above parameter is required for each site to simulate carbon dynamics in the grassland ecosystems in cold climate regions. In future applications, dependencies between this parameter and the environment or species composition could be evaluated so that it does not need to be fitted anymore. This will require more comprehensive datasets with which also the importance of underlying processes can be revealed and model calibration can be carried out, possibly using an optimization procedure such as Monte Carlo simulation (e.g., Van Oijen et al., 2005).
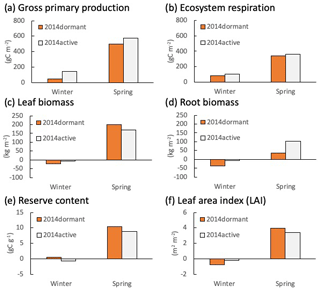
Figure 5Changes in calculated (a) gross primary production (GPP), (b) ecosystem respiration, (c) live leaf and (d) root biomasses, (e) reserve content, and (f) leaf area index (LAI) at Fendt during the winter (from December to February) and spring (from March to May) in 2014 in active (grey bars) and dormant cases (orange bars).
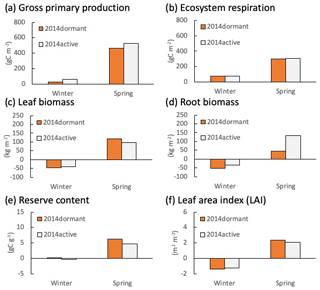
Figure 6Changes in calculated (a) gross primary production (GPP), (b) ecosystem respiration, (c) live leaf and (d) root biomasses, (e) reserve content, and (f) leaf area index (LAI) at Graswang during the winter (from December to February) and spring (from March to May) in 2014 in active (grey bars) and dormant cases (orange bars).
Our approach uses the manually calibrated Tph values for each site, while only typical (average) values are taken for different plant functional types of grassland vegetation in global biogeochemical models. Numerical experiments using Tph=1 ∘C revealed that the high CO2 uptake rate at low altitude during winter was likely explained by high levels of physiological activity of grasslands (Fig. 4a). In this experiment, the impact of cold acclimation on the CO2 balance for the two pre-Alpine temperate grassland sites was evaluated by manually tuning the threshold temperature of photosynthesis to lower (Tph=1 ∘C) and higher values (Tph=11 ∘C) because the exact mechanism of model response to Tph changes is unclear (Höglind et al., 2011). Such a down-regulation of photosynthesis is justified by numerous observations about acclimation responses particularly after exposure to freezing temperatures (e.g., Huner et al., 1993; Kolari et al., 2007). In fact, the Graswang site was exposed to frost even during the extremely warm winter in 2013–2014 (Zeeman et al., 2017). In our simulations, we treated these acclimation responses as a parameter change, although in future developments they might be described mechanistically depending on temperature development (Kumarathunge et al., 2019; Mediavilla et al., 2016). Other mechanisms are however, already implicitly considered in the photosynthesis model. For example, the limitation of photosynthesis and thus the optimum temperature shifts under low air temperature from electron-transport-limited to Rubisco-limited (Sage and Kubien, 2007). Further observational work is required at various grassland ecosystems in order to evaluate this hypothesis.
The high CO2 uptake rate during snow-free conditions was not limited to the Fendt site, but is likely a widespread phenomenon in other mountain grasslands in Europe. This statement is supported by Table 1, which summarizes full-year observational studies that include wintertime CO2 flux at European mountains. Indeed, except for the Austrian site of Rotholz, which has a long grazing period that may intensively reduce grass productivity (Wohlfahrt et al., 2010), high CO2 uptake during snow-free periods was observed at all altitudes below 760 m, corresponding to annual mean air temperature (MAT) of more than 8 ∘C. If the altitude or MAT is considered as a threshold of cold acclimation of grasses, the snow-free wintertime CO2 uptake may have a large impact on the carbon balance of grassland ecosystems over the European Alps. Since a rise in the snowline and wintertime air temperature of up to 300–600 m and 2–4 ∘C, respectively, has been predicted for the latter part of the 21st century, the effect is likely to even increase (Gobiet et al., 2014). It should be noted, however, that other indicators of the level of cold acclimation might be superior to the use of MAT because physiological activities of grassland vegetation are often triggered by temperatures during specific development stages. If, however, such activities are rather closely related to the MAT (as indicated in Table 1), it is also possible that the differences in phenology and photosynthesis are caused by a different species composition of grasslands. In this case, acclimation speed and management options that facilitate a change towards better adapted ecosystems should be investigated.
The comparison between scenarios shows that root biomass clearly increased in the active simulation compared to the dormant simulation during winter and spring (Fig. 5c and d), which can only be due to CO2 gain by photosynthesis during this time (Fig. 5a). Note that these somewhat counter-intuitive results may be due to the inability of the model to grow specific storage organs that could later be emptied to grow other tissues. Differentiation, however, is not yet possible because relevant observational evidence is not available. Most studies of Alpine grassland ecosystems in Europe have focused on the impact of climate changes on grass yield (i.e., grassland-based food production); for example, in the Nordic region, future CO2 increase, warming, and less snowfall are expected to increase grassland productivity (Ergon et al., 2018). According to this study, CO2 uptake at the Fendt site, estimated as an annual GPP of 100 gC m−2 in 2013–2014, was mainly due to the higher wintertime photosynthetic rate in the active scenario. Thus, it could be assumed that the increase in the aboveground biomass in spring would be higher in the active scenario. However, the aboveground biomass at the first cutting simulated in the active scenario was similar that in the dormant scenario (Fig. 5c). Still, the behavior is consistent with the simulated carbon reserve contents (a potential of carbon allocation to the aboveground biomass) in winter, which were similar in the two simulation scenarios (Fig. 5e). The actual limitation might have internal (e.g., determined growth) or external causes. For example, self-shading could result in the decreasing carbon gain efficiency of new leaves, which might induce a stop to growth or an increase in senescence when the canopy becomes denser. Indeed, calculated LAI values were similar to critical values for self-shading shortly before the first cutting event in 2014 (Fig. 4), which is however not a process considered in the model and is thus the result of a reasonably parametrized determined growth. This is corroborated by a similar degree of leaf senescence in both scenarios (6.9 and −0.7 % at Fendt and Graswang, respectively). These results indicate that grass yield cannot be simply determined by the source-strength (CO2 assimilation due to photosynthesis) and is controlled by the sink demand of the aboveground biomass (foliar, tiller, and stem growth). Indeed, an open-top-chamber warming experiment in the alpine steppe on the north Tibetan Plateau showed that warming significantly increased total root biomass by 28 % at a soil depth of 0–0.01 m in the growing season (Ma et al., 2016), supporting the possibility of larger belowground allocation of organic carbon, as suggested by this study. Therefore, the increased photosynthesis in the warmer winter does not necessarily increase grass yields and thus fodder in mountainous regions. In order to quantify the impact on livestock supply, further research needs to investigate to which degree additional biomass is directed into above- and belowground storages.
Another important implication from our numerical experiments is that carbon stock in/loss from the soil in mountain grasslands may be greater in a warmer future climate. The root biomass simulated for the active scenario was 3 times greater than that for the dormant scenario (Fig. 5d), indicating that more carbon is accumulated in roots, storage organs, or in the soil due to higher input by root litter in warmer winters. Indeed, recent studies suggest that a relatively high MAT accelerates the turnover of roots to produce root litter input in managed mountain grassland ecosystems (Leifeld et al., 2015). This change in the belowground input of carbon in grassland ecosystems is particularly important for the carbon cycle at managed grassland ecosystems because plant-fixed carbon from the aboveground biomass is substantially reduced following cutting. Furthermore, this may enhance carbon loss from the soil due to heterotrophic respiration and leaching of CO2 because grassland vegetation typically has a high density of fine roots that are poorly lignified and with high turnover rates, providing a relatively labile carbon substrate for microbial activity (Garcia-Pausas et al., 2017). The altered SOC dynamics in grassland ecosystems may be of considerable importance for the global carbon cycle since soils of temperate grassland ecosystems are already estimated to hold a large stock of carbon, 7 % of total global soil carbon (Jobbágy and Jackson, 2000). Therefore, we suggest that global terrestrial biosphere models (as reviewed by Fatichi et al., 2019) need to be elaborated with phenological and acclimation processes such as interactions with belowground processes (Gill et al., 2002; Riedo et al., 1998; Soussana et al., 2012) in order to estimate the carbon balance response of managed grassland ecosystems to global warming.
The output data from this study are publicly accessible via contacting the first author.
The supplement related to this article is available online at: https://doi.org/10.5194/bg-17-1071-2020-supplement.
GK developed the model with support from RG and MO, and performed the simulations using the data collected by MM and MJZ. GK prepared the manuscript with contributions from all co-authors.
The authors declare that they have no conflict of interest.
We thank the staff of KIT/IMK-IFU in Germany and of ICAS in Japan for their support. We also express our gratitude to Georg Wohlfahrt of the University of Innsbruck, Austria; Jun Koarashi of the JAEA, Japan; Kentaro Takagi of Hokkaido University, Japan; and Ankur Desai of University of Wisconsin for their helpful comments and suggestions on this study. The German weather data were provided by DWD. Fortran code of BASGRA was provided from https://zenodo.org/record/27867#.XjqoFWbTW70 (last access: 14 February 2020).
This study was supported by the German Research Foundation (grant no. ZE1006/2-1) and the Postdoctoral Fellowship for Research Abroad and Leading Initiative for Excellent Young Researchers, (Japan Society for the Promotion of Science, and the Ministry of Education, Culture, Sports, Science and Technology). The TERENO pre-Alpine infrastructure is funded by the Helmholtz Association and the Federal Ministry of Education and Research.
This paper was edited by Paul Stoy and reviewed by two anonymous referees.
Ammann, C., Spirig, C., Leifeld, J., and Neftel, A.: Assessment of the nitrogen and carbon budget of two managed temperate grassland fields, Agric. Ecosyst. Environ., 133, 150–162, https://doi.org/10.1016/j.agee.2009.05.006, 2009.
Arora, V. K. and Boer, G. J.: A representation of variable root distribution in dynamic vegetation models, Earth Interact., 7, 1–19, https://doi.org/10.1175/1087-3562(2003)007<0001:arovrd>2.0.co;2, 2003.
Arora, V. K. and Boer, G. J.: A parameterization of leaf phenology for the terrestrial ecosystem component of climate models, Global Change Biol., 11, 39–59, https://doi.org/10.1111/j.1365-2486.2004.00890.x, 2005.
Bernacchi, C. J., Singsaas, E. L., Pimentel, C., Portis Jr., A. R., and Long, S. P.: Improved temperature response functions for models of Rubisco-limited photosynthesis, Plant Cell Environ., 24, 253–259, https://doi.org/10.1111/j.1365-3040.2001.00668.x, 2001.
Boisvenue, C. and Running, S. W.: Impacts of climate change on natural forest productivity – evidence since the middle of the 20th century, Global Change Biol., 12, 862–882, https://doi.org/10.1111/j.1365-2486.2006.01134.x, 2006.
Cannell, M. G. R., and Thornley, J. H. M.: N-poor ecosystems may respond more to elevated CO2 than N-rich ones in the long term. A model analysis of grassland, Global Change Biol., 4, 431–442, https://doi.org/10.1046/j.1365-2486.1998.00167.x, 1998.
Desai, A. R., Wohlfahrt, G., Zeeman, M. J., Katata, G., Eugster, W., Montagnani, L., Gianelle, D., Mauder, M., and Schmid, H. P.: Montane ecosystem productivity responds more to global circulation patterns than climatic trends, Environ. Res. Lett., 11, 024013, https://doi.org/10.1088/1748-9326/11/2/024013, 2015.
Ergon, Å., Seddaiu, G., Korhonen, P., Virkajärvi, P., Bellocchi, G., Jørgensen, M., Østrem, L., Reheul, D., and Volaire, F.: How can forage production in Nordic and Mediterranean Europe adapt to the challenges and opportunities arising from climate change?, Eur. J. Agron., 92, 97–106, https://doi.org/10.1016/j.eja.2017.09.016, 2018.
Essery, R., Morin, S., Lejeune, Y., and Menard, C.: A comparison of 1701 snow models using observations from an alpine site, Adv. Water Res., 55, 131–148, https://doi.org/10.1016/j.advwatres.2012.07.013, 2013.
Fatichi, S., Leuzinger, S., and Körner, C.: Moving beyond photosynthesis: from carbon source to sink-driven vegetation modeling, New Phytol., 201, 1086–1095, https://doi.org/10.1111/nph.12614, 2014.
Fatichi, S., Pappas, C., Zscheischler, J., and Leuzinger, S.: Modelling carbon sources and sinks in terrestrial vegetation, New Phytol., 221, 652–668, https://doi.org/10.1111/nph.15451, 2019.
Galvagno, M., Wohlfahrt, G., Cremonese, E., Rossini, M., Colombo, R., Filippa, G., Julitta, T., Manca, G., Siniscalco, C., di Cella, U. M., and Migliavacca, M.: Phenology and carbon dioxide source/sink strength of a subalpine grassland in response to an exceptionally short snow season, Environ. Res. Lett., 8, 025008, https://doi.org/10.1088/1748-9326/8/2/025008, 2013.
Garcia-Pausas, J., Romanyà, J., Montanè, F., Rios, A. I., Taull, M., Rovira, P., and Casals, P.: Are soil carbon stocks in mountain grasslands compromised by land-use changes? edited by: Catalan, J., Ninot, J. M., Aniz, M., High Mountain Conservation in a Changing World, Adv. Global Change Res., 207–230, https://doi.org/10.1007/978-3-319-55982-7_9, 2017.
Gill, R. A., Kelly, R. H., Parton, W. J., Day, K. A., Jackson, R. B., Morgan, J. A., Scurlock, J. M. O., Tieszen, L. L., Castle, J. V., Ojima, D. S., and Zhang, X. S.: Using simple environmental variables to estimate belowground productivity in grasslands, Glob. Ecol. Biogeogr., 11, 79–86, https://doi.org/10.1046/j.1466-822x.2001.00267.x, 2002.
Gobiet, A., Kotlarski, S., Beniston, M., Heinrich, G., Rajczak, J., and Stoffel, M.: 21st century climate change in the European Alps – a review, Sci. Total Environ., 493, 1138–1151, https://doi.org/10.1016/j.scitotenv.2013.07.050, 2014.
Hingerl, L., Kunstmann, H., Wagner, S., Mauder, M., Bliefernicht, J., and Rigon, R.: Spatio-temporal variability of water and energy fluxes? a case study for a mesoscale catchment in pre-alpine environment, Hydrol. Process, 30, 3804–3823, https://doi.org/10.1002/hyp.10893, 2016.
Hirashima, H., Yamaguchi, S., Sati, A., and Lehning, M.: Numerical modeling of liquid water movement through layered snow based on new measurements of the water retention curve, Cold Reg. Sci. Technol., 64, 94–103, https://doi.org/10.1016/j.coldregions.2010.09.003, 2010.
Hoch, G. and Körner, C.: The carbon charging of pines at the climatic treeline: a global comparison, Oecologia, 135, 10–21, https://doi.org/10.1007/s00442-002-1154-7, 2003.
Höglind, M., Hanslin, H. M., and Mortensen, L. M.: Photosynthesis of Lolium perenne L. at low temperatures under low irradiances, J. Exp. Bot., 70, 297–304, https://doi.org/10.1016/j.envexpbot.2010.10.007, 2011.
Höglind, M., Van Oijen, M., Cameron, D., and Persson, T.: Process-based simulation of growth and overwintering of grassland using the BASGRA model, Ecol. Model., 335, 1–15, https://doi.org/10.1016/j.ecolmodel.2016.04.024, 2016.
Huner, N. P. A., Öquist, G., Hurry, V. M., Krol, M., Falk, S., and Griffith, M.: Photosynthesis, photoinhibition and low temperature acclimation in cold tolerant plants, Photosynthesis. Res., 37, 19–39, https://doi.org/10.1007/bf02185436, 1993.
Jing, Q., Bélanger, G., Qian, B., and Baron, V.: Timothy yield and nutritive value with a three-harvest system under the projected future climate in Canada, Can. J. Plant Sci., 94, 213–222, https://doi.org/10.4141/cjps2013-279, 2014.
Jobbágy, E. G. and Jackson, R. B.: The vertical distribution of soil organic carbon and its relation to climate and vegetation, Ecol. Appl., 10, 423–436, https://doi.org/10.2307/2641104, 2000.
Jordan, R.: A one-dimensional temperature model for a snow cover. Technical documentation for SNTHERM.89, CRREL Special Report 91-16; US Army Core of Engineers Cold Regions Research and Engineering Laboratory, Hanover, NH, 49 pp., 1991.
Katata, G.: Improvement of a land surface model for accurate prediction of surface energy and water balances, JAEA-Data/Code, 2008-033, 64, 2009.
Katata, G. and Ota, M.: A terrestrial ecosystem model (SOLVEG) coupled with atmospheric gas and aerosol exchange processes, JAEA-Data/Code, 2016-014, 35, 2017.
Katata, G., Kajino, M., Matsuda, K., Takahashi, A., and Nakaya, K.: A numerical study of the effects of aerosol hygroscopic properties to dry deposition on a broad-leaved forest, Atmos. Environ., 97, 501–510, https://doi.org/10.1016/j.atmosenv.2013.11.028, 2014.
Kattge, J. and Knorr, W.: The temperature dependence of photosynthetic capacity in a photosynthesis model acclimates to plant growth temperature: a re-analysis of data from 36 species, Plant Cell Environ., 30, 1176–1190, https://doi.org/10.1111/j.1365-3040.2007.01690_x, 2007.
Kolari, P., Lappalainen, H. K., Hänninen, H., and Hari, P.: Relationship between temperature and the seasonal course of photosynthesis in Scots pine at northern timberline and in southern boreal zone, Tellus B, 3, 542–552, https://doi.org/10.3402/tellusb.v59i3.17033, 2007.
Körner, C.: Winter crop growth at low temperature may hold the answer for alpine treeline formation, Plant Ecol. Divers., 1, 3–11, https://doi.org/10.1080/17550870802273411, 2008.
Kozlowski, T. T.: Carbohydrate sources and sinks in woody plants, Bot. Rev., 58, 107–122, https://doi.org/10.1007/BF02858600, 1992.
Kumarathunge, D. P., Medlyn, B. E., Drake, J. E., Tjoelker, M. G., Aspinwall, M. J., Battaglia, M., Cano, F. J., Carter, K. R., Cavaleri, M. A., Cernusak, L. A., Chambers, J. Q., Crous, K. Y., De Kauwe, M. G., Dillaway, D. N., Dreyer, E., Ellsworth, D. S., Ghannoum, O., Han, Q., Hikosaka, K., Jensen, A. M., Kelly, J. W. G., Kruger, E. L., Mercado, L. M., Onoda, Y., Reich, P. B., Rogers, A., Slot, M., Smith, N. G., Tarvainen, L., Tissue, D. T., Togashi, H. F., Tribuzy, E. S., Uddling, J., Vårhammar, A., Wallin, G., Warren, J. M., and Way, D. A.: Acclimation and adaptation components of the temperature dependence of plant photosynthesis at the global scale, New Phytol., 222, 768–784, https://doi.org/10.1111/nph.15668, 2019.
Leifeld, J., Meyer, S., Budge, K., Sebastia, M. T., Zimmermann, M., and Fuhrer, J. :Turnover of grassland roots in mountain ecosystems revealed by their radiocarbon signature: Role of temperature and management, PLOS ONE, 10, e0119184, https://doi.org/10.1371/journal.pone.0119184, 2015.
Leuning, R.: Temperature dependence of two parameters in a photosynthesis model, Plant Cell Environ., 25, 1205–1210, https://doi.org/10.1046/j.1365-3040.2002.00898.x, 2002.
Ma, X.-X., Yan, Y., Hong, J.-T., Lu, X.-Y., and Wang, X.-D.: Impacts of warming on root biomass allocation in alpine steppe on the north Tibetan Plateau, J. Mt. Sci., 14, 25, 1615–1623, https://doi.org/10.1007/s11629-016-3966-7, 2017.
Marcolla, B., Cescatti, A., Manca, G., Zorer, R., Cavagna, M., Fiora, A., Gianelle, D., Rodeghiero, M., Sottocornola, M., and Zampedri, R.: Climatic controls and ecosystem responses drive the inter-annual variability of the net ecosystem exchange of an alpine meadow, Agr. Forest Meteorol., 151, 1233–1243, https://doi.org/10.1016/j.agrformet.2011.04.015, 2011.
Mauder, M., Cuntz, M., Drüe, C., Graf, A., Rebmann, C., Schmid, H. P., Schmidt, M., and Steinbrecher, R.: A strategy for quality and uncertainty assessment of long-term eddy-covariance measurements, Agr. Forest Meteorol., 169, 122–135, https://doi.org/10.1016/j.agrformet.2012.09.006, 2013.
Mediavilla, S., González-Zurdo, P., Babiano, J., and Escudero, A.: Responses of photosynthetic parameters to differences in winter temperatures throughout a temperature gradient in two evergreen tree species, Eur. J. Forest Res., 135, 871–883, https://doi.org/10.1007/s10342-016-0980-9, 2016.
Merbold, L., Steinlin, C., and Hagedorn, F.: Winter greenhouse gas fluxes (CO2, CH4 and N2O) from a subalpine grassland, Biogeosciences, 10, 3185–3203, https://doi.org/10.5194/bg-10-3185-2013, 2013.
Monteith, J. L.: Evaporation and Surface-Temperature, Q. J. Roy. Meteor. Soc., 107, 1–27, https://doi.org/10.1002/qj.49710745102, 1981.
Nagai, H.: Atmosphere-soil-vegetation model including CO2 exchange processes: SOLVEG2, JAERI-Data/Code, 2004-014, 2004.
Oleson, K. W., Lawrence, D. M., Bonan, G. B., Flanner, M. G., Kluzek, E., Lawrence, P. J., Levis, S., Swenson, S. C., and Thornton, P. E.: Technical description of version 4.0 of the Community Land Model (CLM), NCAR Technical Note NCAR/TN-461+STR, National Center for Atmospheric Research (NCAR), Boulder, CO, 257, https://doi.org/10.5065/D6FB50WZ, 2010.
Ota, M., Nagai, H., and Koarashi, J.: Root and dissolved organic carbon controls on subsurface soil carbon dynamics: A model approach, J. Geophys. Res., 118, 1646–1659, https://doi.org/10.1002/2013jg002379, 2013.
Pepin, N., Bradley, R. S., Diaz, H. F., Baraer, M., Caceres, E. B., Forsythe, N., Fowler, H., Greenwood, G., Hashmi, M. .Z., Liu, X. D., Miller, J. R., Ning, L., Ohmura, A., Palazzi, E., Rangwala, I., Schöner, W., Severskiy, I., Shahgedanova, M., Wang, M. B., Williamson, S. N., and Yang, D. Q.: Elevation-dependent warming in mountain regions of the world, Nat. Clim. Chang., 5, 424–430, https://doi.org/10.1038/nclimate2563, 2015
Rabenhorst, M. C.: Biologic zero: a soil temperature concept, Wetlands, 25, 616–621, https://doi.org/10.1672/0277-5212(2005)025[0616:BZASTC]2.0.CO;2, 2005.
Rapacz, M., Ergon, Å., Höglind, M., Jørgensen, M., Jurczyk, B., Østrem, L., Rognli, O. A., and Tronsmo, A. M.: Overwintering of herbaceous plants in a changing climate, Still more questions than answers, Plant Sci., 225, 34-44, https://doi.org/10.1016/j.plantsci.2014.05.009, 2014.
Riedo, M., Grub, A., Rosset, M., and Fuhrer, J.: A pasture simulation model for dry matter production, and fluxes of carbon, nitrogen, water and energy, Ecol. Model., 105, 141–183, https://doi.org/10.1016/s0304-3800(97)00110-5, 1998.
Rogiers, N., Eugster, W., Furger, M., and Siegwolf, R.: Effect of land management on ecosystem carbon fluxes at a subalpine grassland site in the Swiss Alps, Theor. Appl. Climatol., 80, 187–203, https://doi.org/10.1007/s00704-004-0099-7, 2005.
Sage, R. F. and Kubien, D. S.: The temperature response of C3 and C4 photosynthesis, Plant Cell Environ., 30, 1086–1106, https://doi.org/10.1111/j.1365-3040.2007.01682.x, 2007.
Schapendonk, A. H. M. C., Stol, W., van Kraalingen, D. W. G., and Bouman, B. A. M.: LINGRA – a source/sink model to simulate grassland productivity in Europe, Eur. J. Agron., 9, 87–100, https://doi.org/10.1016/s1161-0301(98)00027-6, 1998.
Scholz, K., Hammerle, A., Hiltbrunner, E., and Wohlfahrt, G.: Analyzing the effects of growing season length on the net ecosystem production of an Alpine grassland using model-data fusion, Ecosystems, 21, 982–999, https://doi.org/10.1007/s10021-017-0201-5, 2018.
Smith, N. G. and Dukes, J. S.: Plant respiration and photosynthesis in global-scale models: incorporating acclimation to temperature and CO2, Glob. Change Biol., 19, 45–63, https://doi.org/10.1111/j.1365-2486.2012.02797.x, 2013.
Soussana, J. F., Maire, V., Gross, N., Bachelet, B., Pages, L., Martin, R., Hill, D., and Wirth, C.: Gemini: A grassland model simulating the role of plant traits for community dynamics and ecosystem functioning. Parameterization and evaluation, Ecol. Model., 231, 134–145, https://doi.org/10.1016/j.ecolmodel.2012.02.002, 2012.
Tuba, Z., Csintalan, Z., Szente, K., Nagy, Z., Fekete, G., Larcher, W., and Lichtenthaler, H. K.: Winter photosynthetic activity of twenty temperate semi-desert sand grassland species, J. Plant Physiol., 165, 1438–1454, https://doi.org/10.1016/j.jplph.2007.10.017, 2008.
Tubiello, F. N., Soussana, J.-F., and Howden, S. M.: Crop and pasture response to climate change, P. Natl. Acad. Sci. USA, 104, 19686–19690, https://doi.org/10.1073/pnas.0701728104, 2007.
Van Oijen, M., Höglind, M., Hanslin, M. H., and Caldwell, N.: Process-based modeling of timothy regrowth, Agron. J., 97, 1295–1303, https://doi.org/10.2134/agronj2004.0251, 2005.
Van Oijen, M., Bellocchi, G., and Höglind, M.: Effects of climate change on grassland biodiversity and productivity: The need for a diversity of models, Agron., 8, 14 https://doi.org/10.3390/agronomy8020014, 2018.
Wohlfahrt, G., Bahn, M., Tappeiner, U., and Cernusca, A.: A multi-component, multi-species model of vegetation-atmosphere CO2 and energy exchange for mountain grasslands, Agr. Forest Meteorol., 106, 261–287, https://doi.org/10.1016/s0168-1923(00)00224-0, 2001.
Wohlfahrt, G., Hammerle, A., Haslwanter, A., Bahn, M., Tappeiner, U., and Cernusca, A.: Seasonal and inter-annual variability of the net ecosystem CO2 exchange of a temperate mountain grassland: Effects of weather and management, J. Geophys. Res., 113, D08110, https://doi.org/10.1029/2007jd009286, 2008.
Wohlfahrt, G., Pilloni, S., Hörtnagl, L., and Hammerle, A.: Estimating carbon dioxide fluxes from temperate mountain grasslands using broad-band vegetation indices, Biogeosciences, 7, 683–694, https://doi.org/10.5194/bg-7-683-2010, 2010.
Xie, J., Kneubuhler, M., Garonna, I., Notarnicola, C., De Gregorio, L., De Jong, R., Chimani, B., and Schaepman, M. E.: Altitude-dependent influence of snow cover on alpine land surface phenology, J. Geophys. Res., 122, 1107–1122, https://doi.org/10.1002/2016jg003728, 2017.
Zeeman, M. J., Hiller, R., Gilgen, A. K., Michna, P., Pluss, P., Buchmann, N., and Eugster, W.: Management and climate impacts on net CO2 fluxes and carbon budgets of three grasslands along an elevational gradient in Switzerland, Agr. Forest Meteorol., 150, 519–530, https://doi.org/10.1016/j.agrformet.2010.01.011, 2010.
Zeeman, M. J., Mauder, M., Steinbrecher, R., Heidbach, K., Eckart, E., and Schmid, H. P.: Reduced snow cover affects productivity of upland temperate grasslands, Agr. Forest Meteorol., 232, 514–526, https://doi.org/10.1016/j.agrformet.2016.09.002, 2017.
Zeeman, M. J., Shupe, H., Baessler, C., and Ruehr, N. K.: Productivity and vegetation structure of three differently managed temperate grasslands, Agric. Ecosyst. Environ., 270, 129–148, https://doi.org/10.1016/j.agee.2018.10.003, 2019.
Zhang, X., Sun, S., and Xue, Y.: Development and testing of a frozen soil parameterization for cold region studies, J. Hydrometeorol., 8, 690–701, https://doi.org/10.1175/jhm605.1, 2007.