the Creative Commons Attribution 4.0 License.
the Creative Commons Attribution 4.0 License.
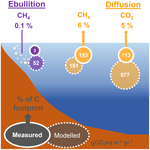
The carbon footprint of a Malaysian tropical reservoir: measured versus modelled estimates highlight the underestimated key role of downstream processes
Yves T. Prairie
Reservoirs are important sources of greenhouse gases (GHGs) to the atmosphere, and their number is rapidly increasing, especially in tropical regions. Accurately predicting their current and future emissions is essential but hindered by fragmented data on the subject, which often fail to include all emission pathways (surface diffusion, ebullition, degassing, and downstream emissions) and the high spatial and temporal flux variability. Here we conducted a comprehensive sampling of Batang Ai reservoir (Malaysia), and compared field-based versus modelled estimates of its annual carbon footprint for each emission pathway. Carbon dioxide (CO2) and methane (CH4) surface diffusion were higher in upstream reaches. Reducing spatial and temporal sampling resolution resulted in up to a 64 % and 33 % change in the flux estimate, respectively. Most GHGs present in discharged water were degassed at the turbines, and the remainder were gradually emitted along the outflow river, leaving time for CH4 to be partly oxidized to CO2. Overall, the reservoir emitted 2475 , with 89 % occurring downstream of the dam, mostly in the form of CH4. These emissions, largely underestimated by predictions, are mitigated by CH4 oxidation upstream and downstream of the dam but could have been drastically reduced by slightly raising the water intake elevation depth. CO2 surface diffusion and CH4 ebullition were lower than predicted, whereas modelled CH4 surface diffusion was accurate. Investigating latter discrepancies, we conclude that exploring morphometry, soil type, and stratification patterns as predictors can improve modelling of reservoir GHG emissions at local and global scales.
- Article
(1215 KB) - Full-text XML
-
Supplement
(1071 KB) - BibTeX
- EndNote
Reservoirs provide a variety of services to humans (water supply, navigation, flood control, hydropower) and cover an estimated area exceeding 0.3 million km2 globally (Lehner et al., 2011). This area is increasing, with an expected rapid growth of the hydroelectric sector in the next two decades (International Hydropower Association (IHA), 2015), mainly in tropical and subtropical regions (Zarfl et al., 2015). The flooding of terrestrial landscapes can transform them into significant greenhouse gas (GHG) sources to the atmosphere (Prairie et al., 2018; Rudd et al., 1993; Teodoru et al., 2012). While part of reservoir GHG emissions would occur naturally (not legitimately attributable to damming), the remainder results from newly created environments favouring carbon (C) mineralization, particularly methane (CH4) production (flooded organic-rich anoxic soils) (Prairie et al., 2018). Field studies have revealed a wide range in measured fluxes, with spatial and temporal variability sometime spanning several orders of magnitude within a single reservoir (Paranaíba et al., 2018; Sherman and Ford, 2011). Moreover, reservoirs can emit GHG through several pathways: through diffusion of gas at the air–water interface (surface diffusion); through the release of gas bubbles formed in the sediments (ebullition); for some reservoirs (mostly hydroelectric) through gas release following pressure drop upon water discharge (degassing); and through evasion of the remaining excess gas in the outflow river (downstream emissions). The relative contribution of these flux pathways to total emissions is extremely variable. While surface diffusion is the most frequently sampled, it is often not the main emission pathway (Demarty and Bastien, 2011). Indeed, measured ebullition, degassing, and downstream emissions range from negligible to several orders of magnitude higher than surface diffusion in different reservoirs (Bastien and Demarty, 2013; DelSontro et al., 2010; Galy-Lacaux et al., 1997; Keller and Stallard, 1994; Kemenes et al., 2007; Teodoru et al., 2012; Venkiteswaran et al., 2013), making it a challenge to model total reservoirs GHG emissions.
Literature syntheses over the past 20 years have yielded highly variable global estimates of reservoirs GHG footprint, ranging from 0.5 to 2.3 Pg CO2 eq yr−1 (Barros et al., 2011; Bastviken et al., 2011; Deemer et al., 2016; St. Louis et al., 2000). These estimates are based on global extrapolations of averages of sampled systems, representing an uneven spatial distribution biased toward North America and Europe, as well as an uneven mixture of emission pathways. Recent studies have highlighted the lack of spatial and temporal resolution as well as the frequent absence of some flux pathways (especially degassing, downstream, and N2O emissions) in most reservoir GHG assessments (Beaulieu et al., 2016; Deemer et al., 2016). More recently, studies have focused on identifying drivers of reservoir GHG flux variability. Using global empirical data, Barros et al. (2011) proposed the first quantitative models for reservoir carbon dioxide (CO2) and CH4 surface diffusion as a negative function of reservoir age, latitude, and mean depth (for CO2 only) and a positive function of dissolved organic carbon (DOC) inputs (Barros et al., 2011). An online tool (G-res) for predicting reservoir CO2 and CH4 emissions was later developed on the basis of a similar empirical modelling approach of measured reservoir fluxes with globally available environmental data (UNESCO/IHA, 2017). Modelling frameworks to predict GHG emissions from existing and future reservoirs are essential tools for reservoir management. However, their accuracy is directly related to available information and inherently affected by gaps and biases of the published literature. For example, while the G-res model predicts reservoir CO2 and CH4 surface diffusion as well as CH4 ebullition and degassing on the basis of temperature, age, percent of littoral zone, and soil organic C, it does not consider N2O emissions, CO2 degassing, and downstream emissions due to scarcity of data. Overall, the paucity of comprehensive empirical studies limits our knowledge of reservoir GHG dynamics at a local scale, introducing uncertainties in large-scale estimates and hindering model development.
The research reported here focuses on building a comprehensive assessment of GHG fluxes of Batang Ai, a tropical reservoir in Southeast Asia (Malaysia), over four sampling campaigns spanning 2 years with an extensive spatial coverage. The main objective of this study is to provide a comprehensive account of CO2, CH4, and N2O fluxes from surface diffusion, ebullition, degassing, and downstream emissions (accounting for riverine CH4 oxidation) to better understand what shapes their relative contributions and their potential mitigation. The second objective is to compare our measured values with modelled estimates from each pathway and gas species to locate where the largest discrepancies are and thereby identify research avenues for improving the current modelling framework.
2.1 Study site and sampling campaigns
Batang Ai is a hydroelectric reservoir located on the island of Borneo in the Sarawak province of Malaysia (latitude 1.16∘ and longitude 111.9∘). The regional climate is tropical equatorial with a relatively constant temperature throughout the year, on average 23 ∘C in the morning to 32 ∘C during the day. Annual rainfall varies between 3300 and 4600 mm with two monsoon seasons: November to February (northeast monsoon) and June to October (southwest) (Sarawak Government, 2019). Batang Ai reservoir was impounded in 1985 with no prior clearing of the vegetation and has a dam wall of 85 m in height, a mean depth of 34 m, and a total area of 68.4 km2. The reservoir catchment consists of 1149 km2 of mostly forested land where human activities are limited to a few traditional habitations and associated croplands, as well as localized aquaculture sites within the reservoir main basin. The reservoir has two major inflows: the Batang Ai and Engkari rivers, which flow into two reservoir branches merging upstream of the main reservoir basin (Fig. 1). Four sampling campaigns were conducted: (1) 14 November to 5 December 2016 (November–December 2016), (2) 19 April to 3 May 2017 (April–May 2017), (3) 28 February to 13 March 2018 (February–March 2018), and (4) 12 to 29 August 2018 (August 2018).
2.2 Water chemistry
Samples for DOC, total phosphorus (TP), total nitrogen (TN), and chlorophyll a (Chl a) analyses were collected from the water surface (<0.5 m) at all surface diffusion sampling sites shown in Fig. 1 and during each campaign. For TP and TN, we collected non-filtered water in acid-washed glass vials stored at 4 ∘C until analysis. TP was measured by spectrophotometry using the standard molybdenum blue method after persulfate digestion at 121 ∘C for 20 min and a calibration with standard solutions from 10 to 100 µg L−1 with a 5 % precision (Wetzel and Likens, 2000). TN analyses were performed by alkaline persulfate digestion to NO3, subsequently measured on a flow Alpkem analyser (OI Analytical Flow Solution 3100) calibrated with standard solutions from 0.05 to 2 mg L−1 with a 5 % precision (Patton and Kryskalla, 2003). Water filtered at 0.45 µm was used for DOC analysis with a total organic carbon analyser 1010-OI following sodium persulfate digestion and calibrated with standard solutions from 1 to 20 mg L−1 with a 5 % precision (detection limit of 0.1 mg L−1). Chl a was analysed through spectrophotometry following filtration on Whatman (GF/F) filters and extraction by hot 90 % ethanol solution (Sartory and Grobbelaar, 1984).
2.3 Surface diffusion
Surface diffusion is the flux of gas between the water surface and the air driven by a gradient in gas partial pressure. Surface diffusion of CO2 and CH4 to the atmosphere was measured at 36 sites in the reservoir and 3 sites in the inflow rivers (Fig. 1), and sampling of the same sites was repeated in each campaign (with a few exceptions). Fluxes were measured using a static airtight floating chamber connected in a closed loop to an ultraportable gas analyser (UGGA from LGR). Surface diffusion rates (Fgas) were derived from the linear change in CO2 and CH4 partial pressures (continuously monitored at 1 Hz for a minimum of 5 min) through time inside the chamber using the following Eq. (1):
where s is the gas accumulation rate in the chamber, V=25 L the chamber volume, A=0.184 m2 the chamber surface area, and m the gas molar volume at current atmospheric pressure.
N2O surface diffusion was estimated at seven of the sampled sites (Fig. 1) using the following Eq. (2) (Lide, 2005):
where kgas is the gas exchange coefficient, Cgas is the gas concentration in the water, and Ceq is the theoretical gas concentration at equilibrium given measured water temperature, atmospheric pressure, and ambient gas concentration. was measured using the headspace technique, with a 1.12 L sealed glass serum bottle containing surface water and a 0.12 L headspace of ambient air. After shaking the bottle for 2 min to achieve air–water equilibrium, the headspace gas was extracted from the bottle with an airtight syringe and injected in the previously evacuated 9 mL glass vial capped with an airtight butyl stopper and aluminium seal. Three analytical replicates and a local sample of ambient air were taken at each site and analysed by gas chromatography using a Shimadzu GC-2040, with a Poropaq Q column to separate gases and an electron capture detector (ECD) calibrated with 0.3, 1, and 3 ppm of N2O certified standard gas. After analysis the original N2O concentration of the water was back-calculated based on the water temperature before and after shaking (for gas solubility), the ambient atmospheric pressure, the ratio of water to air in the sampling bottle, and the headspace N2O concentration before shaking. was derived from measured values obtained by rearranging Eq. (2) for CH4, with known values of Fgas, Cgas, and Ceq. The to transformation was done using the following Eq. (3) (Cole and Caraco, 1998; Ledwell, 1984):
where Sc is the gas Schmidt number (Wanninkhof, 1992).
CH4 and CO2 concentrations in the water were measured using the headspace technique. Surface water was collected in a 60 mL gas-tight plastic syringe in which a 30 mL headspace was created (using either ambient air or carbon-free air). The syringe was shaken for 2 min to achieve gas equilibrium between air and water. The gas phase was then injected in a 12 mL airtight pre-evacuated vial and subsequently analysed through manual injection on a Shimadzu GC-8A gas chromatograph with a flame ionization detector following a calibration curve with certified gas standards (0–10 000 ppm for CO2 and 0–50 000 ppm for CH4). The samples were also analysed for isotopic δ13CO2 and δ13CH4 signatures by manually injecting 18 mL of gas in a cavity ring-down spectrometer (CRDS) equipped with a small sample isotopic module (SSIM A0314, Picarro G2201-i analyser) set in a non-continuous mode with a three-point calibration curve based on certified gas standards (−40 ‰, −3.9 ‰, and 25.3 ‰ for δ13CO2, and −66.5 ‰, −38.3 ‰, and −23.9 ‰ for δ13CH4).
2.4 Ebullition
Ebullition is the process through which gas bubbles formed in the sediments rise through the water column and are released to the atmosphere. Sediment gas ebullition was measured at four sites in the reservoir and two sites in the inflows (Fig. 1) by deploying 0.785 m2 underwater inverted funnel traps at 2 to 3 m deep for approximately 20 d in the reservoir and 1 h in the inflows. The top part of a closed plastic syringe was fixed to the narrow end of the funnel trap where the emerging bubbles accumulated. Upon recovery, bubble gas volume was measured, collected from the syringe, and injected in 12 mL pre-evacuated airtight vials for CO2 and CH4 concentration analyses (using the aforementioned method). Ebullition rate was calculated assuming the original bubble composition was similar to bubbles collected almost right after ascent in the inflows sites, which was 100 % CH4. Hence we considered CO2 and N2O ebullition to be null.
In order to estimate the potential for sediment accumulation fuelling ebullition in the littoral zone, we calculated the mud energy boundary depth (EBD in metres, below which fine-grained sediment accumulation occurs) using the reservoir surface area (E in km2) as the exposure parameter in the following Eq. (4) (Rowan et al., 1992):
Table 1CO2 and CH4 dynamics downstream of the dam: gas export rate from upstream to downstream of the dam, degassing, result of CH4 oxidation (CO2 production and CH4 consumption), downstream emissions, and total emissions to the atmosphere below the dam. Uncertainties based on variation coefficients are reported in parentheses. Units are in of reservoir surface area.
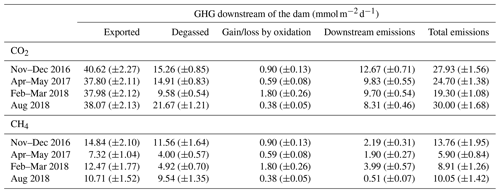
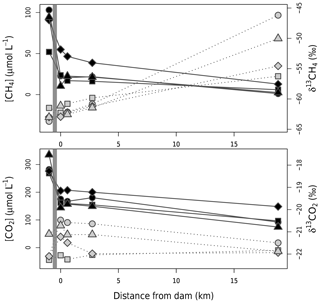
Figure 3Concentrations (black symbols and solid line) and δ13C (grey symbols and dotted lines) of CO2 and CH4 from right upstream of the dam (grey band) to 19 km downstream in the outflow river. Circles, squares, diamonds, and triangles represent values from November–December 2016, April–May 2017, February–Mar 2018, and August 2018 respectively.
2.5 Degassing, downstream emissions, and CH4 oxidation
Degassing of CO2 and CH4 right after water discharge (Fdeg) and downstream emissions of the remaining reservoir-derived GHGs in the outflow river (Fdwn) were calculated using the following Eqs. (5) and (6):
where Q is the water discharge and Cup, C0, and C19 the measured gas concentrations upstream of the dam at the water withdrawal depth, at the powerhouse right after water discharge, and in the outflow 19 km downstream of the dam respectively. Cox is the net change in gas concentration due to oxidation (loss for CH4 and gain for CO2). For downstream emissions, we considered that, after a river stretch of 19 km, all excess gas originating from the reservoir was evaded and gas concentration was representative of the outflow river baseline. This assumption potentially underestimates actual downstream emissions (in the case of remaining excess gas after 19 km). However, given the observed exponential decrease in gas concentration along the outflow (Fig. 3), emissions after 19 km are expected to be small compared to those in the 0 to 19 km river stretch, consistent with observations in other reservoirs (Guérin et al., 2006; Kemenes et al., 2007).
Gas concentrations upstream and downstream of the dam were obtained by measuring, in each campaign, CO2 and CH4 concentrations in a vertical profile right upstream of the dam at a 1 to 3 m interval from 0 to 32 m and at four locations in the outflow: at 0 (power house), 0.6, 2.7, and 19 km downstream of the dam (Fig. 1). Sampling was done using a multi-parameter probe equipped with depth, oxygen, and temperature sensors (Yellow Spring Instruments, YSI model 600XLM-M) attached to a 12 V submersible Tornado pump (Proactive Environmental Products) for water collection. Gas concentration and δ13C were measured as described in Sect. 2.3. Water withdrawal depth ranged from 20 to 23 m and was estimated based on known values of elevations of water intake and water level compared to sea level. Gas concentration in the water exiting the reservoir was defined as the average measured gas concentrations in the ±1 m range of the withdrawal depth.
Estimates of downstream CH4 oxidation were obtained, for each sampling campaign, by calculating the fraction of CH4 oxidized (Fox) using the following Eq. (7):
Equation (7) is based on a non-steady-state isotopic model developed considering evasion (emission to the atmosphere) and oxidation as the two loss processes for CH4 in the outflow river, assuming negligible isotopic fractionation for evasion (Knox et al., 1992) and a fractionation of α=1.02 for oxidation (Coleman et al., 1981) (see derivation in Supplement). [CH4]source, [CH4]resid, δ13CH4source, and δ13CH4resid are the concentrations of CH4 and their corresponding isotopic signatures at the beginning of the outflow (km 0) and 19 km downstream, representing the source and residual pools of CH4 respectively. The amount of CH4 oxidized to CO2 along the 19 km of river stretch for each sampling campaign was calculated as the product of Fox and [CH4]source. The resulting loss of CH4 and gain of CO2 in the outflow were accounted for in downstream emissions (Cox in Eq. 6). Note that downstream N2O emissions were considered null since N2O concentrations measured in the deep reservoir layer were lower than concentrations in the outflow.
2.6 Ecosystem-scale C footprint
Batang Ai annual C footprint was calculated as the sum of surface diffusion, ebullition, degassing, and downstream emissions of CO2, CH4, and N2O considering a greenhouse warming potential of 1, 34, and 298 respectively over a 100-year lifetime period (Myhre et al., 2013). For each flux pathway, annual flux was estimated as the average of the sampling campaigns. Ecosystem-scale estimate of surface diffusion was calculated for each campaign as the average of measured flux rates applied to the reservoir area for N2O, and for CO2 and CH4 it was obtained by spatial interpolation of measured fluxes over the reservoir area based on inverse distance weighting with a power of two (a power of one yields similar averages, CV <11 %) using package gstat version 1.1–6 in the R version 3.4.1 software (Pebesma, 2004; R Core Team, 2017). Ebullition at the reservoir scale was calculated as the average of measured reservoir ebullition rates applied to the littoral area (<3 m deep).
The estimated GHG emissions of Batang Ai based on measured data was compared to values derived from the G-res model (UNESCO/IHA, 2017) and the model presented in Barros et al. (2011). Both models predict surface CO2 and CH4 diffusion as a function of age and account for the effect of temperature using different proxies: the G-res uses effective temperature while the Barros et al. model uses latitude (an indirect proxy that integrates other spatial differences). In terms of CO2 surface diffusion, the G-res uses reservoir area, soil C content, and TP to quantify the effect of C inputs fuelling CO2 production, while the Barros et al. model uses DOC inputs directly (based on in situ DOC concentration). For CH4 surface diffusion, both models account for morphometry using the fraction of littoral area (G-res) or the mean depth (Barros et al. model). Overall, both models predict surface diffusion based on the same conceptual framework but use different proxies. CH4 ebullition and degassing are modelled only by the G-res, being the sole model available to this date. Details on model equations and input variables are presented in the Supplement (Tables S2 and S3).
3.1 Water chemistry
The reservoir is stratified throughout the year with a thermocline at a depth around 13 m and mostly anoxic conditions in the hypolimnion of the main basin (Fig. S1 in the Supplement). The system is oligotrophic, with very low concentrations of DOC, TP, TN, and Chl a averaging 0.9 (SD±0.2) mg L−1, 5.9 (SD±2.4) µg L−1, 0.11 (SD±0.04) mg L−1, and 1.3 (SD±0.7) µg L−1 respectively (Table S1) and high water transparency (Secchi depth >5 m). In the reservoir inflows, concentrations of measured chemical species are slightly higher but still in the oligotrophic range (Table S1); however, the transparency is much lower due to turbidity (Secchi <0.5 m). The oligotrophic status of the reservoir likely results from nutrient-poor soils (Wasli et al., 2011) and a largely undisturbed forested catchment in the protected Batang Ai National Park. The reservoir's low Chl a concentrations are comparable to the neighbouring Bakun reservoir (Ling et al., 2017), and its DOC concentrations are on the low end of the wide range of measured values in nearby rivers (Martin et al., 2018).
3.2 Surface diffusion
Measured CO2 diffusion in the reservoir averaged 7.7 (SD±18.2) (Table S1), which is on the low end compared to other reservoirs (Deemer et al., 2016) and even to natural lakes (Sobek et al., 2005) but similar to CO2 fluxes measured in two reservoirs in Laos (Chanudet et al., 2011). CO2 diffusion across all sites ranged from substantial uptake to high emissions (from −30.8 to 593.9 , Table S1) reflecting a large spatial and temporal variability. Spatially, CO2 fluxes measured in the main basin and branches had similar averages of 7.9 and 7.3 respectively (overall SD±18.2), contrasting with higher and more variable values in the inflows with a mean of 137.3 (SD±192.4) (Fig. 2). Within the reservoir, CO2 fluxes varied (SD±18.2 ) but did not follow a consistent pattern and might reflect pre-flooding landscape heterogeneity (Teodoru et al., 2011). Temporally, highest average reservoir CO2 fluxes were measured in April–May 2017, when no CO2 uptake was observed, contrary to other campaigns, especially February–March and August 2018, when CO2 uptake was common (Fig. S2) and average Chl a concentrations were the highest. This reflects the important role of metabolism (namely CO2 consumption by primary production) in modulating surface CO2 fluxes in Batang Ai.
All CH4 surface diffusion measurements were positive and ranged from 0.03 to 113.4 (Table S1). Spatially, CH4 fluxes were progressively higher moving further upstream (Figs. 2 and S3), with decreasing water depth and increasing connection to the littoral. This gradient in morphometry induces an increasingly greater contact of the water with bottom and littoral sediments, where CH4 is produced, explaining the spatial pattern of CH4 fluxes. CH4 surface diffusion also varied temporally but to a lesser extent than CO2, being on average highest in August 2018 in the reservoir and in November–December 2016 in the inflows.
Reservoir N2O surface diffusion (measured with a limited spatial resolution) averaged −0.2 (SD±2.1) (Table S1). The negative value indicates that the system acts as a slight net sink of N2O, absorbing an estimated 2.1 (Table 2). Atmospheric N2O uptake has previously been reported in aquatic systems and linked to low oxygen and nitrogen content conducive to complete denitrification, which consumes N2O (Soued et al., 2016; Webb et al., 2019). These environmental conditions match observations in Batang Ai, with a low average TN concentration of 0.11 (0.04) mg L−1 (Table S1) and anoxic deep waters (Fig. S1).
3.3 Ebullition
We calculated that CH4 ebullition rates in Batang Ai's littoral area ranged from 0.02 to 0.84 , which contrasts with rates measured in its inflows, which are several orders of magnitude higher (52 to 103 ). Similar patterns were observed in other reservoirs, where inflow arms where bubbling hot spots due to a higher organic C supply driven by terrestrial matter deposition (DelSontro et al., 2011; Grinham et al., 2018). Since ebullition rates are notoriously heterogeneous and were measured at only four sites in the reservoir, they may not reflect ecosystem-scale rates. However, our attempt to manually provoke ebullition at several other sites (by physically disturbing the sediments) did not result in any bubble release, confirming the low potential for ebullition in the reservoir littoral zone. Moreover, we calculated that fine-grained sediment accumulation is unlikely at depths shallower than 9.7 m (estimated EBD) in Batang Ai. This, combined with the reservoir steep slope, prevents the sustained accumulation of organic material in littoral zones (Blais and Kalff, 1995), hence decreasing the potential for CH4 production and bubbling there. Also, apparent littoral sediment composition in the reservoir and dense clay with low porosity may further hinder bubble formation and emission (de Mello et al., 2018).
3.4 Degassing and downstream emissions
Emissions downstream of the dam, expressed on a reservoir-wide areal basis, ranged from 19.3 to 30.0 for CO2 and from 5.9 to 13.8 for CH4 (Table 1). The amount of CO2 exiting the reservoir varied little between sampling campaigns (CV=3 %), contrary to CH4 (CV=28 %, Table 1 and Fig. 3). Higher temporal variability of CH4 concentration in discharged water is likely modulated by microbial CH4 oxidation in the reservoir water column upstream of the dam. Evidence of high CH4 oxidation is apparent in reservoir water column profiles, showing a sharp decline of CH4 concentration and increase in δ13CH4 right around the water withdrawal depth (Fig. S1). This vertical pattern results from higher oxygen availability when moving up in the hypolimnion (Fig. S1), promoting CH4 oxidation at shallower depths.
Once GHGs have exited the reservoir, a large fraction (40 % and 65 % for CO2 and CH4 respectively) is immediately lost to the atmosphere as degassing emissions (Table 1), which is in line with previous literature reports (Kemenes et al., 2016). Along the outflow river, CO2 and CH4 concentrations gradually decreased, δ13CO2 remained stable, and δ13CH4 steadily increased (Fig. 3). Given the very small isotopic fractionation (0.9992) of CH4 during gas evasion (Knox et al., 1992), the only process that can explain the observed δ13CH4 increase is CH4 oxidation (Bastviken et al., 2002; Thottathil et al., 2018). We estimated that riverine CH4 oxidation ranged from 0.38 to 1.80 (expressed per squared metre of reservoir area for comparison), transforming 18 % to 32 % (depending on the sampling campaign) of the CH4 to CO2 within the first 19 km of the outflow. Riverine oxidation rates did not co-vary temporally with water temperature, oxygen availability, or CH4 concentrations (known as typical drivers; Thottathil et al., 2019); hence, they might be regulated by other factors like light and microbial assemblages (Murase and Sugimoto, 2005; Oswald et al., 2015). Overall, riverine oxidation of CH4 to CO2 (which has a 34 times lower warming potential) reduced radiative forcing of downstream emissions (excluding degassing) by, on average, 21 %, and the total annual reservoir C footprint by 7 %. Despite having a measurable impact on reservoir GHG emissions, CH4 oxidation downstream of dams was only considered in three other reservoirs to our knowledge (DelSontro et al., 2016; Guérin and Abril, 2007; Kemenes et al., 2007). Accounting for this process is particularly important in systems where downstream emissions are large, which is a common situation in tropical reservoirs (Demarty and Bastien, 2011). While additional data on the subject are needed, our results provide one of the first basis for understanding CH4 oxidation downstream of dams and eventually integrating this component to global models (from which it is currently absent).
3.5 Importance of sampling resolution
High spatial and temporal sampling resolution have been recently highlighted as an important but often lacking aspect of reservoir C footprint assessments (Deemer et al., 2016; Paranaíba et al., 2018). Reservoir-scale fluxes are usually derived from applying an average of limited flux measurements to the entire reservoir area. For Batang Ai, this method overestimates CO2 and CH4 surface diffusion by 14 % (130 ) and 64 % (251 ) respectively compared to spatial interpolation. This is due to the effect of extreme values that are very constrained in space but have a disproportionate effect on the overall flux average. Also, reducing temporal sampling resolution to one campaign instead of four changes the reservoir C footprint estimate by up to 33 %. An additional source of uncertainty in reservoir flux estimates is the definition of a baseline value representing natural river emissions in order to calculate downstream emissions of excess gas in the outflow attributable to damming. In Batang Ai, downstream emission was estimated assuming the GHG concentration 19 km downstream of the dam is a representative baseline for the outflow; however, measured values in the pre-impounded river would have substantially reduced the estimate uncertainty. Results from Batang Ai reinforce the importance of pre- and post-impoundment sampling resolution and upscaling methods in annual reservoir-scale GHG flux estimates.
Table 2Estimated reservoir and inflow areal and total GHG fluxes to the atmosphere (±standard error for measured values, or 95 % confidence interval based on model standard error for G-res values) from different pathways based on measured and modelled approaches.
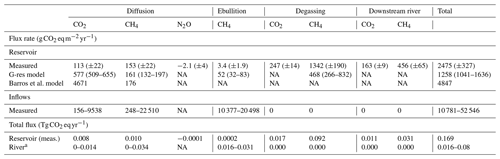
NA: not available. a Represents the estimated pre-impounded river fluxes assuming they were similar to current fluxes from the reservoir inflows.
3.6 Reservoir C footprint and potential mitigation
Most of the Batang Ai emissions occur downstream of the dam through degassing (64.2 %) and downstream emissions (25.0 %), while surface diffusion contributed only 10.6 % and ebullition 0.14 % (Table 2). In all pathways, radiative potential of CH4 fluxes was higher than CO2 and N2O (especially for degassing), accounting for 79.0 % of Batang Ai CO2 eq emissions. This distribution of the flux can be attributed mostly to the accumulation of large quantities of CH4 in the hypolimnion, combined with the fact that the withdrawal depth is located within this layer, allowing the accumulated gas to escape to the atmosphere. Previous studies on reservoirs with similar characteristics to Batang Ai (tropical climate with a permanent thermal stratification and deep water withdrawal) have also found degassing and downstream emissions to be the major emission pathways, especially for CH4 (Galy-Lacaux et al., 1997; Kemenes et al., 2007).
Overall, we estimated that the reservoir emits on average 2475 (±327) , which corresponds to 0.169 Tg CO2 eq yr−1 over the whole system. In comparison, the annual areal emission rate (diffusion and ebullition) of the inflows, based on a more limited sampling resolution, is estimated to range from 10.8 to 52.5 , mainly due to extremely high ebullition. When applied to the approximated surface area of the river before impoundment (1.52 km2), this rate translates to 0.016–0.080 Tg CO2 eq (Table 2), assuming similar flux rates in the current inflows and pre-impoundment river. While the emission rate of the river per unit of area is an order of magnitude higher than for the reservoir, its estimated total flux remains 2.1 to 10.6 times lower due to a much smaller surface. Higher riverine emissions rates are probably due to a shallower depth and higher inputs of terrestrial organic matter, both conducive to CO2 and CH4 production and ebullition. Changing the landscape hydrology to a reservoir drastically reduced areal flux rates, especially ebullition; however, it widely expanded the volume of anoxic environments (sediments and hypolimnion), creating a vast new space for CH4 production. The new hydrological regime also created an opportunity for the large quantities of gas produced in deep layers to easily escape to the atmosphere through the outflow and downstream river.
One way to reduce reservoir GHG emissions is to ensure low CO2 and CH4 concentrations at the water withdrawal depth. In Batang Ai, maximum CO2 and CH4 concentrations are found in the reservoir deep layers and rapidly decrease from 20 to 10 m for CO2 and from 25 to 15 m for CH4 (Fig. S1). This pattern is commonly found in lakes and reservoirs and results from thermal stratification and biological processes (aerobic respiration and CH4 oxidation). Knowing this concentration profile, degassing and downstream emissions could have been reduced in Batang Ai by elevating the water withdrawal depth to avoid hypolimnetic gas release. We calculated that elevating the water withdrawal depth by 1, 3, and 5 m would result in a reduction of degassing and downstream emissions by 1 %, 11 %, and 22 % for CO2 and by 28 %, 92 %, and 100 % for CH4, respectively (Fig. S4). Consequently, a minor change in the dam design could have drastically reduced Batang Ai's C footprint. This should be taken into consideration in future reservoir construction, especially in tropical regions.
3.7 Measured versus modelled fluxes
Based on measurements, Batang Ai emits on average 113 (±22) via surface CO2 diffusion. This value is 41 times lower than predicted by the Barros et al. model (4671 , Table 2) based on reservoir age, DOC inputs (derived from DOC water concentration), and latitude (Barros et al., 2011). The high predicted value for Batang Ai, being a relatively old reservoir with very low DOC concentration, is mainly driven by its low latitude. While reservoirs in low latitudes globally have higher average CO2 fluxes due to higher temperature and often dense flooded biomass (Barros et al., 2011; St. Louis et al., 2000), our results provide a clear example that not all tropical reservoirs have high CO2 emissions by simple virtue of their geographical location. Despite high temperature, Batang Ai's very low water organic matter content (Table S1) offers little substrate for net heterotrophy, and its strong permanent stratification creates a physical barrier potentially retaining CO2 derived from flooded biomass in the hypolimnion. The only three other sampled reservoirs in Southeast Asia (Nam Leuk and Nam Ngum in Laos, and Palasari in Indonesia) also exhibited low organic C concentration (for reservoirs in Laos) and low to negative average surface CO2 diffusion despite their low latitude (Chanudet et al., 2011; Macklin et al., 2018). This suggests that, while additional data are needed, low CO2 diffusion may be common in Southeast Asian reservoirs and is likely linked to the low organic C content.
In comparison, the G-res model predicts a CO2 surface diffusion of 577 (509–655) , which includes the flux naturally sustained by catchment C inputs (397 , predicted flux 100 years after flooding) and the flux derived from organic matter flooding (180 ). While the predicted G-res value is much closer than that predicted from the Barros et al. model, it still overestimates measured flux, mostly the natural baseline (catchment derived) part of it. The G-res predicts baseline CO2 effluxes as a function of soil C content, a proxy for C input to the reservoir. While Batang Ai soil is rich in organic C (∼50 g kg−1), it also has a high clay content (>40 %) (ISRIC – World Soil Information, 2019; Wasli et al., 2011) which is known to bind with organic matter and reduce its leaching to the aquatic environment (Oades, 1988). This may explain the unusually low DOC concentration in the reservoir and its inflows (0.3 to 1.8 mg L−1, Table S1) that are among the lowest reported in freshwaters globally (Sobek et al., 2007). Clay-rich soils are ubiquitous in tropical landscapes (especially in Southeast Asia, Central America, and central and eastern Africa) (ISRIC – World Soil Information, 2019); however, their impact on global-scale patterns of aquatic DOC remains unknown. This may be due to a lack of aquatic DOC data, with the most recently published global study on the subject featuring only one tropical system and a heavy bias towards North America and Europe (Sobek et al., 2007). Exploring the global-scale picture of aquatic DOC and its link to watershed soils characteristics would be a significant step forward in the modelling of reservoir CO2 diffusion. Indeed, had the G-res model been able to capture the baseline emissions more correctly in Batang Ai (close to zero given the very low DOC inputs), predictions would have nearly matched observations. Finally, note that the G-res model is not suitable to predict CO2 uptake, which was observed in 32 % of flux measurements in Batang Ai due to an occasionally net autotrophic surface metabolism favoured under low C inputs (Bogard and del Giorgio, 2016). Improving this aspect of the model depends on the capacity to predict internal metabolism of aquatic systems at a global scale, which is currently lacking. Overall, reservoir CO2 diffusion models may be less performant in certain regions, like Southeast Asia, due to an uneven spatial sampling distribution and a general lack of knowledge and data on C cycling in some parts of the world.
Our field-based estimate of Batang Ai CH4 surface diffusion is 153 (±22) , which differs by only 5 % and 15 % from the G-res and Barros et al. modelled predictions of 161 (132–197) and 176 respectively (Table 2). Both models use as predictors age, a proxy for water temperature (air temperature or latitude), and an indicator of reservoir morphometry (% littoral area or mean depth), and Barros et al. (2011) also uses DOC input (Table S3). Similar predictors were identified in a recent global literature analysis, which also pointed out the role of trophic state in CH4 diffusion, with Batang Ai falling well in the range of flux reported in other oligotrophic reservoirs (Deemer et al., 2016). Overall, our results show that global modelling frameworks for CH4 surface diffusion capture the reality of Batang Ai reasonably well.
Measured estimate of reservoir-scale CH4 ebullition averaged 3.4 (±1.9) (Table 2), which is one of the lowest reported globally in reservoirs (Deemer et al., 2016) and is an order of magnitude lower than the 52 (32–83) predicted by the G-res model (the only available model for reservoir ebullition). This contrasts with the perception that tropical reservoirs consistently have high ebullitive emissions and supports the idea that the supply of sediment organic matter, rather than temperature, is the primary driver of ebullition (Grinham et al., 2018). Batang Ai sediment properties and focusing patterns mentioned earlier could explain the model overestimation of CH4 ebullition. The G-res model considers the fraction of littoral area and horizontal radiance (a proxy for heat input) as predictors of ebullition rate but does not integrate other catchment properties. Building a stronger mechanistic understanding of the effect of sediment composition and accumulation patterns on CH4 bubbling may improve our ability to more accurately predict reservoir ebullition flux.
Our empirical estimate shows that 409 (±23) and 1798 (±255) are emitted as CO2 and CH4 respectively downstream of the dam (including degassing), accounting for 89 % of Batang Ai GHG emissions (Table 2). Currently there is no available model predicting downstream GHG emissions from reservoirs, except the G-res model which is able to predict only the CH4 degassing part of this flux. Modelled CH4 degassing in Batang Ai is 468 (266–832) compared to an estimated 1342 (±190) based on our measurements. Predictive variables used to model CH4 degassing are modelled CH4 surface diffusion (based on % littoral area and temperature) and water retention time (Table S3). In Batang Ai main basin, the strong and permanent stratification favours oxygen depletion in the hypolimnion, which promotes deep CH4 accumulation combined with a decoupling between surface and deep water layers. The model relies strongly on surface CH4 patterns to predict excess CH4 in the deep layer, which could explain why it underestimates CH4 degassing in Batang Ai. Similar strong stratification patterns are ubiquitous in the tropics, with a recent study suggesting a large majority of tropical reservoirs are monomictic or oligomictic (Lehmusluoto et al., 1997; Winton et al., 2019) and hence more often stratified than temperate and boreal ones. This suggests that CH4 degassing is potentially more frequently underestimated in low-latitude reservoirs. The G-res effort to predict CH4 degassing is much needed given the importance of this pathway, and the next step would be to refine this model and develop predictions for other currently missing fluxes like CO2 degassing and downstream emissions in the outflow. Our results suggest that improving latter aspects requires a better capacity to predict GHG accumulation in deep reservoirs layers across a wide range of stratification regimes.
The comprehensive GHG portrait of Batang Ai highlights the importance of spatial and temporal sampling resolution and the inclusion of all flux components in reservoir GHG assessments. Gas dynamics downstream of the dam (degassing, outflow emissions, and CH4 oxidation), commonly not assessed in reservoir GHG studies, are major elements in Batang Ai. We suggest that these emissions could have been greatly diminished with a minor change to the dam design (shallower water withdrawal). Mitigating GHG emissions from future reservoirs depends on the capacity to predict GHG fluxes from all pathways. In this regard, the comparison between Batang Ai measured and modelled GHG flux estimates allowed us to identify knowledge gaps based on which we propose the four following research avenues. (1) Refine the modelling of reservoir CO2 diffusion by studying its link with metabolism and organic matter leaching from different soil types. (2) Examine the potential for CH4 ebullition in littoral zones in relation to patterns of organic matter sedimentation linked to morphometry. (3) Improve the modelling of CH4 degassing by better defining drivers of hypolimnetic CH4 accumulation, namely thermal stratification. (4) Gather additional field data on GHG dynamics downstream of dams (degassing, river emissions, and river CH4 oxidation) in order to incorporate all components of the flux to the modelling of reservoir C footprint.
The dataset related to this article is available online through Zenodo at https://doi.org/10.5281/zenodo.3629898 (Soued and Prairie, 2020).
The supplement related to this article is available online at: https://doi.org/10.5194/bg-17-515-2020-supplement.
CS contributed to the conceptualization, methodology, validation, formal analysis, investigation, data curation, writing of the original draft, review and editing, and project administration. YTP contributed to the methodology, validation, investigation, resources, review and editing, supervision, and funding acquisition.
The authors declare that they have no conflict of interest.
This is a contribution to the UNESCO chair in Global Environmental Change and the GRIL. We are grateful to Karen Lee Suan Ping and Jenny Choo Cheng Yi for their logistic support and participation in sampling campaigns. We also thank Jessica Fong Fung Yee, Amar Ma'aruf Bin Ismawi, Gerald Tawie Anak Thomas, Hilton Bin John, Paula Reis, Sara Mercier-Blais, and Karelle Desrosiers for their help on the field, as well as Katherine Velghe and Marilyne Robidoux for their assistance during laboratory analyses.
This research has been supported by the Natural Sciences and Engineering Research Council of Canada (Discovery Grant to Yves T. Prairie and BES-D scholarship to Cynthia Soued) and Sarawak Energy Berhad (research and development).
This paper was edited by Ji-Hyung Park and reviewed by one anonymous referee.
Barros, N., Cole, J. J., Tranvik, L. J., Prairie, Y. T., Bastviken, D., Huszar, V. L. M., del Giorgio, P., and Roland, F.: Carbon emission from hydroelectric reservoirs linked to reservoir age and latitude, Nat. Geosci., 4, 593–596, https://doi.org/10.1038/ngeo1211, 2011.
Bastien, J. and Demarty, M.: Spatio-temporal variation of gross CO2 and CH4 diffusive emissions from Australian reservoirs and natural aquatic ecosystems, and estimation of net reservoir emissions, Lakes Reserv. Res. Manag., 18, 115–127, https://doi.org/10.1111/lre.12028, 2013.
Bastviken, D., Ejlertsson, J., and Tranvik, L.: Measurement of methane oxidation in lakes: A comparison of methods, Environ. Sci. Technol., 36, 3354–3361, https://doi.org/10.1021/es010311p, 2002.
Bastviken, D., Tranvik, L. J., Downing, J. A., Crill, P. M., and Enrich-Prast, A.: Freshwater Methane Emissions Offset the Continental Carbon Sink, Science, 331, 50–50, https://doi.org/10.1126/science.1196808, 2011.
Beaulieu, J. J., McManus, M. G., and Nietch, C. T.: Estimates of reservoir methane emissions based on a spatially balanced probabilistic-survey, Limnol. Oceanogr., 61, S27–S40, https://doi.org/10.1002/lno.10284, 2016.
Blais, J. M. and Kalff, J.: The influence of lake morphometry on sediment focusing, Limnol. Oceanogr., 40, 582–588, https://doi.org/10.4319/lo.1995.40.3.0582, 1995.
Bogard, M. J. and del Giorgio, P. A.: The role of metabolism in modulating CO2 fluxes in boreal lakes, Global Biogeochem. Cycles, 30, 1509–1525, https://doi.org/10.1002/2016GB005463, 2016.
Chanudet, V., Descloux, S., Harby, A., Sundt, H., Hansen, B. H., Brakstad, O., Serça, D., and Guerin, F.: Gross CO2 and CH4 emissions from the Nam Ngum and Nam Leuk sub-tropical reservoirs in Lao PDR, Sci. Total Environ., 409, 5382–5391, https://doi.org/10.1016/j.scitotenv.2011.09.018, 2011.
Cole, J. J. and Caraco, N. F.: Atmospheric exchange of carbon dioxide in a low-wind oligotrophic lake measured by the addition of SF6, Limnol. Oceanogr., 43, 647–656, https://doi.org/10.4319/lo.1998.43.4.0647, 1998.
Coleman, D. D., Risatti, J. B., and Schoell, M.: Fractionation of carbon and hydrogen isotopes by methane-oxidizing bacteria, Geochim. Cosmochim. Ac., 45, 1033–1037, https://doi.org/10.1016/0016-7037(81)90129-0, 1981.
Deemer, B. R., Harrison, J. A., Li, S., Beaulieu, J. J., DelSontro, T., Barros, N., Bezerra-Neto, J. F., Powers, S. M., dos Santos, M. A., and Vonk, J. A.: Greenhouse Gas Emissions from Reservoir Water Surfaces: A New Global Synthesis, Bioscience, 66, 949–964, https://doi.org/10.1093/biosci/biw117, 2016.
DelSontro, T., McGinnis, D. F., Sobek, S., Ostrovsky, I., and Wehrli, B.: Extreme Methane Emissions from a Swiss Hydropower Reservoir: Contribution from Bubbling Sediments, Environ. Sci. Technol., 44, 2419–2425, https://doi.org/10.1021/es9031369, 2010.
DelSontro, T., Kunz, M. J., Kempter, T., Wüest, A., Wehrli, B., and Senn, D. B.: Spatial Heterogeneity of Methane Ebullition in a Large Tropical Reservoir, Environ. Sci. Technol., 45, 9866–9873, https://doi.org/10.1021/es2005545, 2011.
DelSontro, T., Perez, K. K., Sollberger, S., and Wehrli, B.: Methane dynamics downstream of a temperate run-of-the-river reservoir, Limnol. Oceanogr., 61, S188–S203, https://doi.org/10.1002/lno.10387, 2016.
Demarty, M. and Bastien, J.: GHG emissions from hydroelectric reservoirs in tropical and equatorial regions: Review of 20 years of CH4 emission measurements, Energy Policy, 39, 4197–4206, https://doi.org/10.1016/j.enpol.2011.04.033, 2011.
de Mello, N. A. S. T., Brighenti, L. S., Barbosa, F. A. R., Staehr, P. A., and Bezerra Neto, J. F.: Spatial variability of methane (CH4) ebullition in a tropical hypereutrophic reservoir: silted areas as a bubble hot spot, Lake Reserv. Manag., 34, 105–114, https://doi.org/10.1080/10402381.2017.1390018, 2018.
Galy-Lacaux, C., Delmas, R., Jambert, C., Dumestre, J.-F., Labroue, L., Richard, S., and Gosse, P.: Gaseous emissions and oxygen consumption in hydroelectric dams: A case study in French Guyana, Global Biogeochem. Cycles, 11, 471–483, https://doi.org/10.1029/97GB01625, 1997.
Grinham, A., Dunbabin, M., and Albert, S.: Importance of sediment organic matter to methane ebullition in a sub-tropical freshwater reservoir, Sci. Total Environ., 621, 1199–1207, https://doi.org/10.1016/j.scitotenv.2017.10.108, 2018.
Guérin, F. and Abril, G.: Significance of pelagic aerobic methane oxidation in the methane and carbon budget of a tropical reservoir, J. Geophys. Res.-Biogeo., 112, G3, https://doi.org/10.1029/2006JG000393, 2007.
Guérin, F., Abril, G., Richard, S., Burban, B., Reynouard, C., Seyler, P., and Delmas, R.: Methane and carbon dioxide emissions from tropical reservoirs: Significance of downstream rivers, Geophys. Res. Lett., 33, L21407, https://doi.org/10.1029/2006GL027929, 2006.
International Hydropower Association (IHA): A brief history of hydropower, available at: https://www.hydropower.org/a-brief-history-of-hydropower (last access: 11 July 2019), 2015.
ISRIC – World Soil Information: SoilGrids v0.5.3, available at: https://soilgrids.org/#!/?layer=ORCDRC_M_sl4_250m&vector=1, last access: 1 May 2019.
Keller, M. and Stallard, R. F.: Methane emission by bubbling from Gatun Lake, Panama, J. Geophys. Res., 99, 8307, https://doi.org/10.1029/92JD02170, 1994.
Kemenes, A., Forsberg, B. R., and Melack, J. M.: Methane release below a tropical hydroelectric dam, Geophys. Res. Lett., 34, 1–5, https://doi.org/10.1029/2007GL029479, 2007.
Kemenes, A., Forsberg, B. R., and Melack, J. M.: Downstream emissions of CH4 and CO2 from hydroelectric reservoirs (Tucuruí, Samuel, and Curuá-Una) in the Amazon basin, Inl. Waters, 6, 295–302, https://doi.org/10.1080/IW-6.3.980, 2016.
Knox, M., Quay, P. D., and Wilbur, D.: Kinetic isotopic fractionation during air-water gas transfer of O2, N2, CH4, and H2, J. Geophys. Res., 97, 20335, https://doi.org/10.1029/92JC00949, 1992.
Ledwell, J. J.: The Variation of the Gas Transfer Coefficient with Molecular Diffusity, in: Gas Transfer at Water Surfaces, pp. 293–302, Springer Netherlands, Dordrecht, 1984.
Lehmusluoto, P., Machbub, B., Terangna, N., Rusmiputro, S., Achmad, F., Boer, L., Brahmana, S. S., Priadi, B., Setiadji, B., Sayuman, O., and Margana, A.: National inventory of the major lakes and reservoirs in Indonesia, available at: http://www.kolumbus.fi/pasi.lehmusluoto/210_expedition_indodanau_report1997.PDF (last access: 8 November 2019), 1997.
Lehner, B., Liermann, C. R., Revenga, C., Vörösmarty, C., Fekete, B., Crouzet, P., Döll, P., Endejan, M., Frenken, K., Magome, J., Nilsson, C., Robertson, J. C., Rödel, R., Sindorf, N., and Wisser, D.: High-resolution mapping of the world's reservoirs and dams for sustainable river-flow management, Front. Ecol. Environ., 9, 494–502, https://doi.org/10.1890/100125, 2011.
Lide, D.: CRC Handbook of Chemistry and Physics, edited by: CRC Press, Boca Raton, FL, available at: http://webdelprofesor.ula.ve/ciencias/isolda/libros/handbook.pdf (last access: 30 August 2019), 2005.
Ling, T. Y., Gerunsin, N., Soo, C. L., Nyanti, L., Sim, S. F., and Grinang, J.: Seasonal changes and spatial variation in water quality of a large young tropical reservoir and its downstream river, J. Chem., 2017, 8153246, https://doi.org/10.1155/2017/8153246, 2017.
Macklin, P. A., Gusti Ngurah Agung Suryaputra, I., Maher, D. T., and Santos, I. R.: Carbon dioxide dynamics in a lake and a reservoir on a tropical island (Bali, Indonesia), PLoS One, 13, 6, https://doi.org/10.1371/journal.pone.0198678, 2018.
Martin, P., Cherukuru, N., Tan, A. S. Y., Sanwlani, N., Mujahid, A., and Müller, M.: Distribution and cycling of terrigenous dissolved organic carbon in peatland-draining rivers and coastal waters of Sarawak, Borneo, Biogeosciences, 15, 6847–6865, https://doi.org/10.5194/bg-15-6847-2018, 2018.
Murase, J. and Sugimoto, A.: Inhibitory effect of light on methane oxidation in the pelagic water column of a mesotrophic lake (Lake Biwa, Japan), Limnol. Oceanogr., 50, 1339–1343, https://doi.org/10.4319/lo.2005.50.4.1339, 2005.
Myhre, G., Shindell, D., Bréon, F.-M., Collins, W., Fuglestvedt, J., Huang, J., Koch, D., Lamarque, J.-F., Lee, D., Mendoza, B., Nakajima, T., Robock, A., Stephens, G., Takemura, T., and Zhang, H.: Anthropogenic and Natural Radiative Forcing, in: Climate Change 2013: The Physical Science Basis. Contribution of Working Group I to the Fifth Assessment Report of the Intergovernmental Panel on Climate Change, pp. 659–740, Cambridge, United Kindom, available at: http://www.climatechange2013.org/images/report/WG1AR5_Chapter08_FINAL.pdf (last access: 30 August 2019), 2013.
Oades, J. M.: The retention of organic matter in soils, Biogeochemistry, 5, 35–70, https://doi.org/10.1007/BF02180317, 1988.
Oswald, K., Milucka, J., Brand, A., Littmann, S., Wehrli, B., Kuypers, M. M. M., and Schubert, C. J.: Light-Dependent Aerobic Methane Oxidation Reduces Methane Emissions from Seasonally Stratified Lakes, PLoS One, 10, e0132574, https://doi.org/10.1371/journal.pone.0132574, 2015.
Paranaíba, J. R., Barros, N., Mendonça, R., Linkhorst, A., Isidorova, A., Roland, F., Almeida, R. M., and Sobek, S.: Spatially Resolved Measurements of CO2 and CH4 Concentration and Gas-Exchange Velocity Highly Influence Carbon-Emission Estimates of Reservoirs, Environ. Sci. Technol., 52, 607–615, https://doi.org/10.1021/acs.est.7b05138, 2018.
Patton, C. and Kryskalla, J.: Methods of analysis by the U.S. Geological Survey National Water Quality Laboratory: evaluation of alkaline persulfate digestion as an alternative to Kjeldahl digestion for determination of total and dissolved nitrogen and phosphorus in water, U.S. Government Printing Office, Denver, Colorado, 2003.
Pebesma, E. J.: Multivariable geostatistics in S: the gstat package, Comput. Geosci., 30, 683–691, https://doi.org/10.1016/j.cageo.2004.03.012, 2004.
Prairie, Y. T., Alm, J., Beaulieu, J., Barros, N., Battin, T., Cole, J., del Giorgio, P., DelSontro, T., Guérin, F., Harby, A., Harrison, J., Mercier-Blais, S., Serça, D., Sobek, S., and Vachon, D.: Greenhouse Gas Emissions from Freshwater Reservoirs: What Does the Atmosphere See?, Ecosystems, 21, 1058–1071, https://doi.org/10.1007/s10021-017-0198-9, 2018.
R Core Team: R: A language and environment for statistical computing, available at: https://www.r-project.org/ (last access: 9 December 2019), 2017.
Rowan, D. J., Kalff, J., and Rasmussen, J. B.: Estimating the Mud Deposition Boundary Depth in Lakes from Wave Theory, Can. J. Fish. Aquat. Sci., 49, 2490–2497, https://doi.org/10.1139/f92-275, 1992.
Rudd, J., Harris, R., and Kelly, C. A.: Are Hydroelectric Reservoirs Signficant Sources of Greenhouse Gases?, Ambio, 22, 246–248, 1993.
Sarawak Government: The Geography of Sarawak, available at: https://www.sarawak.gov.my/web/home/article_view/159/176/, last access: 3 May 2019.
Sartory, D. P. and Grobbelaar, J. U.: Extraction of chlorophyll a from freshwater phytoplankton for spectrophotometric analysis, Hydrobiologia, 114, 177–187, https://doi.org/10.1007/BF00031869, 1984.
Sherman, B. and Ford, P.: Methane Emissions from Two reservoirs in a steep, sub- Tropical rainforest catchment, in: Science Forum and Stakeholder Engagement: Building Linkages, Collaboration and Science Quality, edited by: Begbie, D. K. and Wakem, S. L., pp. 1–9, Urban Water Security Research Alliance, Brisbane, Queensland, 2011.
Sobek, S., Tranvik, L. J., and Cole, J. J.: Temperature independence of carbon dioxide supersaturation in global lakes, Global Biogeochem. Cycles, 19, 2, https://doi.org/10.1029/2004GB002264, 2005.
Sobek, S., Tranvik, L. J., Prairie, Y. T., Kortelainen, P., and Cole, J. J.: Patterns and regulation of dissolved organic carbon: An analysis of 7,500 widely distributed lakes, Limnol. Oceanogr., 52, 1208–1219, https://doi.org/10.4319/lo.2007.52.3.1208, 2007.
Soued, C. and Prairie, Y.: Carbon dioxide, methane, and chemical data from Batang Ai reservoir (Version 1.0.0), Zenodo, https://doi.org/10.5281/zenodo.3629898, 2020.
Soued, C., del Giorgio, P. A., and Maranger, R.: Nitrous oxide sinks and emissions in boreal aquatic networks in Québec, Nat. Geosci., 9, 116–120, https://doi.org/10.1038/ngeo2611, 2016.
St. Louis, V. L., Kelly, C. A., Duchemin, É., Rudd, J. W. M., and Rosenberg, D. M.: Reservoir Surfaces as Sources of Greenhouse Gases to the Atmosphere: A Global Estimate, Bioscience, 50, 766, https://doi.org/10.1641/0006-3568(2000)050[0766:RSASOG]2.0.CO;2, 2000.
Teodoru, C. R., Prairie, Y. T., and del Giorgio, P. A.: Spatial Heterogeneity of Surface CO2 Fluxes in a Newly Created Eastmain-1 Reservoir in Northern Quebec, Canada, Ecosystems, 14, 28–46, https://doi.org/10.1007/s10021-010-9393-7, 2011.
Teodoru, C. R., Bastien, J., Bonneville, M.-C., del Giorgio, P. A., Demarty, M., Garneau, M., Hélie, J.-F., Pelletier, L., Prairie, Y. T., Roulet, N. T., Strachan, I. B., and Tremblay, A.: The net carbon footprint of a newly created boreal hydroelectric reservoir, Global Biogeochem. Cycles, 26, 2, https://doi.org/10.1029/2011GB004187, 2012.
Thottathil, S. D., Reis, P. C. J., del Giorgio, P. A., and Prairie, Y. T.: The Extent and Regulation of Summer Methane Oxidation in Northern Lakes, J. Geophys. Res.-Biogeo., 123, 3216–3230, https://doi.org/10.1029/2018JG004464, 2018.
Thottathil, S. D., Reis, P. C. J., and Prairie, Y. T.: Methane oxidation kinetics in northern freshwater lakes, Biogeochemistry, 143, 105–116, https://doi.org/10.1007/s10533-019-00552-x, 2019.
UNESCO/IHA: The GHG Reservoir Tool (G- res), available at: https://g-res.hydropower.org/ (last access: 1 May 2019), 2017.
Venkiteswaran, J. J., Schiff, S. L., St. Louis, V. L., Matthews, C. J. D., Boudreau, N. M., Joyce, E. M., Beaty, K. G., and Bodaly, R. A.: Processes affecting greenhouse gas production in experimental boreal reservoirs, Global Biogeochem. Cycles, 27, 567–577, https://doi.org/10.1002/gbc.20046, 2013.
Wanninkhof, R.: Relationship between wind speed and gas exchange over the ocean, J. Geophys. Res., 97, 7373, https://doi.org/10.1029/92JC00188, 1992.
Wasli, M. E., Tanaka, S., Kendawang, J. J., Abdu, A., Lat, J., Morooka, Y., Long, S. M., and Sakurai, K.: Soils and Vegetation Condition of Natural Forests and Secondary Fallow Forests within Batang Ai National Park Boundary, Sarawak, Malaysia, Kuroshio Sci., 5, 67–76, 2011.
Webb, J. R., Hayes, N. M., Simpson, G. L., Leavitt, P. R., Baulch, H. M., and Finlay, K.: Widespread nitrous oxide undersaturation in farm waterbodies creates an unexpected greenhouse gas sink, P. Natl. Acad. Sci. USA, 116, 201820389, https://doi.org/10.1073/pnas.1820389116, 2019.
Wetzel, R. G. and Likens, G. E.: Limnological Analyses, 3rd ed., Springer New York, New York, NY, 2000.
Winton, R. S., Calamita, E., and Wehrli, B.: Reviews and syntheses: Dams, water quality and tropical reservoir stratification, Biogeosciences, 16, 1657–1671, https://doi.org/10.5194/bg-16-1657-2019, 2019.
Zarfl, C., Lumsdon, A. E., Berlekamp, J., Tydecks, L., and Tockner, K.: A global boom in hydropower dam construction, Aquat. Sci., 77, 161–170, https://doi.org/10.1007/s00027-014-0377-0, 2015.