the Creative Commons Attribution 4.0 License.
the Creative Commons Attribution 4.0 License.
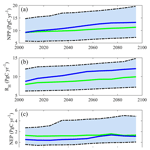
Microbial dormancy and its impacts on northern temperate and boreal terrestrial ecosystem carbon budget
Junrong Zha
A large amount of soil carbon in northern temperate and boreal regions could be emitted as greenhouse gases in a warming future. However, lacking detailed microbial processes such as microbial dormancy in current biogeochemistry models might have biased the quantification of the regional carbon dynamics. Here the effect of microbial dormancy was incorporated into a biogeochemistry model to improve the quantification for the last century and this century. Compared with the previous model without considering the microbial dormancy, the new model estimated the regional soils stored 75.9 Pg more C in the terrestrial ecosystems during the last century and will store 50.4 and 125.2 Pg more C under the RCP8.5 and RCP2.6 scenarios, respectively, in this century. This study highlights the importance of the representation of microbial dormancy in earth system models to adequately quantify the carbon dynamics in the northern temperate and boreal natural terrestrial ecosystems.
The land ecosystems in northern temperate and boreal regions (>45∘ N) occupy 22 % of the global surface and store over 40 % of the global soil organic carbon (SOC) (McGuire and Hobbie, 1997; Melillo et al., 1993; Tarnocai et al., 2009; Hugelius et al., 2014). During the past decades, a greening accompanying a warming in the region has been documented (Zhou et al., 2001; Lloyd et al., 2002; Stow et al., 2004; Callaghan et al., 2005; Tape et al., 2006). The regional carbon dynamics are expected to loom large in the global carbon cycle and exert large feedbacks to the global climate system (McGuire et al., 2009; Davidson and Janssens, 2006; Bond-Lamberty and Thomson, 2010).
To date, numerous ecosystem models have been developed to project the feedbacks between terrestrial ecosystem carbon cycling and climate (Raich et al., 1991; Zhuang et al., 2001, 2002, 2015; Parton et al., 1993; Knorr et al., 2005; Running and Coughlan, 1988), but they can bias their quantifications due to missing detailed microbial mechanisms in these models (Schmidt et al., 2011; Todd-Brown et al., 2013; Conant et al., 2011; Treseder et al., 2011). Microorganisms play a central role in decomposition of litter and soil organic carbon, which further governs the global carbon cycling and climate change (Xu et al., 2014; Treseder et al., 2011; Wang et al., 2015). An emerging field of research has begun to incorporate microbial ecology into existing process-based models to represent decomposition in ways that include important microbial processes that were previously ignored (Zha and Zhuang, 2018; Schimel and Weintraub, 2003; Allison et al., 2010; German et al., 2012). These microbe-based models tend to better reproduce field and satellite observations than traditional ones that treat soil decomposition as a first-order decay process without considering microbial activities (Treseder et al., 2011; Wieder et al., 2013; Todd-Brown et al., 2011; Lawrence et al., 2009; Moorhead and Sinsabaugh, 2006). However, some vital microbial traits such as microbial dormancy and community shifts are still rarely explicitly considered in large-scale ecosystem models (Wieder et al., 2015), and this may introduce notable uncertainties (Graham et al., 2014, 2016; Wang et al., 2015; Bouskill et al., 2012; Kaiser et al., 2014).
Dormancy is broadly recognized as a strategy for microorganisms to cope with periodical environmental stresses (Harder and Dijkhuizen, 1983). When environmental conditions are unfavorable for growth, microbes switch to a dormant state, which is a reversible state of low to zero metabolic activity (Stolpovsky et al., 2011; Lennon and Jones, 2011). In this state, biogeochemical processes such as soil decomposition are slow (Blagodatskaya and Kuzyakov, 2013). At any given time, there is only a fraction of, likely below 50 %, metabolically active microbes in natural soils (Wang et al., 2015; Stolpovsky et al., 2011). Soil decomposition and nutrient cycling mainly depend on these active microbes because only active ones can consume organic matter and replicate themselves (Wang et al., 2015; Blagodatskaya et al., 2014). To date, most existing biogeochemistry models use total rather than active microbial biomass as an indicator of microbial activities (Wieder et al., 2015), which could bias the estimates of soil decomposition and ecosystem carbon budget (Hagerty et al., 2014; He et al., 2015). In particular, the northern temperate and boreal terrestrial ecosystems are nitrogen-limited; neglecting microbial dormancy will lead to incorrect estimates of nitrogen availability through soil decomposition, failing to capture nitrogen feedbacks to carbon dynamics (Wang et al., 2015; Stolpovsky et al., 2011; Thullner et al., 2005). Furthermore, these ecosystems have experienced a marked seasonality of active and dormant microbial cycles and the above-global-average warming, which might have increased the proportion of active microbes in soils (He et al., 2015). Thus, incorporating dormancy effects will improve model realism to provide a better projection of the northern temperate and boreal terrestrial ecosystem carbon dynamics.
This study incorporated the effects of the microbial dormancy trait into an extant process-based biogeochemistry model (MIC-TEM) (Zha and Zhuang, 2018; He et al., 2015). The dormant and active microbial physiology has been considered explicitly in the new version of the model (MIC-TEM-dormancy). The revised model was parameterized, validated, and then applied to evaluate the carbon dynamics during the last century and this century in the northern temperate and boreal terrestrial ecosystems (north 45∘ N above). By comparing the results of MIC-TEM-dormancy and MIC-TEM, we can show that incorporating microbial dormancy may produce a much different prediction in the historical and future carbon budget.
2.1 Overview
Due to the importance of microbial dormancy, some recent work has been done to consider the metabolic activation and deactivation of microbes in soil and its effects on soil carbon (C) dynamics and climate feedbacks. For example, Wang et al. (2015) have incorporated transformation processes between active and dormant states to develop two versions of MEND, that is, MEND with and without dormancy. The two versions of the model have been applied to quantify the carbon decomposition in laboratory incubations of four soils. Salazar et al. (2018) have also taken microbially dormancy into account to compare their predictions of microbial biomass and soil heterotrophic respiration (RH) under simulated cycles of stressful (dryness) and favorable (wet pulses) conditions. Our study extends those modeling studies to the northern temperate and boreal terrestrial ecosystems by developing a more detailed biogeochemistry model considering the dormancy impacts. Below, we first describe how we developed the new model (MIC-TEM-dormancy) by incorporating the microbial dormancy trait into an existing microbe-based biogeochemistry model (MIC-TEM). Second, we discuss how parameterization and validation of the MIC-TEM-dormancy model were conducted using observed net ecosystem exchange data and heterotrophic respiration data at representative sites. Third, we presented how the model was applied to natural ecosystems in the region (above 45∘ N) for the 20th and 21st centuries and discussed the dormancy effects on their regional carbon budget.
2.2 Model description
A non-dormancy version of the biogeochemistry model (MIC-TEM) has been developed by incorporating a microbial module (Allison et al., 2010) into an extant large-scale biogeochemical model (TEM) to explicitly (Zhuang et al., 2015) consider the effects of microbial dynamics and enzyme kinetics on carbon dynamics (Zha and Zhuang, 2018). Here we further advanced the MIC-TEM by incorporating algorithms that describe the effects of microbial dormancy dynamics based on He et al. (2015). Different from He et al. (2015), in which the microbial module was driven with existing data of carbon stocks and fluxes, our study incorporated the microbial module into an extant MIC-TEM that simulates carbon data dynamically. This coupling enables us to extrapolate our model to northern temperate and boreal terrestrial ecosystems, rather than only for temperate forest regions in He et al. (2015). In our new model (MIC-TEM-dormancy), the microbial biomass pool was divided into two fractions, including the dormant and active microbial biomass pools. The two microbial biomass pools and the reversible transition between them have been considered explicitly in the new model (Fig. 1) but were ignored in MIC-TEM.
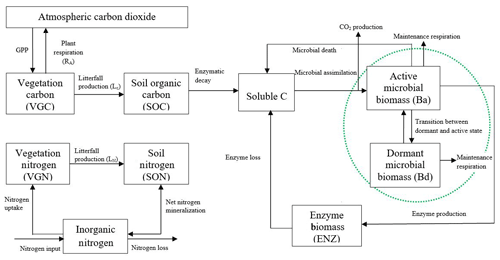
Figure 1Framework of the dormancy model: microbial biomass is split into two parts, active microbial biomass and dormant microbial biomass (shown in the green dashed circle). Maintenance respiration from these two parts and the CO2 production through microbial assimilation contribute to heterotrophic respiration. The model was revised based on Zha and Zhuang (2018).
In previous MIC-TEM, heterotrophic respiration (RH) is simply calculated as the product of ASSIM and CUE, which are microbial assimilation and carbon use efficiency, respectively. For detailed carbon dynamics in MIC-TEM, see Zha and Zhuang (2018).
Here we revised MIC-TEM by incorporating microbial dormancy dynamics according to He et al. (2015). In MIC-TEM-dormancy, the soil heterotrophic respiration RH is revised to include three parts: the maintenance respiration from the active and dormant microorganisms and the CO2 production through the process of microbial assimilation (He et al., 2015):
where the first two terms are maintenance respiration from the active and dormant microorganisms. The last term is the CO2 produced during the process of microbial assimilation.
For the first two terms, Ba and Bd represent the active and dormant microbial biomass pool, respectively. The parameter mR denotes the specific maintenance rate in an active state (h−1), and β is the ratio of dormant maintenance rate to active maintenance rate. Thus, βmR denotes the maximum specific maintenance rate in a dormant state. Temperature sensitivity was expressed as the Q10 function , where temp is soil temperature in the top 20 cm (degrees Celsius).
For the third term, the CO2 produced through microbial assimilation is calculated as in He et al. (2015) and Allison et al. (2010):
where ASSIM represents the microbial assimilation, and the parameter Yg represents carbon use efficiency. Microbial assimilation (ASSIM) is calculated as in He et al. (2015):
Here parameter α is maintenance weight (h−1); CNsoil and CNmic denote the C:N ratios of soil and that of microbial biomass. In addition, Φ is the substrate saturation level and defined as in He et al. (2015) and Wang et al. (2014):
where Ks is the half saturation constant for substrate uptake as indicated by the Michaelis–Menten kinetic, and S is soluble C substrates that are directly accessible for microbial assimilation (Wang et al., 2014). Here we quantified concentration of soluble C substrates that are directly accessible for microbial assimilation by using the conceptual framework from Davidson et al. (2012):
The term “soluble C” denotes the state variable of the soluble carbon pool. Dliq is the diffusion coefficient of the substrate in the liquid phase and is formulated as
where BD is the bulk density and PD is the soil particle density. θ is the volumetric soil moisture.
Different from MIC-TEM, the transitions between active and dormant microbial biomass are included in MIC-TEM-dormancy.
where Ba→d and Bd→a denote the transition from the active to dormant microbe and from the dormant to active microbe, respectively (He et al., 2015; Wang et al., 2014). Thus, dormancy rate is affected by active and dormant biomass, soil temperature (temp), and soil moisture (θ in Φ).
The active microbial biomass (Ba) is modeled as (He et al., 2015; Wang et al., 2014)
where DEATH and EPROD denote microbial biomass death and enzyme production, which are modeled as proportional to active microbial biomass with constant rates rdeath and rEnzProd (Allison et al., 2010):
where rdeath and rEnzProd are the rate constants of microbial death and enzyme production, respectively.
The dormant microbial biomass (Bd) is modeled as (He et al., 2015; Wang et al., 2014)
The soluble C pool is modeled as (He et al., 2015; Allison et al., 2010)
where DECAY represents the enzymatic decay of soil organic carbon (SOC), and ELOSS represents the loss of enzyme.
DECAY is regulated by enzyme biomass (ENZ), soil organic carbon (SOC), soil temperature, and substrate quality (He et al., 2015):
where Vmax is the maximum SOC decay rate, and Kmuptake is the half-saturation constant for enzymatic decay.
ELOSS is modeled as a first-order process (Allison et al., 2010) to represent enzyme turnover:
where renzloss is the rate constant of enzyme loss.
The soil organic carbon pool (SOC) is modeled as
where litterfall is estimated as a function of vegetation carbon (Zhuang et al., 2010).
Last, the enzyme pool (ENZ) is modeled as
With the modification of microbial carbon dynamics by considering microbial life history traits, soil decomposition is changed since it is controlled by microbes. When microbial dormancy is considered, the number of active microbes that participate in soil decomposition is much less. The changes in soil decomposition directly influence the amount of soil respiration and further influence soil nitrogen (N) mineralization that determines soil N availability for plants, affecting gross primary production (GPP). Since both GPP and RH can be affected by microbial dormancy, net ecosystem production (NEP) will also be affected.
2.3 Model parameterization and validation
The detailed description of parameters that are related to microbial dormancy can be found in He et al. (2015) (Table 1). Here we calibrated the MIC-TEM-dormancy at six representative sites with gap-filled monthly net ecosystem productivity (NEP, gC m−2 per month) data in northern temperate and boreal regions (Table 2). Site-level climatic data and soil texture data were organized for driving the model. All site information can be found on the AmeriFlux network (Davidson et al., 2000). The results for model parameterization were presented in Fig. 2. We conducted the parameterization using a global optimization algorithm known as the SCE-UA (Shuffled complex evolution) method (Duan et al., 1994). An ensemble of 50 independent sets of parameters were performed based on prior ranges from literature (Table 1) to minimize the difference between the monthly simulated and measured NEP at the chosen sites. The cost function of the minimization is
where NEPobs,i and NEPsim,i are the observed and simulated NEP, respectively. k is the number of data pairs for comparison. Except for the parameters of microbial dormancy, other parameters are derived directly from MIC-TEM (Zha and Zhuang, 2018). The optimized parameters were used for model validation and regional simulations.
For model validation, we chose another six sites that contain monthly NEP data from the AmeriFlux network (Table 3). Four of these six sites were also used for parameterization (Table 2). However, we used the data of different observation periods for model validation for those overlapped sites. Moreover, we also conducted site-level validations with monthly soil respiration data from the AmeriFlux network and Fluxnet dataset. The site information was provided in Table 4. For these sites, we assumed 50 % of soil respiration was heterotrophic respiration (RH) for forest (Hanson et al., 2000) and 60 % and 70 % of that was RH for grassland (Wang et al., 2009) and tundra (Billings et al., 1977). Because there is a limited amount of available heterotrophic respiration (RH) data, we could not conduct a regional validation for all pixels in northern temperate and boreal regions. Instead, we extracted 61 sites providing data of average annual heterotrophic respiration from the ORNL global Soil Respiration Dataset (https://daac.ornl.gov/SOILS/guides/SRDB_V4.html, last access: 9 December 2020, Bond-Lamberty et al., 2018) for model validation. The site-level observed average annual RH was used to compare with simulated annual RH by MIC-TEM-dormancy and MIC-TEM. The MIC-TEM-dormancy was run at monthly time steps to keep consistent with the time step of MIC-TEM. Although microbial dynamics occur at fine temporal scales (Tang and Riley, 2014), we can still quantify the cumulative impacts of microbial dynamics on carbon and nitrogen cycling at monthly time steps by not changing the model structure.
2.4 Spatial extrapolation
For historical simulations during the 20th century, two sets of regional simulations using MIC-TEM-dormancy and MIC-TEM at a spatial resolution of 0.5∘ latitude × 0.5∘ longitude were conducted. Our model simulation contains two parts: spin-up and transient simulation. A typical spin-up was conducted to get the model to a steady state for each spatial location, which will be used as initial conditions for transient simulations (McGuire et al., 1992). During spin-up procedure, cyclic forcing data were used to force the model run and repeated continuously until dynamic equilibrium was achieved at which the modeled state variables show a cyclic pattern or become constant. Specifically, this study used the monthly historical climate data from 1900 to 1940 to repeatedly drive the model for the spin-up. Before spin-up procedure, the model was initialized with default built-in carbon stocks (Raich et al., 1991). During transient simulations, the calibrated ecosystem-specific parameters were used for regional simulations. The previous dynamic equilibrium was used as an initial value for transient simulation. The historical climatic forcing data, including the monthly air temperature, precipitation, cloudiness, and atmospheric CO2 concentrations, were organized from the Climatic Research Unit (CRU TS3.1) from the University of East Anglia (Harris et al., 2014). We also used gridded data of soil texture (Zhuang et al., 2015), elevation (Zhuang et al., 2015), and potential natural vegetation (Melillo et al., 1993) from literature. In our model, we assumed that soil texture, elevation, and potential natural vegetation data only vary spatially, not vary over time (Zhuang et al., 2015).
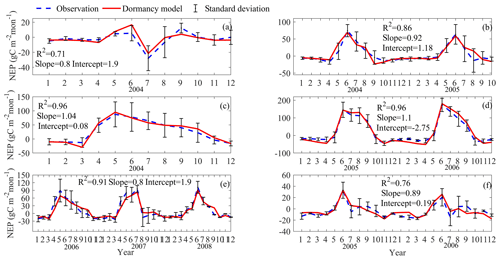
Figure 2Comparison between observed and simulated NEP (gC m−2 per month) at (a) Ivotuk (alpine tundra), (b) UCI-1964 burn site (boreal forest), (c) Howland Forest (main tower) (temperate coniferous forest), (d) Univ. of Mich. Biological Station (temperate deciduous forest), (e) KUOM turf-grass field (grassland), and (f) Atqasuk (wet tundra). Note: scales are different. Error bars represent standard errors among daily measured data in 1 month.
In addition, regional simulations over the 21st century were conducted under two Intergovernmental Panel on Climate Change (IPCC) climate scenarios (RCP2.6 and RCP8.5). The future climatic forcing data under these two climate change scenarios were derived from the HadGEM2-ES model, which is a member of CMIP5project213 (https://esgf-node.llnl.gov/search/cmip5/, last access: 9 December 2020). Then the regional estimations were obtained by summing up the gridded outputs for our study region. The positive simulated NEP represents a CO2 sink from the atmosphere to terrestrial ecosystems, while a negative value represents a source of CO2 from terrestrial ecosystems to the atmosphere.
2.5 Parameter equifinality effects
Our previous studies using TEM have demonstrated that equifinality derived from site-level parameterization will affect the uncertainty in the estimation of regional carbon dynamics (Tang and Zhuang, 2008, 2009). Here equifinality refers to the fact that a number of sets of parameters result in model simulations that all match the data similarly well. To quantify this effect on our simulation uncertainty, we conducted ensemble regional simulations with 50 sets of parameters for both historical and future studies. The 50 sets of parameters were obtained according to the method in Tang and Zhuang (2008).
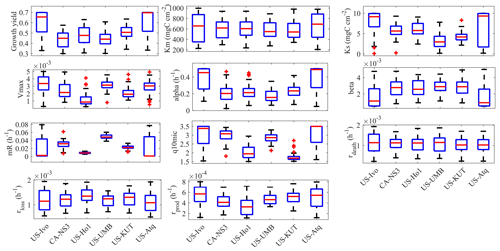
Figure 3Box plot of parameter posterior distributions that are obtained after ensemble inverse modeling for MIC-TEM-dormancy for all six sites: US-Ivo: Ivotuk (alpine tundra), CA-NS3: UCI-1964 burn site (boreal forest), US-Ho1: Howland Forest (temperate coniferous forest), US-UMB: Univ. of Mich. Biological Station (temperate deciduous forest), US-KUT: KUOM turf-grass field (grassland), US-Atq: Atqasuk (wet tundra).
3.1 Inversed model parameters and model validation
Using the SCE-UA ensemble method, 50 independent sets of parameters were converged to minimize the objective function. Then the optimized parameters are calculated as the mean of these 50 sets of inversed parameters. The box plot of parameter posterior distributions reflects different ecosystem properties at these sites (Fig. 3). For instance, growth yield was higher in tundra types than in forests, meaning microorganisms in environments with higher energy limitation tend to enhance the efficiency of energy transportation. In addition, alpha, the maintenance weight, was also higher in tundra types than in forests. From the plot for the parameter beta, the ratio of dormant maintenance rate to specific maintenance rate for active biomass in tundra types is lower than that in forest types. Other microbially related parameters did not differentiate much among different vegetation types.
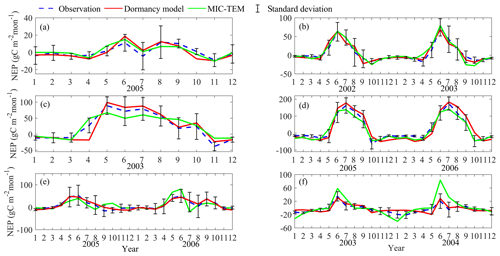
Figure 4Comparison between observed and simulated NEP (gC m−2 per month) at (a) Ivotuk (alpine tundra), (b) UCI-1964 burn site (boreal forest), (c) Howland Forest (main tower) (temperate coniferous forest), (d) Bartlett Experimental Forest (temperate deciduous forest), (e) Brookings (grassland), and (f) Atqasuk (wet tundra). Note: scales are different.
After parameterization, the MIC-TEM-dormancy was validated with monthly NEP data for six representative ecosystems, and the comparisons between monthly observed NEP and simulated NEP were presented in Fig. 4. With the optimized parameters, the dormancy-based model was used to reproduce NEP to compare with the measured NEP (Table 5). The R2 ranges from 0.67 for Atqasuk to 0.93 for Bartlett Experimental Forest (Table 5). Generally, our new model performs better for forest ecosystems than for tundra ecosystems. Compared with MIC-TEM, the dormancy model performs better for alpine tundra, temperate coniferous forest, and grassland. For other sites, both models show similar performance (Table 5). In addition, a set of monthly soil respiration data were selected to evaluate the estimated RH. The comparisons between monthly observed RH and simulated RH from two contrasting models were conducted (Fig. 5). MIC-TEM-dormancy has higher R2 and lower root-mean-square error (RMSE) (Table 6). Sixty-one sites with average annual RH in northern temperate and boreal regions were used to further evaluate the new model performance. The dormancy model has lower intercept and slope with R2 of 0.45, while R2 of MIC-TEM is 0.3 (Fig. 6). These analyses indicate that the new model is more realistic in representing RH by considering microbial dormancy.
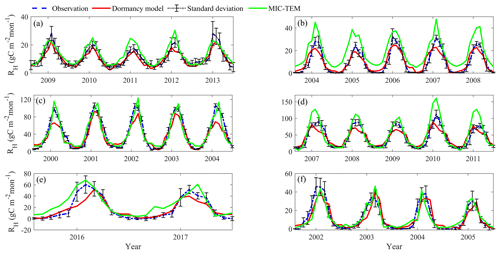
Figure 5Comparison between observed and simulated RH (gC m−2 per month) at (a) US-EML (alpine tundra), (b) CA-SJ2 (boreal forest), (c) US-Ho2 (temperate coniferous forest), (d) US-UMB (temperate deciduous forest), (e) US-Ro4 (grassland), and (f) RU-Che (wet tundra). Note: scales are different.
3.2 Regional carbon dynamics during the 20th century
Regional extrapolation with both models estimated a regional terrestrial ecosystem carbon sink but with different magnitudes (Fig. 7c). With optimized parameters, MIC-TEM estimated a regional carbon sink of 77.6 Pg with the interannual standard deviation of 0.21 Pg C yr−1 during the 20th century. However, MIC-TEM-dormancy nearly doubles the sink at 153.5 Pg with the interannual standard deviation of 0.12 Pg C yr−1 during the last century (Fig. 7c). At the end of the century, MIC-TEM estimated that NEP reaches 1.0 Pg C yr−1 in comparison with MIC-TEM-dormancy estimates of 1.5 Pg C yr−1 (Fig. 7c). Both models simulated similar trends for regional net primary production (NPP), RH, and NEP (Fig. 7). Generally, they show an increasing trend in the 20th century (Fig. 7). Meanwhile, with optimized parameters, MIC-TEM-dormancy estimated NPP and RH at 7.94 and 6.4 Pg C yr−1, which are 5.8 % and 16.3 % less than the estimations from MIC-TEM, respectively (Fig. 7a and b). This pronounced difference of NEP between two models comes from the disparity between the simulated NPP and RH with them since NEP is calculated as the difference between NPP and RH. Without considering dormancy, MIC-TEM estimates more active microbial biomass, hence overestimating both RH and NPP (due to higher simulated N mineralization and uptake by plants), but resulting in lower NEP than that calculated by MIC-TEM-dormancy.
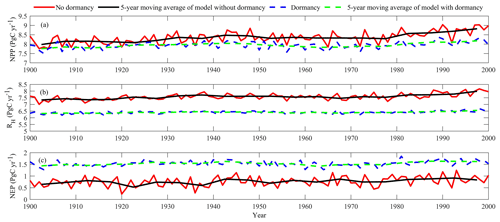
Figure 7Simulated annual net primary production (NPP, a), heterotrophic respiration (RH, b), and net ecosystem production (NEP, c) during the 20th century by the dormancy model and MIC-TEM.
Temporally, both models projected higher NPP and RH in summer than in winter (Fig. 8a and b) due to higher soil temperature and moisture (McGuire et al., 1992). Setting the RH projection from MIC-TEM as a baseline, MIC-TEM-dormancy projected 33 % less RH in summer (May to September) and 30 % more in winter (other months) (Fig. 8b), indicating that without dormancy, the model tends to estimate lower soil respiration due to ignorance of dormant respiration in winter but higher soil respiration due to higher active biomass in summer. NEP seasonality estimated with two models are close to each other (Fig. 8c), but the dormancy model projected slightly higher NEP in summer.
3.3 Regional carbon dynamics during the 21st century
Under the RCP8.5 scenario, both models estimated the regional natural terrestrial ecosystems act as a carbon sink (Fig. 9). The MIC-TEM-dormancy predicted a C accumulation of 129.9 Pg by the end of this century, with the interannual standard deviation of 0.13 Pg C yr−1, whereas MIC-TEM estimates a C accumulation of 79.5 Pg with the interannual standard deviation of 0.37 Pg C yr−1 during the 21st century (Fig. 9). Thus, MIC-TEM-dormancy estimates an increase of 50.4 Pg regional carbon sequestration relative to MIC-TEM, with less interannual variation (Fig. 9). Under this scenario, both models predict similar temporal trends for NEP, namely increasing from the 2000s and then decreasing from the 2070s onward (Fig. 9). MIC-TEM-dormancy predicts that the carbon sink reaches 1.36 Pg C yr−1 in the 2090s, which is 0.26 Pg C yr−1 more than the projection of MIC-TEM. Moreover, MIC-TEM-dormancy estimated NPP and RH at 10.2 and 8.9 Pg C yr−1, which are 1.3 and 1.8 Pg C yr−1 less than the estimations from MIC-TEM, respectively (Fig. 9).
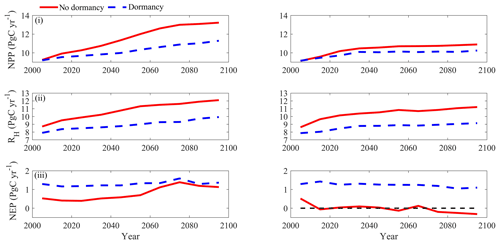
Figure 9Predicted changes in carbon fluxes: (i) NPP, (ii) RH, and (iii) NEP for all land areas north of 45∘ N in response to transient climate change under the RCP8.5 scenario (left panels) and RCP2.6 scenario (right panels) with the dormancy model and MIC-TEM, respectively. The decadal running mean is applied.
Under the RCP2.6 scenario, the cumulative NEP from two models diverged by 125.2 Pg C by 2100. The trajectory of interannual NEP estimated with the two models also diverged. The MIC-TEM predicted the region fluctuates between carbon sinks and sources and totally acts as a carbon source of 1.6 Pg C with the interannual standard deviation of 0.24 Pg C yr−1 during the 21st century. In contrast, MIC-TEM-dormancy projected the region acts as a carbon sink of 123.6 Pg C with an interannual standard deviation of 0.1 Pg C yr−1 (Fig. 9). MIC-TEM-dormancy estimates NPP and RH at 9.9 and 8.7 Pg C yr−1, which are 0.5 and 1.7 Pg C yr−1 less than the estimations from MIC-TEM, respectively (Fig. 9). Moreover, simulations under the two contrasting climate scenarios (RCP2.6 and RCP8.5) exhibit a large difference of 81.1 Pg C of cumulative NEP during the 21st century by MIC-TEM, but only 6.3 Pg C of that by MIC-TEM-dormancy.
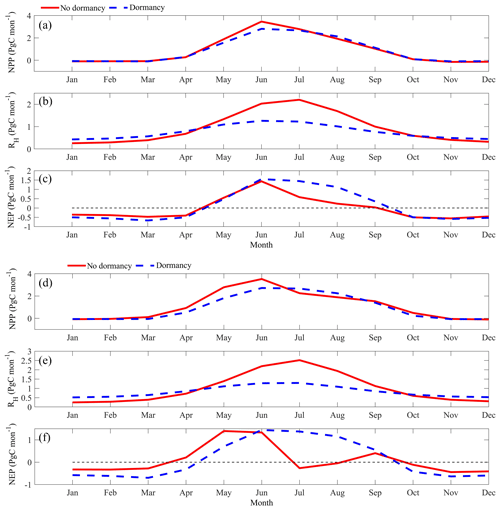
Figure 10Regional annual seasonal pattern of simulated net primary production (NPP, a, d), heterotrophic respiration (RH, b, e), and net ecosystem production (NEP, c, f) during the 2090s from the dormancy model and MIC-TEM under (a, b, c) the RCP2.6 scenario and (d, e, f) the RCP8.5 scenario. The region is all land areas north of 45∘ N.
MIC-TEM-dormancy estimated higher RH in winter, but lower RH in summer under both future scenarios in the 2090s (Fig. 10). NPP is the same in winter with or without dormancy, and in the late summer it is higher than that without dormancy, especially in the RCP8.5 scenario. The combined flattening patterns of NPP and RH result in different patterns for NEP. Under the RCP2.6 scenario, MIC-TEM-dormancy predicts higher NEP from June to October but lower NEP from January to April compared to MIC-TEM (Fig. 10). Under the RCP8.5 scenario, MIC-TEM-dormancy predicts higher NEP from June to September but much lower NEP in other months than MIC-TEM (Fig. 10).
3.4 Regional uncertainty considering equifinality effects during 20th and 21st centuries
The ensemble simulations for the 20th century are shown in Fig. 11. Given the uncertainty in parameters, MIC-TEM-dormancy predicts that the regional cumulative carbon ranges from a carbon loss of 28.2 Pg to a carbon sink of 362.1 Pg by different ensemble members, with a mean of 71.2±54.8 Pg (Fig. 11). For the 21st century, MIC-TEM-dormancy predicts that the region acts from a carbon source of 49.3 Pg C to a carbon sink of 296.5 Pg C, with a mean of 112.7±116.5 Pg under RCP2.6 scenario (Fig. 12). Under the RCP8.5 scenario, MIC-TEM-dormancy predicts that the region acts from a carbon source of 27.1 Pg C to a carbon sink of 401.3 Pg C, with a mean of 143.1±162.5 Pg (Fig. 12).
Our regional simulations with two contrasting models (MIC-TEM, MIC-TEM-dormancy) indicate the regional natural terrestrial ecosystems acted as a carbon sink in past decades, which is consistent with results from other process-based models (White et al., 2000; McGuire et al., 2009; Schimel, 2013). However, the magnitudes of this sink are quite different in two models. Moreover, MIC-TEM-dormancy predicts the sink will decrease under both RCP8.5 and RCP2.6 scenarios during the 21st century, while MIC-TEM projects that the sink will increase under the RCP8.5 but change to a carbon source under the RCP2.6 scenario. Estimations based on models without dormancy could fit observations of RH as well as estimations with dormancy, but at the cost of underestimating microbial biomass (Wang et al., 2014). Differences in predicted RH with and without dormancy increase with temperature and with the length of the dry periods between wetting events (Salazar et al., 2018). The large difference in the two models suggests the importance of incorporating microbial dormancy effects.
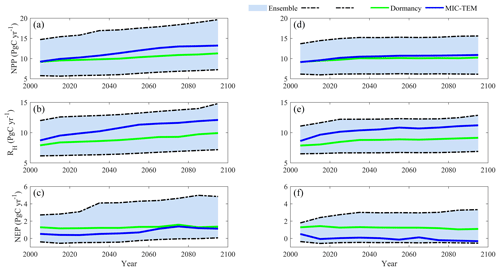
Figure 12Simulated annual net primary production (NPP, a, d), heterotrophic respiration (RH, b, e), and net ecosystem production (NEP, c, f) under the RCP8.5 scenario (a–c) and RCP2.6 scenario (d–f) by MIC-TEM-dormancy with an ensemble of parameters. The decadal running mean is applied. The grey area represents the upper and lower bounds of simulations.
The large bias between dormancy and non-dormancy models mainly comes from two parts. First, many important microbial activities such as soil organic carbon decomposition and nutrient cycling largely depend on the active fraction of microbial communities, not total microbial biomass (Wang et al., 2014; Blagodatsky et al., 2000). However, only a small part (about 0.1 %–2 %, seldom exceed 5 %) of the total soil microbial biomass is recognized to be active under natural conditions (Blagodatsky et al., 2011; Werf and Verstraete, 1987). Thus, dormancy could be a prominent feature in soil systems (Wang et al., 2014). Without considering dormancy, the “effective” microbial biomass for soil decomposition could be overestimated, resulting in overestimation of heterotrophic respiration (He et al., 2015). He et al. (2015) predicted total soil RH of all temperate forests (25–50∘ N) from the dormancy model amounted to 7.28 and 8.83 Pg C yr−1 from a no-dormancy model, which is 21.3 % higher than the dormancy model. Although their study region and simulation period are different from our study, the results can still be comparable. Both studies indicated that the magnitude of RH from the no-dormancy model is higher than for dormancy models. Second, high soil respiration stimulates N mineralization in soils (Zhuang et al., 2001, 2002), making more nutrients for photosynthesis of plants (Raich et al., 1991; McGuire et al., 1995; Zhuang et al., 2015; Zha and Zhuang, 2018; Thullner et al., 2005).
Therefore, NPP will be higher due to the N enrichment from higher RH. However, how NEP will change is still unclear. Our estimates of the northern extratropical NEP in the 1980s (1.61 Pg C yr−1 with MIC-TEM-dormancy and 0.84 Pg C yr−1 with MIC-TEM) are within ranges (0.6 to 2.3 Pg C yr−1) reported in the literature for northern regions (Schimel et al., 2001). Moreover, our predicted time trajectory of NEP in the 21st century under the RCP2.6 scenario is very similar to the finding of White et al. (2000), indicating that NEP increases from the 2000s to the 2070s and then decreases in the 2090s. Although our dormancy model can project reasonable carbon fluxes and indicates the importance of incorporating microbial dormancy when compared with MIC-TEM (Zha and Zhuang et al., 2018), there are some other microbial traits that have not yet been considered in our model. For instance, one vital common evolutionary trait of microbes is the community shift (Wang et al., 2015) with changing environment, including warming, N fertilization, and precipitation (Treseder et al., 2011; Frey et al., 2013; Allison et al., 2009; Evans and Wallenstein, 2011). Community shift will influence microbial physiology, temperature sensitivity, and growth rates (Classen et al., 2015), which will further affect the rate of soil decomposition and other carbon dynamics (Treseder et al., 2011; Schimel and Schaeffer, 2012; Todd-Brown et al., 2011). In addition, microbial community composition was ignored in our model. We did not separate among functional microbial groups, but gather microbes into one “box”. However, microbial community composition could influence ecosystem functioning, and their variance in responses to environmental conditions could alter the prediction of the rates of decomposition of organic material (Balser et al., 2002; Fierer et al., 2007). In particular, some narrowly distributed functions can be more sensitive to microbial community composition, and these might benefit most from explicit consideration of distinguishing functional groups in ecosystem models (McGuire and Treseder, 2010; Schimel, 1995). Thus, functional dissimilarity in microbial communities can be considered the next step for model development (Strickland et al., 2009; Moorhead et al., 2006). Moreover, microbial acclimation, a mechanism of adaption to a new temperature regime, is another important trait to affect soil decomposition. Recent studies have found that the warming-induced elevated respiration of the microbial community could decrease over time because of acclimation (Melillo et al., 1993; Todd-Brown et al., 2011). This mechanism shall be factored into future soil decomposition analysis.
Except for the model limitations mentioned above, additional uncertainties may come from inadequate model parameterization and model assumptions. For example, a critical microbial parameter, carbon use efficiency (CUE), is a primary control to soil CO2 efflux. Higher CUE indicates more microbial growth and more carbon uptake by plants, while lower CUE indicates higher soil decomposition (Manzoni et al., 2012). Theoretical and empirical studies have suggested that CUE depends on both temperature and substrate quality (Frey et al., 2013) and decreases as temperature increases and nutrient availability decreases (Manzoni et al., 2012). Our study considered the CUE sensitivity to temperature, but not nutrient availability. On the other hand, some model assumptions can also cause uncertainties. For example, we assumed that vegetation will not change during the transient simulation. However, over the past few decades in northern temperate and boreal regions, temperature increases have led to vegetation shift from one type to another (Hansen et al., 2006; White et al., 2000). The vegetation changes will affect carbon cycling in these ecosystems.
While our analysis suggests it is important to incorporate microbial dormancy dynamics into a process-based biogeochemistry model to more adequately simulate carbon dynamics in northern temperate and boreal regions, we do confront modeling dilemmas. First, our process-based models have a relatively large number of parameters, which unavoidably creates the “equifinality” problem as recognized in our previous studies for the model (e.g., Tang and Zhuang, 2008, 2009). To alleviate this problem in this analysis, we have conducted parameter ensemble simulations at both site and regional levels and presented our results with uncertainties, which could be a standard approach for process-based complex biogeochemistry modeling analyses. Second, incorporating more ecosystem processes increases the number of parameters in our model, inducing even larger uncertainties for both site-level and regional simulations. On the one hand, the more complex model to a certain degree helps capture observations; on the other hand, the model uncertainty has not been constrained or even enlarged. We highlight the need to further investigate this trade-off within the modeling research community.
This study incorporated microbial dormancy into a detailed microbe-based soil decomposition biogeochemistry model to examine the fate of large soil carbon storage in northern temperate and boreal natural terrestrial ecosystems under changing climate conditions. Regional simulations using MIC-TEM-dormancy indicated that, over the 20th century, the region is a carbon sink of 166.8±97.7 Pg. This sink could decrease to 175.9±105.4 Pg under the RCP8.5 scenario or 125.4±85.5 Pg under the RCP2.6 scenario during the 21st century. Whether considering microbial dormancy or not can cause large differences in soil decomposition estimation between two models. Meanwhile, due to available nitrogen affected by soil decomposition, net primary production is consequently influenced in these two centuries. The combined changes in soil decomposition and net primary production led to large differences in carbon budget estimation between two models. Compared with MIC-TEM, MIC-TEM-dormancy projected 75.9 Pg more C stored in the terrestrial ecosystems over the last century and 50.4 and 125.2 Pg more C under the RCP8.5 and RCP2.6 scenarios, respectively. This study highlights the importance of the representation of microbial dormancy in earth system models in order to adequately quantify the carbon dynamics of natural terrestrial ecosystems in northern temperate and boreal regions.
All data used in this study are available from the authors upon request.
QZ designed the study. JZ conducted model development, simulation, and analysis. JZ and QZ wrote the paper.
The authors declare that they have no conflict of interest.
This research was supported by a NSF project (IIS-1027955), a DOE project (DE-SC0008092), and a NASA LCLUC project (NNX09AI26G) to Qianlai Zhuang. We acknowledge the Rosen High Performance Computing Center at Purdue for computing support. We thank the National Snow and Ice Data center for providing Global Monthly EASE-Grid snow water equivalent data and the National Oceanic and Atmospheric Administration for North American Regional Reanalysis (NARR). We also acknowledge the World Climate Research Programme's Working Group on Coupled Modeling Intercomparison Project CMIP5, and we thank the climate modeling groups for producing and making available their model output. The data presented in this paper can be accessed through our research website (http://www.eaps.purdue.edu/ebdl/, last access: 9 December 2020).
This research has been supported by the NSF (grant no. IIS-1027955), the DOE (grant no. DE-SC0008092), NASA LCLUC (grant no. NNX09AI26G), and NASA (NNX17AK20G).
This paper was edited by Jens-Arne Subke and reviewed by Thomas Wutzler, Alejandro Salazar, and one anonymous referee.
Allison, E. H., Perry, A. L., Badjeck, M.-C., Neil Adger, W., Brown, K., Conway, D., Halls, A. S., Pilling, G. M., Reynolds, J. D., Andrew, N. L., and Dulvy, N. K.: Vulnerability of national economies to the impacts of climate change on fisheries, Fish Fish., 10, 173–196, https://doi.org/10.1111/j.1467-2979.2008.00310.x, 2009.
Allison, S. D., Wallenstein, M. D., and Bradford, M. A.: Soil-carbon response to warming dependent on microbial physiology, Nat. Geosci., 3, 336–340, https://doi.org/10.1038/ngeo846, 2010.
Balser, T. C., Kinzig, A. P., and Firestone, M. K.: Linking soil microbial communities and ecosystem functioning, The functional consequences of biodiversity: Empirical progress and theoretical extensions, Biogeochemistry, 73, 265–293, 2002.
Billings, W. D., Peterson, K. M., Shaver, G. R., and Trent, A. W.: Root Growth, Respiration, and Carbon Dioxide Evolution in an Arctic Tundra Soil, Arctic Alpine Res., 9, 129–137, https://doi.org/10.1080/00040851.1977.12003908, 1977.
Blagodatskaya, E. and Kuzyakov, Y.: Active microorganisms in soil: Critical review of estimation criteria and approaches, Soil Biol. Biochem., 67, 192–211, https://doi.org/10.1016/j.soilbio.2013.08.024, 2013.
Blagodatskaya, E., Khomyakov, N., Myachina, O., Bogomolova, I., Blagodatsky, S., and Kuzyakov, Y.: Microbial interactions affect sources of priming induced by cellulose, Soil Biol. Biochem., 74, 39–49, https://doi.org/10.1016/j.soilbio.2014.02.017, 2014.
Blagodatsky, S., Grote, R., Kiese, R., Werner, C., and Butterbach-Bahl, K.: Modelling of microbial carbon and nitrogen turnover in soil with special emphasis on N-trace gases emission, Plant Soil, 346, 297–330, https://doi.org/10.1007/s11104-011-0821-z, 2011.
Blagodatsky, S. A., Heinemeyer, O., and Richter, J.: Estimating the active and total soil microbial biomass by kinetic respiration analysis, Biol. Fertil. Soils, 32, 73–81, 2000.
Bond-Lamberty, B. and Thomson, A.: Temperature-associated increases in the global soil respiration record, Nature, 464, 579–582, https://doi.org/10.1038/nature08930, 2010.
Bond-Lamberty, B., Bailey, V. L., Chen, M., Gough, C. M., and Vargas, R.: Globally rising soil heterotrophic respiration over recent decades, Nature, 560, 80–83, https://doi.org/10.1038/s41586-018-0358-x, 2018.
Bouskill, N. J., Tang, J., Riley, W. J., and Brodie, E. L.: Trait-based representation of biological nitrification: model development, testing, and predicted community composition, Front. Microb., 3, 364, https://doi.org/10.3389/fmicb.2012.00364, 2012.
Callaghan, T., Björn, L. O., Chernov, Y., Chapin, T., Christensen, T. R., Huntley, B., Ims, R., Jolly, D., Jonasson, S., Matveyeva, N., Panikov, N., Oechel, W., and Shaver, G.: Arctic tundra and polar desert ecosystems, Arctic climate impact assessment, Cambridge University Press, New York, 243–352, 2005.
Classen, A. T., Sundqvist, M. K., Henning, J. A., Newman, G. S., Moore, J. A. M., Cregger, M. A., Moorhead, L. C., and Patterson, C. M.: Direct and indirect effects of climate change on soil microbial and soil microbial-plant interactions: What lies ahead?, Ecosphere, 6, 130, https://doi.org/10.1890/es15-00217.1, 2015.
Conant, R. T., Ryan, M. G., Ågren, G. I., Birge, H. E., Davidson, E. A., Eliasson, P. E., Evans, S. E., Frey, S. D., Giardina, C. P., Hopkins, F. M., Hyvönen, R., Kirschbaum, M. U. F., Lavallee, J. M., Leifeld, J., Parton, W. J., Megan Steinweg, J., Wallenstein, M. D., Martin Wetterstedt, J. Å., and Bradford, M. A.: Temperature and soil organic matter decomposition rates – synthesis of current knowledge and a way forward, Glob. Change Biol., 17, 3392–3404, https://doi.org/10.1111/j.1365-2486.2011.02496.x, 2011.
Coursolle, C., Margolis, H. A., Barr, A. G., Black, T. A., Amiro, B. D., McCaughey, J. H., Flanagan, L. B., Lafleur, P. M., Roulet, N. T., Bourque, C. P. A., Arain, M. A., Wofsy, S. C., Dunn, A., Morgenstern, K., Orchansky, A. L., Bernier, P. Y., Chen, J. M., Kidston, J., Saigusa, N., and Hedstrom, N.: Late-summer carbon fluxes from Canadian forests and peatlands along an east–west continental transect, Can. J. Forest Res., 36, 783–800, https://doi.org/10.1139/x05-270, 2006.
Davidson, E. A., Trumbore, S. E., and Amundson, R.: Biogeochemistry: soil warming and organic carbon content, Nature, 408, 789–790, https://doi.org/10.1038/35048672, 2000.
Davidson, E. A. and Janssens, I. A.: Temperature sensitivity of soil carbon decomposition and feedbacks to climate change, Nature, 440, 165–173, https://doi.org/10.1038/nature04514, 2006.
Davidson, E. A., Janssens, I. A., and Luo, Y.: On the variability of respiration in terrestrial ecosystems: moving beyond Q10, Glob. Change Biol., 12, 154–164, https://doi.org/10.1111/j.1365-2486.2005.01065.x, 2006.
Davidson, E. A., Samanta, S., Caramori, S. S., and Savage, K.: The Dual Arrhenius and Michaelis-Menten kinetics model for decomposition of soil organic matter at hourly to seasonal time scales, Glob. Change Biol., 18, 371–384, https://doi.org/10.1111/j.1365-2486.2011.02546.x, 2012.
Duan, Q., Sorooshian, S., and Gupta, V. K.: Optimal use of the SCE-UA global optimization method for calibrating watershed models, J. Hydrol., 158, 265–284, 1994.
Evans, S. E. and Wallenstein, M. D.: Soil microbial community response to drying and rewetting stress: does historical precipitation regime matter?, Biogeochemistry, 109, 101–116, https://doi.org/10.1007/s10533-011-9638-3, 2011.
Fierer, N., Morse, J. L., Berthrong, S. T., Bernhardt, E. S., and Jackson, R. B.: Environmental controls on the landscape – scale biogeography of stream bacterial communities, Ecology, 88, 2162–2173, 2007.
Frey, S. D., Lee, J., Melillo, J. M., and Six, J.: The temperature response of soil microbial efficiency and its feedback to climate, Nat. Clim. Change, 3, 395–398, https://doi.org/10.1038/nclimate1796, 2013.
German, D. P., Marcelo, K. R. B., Stone, M. M., and Allison, S. D.: The Michaelis-Menten kinetics of soil extracellular enzymes in response to temperature: a cross-latitudinal study, Glob. Change Biol., 18, 1468–1479, https://doi.org/10.1111/j.1365-2486.2011.02615.x, 2012.
Gilmanov, T. G., Tieszen, L. L., Wylie, B. K., Flanagan, L. B., Frank, A. B., Haferkamp, M. R., Meyers, T. P., and Morgan, J. A.: Integration of CO2flux and remotely-sensed data for primary production and ecosystem respiration analyses in the Northern Great Plains: potential for quantitative spatial extrapolation, Glob. Ecol. Biogeogr., 14, 271–292, https://doi.org/10.1111/j.1466-822X.2005.00151.x, 2005.
Gough, C. M., Hardiman, B. S., Nave, L. E., Bohrer, G., Maurer, K. D., Vogel, C. S., Nadelhoffer, K. J., and Curtis, P. S.: Sustained carbon uptake and storage following moderate disturbancein a Great Lakes forest, Ecol. Appl., 23, 1202–1215, 2013.
Goulden, M. L., Winston, G. C., McMillan, A. M. S., Litvak, M. E., Read, E. L., Rocha, A. V., and Rob Elliot, J.: An eddy covariance mesonet to measure the effect of forest age on land-atmosphere exchange, Glob. Change Biol., 12, 2146–2162, https://doi.org/10.1111/j.1365-2486.2006.01251.x, 2006.
Graham, E. B., Wieder, W. R., Leff, J. W., Weintraub, S. R., Townsend, A. R., Cleveland, C. C., Philippot, L., and Nemergut, D. R.: Do we need to understand microbial communities to predict ecosystem function? A comparison of statistical models of nitrogen cycling processes, Soil Biol. Biochem., 68, 279–282, https://doi.org/10.1016/j.soilbio.2013.08.023, 2014.
Graham, E. B., Knelman, J. E., Schindlbacher, A., Siciliano, S., Breulmann, M., Yannarell, A., Beman, J. M., Abell, G., Philippot, L., Prosser, J., Foulquier, A., Yuste, J. C., Glanville, H. C., Jones, D. L., Angel, R., Salminen, J., Newton, R. J., Burgmann, H., Ingram, L. J., Hamer, U., Siljanen, H. M., Peltoniemi, K., Potthast, K., Baneras, L., Hartmann, M., Banerjee, S., Yu, R. Q., Nogaro, G., Richter, A., Koranda, M., Castle, S. C., Goberna, M., Song, B., Chatterjee, A., Nunes, O. C., Lopes, A. R., Cao, Y., Kaisermann, A., Hallin, S., Strickland, M. S., Garcia-Pausas, J., Barba, J., Kang, H., Isobe, K., Papaspyrou, S., Pastorelli, R., Lagomarsino, A., Lindstrom, E. S., Basiliko, N., and Nemergut, D. R.: Microbes as Engines of Ecosystem Function: When Does Community Structure Enhance Predictions of Ecosystem Processes?, Front. Microb., 7, 214, https://doi.org/10.3389/fmicb.2016.00214, 2016.
Griffis, T. J., Lee, X., Baker, J. M., Billmark, K., Schultz, N., Erickson, M., Zhang, X., Fassbinder, J., Xiao, W., and Hu, N.: Oxygen isotope composition of evapotranspiration and its relation to C4photosynthetic discrimination, J. Geophys. Res., 116, https://doi.org/10.1029/2010jg001514, 2011.
Hagerty, S. B., van Groenigen, K. J., Allison, S. D., Hungate, B. A., Schwartz, E., Koch, G. W., Kolka, R. K., and Dijkstra, P.: Accelerated microbial turnover but constant growth efficiency with warming in soil, Nat. Clim. Change, 4, 903–906, https://doi.org/10.1038/nclimate2361, 2014.
Hanson, P. J., Edwards, N. T., Garten, C. T., and Andrews, J. A.: Separating root and soil microbial contributions to soil respiration: A review of methods and observations, Biogeochemistry, 48, 115–146, 2000.
Hansen, J., Sato, M., Ruedy, R., Lo, K., Lea, D. W., and Medina-Elizade, M.: Global temperature change, P. Natl. Acad. Sci. USA, 103, 14288–14293, https://doi.org/10.1073/pnas.0606291103, 2006.
Harder, W. and Dijkhuizen, L.: Physiological responses to nutrient limitation, Annu. Rev. Microb., 37, 1–23, 1983.
Harris, I., Jones, P. D., Osborn, T. J., and Lister, D. H.: Updated high-resolution grids of monthly climatic observations – the CRU TS3.10 Dataset, Int. J. Climatol., 34, 623–642, https://doi.org/10.1002/joc.3711, 2014.
He, Y., Yang, J., Zhuang, Q., Harden, J. W., McGuire, A. D., Liu, Y., Wang, G., and Gu, L.: Incorporating microbial dormancy dynamics into soil decomposition models to improve quantification of soil carbon dynamics of northern temperate forests, J. Geophys. Res.-Biogeo., 120, 2596–2611, https://doi.org/10.1002/2015jg003130, 2015.
Hiller, R. V., McFadden, J. P., and Kljun, N.: Interpreting CO2 Fluxes Over a Suburban Lawn: The Influence of Traffic Emissions, Bound.-Lay. Meteorol., 138, 215–230, https://doi.org/10.1007/s10546-010-9558-0, 2010.
Houghton, R. A.: Balancing the Global Carbon Budget, Annu. Rev. Earth Pl. Sc., 35, 313–347, https://doi.org/10.1146/annurev.earth.35.031306.140057, 2007.
Hugelius, G., Strauss, J., Zubrzycki, S., Harden, J. W., Schuur, E. A. G., Ping, C.-L., Schirrmeister, L., Grosse, G., Michaelson, G. J., Koven, C. D., O'Donnell, J. A., Elberling, B., Mishra, U., Camill, P., Yu, Z., Palmtag, J., and Kuhry, P.: Estimated stocks of circumpolar permafrost carbon with quantified uncertainty ranges and identified data gaps, Biogeosciences, 11, 6573–6593, https://doi.org/10.5194/bg-11-6573-2014, 2014.
Jenkins, J. P., Richardson, A. D., Braswell, B. H., Ollinger, S. V., Hollinger, D. Y., and Smith, M. L.: Refining light-use efficiency calculations for a deciduous forest canopy using simultaneous tower-based carbon flux and radiometric measurements, Agr. Forest Meteorol., 143, 64–79, https://doi.org/10.1016/j.agrformet.2006.11.008, 2007.
Kaiser, C., Franklin, O., Dieckmann, U., and Richter, A.: Microbial community dynamics alleviate stoichiometric constraints during litter decay, Ecol. Lett., 17, 680–690, https://doi.org/10.1111/ele.12269, 2014.
Knorr, W., Prentice, I. C., House, J. I., and Holland, E. A.: Long-term sensitivity of soil carbon turnover to warming, Nature, 433, 298–301, https://doi.org/10.1038/nature03226, 2005.
Lawrence, C. R., Neff, J. C., and Schimel, J. P.: Does adding microbial mechanisms of decomposition improve soil organic matter models?, A comparison of four models using data from a pulsed rewetting experiment, Soil Biol. Biochem., 41, 1923–1934, https://doi.org/10.1016/j.soilbio.2009.06.016, 2009.
Lennon, J. T. and Jones, S. E.: Microbial seed banks: the ecological and evolutionary implications of dormancy, Nature reviews, Microbiology, 9, 119–130, 10.1038/nrmicro2504, 2011.
Lloyd, A. H., Rupp, T. S., Fastie, C. L., and Starfield, A. M.: Patterns and dynamics of treeline advance on the Seward Peninsula, Alaska, J. Geophys. Res., 108, 8161, https://doi.org/10.1029/2001jd000852, 2002.
Manzoni, S., Taylor, P., Richter, A., Porporato, A., and Agren, G. I.: Environmental and stoichiometric controls on microbial carbon-use efficiency in soils, New Phytol., 196, 79–91, https://doi.org/10.1111/j.1469-8137.2012.04225.x, 2012.
McEwing, K. R., Fisher, J. P., and Zona, D.: Environmental and vegetation controls on the spatial variability of CH4 emission from wet-sedge and tussock tundra ecosystems in the Arctic, Plant Soil, 388, 37–52, https://doi.org/10.1007/s11104-014-2377-1, 2015.
McGuire, A. D., Melillo, J. M., Joyce, L. A., Kicklighter, D. W., Grace, A. L., III, B. M., and Vorosmarty, C. J.: Interactions between carbon and nitrogen dynamics in estimating net primary productivity for potential vegetation in North America, Global Biogeochem. Cy., 6, 101–124, 1992.
McGuire, A. D., Melillo, J. M., Kicklighter, D. W., and Joyce, L. A.: Equilibrium responses of soil carbon to climate change: Empirical and process-based estimates, J. Biogeogr., 22, 785–796, 1995.
McGuire, A. D. and Hobbie, J. E.: Global climate change and the equilibrium responses of carbon storage in arctic and subarctic regions, in: Modeling the Arctic system: A workshop report on the state of modeling in the Arctic System Science program, 53–54, 1997.
McGuire, A. D., Anderson, L. G., Christensen, T. R., Dallimore, S., Guo, L., Hayes, D. J., Heimann, M., Lorenson, T. D., Macdonald, R. W., and Roulet, N.: Sensitivity of the carbon cycle in the Arctic to climate change, Ecol. Monogr., 79, 523–555, 2009.
McGuire, K. L. and Treseder, K. K.: Microbial communities and their relevance for ecosystem models: Decomposition as a case study, Soil Biol. Biochem., 42, 529–535, https://doi.org/10.1016/j.soilbio.2009.11.016, 2010.
Melillo, J. M., McGuire, A. D., Kicklighter, D. W., Moore III, B., Vorosmarty, C. J., and Schloss, A. L.: Global climate change and terrestrial net primary production, Nature, 363, 234–240, https://doi.org/10.1038/363234a0, 1993.
Merbold, L., Kutsch, W. L., Corradi, C., Kolle, O., Rebmann, C., Stoy, P. C., Zimov, S. A., and Schulze, E. D.: Artificial drainage and associated carbon fluxes (CO2∕CH4) in a tundra ecosystem, Glob. Change Biol., 15, 2599–2614, https://doi.org/10.1111/j.1365-2486.2009.01962.x, 2009.
Moorhead, D. L. and Sinsabaugh, R. L.: A theoretical model of litter decay and microbial interaction, Ecol. Monogr., 76, 151–174, 2006.
Oechel, W. C., Laskowski, C. A., Burba, G., Gioli, B., and Kalhori, A. A. M.: Annual patterns and budget of CO2flux in an Arctic tussock tundra ecosystem, J. Geophys. Res.-Biogeo., 119, 323–339, https://doi.org/10.1002/2013jg002431, 2014.
Parton, W. J., Scurlock, J. M. O., Ojima, D. S., Gilmanov, T. G., Scholes, R. J., Schimel, D. S., Kirchner, T., Menaut, J. C., Seastedt, T., Moya, E. G., Kamnalrut, A., and Kinyamario, J. I.: Observations and modeling of biomass and soil organic matter dynamics for the grassland biome worldwide, Global Biogeochem. Cy., 7, 785–809, 1993.
Raich, J. W., Rastetter, E. B., Melillo, J. M., Kicklighter, D. W., Steudler, P. A., Peterson, B. J., Grace, A. L., Moore, B., and Vorosmary, C. J.: Potential net primary productivity in South America: application of a global model, Ecol. Appl., 1, 399–429, https://doi.org/10.2307/1941899, 1991.
Richardson, A. D., Jenkins, J. P., Braswell, B. H., Hollinger, D. Y., Ollinger, S. V., and Smith, M. L.: Use of digital webcam images to track spring green-up in a deciduous broadleaf forest, Oecologia, 152, 323–334, https://doi.org/10.1007/s00442-006-0657-z, 2007.
Running, S. W. and Coughlan, J. C.: A general model of forest ecosystem processes for regional applications I. Hydrologic balance, canopy gas exchange and primary production processes, Ecol. Modell., 42, 125–154, 1988.
Salazar, A., Sulman, B. N., and Dukes, J. S.: Microbial dormancy promotes microbial biomass and respiration across pulses of drying-wetting stress, Soil Biol. Biochem., 116, 237–244, https://doi.org/10.1016/j.soilbio.2017.10.017, 2018.
Schimel, D. S.: Terrestrial ecosystems and the carbon cycle, Glob. Change Biol., 1, 77–91, 1995.
Schimel, D. S., House, J. I., Hibbard, K. A., Bousquet, P., Ciais, P., Peylin, P., Braswell, B. H., Apps, M. J., Baker, D., Bondeau, A., Canadell, J., Churkina, G., Cramer, W., Denning, A. S., Field, C. B., Friedlingstein, P., Goodale, C., Heimann, M., Houghton, R. A., Melillo, J. M., III, B. M., Murdiyarso, D., Noble, I., Pacala, S. W., Prentice, I. C., Raupach, M. R., Rayner, P. J., Scholes, R. J., Steffen, W. L., and Wirth, C.: Recent patterns and mechanisms of carbon exchange by terrestrial ecosystems, Nature, 414, 169–172, https://doi.org/10.1038/3510250, 2001.
Schimel, J.: Microbes and global carbon, Nat. Clim. Change, 3, 867–868, https://doi.org/10.1038/nclimate2015, 2013.
Schimel, J. P. and Weintraub, M. N.: The implications of exoenzyme activity on microbial carbon and nitrogen limitation in soil: a theoretical model, Soil Biol. Biochem., 35, 549–563, https://doi.org/10.1016/s0038-0717(03)00015-4, 2003.
Schimel, J. P. and Schaeffer, S. M.: Microbial control over carbon cycling in soil, Front. Microb., 3, 348, https://doi.org/10.3389/fmicb.2012.00348, 2012.
Schmidt, M. W., Torn, M. S., Abiven, S., Dittmar, T., Guggenberger, G., Janssens, I. A., Kleber, M., Kogel-Knabner, I., Lehmann, J., Manning, D. A., Nannipieri, P., Rasse, D. P., Weiner, S., and Trumbore, S. E.: Persistence of soil organic matter as an ecosystem property, Nature, 478, 49–56, https://doi.org/10.1038/nature10386, 2011.
Stolpovsky, K., Martinez-Lavanchy, P., Heipieper, H. J., Van Cappellen, P., and Thullner, M.: Incorporating dormancy in dynamic microbial community models, Ecol. Modell., 222, 3092–3102, https://doi.org/10.1016/j.ecolmodel.2011.07.006, 2011.
Stow, D. A., Hope, A., McGuire, D., Verbyla, D., Gamon, J., Huemmrich, F., Houston, S., Racine, C., Sturm, M., Tape, K., Hinzman, L., Yoshikawa, K., Tweedie, C., Noyle, B., Silapaswan, C., Douglas, D., Griffith, B., Jia, G., Epstein, H., Walker, D., Daeschner, S., Petersen, A., Zhou, L., and Myneni, R.: Remote sensing of vegetation and land-cover change in Arctic Tundra Ecosystems, Remote Sens. Environ., 89, 281–308, https://doi.org/10.1016/j.rse.2003.10.018, 2004.
Strickland, M. S., Lauber, C., Fierer, N., and Bradford, M. A.: Testing the functional signi?canceof microbial community composition, Ecology, 90, 441–451, 2009.
Tang, J. and Zhuang, Q.: Equifinality in parameterization of process-based biogeochemistry models: A significant uncertainty source to the estimation of regional carbon dynamics, J. Geophys. Res., 113, G04010, https://doi.org/10.1029/2008JG000757, 2008.
Tang, J. and Zhuang, Q.: A global sensitivity analysis and Bayesian inference framework for improving the parameter estimation and prediction of a process-based Terrestrial Ecosystem Model, J. Geophys. Res., 114, D15303, https://doi.org/10.1029/2009JD011724, 2009.
Tang, J. and Riley, W. J.: Weaker soil carbon–climate feedbacks resulting from microbial and abiotic interactions, Nat. Clim. Change, 5, 56–60, https://doi.org/10.1038/nclimate2438, 2014.
Tape, K. E. N., Sturm, M., and Racine, C.: The evidence for shrub expansion in Northern Alaska and the Pan-Arctic, Glob. Change Biol., 12, 686–702, https://doi.org/10.1111/j.1365-2486.2006.01128.x, 2006.
Tarnocai, C., Canadell, J. G., Schuur, E. A. G., Kuhry, P., Mazhitova, G., and Zimov, S.: Soil organic carbon pools in the northern circumpolar permafrost region, Global Biogeochem. Cy., 23, GB2023, https://doi.org/10.1029/2008gb003327, 2009.
Thullner, M., Van Cappellen, P., and Regnier, P.: Modeling the impact of microbial activity on redox dynamics in porous media, Geochim. Cosmochim. Ac., 69, 5005–5019, https://doi.org/10.1016/j.gca.2005.04.026, 2005.
Todd-Brown, K. E. O., Hopkins, F. M., Kivlin, S. N., Talbot, J. M., and Allison, S. D.: A framework for representing microbial decomposition in coupled climate models, Biogeochemistry, 109, 19–33, https://doi.org/10.1007/s10533-011-9635-6, 2011.
Todd-Brown, K. E. O., Randerson, J. T., Post, W. M., Hoffman, F. M., Tarnocai, C., Schuur, E. A. G., and Allison, S. D.: Causes of variation in soil carbon simulations from CMIP5 Earth system models and comparison with observations, Biogeosciences, 10, 1717–1736, https://doi.org/10.5194/bg-10-1717-2013, 2013.
Treseder, K. K., Balser, T. C., Bradford, M. A., Brodie, E. L., Dubinsky, E. A., Eviner, V. T., Hofmockel, K. S., Lennon, J. T., Levine, U. Y., MacGregor, B. J., Pett-Ridge, J., and Waldrop, M. P.: Integrating microbial ecology into ecosystem models: challenges and priorities, Biogeochemistry, 109, 7–18, https://doi.org/10.1007/s10533-011-9636-5, 2011.
Wang, G., Mayes, M. A., Gu, L., and Schadt, C. W.: Representation of Dormant and Active Microbial Dynamics for Ecosystem Modeling, Public Library of Science, 9, e89252, https://doi.org/10.1371/journal.pone.0089252.g001, 2014.
Wang, G., Jagadamma, S., Mayes, M. A., Schadt, C. W., Steinweg, J. M., Gu, L., and Post, W. M.: Microbial dormancy improves development and experimental validation of ecosystem model, ISME J., 9, 226–237, https://doi.org/10.1038/ismej.2014.120, 2015.
Wang, W., Feng, J., and Oikawa, T.: Contribution of Root and Microbial Respiration to Soil CO2 Efflux and Their Environmental Controls in a Humid Temperate Grassland of Japan, Pedosphere, 19, 31–39, 2009.
Werf, H. V. D. and Verstraete, W.: Estimation of active soil microbial biomass by mathematical analysis of respiration curves: relation to conventional estimation of total biomass, Soil Biol. Biochem., 19, 267–271, 1987.
White, A., Cannell, M. G. R., and Friend, A. D.: The high-latitude terrestrial carbon sink: a model analysis Glob. Change Biol., 6, 227–245, 2000.
Wieder, W. R., Allison, S. D., Davidson, E. A., Georgiou, K., Hararuk, O., He, Y., Hopkins, F., Luo, Y., Smith, M. J., Sulman, B., Todd-Brown, K., Wang, Y. P., Xia, J., and Xu, X.: Explicitly representing soil microbial processes in Earth system models, Global Biogeochem. Cy., 29, 1782–1800, https://doi.org/10.1002/2015GB005188, 2015.
Wieder, W. R., Bonan, G. B., and Allison, S. D.: Global soil carbon projections are improved by modelling microbial processes, Nat. Clim. Change, 3, 909–912, https://doi.org/10.1038/nclimate1951, 2013.
Xu, X., Schimel, J. P., Thornton, P. E., Song, X., Yuan, F., and Goswami, S.: Substrate and environmental controls on microbial assimilation of soil organic carbon: a framework for Earth system models, Ecol. Lett., 17, 547–555, https://doi.org/10.1111/ele.12254, 2014.
Zha, J. and Zhuang, Q.: Microbial decomposition processes and vulnerable arctic soil organic carbon in the 21st century, Biogeosciences, 15, 5621–5634, https://doi.org/10.5194/bg-15-5621-2018, 2018.
Zhou, L., Tucker, C. J., Kaufmann, R. K., Slayback, D., Shabanov, N. V., and Myneni, R. B.: Variations in northern vegetation activity inferred from satellite data of vegetation index during 1981 to 1999, J. Geophys. Res.-Atmos., 106, 20069–20083, https://doi.org/10.1029/2000jd000115, 2001.
Zhuang, Q., Romanovsky, V. E., and McGuire, A. D.: Incorporation of a permafrost model into a large-scale ecosystem model: Evaluation of temporal and spatial scaling issues in simulating soil thermal dynamics, J. Geophys. Res.:-Atmos., 106, 33649–33670, https://doi.org/10.1029/2001jd900151, 2001.
Zhuang, Q., McGuire, A. D., O'Neill, K. P., Harden, J. W., Romanovsky, V. E., and Yarie, J.: Modeling soil thermal and carbon dynamics of a fire chronosequence in interior Alaska, J. Geophys. Res., 108, FFR 3-1-FFR-3-26, https://doi.org/10.1029/2001jd001244, 2002.
Zhuang, Q., He, J., Lu, Y., Ji, L., Xiao, J., and Luo, T.: Carbon dynamics of terrestrial ecosystems on the Tibetan Plateau during the 20th century: An analysis with a process-based biogeochemical model, Glob. Ecol. Biogeogr., 19, 649–662, https://doi.org/10.1111/j.1466-8238.2010.00559.x, 2010.
Zhuang, Q., Zhu, X., He, Y., Prigent, C., Melillo, J. M., David McGuire, A., Prinn, R. G., and Kicklighter, D. W.: Influence of changes in wetland inundation extent on net fluxes of carbon dioxide and methane in northern high latitudes from 1993 to 2004, Environ. Res. Lett., 10, 095009, https://doi.org/10.1088/1748-9326/10/9/095009, 2015.
Zhuang, Q., McGuire, A. D., Melillo, J. M., Clein, J. S., Dargaville, R. J., Kicklighter, D. W., Myneni, R. B., Dong, J., Romanovsky, V. E., Harden, J., and Hobbie, J. E.: Carbon cycling in extratropical terrestrial ecosystems of the Northern Hemisphere during the 20th century: a modeling analysis of the influences of soil thermal dynamics, Tellus B, 55, 751–776, https://doi.org/10.3402/tellusb.v55i3.16368, 2016.