the Creative Commons Attribution 4.0 License.
the Creative Commons Attribution 4.0 License.
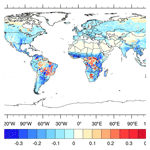
Global evaluation of terrestrial biogeochemistry in the Energy Exascale Earth System Model (E3SM) and the role of the phosphorus cycle in the historical terrestrial carbon balance
Xiaojuan Yang
Peter Thornton
Daniel Ricciuto
Yilong Wang
Forrest Hoffman
The importance of carbon (C)–nutrient interactions to the prediction of future C uptake has long been recognized. The Energy Exascale Earth System Model (E3SM) land model (ELM) version 1 is one of the few land surface models that include both N and P cycling and limitation (ELMv1-CNP). Here we provide a global-scale evaluation of ELMv1-CNP using the International Land Model Benchmarking (ILAMB) system. We show that ELMv1-CNP produces realistic estimates of present-day carbon pools and fluxes. Compared to simulations with optimal P availability, simulations with ELMv1-CNP produce better performance, particularly for simulated biomass, leaf area index (LAI), and global net C balance. We also show ELMv1-CNP-simulated N and P cycling is in good agreement with data-driven estimates. We compared the ELMv1-CNP-simulated response to CO2 enrichment with meta-analysis of observations from similar manipulation experiments. We show that ELMv1-CNP is able to capture the field-observed responses for photosynthesis, growth, and LAI. We investigated the role of P limitation in the historical balance and show that global C sources and sinks are significantly affected by P limitation, as the historical CO2 fertilization effect was reduced by 20 % and C emission due to land use and land cover change was 11 % lower when P limitation was considered. Our simulations suggest that the introduction of P cycle dynamics and C–N–P coupling will likely have substantial consequences for projections of future C uptake.
- Article
(6683 KB) -
Supplement
(2683 KB) - BibTeX
- EndNote
This manuscript has been authored by UT-Battelle, LLC under contract no. DE-AC05-00OR22725 with the U.S. Department of Energy. The United States Government retains and the publisher, by accepting the article for publication, acknowledges that the United States Government retains a non-exclusive, paid-up, irrevocable, world-wide license to publish or reproduce the published form of this manuscript, or allow others to do so, for United States Government purposes. The Department of Energy will provide public access to these results of federally sponsored research in accordance with the DOE Public Access Plan (http://energy.gov/downloads/doe-public-access-plan, last access: 12 September 2019).
The recent global carbon (C) budget showed that over the last half century global fossil CO2 emissions have increased from about 3 Pg C yr−1 in the 1960s to about 9.5 Pg C yr−1 in the last decade (Friedlingstein et al., 2019). It has also been shown that land ecosystems play important roles in controlling the fractions of CO2 emissions that remain in the atmosphere by taking up about 29 % of total emissions (Le Quéré et al., 2018). Large uncertainties remain on the net land–atmosphere C exchange, mainly due to difficulties in quantifying the complex C cycle processes such as CO2 fertilization effects, responses of carbon fluxes to temperature and precipitation variation, and C emissions associated with land use and land cover change (LULCC). These uncertainties will very likely hamper our ability to predict the future trajectories of atmospheric CO2.
One of the important uncertainties relates to our understanding of C–nutrient interactions and nutrient limitation and how they are represented in models. The importance of nitrogen (N) availability to predicted land C storage has been long recognized (Hungate et al., 2003). Although there were only two models in CMIP5 (the Coupled Model Intercomparison Project Phase 5) that accounted for N dynamics and N limitation (Thornton et al., 2007, 2009; Arora et al., 2013), many ESMs participating in CMIP6 (the Coupled Model Intercomparison Project Phase 6) now include N cycle and C–N interactions (Davies-Barnard et al., 2020; Lawrence et al., 2019; Goll et al., 2017a; Smith et al., 2014; Sellar et al., 2019). The comparisons between these models have been summarized in Arora et al. (2020) and Davies-Barnard et al. (2020). In recent years, significant efforts have also gone into understanding phosphorus (P) cycle dynamics and the role of P limitation in land C storage (Jiang et al., 2019; Hou et al., 2020; Reed et al., 2015; Wieder et al., 2015b; Sun et al., 2017). Increasing numbers of models have developed the capability to include P cycle processes and C–N–P interactions (Wang et al., 2010; Goll et al., 2012; Thum et al., 2019; Goll et al., 2017b; Yang et al., 2014, 2019; Sun et al., 2021). It has been shown that considering P cycle dynamics and C–N–P interactions improves process representation and model fidelity compared with observational and experimental data in most models (Goll et al., 2017b; Yang et al., 2014). Model simulations have also demonstrated the importance of P limitation to land C uptake (Zhang et al., 2014; Goll et al., 2012; Yang et al., 2016, 2019; Sun et al., 2021). Using an ensemble of 14 terrestrial ecosystem models to simulate the planned free-air CO2 enrichment experiment AmazonFACE, Fleischer et al. (2019) showed that P availability reduced the projected CO2-induced C sink by about 50 % compared to estimates from models assuming no phosphorus limitation. Taken together, understanding and representation of the role of P cycle dynamics in affecting terrestrial C balance are essential for the prediction of future terrestrial carbon uptake and atmospheric CO2 concentration.
Field and modeling studies have shown that forest productivity tends to increase with increasing soil phosphorus availability (Vicca et al., 2012; Aragão et al., 2009; Wang et al., 2010). Despite these recent efforts, P cycle dynamics and C–N–P interactions are not yet included in most CMIP6 models. The Energy Exascale Earth System Model (E3SM) is one of the few models that have been developed with a coupled C–N–P capability in the land component in CMIP6 (Burrows et al., 2020). The land model in E3SM, herein referred to as ELMv1-CNP, has been first applied in the Amazon region to test its capability and to evaluate the importance of P limitation in this region (Yang et al., 2019). Yang et al. (2019) provides an in-depth evaluation of ELMv1-CNP for the Amazon rainforest using field observational data, with a focus on how the introduction of P cycle dynamics and P limitation improved model-simulated spatial variation of productivity. They show that effects of P limitation on C sources and sinks in the Amazon region are significant, reducing simulated CO2 fertilization of new carbon uptake by as much as 31 %.
This study expands the analysis in the Amazon region to the global scale and has two main aims: (1) to provide an evaluation of ELMv1-CNP performance on the global scale using both observational and experimental data and (2) to quantify the role of P cycle dynamics and P limitation in affecting simulated C sources and sinks globally. We first evaluate the performance of ELMv1-CNP using the International Land Model Benchmarking (ILAMB) benchmarking system (Collier et al., 2018), which has been widely used in the evaluation of land surface models and ESMs (Lawrence et al., 2019; Bonan et al., 2019; Zhu et al., 2019; Friedlingstein et al., 2019). We then evaluate ELMv1-CNP-simulated N and P pools and fluxes with an observation-based dataset. Realizing that the static benchmarking may not help constrain future model projections, we further evaluate ELMv1-CNP using experimental manipulations of atmospheric CO2. Finally, we take advantage of the P-enabled capability in ELMv1-CNP to quantify the effect of P dynamics on the simulated ecosystem responses to increasing atmospheric CO2, increasing N deposition, LULCC, and climate change on the global scale.
2.1 Model overview
ELMv1-CNP is based on the Community Land Model version 4.5 (CLM4.5), which includes coupled C–N biogeochemistry from CLM4 (Thornton et al., 2007) and improvements to canopy photosynthesis, soil biogeochemistry, and representation of nitrogen cycle dynamics (Koven et al., 2013; Bonan et al., 2011; Oleson et al., 2013). Recognizing the critical role of the tropical forests in the global carbon cycle and C–climate interactions and the important role of P cycle dynamics and P limitation in tropical forests, we implemented a fully prognostic P cycle and C–N–P interactions into ELMv1-CNP, enabling ELMv1-CNP to be one of the few land surface models that include both N and P cycle dynamics and limitation. The main model features include (1) a fully prognostic P cycle tracking various soil inorganic P pools, vegetation P pools, and litter and soil organic P pools; (2) the representation of P limitation on plant productivity and litter and soil organic matter decomposition based on a supply–demand approach; (3) resolving N vs. P limitation using the Liebig law; (4) the vertically resolved soil inorganic and organic P dynamics; (5) the decoupling of the P cycle from the C and N cycle during decomposition due to phosphatase activity; and (6) the representation of adsorption–desorption dynamics based on soil order.
Besides the P cycling processes, the other important difference of ELMv1-CNP from CLM4.5 is the removal of instantaneous downregulation of photosynthesis from nutrient limitation. Instead, longer-term downregulation of productivity is enabled through the implementation of C, N, and P nonstructural vegetation storage pools. In CLM4.5, nutrient limitation is calculated at each time step as a function of potential gross primary productivity (GPP), stoichiometry of plant tissues, and nitrogen uptake. Any excess carbon due to nitrogen limitation is immediately released to the atmosphere through instantaneous downregulation. This nutrient limitation can be highly variable over time and affects diurnal and seasonal cycles of gross primary productivity, which is not consistent with flux tower observations (Ghimire et al., 2016) or with short-term elevated CO2 experiments that were done with and without nutrient fertilization (Metcalfe et al., 2017). In the current model, competition for available nutrients and plant uptake still occur every time step given instantaneous demand that is a function of plant GPP and microbial nutrient immobilization (Oleson et al., 2013). However, nutrients taken up by plants are now first allocated to non-structural N and P storage pools instead of directly to structural pools. Nutrient limitation to allocation is determined by comparing plant nutrient demand (given GPP and stoichiometry) and the nutrient availability from the non-structural nutrient pools, which is a function of the pool size in relation to long-term demand. The excess carbon flux, which cannot be allocated due to nutrient limitation, is directed to the non-structural plant carbon (NSC) pool instead of downregulating GPP. This pool respires to the atmosphere with a given turnover time. Details about the representation of NSC can be found in the supporting information (Text S1)
The model version used in this study is the publicly released ELMv1 and can be downloaded along with all the parameter files at https://github.com/E3SM-Project/E3SM, last access: 12 September 2019. In this version of the model, the fire module is activated by default. The soil erosion module is not activated. We assume soil C, N, and P cycling can take place up to a 3.8 m depth as the assumption in CLM4.5 (Koven et al., 2013). We also provide the key model parameters in Table S1 (PFT specific) and Table S2 (soil order specific). We note that only leaf parameters vary with plant functional type (PFT), but we include all other tissues in Table S1 to provide all parameters in the consistent format.
2.2 Simulations
The simulations presented here were first spun up to bring C, N, and P pools to equilibrium by recycling the GSWP3 (Global Soil Wetness Project Phase 3) climate forcing data (http://hydro.iis.u-tokyo.ac.jp/GSWP3/, last access: 13 August 2019) between 1901–1920, along with constant atmospheric CO2, N deposition, and land cover type for the year of 1850. Spinup was accomplished through two steps: accelerated decomposition (AD) spinup and regular spinup. We ran the model for 250 years in the AD spinup mode. The purpose of the AD spinup is to accelerate the decomposition process and speed up the spinup process of the carbon and nutrient cycles. The AD spinup procedure was modified from that originally described by Thornton and Rosenbloom (2005), which used spatially invariant acceleration factors to accelerate decomposition in soil organic matter (SOM) pools. Here we updated the AD spinup by including the impacts of temperature and soil moisture on the acceleration factor. This resulted in higher acceleration factors in cool and/or dry climates, which are typically slower to achieve steady state. In addition, vegetation dead stem and coarse root mortality were accelerated by a factor of 10 to achieve steady-state biomass more quickly. The factor of 10 was chosen to have a good balance between faster acceleration and the disequilibrium between accelerated and non-accelerated steady states that requires a longer regular spinup following Koven et al. (2013). In the AD spinup, supplemental soil mineral P was applied for the entire simulation such that there was no P limitation on C and N dynamics. During the transition between AD spinup and regular spinup, we initialized the soil inorganic pools using global P maps developed by Yang et al. (2013). For the grid cells that do not have values in Yang et al. (2013), we applied the nearest-neighbor method to estimate the values. Since the P cycle involves both biological and geochemical processes that occur on geological timescales, the initialization of P pools provides some reasonable estimates of soil P pools without running the model for millions of simulated years. More details regarding the rationale of using the developed P maps for initialization can be found in Yang et al. (2013). We then ran normal spinup for 600 years with active C, N, and P coupled biogeochemistry until C, N, and P pools reached equilibrium. The criteria for equilibrium are for global total net ecosystem exchange (NEE) less than 0.1 Pg C yr−1 averaged over 100 years, the threshold recommended for the C4MIP (Jones et al., 2016). We also ran a control simulation between 1850–2010 as a continuation of the normal spinup. We added the time series of labile P, secondary mineral P, and occluded P for the control simulation (Fig. S1). There are very few changes in the inorganic P pools during the 161 years control simulation, suggesting that these pools can be considered in equilibrium for the timescale of our interest.
After the model was spun up, we ran the global historical transient simulations (1850–2010) at 0.5∘ spatial resolution using GSWP3 v2 climate forcing data, along with historical transient atmospheric CO2 concentration, N deposition, and land use and land cover change that are part of the CMIP6 protocols (https://luh.umd.edu/data.shtml, last access: 13 August 2019). Input data and references are summarized in Table S3. We also ran a suite of single-factor simulations to examine the individual effects of changing environmental factors (atmospheric CO2, land use and land cover change, climate, and nitrogen deposition, Table 1). In addition to the ELMv1 simulations with a fully active P cycle, we also performed historical transient and single-factor simulations with P limitation switched off (supplementing P availability to fully meet demand at each grid cell and for each time step so there is no P limitation on productivity and decomposition). We denoted the default ELMv1 simulations that have an active P cycle as the CNP configuration (ELMv1-CNP) and simulations assuming no P limitation as the CN configuration (ELMv1-CN).
Table 1Summary of model simulations.
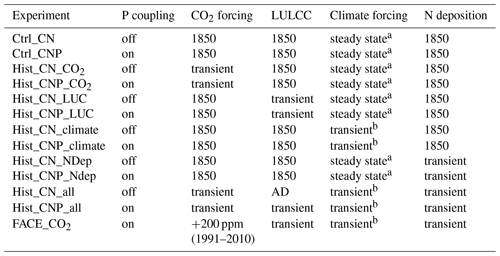
a Cycling of 20-year time series of GSWP3 reanalysis product (1901–1920). b Historical time series of GSWP3 reanalysis product (1901–2010).
We also performed one additional simulation where we initiated a global step increase in atmospheric CO2 concentration, by +200 ppm, starting from 2001 and continuing through 2010. These simulations are designed to mimic the Free Air CO2 Enrichment (FACE) experiments (Ainsworth and Long, 2005). To quantify model sensitivities to elevated CO2, we calculated the effect size (treatment divided by control) over the 10 years of simulation (2001–2010). We then evaluated model sensitivities to elevated CO2 against meta-analysis from FACE experiments (Ainsworth and Long, 2005).
All of the simulations are summarized in Table 1.
2.3 ILAMB
We used the International Land Model Benchmarking system (Collier et al., 2018; Luo et al., 2012; Hoffman et al., 2017) to assess the model performance. ILAMB was designed to use a wide array of observational data to constrain model results, including various land carbon pools and fluxes, inferred CO2 concentration variability, and functional relationships. For each variable, ILAMB scores model performance for period mean, bias, root-mean-square error (RMSE), spatial distribution, interannual coefficient of variation, seasonal cycle, and long-term trend. These scores are aggregated into an overall score representing multiple aspects of model performance for each variable. These aggregated absolute scores are then used to calculate the relative score, which indicates the relative performance of each model with respect to other models used in the same analysis. The observational datasets used for the evaluation of carbon cycle in ILAMB are listed in Table S4.
In order to understand how the implementation of P cycling dynamics affects model performance, we evaluated the performance of both ELMv1-CNP and ELMv1-CN. In order to provide a context in terms of model performance in ILAMB, we provide the ILAMB evaluation of several other land models included in the Land Surface, Snow and Soil Moisture Model Intercomparison Project (LS3MIP) as part of CMIP6 (https://www.wcrp-climate.org/wgcm-cmip/wgcm-cmip6, last access: 16 July 2020). LS3MIP includes a collection of model experiments including both offline land model experiments and coupled experiments (van den Hurk et al., 2016). We used the results from the offline land model experiments. Like our simulations, these experiments were performed at 0.5∘ by 0.5∘ spatial resolution and using the GSWP3 forcing data. Other model configurations in LS3MIP are identical to that used in CMIP6 historical simulations, which we used for the simulations in this study.
2.4 GOLUM-CNP
Since there is no nutrient cycle metric in ILAMB, we also compared major N and P pools and fluxes along with nutrient use efficiencies from ELMv1-CNP with the data-driven estimates of N and P pools and fluxes from the Global Observation-based Land-ecosystems Utilization Model of Carbon, Nitrogen, and Phosphorus (GOLUM-CNP) (Wang et al., 2018). GOLUM-CNP combines data-driven estimates of N and P inputs and outputs and observed stoichiometric ratios with a steady-state diagnostic model, providing global steady-state N and P pools and fluxes for large biomes. Despite large uncertainties and the steady-state assumptions, GOLUM-CNP provides a global data-driven product that can be used to test nutrient cycles in land surface models. GOLUM-CNP has also been used in the evaluation of other land surface models (Sun et al., 2021).
3.1 Evaluations of ELMv1 using ILAMB
ILAMB includes many metrics that cover water, energy, and carbon pools and fluxes on both regional and global scales. Figure 1 shows ILAMB benchmarking scores for ELMv1-CNP and ELMv1-CN, along with several other land models in CMIP6, which are provided to contextualize ILAMB scores for ELMv1-CNP. The relative model performance scores are shown in Fig. 1, indicating which model version performs better with respect to others. The full results produced by the ILAMB package can be found at https://compy-dtn.pnl.gov/yang954/_build/, last access: 24 July 2020.
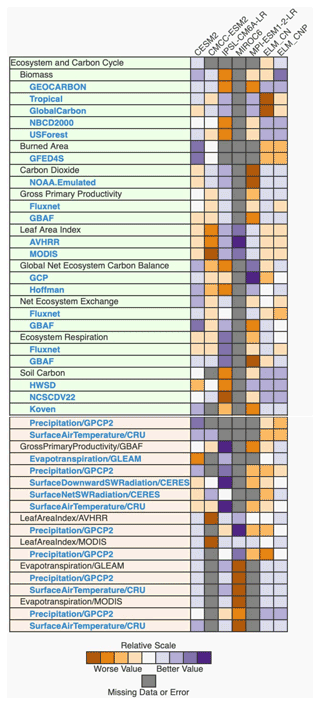
Figure 1ILAMB carbon cycle scores for ELMv1-CNP and ELM-CN and a few land models in CMIP6. Shown here is the relative score, indicating the performance of each model relative to other models. References for benchmarking data for each variable are provided in Table S4. The datasets that are in green boxes are either carbon pools or fluxes, while the datasets in orange boxes are relationships between carbon pools/fluxes and environmental variables such as precipitation or temperature. Outputs for other land models are from the LS3MIP offline simulations archive in CMIP6. These simulations were performed using the same resolution and forcing data as this study. CLM4.5 is the land model in CMCC-ESM2. CLM5 is the land model for CESM2. ORCHIDEE is the land model for IPSL. JSBACH is the land model for MPI-ESM1.2. VISIT is the land model for MIROC6.
Figure 1 shows that the performance of ELMv1-CNP is comparable to other land models in CMIP6. ELMv1-CNP exhibits performance similar to CLM5 (CESM2) in terms of aggregated scores for carbon cycle metrics, while CLM5 shows better performance with respect to overall functional relationships, mainly due to a better score for the functional relationship of burned area. The performance of each model varies for different variables. For example, the ORCHIDEE land surface model in IPSL-CM6A-LR performs relatively well in inferred atmospheric carbon dioxide, leaf area index, and GPP relationships.
Figure 1 also shows the comparison between ELMv1-CNP and ELMv1-CN, allowing us to quantify the impacts of including a prognostic P cycle and realistic P availability on model performance. For metrics in Fig. 1 that show the greatest differences between ELMv1-CNP and ELMv1-CN, the CNP version always has a higher score than CN. This is reflected in the relatively higher aggregated scores for carbon cycle variables and functional relationships in ELMv1-CNP.
Figure 2 shows the global net ecosystem carbon balance metric in ILAMB for ELMv1-CNP and ELMv1-CN. The observational datasets for this metric are from the Global Carbon Project (Fig. 2a) (Le Quéré et al., 2016) and from the inversion-based estimate (Hoffman et al., 2014), both providing global totals of land carbon accumulation but for different historical time periods (1850–2010 for Hoffman et al., 2014, and 1959–2010 for Le Quéré et al., 2016). The simulated global C balance by both ELMv1-CNP and ELMv1-CN is in the range of uncertainty of observational estimates, with ELMv1-CNP-simulated historical global carbon accumulation being a better match with mean observational estimates, particularly after 1950. ELMv1-CN estimated a net accumulation of land carbon of 22 Pg C over the period 1850–2010, which is much higher than the mean observational estimate of −8 Pg C. ELMv1-CNP estimated a land carbon accumulation of 7 Pg C.
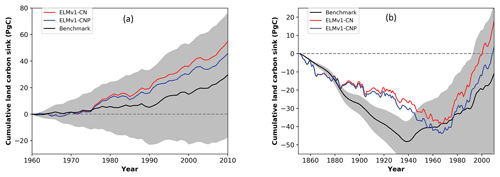
Figure 2ELMv1-CNP and ELMv1-CN-simulated global land carbon accumulation for the time period (a) 1960–2010 and (b) and 1850–2010. Benchmark data (black lines with uncertainty estimate in grey) are from (a) Global Carbon Project (Le Quéré et al., 2016) and (b) Hoffman et al. (2014).
Figure 3 shows the spatial distribution of vegetation biomass for the benchmark data and model bias in ILAMB. Overall both ELMv1-CN and ELMv1-CNP tend to overestimate biomass, compared to this specific global product of biomass (GEOCARBON). The high bias in the tropical region is much reduced in ELMv1-CNP simulations (Fig. 3a, b and c). The better performance of ELMv1-CNP is also reflected in the spatial Taylor diagram for biomass (Fig. 3d).
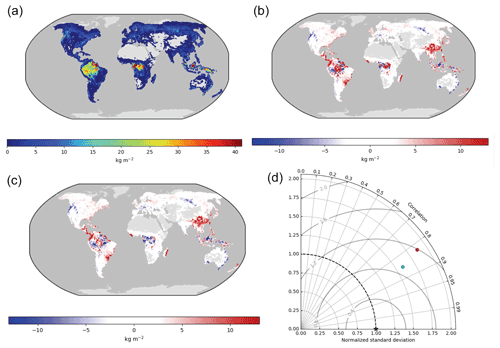
Figure 3Global pattern of simulated biomass: (a) benchmark data, (b) ELMv1-CN bias, (c) ELMv1-CNP bias, and (d) spatial Taylor diagram for model–benchmark comparison (red dot is for ELMv1-CN and blue dot is for ELMv1-CNP). Benchmark data here are from the GEOCARBON product (Saatchi et al., 2011).
Another important benchmark in ILAMB is the functional relationships between two variables, for example the relationship between GPP and precipitation and the relationship between annual mean leaf area index (LAI) and precipitation. An accurate simulation of these relationships in addition to individual benchmarks is an indication that the models are representing the underlying processes correctly. ELMv1-CNP produces a better functional relationship compared to ELMv1-CN. For example, for the relationship between LAI and precipitation, ELMv1-CN overestimated LAI, particularly in regions with high precipitation, while the ELMv1-CNP configuration shows an improved relationship (Fig. 4). The improvement of the functional relationship is mainly due to the improvement in high-precipitation regions, e.g., lowland tropical forest regions. In these regions, inclusion of P dynamics and P limitation reduced simulated bias in GPP and LAI, therefore leading to a better match with the observations.
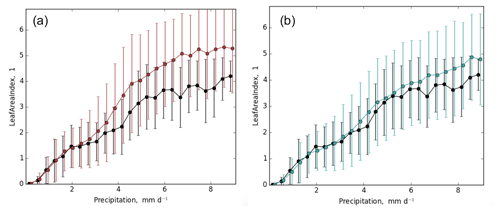
Figure 4ILAMB relationship plot between LAI and climatological annual precipitation and (a) ELMv1-CN (b) ELMv1-CNP. Black line is the observationally derived relationship. Error bars indicate 1 standard deviation of LAI for all grid cells within the precipitation bin. Observed LAI is from the MODIS LAI product.
3.2 Evaluation of N and P cycling in ELMv1-CNP
We evaluated simulated nutrient use efficiencies against that from the GOLUM-CNP product on the biome level. Here we define nutrient use efficiency as the ratio between annual net primary productivity (NPP) and annual nutrient uptake (for both N and P), with NUE for nitrogen use efficiency and PUE for phosphorus use efficiency (Finzi et al., 2007). ELMv1-CNP-simulated NUE is higher in temperate and boreal forests and lower in tropical grassland and tundra, which is consistent with GOLUM-CNP (Fig. 5a). Temperate grassland NUE and PUE in ELMv1-CNP are higher in distribution because of the higher variation in NPP allocation to non-structural carbon pools. ELMv1-CNP predicted higher NUE in tropical lowland forests than GOLUM-CNP. ELMv1-CNP-simulated PUE is generally consistent with GOLUM-CNP (Fig. 5b). However, ELMv1-CNP-simulated PUE in tropical forests is much lower than that from GOLUM-CNP.
We also evaluated ELMv1-CNP-simulated N and P pools and major fluxes on the global scale for the period of 2001–2010 with the observationally derived products in GOLUM-CNP. Figure S2 shows the comparison of N and P uptake from ELMv1-CNP and GOLUM-CNP at the biome level. ELMv1-CNP-simulated plant N and P uptake is in agreement with GOLUM-CNP, with higher uptake fluxes in tropical forests and lower uptake in temperate and boreal forests. ELMv1-CNP-simulated N uptake is lower in the tropical forests, compared to GOLUM-CNP (Fig. S2a). Conversely, simulated P uptake is higher than GOLUM-CNP estimates across the tropics (Fig. S2b).
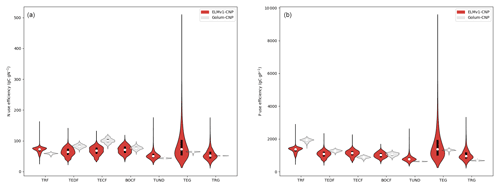
Figure 5Violin plots of (a) nitrogen use efficiency (NUE) and (b) phosphorus use efficiency (PUE) from ELMv1-CNP and GOLUM-CNP for seven biomes: tropical rainforest (TRF), temperate deciduous forest (TEDF), temperate coniferous forest (TECF), boreal coniferous forest (BOCF), temperate grassland (TEG), and tropical grassland (TRG). Plots show the medians of all grid cells in each biome (open circles) and the probability density distribution (balloons).
3.3 Evaluations using CO2 manipulation experiment
Relative to the control simulation, increasing atmospheric CO2 concentration by 200 ppm increased gross primary productivity by 23 % (global mean) over the 10 years of simulation (2001–2010). Nearly all PFTs showed more than a 10 % increase in productivity, with more significant increases occurring in tropical regions and middle latitudes (Fig. 6a). The modeled response ratio of NPP also shows widespread increases, and on the global scale our results showed a 25.8 % increase in NPP in response to CO2 enrichment (Fig. 6b). The simulated increases in GPP and NPP, to a large extent, translated into increases in vegetation carbon (Fig. 6c), with a global average response ratio of 18 %. The modeled response ratio of LAI is much smaller, with a 5 % increase globally (Fig. 6d). The globally aggregated simulated effect size of CO2 enrichment from ELMv1-CNP on GPP, NPP, LAI, and NSC compares well to the observations from the meta-analysis (Fig. 7), particularly for GPP and LAI. ELMv1-CNP overestimated the responses of NPP. Both observations and simulations show large sensitivity of NSC to CO2 enrichment, with larger variability in the model simulations.
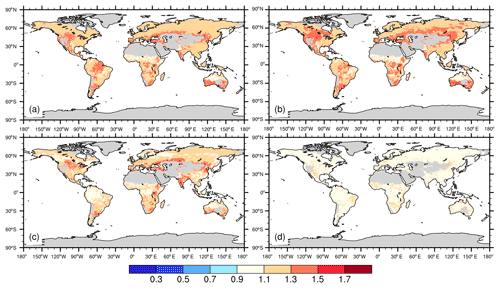
Figure 6Spatial distribution of the effect size of CO2 enrichment on (a) GPP, (b) NPP, (c) vegetation carbon, and (d) LAI. Effect sizes were calculated for each grid cell as the mean annual values of GPP, NPP, vegetation carbon, and LAI from CO2 enrichment simulation divided by those from the control simulations between 2001–2010.
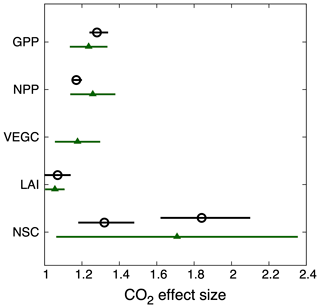
Figure 7Observed (open circles) and simulated (green triangles) effect size of CO2 enrichment on GPP, NPP, LAI, vegetation carbon, and non-structural carbon. Observations show the mean (± 95 % confidence interval; Ainsworth and Long, 2005). There are two observations of NSC shown here: one is for sugar with a mean value of 1.3 and the other is for starch with a mean value of 1.8, while model conceptualization of NSC includes both sugar and starch. Simulated responses show the global mean effect sizes (± standard deviation; calculated to provide an estimate of spatial variation).
3.4 Carbon, nitrogen, and phosphorus pools and fluxes
3.4.1 Carbon budget
Major components of the global land C budget for the present day (mean of 2001–2010) in ELMv1-CNP are shown in Fig. 8a. These are from historical simulations with transient climate forcing, atmospheric CO2 concentration, land use and land cover change, and N deposition. For the present day, model-simulated total ecosystem C is 2588.73 Pg C, with about 22 % stored in vegetation (575.45 Pg C), about 5 % stored in litter and coarse wood debris (122.5 Pg C), and 73 % stored in soil organic matter (1890.78 Pg C). Model-simulated vegetation C is within the range of inventory-based estimates from IPCC AR5 (450–650 Pg C). Our simulated vegetation C is also comparable to or slightly higher than observational estimates from the literature: 455 Pg C (GEOCARBON, Avitabile et al., 2016; Santoro et al., 2015), 550 ± 100 Pg C (Houghton, 2003), 560 ± 94 Pg C (Defries et al., 1999), and 450 Pg C (Erb et al., 2018). Model-simulated total soil C is within the range of estimates from IPCC AR5 (1500–2400 Pg C) and that from Jobbágy and Jackson (2000) (1750 ± 250 Pg C). Model-simulated total soil C is lower than several other observational estimates from the literature: 2376–2456 Pg C (Batjes, 2014) and 3000 Pg C (Köchy et al., 2015), which could be because ELMv1-CNP has yet to include an explicit representation of peatland carbon dynamics. As for the top 1 m of soil carbon, model-simulated values of 1134.41 Pg C are within the range of estimates from the Harmonized World Soil Database (HWSD) (FAO/IIASA/ISRIC/ISSCAS/JRC, 2012) as reported by Todd-Brown et al. (2013) (890–1660 Pg C) but lower than the observation-based estimate of 1462–1548 Pg C from Batjes (2014) and 1325 Pg C from Köchy et al. (2015). Model-simulated litter C (22.9 Pg C) is lower than the observation-based estimate: 68 Pg C (Matthews, 1997) and 43 ± 3 Pg C (Pan et al., 2011). Model-simulated coarse wood debris C stock (99.6 Pg C) is higher than the observation-based estimate: 75 Pg C (Matthews, 1997) and 73 ± 6 Pg C (Pan et al., 2011).
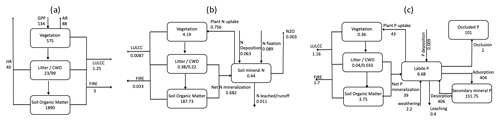
Figure 8(a) Terrestrial C cycle, (b) N cycle, and (c) P cycle as simulated by ELMv1-CNP, shown here are mean values between 2001–2010. Vegetation and soil C, N, and P pools are in units of petagrams of carbon (Pg C), petagrams of nitrogen (Pg N), and petagrams of phosphorus (Pg P), respectively. C and N fluxes are given in petagrams of carbon per year (Pg C yr−1) and petagrams of nitrogen per year (Pg N yr−1), and P fluxes are given in teragrams of phosphorus per year (Tg P yr−1). AR stands for autotrophic respiration and HR stands for heterotrophic respiration.
Model-simulated present-day GPP (134.15 Pg C yr−1) is slightly higher than the observation-based estimate, 123 ± 8 Pg C yr−1 (Beer et al., 2010), 119 ± 6 Pg C yr−1 (Jung et al., 2011), and 123 Pg C yr−1 (IPCC AR5), and lower than 150–175 Pg C yr−1 from Welp et al. (2011) that is derived based on oxygen isotopes of atmospheric CO2. A recent study based on satellite data suggested a global GPP of 140 Pg C yr−1 for year 2007 (Joiner et al., 2018). The comparisons between simulated carbon pools and fluxes and available observations are also included in Table 2.
3.4.2 Nitrogen budget
The ELMv1-CNP-estimated N budget for the present day (2001–2010) is summarized in Fig. 8b. Compared to the C cycle, there are fewer observational estimates for N pools and fluxes. Most of the literature values are from other model simulations. Although not appropriate for direct model evaluation, these modeling estimates from the literature provide a broad context for us to evaluate our simulated pools and fluxes.
Model-simulated vegetation N is 4.36 Pg N, which is comparable to the estimates from some other modeling studies, 3.8 Pg N (Zaehle et al., 2010) and 5.3 Pg N (Xu and Prentice, 2008), and lower than the estimates of 16 Pg N (Lin et al., 2000) and 18 Pg N (Yang et al., 2009). Model-simulated total soil organic matter N is 188.79 Pg N, which is reasonable considering the observation-based estimate for 1 m of 95 Pg N (Post et al., 1985) and 133–140 Pg N (Batjes, 2014). The ELMv1-CNP-estimated biological nitrogen fixation (BNF) of 89 Tg N yr−1 is within the range of estimates from the literature. Vitousek et al. (2013) estimated that global BNF ranges between 40–100 Tg N yr−1 using a mass-balance approach. A meta-analysis by Davies-Barnard and Friedlingstein (2020) suggested that global inputs of BNF in natural ecosystems range between 52 and 130 Tg N yr−1, with a median global value of 88 Tg N yr−1. For the purpose of comparison, the BNF estimate from CLM5 is 96.4 Tg N yr−1, which is slightly higher than our estimate. The comparisons between simulated N pools and fluxes and available observations are also included in Table 2.
3.4.3 Phosphorus budget
The ELMv1-CNP-estimated P budget for the present day (2001–2010) is summarized in Fig. 8c. Very few observational data are available for P on the global scale. The only observation-based global product is the global P maps developed by Yang et al. (2013). Model-simulated vegetation P is 0.36 Pg P, which is comparable to the estimates from other modeling studies ranging from 0.23 to 3 Pg P (Goll et al., 2012; Wang et al., 2010; Jahnke, 1992). Model-simulated soil organic P is 3.75 Pg P, which is slightly lower than previous studies: 5.74 Pg P (Goll et al., 2012), 5–10 Pg P (Smil, 2000), and 8.6 Pg (Yang et al., 2013). Model-simulated soil mineral P for the top 40 and 60 cm is 63.24 and 81.32 Pg P respectively, which are generally comparable to the estimate of 45 Pg P for the top 50 cm of soil from Yang et al. (2013). The comparisons between simulated P pools and fluxes and available observations are also included in Table 2.
3.5 The effects of P limitation on the historical carbon cycle
ELMv1-CNP calculates the extent of both N and P limitation for plant growth on the global scale (Fig. 9a and b). Generally speaking, P is a more limiting nutrient in tropical evergreen forests and savannas in South America and Africa, while N is more limiting in temperate regions (Fig. 9a). The ratio between the P limitation factor and N limitation factor illustrates the degree of N–P co-limitation (Fig. 9b). N and P are co-limiting productivity in tundra, boreal forests, and deserts.
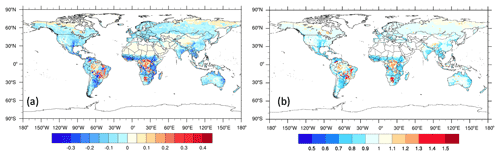
Figure 9(a) Spatial variation of the extent of nutrient limitation on plant growth. Regions with a negative value are more limited by N, while regions with a positive value are more limited by P. Larger absolute values are associated with stronger limitation. Values plotted are the proportion by which plant growth is reduced due to N limitation or P limitation: 1−fP when fP<fN and fN−1 when fN<fP, where fP is the limitation factor on plant growth considering P supply and demand, while fN is the limitation factor on plant growth considering N supply and demand (Yang et al., 2014). (b) Spatial variation of the ratios between P limitation and N limitation indicating the degree of co-limitation. Values plotted are the ratios between fN and . Regions with values less than 1 indicate more N limitation, and regions with values greater than 1 are more limited by P. Values close to 1 indicate NP co-limitation. The definition of co-limitation is subjective here, but a difference of 10 % or less between the values for fN and fP would lead to a range of about 0.9 to 1.1 in the plotted ratio.
Figure 10 shows the simulated spatial patterns of productivity and carbon storage and how they are affected by P dynamics and limitation. P dynamics strongly control land carbon uptake and storage, particularly in tropical regions. Globally NPP is highest in tropical evergreen forests and lower in middle- to high-latitude regions. Plant growth in tropical regions, however, is generally limited by P availability, particularly in the central and eastern Amazon basin and tropical Africa. The reduced productivity due to P limitation translates into reduced vegetation carbon storage and soil carbon storage, with the exception of tropical savannas, where fire dynamics also play an important role in vegetation and soil carbon storage.
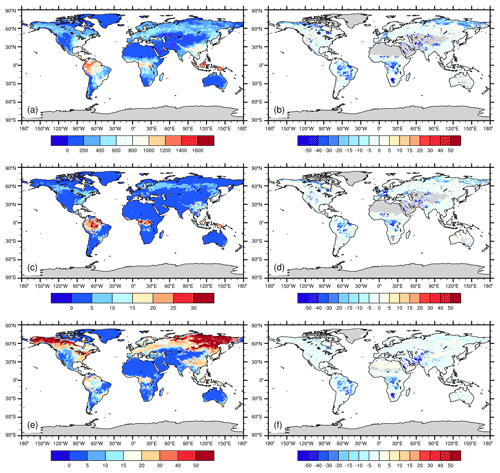
Figure 10Average estimates of (a) net primary productivity (g C m−2 yr−1), (c) vegetation carbon (kg C m−2), and (e) soil organic carbon (kg C m−2) for the years 2001–2010 and the effects of phosphorus dynamics (expressed as percentage deviation between CNP and CN configurations, unitless) on (b) net primary productivity, (d) vegetation carbon, and (f) soil carbon as estimated by ELMv1.
Figure 11 shows the effects of P dynamics on historical global land carbon accumulation. The introduction of P dynamics leads to a 19.5 % reduction in global C storage due to CO2 fertilization between 1850 and 2010. The consideration of P dynamics also leads to a lower estimate of land use emissions on the global scale (143.89 Pg C vs. 161.21 Pg C) as CNP simulations generally show lower initial vegetation biomass. Increasing N deposition generally leads to a small carbon accumulation between 1850 and 2010 in both CN and CNP simulations globally. With P limitation, however, the global carbon accumulation from N deposition is reduced by about a third. Climate, although responsible for the large seasonal and interannual variability of carbon fluxes, has only minor impacts on historical carbon accumulation on the global scale for both CN and CNP simulations. When changes of all environmental factors are considered, the impact of P dynamics on carbon accumulation is the balance between a smaller CO2 fertilization effect and lower land use emissions, with the net effect being slightly lower historical carbon accumulation globally.
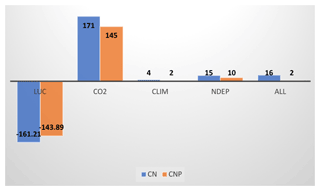
Figure 11Cumulative global carbon storage (Pg C) from 1850–2010 from ELMv1-CN and ELMv1-CNP simulations with changes in land use and land cover change (LUC), atmospheric CO2 (CO2), climate (CLIM), N deposition (NDEP), and all factors combined (ALL). These are calculated as the accumulation of NEE between 1850 and 2010 for the historical transient model simulations listed in Table 1.
4.1 ILAMB benchmarking
This study presents a global assessment of the ELMv1-CNP. Yang et al. (2019) evaluated the performance of ELMv1-CNP in the Amazon region using plot-level observations from the RAINFOR network and found that the model captures well the observed productivity and biomass gradient across the Amazon basin. Here we further evaluate the global model performance using the ILAMB benchmarking system – an open-source land model evaluation system that is designed to assess model performance at site-level, regional, and global scales in an integrated and comprehensive way.
We include several other land models in CMIP6 in our ILAMB analysis with the goal of providing a context for the performance of ELMv1-CNP. We found that ELMv1-CNP exhibits similar performance to other models. It is challenging to demonstrate a clear improvement or degradation for complex land surface models in ILAMB. For example, our analysis indicates that ELMv1-CNP performance is comparable to CLM5 in terms of the overall carbon cycle. Both ELMv1-CNP and CLM5 have a common ancestor CLM4.5, but they took very different approaches for further development. CLM5 had significant efforts undertaken in improving the representation of the nitrogen cycle, while ELMv1-CNP was more focused on implementing a prognostic phosphorus cycle and C–N–P interactions. Model development activities in both models helped improve model performance through the lens of ILAMB, but the sources of improvements are quite different. This highlights the need to include more process-level evaluations in ILAMB for the purpose of evaluating the impact of specific model improvements.
Although CLM5 and ELM-CNP perform similarly in terms of ILAMB scores, it is worth noting the unique role of P cycle dynamics in affecting C cycling and the importance of including P cycle limitation in earth system models for better prediction of carbon–climate feedbacks. The important role of soil P availability in affecting plant growth in tropical forests residing on highly weathered soils has long been recognized (Walker and Syers, 1976; Vitousek et al., 2010; Butler et al., 2018; Elser et al., 2007). Recent work has also explored how increasing demand for P may attenuate predicted increase in NPP conceptually by comparing potential demand with potential nutrient availably in the 21st Century (Wieder et al., 2015b; Sun et al., 2017). Increasing numbers of land models have incorporated P cycle dynamics and P limitations (Sun et al., 2021; Nakhavali et al., 2021). Although both N and P limitation acts through reducing NPP, it is critical to include P cycling explicitly in models since P cycle dynamics are very different from the N cycling dynamics. The primary input for P is through rock weathering, which makes it a very much non-renewable nutrient for the terrestrial ecosystems, whereas N fixation, the primary input for N, is more biologically driven. P cycling involves the transformation of various forms of P through a series of biological, enzymatical, and geochemical processes with the turnover time ranging from seconds to millions of years. N cycle dynamics are relatively simpler, with two inorganic forms and mostly biological and enzymatical processes involved. In addition, the interactions between N and P cycling also point to the need to include P cycle explicitly in land models. Increasing numbers of studies have shown that biological N fixation could be constrained by soil P availability (Hungate et al., 2004; Reed et al., 2013; Barron et al., 2008; Edwards et al., 2006; Crews et al., 2000). On the other hand, studies have also shown that increases in N availability can promote phosphatase activity and enhance biochemical mineralization and therefore accelerate P cycling (Mcgill and Cole, 1981; Wang et al., 2007; Houlton et al., 2008; Olander and Vitousek, 2000; Treseder and Vitousek, 2001; Marklein and Houlton, 2012). We will continue to refine and improve the representation of the C–N–P interactions in the future development of ELM.
Also, ILAMB, despite being a comprehensive benchmarking tool for land surface models, is limited in scope in terms of the benchmarking data included. For example, Quesada et al. (2012) found that the decreasing west–east gradient in productivity is mostly related to total soil P across the Amazon basin. Yang et al. (2019) showed that consideration of soil P availability improved model-simulated productivity, enabling the model to capture the productivity gradient from west to east across the Amazon basin. The problem is that this productivity gradient across the Amazon basin is not captured in ILAMB benchmark data so the failure of a CN model would not be captured by ILAMB.
We show that the model performance generally improved with realistic P availability through the implementation of a prognostic P cycle in ELM. Compared to ELMv1-CN, ELMv1-CNP-simulated biomass has lower bias across the tropical regions as P limitation leads to lower productivity and hence lower biomass. ELMv1-CNP produces better ILAMB scores on the functional relationships between GPP, LAI, and other forcing variables, mainly due to improved estimates of GPP and LAI in tropical regions. ELMv1-CNP also produces higher ILAMB scores for the integrated benchmarks such as global net ecosystem carbon balance and carbon dioxide concentration. We note that satisfactory performance for these two integrated metrics is most critical to a land model in ESMs as they are most relevant to the coupling between land ecosystems and radiatively forced climate change.
ELMv1-CNP is not always better than ELMv1-CN from the benchmarks in the current ILAMB system. One of the benefits of a multi-metric analysis package like ILAMB is that we can compare performance at different levels of granularity, and it is rare that any one model has uniformly improved performance over any other single model on every fine-grained metric. By having multiple data sources for a given metric we can often see improvement against one data source and degradation compared to another for the same model output. For example, the ELMv1-CN model performs better than ELMv1-CNP for ecosystem respiration when comparing the FLUXNET metric, but ELMv1-CNP does better than ELMv1-CN for the GBAF metric on the same output variable. In the case of GPP and NEE, although ELMv1-CN performs better or the same as ELMv1-CNP for both FLUXNET and GBAF metrics, the overall better scores of the ELMv1-CNP model for the relationship metrics connected to GPP give us more confidence that ELMv1-CNP is actually an improvement. Each metric has its own advantages and disadvantages, and there is still considerable subjectivity in how to interpret the multi-metric collection. For example, the site-level evaluations in ILAMB do not take into account site-specific disturbance histories, which can be an important driver of NEE variability over time at a given site.
Although the ILAMB benchmarking system is very useful for evaluating model performance from different aspects simultaneously, interpretation of ILAMB scores deserves extra caution with known observational bias considered. For example, ILAMB uses LAI estimated from remote sensing observations from the Moderate Resolution Imaging Spectroradiometer (MODIS) as benchmarking data, while studies have suggested that MODIS LAI may be biased low due to reflectance saturation in dense canopies in the tropical forests (Shabanov et al., 2005; Huete et al., 2002; Kobayashi and Dye, 2005). Another example is the observational data for biomass. There are significant differences between the tropical dataset and the GEOCARBON dataset for tropical biomass, but they were given about the same default weight in the ILAMB scoring system. Mitchard et al. (2014) investigated the marked differences between different estimates of Amazon biomass and suggested the regional biases in some remote sensing products might be due to the lack of consideration of ecological variation in tree wood density and allometry. Further investigation of these datasets is needed to ensure the quality of biomass benchmarking data.
The current version of ILAMB includes the analysis of 28 variables using more than 60 datasets or data products. None of these variables, however, are directly related to nutrient cycles. As more land surface models are implementing N and P dynamics, it is becoming increasingly important to include metrics for nutrient stocks and fluxes. Davies-Barnard et al. (2020) assessed five nitrogen-enabled land surface models in CMIP6 and called out the need to have better constraints of nitrogen cycle processes. The need is equally urgent, if not more, to synthesize more observations to better constrain the P cycle processes, as less synthesized data are available for P. Encouragingly, recent studies have started to develop observational datasets based estimate of N and P cycling on the global scale for model evaluation, such as the GOLUM-CNP dataset we used in this study. We hope to highlight the need and engage the broader community in developing additional nutrient datasets that can be included in ILAMB.
Other metrics that would be useful are the responses from N and P addition experiments. As Yang et al. (2014) showed, fertilization experiments at sites along the Hawaii chronosequence provided a useful evaluation test bed to assess model-simulated responses to N and P fertilization effects. FACE experiments are useful for model evaluation as shown here (Sect. 4.2) and in other studies (Wieder et al., 2019; Davies-Barnard et al., 2020). Warming studies that include an explicit focus on nutrient cycle responses will be another good evaluation opportunity (Melillo et al., 2002). An existing challenge is to provide a common protocol to use these types of experiments in the ILAMB benchmarking system.
4.2 Evaluations using GOLUM-CNP
On the biome level ELMv1-CNP-simulated nutrient use efficiencies are consistent with the observation-based estimates from GOLUM-CNP. This indicates that the representation of N and P cycling and C–N–P coupling is reasonable in ELMv1-CNP. In terms of nutrient uptake, both show the highest N and P uptake in tropical forests, due to the high N and P demand associated with high productivity. ELMv1-CNP predicted lower N uptake in the tropical forests, compared to GOLUM-CNP. Nutrient uptake in ELMv1-CNP is a function of nutrient availability and nutrient demand, with demand being determined by available carbon for allocation, allocation fractions to different plant tissues, and plant tissue stoichiometry. The simulated NPP at the biome level matches well with NPP from GOLUM-CNP except for Tundra (Fig. S3). The different C : N and C : P stoichiometric ratios for vegetation tissues used in ELMv1-CNP and GOLUM-CNP could contribute to the difference in nutrient uptake. C : N ratios of leaf, wood, and fine root in GOLUM-CNP are all lower than ELMv1-CNP (21, 126, and 40 in GOLUM vs. 30, 500, and 42 in ELMv1-CNP). This suggests for a given amount of carbon allocation, N uptake would be lower in ELMv1-CNP. Soil P availability might be overestimated considering ELMv1-CNP-estimated P leaching is much lower than the estimate of Wang et al. (2018), therefore leading to relatively higher P uptake in ELMv1-CNP. Differences in allocation factors could also be contributing to the differences in nutrient uptake between ELMv1-CNP and GOLUM-CNP. For example, the mean allocation fraction to fine root is higher in GOLUM-CNP compared to ELM-CNP, while allocation fraction to leaf is lower in GOLUM-CNP, particularly in forest ecosystems (Figs. S4 and S6). GOLUM-CNP also has higher NPP allocation fraction to woody biomass in boreal forests (Fig. S5)
4.3 Evaluations using CO2 manipulation experiments
Our simulated large increase in GPP with CO2 enrichment (23 %) is in agreement with field observations that photosynthetic assimilation increased 28 % under elevated CO2 (Ainsworth and Long, 2005). Our simulated 26 % increase in NPP is higher than the 17 % observed increase in dry matter production in the FACE experiments (Ainsworth and Long, 2005; Wieder et al., 2019). Our simulated 18 % increase in biomass is higher than the estimates from Terrer et al. (2019), which provide a data-driven estimate of global CO2 fertilization effect on biomass and show a relative increase in biomass of 12 ± 3 % for a 250 ppm CO2 increase. A meta-analysis of woody-plant responses to elevated CO2 shows a mean effect of 22.3 % on biomass (Baig et al., 2015). Among CLM4, CLM4.5, and CLM5, ELMv1-CNP is more comparable to CLM5 with a strong simulated response of GPP, NPP, and vegetation carbon in response to CO2 enrichment, while CLM4 and CLM4.5 showed very weak CO2 effects (Wieder et al., 2019).
The much stronger sensitivity of photosynthesis to elevated CO2 in ELMv1-CNP is due to the removal of instantaneous downregulation of photosynthesis as a response to nutrient limitation. The instantaneous downregulation assumption in CLM4 and CLM4.5 has been shown to be inconsistent with experimental results (Metcalfe et al., 2017). Despite large uncertainty, it is encouraging that simulated NSC response to elevated CO2 is largely consistent with the observational data (Fig. 7). The low sensitivity of LAI in ELMv1-CNP is also consistent with field observations. Our results suggest the assumption we made regarding the fate of photosynthate is reasonable. Yang et al. (2016) showed that enhanced phosphatase enzyme production response to increasing CO2 could have important impacts on P availability and sustain forest productivity under elevated CO2. In simulating the planned free-air CO2 enrichment experiment AmazonFACE, ELMv1-CNP-simulated phosphatase activity increased about 20 % over 15 years (Fleischer et al., 2019). Here we show that introduction of NSC pools further improves the response of vegetation processes to changes in P availability and P limitation.
Our findings are consistent with field studies that show the strong increase in NSC under elevated CO2 condition (eCO2), particularly when nutrient availability is low (Wong, 1990; Körner et al., 2005). Several studies evaluating CLM4.5 using carbon isotope data also suggested that model performance would be better with the introduction of an NSC pool (Mao et al., 2016; Raczka et al., 2016; Duarte et al., 2017). However, large uncertainties remain regarding the turnover rate of the NSC pool. Further syntheses of field measurements on NSC in CO2 enrichment experiments are needed to evaluate and constrain the representation of NSC in models.
Our simulated strong sensitivity of photosynthesis to CO2 enrichment is consistent with recent studies that show large GPP growth during the 20th century (Campbell et al., 2017; Haverd et al., 2020; Ehlers et al., 2015). Ellsworth et al. (2017) also showed a large increase in photosynthesis in response to elevated CO2 in a temperate forest FACE experiment.
The increased sensitivity of GPP and NPP to CO2 enrichment in ELMv1-CNP, compared with the predecessors CLM4 and CLM4.5, will very likely reduce the bias in the atmospheric fraction of human CO2 emissions in previous coupled simulations as noted by Hoffman et al. (2014). In fact, CO2 concentration metrics in ILAMB, which translate model-simulated NEE into atmospheric CO2 signal using an atmospheric transport model (Collier et al., 2018), are intended for the evaluation of this sensitivity. The inferred atmospheric CO2 concentration from ELMv1 is very reasonable compared with observed NOAA flask data (Figs. S7 and S8).
4.4 Model-estimated carbon, nitrogen, and phosphorus pools and fluxes
Global C, N, and P pools in our ELMv1-CNP simulation are in good agreement with recent independent global estimates, indicating that ELMv1-CNP is capable of simulating the contemporary C, N, and P cycles. In Yang et al. (2019) it was shown that introduction of more realistic mortality processes improved the model representation of longitudinal spatial patterns of biomass across the Amazon basin. Here we show that an overall high bias in biomass production is corrected through limits of vegetation production in response to P availability, without compromising the improved spatial gradients obtained through the mortality mechanism. It is worth mentioning that our understanding of nutrient stocks and fluxes is much less advanced in comparison with the global C cycle. This has been increasingly acknowledged for the global N cycle as increasing numbers of land surface models have incorporated N cycle dynamics and C–N interactions (Zaehle et al., 2010; Wieder et al., 2019; Davies-Barnard et al., 2020; Smith et al., 2014; Sellar et al., 2019; Goll et al., 2017a; Gerber et al., 2010). Biological N fixation and N-use efficiency have been identified as the key processes that need to be better constrained for land surface models (Davies-Barnard et al., 2020).
Our understanding of P stocks and fluxes are even less advanced than that for the N cycle, as shown in this study and other modeling studies that include P as a limiting nutrient. This is mainly due to (1) various forms of P with different level of availability for plants and microbes, (2) geochemical processes in conjunction with biological processes controlling P availability, and (3) technical challenges in measuring soil P. For example, Hedley fractionation data provide a comprehensive picture of different P forms in soils and have been used for model evaluation and/or initialization in all the land surface models that include a prognostic phosphorus cycle (Wang et al., 2010; Goll et al., 2012; Yang et al., 2014, 2019). However, this extraction method is time-consuming and challenging, and not many routine measurements have been made using this technique. As such, observational estimates of P pools and fluxes are extremely limited. Although recent global Hedley database development (Yang and Post, 2011; Hou et al., 2018) has been helpful in global model development and evaluation, more observational data on P stocks and fluxes are needed to better constrain P-enabled models.
4.5 Effects of accounting for the P cycle dynamics on simulated carbon balance
4.5.1 Spatial variation of nutrient limitation
Our simulated nutrient limitation pattern broadly agrees with the findings from Elser et al. (2007), which supports the generally accepted notion that tropical ecosystems residing on highly weathered soils are P limited (Walker and Syers, 1976; Lebauer and Treseder, 2008). A recent study that predicted spatial patterns of N and P limitation using the ratios of leaf N and P resorption efficiencies also found a shift from P limitation to N limitation with increasing latitude (Du et al., 2020). Lebauer and Treseder (2008) showed that N limitation is widespread, even in tropical regions. This is consistent with our model simulations which show that although P is more limiting in tropical forests, N is also a limiting nutrient. The geographic distribution of nutrient limitation is generally in agreement with that from Goll et al. (2012) and Wang et al. (2010). Goll et al. (2012) suggests that P limits C uptake mainly in low-latitude regions and high latitudes, while N is the limiting nutrient in temperate regions. It is worth mentioning that in Goll et al. (2012) N and P limitation generally have distinct geographic occurrence, while this study suggests NP co-limitation occurs in many parts of the world. Wang et al. (2010) also showed that productivity in tropical forests and savanna is limited by P, while most other biomes are limited by N. This is broadly consistent with our results but with a few key differences. Wang et al. (2010) suggests that P is the limiting nutrient for savannas, whereas our results show savannas are more limited by N. This may have to do with the lack of representation of fire disturbance in Wang et al. (2010). Savannas are subject to regular wildfires, which could have significant effects on nutrient cycle dynamics and nutrient limitation. For example, it has been suggested that while combustion causes significant gaseous losses of N from burned ecosystems, P is largely retained as ash (Herbert et al., 2003). Braakhekke et al. (2017) also showed that there are strong losses of N due to fire. Furthermore, Wang et al. (2010) suggested that tropical forests are limited only by P, not by N, whereas our results indicate that N and P both limit tropical forest productivity, although P limitation is more dominant in most of the lowland tropical forests. This is consistent with a recent meta-analysis of nutrient fertilization experiments in tropical forests (Wright et al., 2018).
4.5.2 The implications for global carbon cycle and climate
Historical C accumulation is a result of many complex and sometimes counteracting processes controlling C fluxes and stocks (Lawrence et al., 2019), including accumulation of carbon on land due to CO2 fertilization, accumulation due to nitrogen deposition, carbon fluxes due to climate variability and climate change, and losses and gains due to land cover conversion and regrowth following historical land cover changes (LULCC fluxes). Over the long term, two of the dominant processes controlling C accumulation in terrestrial ecosystems are C emissions due to LULCC and C uptake due to the CO2 fertilization effect. P cycle dynamics have important impacts on both of these processes, but with opposite sign. Globally, considering P cycle dynamics leads to lower carbon emissions associated with deforestation by about 11 % (161.21 Pg in CN vs. 143.89 in CNP). Conversely, CO2 fertilization at the global scale is reduced by 20 % when P limitation is included during the historical time period (134 Pg C vs. 108 Pg C). In general, the ELMv1-CN simulation shows a CO2 fertilization effect on biomass that is too strong, which leads to a stronger-than-observed carbon sink compared to observational constraints from both Hoffman et al. (2014) and Le Quéré et al. (2016). ELMv1-CN simulation also produces stronger carbon emissions from LULCC due to having higher biomass compared to ELMv1-CNP. The CO2 fertilization effect in the ELMv1-CN simulations is strong enough to overcome the LULCC losses, with the net result being too large of a sink throughout the historical time period for the CN model. Both model configurations lose carbon too slowly due to LULCC in the period from 1850–1940, when compared to the Hoffman et al. (2014) global estimate. Both models also predict continued losses over the period 1940–1965, while the Hoffman et al. (2014) estimate switches from net carbon loss to net carbon accumulation around 1940. These are clearly shown in Fig. S9, which shows the time series of simulated change in land carbon storage in response to changes in CO2, LULCC, N deposition, and climate during 1850–2010. The ELMv1-CN and ELMv1-CNP models are similar to many other CMIP6 models with respect to this bias in the timing of transition from net land carbon source to net land sink as shown in our ILAMB analysis of other land models in CMIP6.
We also note that, over the historical time period, P became more limiting as simulated historical C accumulations became increasingly divergent between CN and CNP simulations. This is mainly caused by stimulated plant productivity under higher atmospheric CO2, which leads to higher plant demand for P that is not balanced by increased supply of newly mineralized P from the soil. This is consistent with other global modeling studies with explicit representation of P cycle dynamics (Goll et al., 2012; Zhang et al., 2014), as well as diagnostic studies that evaluated how CO2 fertilization simulated by CMIP5 models could be constrained by soil P availability using a mass-balance approach (Wieder et al., 2015b; Sun et al., 2017). Taken together, the limiting effect of P availability on C uptake will likely have substantial consequences for projections of future C uptake.
4.6 Limitations and future development
While the ELMv1-CNP simulations presented here show that the model is capable of representing contemporary C, N, and P stocks and fluxes and capturing the observed ecosystem responses to changes in atmospheric CO2, the current configuration does have limitations.
While the model represents disturbances such as fire and the interactions between disturbances and nutrient cycle dynamics, these interactions and how they affect carbon cycle processes have not been well constrained with observational data. There is a growing body of literature investigating the biogeochemical signature of fire. For example, a meta-analysis by Butler et al. (2018) shows that fire led to significantly higher concentration of soil mineral P and lower soil and litter C : P and N : P ratios, therefore decoupling the P cycle from the C and N cycles. We will take advantage of these recent findings to improve model fidelity on this front.
Another area that needs to be improved is the treatment of N fixation and how that is linked to P availability. N fixation in ELMv1-CNP is represented as a function of NPP (Cleveland et al., 1999). While providing a reasonable global estimate of N fixation, the approach ignores the existing mechanistic understanding of nitrogen fixation processes (Wieder et al., 2015a). Furthermore, several lines of evidence suggest that both symbiotic and free-living N fixation rates depend on the availability of other elements, such as P and molybdenum (Reed et al., 2013; Nasto et al., 2014). N fixation could have important implications for the spatial distribution of N limitation vs. P limitation. In the future we plan to have a more mechanistic representation of N fixation in ELM.
In ELMv1-CNP, P limitation is represented by downregulating plant growth when P demand is greater than soil P availability. The mechanisms by which P fundamentally limits ecosystem productivity remain uncertain (Jiang et al., 2019). Some studies proposed that there are linear or log-linear relationships between leaf P concentration and photosynthetic parameters, although the relationship has been shown to be weak (Walker et al., 2014). P fertilization experiments in P-limited ecosystems do not support this proposed relationship. A P fertilization experiment on highly weathered soils in Australia showed that although leaf P concentration increased significantly (+50 %) compared to unfertilized trees, photosynthetic capacity was unaffected (Crous et al., 2015). Another fertilization experiment in Hawaii showed that the increase in aboveground NPP with P fertilization was caused mainly by increases in LAI instead of photosynthesis per unit leaf area (Herbert and Fownes, 1995). Further laboratory and field experiments are needed to help us better understand and represent the role of P in photosynthesis. Investigating the detailed mechanisms through which leaf P concentration affects photosynthesis is an active field of research (Jiang et al., 2019; Norby et al., 2017; Crous et al., 2015), and representing these relationships in land models remains an outstanding challenge.
Uncertainty also remains regarding the ELMv1-CNP representation of sorption dynamics and biochemical mineralization and their responses to changes in atmospheric CO2 and climate (Fleischer et al., 2019). Motivated by our previous modeling studies, several recent field studies have started focusing on improving our mechanistic understanding and providing quantitative relationships for modeling these processes (Cabugao et al., 2017; Brenner et al., 2019). A recent study that upscaled site measurements of potential phosphatase activity to continental Europe using a machine-learning technique provides a potential pathway toward generating benchmark data for biochemical mineralization on a regional to global scale (Sun et al., 2020). ELMv1-CNP is likely underestimating P leaching, in comparison to the estimate of Wang et al. (2018), which could contribute to the underestimate of P uptake and overestimate of land carbon sink. We will further improve the representation of P leaching in ELMv1. There are other mechanisms that could sustain productivity with increasing P limitation but were not considered in ELMv1-CNP, such as flexible stoichiometry and dynamic allocation. These will be investigated further in future versions of E3SM. However, as Fleischer et al. (2019) pointed out, since plant N : P ratios in highly P limited tropical forests are already at the high end of the observed spectrum, the role of stoichiometry plasticity in sustaining tropical productivity could be limited.
While the representation of NSC has helped ELMv1-CNP to capture the interannual variability of atmospheric CO2 and to generate ecosystem responses to elevated CO2 consistent with FACE measurements, the sizes and turnover times of NSC pools are not well constrained. We will synthesize limited measurements on NSC from the literature that include observational and experimental data as well as measurements from isotopic studies to better understand the dynamics of the NSC pool and to evaluate and refine its representation in ELM. We also advocate for more measurements on NSC and how they respond to environmental changes in diverse ecosystems to have a more complete understanding and quantification of NSC.
Finally, although models such as ELMv1-CNP and CLM5 perform similarly when evaluated against present-day metrics as gathered in ILAMB, we expect that the differences among models in their representation of observed processes and in their assumptions about how changes in atmospheric composition and climate will impact ecosystem processes will lead to diverging predictions under future climate scenarios. We will explore those differences and their consequences in future work.
In this study, we provide an evaluation of ELMv1-CNP using the ILAMB benchmarking system, comparison with CO2 manipulation experiments, and comparison with other observational and modeling studies. Benchmarking with ILAMB indicates ELMv1-CNP produces realistic estimates of present-day carbon pools and fluxes. Compared to a simulation with optimal P availability, ELMv1-CNP produces better performance, particularly for the metrics that are most relevant to land–atmosphere exchange. Our results from CO2 manipulation experiments suggest that ELMv1-CNP is able to capture observed responses to elevated CO2, including those for GPP, NPP, vegetation C, and LAI. Further analysis suggests that the introduction of a non-structural carbon pool in ELMv1-CNP is largely responsible for these improvements. Evaluating global C, N, and P pools and fluxes in the context of literature values suggests that ELMv1-CNP provides a reasonable representation of contemporary global-scale C, N, and P cycles.
We highlight the data needs for global land model evaluation, particularly the need for more synthesis datasets on nutrient pools and fluxes, as well as observations from manipulation experiments that provide additional benchmark data for nutrient cycle evaluation. This need is becoming increasingly pressing as more land models are including N and P cycle dynamics and C–N–P interactions. We also identify challenges in constraining P cycle dynamics and point to the need for soil P measurements.
Our simulations suggest, probably not surprisingly, that in general P is the more limiting nutrient in the tropical regions while N is more limiting in the middle to high latitudes. However, our results also suggest widespread N and P co-limitation, even in the tropical regions where P limitation is more dominant. Our results show that C sources and sinks are significantly affected by P limitation, as the historical CO2 fertilization effect was reduced by 20 % and C emission due to LULCC was 11 % lower when P limitation was considered. We conclude that introduction of P cycle dynamics and C–N–P coupling will likely have substantial consequences for projections of future C uptake.
The E3SM model can be accessed at https://www.osti.gov/doecode/biblio/10475, last access: 12 September 2019 (https://doi.org/10.11578/E3SM/dc.20180418.36, E3SM Project, 2018). The input data are available at https://web.lcrc.anl.gov/public/e3sm/inputdata/, last access: 12 September 2019. The model outputs used in this study can be downloaded at https://doi.org/10.6084/m9.figshare.12021348 (Yang, 2020).
The supplement related to this article is available online at: https://doi.org/10.5194/bg-20-2813-2023-supplement.
XY, PET, and DMR designed the research. XY ran the model simulations and worked with PET, DMR, and FHM to analyze and interpret the model outputs. YW provided the GOLUM-CNP datasets. XY prepared the manuscript with contributions from all authors.
The contact author has declared that none of the authors has any competing interests.
Publisher’s note: Copernicus Publications remains neutral with regard to jurisdictional claims in published maps and institutional affiliations.
This research was supported as part of the Energy Exascale Earth System Model (E3SM) project, funded by the Office of Biological and Environmental Research, Office of Science, U.S. Department of Energy. This research was also supported by the Oak Ridge National Laboratory's (ORNL) Terrestrial Ecosystem Science Focus Area (TES SFA). Xiaojuan Yang was also supported as part of the Next Generation Ecosystem Experiments–Tropics project. Forrest Hoffman was supported by the Reducing Uncertainties in Biogeochemical Interactions through Synthesis and Computation Scientific Focus Area (RUBISCO SFA), which is sponsored by the Regional and Global Model Analysis (RGMA) Program in the Climate and Environmental Sciences Division (CESD) of the Office of Biological and Environmental Research (BER) in the U.S. Department of Energy Office of Science. We thank Min Xu for his help in running ILAMB.
This research has been supported by the Office of Biological and Environmental Research (grant no. 3ERKP848).
This paper was edited by David Medvigy and reviewed by two anonymous referees.
Ainsworth, E. A. and Long, S. P.: What have we learned from 15 years of free-air CO2 enrichment (FACE)? A meta-analytic review of the responses of photosynthesis, canopy properties and plant production to rising CO2, New Phytol., 165, 351–372, https://doi.org/10.1111/j.1469-8137.2004.01224.x, 2005.
Aragão, L. E. O. C., Malhi, Y., Metcalfe, D. B., Silva-Espejo, J. E., Jiménez, E., Navarrete, D., Almeida, S., Costa, A. C. L., Salinas, N., Phillips, O. L., Anderson, L. O., Alvarez, E., Baker, T. R., Goncalvez, P. H., Huamán-Ovalle, J., Mamani-Solórzano, M., Meir, P., Monteagudo, A., Patiño, S., Peñuela, M. C., Prieto, A., Quesada, C. A., Rozas-Dávila, A., Rudas, A., Silva Jr., J. A., and Vásquez, R.: Above- and below-ground net primary productivity across ten Amazonian forests on contrasting soils, Biogeosciences, 6, 2759–2778, https://doi.org/10.5194/bg-6-2759-2009, 2009.
Arora, V. K., Boer, G. J., Friedlingstein, P., Eby, M., Jones, C. D., Christian, J. R., Bonan, G., Bopp, L., Brovkin, V., and Cadule, P.: Carbon–concentration and carbon–climate feedbacks in CMIP5 Earth system models, J. Clim., 26, 5289–5314, 2013.
Arora, V. K., Katavouta, A., Williams, R. G., Jones, C. D., Brovkin, V., Friedlingstein, P., Schwinger, J., Bopp, L., Boucher, O., Cadule, P., Chamberlain, M. A., Christian, J. R., Delire, C., Fisher, R. A., Hajima, T., Ilyina, T., Joetzjer, E., Kawamiya, M., Koven, C. D., Krasting, J. P., Law, R. M., Lawrence, D. M., Lenton, A., Lindsay, K., Pongratz, J., Raddatz, T., Séférian, R., Tachiiri, K., Tjiputra, J. F., Wiltshire, A., Wu, T., and Ziehn, T.: Carbon–concentration and carbon–climate feedbacks in CMIP6 models and their comparison to CMIP5 models, Biogeosciences, 17, 4173–4222, https://doi.org/10.5194/bg-17-4173-2020, 2020.
Avitabile, V., Herold, M., Heuvelink, G. B., Lewis, S. L., Phillips, O. L., Asner, G. P., Armston, J., Ashton, P. S., Banin, L., and Bayol, N.: An integrated pan-tropical biomass map using multiple reference datasets, Glob. Change Biol., 22, 1406–1420, 2016.
Baig, S., Medlyn, B. E., Mercado, L. M., and Zaehle, S.: Does the growth response of woody plants to elevated CO2 increase with temperature? A model-oriented meta-analysis, Glob. Change Biol., 21, 4303–4319, 2015.
Barron, A. R., Wurzburger, N., Bellenger, J. P., Wright, S. J., Kraepiel, A. M., and Hedin, L. O.: Molybdenum limitation of asymbiotic nitrogen fixation in tropical forest soils, Nat. Geosci., 2, 42–45, 2008.
Batjes, N. H.: Total carbon and nitrogen in the soils of the world, Eur. J. Soil Sci., 65, 10–21, 2014.
Beer, C., Reichstein, M., Tomelleri, E., Ciais, P., Jung, M., Carvalhais, N., Rödenbeck, C., Arain, M. A., Baldocchi, D., and Bonan, G. B.: Terrestrial gross carbon dioxide uptake: global distribution and covariation with climate, Science, 329, 834–838, 2010.
Bonan, G. B., Lawrence, P. J., Oleson, K. W., Levis, S., Jung, M., Reichstein, M., Lawrence, D. M., and Swenson, S. C.: Improving canopy processes in the Community Land Model version 4 (CLM4) using global flux fields empirically inferred from FLUXNET data, J. Geophys. Res.-Biogeo., 116, https://doi.org/10.1029/2010JG001593, 2011.
Bonan, G. B., Lombardozzi, D. L., Wieder, W. R., Oleson, K. W., Lawrence, D. M., Hoffman, F. M., and Collier, N.: Model structure and climate data uncertainty in historical simulations of the terrestrial carbon cycle (1850–2014), Global Biogeochem. Cy., 33, 1310–1326, 2019.
Braakhekke, M. C., Rebel, K. T., Dekker, S. C., Smith, B., Beusen, A. H. W., and Wassen, M. J.: Nitrogen leaching from natural ecosystems under global change: a modelling study, Earth Syst. Dynam., 8, 1121–1139, https://doi.org/10.5194/esd-8-1121-2017, 2017.
Brenner, J., Porter, W., Phillips, J. R., Childs, J., Yang, X., and Mayes, M. A.: Phosphorus sorption on tropical soils with relevance to Earth system model needs, Soil Res., 57, 17–27, 2019.
Burrows, S., Maltrud, M., Yang, X., Zhu, Q., Jeffery, N., Shi, X., Ricciuto, D., Wang, S., Bisht, G., and Tang, J.: The DOE E3SM v1. 1 Biogeochemistry Configuration: Description and Simulated Ecosystem-Climate Responses to Historical Changes in Forcing, J. Adv. Model. Earth Syst., 12, e2019MS001766, https://doi.org/10.1029/2019MS001766, 2020.
Butler, O. M., Elser, J. J., Lewis, T., Mackey, B., and Chen, C.: The phosphorus-rich signature of fire in the soil–plant system: a global meta-analysis, Ecol. Lett., 21, 335–344, 2018.
Cabugao, K. G., Timm, C. M., Carrell, A. A., Childs, J., Lu, T.-Y. S., Pelletier, D. A., Weston, D. J., and Norby, R. J.: Root and rhizosphere bacterial phosphatase activity varies with tree species and soil phosphorus availability in Puerto Rico tropical forest, Front. Plant Sci., 8, 1834, https://doi.org/10.3389/fpls.2017.01834, 2017.
Campbell, J., Berry, J., Seibt, U., Smith, S. J., Montzka, S., Launois, T., Belviso, S., Bopp, L., and Laine, M.: Large historical growth in global terrestrial gross primary production, Nature, 544, 84–87, 2017.
Cleveland, C., Townsend, A., Schimel, D., Fisher, H., Howarth, R., Hedin, L., Perakis, S., Latty, E., Von Fischer, J., and Elseroad, A.: Global patterns of terrestrial biological nitrogen (N2) fixation in natural ecosystems, Global Biogeochem. Cy., 13, 623–645, 1999.
Collier, N., Hoffman, F. M., Lawrence, D. M., Keppel-Aleks, G., Koven, C. D., Riley, W. J., Mu, M., and Randerson, J. T.: The International Land Model Benchmarking (ILAMB) system: design, theory, and implementation, J. Adv. Model. Earth Syst., 10, 2731–2754, 2018.
Crews, T. E., Farrington, H., and Vitousek, P. M.: Changes in asymbiotic, heterotrophic nitrogen fixation on leaf litter of Metrosideros polymorpha with long-term ecosystem development in Hawaii, Ecosystems, 3, 386–395, 2000.
Crous, K. Y., Osvaldsson, A., and Ellsworth, D. S.: Is phosphorus limiting in a mature Eucalyptus woodland? Phosphorus fertilisation stimulates stem growth, Plant Soil, 391, 293–305, 2015.
Davies-Barnard, T. and Friedlingstein, P.: The Global Distribution of Biological Nitrogen Fixation in Terrestrial Natural Ecosystems, Global Biogeochem. Cy., 34, e2019GB006387, https://doi.org/10.1029/2019gb006387, 2020.
Davies-Barnard, T., Meyerholt, J., Zaehle, S., Friedlingstein, P., Brovkin, V., Fan, Y., Fisher, R. A., Jones, C. D., Lee, H., Peano, D., Smith, B., Wårlind, D., and Wiltshire, A. J.: Nitrogen cycling in CMIP6 land surface models: progress and limitations, Biogeosciences, 17, 5129–5148, https://doi.org/10.5194/bg-17-5129-2020, 2020.
DeFries, R., Field, C., Fung, I., Collatz, G., and Bounoua, L.: Combining satellite data and biogeochemical models to estimate global effects of human-induced land cover change on carbon emissions and primary productivity, Global Biogeochem. Cy., 13, 803–815, 1999.
Du, E., Terrer, C., Pellegrini, A. F., Ahlström, A., van Lissa, C. J., Zhao, X., Xia, N., Wu, X., and Jackson, R. B.: Global patterns of terrestrial nitrogen and phosphorus limitation, Nat. Geosci., 13, 221–226, 2020.
Duarte, H. F., Raczka, B. M., Ricciuto, D. M., Lin, J. C., Koven, C. D., Thornton, P. E., Bowling, D. R., Lai, C.-T., Bible, K. J., and Ehleringer, J. R.: Evaluating the Community Land Model (CLM4.5) at a coniferous forest site in northwestern United States using flux and carbon-isotope measurements, Biogeosciences, 14, 4315–4340, https://doi.org/10.5194/bg-14-4315-2017, 2017.
E3SM Project (DOE): Energy Exascale Earth System Model v1.0, Computer software [code], https://doi.org/10.11578/E3SM/dc.20180418.36, 2018.
Edwards, E., McCaffery, S., and Evans, J.: Phosphorus availability and elevated CO2 affect biological nitrogen fixation and nutrient fluxes in a clover dominated sward, New Phytol., 169, 157–167, 2006.
Ehlers, I., Augusti, A., Betson, T. R., Nilsson, M. B., Marshall, J. D., and Schleucher, J.: Detecting long-term metabolic shifts using isotopomers: CO2-driven suppression of photorespiration in C3 plants over the 20th century, P. Natl. Acad. Sci. USA, 112, 15585–15590, 2015.
Ellsworth, D. S., Anderson, I. C., Crous, K. Y., Cooke, J., Drake, J. E., Gherlenda, A. N., Gimeno, T. E., Macdonald, C. A., Medlyn, B. E., and Powell, J. R.: Elevated CO2 does not increase eucalypt forest productivity on a low-phosphorus soil, Nat. Clim. Change, 7, 279–282, 2017.
Elser, J. J., Bracken, M. E. S., Cleland, E. E., Gruner, D. S., Harpole, W. S., Hillebrand, H., Ngai, J. T., Seabloom, E. W., Shurin, J. B., and Smith, J. E.: Global analysis of nitrogen and phosphorus limitation of primary producers in freshwater, marine and terrestrial ecosystems, Ecol. Lett., 10, 1135–1142, https://doi.org/10.1111/j.1461-0248.2007.01113.x, 2007.
Erb, K.-H., Kastner, T., Plutzar, C., Bais, A. L. S., Carvalhais, N., Fetzel, T., Gingrich, S., Haberl, H., Lauk, C., and Niedertscheider, M.: Unexpectedly large impact of forest management and grazing on global vegetation biomass, Nature, 553, 73–76, 2018.
Finzi, A. C., Norby, R. J., Calfapietra, C., Gallet-Budynek, A., Gielen, B., Holmes, W. E., Hoosbeek, M. R., Iversen, C. M., Jackson, R. B., and Kubiske, M. E.: Increases in nitrogen uptake rather than nitrogen-use efficiency support higher rates of temperate forest productivity under elevated CO2, P. Natl. Acad. Sci. USA, 104, 14014–14019, 2007.
Fleischer, K., Rammig, A., De Kauwe, M. G., Walker, A. P., Domingues, T. F., Fuchslueger, L., Garcia, S., Goll, D. S., Grandis, A., and Jiang, M.: Amazon forest response to CO2 fertilization dependent on plant phosphorus acquisition, Nat. Geosci., 12, 736–741, 2019.
Friedlingstein, P., Jones, M. W., O'Sullivan, M., Andrew, R. M., Hauck, J., Peters, G. P., Peters, W., Pongratz, J., Sitch, S., Le Quéré, C., Bakker, D. C. E., Canadell, J. G., Ciais, P., Jackson, R. B., Anthoni, P., Barbero, L., Bastos, A., Bastrikov, V., Becker, M., Bopp, L., Buitenhuis, E., Chandra, N., Chevallier, F., Chini, L. P., Currie, K. I., Feely, R. A., Gehlen, M., Gilfillan, D., Gkritzalis, T., Goll, D. S., Gruber, N., Gutekunst, S., Harris, I., Haverd, V., Houghton, R. A., Hurtt, G., Ilyina, T., Jain, A. K., Joetzjer, E., Kaplan, J. O., Kato, E., Klein Goldewijk, K., Korsbakken, J. I., Landschützer, P., Lauvset, S. K., Lefèvre, N., Lenton, A., Lienert, S., Lombardozzi, D., Marland, G., McGuire, P. C., Melton, J. R., Metzl, N., Munro, D. R., Nabel, J. E. M. S., Nakaoka, S.-I., Neill, C., Omar, A. M., Ono, T., Peregon, A., Pierrot, D., Poulter, B., Rehder, G., Resplandy, L., Robertson, E., Rödenbeck, C., Séférian, R., Schwinger, J., Smith, N., Tans, P. P., Tian, H., Tilbrook, B., Tubiello, F. N., van der Werf, G. R., Wiltshire, A. J., and Zaehle, S.: Global Carbon Budget 2019, Earth Syst. Sci. Data, 11, 1783–1838, https://doi.org/10.5194/essd-11-1783-2019, 2019.
Gerber, S., Hedin, L. O., Oppenheimer, M., Pacala, S. W., and Shevliakova, E.: Nitrogen cycling and feedbacks in a global dynamic land model, Global Biogeochem. Cy., 24, GB1001, https://doi.org/10.1029/2008gb003336, 2010.
Ghimire, B., Riley, W. J., Koven, C. D., Mu, M., and Randerson, J. T.: Representing leaf and root physiological traits in CLM improves global carbon and nitrogen cycling predictions, J. Adv. Model. Earth Syst., 8, 598–613, 2016.
Goll, D. S., Brovkin, V., Parida, B. R., Reick, C. H., Kattge, J., Reich, P. B., van Bodegom, P. M., and Niinemets, Ü.: Nutrient limitation reduces land carbon uptake in simulations with a model of combined carbon, nitrogen and phosphorus cycling, Biogeosciences, 9, 3547–3569, https://doi.org/10.5194/bg-9-3547-2012, 2012.
Goll, D. S., Winkler, A. J., Raddatz, T., Dong, N., Prentice, I. C., Ciais, P., and Brovkin, V.: Carbon–nitrogen interactions in idealized simulations with JSBACH (version 3.10), Geosci. Model Dev., 10, 2009–2030, https://doi.org/10.5194/gmd-10-2009-2017, 2017a.
Goll, D. S., Vuichard, N., Maignan, F., Jornet-Puig, A., Sardans, J., Violette, A., Peng, S., Sun, Y., Kvakic, M., Guimberteau, M., Guenet, B., Zaehle, S., Penuelas, J., Janssens, I., and Ciais, P.: A representation of the phosphorus cycle for ORCHIDEE (revision 4520), Geosci. Model Dev., 10, 3745–3770, https://doi.org/10.5194/gmd-10-3745-2017, 2017b.
Haverd, V., Smith, B., Canadell, J. G., Cuntz, M., Mikaloff-Fletcher, S., Farquhar, G., Woodgate, W., Briggs, P. R., and Trudinger, C. M.: Higher than expected CO2 fertilization inferred from leaf to global observations, Glob. Change Biol., 26, 2390–2402, 2020.
Herbert, D. A. and Fownes, J. H.: Phosphorus limitation of forest leaf area and net primary production on a highly weathered soil, Biogeochemistry, 29, 223–235, 1995.
Herbert, D. A., Williams, M., and Rastetter, E. B.: A model analysis of N and P limitation on carbon accumulation in Amazonian secondary forest after alternate land-use abandonment, Biogeochemistry, 65, 121–150, 2003.
Hoffman, F., Koven, C., Keppel-Aleks, G., Lawrence, D., Riley, W., Randerson, J., Ahlström, A., Abramowitz, G., Baldocchi, D., and Best, M.: International land model benchmarking (ILAMB) 2016 Workshop Report, US Department of Energy, Office of Science, 10, 1330803, https://doi.org/10.2172/1330803, 2017.
Hoffman, F. M., Randerson, J. T., Arora, V. K., Bao, Q., Cadule, P., Ji, D., Jones, C. D., Kawamiya, M., Khatiwala, S., and Lindsay, K.: Causes and implications of persistent atmospheric carbon dioxide biases in Earth System Models, J. Geophys. Res.-Biogeo., 119, 141–162, 2014.
Hou, E., Tan, X., Heenan, M., and Wen, D.: A global dataset of plant available and unavailable phosphorus in natural soils derived by Hedley method, Sci. Data, 5, 180166, https://doi.org/10.1038/sdata.2018.166, 2018.
Hou, E., Luo, Y., Kuang, Y., Chen, C., Lu, X., Jiang, L., Luo, X., and Wen, D.: Global meta-analysis shows pervasive phosphorus limitation of aboveground plant production in natural terrestrial ecosystems, Nat. Commun., 11, 1–9, 2020.
Houghton, R. A.: The contemporary carbon cycle, Treat. Geochem., 8, 473–513, 2003.
Houlton, B. Z., Wang, Y.-P., Vitousek, P. M., and Field, C. B.: A unifying framework for dinitrogen fixation in the terrestrial biosphere, Nature, 454, 327–330, https://doi.org/10.1038/nature07028, 2008.
Huete, A., Didan, K., Miura, T., Rodriguez, E. P., Gao, X., and Ferreira, L. G.: Overview of the radiometric and biophysical performance of the MODIS vegetation indices, Remote Sens. Environ., 83, 195–213, 2002.
Hungate, B. A., Dukes, J. S., Shaw, M. R., Luo, Y., and Field, C. B.: Nitrogen and climate change, Science, 302, 1512–1513, 2003.
Hungate, B. A., Stiling, P. D., Dijkstra, P., Johnson, D. W., Ketterer, M. E., Hymus, G. J., Hinkle, C. R., and Drake, B. G.: CO2 elicits long-term decline in nitrogen fixation, Science, 304, 1291, https://doi.org/10.1126/science.1095549, 2004.
Jahnke, R. A.: The phosphorus cycle, in: Global Biogeochemical Cycles, edited by: Butcher, S. S., Charlson, R. J., Orians, G. H., and Wolfe, G. V., Academic Press, London, 301–315, 1992.
Jiang, M., Caldararu, S., Zaehle, S., Ellsworth, D. S., and Medlyn, B. E.: Towards a more physiological representation of vegetation phosphorus processes in land surface models, New Phytol., 222, 1223–1229, 2019.
Jobbágy, E. G. and Jackson, R. B.: The vertical distribution of soil organic carbon and its relation to climate and vegetation, Ecol. Appl., 10, 423–436, 2000.
Joiner, J., Yoshida, Y., Zhang, Y., Duveiller, G., Jung, M., Lyapustin, A., Wang, Y., and Tucker, C. J.: Estimation of terrestrial global gross primary production (GPP) with satellite data-driven models and eddy covariance flux data, Remote Sens., 10, 1346, https://doi.org/10.3390/rs10091346, 2018.
Jones, C. D., Arora, V., Friedlingstein, P., Bopp, L., Brovkin, V., Dunne, J., Graven, H., Hoffman, F., Ilyina, T., John, J. G., Jung, M., Kawamiya, M., Koven, C., Pongratz, J., Raddatz, T., Randerson, J. T., and Zaehle, S.: C4MIP – The Coupled Climate–Carbon Cycle Model Intercomparison Project: experimental protocol for CMIP6, Geosci. Model Dev., 9, 2853–2880, https://doi.org/10.5194/gmd-9-2853-2016, 2016.
Jung, C.-G., Shin, H.-J., Park, M.-J., Joh, H.-K., and Kim, S.-J.: Evaluation of MODIS Gross Primary Production (GPP) by Comparing with GPP from CO2 Flux Data Measured in a Mixed Forest Area, J. Korean Soc. Agr. Eng., 53, 1–8, 2011.
Kobayashi, H. and Dye, D. G.: Atmospheric conditions for monitoring the long-term vegetation dynamics in the Amazon using normalized difference vegetation index, Remote Sens. Environ., 97, 519–525, 2005.
Köchy, M., Hiederer, R., and Freibauer, A.: Global distribution of soil organic carbon – Part 1: Masses and frequency distributions of SOC stocks for the tropics, permafrost regions, wetlands, and the world, SOIL, 1, 351–365, https://doi.org/10.5194/soil-1-351-2015, 2015.
Körner, C., Asshoff, R., Bignucolo, O., Hättenschwiler, S., Keel, S. G., Peláez-Riedl, S., Pepin, S., Siegwolf, R. T., and Zotz, G.: Carbon flux and growth in mature deciduous forest trees exposed to elevated CO2, Science, 309, 1360–1362, 2005.
Koven, C. D., Riley, W. J., Subin, Z. M., Tang, J. Y., Torn, M. S., Collins, W. D., Bonan, G. B., Lawrence, D. M., and Swenson, S. C.: The effect of vertically resolved soil biogeochemistry and alternate soil C and N models on C dynamics of CLM4, Biogeosciences, 10, 7109–7131, https://doi.org/10.5194/bg-10-7109-2013, 2013.
Lawrence, D. M., Fisher, R. A., Koven, C. D., Oleson, K. W., Swenson, S. C., Bonan, G., Collier, N., Ghimire, B., van Kampenhout, L., and Kennedy, D.: The Community Land Model version 5: Description of new features, benchmarking, and impact of forcing uncertainty, J. Adv. Model. Earth Syst., https://doi.org/10.1029/2018MS001583, 2019.
Le Quéré, C., Andrew, R. M., Canadell, J. G., Sitch, S., Korsbakken, J. I., Peters, G. P., Manning, A. C., Boden, T. A., Tans, P. P., Houghton, R. A., Keeling, R. F., Alin, S., Andrews, O. D., Anthoni, P., Barbero, L., Bopp, L., Chevallier, F., Chini, L. P., Ciais, P., Currie, K., Delire, C., Doney, S. C., Friedlingstein, P., Gkritzalis, T., Harris, I., Hauck, J., Haverd, V., Hoppema, M., Klein Goldewijk, K., Jain, A. K., Kato, E., Körtzinger, A., Landschützer, P., Lefèvre, N., Lenton, A., Lienert, S., Lombardozzi, D., Melton, J. R., Metzl, N., Millero, F., Monteiro, P. M. S., Munro, D. R., Nabel, J. E. M. S., Nakaoka, S., O'Brien, K., Olsen, A., Omar, A. M., Ono, T., Pierrot, D., Poulter, B., Rödenbeck, C., Salisbury, J., Schuster, U., Schwinger, J., Séférian, R., Skjelvan, I., Stocker, B. D., Sutton, A. J., Takahashi, T., Tian, H., Tilbrook, B., van der Laan-Luijkx, I. T., van der Werf, G. R., Viovy, N., Walker, A. P., Wiltshire, A. J., and Zaehle, S.: Global Carbon Budget 2016, Earth Syst. Sci. Data, 8, 605–649, https://doi.org/10.5194/essd-8-605-2016, 2016.
Le Quéré, C., Andrew, R. M., Friedlingstein, P., Sitch, S., Hauck, J., Pongratz, J., Pickers, P. A., Korsbakken, J. I., Peters, G. P., Canadell, J. G., Arneth, A., Arora, V. K., Barbero, L., Bastos, A., Bopp, L., Chevallier, F., Chini, L. P., Ciais, P., Doney, S. C., Gkritzalis, T., Goll, D. S., Harris, I., Haverd, V., Hoffman, F. M., Hoppema, M., Houghton, R. A., Hurtt, G., Ilyina, T., Jain, A. K., Johannessen, T., Jones, C. D., Kato, E., Keeling, R. F., Goldewijk, K. K., Landschützer, P., Lefèvre, N., Lienert, S., Liu, Z., Lombardozzi, D., Metzl, N., Munro, D. R., Nabel, J. E. M. S., Nakaoka, S., Neill, C., Olsen, A., Ono, T., Patra, P., Peregon, A., Peters, W., Peylin, P., Pfeil, B., Pierrot, D., Poulter, B., Rehder, G., Resplandy, L., Robertson, E., Rocher, M., Rödenbeck, C., Schuster, U., Schwinger, J., Séférian, R., Skjelvan, I., Steinhoff, T., Sutton, A., Tans, P. P., Tian, H., Tilbrook, B., Tubiello, F. N., van der Laan-Luijkx, I. T., van der Werf, G. R., Viovy, N., Walker, A. P., Wiltshire, A. J., Wright, R., Zaehle, S., and Zheng, B.: Global Carbon Budget 2018, Earth Syst. Sci. Data, 10, 2141–2194, https://doi.org/10.5194/essd-10-2141-2018, 2018.
LeBauer, D. S. and Treseder, K. K.: Nitrogen limitation of net primary productivity in terrestrial ecosystems is globally distributed, Ecology, 89, 371–379, 2008.
Lin, B.-L., Sakoda, A., Shibasaki, R., Goto, N., and Suzuki, M.: Modelling a global biogeochemical nitrogen cycle in terrestrial ecosystems, Ecol. Model., 135, 89–110, 2000.
Luo, Y. Q., Randerson, J. T., Abramowitz, G., Bacour, C., Blyth, E., Carvalhais, N., Ciais, P., Dalmonech, D., Fisher, J. B., Fisher, R., Friedlingstein, P., Hibbard, K., Hoffman, F., Huntzinger, D., Jones, C. D., Koven, C., Lawrence, D., Li, D. J., Mahecha, M., Niu, S. L., Norby, R., Piao, S. L., Qi, X., Peylin, P., Prentice, I. C., Riley, W., Reichstein, M., Schwalm, C., Wang, Y. P., Xia, J. Y., Zaehle, S., and Zhou, X. H.: A framework for benchmarking land models, Biogeosciences, 9, 3857–3874, https://doi.org/10.5194/bg-9-3857-2012, 2012.
Mao, J., Ricciuto, D. M., Thornton, P. E., Warren, J. M., King, A. W., Shi, X., Iversen, C. M., and Norby, R. J.: Evaluating the Community Land Model in a pine stand with shading manipulations and 13CO2 labeling, Biogeosciences, 13, 641–657, https://doi.org/10.5194/bg-13-641-2016, 2016.
Marklein, A. R. and Houlton, B. Z.: Nitrogen inputs accelerate phosphorus cycling rates across a wide variety of terrestrial ecosystems, New Phytol., 193, 696–704, 2012.
Matthews, E.: Global litter production, pools, and turnover times: Estimates from measurement data and regression models, J. Geophys. Res.-Atmos., 102, 18771–18800, 1997.
McGill, W. and Cole, C.: Comparative aspects of cycling of organic C, N, S and P through soil organic matter, Geoderma, 26, 267–286, 1981.
Melillo, J., Steudler, P., Aber, J., Newkirk, K., Lux, H., Bowles, F., Catricala, C., Magill, A., Ahrens, T., and Morrisseau, S.: Soil warming and carbon-cycle feedbacks to the climate system, Science, 298, 2173–2176, 2002.
Metcalfe, D. B., Ricciuto, D., Palmroth, S., Campbell, C., Hurry, V., Mao, J., Keel, S. G., Linder, S., Shi, X., and Näsholm, T.: Informing climate models with rapid chamber measurements of forest carbon uptake, Glob. Change Biol., 23, 2130–2139, 2017.
Mitchard, E. T., Feldpausch, T. R., Brienen, R. J., Lopez-Gonzalez, G., Monteagudo, A., Baker, T. R., Lewis, S. L., Lloyd, J., Quesada, C. A., and Gloor, M.: Markedly divergent estimates of A mazon forest carbon density from ground plots and satellites, Global Ecol. Biogeogr., 23, 935–946, 2014.
Nakhavali, M. A., Mercado, L. M., Hartley, I. P., Sitch, S., Cunha, F. V., di Ponzio, R., Lugli, L. F., Quesada, C. A., Andersen, K. M., Chadburn, S. E., Wiltshire, A. J., Clark, D. B., Ribeiro, G., Siebert, L., Moraes, A. C. M., Schmeisk Rosa, J., Assis, R., and Camargo, J. L.: Representation of the phosphorus cycle in the Joint UK Land Environment Simulator (vn5.5_JULES-CNP), Geosci. Model Dev., 15, 5241–5269, https://doi.org/10.5194/gmd-15-5241-2022, 2022.
Nasto, M. K., Alvarez-Clare, S., Lekberg, Y., Sullivan, B. W., Townsend, A. R., and Cleveland, C. C.: Interactions among nitrogen fixation and soil phosphorus acquisition strategies in lowland tropical rain forests, Ecol. Lett., 17, 1282–1289, 2014.
Norby, R. J., Gu, L., Haworth, I. C., Jensen, A. M., Turner, B. L., Walker, A. P., Warren, J. M., Weston, D. J., Xu, C., and Winter, K.: Informing models through empirical relationships between foliar phosphorus, nitrogen and photosynthesis across diverse woody species in tropical forests of Panama, New Phytol., 215, 1425–1437, 2017.
Olander, L. P. and Vitousek, P. M.: Regulation of soil phosphatase and chitinase activityby N and P availability, Biogeochemistry, 49, 175–191, 2000.
Oleson, K., Lawrence, D., Bonan, G., Drewniak, B., Huang, M., Koven, C., Levis, S., Li, F., Riley, W., and Subin, Z.: Technical Description of version 4.5 of the Community Land Model (CLM)(NCAR Technical Note No. NCAR/TN-503+ STR). Citeseer, National Center for Atmospheric Research, P.O. Box, 3000, https://doi.org/10.5065/D6RR1W7M, 2013.
Pan, Y., Birdsey, R. A., Fang, J., Houghton, R., Kauppi, P. E., Kurz, W. A., Phillips, O. L., Shvidenko, A., Lewis, S. L., and Canadell, J. G.: A large and persistent carbon sink in the world's forests, Science, 333, 988–993, 2011.
Post, W. M., Pastor, J., Zinke, P. J., and Stangenberger, A. G.: Global patterns of soil nitrogen storage, Nature, 317, 613–616, 1985.
Quesada, C. A., Phillips, O. L., Schwarz, M., Czimczik, C. I., Baker, T. R., Patiño, S., Fyllas, N. M., Hodnett, M. G., Herrera, R., Almeida, S., Alvarez Dávila, E., Arneth, A., Arroyo, L., Chao, K. J., Dezzeo, N., Erwin, T., di Fiore, A., Higuchi, N., Honorio Coronado, E., Jimenez, E. M., Killeen, T., Lezama, A. T., Lloyd, G., López-González, G., Luizão, F. J., Malhi, Y., Monteagudo, A., Neill, D. A., Núñez Vargas, P., Paiva, R., Peacock, J., Peñuela, M. C., Peña Cruz, A., Pitman, N., Priante Filho, N., Prieto, A., Ramírez, H., Rudas, A., Salomão, R., Santos, A. J. B., Schmerler, J., Silva, N., Silveira, M., Vásquez, R., Vieira, I., Terborgh, J., and Lloyd, J.: Basin-wide variations in Amazon forest structure and function are mediated by both soils and climate, Biogeosciences, 9, 2203–2246, https://doi.org/10.5194/bg-9-2203-2012, 2012.
Raczka, B., Duarte, H. F., Koven, C. D., Ricciuto, D., Thornton, P. E., Lin, J. C., and Bowling, D. R.: An observational constraint on stomatal function in forests: evaluating coupled carbon and water vapor exchange with carbon isotopes in the Community Land Model (CLM4.5), Biogeosciences, 13, 5183–5204, https://doi.org/10.5194/bg-13-5183-2016, 2016.
Reed, S. C., Cleveland, C. C., and Townsend, A. R.: Relationships among phosphorus, molybdenum and free-living nitrogen fixation in tropical rain forests: results from observational and experimental analyses, Biogeochemistry, 1–13, https://doi.org/10.1007/s10533-013-9835-3, 2013.
Reed, S. C., Yang, X., and Thornton, P. E.: Incorporating phosphorus cycling into global modeling efforts: a worthwhile, tractable endeavor, New Phytol., 208, 324–329, 2015.
Santoro, M., Beaudoin, A., Beer, C., Cartus, O., Fransson, J. E., Hall, R. J., Pathe, C., Schmullius, C., Schepaschenko, D., and Shvidenko, A.: Forest growing stock volume of the northern hemisphere: Spatially explicit estimates for 2010 derived from Envisat ASAR, Remote Sens. Environ., 168, 316–334, 2015.
Sellar, A. A., Jones, C. G., Mulcahy, J., Tang, Y., Yool, A., Wiltshire, A., O'connor, F. M., Stringer, M., Hill, R., and Palmieri, J.: UKESM1: Description and evaluation of the UK Earth System Model, J. Adv. Model. Earth Syst., https://doi.org/10.1029/2019MS001739, 2019.
Shabanov, N. V., Huang, D., Yang, W., Tan, B., Knyazikhin, Y., Myneni, R. B., Ahl, D. E., Gower, S. T., Huete, A. R., and Aragão, L. E. O.: Analysis and optimization of the MODIS leaf area index algorithm retrievals over broadleaf forests, IEEE T. Geosci. Remote Sens., 43, 1855–1865, 2005.
Smil, V.: P Phosphorus in the Environment: Natural Flows and Human Interferences, Ann. Rev. Energ. Environ., 25, 53–88, 2000.
Smith, B., Wårlind, D., Arneth, A., Hickler, T., Leadley, P., Siltberg, J., and Zaehle, S.: Implications of incorporating N cycling and N limitations on primary production in an individual-based dynamic vegetation model, Biogeosciences, 11, 2027–2054, https://doi.org/10.5194/bg-11-2027-2014, 2014.
Sun, Y., Peng, S., Goll, D. S., Ciais, P., Guenet, B., Guimberteau, M., Hinsinger, P., Janssens, I. A., Peñuelas, J., and Piao, S.: Diagnosing phosphorus limitations in natural terrestrial ecosystems in carbon cycle models, Earth's Future, 5, 730–749, 2017.
Sun, Y., Goll, D. S., Ciais, P., Peng, S., Margalef, O., Asensio, D., Sardans, J., and Peñuelas, J.: Spatial pattern and environmental drivers of acid phosphatase activity in Europe, Front. Big Data, 2, 2019, https://doi.org/10.3389/fdata.2019.00051, 2020.
Sun, Y., Goll, D. S., Chang, J., Ciais, P., Guenet, B., Helfenstein, J., Huang, Y., Lauerwald, R., Maignan, F., Naipal, V., Wang, Y., Yang, H., and Zhang, H.: Global evaluation of the nutrient-enabled version of the land surface model ORCHIDEE-CNP v1.2 (r5986), Geosci. Model Dev., 14, 1987–2010, https://doi.org/10.5194/gmd-14-1987-2021, 2021.
Terrer, C., Jackson, R. B., Prentice, I. C., Keenan, T. F., Kaiser, C., Vicca, S., Fisher, J. B., Reich, P. B., Stocker, B. D., and Hungate, B. A.: Nitrogen and phosphorus constrain the CO2 fertilization of global plant biomass, Nat. Clim. Change, 9, 684–689, 2019.
Thornton, P. E., Doney, S. C., Lindsay, K., Moore, J. K., Mahowald, N., Randerson, J. T., Fung, I., Lamarque, J.-F., Feddema, J. J., and Lee, Y.-H.: Carbon-nitrogen interactions regulate climate-carbon cycle feedbacks: results from an atmosphere-ocean general circulation model, Biogeosciences, 6, 2099–2120, https://doi.org/10.5194/bg-6-2099-2009, 2009.
Thornton, P. E., Lamarque, J.-F., Rosenbloom, N. A., and Mahowald, N. M.: Influence of carbon-nitrogen cycle coupling on land model response to CO2 fertilization and climate variability, Global Biogeochem. Cy., 21, GB4018, https://doi.org/10.1029/2006gb002868, 2007.
Thum, T., Caldararu, S., Engel, J., Kern, M., Pallandt, M., Schnur, R., Yu, L., and Zaehle, S.: A new model of the coupled carbon, nitrogen, and phosphorus cycles in the terrestrial biosphere (QUINCY v1.0; revision 1996), Geosci. Model Dev., 12, 4781–4802, https://doi.org/10.5194/gmd-12-4781-2019, 2019.
Todd-Brown, K. E. O., Randerson, J. T., Post, W. M., Hoffman, F. M., Tarnocai, C., Schuur, E. A. G., and Allison, S. D.: Causes of variation in soil carbon simulations from CMIP5 Earth system models and comparison with observations, Biogeosciences, 10, 1717–1736, https://doi.org/10.5194/bg-10-1717-2013, 2013.
Treseder, K. K. and Vitousek, P. M.: Effects of soil nutrient availability on investment in acquisition of N and P in Hawaiian rain forests, Ecology, 82, 946–954, 2001.
van den Hurk, B., Kim, H., Krinner, G., Seneviratne, S. I., Derksen, C., Oki, T., Douville, H., Colin, J., Ducharne, A., Cheruy, F., Viovy, N., Puma, M. J., Wada, Y., Li, W., Jia, B., Alessandri, A., Lawrence, D. M., Weedon, G. P., Ellis, R., Hagemann, S., Mao, J., Flanner, M. G., Zampieri, M., Materia, S., Law, R. M., and Sheffield, J.: LS3MIP (v1.0) contribution to CMIP6: the Land Surface, Snow and Soil moisture Model Intercomparison Project – aims, setup and expected outcome, Geosci. Model Dev., 9, 2809–2832, https://doi.org/10.5194/gmd-9-2809-2016, 2016.
Vicca, S., Luyssaert, S., Penuelas, J., Campioli, M., Chapin III, F., Ciais, P., Heinemeyer, A., Högberg, P., Kutsch, W., and Law, B. E.: Fertile forests produce biomass more efficiently, Ecol. Lett., 15, 520–526, 2012.
Vitousek, P. M., Porder, S., Houlton, B. Z., and Chadwick, O. A.: Terrestrial phosphorus limitation: mechanisms, implications, and nitrogen-phosphorus interactions, Ecol. Appl., 20, 5–15, 2010.
Vitousek, P. M., Menge, D. N., Reed, S. C., and Cleveland, C. C.: Biological nitrogen fixation: rates, patterns and ecological controls in terrestrial ecosystems, Philos. T. R. Soc. B, 368, 20130119, https://doi.org/10.1098/rstb.2013.0119, 2013.
Walker, A. P., Beckerman, A. P., Gu, L., Kattge, J., Cernusak, L. A., Domingues, T. F., Scales, J. C., Wohlfahrt, G., Wullschleger, S. D., and Woodward, F. I.: The relationship of leaf photosynthetic traits–Vcmax and Jmax–to leaf nitrogen, leaf phosphorus, and specific leaf area: a meta-analysis and modeling study, Ecol. Evol., 4, 3218–3235, 2014.
Walker, T. and Syers, J.: The fate of phosphorus during pedogenesis, Geoderma, 15, 1–19, 1976.
Wang, Y., Ciais, P., Goll, D., Huang, Y., Luo, Y., Wang, Y.-P., Bloom, A. A., Broquet, G., Hartmann, J., Peng, S., Penuelas, J., Piao, S., Sardans, J., Stocker, B. D., Wang, R., Zaehle, S., and Zechmeister-Boltenstern, S.: GOLUM-CNP v1.0: a data-driven modeling of carbon, nitrogen and phosphorus cycles in major terrestrial biomes, Geosci. Model Dev., 11, 3903–3928, https://doi.org/10.5194/gmd-11-3903-2018, 2018.
Wang, Y. P., Houlton, B. Z., and Field, C. B.: A model of biogeochemical cycles of carbon, nitrogen, and phosphorus including symbiotic nitrogen fixation and phosphatase production, Global Biogeochem. Cy., 21, GB1018, https://doi.org/10.1029/2006gb002797, 2007.
Wang, Y.-P., Law, R. M., and Pak, B.: A global model of carbon, nitrogen and phosphorus cycles for the terrestrial biosphere, Biogeosciences, 7, 2261–2282, https://doi.org/10.5194/bg-7-2261-2010, 2010.
Welp, L. R., Keeling, R. F., Meijer, H. A., Bollenbacher, A. F., Piper, S. C., Yoshimura, K., Francey, R. J., Allison, C. E., and Wahlen, M.: Interannual variability in the oxygen isotopes of atmospheric CO2 driven by El Niño, Nature, 477, 579–582, 2011.
Wieder, W. R., Cleveland, C. C., Lawrence, D. M., and Bonan, G. B.: Effects of model structural uncertainty on carbon cycle projections: biological nitrogen fixation as a case study, Environ. Res. Lett., 10, 044016, https://doi.org/10.1088/1748-9326/10/4/044016, 2015a.
Wieder, W. R., Cleveland, C. C., Smith, W. K., and Todd-Brown, K.: Future productivity and carbon storage limited by terrestrial nutrient availability, Nat. Geosci., 8, 441, https://doi.org/10.1038/ngeo2413, 2015b.
Wieder, W. R., Lawrence, D. M., Fisher, R. A., Bonan, G. B., Cheng, S. J., Goodale, C. L., Grandy, A. S., Koven, C. D., Lombardozzi, D. L., and Oleson, K. W.: Beyond static benchmarking: Using experimental manipulations to evaluate land model assumptions, Global Biogeochem. Cy., 33, 1289–1309, 2019.
Wong, S.-C.: Elevated atmospheric partial pressure of CO2 and plant growth: II. Non-structural carbohydrate content in cotton plants and its effect on growth parameters, Photosyn. Res., 23, 171–180, 1990.
Wright, S. J., Turner, B. L., Yavitt, J. B., Harms, K. E., Kaspari, M., Tanner, E. V., Bujan, J., Griffin, E. A., Mayor, J. R., and Pasquini, S. C.: Plant responses to fertilization experiments in lowland, species-rich, tropical forests, Ecology, 99, 1129–1138, 2018.
Xu, R. I. and Prentice, I. C.: Terrestrial nitrogen cycle simulation with a dynamic global vegetation model, Glob. Change Biol., 14, 1745–1764, https://doi.org/10.1111/j.1365-2486.2008.01625.x, 2008.
Yang, X.: ELMv1 outputs for global scale evaluation, figshare [data set], https://doi.org/10.6084/m9.figshare.12021348.v1, 2020.
Yang, X. and Post, W. M.: Phosphorus transformations as a function of pedogenesis: A synthesis of soil phosphorus data using Hedley fractionation method, Biogeosciences, 8, 2907–2916, https://doi.org/10.5194/bg-8-2907-2011, 2011.
Yang, X., Wittig, V., Jain, A., and Post, W.: Integration of nitrogen cycle dynamics into the Integrated Science Assessment Model for the study of terrestrial ecosystem responses to global change, Global Biogeochem. Cy., 23, https://doi.org/10.1029/2009GB003474, 2009.
Yang, X., Post, W. M., Thornton, P. E., and Jain, A.: The distribution of soil phosphorus for global biogeochemical modeling, Biogeosciences, 10, 2525–2537, https://doi.org/10.5194/bg-10-2525-2013, 2013.
Yang, X., Thornton, P. E., Ricciuto, D. M., and Post, W. M.: The role of phosphorus dynamics in tropical forests – a modeling study using CLM-CNP, Biogeosciences, 11, 1667–1681, https://doi.org/10.5194/bg-11-1667-2014, 2014.
Yang, X., Thornton, P. E., Ricciuto, D. M., and Hoffman, F. M.: Phosphorus feedbacks constraining tropical ecosystem responses to changes in atmospheric CO2 and climate, Geophys. Res. Lett., 43, 7205–7214, https://doi.org/10.1002/2016GL069241, 2016.
Yang, X., Ricciuto, D. M., Thornton, P. E., Shi, X., Xu, M., Hoffman, F., and Norby, R. J.: The effects of phosphorus cycle dynamics on carbon sources and sinks in the Amazon region: a modeling study using ELM v1, J. Geophys. Res.-Biogeo., 124, https://doi.org/10.1029/2019JG005082, 2019.
Zaehle, S., Friend, A., Friedlingstein, P., Dentener, F., Peylin, P., and Schulz, M.: Carbon and nitrogen cycle dynamics in the O-CN land surface model: 2. Role of the nitrogen cycle in the historical terrestrial carbon balance, Global Biogeochem. Cy., 24, https://doi.org/10.1029/2009GB003522, 2010.
Zhang, Q., Wang, Y.-P., Matear, R., Pitman, A., and Dai, Y.: Nitrogen and phosphorous limitations significantly reduce future allowable CO2 emissions, Geophys. Res. Lett., 41, 632–637, 2014.
Zhu, Q., Riley, W. J., Tang, J., Collier, N., Hoffman, F. M., Yang, X., and Bisht, G.: Representing nitrogen, phosphorus, and carbon interactions in the E3SM land model: Development and global benchmarking, J. Adv. Model. Earth Syst., 11, 2238–2258, 2019.