the Creative Commons Attribution 4.0 License.
the Creative Commons Attribution 4.0 License.
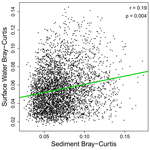
Organic matter transformations are disconnected between surface water and the hyporheic zone
Sarah J. Fansler
Malak M. Tfaily
Vanessa A. Garayburu-Caruso
Amy E. Goldman
Robert E. Danczak
Rosalie K. Chu
Lupita Renteria
Jerry Tagestad
Jason Toyoda
Biochemical transformations of organic matter (OM) are a primary driver of river corridor biogeochemistry, thereby modulating ecosystem processes at local to global scales. OM transformations are driven by diverse biotic and abiotic processes, but we lack knowledge of how the diversity of those processes varies across river corridors and across surface and subsurface components of river corridors. To fill this gap we quantified the number of putative biotic and abiotic transformations of organic molecules across diverse river corridors using ultra-high-resolution mass spectrometry. The number of unique transformations is used here as a proxy for the diversity of biochemical processes underlying observed profiles of organic molecules. For this, we use public data spanning the contiguous United States (ConUS) from the Worldwide Hydrobiogeochemical Observation Network for Dynamic River Systems (WHONDRS) consortium. Our results show that surface water OM had more biotic and abiotic transformations than OM from shallow hyporheic zone sediments (1–3 cm depth). We observed substantially more biotic than abiotic transformations, and the numbers of biotic and abiotic transformations were highly correlated with each other. We found no relationship between the number of transformations in surface water and sediments and no meaningful relationships with latitude, longitude, or climate. We also found that the composition of transformations in sediments was not linked with transformation composition in adjacent surface waters. We infer that OM transformations represented in surface water are an integrated signal of diverse processes occurring throughout the upstream catchment. In contrast, OM transformations in sediments likely reflect a narrower range of processes within the sampled volume. This indicates decoupling between the processes influencing surface water and sediment OM, despite the potential for hydrologic exchange to homogenize OM. We infer that the processes influencing OM transformations and the scales at which they operate diverge between surface water and sediments.
- Article
(3154 KB) - Full-text XML
-
Supplement
(448 KB) - BibTeX
- EndNote
River corridors are an important component of the integrated Earth system that have large influences on the flux of materials and energy across local to global scales (Harvey and Gooseff, 2015; Schlünz and Schneider, 2000; Schlesinger and Melack, 1981). The biogeochemical function of river corridors (e.g., rates of contaminate transformations) is the outcome of both biotic and abiotic processes (e.g., He et al., 2016; Bowen et al., 2020). On the biological side, microbial communities in areas where groundwater and surface water mix (i.e., hyporheic zones) can, for example, contribute substantially to river corridor respiration rates (Jones Jr, 1995; Naegeli and Uehlinger, 1997; Battin et al., 2003; Fischer et al., 2005; but see Ward et al., 2018). In these areas, microbial metabolism can be heavily modified by hydrologic mixing (e.g., McClain et al., 2003; Stegen et al., 2016, 2018). On the abiotic side, light-driven organic matter (OM) transformations, for example, can consume significant amounts of dissolved organic carbon in river systems (e.g., Amon and Benner, 1996) and heavily modify OM profiles (e.g., Holt et al., 2021). The integration of biotic and abiotic processes ultimately leads to variation in water quality and ecosystem fluxes that are relevant to local communities and global fluxes.
Within river corridors, OM serves as a primary energy source fueling aerobic and anaerobic heterotrophic respiration (Fisher and Likens, 1973; Wetzel, 1995; Cole et al., 2007; Creed et al., 2015). The chemistry of OM in river corridors is particularly important, with a multitude of influences over biogeochemical rates and ecosystem fluxes. For example, through field, lab, and mechanistic modeling, thermodynamic properties of OM have been shown to influence microbial respiration in both aerobic and anaerobic river corridor settings (Boye et al., 2017; Stegen et al., 2018; Graham et al., 2018; Garayburu-Caruso et al., 2020a; Song et al., 2020; Sengupta et al., 2021). This has also recently been shown in soil systems as well (Hough et al., 2022). Other attributes of OM chemistry, such as the carbon-to-nitrogen ratio, also have strong influences over river corridor rates and fluxes (Bauer et al., 2013; Liu et al., 2020). As is the case for nearly all attributes of river corridors, the spatial variation in and temporal dynamics of OM chemistry emerge through the integration of biotic and abiotic processes.
Biotic and abiotic processes influence river corridor OM chemistry by modifying rates of production, transformation, sorption and desorption, and/or spatial movement (Danczak et al., 2020). All these factors have been studied to some degree in river corridors, and advances in cheminformatics techniques can provide further insights specifically into the biotic and abiotic components of OM transformations. More specifically, Fudyma et al. (2021) used the ultra-high-mass resolution of Fourier transform ion cyclotron resonance mass spectrometry (FTICR-MS) data (Marshall et al., 1998; Bahureksa et al., 2021) to infer putative abiotic and abiotic transformations of OM in a river corridor system. This extended previously developed cheminformatics techniques (e.g., Breitling et al., 2006; Stegen et al., 2018; Danczak et al., 2020, 2021) to include abiotic transformations. Fudyma et al. (2021) found that abiotic OM transformations, such as those driven by sunlight and photooxidation, may alter bioavailability of OM in groundwater and surface water. These observations were collected across different subsurface hydrologic mixing conditions and suggest that changes in the bioavailability of OM lead to enhanced microbial activity in subsurface domains like the hyporheic zone. This emphasizes the need to consider abiotic OM transformations as a key complement to biotic OM transformations in river corridors (Amon and Benner, 1996; Bowen et al., 2020; Holt et al., 2021; Hu et al., 2021).
While both biotic and abiotic OM transformations are important in river corridors, we lack broad cross-system understanding of how these two classes of transformations relate to each other and how they vary between hyporheic zone sediments and surface water. Resolving these knowledge gaps is useful from a number of perspectives; for example, it was recently proposed that surface water chemistry can be used as a mirror to understand subsurface chemistry and associated processes (Stewart et al., 2021). With that idea in mind, if transformation numbers or profiles in surface water are statistically associated with transformation numbers or profiles in sediments, we could use surface water data (easier to generate) to infer properties and processes in the subsurface (much harder to study). In addition, such correspondence would indicate that surface–subsurface hydrologic exchange in river corridors is sufficient to overcome localized processes, thereby at least partially homogenizing OM across river corridor compartments. On the other hand, lack of correspondence between surface water and sediment OM transformations would indicate that deterministic processes (sensu Danczak et al., 2020) in the subsurface overwhelm transport mechanisms in governing OM chemistry. Either outcome is highly informative for fundamental understanding and for mechanistic modeling efforts that couple surface–subsurface hydrology and biogeochemistry (e.g., hyporheicFoam; Li et al., 2020).
Here we aim to help fill knowledge gaps associated with OM transformation counts and composition across surface and subsurface components of river corridors distributed across the contiguous United States (ConUS). We specifically compare the numbers of biotic and abiotic OM transformations in sediments and surface waters and evaluate the potential for continental-scale spatial patterns in biochemical transformation counts and composition. To do so, we use publicly available FTICR-MS data provided by the Worldwide Hydrobiogeochemistry Observation Network for Dynamic River Systems (WHONDRS) consortium (Stegen and Goldman, 2018). One key outcome of our analyses is that OM transformations in sediments are not related to OM transformations in adjacent surface water, which suggests divergent governing processes despite hydrologic connectivity between these river corridor subsystems.
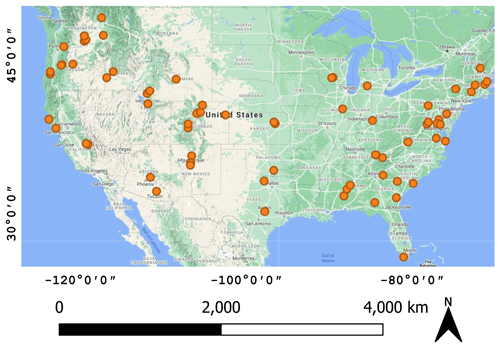
Figure 1Map of sampling locations distributed across the contiguous United States (ConUS). Surface water and sediments were collected at each site using a crowdsourced approach via the WHONDRS consortium. Physical factors such as stream order were not constrained. Figure generated by Sophia McKever using QGIS. The base map is copyrighted: ©OpenStreetMap contributors 2022. Distributed under the Open Data Commons Open Database 504 License (ODbL) v1.0.
Data generation
The samples used for data generation were collected and processed in 2019 as part of the WHONDRS consortium (Stegen and Goldman, 2018), and the data were retrieved from publicly available data packages (Toyoda et al., 2020; Goldman et al., 2020). Full details on sample and metadata collection are provided in Garayburu-Caruso et al. (2020b); some additional sample data are used here that were not used in Garayburu-Caruso et al. (2020b), but all methods are consistent. In short, at each site (Fig. 1) three depositional zones within ∼ 10 m of each other were sampled for shallow sediments (∼ 1–3 cm into the riverbed). Prior to sediment collection, surface water was collected at the most downstream sediment sampling location. The samples were shipped to the Pacific Northwest National Laboratory (PNNL) campus in Richland, WA (USA), on blue ice within 24 h of collection. Untargeted characterization of OM was done using ultra-high-resolution FTICR-MS. In preparation for FTICR-MS analysis, sediments were extracted with Milli-Q deionized (DI) water, and the resulting supernatant was filtered prior to measurement of non-purgeable organic carbon (NPOC). NPOC concentrations were normalized to 1.5 mg C L−1 by adding Milli-Q DI water. To remove salts and minerals, 15 mL of each sample was then passed through PPL (styrene-divinylbenzene polymer sorbent modified with a proprietary nonpolar surface) cartridges (Bond Elut). FTICR-MS analyses were performed at the Environmental Molecular Science Laboratory (EMSL) in Richland, WA, using a 12 Tesla Bruker SolariX FTICR mass spectrometer (Bruker, SolariX, Billerica, MA, USA) in negative ionization mode. FTICR-MS spectra were processed to assign molecular formulae as described in Garayburu-Caruso et al. (2020b). Briefly, to convert raw FTICR-MS spectra into a list of mass-to-charge ratios (i.e., values) we used Bruker Daltonics Data Analysis (version 4.2). We specifically applied the Fourier transform mass spectrometry (FTMS) peak picker module with a signal-to-noise ratio () threshold of 7 and absolute intensity threshold of 100. We then used Formularity (Tolić et al., 2017) to align peaks with a 0.5 ppm threshold and assign chemical formulas. Within Formularity we specifically used the compound identification algorithm with > 7 and mass measurement error of < 0.5 ppm. The compound identification algorithm allows for C, H, O, N, S, and P within the assigned formula while excluding other elements.
FTICR-MS data were used as presence–absence due to peak intensities providing unreliable estimates of absolute or relative concentrations, which is a limitation inherent to FTICR-MS analysis. While FTICR-MS provides the most comprehensive OM chemistry characterization currently available, it has constraints such as not being quantitative and missing low-molecular-weight compounds (≲ 200 Da) that need to be taken into consideration. FTICR-MS nonetheless provides a robust approach for conducting untargeted characterization of environmental OM.
In addition to the FTICR-MS data, we used a suite of environmental variables in an attempt to explain variation in OM transformation counts. These variables included actual evapotranspiration, mean annual precipitation, mean annual temperature, and potential evapotranspiration. Global datasets for these variables were acquired from two sources as geospatial raster datasets: the historical mean annual temperature and mean annual precipitation were downloaded from https://worldclim.org/ (last access: 7 June 2020) (Fick and Hijmans, 2017), and the evapotranspiration and potential evapotranspiration were available as geospatial rasters from the MOD16 Global Evapotranspiration Product database (Running et al., 2017). The environmental variable values were associated with each sample location using the ArcGIS function Extract Values to Points. The output was a table of climate and evapotranspiration values for each sample location.
Biochemical transformation analyses and statistics
Biochemical transformations of OM were inferred as in Fudyma et al. (2021), and full details of the method can be found in that publication. In brief, we used a list of common biochemical transformations (see file “Biotic-abiotic-transfromation-classification.csv” in the Stegen et al, 2021, data package) to putatively infer the identity (e.g., hydrogenation, loss/gain of an alanine) and number of occurrences of each transformation in each sample. A given transformation was inferred each time we observed the corresponding mass shift between a pair of peaks within each sample. This analysis does not provide direct information about where or when a given transformation may have occurred, and it is likely that they occurred prior to the sample being taken and outside of the sampled volume. For example, surface water acts as an integrator whereby transformations inferred in surface water samples likely occurred throughout the upstream catchment. What is observed in surface water samples is therefore the cumulative result of processes throughout the upstream catchment. Similarly, biochemical transformations inferred from sediment samples may have occurred along subsurface flow paths beyond the sampled volume.
In each sample, we counted the number of times each transformation was inferred to have occurred. We then designated each transformation as biotic, abiotic, or both, reflecting the potential chemical reaction sources as in Fudyma et al. (2021). Next, the samples were parsed into sediment or surface water categories. Then we compared the total number of transformations, the number of abiotic transformations, the number of biotic transformations, and the ratio of abiotic to biotic transformation numbers for each sample. Distributions based on the number of transformations or their ratio were compared between surface water and sediments using Wilcox signed rank tests. Transformation numbers and their ratio were related to each other and to spatial and environmental variables using ordinary least squares regression. Spatial and environmental variables included latitude, longitude, and the environmental variables listed above.
In addition to studying transformation numbers, we examined the composition of transformations and related these compositional profiles between surface water and sediments. The purpose of this analysis was to evaluate the degree to which hydrologic exchange homogenizes OM between sediments and physically adjacent surface water. The compositional profile for each sample was characterized by the number of times each transformation was inferred. For each site, the three surface water samples were combined by adding together the number of observations for each transformation and then computing the relative abundance of each transformation. The same process was done for the three sediment samples within each site. Doing this across all sites provided the equivalent of an ecological “species-by-site” matrix, but with transformations as “species” and samples as “sites” and the entries as the site-level relative abundance of each transformation in each sample. In turn, we calculated Bray–Curtis dissimilarity among all sediment samples and, separately, among all surface water samples. The relationship between surface water and sediment Bray–Curtis dissimilarities was then evaluated using distance matrix regression and a Mantel test to account for non-independence of the pairwise comparisons. For this, the Bray–Curtis values from surface water from a given site were linked with the Bray–Curtis values for the sediment data from the same site. Each data point used in the regression is therefore based on surface water and sediment from the same site compared to data from a different, but common, site. For example, in the case of three sites (A, B, and C), a single data point in the regression would be based on water from A compared to water from B and sediments from A compared to sediments from B. Another data point would be water from A compared to water from C and sediments from A compared to sediments from C, and so on. If hydrologic transport between surface water and sediments homogenizes organic molecules between water and sediments, water Bray–Curtis should increase with sediment Bray–Curtis. The stronger the homogenization, the stronger the Bray–Curtis relationship should be. If hydrologic transport does not homogenize OM between sediments and the physically adjacent surface water, no relationship will be observed between surface water and sediment Bray–Curtis values.
Examining ConUS-scale distributions for the number of putative biotic and abiotic transformations showed that surface water OM had significantly more biotic (W = 12 360, p ≪ 0.0001; Fig. 2a) and abiotic (W = 12 978, p ≪ 0.0001; Fig. 2b) transformations than sediment OM. In addition, there were far fewer abiotic transformations (∼ 50–800 per sample) than biotic transformations (∼ 5000 to 80 000) within the ConUS-scale distributions (cf., Fig. 2a and b). On a per-sample basis the abiotic-to-biotic ratio ranged from ∼ 0.01 to 0.02, and sediments had a significantly higher ratio than surface water (W = 46 627, p ≪ 0.0001; Fig. 2c). As a key methodological detail – as described in the “Methods” section – we note that all samples were normalized to a constant organic carbon concentration prior to FTICR-MS analysis such that comparisons can be made directly among all samples, including between surface water and sediments.
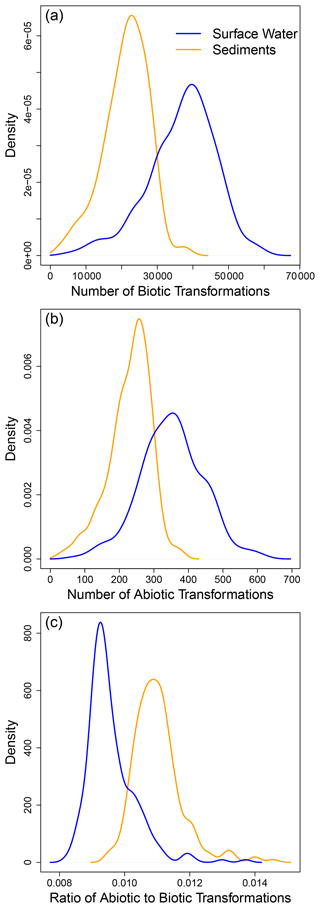
Figure 2Examining the ConUS-scale distributions of biotic and abiotic transformation numbers reveals more transformations in surface water than sediment organic matter. Kernel density functions for ConUS-scale biotic (a) and abiotic (b) transformations and their ratio (c) in sediment (orange lines) and surface water (blue lines) organic matter. The median values of the distributions significantly diverge within each panel (see text for statistics).
The larger number of putative biotic and abiotic transformations in surface water is, at first, surprising given that hyporheic zone sediments are very biogeochemically active (Naegeli and Uehlinger, 1997; McClain et al., 2003) and are often considered to be ecosystem control points within river corridors (Bernhardt et al., 2017). We might therefore expect there to be more OM transformations in hyporheic zone sediments. It is important to consider, however, that the number of transformations (as quantified here) is a reflection of transformation diversity, not the rate of OM transformations. For example, a system may experience a very high rate of OM transformation but have a low number of unique types of transformations. Such a situation would result in a low transformation count due to the FTICR-MS data being used to indicate the presence or absence of organic molecules (i.e., there is no information on abundance).
Given that the number of putative transformations does not indicate the rate of transformation, the larger number in surface water may result from surface water OM being an integrated signature of processes occurring across upstream catchments (Vannote et al., 1980; Xenopoulos et al., 2017). In comparison, sediment OM may reflect processes occurring within and/or much closer to the sampled volume. That is, a larger diversity of transformations may accumulate as surface water OM integrates processes and sources from across the stream network, which is conceptually consistent with previous work using the same data that found higher molecular richness in surface water than in sediment OM (Garayburu-Caruso et al., 2020b). This highlights that inferred transformations likely occurred prior to sampling and outside of the sampled volume (e.g., in the upstream catchment for surface water data and along subsurface flow paths for sediment data). Our interpretation furthermore sets up the emergent (i.e., post hoc) hypothesis that the number of transformations may increase with catchment area. This hypothesis could be evaluated by combining the dataset analyzed here with quantification of upstream catchment areas. Furthermore, this points to a need to compare drivers of transformation counts with drivers of OM functional diversity. For example, Kida et al. (2021) recently found OM functional diversity to increase, decrease, or stay steady moving down a stream network (i.e., as upstream catchment area increased). Those authors tied variability in the patterns to context dependencies in environmental characteristics. ConUS-scale consistency in the patterns observed here for OM transformation contrasts with the context dependencies observed for OM functional diversity in Kida et al. (2021). We therefore encourage future studies to elucidate relationships between OM transformations and functional diversity.
While the number of abiotic transformations was far lower than biotic transformations both locally (i.e., within each site) and at the ConUS-scale (Fig. 2), abiotic transformations nonetheless play an important role in river corridors (Judd et al., 2007; Ward et al., 2017). For example, Fudyma et al. (2021) examined biochemical transformations in the river corridor and found that abiotic transformations in surface water modified the chemistry of OM entering the hyporheic zone, with subsequent impacts on respiration rates. Soares et al. (2019) also recently found that abiotic transformations of OM can lead to increases in bioavailable OM as residence time of surface water increases. These demonstrations of the importance of abiotic transformations further emphasize that the number of transformations observed here is a quantification of transformation diversity, not functional importance. That is, small sets of transformations can serve vital functional roles and can connect sets or “modules” of transformations together (Fudyma et al., 2021).
As noted above, our results suggest that OM transformations in surface water may reflect processes occurring across the upstream catchment, while OM transformations in sediment may reflect processes within the sampled volume. This inference was further supported by non-significant relationships between surface water and sediments in terms of transformation counts (Fig. 3). That is, the number of abiotic transformations in surface water was not related to the number of abiotic transformations in sediments. This analysis was done on paired samples, with data for surface water coming from the same stream reach as data for sediments. This allowed for regression-based analyses. The number of biotic transformations and the abiotic-to-biotic ratio were also uncorrelated between surface water and sediments. Extending the analyses to transformation composition further supports a disconnect between surface water and sediment OM transformation profiles. That is, we observed no meaningful relationship between surface water and sediment OM transformation compositional dissimilarity (Figs. 4 and S1 in the Supplement). As discussed in the “Methods” section, if hydrologic transport was overwhelming localized processes, we would have observed a clear positive relationship. Instead, a very weak relationship was observed (R2 = 0.04), indicating that influences of transport are very small relative to localized processes. This may be conceptualized similarly to the Damköhler number, whereby the ratio of the reaction influence to the transport influence is very large.
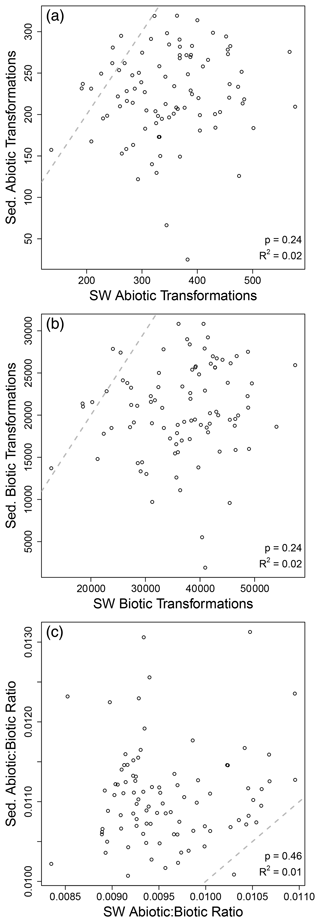
Figure 3Sediment (Sed.) and surface water (SW) transformation counts were not related to each other. Regression analysis of the number of abiotic (a) and biotic (b) transformations and their ratio (c). Each open circle is from one sampling site at which surface water and sediments were both collected. Regression statistics are provided in each panel, and the dashed line is the 1-to-1 line; no regressions were significant.
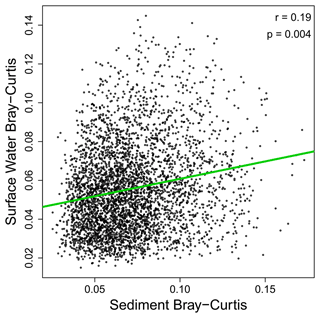
Figure 4Transformation profiles of OM in sediments and surface water were weakly related to each other. Bray–Curtis dissimilarities in surface water and sediments are plotted against each other, with their relationship evaluated via the Mantel test to control for non-independence among data points (see “Methods” section). The Pearson correlation coefficient and the Mantel-based p value are provided in the panel. While significant, the relationship is extremely weak, suggesting lack of a meaningful relationship. One outlier sample was discovered and excluded from this analysis. Figure S1 includes the outlier, which does not change the interpretation; it only makes it harder to see the data.
The lack of correlation between transformation counts and composition between surface water and sediment OM indicates at least a partial decoupling of the processes governing OM transformations in surface water and sediments. In this case, bi-directional exchanges (i.e., hyporheic exchange) (Harvey and Gooseff, 2015) of water and OM between surface water and the sediments are not strong enough to overwhelm processes occurring within each subsystem. It was recently proposed that OM assemblages can be thought of in terms of ecological community assembly processes including stochastic dispersal and deterministic selection (Danczak et al., 2020, 2021). From this ecological perspective, our results indicate that the rate of dispersal (i.e., transport) of OM from surface water into sediments is not sufficient to overcome the influences of localized, deterministic processes that cause systematic differences (among molecules) in the rates of production and transformation. Here, OM production and transformation are analogous to organismal birth and death, respectively (Danczak et al., 2020). It is unclear, however, what factors and processes within the sediments impose deterministic selection over molecular production and transformation. We hypothesize that a suite of factors are at work, such as redox conditions and sediment mineralogy. For example, the profile of organic molecules can be influenced by sorption, desorption, and transformations associated with organo-mineral interactions (Mead and Goñi, 2008; Zhou and Broodbank, 2014; Le Gaudu et al., 2022). It is also plausible that lower OM diversity in sediments, relative to surface water (Garayburu-Caruso et al., 2020b), could be due to organo-mineral interactions selecting for and against certain types of organic molecules (Aufdenkampe et al., 2007; Kleber et al., 2007, 2021). It is these kinds of localized interactions that we propose as overcoming strong coherence between surface water and sediment OM that may otherwise occur via transport and mixing. Spatial variation in mineralogy, redox, and other physicochemical properties may therefore help explain variation across sediments in the number of observed transformations.
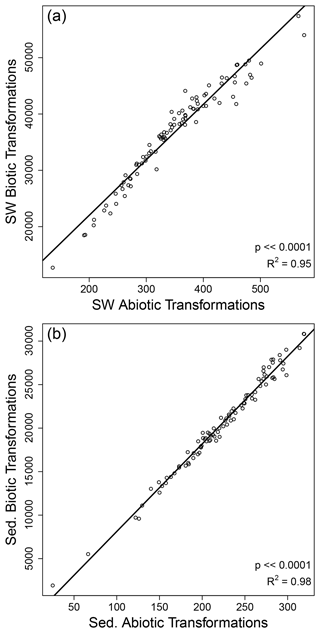
Figure 5Strong correlations were observed between the number of biotic and abiotic organic matter transformations within surface water (SW) and within sediment (Sed.). Each circle represents one sampled site for surface water (a) and sediments (b). The solid black line is the regression model, and statistics are provided in each panel.
In contrast to the decoupling between OM transformations in surface water and sediments, we observed strong correlations between the number of biotic and abiotic transformations within surface water and within sediment (Fig. 5). As discussed above, the number of transformations is best interpreted as a measure of transformation richness, as opposed to an indication of rates. The strong correlation between biotic and abiotic transformation counts therefore indicates that the diversity of biotic transformations tracks closely with the diversity of abiotic transformations. This suggests that systems in which a larger range of biochemical mechanisms contribute to OM production and transformation are also characterized by a larger range of abiotic mechanisms contributing to OM transformations. In considering this inference, it is important to recognize that the correlation between biotic and abiotic transformation counts may be influenced by among-sample variation in the number of observed molecules. However, among-sample variation in the number of observed molecules is not an artifact. This is because higher OM transformation richness should lead to a larger number of unique organic molecules. That is, the number of observed molecules and the level of OM transformation richness are mechanistically linked to each other, whereby richness can beget more richness. This lends credence to our inferences above but also emphasizes that additional insights can be gleaned by controlling for among-sample variation in the number of observed molecules.
To control for among-sample variation in the number of observed molecules we quantified the within-site abiotic-to-biotic ratio. This ratio was significantly higher in sediments than in surface water. The close spatial proximity between OM and mineral surfaces in sediments may contribute to relatively higher frequency of abiotic transformations in sediments. This may be associated, in part, with sorption and desorption processes (Kleber et al., 2021), though OM compositional change associated with desorption in the hyporheic zone can be strongly linked to microbially mediated transformations (Zhou et al., 2019). In addition, a larger diversity of redox conditions and thus more diverse redox species (Briggs et al., 2013; Boano et al., 2014; Lewandowski et al., 2019) in sediments could also contribute to the larger relative contribution of abiotic transformations in sediments. This does not discount the important role of abiotic transformations in surface water, such as those associated with photooxidation. Indeed, it is well known that abiotic transformations in surface water can strongly influence watershed carbon cycling fluxes (Ward et al., 2017; Bowen et al., 2020; Hu et al., 2021).
In addition to comparing transformations across river corridor subsystems, we conducted a preliminary investigation of spatial and climate correlates (e.g., mean annual temperature) of transformation numbers. This revealed non-significant (p > 0.05) or very weak (R2 < 0.1) relationships in all cases (see figures in the Supplement). We also performed multiple-regression analyses, and even models with five spatial and climate variables showed very low explanatory power (e.g., R2 < 0.08 for the model explaining variation in total transformations). Low explanatory power of space and climate is surprising given the continental-scale variation in OM chemistry revealed in the same dataset used here. That is, Garayburu-Caruso et al. (2020b) found a significant increase in sediment mean nominal oxidation state of organic carbon (NOSC) in the eastern US, relative to the western US. The lack of relationships shown here indicates that large-scale drivers of OM chemistry are not the same factors that drive variation in the number of transformations or the abiotic-to-biotic transformation ratio. A major remaining challenge is, therefore, to elucidate what drives variation in the absolute and relative numbers of abiotic and biotic OM transformations and understand relationships between transformations and functional diversity of attributes such as NOSC.
While it is unclear what drives variation in transformation numbers across river corridors, our ConUS-scale analyses provided insights that are likely applicable across all river corridors. In particular, processes governing OM transformations appear to be distinct between surface water and hyporheic zone sediments. This is unexpected given the bidirectional exchange of materials between surface water and sediments (Boano et al., 2014; Harvey and Gooseff, 2015). It also highlights that while hydrologically driven mixing can stimulate biogeochemical processes in hyporheic zones (McClain et al., 2003; Stegen et al., 2016), it generally does not homogenize OM between surface water and sediments (Stegen et al., 2018; Fudyma et al., 2021). Instead, we propose that OM observed in each subsystem is the result of biochemical transformations mediated by distinct processes. We emphasize that this inference extends only to the analytical limits of the FTICR-MS data used here, which do not provide a comprehensive survey of all possible transformations. However, no analytical method can provide a comprehensive survey. Among currently available methods, FTICR-MS provides the highest resolving power to enable the most comprehensive non-targeted surveys of organic molecules in environmental samples (Bahureksa et al., 2021). As such, using additional methods (e.g., liquid chromatography MS) will increase the number of putative transformations inferred in each sample, but the total number of transformations should be dominated by those inferred from FTICR-MS data. We encourage use of multiple complementary methods in future studies as this can be a powerful approach (Kim et al., 2006; Hagel and Facchini, 2008; Wolfender et al., 2015; Wilson and Tfaily, 2018; Kamjunke et al., 2019; Tfaily et al., 2019). We hypothesize, however, that using multiple methods will not modify our primary inference. That is, surface OM transformation counts are likely influenced by upstream catchment processes, while sediment OM is likely influenced by processes local to the sample volume. These observations further highlight the need to study and model river corridors through a multi-scale perspective.
Scripts to reproduce the primary results of this paper are available in Stegen et al. (2021) (https://doi.org/10.15485/1839188).
Data to reproduce the primary results of this paper are available in Stegen et al. (2021) (https://doi.org/10.15485/1839188). The data were retrieved from published data packages (Toyoda et al., 2020, https://doi.org/10.15485/1603775; Goldman et al., 2020, https://doi.org/10.15485/1729719).
The supplement related to this article is available online at: https://doi.org/10.5194/bg-19-3099-2022-supplement.
JCS contributed to conceptualization, formal analysis, funding acquisition, investigation, methodology, project administration, software, supervision, validation, visualization, and writing (original draft, review, and editing). SJF contributed to conceptualization, formal analysis, investigation, methodology, software, validation, visualization, and writing (original draft, review, and editing). MMT contributed to conceptualization, investigation, methodology, and writing (review and editing). VAG-C contributed to data curation, investigation, and writing (review and editing). AEG contributed to data curation, investigation, and writing (review and editing). RED contributed to data curation, investigation, software, and writing (review and editing). RKC contributed to data curation, investigation, and writing (review and editing). LR contributed to data curation, investigation, and writing (review and editing). JeT contributed to data curation, investigation, and writing (review and editing). JaT contributed to data curation, investigation, and writing (review and editing).
The contact author has declared that neither they nor their co-authors have any competing interests.
Publisher's note: Copernicus Publications remains neutral with regard to jurisdictional claims in published maps and institutional affiliations.
This work was supported by the US Department of Energy (DOE) Office of Science Early Career Research Program at Pacific Northwest National Laboratory (PNNL). PNNL is operated by Battelle for the US DOE under contract DE-AC05-76RL01830. This study used data from the Worldwide Hydrobiogeochemistry Observation Network for Dynamic River Systems (WHONDRS) under the River Corridor Science Focus Area (SFA) at PNNL. The SFA is supported by the US DOE, Office of Biological and Environmental Research (BER), Environmental System Science (ESS) program. A portion of this research was performed at the Environmental Molecular Sciences Laboratory, a DOE Office of Science user facility sponsored by the Biological and Environmental Research program under contract no. DE-AC05-76RL01830 and user proposal 51180. We thank Sophia McKever for generating Fig. 1.
This research has been supported by the US Department of Energy (grant no. 74193).
This paper was edited by Ji-Hyung Park and reviewed by Peter Herzsprung and one anonymous referee.
Amon, R. M. W. and Benner, R.: Photochemical and microbial consumption of dissolved organic carbon and dissolved oxygen in the Amazon River system, Geochim. Cosmochim. Ac., 60, 1783–1792, https://doi.org/10.1016/0016-7037(96)00055-5, 1996.
Aufdenkampe, A. K., Mayorga, E., Hedges, J. I., Llerena, C., Quay, P. D., Gudeman, J., Krusche, A. V., and Richey, J. E.: Organic matter in the Peruvian headwaters of the Amazon: Compositional evolution from the Andes to the lowland Amazon mainstem, Org. Geochem., 38, 337–364, https://doi.org/10.1016/j.orggeochem.2006.06.003, 2007.
Bahureksa, W., Tfaily, M. M., Boiteau, R. M., Young, R. B., Logan, M. N., McKenna, A. M., and Borch, T.: Soil Organic Matter Characterization by Fourier Transform Ion Cyclotron Resonance Mass Spectrometry (FTICR MS): A Critical Review of Sample Preparation, Analysis, and Data Interpretation, Environ. Sci. Technol., 55, 9637–9656, https://doi.org/10.1021/acs.est.1c01135, 2021.
Battin, T. J., Kaplan, L. A., Newbold, J. D., and Hendricks, S. P.: A mixing model analysis of stream solute dynamics and the contribution of a hyporheic zone to ecosystem function*, Freshwater Biol., 48, 995–1014, https://doi.org/10.1046/j.1365-2427.2003.01062.x, 2003.
Bauer, J. E., Cai, W.-J., Raymond, P. A., Bianchi, T. S., Hopkinson, C. S., and Regnier, P. A. G.: The changing carbon cycle of the coastal ocean, Nature, 504, 61–70, https://doi.org/10.1038/nature12857, 2013.
Bernhardt, E. S., Blaszczak, J. R., Ficken, C. D., Fork, M. L., Kaiser, K. E., and Seybold, E. C.: Control Points in Ecosystems: Moving Beyond the Hot Spot Hot Moment Concept, Ecosystems, 20, 665–682, https://doi.org/10.1007/s10021-016-0103-y, 2017.
Boano, F., Harvey, J. W., Marion, A., Packman, A. I., Revelli, R., Ridolfi, L., and Wörman, A.: Hyporheic flow and transport processes: Mechanisms, models, and biogeochemical implications, Rev. Geophys., 52, 603–679, https://doi.org/10.1002/2012RG000417, 2014.
Bowen, J. C., Kaplan, L. A., and Cory, R. M.: Photodegradation disproportionately impacts biodegradation of semi-labile DOM in streams, Limnol. Oceanogr., 65, 13–26, https://doi.org/10.1002/lno.11244, 2020.
Boye, K., Noël, V., Tfaily, M. M., Bone, S. E., Williams, K. H., Bargar, J. R., and Fendorf, S.: Thermodynamically controlled preservation of organic carbon in floodplains, Nat. Geosci., 10, 415–419, https://doi.org/10.1038/ngeo2940, 2017.
Breitling, R., Ritchie, S., Goodenowe, D., Stewart, M. L., and Barrett, M. P.: Ab initio prediction of metabolic networks using Fourier transform mass spectrometry data, Metabolomics, 2, 155–164, https://doi.org/10.1007/s11306-006-0029-z, 2006.
Briggs, M. A., Lautz, L. K., Hare, D. K., and González-Pinzón, R.: Relating hyporheic fluxes, residence times, and redox-sensitive biogeochemical processes upstream of beaver dams, Freshw. Sci., 32, 622–641, https://doi.org/10.1899/12-110.1, 2013.
Cole, J. J., Prairie, Y. T., Caraco, N. F., McDowell, W. H., Tranvik, L. J., Striegl, R. G., Duarte, C. M., Kortelainen, P., Downing, J. A., Middelburg, J. J., and Melack, J.: Plumbing the Global Carbon Cycle: Integrating Inland Waters into the Terrestrial Carbon Budget, Ecosystems, 10, 172–185, https://doi.org/10.1007/s10021-006-9013-8, 2007.
Creed, I. F., McKnight, D. M., Pellerin, B. A., Green, M. B., Bergamaschi, B. A., Aiken, G. R., Burns, D. A., Findlay, S. E. G., Shanley, J. B., Striegl, R. G., Aulenbach, B. T., Clow, D. W., Laudon, H., McGlynn, B. L., McGuire, K. J., Smith, R. A., and Stackpoole, S. M.: The river as a chemostat: fresh perspectives on dissolved organic matter flowing down the river continuum, Can. J. Fish. Aquat. Sci., 72, 1272–1285, https://doi.org/10.1139/cjfas-2014-0400, 2015.
Danczak, R. E., Chu, R. K., Fansler, S. J., Goldman, A. E., Graham, E. B., Tfaily, M. M., Toyoda, J., and Stegen, J. C.: Using metacommunity ecology to understand environmental metabolomes, Nat. Commun., 11, 6369, https://doi.org/10.1038/s41467-020-19989-y, 2020.
Danczak, R. E., Goldman, A. E., Chu, R. K., Toyoda, J. G., Garayburu-Caruso, V. A., Tolić, N., Graham, E. B., Morad, J. W., Renteria, L., Wells, J. R., Herzog, S. P., Ward, A. S., and Stegen, J. C.: Ecological theory applied to environmental metabolomes reveals compositional divergence despite conserved molecular properties, Sci. Total Environ., 788, 147409, https://doi.org/10.1016/j.scitotenv.2021.147409, 2021.
Fick, S. E. and Hijmans, R. J.: WorldClim 2: new 1-km spatial resolution climate surfaces for global land areas, Int. J. Climatol., 37, 4302–4315, https://doi.org/10.1002/joc.5086, 2017.
Fischer, H., Kloep, F., Wilzcek, S., and Pusch, M. T.: A river's liver–microbial processes within the hyporheic zone of a large lowland river, Biogeochemistry, 76, 349–371, 2005.
Fisher, S. G. and Likens, G. E.: Energy Flow in Bear Brook, New Hampshire: An Integrative Approach to Stream Ecosystem Metabolism, Ecol. Monogr., 43, 421–439, https://doi.org/10.2307/1942301, 1973.
Fudyma, J. D., Chu, R. K., Graf Grachet, N., Stegen, J. C., and Tfaily, M. M.: Coupled Biotic-Abiotic Processes Control Biogeochemical Cycling of Dissolved Organic Matter in the Columbia River Hyporheic Zone, Frontiers in Water, 2, 574692, https://doi.org/10.3389/frwa.2020.574692, 2021.
Garayburu-Caruso, V. A., Stegen, J. C., Song, H.-S., Renteria, L., Wells, J., Garcia, W., Resch, C. T., Goldman, A. E., Chu, R. K., Toyoda, J., and Graham, E. B.: Carbon Limitation Leads to Thermodynamic Regulation of Aerobic Metabolism, Environ. Sci. Technol. Letters, 7, 517–524, https://doi.org/10.1021/acs.estlett.0c00258, 2020a.
Garayburu-Caruso, V. A., Danczak, R. E., Stegen, J. C., Renteria, L., Mccall, M., Goldman, A. E., Chu, R. K., Toyoda, J., Resch, C. T., Torgeson, J. M., Wells, J., Fansler, S., Kumar, S., and Graham, E. B.: Using Community Science to Reveal the Global Chemogeography of River Metabolomes, Metabolites, 10, 518, https://doi.org/10.3390/metabo10120518, 2020b.
Goldman, A. E., Chu, R. K., Danczak, R. E., Daly, R. A., Fansler, S., Garayburu-Caruso, V. A., Graham, E. B., McCall, M. L., Ren, H., and Renteria, L.:WHONDRS Summer 2019 Sampling Campaign: Global River Corridor Sediment FTICR-MS, NPOC, and Aerobic Respiration, [data set], ESS-DIVE, https://doi.org/10.15485/1729719, 2020.
Graham, E. B., Crump, A. R., Kennedy, D. W., Arntzen, E., Fansler, S., Purvine, S. O., Nicora, C. D., Nelson, W., Tfaily, M. M., and Stegen, J. C.: Multi 'omics comparison reveals metabolome biochemistry, not microbiome composition or gene expression, corresponds to elevated biogeochemical function in the hyporheic zone, Sci. Total Environ., 642, 742–753, https://doi.org/10.1016/j.scitotenv.2018.05.256, 2018.
Hagel, J. M. and Facchini, P. J.: Plant metabolomics: analytical platforms and integration with functional genomics, Phytochem. Rev., 7, 479–497, https://doi.org/10.1007/s11101-007-9086-9, 2008.
Harvey, J. and Gooseff, M.: River corridor science: Hydrologic exchange and ecological consequences from bedforms to basins, Water Resour. Res., 51, 6893–6922, https://doi.org/10.1002/2015WR017617, 2015.
He, W., Choi, I., Lee, J.-J., and Hur, J.: Coupling effects of abiotic and biotic factors on molecular composition of dissolved organic matter in a freshwater wetland, Sci. Total Environ., 544, 525–534, https://doi.org/10.1016/j.scitotenv.2015.12.008, 2016.
Holt, A. D., Kellerman, A. M., Li, W., Stubbins, A., Wagner, S., McKenna, A., Fellman, J., Hood, E., and Spencer, R. G. M.: Assessing the Role of Photochemistry in Driving the Composition of Dissolved Organic Matter in Glacier Runoff, J. Geophys. Res.-Biogeo., 126, e2021JG006516, https://doi.org/10.1029/2021JG006516, 2021.
Hough, M., McCabe, S., Vining, S. R., Pedersen, E. P., Wilson, R. M., Lawrence, R., Chang, K., Bohrer, G., The IsoGenie Coordinators, Riley, W., Crill, P., Varner, R. K., Blazewicz, S. J., Dorrepaal, E., Tfaily, M. M., Saleska, S. R., and Rich, V.: Coupling plant litter quantity to a novel metric for litter quality explains C storage changes in a thawing permafrost peatland, Glob. Change Biol., 28, 950–968, https://doi.org/10.1111/gcb.15970, 2022.
Hu, B., Wang, P., Wang, C., and Bao, T.: Photogeochemistry of particulate organic matter in aquatic systems: A review, Sci. Total Environ., 806, 150467, https://doi.org/10.1016/j.scitotenv.2021.150467, 2021.
Jones Jr, J. B.: Factors controlling hyporheic respiration in a desert stream, Freshwater Biol., 34, 91–99, https://doi.org/10.1111/j.1365-2427.1995.tb00426.x, 1995.
Judd, K. E., Crump, B. C., and Kling, G. W.: Bacterial responses in activity and community composition to photo-oxidation of dissolved organic matter from soil and surface waters, Aquat. Sci., 69, 96–107, https://doi.org/10.1007/s00027-006-0908-4, 2007.
Kamjunke, N., Hertkorn, N., Harir, M., Schmitt-Kopplin, P., Griebler, C., Brauns, M., von Tümpling, W., Weitere, M., and Herzsprung, P.: Molecular change of dissolved organic matter and patterns of bacterial activity in a stream along a land-use gradient, Water Res., 164, 114919, https://doi.org/10.1016/j.watres.2019.114919, 2019.
Kida, M., Fujitake, N., Kojima, T., Tanabe, Y., Hayashi, K., Kudoh, S., and Dittmar, T.: Dissolved Organic Matter Processing in Pristine Antarctic Streams, Environ. Sci. Technol., 55, 10175–10185, https://doi.org/10.1021/acs.est.1c03163, 2021.
Kim, S., Kaplan, L. A., and Hatcher, P. G.: Biodegradable dissolved organic matter in a temperate and a tropical stream determined from ultra-high resolution mass spectrometry, Limnol. Oceanogr., 51, 1054–1063, https://doi.org/10.4319/lo.2006.51.2.1054, 2006.
Kleber, M., Sollins, P., and Sutton, R.: A conceptual model of organo-mineral interactions in soils: self-assembly of organic molecular fragments into zonal structures on mineral surfaces, Biogeochemistry, 85, 9–24, https://doi.org/10.1007/s10533-007-9103-5, 2007.
Kleber, M., Bourg, I. C., Coward, E. K., Hansel, C. M., Myneni, S. C. B., and Nunan, N.: Dynamic interactions at the mineral–organic matter interface, Nature Reviews Earth & Environment, 2, 402–421, https://doi.org/10.1038/s43017-021-00162-y, 2021.
Le Gaudu, M., Thiebault, T., Quénéa, K., Alliot, F., Guigon, E., and Le Callonnec, L.: Trace organic contaminants within solid matrices along an anthropized watercourse: Organo-mineral controls on their spatial distribution, Sci. Total Environ., 822, 153601, https://doi.org/10.1016/j.scitotenv.2022.153601, 2022.
Lewandowski, J., Arnon, S., Banks, E., Batelaan, O., Betterle, A., Broecker, T., Coll, C., Drummond, J. D., Gaona Garcia, J., Galloway, J., Gomez-Velez, J., Grabowski, R. C., Herzog, S. P., Hinkelmann, R., Höhne, A., Hollender, J., Horn, M. A., Jaeger, A., Krause, S., Löchner Prats, A., Magliozzi, C., Meinikmann, K., Mojarrad, B. B., Mueller, B. M., Peralta-Maraver, I., Popp, A. L., Posselt, M., Putschew, A., Radke, M., Raza, M., Riml, J., Robertson, A., Rutere, C., Schaper, J. L., Schirmer, M., Schulz, H., Shanafield, M., Singh, T., Ward, A. S., Wolke, P., Wörman, A., and Wu, L.: Is the Hyporheic Zone Relevant beyond the Scientific Community?, Water, 11, 2230, https://doi.org/10.3390/w11112230, 2019.
Li, B., Liu, X., Kaufman, M. H., Turetcaia, A., Chen, X., and Cardenas, M. B.: Flexible and Modular Simultaneous Modeling of Flow and Reactive Transport in Rivers and Hyporheic Zones, Water Resour. Res., 56, e2019WR026528, https://doi.org/10.1029/2019WR026528, 2020.
Liu, Q., Liang, Y., Cai, W.-J., Wang, K., Wang, J., and Yin, K.: Changing riverine organic C:N ratios along the Pearl River: Implications for estuarine and coastal carbon cycles, Sci. Total Environ., 709, 136052, https://doi.org/10.1016/j.scitotenv.2019.136052, 2020.
Marshall, A. G., Hendrickson, C. L., and Jackson, G. S.: Fourier transform ion cyclotron resonance mass spectrometry: A primer, Mass Spectrom. Rev., 17, 1–35, https://doi.org/10.1002/(SICI)1098-2787(1998)17:1<1::AID-MAS1>3.0.CO;2-K, 1998.
McClain, M. E., Boyer, E. W., Dent, C. L., Gergel, S. E., Grimm, N. B., Groffman, P. M., Hart, S. C., Harvey, J. W., Johnston, C. A., and Mayorga, E.: Biogeochemical hot spots and hot moments at the interface of terrestrial and aquatic ecosystems, Ecosystems, 6, 301–312, 2003.
Mead, R. N. and Goñi, M. A.: Matrix protected organic matter in a river dominated margin: A possible mechanism to sequester terrestrial organic matter?, Geochim. Cosmochim. Ac., 72, 2673–2686, https://doi.org/10.1016/j.gca.2008.03.007, 2008.
Naegeli, M. W. and Uehlinger, U.: Contribution of the Hyporheic Zone to Ecosystem Metabolism in a Prealpine Gravel-Bed-River, J. N. Am. Benthol. Soc., 16, 794–804, https://doi.org/10.2307/1468172, 1997.
Running, S., Mu, Q., and Zhao, M.: MOD16A3 MODIS/Terra Net Evapotranspiration Yearly L4 Global 500m SIN Grid V006, https://doi.org/10.5067/MODIS/MOD16A3.006, 2017.
Schlesinger, W. H. and Melack, J. M.: Transport of organic carbon in the world's rivers, Tellus, 33, 172–187, https://doi.org/10.3402/tellusa.v33i2.10706, 1981.
Schlünz, B. and Schneider, R. R.: Transport of terrestrial organic carbon to the oceans by rivers: re-estimating flux- and burial rates, Int. J. Earth Sci., 88, 599–606, https://doi.org/10.1007/s005310050290, 2000.
Sengupta, A., Fansler, S. J., Chu, R. K., Danczak, R. E., Garayburu-Caruso, V. A., Renteria, L., Song, H.-S., Toyoda, J., Wells, J., and Stegen, J. C.: Disturbance triggers non-linear microbe–environment feedbacks, Biogeosciences, 18, 4773–4789, https://doi.org/10.5194/bg-18-4773-2021, 2021.
Soares, A. R. A., Lapierre, J.-F., Selvam, B. P., Lindström, G., and Berggren, M.: Controls on Dissolved Organic Carbon Bioreactivity in River Systems, Sci. Rep., 9, 14897, https://doi.org/10.1038/s41598-019-50552-y, 2019.
Song, H.-S., Stegen, J. C., Graham, E. B., Lee, J.-Y., Garayburu-Caruso, V. A., Nelson, W. C., Chen, X., Moulton, J. D., and Scheibe, T. D.: Representing Organic Matter Thermodynamics in Biogeochemical Reactions via Substrate-Explicit Modeling, Front. Microbiol., 11, 531756, https://doi.org/10.3389/fmicb.2020.531756, 2020.
Stegen, J. C. and Goldman, A. E.: WHONDRS: a Community Resource for Studying Dynamic River Corridors, mSystems, 3, e00151-18, https://doi.org/10.1128/mSystems.00151-18, 2018.
Stegen, J. C., Fredrickson, J. K., Wilkins, M. J., Konopka, A. E., Nelson, W. C., Arntzen, E. V., Chrisler, W. B., Chu, R. K., Danczak, R. E., Fansler, S. J., Kennedy, D. W., Resch, C. T., and Tfaily, M.: Groundwater–surface water mixing shifts ecological assembly processes and stimulates organic carbon turnover, Nat. Commun., 7, 11237, https://doi.org/10.1038/ncomms11237, 2016.
Stegen, J. C., Johnson, T., Fredrickson, J. K., Wilkins, M. J., Konopka, A. E., Nelson, W. C., Arntzen, E. V., Chrisler, W. B., Chu, R. K., Fansler, S. J., Graham, E. B., Kennedy, D. W., Resch, C. T., Tfaily, M., and Zachara, J.: Influences of organic carbon speciation on hyporheic corridor biogeochemistry and microbial ecology, Nat. Commun., 9, 585, https://doi.org/10.1038/s41467-018-02922-9, 2018.
Stegen, J. C., Fansler, S. J., Tfaily, M. M., Garayburu-Caruso, V. A., Goldman, A. E., Danczak, R. E., Toyoda, J. G., Chu, R., Renteria, L., and Tagestad, J. D.: FTICR-MS and biochemical transformation data from global inland river water and sediment associated with: “Organic matter transformations are disconnected between surface water and the hyporheic zone”, [data set], ESS-DIVE, https://doi.org/10.15485/1839188, 2021.
Stewart, B., Shanley, J. B., Kirchner, J. W., Norris, D., Adler, T., Bristol, C., Harpold, A. A., Perdrial, J. N., Rizzo, D. M., Sterle, G., Underwood, K. L., Wen, H., and Li, L.: Streams as mirrors: reading subsurface water chemistry from stream chemistry, Water Resour. Res., 58, e2021WR029931, https://doi.org/10.1029/2021WR029931, 2021.
Tfaily, M. M., Wilson, R. M., Brewer, H. M., Chu, R. K., Heyman, H. M., Hoyt, D. W., Kyle, J. E., and Purvine, S. O.: Single-throughput Complementary High-resolution Analytical Techniques for Characterizing Complex Natural Organic Matter Mixtures, JoVE (Journal of Visualized Experiments), e59035, https://doi.org/10.3791/59035, 2019.
Tolić, N., Liu, Y., Liyu, A., Shen, Y., Tfaily, M. M., Kujawinski, E. B., Longnecker, K., Kuo, L.-J., Robinson, E. W., Paša-Tolić, L., and Hess, N. J.: Formularity: Software for Automated Formula Assignment of Natural and Other Organic Matter from Ultrahigh-Resolution Mass Spectra, Anal. Chem., 89, 12659–12665, https://doi.org/10.1021/acs.analchem.7b03318, 2017.
Toyoda, J. G., Goldman, A. E., Chu, R. K., and Danczak, R. E.: WHONDRS Summer 2019 Sampling Campaign: Global River Corridor Surface Water FTICR-MS and Stable Isotopes [data set], ESS-DIVE, https://doi.org/10.15485/1603775, 2020.
Vannote, R. L., Minshall, G. W., Cummins, K. W., Sedell, J. R., and Cushing, C. E.: The River Continuum Concept, Can. J. Fish. Aquat. Sci., 37, 130–137, https://doi.org/10.1139/f80-017, 1980.
Ward, C. P., Nalven, S. G., Crump, B. C., Kling, G. W., and Cory, R. M.: Photochemical alteration of organic carbon draining permafrost soils shifts microbial metabolic pathways and stimulates respiration, Nat. Commun., 8, 772, https://doi.org/10.1038/s41467-017-00759-2, 2017.
Ward, N. D., Sawakuchi, H. O., Neu, V., Less, D. F. S., Valerio, A. M., Cunha, A. C., Kampel, M., Bianchi, T. S., Krusche, A. V., Richey, J. E., and Keil, R. G.: Velocity-amplified microbial respiration rates in the lower Amazon River, Limnol. Oceanogr. Letters, 3, 265–274, https://doi.org/10.1002/lol2.10062, 2018.
Wetzel, R. G.: Death, detritus, and energy flow in aquatic ecosystems, Freshwater Biol., 33, 83–89, https://doi.org/10.1111/j.1365-2427.1995.tb00388.x, 1995.
Wilson, R. M. and Tfaily, M. M.: Advanced Molecular Techniques Provide New Rigorous Tools for Characterizing Organic Matter Quality in Complex Systems, J. Geophys. Res.-Biogeo., 123, 1790–1795, https://doi.org/10.1029/2018JG004525, 2018.
Wolfender, J.-L., Marti, G., Thomas, A., and Bertrand, S.: Current approaches and challenges for the metabolite profiling of complex natural extracts, J. Chromatogr. A, 1382, 136–164, https://doi.org/10.1016/j.chroma.2014.10.091, 2015.
Xenopoulos, M. A., Downing, J. A., Kumar, M. D., Menden-Deuer, S., and Voss, M.: Headwaters to oceans: Ecological and biogeochemical contrasts across the aquatic continuum, Limnol. Oceanogr., 62, 3–14, https://doi.org/10.1002/lno.10721, 2017.
Zhou, C., Liu, Y., Liu, C., Liu, Y., and Tfaily, M. M.: Compositional changes of dissolved organic carbon during its dynamic desorption from hyporheic zone sediments, Sci. Total Environ., 658, 16–23, https://doi.org/10.1016/j.scitotenv.2018.12.189, 2019.
Zhou, J. and Broodbank, N.: Sediment-water interactions of pharmaceutical residues in the river environment, Water Res., 48, 61–70, https://doi.org/10.1016/j.watres.2013.09.026, 2014.