the Creative Commons Attribution 4.0 License.
the Creative Commons Attribution 4.0 License.
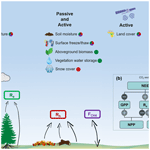
Reviews and syntheses: Recent advances in microwave remote sensing in support of terrestrial carbon cycle science in Arctic–boreal regions
Alex Mavrovic
Oliver Sonnentag
Juha Lemmetyinen
Jennifer L. Baltzer
Christophe Kinnard
Alexandre Roy
Spaceborne microwave remote sensing (300 MHz–100 GHz) provides a valuable method for characterizing environmental changes, especially in Arctic–boreal regions (ABRs) where ground observations are generally spatially and temporally scarce. Although direct measurements of carbon fluxes are not feasible, spaceborne microwave radiometers and radar can monitor various important surface and near-surface variables that affect terrestrial carbon cycle processes such as respiratory carbon dioxide (CO2) fluxes; photosynthetic CO2 uptake; and processes related to net methane (CH4) exchange including CH4 production, transport and consumption. Examples of such controls include soil moisture and temperature, surface freeze–thaw cycles, vegetation water storage, snowpack properties and land cover. Microwave remote sensing also provides a means for independent aboveground biomass estimates that can be used to estimate aboveground carbon stocks. The microwave data record spans multiple decades going back to the 1970s with frequent (daily to weekly) global coverage independent of atmospheric conditions and solar illumination. Collectively, these advantages hold substantial untapped potential to monitor and better understand carbon cycle processes across ABRs. Given rapid climate warming across ABRs and the associated carbon cycle feedbacks to the global climate system, this review argues for the importance of rapid integration of microwave information into ABR terrestrial carbon cycle science.
Northern regions host two important terrestrial biomes, the boreal and the Arctic (hereafter called Arctic–boreal regions, ABRs). Belowground carbon (C) stocks in ABRs comprise 30 %–40 % of the planetary terrestrial carbon and, as such, understanding changes in carbon cycle processes in this vast region has global importance (Pan et al., 2011; Tarnocai et al., 2009). Arctic–boreal regions store substantial quantities of belowground C due to their inherently slow decomposition rates, largely attributable to cold temperatures (Ravn et al., 2020). A large portion of ABRs is underlain by permafrost (perennially frozen ground), which contains approximately half of the world's belowground C stocks (1672 Pg C in the top 3 m of soil; Tarnocai et al., 2009; van Huissteden and Dolman, 2012). In contrast, C stocks in aboveground biomass in ABRs are relatively trivial. For example, although the boreal biome constitutes the second-largest terrestrial biome with a third of the world's forested area, it contains only approximately 15 % of the global forest aboveground biomass (FAO, 2001; Pan et al., 2011, 2013; Carreiras et al., 2017) but 32 % of the global forest carbon stocks (Pan et al., 2011).
Above- and belowground C pools in ABRs are vulnerable to climate change (Grosse et al., 2011). Arctic–boreal regions are warming at a disproportional rate compared to the rest of the planet with potential feedbacks to the global climate system (Box et al., 2019; Derksen et al., 2019; IPCC, 2019; Rantanen et al., 2022). In conjunction with warming temperatures, ABRs are experiencing altered precipitation regimes (Callaghan et al., 2011; Bokhorst et al., 2016; Dolant et al., 2018); permafrost thawing and deepening of the hydrologically and biogeochemically active layer (Liljedahl et al., 2016; Miner et al., 2022); spatially variable vegetation responses associated with “greening” and “browning” (i.e., increasing and decreasing productivity, respectively; Sulla-Menashe et al., 2018; Myers-Smith et al., 2020); earlier spring thaw; later freeze-up and lengthening of the growing season (Kimball et al., 2004a; Euskirchen et al., 2006; Kim et al., 2012); modifications of land cover (Wang et al., 2020); and intensifying disturbance regimes such as fire, drought, pervasive insect infestation and disease (Peng et al., 2011; Yi et al., 2013; Foster et al., 2022). Although ongoing warming has the potential to enhance photosynthesis and plant growth across ABRs, which would also increase aboveground C storage (Sturm et al., 2005; McMahon et al., 2010; Myers-Smith et al., 2020), the vegetation response to climate change is variable and complex. Furthermore, warmer air and soil temperatures enhance soil organic matter decomposition and the subsequent release of carbon dioxide (CO2) via respiration (Schädel et al., 2016). Large uncertainties remain in terrestrial biosphere models used to estimate CO2 and CH4 fluxes in ABRs (Tei and Sugimoto, 2020; Fisher et al., 2018), including the amount of CO2 and CH4 released during winter (Natali et al., 2019; Zona et al., 2015). If increases in ecosystem respiration exceed those of photosynthetic CO2 uptake from enhanced plant growth, ABRs may shift from a weak net CO2 sink to a net CO2 source, thereby generating a potentially non-negligible, positive feedback to the global climate system. This potential change underlines the importance of understanding changes in ABR C pools and fluxes (Hayes et al., 2011; Schuur et al., 2015; Gauthier et al., 2015; Virkkala et al., 2021).
The vastness and remoteness of ABRs make in situ observations challenging and costly. For example, although FLUXNET, the global initiative of tower-based eddy covariance flux observations (Baldocchi et al., 2001; Pastorello et al., 2020), is the most broadly used reference for C-flux measurements at the ecosystem scale, ABRs are notoriously underrepresented in this network (Fig. 1; Baldocchi et al., 2001; Pastorello et al., 2020; Pallandt et al., 2022). Satellite remote sensing and terrestrial biosphere models show promise for monitoring land surface–atmosphere interactions across ABRs (Fisher et al., 2018; Lees et al., 2018). Although direct measurement of C fluxes is not yet possible through remote sensing, it is possible to use spaceborne sensors to monitor variables and ecosystem structural parameters that exert control over various ecosystem processes (J. Du et al., 2019). In the last decade, radiometers sensitive to wavelengths in the visible and infrared portions of the electromagnetic spectrum have been widely used to support C-cycle science in ABRs (Turner et al., 2004; Mao et al., 2016; Lees et al., 2018; Xiao et al., 2019). Visible and infrared remote sensing observations have been used in C-cycle science to map spectral vegetation indices as proxies for vegetation abundance and productivity (e.g., normalized difference vegetation index, NDVI; Tucker, 1979; Lees et al., 2018; S. Du et al., 2019), forest disturbance (e.g., fire, tree mortality; Kim et al., 2012), land cover (Kimball et al., 2009), vegetation structure (e.g., light detection and ranging, lidar; Xiao et al., 2019), snow cover extent (Hori et al., 2017), land surface temperature (Sitch et al., 2007; Xiao et al., 2019), albedo (Xiao et al., 2019), solar-induced chlorophyll fluorescence (Wohlfahrt et al., 2018; Magney et al., 2019), and atmospheric CO2 concentration (Buchwitz et al., 2007; Tu et al., 2020; Lorente et al., 2021). However, spaceborne visible and infrared radiometers are affected by signal degradation from atmospheric effects, have minimal signal penetration depth in vegetation and depend on solar illumination meaning they are restricted to daytime, clear-sky observations (Kim et al., 2012).
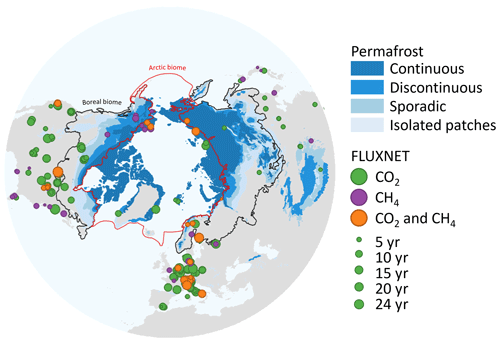
Figure 1Permafrost extent (Brown et al., 2002) and distribution of eddy covariance sites where ecosystem fluxes are monitored continuously (Baldocchi et al., 2001; Pallandt et al., 2022; dot size represents the number of years of available data). The Arctic biome is delineated following the Conservation of Arctic Flora and Fauna working group of the Arctic Council, and the boreal biome is delineated following Potapov et al. (2008). Permafrost extent is estimated in percent areal coverage: continuous (> 90 %–100 % areal extent), discontinuous (> 50 %–90 %), sporadic (10 %–50 %) and isolated patches (< 10 %).
Spaceborne microwave remote sensing can be used in synergy with visible and infrared radiometers to maximize the benefits of a wider frequency span in the electromagnetic spectrum (Sitch et al., 2007; Kimball et al., 2009; Arslan et al., 2011; Kim et al., 2012; Xiao et al., 2019). Microwaves encompass electromagnetic radiation within the frequency range of 0.3–300 GHz, which corresponds to wavelengths from 1 mm to 1 m. Microwave remote sensing provides several advantages such as its relative insensitivity to atmospheric attenuation, cloud cover and solar illumination at frequencies below 100 GHz, which is essential during winter months in regions undergoing polar night (Sitch et al., 2007; J. Du et al., 2019). The spatial resolution of microwave imagery ranges from coarse (several-kilometer scale) to fine (meter scale) with varying temporal resolutions that can reach more than one observation per day. Microwave remote sensing is particularly suitable for ABRs since the signal penetration depth range allows for the retrieval of (1) volumetric information such as certain snow properties including density and microstructure (e.g., Nagler and Rott, 2000; Takala et al., 2011; Lievens et al., 2019) and vegetation optical depth (VOD), which relates to aboveground biomass and vegetation liquid water content (Konings et al., 2017, 2019; Mialon et al., 2020), and (2) near-surface information on variables such as soil moisture (Kerr et al., 2012; Colliander et al., 2017) and the freeze–thaw state (Kim et al., 2012; Roy et al., 2015; Rautiainen et al., 2016; Derksen et al., 2017; Prince et al., 2019) because of its sensitivity to liquid water.
This paper aims to introduce to the C-cycle science community the potential of spaceborne microwave remote sensing to help overcome some of the challenges specifically posed by ABRs for terrestrial C-cycle science and monitoring. We focus on the main vertical C fluxes between the land surface and the atmosphere, specifically, respiratory CO2 fluxes; photosynthetic CO2 uptake; and processes related to net methane (CH4) fluxes governed by CH4 production, transport and consumption. After summarizing the principles of microwave remote sensing (Sect. 2), we review how spaceborne microwave remote sensing can be exploited to help monitor key variables important for CO2 and CH4 fluxes in ABRs. These include soil moisture (Sect. 3.1) and temperature (Sect. 3.2), the surface freeze–thaw status (Sect. 3.3), aboveground biomass (Sect. 3.4), the vegetation water status (Sect. 3.5), land cover (Sect. 3.6), and snow cover (Sect. 3.7). For each variable, we will first explain how the variable at least partially governs C exchanges, outline the potential of microwave remote sensing to monitor the key variable and then introduce presently available microwave remote sensing products related to each variable.
Microwave remote sensing can be used passively, by measuring the natural microwave emission from the planetary surface (using radiometers; receiving only), or actively, by measuring the backscattering of a previously emitted signal (using radar instruments; emitting and receiving).
Radiometers measure the natural microwave emission coming from the planetary surface. This emission is quantified as the brightness temperature (TB; Fig. 2b), which corresponds to the temperature of a blackbody delivering the same luminance as the studied surface. Brightness temperature is related to the physical temperature of a surface () through emissivity (e; unitless). Emissivity is an inherent material property ranging from 0, for a perfectly non-emitting material, to 1, for a purely emitting material (blackbody). The microwave electric field generally displays a preferred orientation, called the microwave polarization, and is often related to the geometric structure of the source or target (Ulaby et al., 1982, 1983). The spatial resolution of passive microwave radiometers is generally coarser than many visible and infrared radiometers owing to the longer wavelengths and practical restrictions of the receiving antenna dimensions on spacecraft (Jenson, 2006). Spaceborne passive microwave radiometers typically have spatial resolutions in the 10–100 km range. Although passive microwave spatial resolutions are often too coarse to capture small-scale landscape heterogeneity (meter to kilometer scale), it is not an obstacle for regional or global applications of terrestrial biosphere models commonly run at spatial resolutions of several kilometers. The temporal resolution of most microwave instruments is generally less than 2–3 d, and in polar regions several overpasses a day may be achieved. The fine temporal resolution might be more critical for terrestrial biosphere models than good spatial resolution since computational power limits the spatial resolution of such models (Washington et al., 2009; Schär et al., 2020).
Radars emit electromagnetic waves to calculate the backscattering coefficient (σ; Fig. 2b) of a target area from the power ratio between the emitted and returned pulse (Ulaby et al., 1982, 1986). The return signal of a radar carries three main pieces of information: the magnitude of σ; the phase of the electromagnetic wave returning; and a delay between the emitted and received signal, which is related to the distance between the radar and the target area. Scatterometers focus particularly on the magnitude of the backscattered signal by a medium to extract backscattering coefficient (Figa-Saldaña et al., 2002). Synthetic-aperture radar (SAR) measures backscattering coefficients using a radar technique that can achieve spatial resolutions at a meter scale by combining scenes of the target area from multiple points of view (Tomiyasu, 1978; Bamler, 2000). Synthetic-aperture radars achieve comparable resolutions to visible and infrared radiometers (i.e., below 10 m of spatial resolution). However, radar transmitters are much more energy-consuming than radiometers because of the power requirement for emitting microwaves. Because of high energy demands, most SAR transmitters operate during a small fraction (1 %–30 %; Grasso et al., 2021; Leanza et al., 2019; Dubock et al., 2001) of their orbit around Earth. The lower the transmission time, the greater the duration between measurements at a given point, often several days (Marghany, 2019). This is currently being overcome via deployment of several satellites equipped with SAR instruments flying in tandem (Sentinel-1; RADARSAT Constellation Mission).
Unlike visible and infrared remote sensing that are limited to information from the surface of any target, the longer wavelengths of microwaves allow for microwaves to penetrate vegetation, ground and snowpack, allowing for subsurface measurements (Ulaby et al., 1986); the longer the microwave, the deeper the penetration depth. However, deeper penetration means that the received signal is a combination of different contributing components, i.e., usually vegetation, soil, snow and atmosphere (Kerr et al., 2012; Roy et al., 2012, 2014), challenging the interpretation of the signal. Consequently, microwave remote sensing is often used together with radiative transfer models to decouple and extract the information on terrestrial surface conditions from microwave observations. These models generally calculate the scattering, reflection and attenuation of the electromagnetic waves of the different components of the surface (Fig. 2) (Mo et al., 1982; El-Rayes and Ulaby, 1987; Huang et al., 2017; Picard et al., 2018). By considering the contribution of each component of the surface (i.e., soil, vegetation and/or snow), radiative transfer models allow for disentangling microwave signals to retrieve key surface state variables of interest (Wigneron et al., 2007).
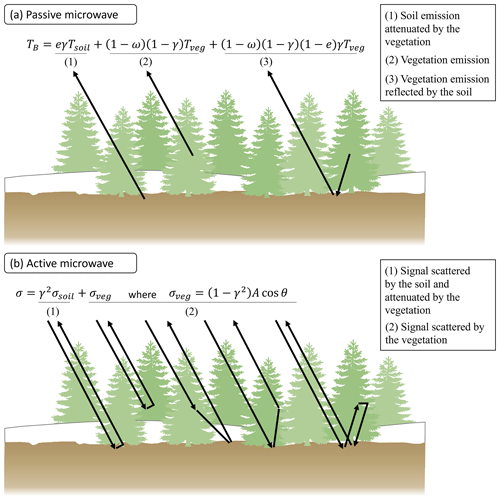
Figure 2Microwave-signal decoupling through electromagnetic wave interaction with matter. (a) Mo et al. (1982) model for passive microwaves with TB representing the brightness temperature, e being the soil emissivity, γ being the vegetation attenuation, ω being the vegetation single-scattering albedo, and T being the thermodynamic temperature of soil and vegetation (Mo et al., 1982). (b) Water cloud model for active microwaves with σ representing the backscattering coefficient, γ being vegetation attenuation, A being a vegetation empirical parameter and θ being the radar incident angle (Attema and Ulaby, 1978). The σveg term is an approximation for the first-order vegetation scattering processes. Snow increases the complexity of the interaction between the microwave and the ground level because it is acting as an additional semi-opaque layer comparable to the vegetation layer. Snow and atmosphere are not accounted for in the equations presented.
Multiple microwave radiometers have been launched in recent decades covering most of the microwave portion of the electromagnetic spectrum: L-band (1–2 GHz), C-band (4–8 GHz), X-band (8–12 GHz), K-band (18–26.5 GHz), Ka-band (26.5–40 GHz) and W-band (75–110 GHz) (Table A1 in Appendix A). There is a nearly continuous publicly available radiometric dataset from 1978 to the present covering the microwave bands from the C-band to the W-band (Fig. 3a). Additionally, in the last decade, L-band data have become accessible through the launch of the Soil Moisture Ocean Salinity mission (SMOS; Kerr et al., 2010), the Aquarius mission (Brucker et al., 2004) and the NASA (National Aeronautics and Space Administration) Soil Moisture Active Passive mission (SMAP; Entekhabi et al., 2010). An exhaustive list of radars would be too long to present here; instead we focus on a selection of recent radar missions (Fig. 3b). These spaceborne radars were selected for the purpose of the review to represent a range of frequencies and available temporal coverage.
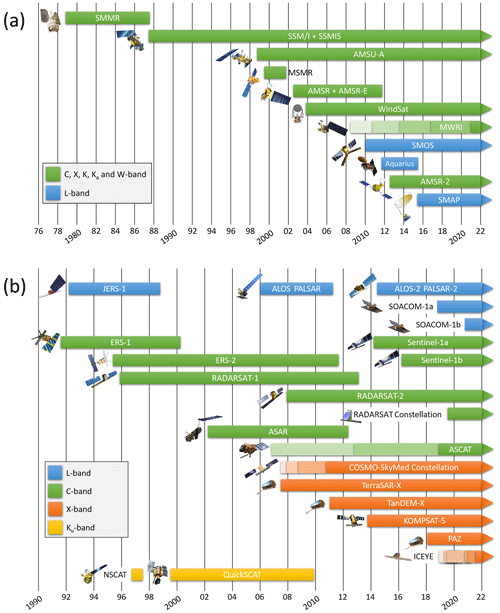
Figure 3(a) Temporal coverage of spaceborne passive microwave instruments (radiometers between 1–100 GHz, sun-synchronous nearly polar orbits; more details in Table A1). (b) Selected number of spaceborne radar missions (active sensors) covering the L-band (1–2 GHz), C-band (4–8 GHz), X-band (8–12 GHz) and Ku-band (12–18 GHz). More details are provided in Table A2.
Although direct measurements of vertical C fluxes are not feasible through microwave remote sensing, it is possible to use spaceborne radiometers and radar to monitor key variables important for different C-cycle processes (Fig. 4). Carbon dioxide and CH4 fluxes, two of the most potent greenhouse gases, are continuously exchanged between Earth's surface and the atmosphere. Carbon dioxide is absorbed through photosynthesis by vegetation and is released by plants and soil through respiration (i.e., autotrophic respiration, Ra, and heterotrophic respiration, Rh, respectively) (Chapin et al., 2006). In ecosystems, CH4 is produced by methanogens under anaerobic conditions and consumed during oxidation by methanotrophs under aerobic conditions (Lai, 2009). Methane transport through the soil column and to the atmosphere occurs through diffusion, ebullition and plant-mediated transport (Lai, 2009).
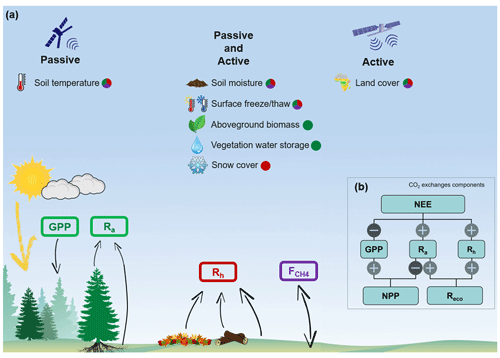
Figure 4(a) Carbon dioxide and methane fluxes between the land surface and the atmosphere including gross primary production (GPP), heterotrophic respiration (Rh), autotrophic respiration (Ra) and net methane fluxes () (units: gC m−2 yr−1). The fluxes are color-coded (green: GPP and Ra; red: Rh; purple: ) to match relevant key variables important for CO2 and CH4 fluxes and derivable with microwave remote sensing. (b) Relationships of net primary productivity and net ecosystem productivity (NEP: negative net ecosystem exchange, NEE) and their component fluxes with ecosystem respiration (ER = Ra+Rh).
3.1 Soil moisture
In ABRs, water availability and air and soil temperatures are considered important environmental constraints on photosynthesis and thus C sequestration (Lieffers and Rothwell, 1987; Angert et al., 2005; Jones et al., 2017). For example, both heat stress and drought can limit tree growth across latitudes in boreal forest stands (Walker and Johnstone, 2014; Sniderhan et al., 2021). Near-surface soil moisture also affects methanogenesis and methanotrophy, which depends strongly on oxygen availability (Lai, 2009). Environmental controls on soil respiration, i.e., the sum of belowground Ra and Rh, include soil moisture and temperature, which in ABRs are both influenced by permafrost and active (seasonally thawed) layer dynamics (Huntzinger et al., 2020). Hence, in a changing climate where soils across ABRs are generally expected to be drying (e.g., Gauthier et al., 2015; Andresen et al., 2020), reliably quantifying soil moisture dynamics and thus water availability will become essential for a predictive understanding of C-cycle dynamics.
The sensitivity of the microwave signal to soil moisture content has been widely demonstrated previously, but several challenges remain including accounting for vegetation attenuation and scattering related to the surface roughness (Das and Paul, 2015; Colliander et al., 2022), as well as organic soil model parametrization (Mironov and Savin, 2015; Bircher et al., 2016). The sensitivity to soil moisture generally increases with lower microwave frequencies, making the L-band the most sensitive to soil moisture content. The depth over which soil moisture content can be estimated from microwave remote sensing is limited at best to the top 5 cm of the soil profile (roots are most dense in the top 20 cm). Measurement depth depends on the frequency used (longer wavelengths realize greater depths; Adams et al., 2015), the soil moisture content, and the vegetation type and density (Wigneron et al., 2007). These measurement depths do not capture the water availability in the full rooting zone, which will be most relevant to predicting the photosynthetic CO2 update. However, it remains possible to estimate root zone soil moisture content from near-surface soil moisture content using pedotransfer equations, meaning these surface measurements can be quite useful (Stefan et al., 2021; Dimitrov et al., 2022).
Extensive methodological research to develop soil moisture retrievals from microwave remote sensing has been motivated for agricultural applications (Engman, 1991; Wigneron et al., 2007; Lakhankar et al., 2009). Soil moisture retrieval was the main motivation behind several passive L-band satellite missions, including SMAP and SMOS. The resulting expertise and soil moisture products present a promising data stream to inform terrestrial biosphere models (see Sect. 4). Table 1 presents several available soil moisture products. The European Space Agency (ESA) Climate Change Initiative (CCI) project produces a soil moisture product merging active and passive microwave sensors, exploiting the microwave instruments temporal coverage since 1978 (Gruber et al., 2019).
Table 1Presently available soil moisture products from spaceborne microwave remote sensing.
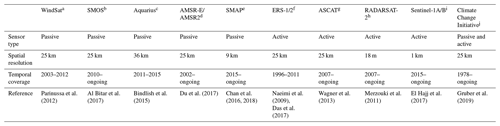
a WindSat/Coriolis surface soil moisture (LPRM) L3 1 day 25 km × 25 km daytime V001. b SMOS L1 and L2 Science data. c Aquarius L3 Weekly Polar-Gridded Sea Surface Salinity, Version 5 (AQ3_SSS). d AMSR-E/AMSR2 Unified L2B Half-Orbit 25 km EASE-Grid Surface Soil Moisture, Version 1 (AU_Land). e SMAP L4 Global 3-hourly 9 km EASE-Grid Surface and Root Zone Soil Moisture Geophysical Data, Version 6 (SPL4SMGP). f ERS-2 SCATTEROMETER Surface Soil Moisture Time Series and Orbit product in High and Nominal Resolution. g ASCAT Soil Moisture at 25 km Swath Grid in NRT – Metop. h RADARSAT-2 Surface Soil Moisture. i 1 km SSM (surface soil moisture) Version 1 product (SSM1km). j ESA CCI SM.
3.2 Soil temperature
Rates of photosynthesis and ecosystem respiration (Reco; Fig. 4) are generally strongly controlled by soil temperature (Angert et al., 2005; Jones et al., 2017; Stocker et al., 2018). Similarly, laboratory and field observations have shown that Rh-related CO2 fluxes are non-negligible below the freezing point and increase with warming soil temperatures (Fahnestock et al., 1998, 1999; Welker et al., 2000; Mikan et al., 2002; Panikov et al., 2006; Natali et al., 2019). Despite lower fluxes, winter CO2 emissions from soil respiration may constitute an important contribution to annual net ecosystem productivity (NEP), especially in ABRs with very short growing seasons (Elberling et al., 2007; Webb et al., 2016; Natali et al., 2019).
Microwave TB depends on thermodynamic temperature (); thus it is possible to retrieve land surface temperature (LST) from radiometric data (Duan et al., 2020). Retrieval of LST from microwave remote sensing is technically more challenging and results in lower precision than similar products from thermal infrared remote sensing, 1–5 K for microwave LST vs. 0.2–2 K for thermal infrared (Jiménez-Muñoz and Sobrino, 2006; Jones et al., 2010; Osińska-Skotak, 2007; Krishnan et al., 2020; Zhang and Cheng, 2020). Microwave emissivity is also more sensitive to environmental changes (e.g., liquid water content) than thermal infrared emissivity. Although infrared LST is more precise than microwave LST, there are instances where microwave LST may be important. Specifically, microwave LST can support gap filling when thermal infrared LST is unavailable because of extended periods of cloud cover or when fine temporal resolution is required. Microwave can be used to sense soil temperature up to a few centimeters deep because of its soil penetration depth. It is an important advantage over thermal infrared remote sensing, which can only detect LST and is subject to significant atmospheric contamination (Jones et al., 2007). Some studies have also shown the potential to use microwave observations to retrieve soil temperature under the snow (Kohn and Royer, 2010; Marchand et al., 2018).
Although several studies have obtained LST from microwave data, no operational microwave LST product is operationally available. Microwave LST algorithms typically achieve a precision of 2–5 K using data from AMSR-E/AMSR2 (Jones et al., 2010; Zhang and Cheng, 2020) and SMM/I and SMMR (Pulliainen et al., 1997; Basist et al., 1998; Fily et al., 2003; Mialon et al., 2007; Royer and Poirier, 2010). Other studies have used machine learning approaches, obtaining precision around 1–3 K with AMSR2 data (Aires et al., 2001; Mao et al., 2018).
3.3 Surface freeze–thaw state
During the short growing season in ABRs, photosynthetic CO2 uptake exceeds the respiratory CO2 losses; thus ABRs generally act as net growing season CO2 sinks (Ciais et al., 2019; Virkkala et al., 2021). However, in winter, plants become dormant and photosynthesis and Ra cease (gross primary productivity, GPP = 0; Ra≈0) owing to the cold temperatures and highly reduced photoperiod (Kimball et al., 2004b; Rafat et al., 2021). Although Rh may continue in frozen soil, it decreases substantially (Natali et al., 2019). Modeling annual NEP in areas undergoing seasonal surface freeze–thaw cycles requires the ability to estimate the length and timing of the growing season (Seiler et al., 2022; El-Amine et al., 2022). The growing season length has a direct impact on annual GPP and thus NEP, but there is also a strong relationship between surface freeze–thaw timing and photosynthetic CO2 uptake (Frolking et al., 1996; McDonald et al., 2004; Kim et al., 2012; Fu et al., 2017; Pierrat et al., 2021). Changes in the timing of spring thaw can create a shift in growing conditions when photosynthesis is initiated (Jarvis and Linder, 2000; Tanja et al., 2003; Kimball et al., 2004a; Piao et al., 2008; Kim et al., 2012; El-Amine et al., 2022). The timing of the start of the growing season has been shown to be more important to annual GPP than the timing of the end because of the superior light and water availability during the spring period (Tanja et al., 2003; El-Amine et al., 2022). The surface freeze–thaw state is a useful proxy for the timing and thus duration of photosynthetic activity (Harrison et al., 2020) and can potentially be used to track CH4 emissions in ABRs (Tenkanen et al., 2021).
Satellite detection of the surface freeze–thaw state is based on the dielectric contrast between water and ice at microwave frequencies. Therefore, soil emissivity is highly sensitive to phase state changes in its liquid content. When water freezes, εsoil drops drastically as liquid water changes to ice because of the crystalline structure of frozen water. The rapid decrease in εsoil in freezing soils translates into a much higher microwave emission and backscattering from the surface. This allows for surface freeze–thaw state retrieval from passive and active microwave measurements using temporal change detection algorithms (Mortin et al., 2012; Rautiainen et al., 2012; Roy et al., 2015; Chen et al., 2019) and threshold-based methods (Kim et al., 2012, 2017; Derksen et al., 2017). Furthermore, for oblique incidence angles, horizontal polarization is more affected than its vertical counterpart during the surface freeze–thaw transition, which favors the use of a polarization ratio as an effective tool for determining the surface freeze–thaw state (Rautiainen et al., 2016; Roy et al., 2017a, b).
The use of passive and active microwave remote sensing for surface freeze–thaw state detection has been widely studied, improving quickly and steadily in the last decade and resulting in various publicly available products (Table 2). As for soil moisture studies, lower microwave frequencies such as the L-band, have been the most exploited because of the prominent water phase dielectric contrast and reduced attenuation from aboveground vegetation and snow (Rautiainen et al., 2016). Surface freeze–thaw state products offer the opportunity to constrain the vegetation growing season timing and photosynthetic CO2 uptake in terrestrial biosphere models.
Table 2Available surface freeze–thaw state products from spaceborne microwave remote sensing.
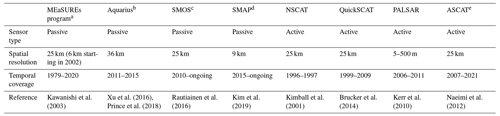
a Sensors: SMMR, SSM/I, SSMIS, AMSR-E and AMSR2. b Aquarius L3 Weekly Polar-Gridded Landscape Freeze/Thaw Data, Version 5 (AQ3_FT). c SMOS Level 3 Freeze and Thaw (F/T). d SMAP Enhanced L3 Radiometer Global and Northern Hemisphere Daily 9 km EASE-Grid Freeze/Thaw State, Version 3 (SPL3FTP_E). e ASCAT Surface Soil Moisture/Freeze-Thaw V2 product.
3.4 Aboveground biomass
Although not a direct control on C-cycle processes, aboveground biomass (AGB) can be used to estimate aboveground C stocks. The annual net gain or loss of C by vegetation (NPP) leads to a proportional increase or decrease in AGB, respectively (Turner et al., 2004; Gough, 2011). Therefore, C storage in aboveground vegetation over a defined period can be inferred from AGB estimates from either terrestrial, aerial or spaceborne techniques (Das et al., 2021). However, using AGB only provides average C exchanges between two data acquisitions and provides no understanding of the underlying ecophysiological and biogeochemical processes. It should be noted that AGB is a minor component of the C stocks in ABRs, where most of the C is stored belowground (Houghton, 2005; Pappas et al., 2020; Walker et al., 2020). Information on AGB allows for initialization, parameterization and evaluation of terrestrial biosphere models and helps to understand, for example, the impact of discrete disturbances such as wildfire and insect outbreaks (Chirici et al., 2016).
Microwave remote sensing can provide information on AGB since microwave wavelengths typically penetrate and interact with moderately dense vegetation cover, depending on the microwave length (Liu et al., 2011a, b). Vegetation attenuation of microwaves is characterized by the vegetation optical depth (VOD; ), which is proportional to vegetation density (i.e., biomass) and water content. Vegetation optical depth is frequency-dependent and affected by the geometry (e.g., canopy structure) of the vegetation (Ulaby et al., 1990). The impact of vegetation geometry on attenuation is challenging to parametrize because of the complexity of forest canopies; therefore it is typically accounted for through vegetation type-dependent, empirically determined parameters introduced in the relationship between VOD, vegetation water content and biomass (Jackson and Schmugge, 1991; Konings et al., 2019). Microwave VOD is better suited to directly monitoring vegetation compared to spectral vegetation indices obtained using visible and infrared wavelengths such as NDVI that saturate at relatively low AGB of around 50–80 Mg ha−1 (Rodríguez-Fernández et al., 2018; Mialon et al., 2020; Turner et al., 2004). In contrast, VOD was shown to saturate AGB of up to 350 Mg ha−1 (Vittucci et al., 2019). It has also been shown that there is a significant difference in what phenological aspects of the growing season are captured by vegetation indices such as NDVI vs. microwave VOD (Milaon et al., 2020). VOD seems to correspond better to key physiological processes such as sap flow and vegetation water storage than vegetation indices such as NDVI, which better capture dynamics of canopy phenology (Lawrence et al., 2014; Cui et al., 2015; Tian et al., 2016; Holtzman et al., 2021; Wigneron et al., 2021).
There is clear potential to improve C-cycle science in boreal forests using VOD-derived AGB (Rodríguez-Fernández et al., 2018). Several recent studies have also shown good correlations between VOD and GPP (Teubner et al., 2018, 2019). The L-band spaceborne radiometer record goes back to 2010 with SMOS, while higher-frequency VOD estimates extend back to the early 1980s. Table 3 presents several VOD products available. Santoro and Cartus (2018) counted 221 studies on SAR data applied to AGB retrieval from 1987 to 2017 using frequencies from 30 MHz up to 12 GHz. AGB investigations using active sensors can achieve a much finer spatial resolution than their passive counterparts, down to the 10 m scale. Also, recent promising advances in SAR interferometry (InSAR), polarimetric InSAR (PolInSAR) and SAR tomography (TomoSAR) techniques provide new opportunities for AGB estimates by surveying the 3D structure of vegetation (Neumann et al., 2012; Tebaldini et al., 2019). InSAR can be used to measure the vertical motion in peatlands, which is a direct indicator of the mass gain or loss by those ecosystems, which constitute a major global C pool (Alshammari et al., 2019; Zhou et al., 2019; Loisel et al., 2021). Some global biomass surveys have also exploited the multi-frequency synergy of data products in the microwave, visible and infrared wavelengths and lidar technologies (e.g., GlobBiomass: Santoro et al., 2018; Mialon et al., 2020). Although the low AGB of the Arctic tundra is challenging to monitor from microwave observations, studies have shown that it is possible to estimate the upper soil organic C (up to 30 cm from the soil–atmosphere interface) using active and passive microwave observations (Bartsch et al., 2016; Yi et al., 2022).
3.5 Vegetation water storage
Water availability is considered an important environmental limitation on photosynthetic processes in ABRs (Ruiz-Pérez and Vico, 2020). In terrestrial biosphere models, a lack of water availability is an environmental stress reducing the photosynthetic capacity (i.e., NPP) (Mu et al., 2007). Water stored in vegetation is critical for stomatal regulation; therefore it is strongly correlated to vegetation growth (Köcher et al., 2013; Matheny et al., 2015). In addition, vegetation water storage can act as a buffer for the daily demands of transpiration (Matheny et al., 2015). However, soil moisture and/or precipitation are generally used to estimate water availability since vegetation water storage estimates are rarely available (Zhang et al., 2015; Stocker et al., 2018).
Since vegetation water storage strongly affects microwave VOD because of the high absorption of microwave by water (Konings et al., 2019), microwave attenuation holds potential for estimating vegetation water storage, which can be used to evaluate vegetation water stress (Holtzman et al., 2021). To the best of our knowledge, no large-scale vegetation water storage product yet exists, but efforts toward this goal are underway (Y. Liu et al., 2021). The microwave VOD sensitivity to both AGB and the vegetation water status complicates its interpretation, although the study of the temporal and spatial trends of VOD can allow for disentangling AGB vs. the vegetation water content (Dou et al., 2023). At short timescales (i.e., diurnal), biomass variation is small and VOD trends can largely be attributed to vegetation water status. At longer timescales (i.e., annual), VOD trends come mostly from biomass dynamics (Mialon et al., 2020). Another method to distinguish water storage and biomass-related VOD changes is to use periods with similar water stress levels for VOD comparison (Konings et al., 2019).
3.6 Land cover
Regional and global studies on C exchanges require information on land cover (Gasser et al., 2020). Repeated satellite-based image classification provides large-scale monitoring of land cover evolution (Wang et al., 2019). Land cover and wetland classifications based on SAR imagery have been developed with the same supervised or unsupervised classification algorithms used for classifying imagery obtained with visible and infrared remote sensing (van Zyl, 1989; Pierce et al., 1994; Dobson et al., 1995; Ranson and Sun, 2000; Bartsch et al., 2007; Whitcomb et al., 2009; Lönnqvist et al., 2010; Merchant et al., 2017, 2022). Recent studies have explored the use of machine learning for land cover classification based on SAR data (Merchant et al., 2019). SAR land cover classifications are enhanced when benefiting from multi-frequency instruments (Saatchi and Rignot, 1997) and multiple polarizations (Lee et al., 2001). The complementarity of SAR and visible/infrared imagery has already been exploited to reinforce spatial and temporal coverage and improve precision of land cover classifications (Töyrä et al., 2001; Ullmann et al., 2014; Merchant et al., 2019). SAR imagery has shown to be especially useful for delineating inundated areas (Bowling et al., 2003) or wet and moist tundra (Morrissey et al., 1996; Merchant et al., 2022), which has a strong impact on CH4 emission (Watts et al., 2014). Microwave observations can also be used to monitor freshwater (FW) waterbody extent dynamics (Murfitt and Duguay, 2021). FW can act as important CH4 emissions sources, especially during ice melt, but aquatic carbon cycle processes are very different than terrestrial carbon processes and were not within of the scope of this review (Matthews et al., 2020).
3.7 Snow cover
Unlike photosynthesis and Ra, Rh can continue through winter in the cold regions. The insulating properties of snow cover have an important indirect impact on C fluxes, keeping the ground warmer than the air during winter, thereby maintaining microbial activity and therefore Rh (Brooks et al., 1997; Brooks and Williams, 1999; Welker et al., 2000; Elberling et al., 2007; Ravn et al., 2020). Through modeling the land surface energy exchanges, the impact of snow on the soil thermal regime can be estimated (Melton et al., 2020). Several studies have demonstrated a correlation between C fluxes and snow depth due to the insulating properties of snow (Björkman et al., 2010; Rogers et al., 2010; Natali et al., 2019). Furthermore, snow density is the main factor controlling snow thermal conductivity (Sturm et al., 1997), which should consequently influence soil temperature, winter CO2 and CH4 fluxes.
Estimating snow accumulation in ABRs represents a challenge for microwave remote sensing. Microwaves are partially absorbed and scattered by snow cover, with an additional challenge in the boreal forest because of snow interception by the forest canopy (Li et al., 2019). Active and passive microwaves can retrieve information about the snowpack status based on microwave interaction with the snow microstructure (Picard et al., 2018). Because multiple snowpack characteristics influence microwaves in many ways (i.e., height, density, microstructure, layering, liquid water content), retrieving snowpack characteristics often leads to an underdetermined equation system (i.e., fewer equations than unknowns). During springtime and winter rain-on-snow (ROS) events, wet snow becomes a major limitation for microwave remote sensing. Liquid water absorbs microwave radiation, reducing microwave penetration depth in wet snow and preventing acquisition of information about either the snow or the underlying ground conditions. This is especially problematic for the retrieval of the surface freeze–thaw state during the spring thaw (Rautiainen et al., 2016). However, snowmelt is easily detected (Forster et al., 2001) and can serve as a proxy for the beginning of the growing season and the resulting initiation of C uptake (Pulliainen et al., 2017).
Estimating the snow water equivalent (SWE; i.e., the product of snow depth and density) is widely used to monitor bulk snow cover using microwaves (Chang et al., 1982; Pulliainen, 2006; Shi et al., 2016; Pulliainen et al., 2020; Saberi et al., 2020; Table 4). It should be noted that uncertainties are higher in the presence of vegetation because of the ensuing microwave attenuation (Mortimer et al., 2020) and for deep snow conditions (SWE > 150 mm: Larue et al., 2017).
Microwave remote sensing has the potential to greatly improve predictions of C fluxes in terrestrial biosphere models. However, the use of key variables obtained from microwave remote sensing to inform terrestrial biosphere models is still limited (Lees et al., 2018). A recent effort assimilates a microwave remote sensing soil moisture data product into a simple C-cycle model to compute the SMAP L4 global daily 9 km EASE-Grid Carbon Net Ecosystem Exchange product (SPL4CMDL; Jones et al., 2017; Fig. 5). The SPL4CMDL product provides daily NEE, GPP, Rh, soil organic C and environmental constraints for eight plant functional types at 9 km spatial resolution. The product is publicly available with data starting in 2015. The L4C carbon exchange model estimates GPP using a light-use efficiency model, where Ra is defined as a fraction of GPP and Rh is estimated using a soil decomposition model with cascading soil organic C. The GEOS-5 atmospheric model is used for the meteorological inputs, and MODIS visible/infrared products are used for land cover and photosynthetically active radiation. There is ongoing work to improve L4C estimates by integrating surface freeze–thaw state information from microwave remote sensing. The SMAP L4C product is currently the only operational C-exchange model using satellite microwave information, which provides direct information for studying seasonal cycles and long-term trends in ecosystem C exchange. SMOS soil moisture data have similarly been used in terrestrial biosphere models but for a shorter period (2010–2015; Wu et al., 2020). Lastly, the terrestrial C-flux (TCF; Kimball et al., 2009) model used AMSR-E soil moisture and temperature information to provide environmental constraints on Rh in Arctic–boreal regions (Baldocchi et al., 2001; Pastorello et al., 2020). Although limited so far, those examples show the potential of integrating microwave products in terrestrial biosphere models.
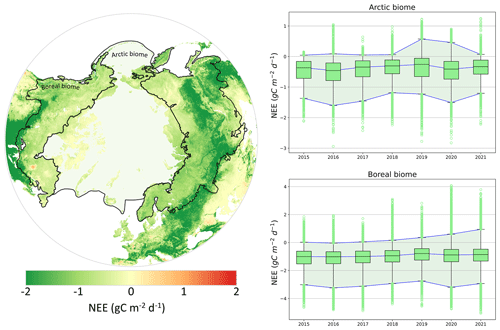
Figure 5Mean monthly net ecosystem exchange (NEE) from the SPL4CMDL for July and August (i.e., peak growing season) between 2015 and 2021. The Arctic biome is delimited following the Conservation of Arctic Flora and Fauna (CAFF) working group of the Arctic Council, and the boreal biome is delineated following Potapov et al. (2008). The yearly mean NEE for the Arctic (Npixel = 282 288) and boreal regions (Npixel = 187 003) is presented as boxplots for the 25th–75th percentiles (box) and the 1st–99th percentiles (bracket). SMAP L4C reference: Kimball et al. (2017).
Although microwave remote sensing data processing is reaching a certain maturity after decades of development, challenges remain in the advancement of microwave data and their assimilation into terrestrial biosphere models (Jones et al., 2017).
5.1 Disentangling the integrated microwave signal
Disentangling the integrated microwave signal originating from varying mixtures of soil, vegetation and snow remains challenging for all microwave remote sensing applications (Kerr et al., 2012; Roy et al., 2012, 2014). Recent advances have been made for both passive and active data to decouple the signal in boreal forests by exploiting multi-polarization, multi-angular and multi-frequency measurements (Larue et al., 2018; Cohen et al., 2019; Konings et al., 2019; Roy et al., 2020). Even in the Arctic biome, where AGB is relatively low, the plot-scale heterogeneity in vegetation composition and structure can pose a challenge since repeated field estimates of vegetation distribution are limited (Myers-Smith et al., 2011; J. Du et al., 2019). Furthermore, the low C fluxes of ABRs can be more challenging to estimate, and vegetation production trends can be lost in interannual variability fluctuations (i.e., low signal-to-noise ratio). The decoupling challenge can be exemplified clearly with the respective effects of soil and vegetation on surface freeze–thaw state detection since soil and vegetation might not freeze or thaw concurrently with implications for detection of surface freeze–thaw events (Roy et al., 2020). Microwave data processing must account for the high density of shallow waterbodies in ABRs, and recent efforts have sought to remove their effect on passive microwave observations in mixed pixels (Touati et al., 2019). These challenges highlight the importance of continued efforts to improve radiative transfer modeling in a way that will allow for the decoupling of the contribution of different components to the microwave signal.
5.2 Intra-pixel variability
Due to the coarse spatial resolution of passive microwave data products, intra-pixel variability and downscaling are important considerations. For example, a recent study from Prince et al. (2019) upholds that the time span of the surface freeze–thaw signal transition in passive microwave measurements might be related to the spatial variability of the soil state (frozen or thawed) through this transition (i.e., patchy frozen soil). Intra-pixel variability also includes the partial presence of snow during transition seasons, vegetation type mixing and snow depth distribution (Meloche et al., 2022). A recent study from Du et al. (2022) showed promising results in downscaling soil moisture to 3 m spatial resolution using machine learning with microwave spaceborne data. Although challenging for subregional studies, the typical passive microwave remote sensing spatial resolution is well suited for regional and global climate studies. Still, most of the terrestrial biosphere model performance evaluation is done using eddy covariance data which are the most trusted and widely used reference for C-flux measurements at large scales. However, the sparsity of the measurement network in ABRs reduces our capacity to represent ABR heterogeneity in the terrestrial biosphere model (Fig. 1; Fisher et al., 2018). However, the scale mismatch between measurements is important to keep in mind; tower-based measurements of C exchanges represent footprints of approximately 1 km or less, which is a much smaller scale than many spaceborne microwave remote sensing data products.
5.3 Potential and upcoming spaceborne microwave remote sensing missions
Upcoming spaceborne microwave remote sensing missions will provide new microwave sensors with additional frequencies and generally improved radiometric and spatial resolution (Table 5). These upcoming missions will increase remote sensing capabilities and extend the continuous microwave coverage over the next decade. The technical and scientific advances made through these missions should be seen as an additional motivation to actively identify ways to integrate microwave data products in C-cycle science. Furthermore, the novel approach of the opportunistic use of spaceborne reflectometry of the global navigation satellite system (GNSS) (W. Li et al., 2022; Yu et al., 2022) already showed promising results in evaluating soil moisture (Edokossi et al., 2020), the soil freeze–thaw state (Rautiainen et al., 2022) and snow water equivalent (Royer et al., 2021).
Microwave remote sensing is an efficient tool for monitoring soil, seasonal snow and vegetation properties affecting water and C-cycle processes and thus has great potential to enhance our understanding of rapidly changing Arctic–boreal regions. Understanding C-cycle processes in Arctic–boreal regions will greatly benefit from an increased use of microwave data, which can only result from expanded collaboration between the microwave remote sensing and C-cycle science communities. The potential applications of satellite-based microwave remote sensing in support of C-cycle science have not been fully realized. We propose four key aspects where increased use of microwave remote sensing could support advances in C-cycle science and monitoring: (1) improve radiative transfer model skills and capabilities and the understanding of microwave signals associated with different surface material; (2) improve assimilation approaches of microwave products in terrestrial biosphere models to overcome the challenges associated with remote cold regions where ground observations are spatially and temporally sparse; (3) develop and maintain long-term, spatially distributed, land-based measurement networks in Arctic–boreal regions to improve microwave-based products; and (4) keep a long-term perspective and coherency between space agencies to maintain the historical trends of microwave observations.
ASAR | Advanced Synthetic Aperture Radar |
ASCAT | Advanced SCATterometer |
AGB | Aboveground biomass |
AMSR | Advanced Microwave Scanning Radiometer |
AMSR-E | AMSR for Earth Observing System |
CARDAMOM | Carbon data model framework |
CCI | Climate Change Initiative |
CIMR | Copernicus Imaging Microwave Radiometer |
CNES | Centre National d'Études Spatiales |
CO2 | Carbon dioxide |
CH4 | Methane |
DLR | German Aerospace Center |
e | Soil emissivity |
ERS | European Remote Sensing satellites |
ESA | European Space Agency |
FAO | Food and Agriculture Organization of the United Nations |
FW | Freshwater |
GEOS-5 | Goddard Earth Observing System, Version 5 |
GNSS | Global navigation satellite system |
GPP | Gross primary production |
H2O | Water |
IFOV | Instantaneous field of view |
InSAR | SAR interferometry |
ISRO | Indian Space Research Organisation |
LAI | Leaf area index |
Lidar | Light detection and ranging |
LST | Land surface temperature |
MEaSUREs | Making Earth System Data Records for Use in Research Environments |
MODIS | Moderate Resolution Imaging Spectroradiometer |
MWRI | Micro-Wave Radiation Imager |
NASA | National Aeronautics and Space Administration |
NDVI | Normalized difference vegetation index |
NEE | Net ecosystem exchange |
NEP | Net ecosystem production |
NISAR | NASA–ISRO SAR mission |
NPP | Net primary production |
NSCAT | NASA Scatterometer |
PALSAR | Phased Array type L-band SAR |
PolInSAR | Polarimetric InSAR |
QuickSCAT | Quick Scatterometer |
Ra | Autotrophic respiration |
Reco | Ecosystem respiration |
Rh | Heterotrophic respiration |
REP | Red-edge position |
ROS | Rain-on-snow events |
ROSE-L | Radar Observing System for Europe – L-Band |
SAR | Synthetic-aperture radar |
SMAP | Soil Moisture Active Passive |
SMAP L4C | SMAP Level-4 carbon product |
SMMR | Scanning Multichannel Microwave Radiometer |
SMOS | Soil Moisture and Ocean Salinity |
SMOS-HR | SMOS High-Resolution |
SWE | Snow water equivalent |
SSM/I | Special Sensor Microwave/Imager |
SSMIS | Special Sensor Microwave Imager/Sounder |
TB | Brightness temperature |
TCF | Terrestrial carbon flux |
TomoSAR | SAR tomography |
VOD | Vegetation optical depth |
γ | Vegetation attenuation |
η | Light conversion efficiency |
ηmax | Light conversion efficiency maximum in optimal conditions |
ε | Relative permittivity |
θ | Incident angle |
σ | Backscattering coefficient |
τ | Optical depth |
Ω | Vegetation single-scattering albedo |
The SMAP L4C product used to generate Fig. 5 is available at https://gmao.gsfc.nasa.gov/pubs/docs/Kimball852.pdf (Kimball et al., 2017).
AM, OS and AR conceptualized the manuscript goal and objectives. AM prepared the manuscript with contributions from all co-authors.
The contact author has declared that none of the authors has any competing interests.
Publisher’s note: Copernicus Publications remains neutral with regard to jurisdictional claims in published maps and institutional affiliations.
This work was made possible thanks to a PhD scholarship obtained from the Natural Sciences and Engineering Research Council of Canada (NSERC) and funding obtained from the Fonds Québécois de la Recherche sur la Nature et les Technologies (FQRNT) and the Canadian Space Agency (CSA).
This research has been supported by the Natural Sciences and Engineering Research Council of Canada (grant no. BESD3-546985-2020) and the Fonds Québécois de la Recherche sur la Nature et les Technologies (grant no. 273500).
This paper was edited by Kirsten Thonicke and reviewed by two anonymous referees.
Adams, J., McNairn, H., Berg, A., and Champagne, C.: Evaluation of near-surface soil moisture data from an AAFC monitoring network in Manitoba, Canada: implications for L-band satellite validation, J. Hydrol., 521, 582–592, https://doi.org/10.1016/j.jhydrol.2014.10.024, 2015.
Aires, F., Prigent, C., Rossow, W., and Rothtein, M.: A new neural network approach including first guess for retrieval of atmospheric water vapor, cloud liquid water path, surface temperature, and emissivities over land from satellite microwave observations, J. Geophys. Res.-Atmos., 106, 14887–14907, https://doi.org/10.1029/2001JD900085, 2001.
Al Bitar, A., Mialon, A., Kerr, Y. H., Cabot, F., Richaume, P., Jacquette, E., Quesney, A., Mahmoodi, A., Tarot, S., Parrens, M., Al-Yaari, A., Pellarin, T., Rodriguez-Fernandez, N., and Wigneron, J.-P.: The global SMOS Level 3 daily soil moisture and brightness temperature maps, Earth Syst. Sci. Data, 9, 293–315, https://doi.org/10.5194/essd-9-293-2017, 2017.
Alshammari, L., Boyd, D., Sowter, A., Marshall, C., Anderson, R., Gilbert, P., Marsh, S., and Large, D.: Use of Surface Motion Characteristics Determined by InSAR to Assess Peatland Condition, J. Geophys. Res.-Biogeo., 125, 293–315, https://doi.org/10.1029/2018JG004953, 2019.
Alvarez-Salazar, O., Hatch, S., Rocca, J., Rosen, P., Shaffer, S., Shen, Y., Sweetser, T., and Xaypraseuth, P.: Mission design for NISAR repeat-pass Interferometric SAR. Sensors, Systems, and Next-Generation Satellites XVIII, 92410C, 11 November 2014, Amsterdam, the Netherlands, 2014.
Andresen, C. G., Lawrence, D. M., Wilson, C. J., McGuire, A. D., Koven, C., Schaefer, K., Jafarov, E., Peng, S., Chen, X., Gouttevin, I., Burke, E., Chadburn, S., Ji, D., Chen, G., Hayes, D., and Zhang, W.: Soil moisture and hydrology projections of the permafrost region – a model intercomparison, The Cryosphere, 14, 445–459, https://doi.org/10.5194/tc-14-445-2020, 2020.
Angert, A., Biraud, S., Bonfils, C., Henning, C., Buermann, W., Pinzon, J., Tucker, C., and Fung, I.: Drier summers cancel out the CO2 uptake enhancement induced by warmer springs, P. Natl. Acad. Sci. USA, 102, 10823–10827, https://doi.org/10.1073/pnas.0501647102, 2005.
Arslan, A., Mattila, O.-P., Markkanen, T., Böttcher, K., Susiluoto, J., Törmä, M., Lemmetyinen, J., Metsämäki, S., Aurela, M., Kervinen, M., Takala, M., Härmä, P, Aalto, T., Laurila, T., and Pulliainen, J.: SNOWCARBO: Monitoring and assessment of carbon balance related phenomena in Finland and northern Eurasia, 2011 IEEE International Geoscience and Remote Sensing Symposium, Vancouver, BC, Canada, 3206–3209, https://doi.org/10.1109/IGARSS.2011.6049901, 2011.
Attema, E. and Ulaby, F.: Vegetation modeled as a water cloud, Radio Sci., 13, 357–364, https://doi.org/10.1029/RS013i002p00357, 1978.
Bachmann, M., Borla Tridon, D., De Zan, F., Krieger, G., and Zink, M.: Tandem-L observation concept – An acquisition scenario for the global scientific mapping machine, Proceedings of EUSAR 2016: 11th European Conference on Synthetic Aperture Radar, 6–9 June 2016, Hamburg, Germany, 1–5, 2016.
Baldocchi, D., Falge, E., Gu, L., Olson, R., Hollinger, D., Running, S., Anthoni, P., Bernhofer, C., Davis, K., Evans, R., Fuentes, J., Goldstein, A., Katul, G., Law, B., Lee, X., Malhi, Y., Meyers, T., Munger, W., Oechel, W., Paw U, K., Pilegaard, K., Schmid, H., Valentini, R., Verma, S., Vesala, T., Wilson, K., and Wofsy, S.: FLUXNET: A New Tool to Study the Temporal and Spatial Variability of Ecosystem–Scale Carbon Dioxide, Water Vapor, and Energy Flux Densities, B. Am. Meteorol. Soc., 82, 2415–2434, https://doi.org/10.1175/1520-0477(2001)082<2415:fantts>2.3.co;2, 2001.
Bamler, R.: Principles of Synthetic Aperture Radar, Surv. Geophys., 21, 147–157, https://doi.org/10.1023/A:1006790026612, 2000.
Bartsch, A., Kidd, R., Pathe, C., Scipal, K., and Wagner, W.: Satellite radar imagery for monitoring inland wetlands in boreal and sub-arctic environments, Aquat. Conserv., 17, 305–317, https://doi.org/10.1002/aqc.836, 2007.
Bartsch, A., Widhalm, B., Kuhry, P., Hugelius, G., Palmtag, J., and Siewert, M. B.: Can C-band synthetic aperture radar be used to estimate soil organic carbon storage in tundra?, Biogeosciences, 13, 5453–5470, https://doi.org/10.5194/bg-13-5453-2016, 2016.
Basist, A., Grody, N., Peterson, T., and Williams, C.: Using the special sensor microwave/imager to monitor land surface temperatures, wetness, and snow cover, J. Appl. Meteorol. Clim., 37, 888–911, https://doi.org/10.1175/1520-0450(1998)037<0888:UTSSMI>2.0.CO;2, 1998.
Bindlish, R., Jackson, T., Cosh, M., Zhao, T., and O'Neill, P.: Global soil moisture from the Aquarius/SAC-D satellite: description and initial assessment, IEEE T. Geosci. Remote Sens., 12, 923–927, https://doi.org/10.1109/LGRS.2014.2364151, 2015.
Bircher, S., Demontoux, F., Razafindratsima, S., Zakharova, E., Drusch, M., Wigneron, J.-P., and Kerr, Y.: L-Band Relative Permittivity of Organic Soil Surface Layers – A New Dataset of Resonant Cavity Measurements and Model Evaluation, Remote Sens., 8, 1024, https://doi.org/10.3390/rs8121024, 2016.
Björkman, M., Morgner, E., Cooper, E., Elberling, B., Klemedtsson, L., and Björk, R.: Winter carbon dioxide effluxes from Arctic ecosystems : An overview and comparison of methodologies, Gobal Biogeochem. Cy., 24, GB3010, https://doi.org/10.1029/2009GB003667, 2010.
Bokhorst, S., Pedersen, S., Brucker, L., Anisimov, O., Bjerke, J., Brown, R., Ehrich, D., Essery, R., Heilig, A., Ingvander, S., Johansson, C., Johansson, M., Jónsdóttir, I. S., Inga, N., Luojus, K., Macelloni, G., Mariash, H., McLennan, D., Rosqvist, G., Sato, A., Savela, H., Schneebeli, M., Sokolov, A., Sokratov, S., Terzago, S., Vikhamar-Schuler, D., Williamson, S., Qiu, Y., and Callaghan, T.: Changing Arctic snow cover: A review of recent developments and assessment of future needs for observations, modeling, and impacts, Ambio, 45, 516–537, https://doi.org/10.1007/s13280-016-0770-0, 2016.
Bowling, L., Kane, D., Gieck, R., Hinzman, L., and Lettenmaier, D.: The role of surface storage in a low-gradient Arctic watershed, Water Resour. Res., 39, 1087, https://doi.org/10.1029/2002WR001466, 2003.
Box, J., Colgan, W., Christensen, T. R., Schmidt, N. M., Lund, M., Parmentier, F.-J., Brown, R., Bhatt, U., Euskirchen, E., and Romanovsky, V.: Key indicators of Arctic climate change: 1971–2017, Environ. Res. Lett., 14, 045010, https://doi.org/10.1088/1748-9326/aafc1b, 2019.
Brooks, P. and Williams, M.: Snowpack controls on nitrogen cycling and export in seasonally snow-covered catchments, Hydrol. Process., 13, 2177–2190, https://doi.org/10.1002/(SICI)1099-1085(199910)13:14/15<2177::AID-HYP850>3.0.CO;2-V, 1999.
Brooks, P., Schmidt, S., and Williams, M.: Winter production of CO2 and N2O from alpine tundra: Environmental controls and relationship to inter-system C and N fluxes, Oecologia, 110, 403–413, https://doi.org/10.1007/PL00008814, 1997.
Brown, J., Ferrians, O., Heginbottom, J., and Melnikov, E.: Circum-Arctic Map of Permafrost and Ground-Ice Conditions, Version 2. Boulder, Colorado USA, NSIDC, National Snow and Ice Data Center [data set], https://doi.org/10.7265/skbg-kf16, 2002.
Brucker, L., Dinnat, E. P., and Koenig, L. S.: Weekly gridded Aquarius L-band radiometer/scatterometer observations and salinity retrievals over the polar regions – Part 1: Product description, The Cryosphere, 8, 905–913, https://doi.org/10.5194/tc-8-905-2014, 2014.
Buchwitz, M., Schneising, O., Burrows, J. P., Bovensmann, H., Reuter, M., and Notholt, J.: First direct observation of the atmospheric CO2 year-to-year increase from space, Atmos. Chem. Phys., 7, 4249–4256, https://doi.org/10.5194/acp-7-4249-2007, 2007.
Callaghan, T., Johansson, M., Brown, R., Groisman, P., Labba, N., Radionov, V., Bradley, R., Blangy, S., Bulygina, O., Christensen, T., Colman, J., Essery, R., Forbes, B., Forchhammer, M., Golubev, V., Honrath, R., Juday, G., Meshcherskaya, A., Phoenix, G., Pomeroy, J., Rautio, A., Robinson, D., Schmidt, N., Serreze, M., Shevchenko, V., Shiklomanov, A., Shmakin, A., Sköld, P., Sturm, M., Woo, M., Woodm E.: Multiple effects of changes in Arctic snow cover, Ambio, 40, 32–45, https://doi.org/10.1007/s13280-011-0213-x, 2011.
Carreiras, J., Quegan, S., Le Toan, T., Ho Tong Minh, D., Saatchi, S., Carvalhais, N., Reichstein, M., and Scipal, K.: Coverage of high biomass forests by the ESA BIOMASS mission under defense restrictions, Remote Sens. Environ., 196, 154–162, https://doi.org/10.1016/j.rse.2017.05.003, 2017.
Chan, S., Bindlish, R., O'Neill, P., Njoku, E., Jackson, T., Colliander, A., Chen, F., Burgin, M., Dunbar, S., Piepmeier, J., Yueh, S., Entekhabi, D., Cosh, M., Caldwell, T., Walker, J., Berg, A., Rowlandson, T., Pacheco, A., McNairn, H., Thibeault, M., Martinez-Fernández, J., González-Zamora, A., Bosch, D., Starks, P., Goodrich, D., Prueger, J., Palecki, M., Small, E., Zreda, M., Calvet, J., Crow, W., and Kerr., Y.: Assessment of the SMAP passive soil moisture product, IEEE T. Geosci. Remote, 54, 4994–5007, https://doi.org/10.1109/TGRS.2016.2561938, 2016.
Chan, S., Bindlish,, R., O'Neill, P., Jackson, T., Njoku, E., Dunbar, S., Chaubell, J., Piepmeier, J., Yueh, S., Entekhabi, D., Colliander, A., Chen, F., Cosh, M., Caldwell, T., Walker, J., Berg, A., McNairn, H., Thibeault, M., Martinez- Fernández, J., Uldall, F., Seyfried, M., Bosch, D., Starks, P., Collins, C., Prueger, J., Van der Velde, R., Asanuma, J., Palecki, M., Small, E., Zreda, M., Calvet, J., Crow, W., and Kerr, Y.: Development and assessment of the SMAP enhanced passive soil moisture product. Remote Sens. Environ., 204, 931–941, https://doi.org/10.1016/j.rse.2017.08.025, 2018.
Chang, A., Foster, J., Hall, D., Rango, A., and Hartline, B.: Snow water equivalent estimation by microwave radiometry, Cold Reg. Sci. Technol., 5, 259–267, https://doi.org/10.1016/j.jag.2011.10.014, 1982.
Chapin III, F., Woodwell, G., Randerson, J., Rastetter, E., Lovett, G., Baldocchi, D., Clark, D., Harmon, M., Schimel, D., Valentini, R., Wirth, C., Aber, J., Cole, J., Goulden, M., Harden, J., Heimann, M., Howarth, R., Matson, P., McGuire, A., Melillo, J., Mooney, H., Neff, J., Houghton, R., Pace, M., Ryan, M., Running, S., Sala, O., Schlesinger, W., and Schulze, E.-D.: Reconciling carbon-cycle concepts, terminology, and methods, Ecosystems, 9, 1041–1050, https://doi.org/10.1007/s10021-005-0105-7, 2006.
Chen, X., Liu, L., and Bartsch, A.: Detecting soil freeze/thaw onsets in Alaska using SMAP and ASCAT data, Remote Sens. Environ., 220, 59–70, https://doi.org/10.1016/j.rse.2018.10.010, 2019.
Chirici, G., Chiesi, M., Corona, P., Salvati, R., Papale, D., Fibbi, L., Sirca, C., Spano, D., Duce, P., Marras, S., Matteucci, G., Cescatti, A., and Maselli, F.: Estimating daily forest carbon fluxes using a combination of ground and remotely sensed data, J. Geophys. Res.-Biogeo., 121, 266–279, https://doi.org/10.1002/2015JG003019, 2016.
Ciais, P., Tan, J., Wang, X., Roedenbeck, C., Chevallier, F., Piao, S.-L., Moriarty, R., Broquet, G., Le Quéré, C., Canadell, J., Peng, S., Poulter, B., Liu, Z., and Tans, P.: Five decades of northern land carbon uptake revealed by the interhemispheric CO2 gradient, Nature, 568, 221–225, https://doi.org/10.1038/s41586-019-1078-6, 2019.
Cohen, J., Rautiainen, K., Ikonen, J., Lemmetyinen, J., Smolander, T., Vehvilêinen, J., and Pulliainen, J.: A modeling-based approach for soil frost detection in the northern boreal forest region with C-Band SAR, IEEE T. Geosci. Remote, 57, 1069–1083, https://doi.org/10.1109/TGRS.2018.2864635, 2019.
Colliander, A., Jackson, T., Bindlish, R., Chan, S., Das, N., Kim, S., Cosh, M., Dunbar, R., Dang, L., Pashaian, L., Asanuma, J., Aida, K., Berg, A., Rowlandson, T., Bosch, D., Caldwell, T., Caylor, K., Goodrich, D., al Jassar, H., Lopez-Baeza, E., Martínez Fernández, J., González-Zamora, A., Livingston, S., McNairn, H., Pacheco, A., Moghaddam, M., Montzka, C., Notarnicola, C., Niedrist, G., Pellarin, T., Prueger, J., Pulliainen, J., Rautiainen, K., Ramos, J., Seyfried, M., Starks, P., Su, Z., Zeng, Y., van der Velde, R., Thibeault, M., Dorigo, W., Vreugdenhil, M., Walker, J. P., Wu, X., Monerris, A., O'Neill, P. E., Entekhabi, D., Njoku, E. G., Yueha, S.: Validation of SMAP surface soil moisture products with core validation sites, Remote Sens. Environ., 191, 215–231, https://doi.org/10.1016/j.rse.2017.01.021, 2017.
Colliander, A., Reichle, R., Crow, W., Cosh, M., Chen, F., Chan, S., Das, N., Bindlish, R., Chaubell, J., Kim, S., Liu, Q., O'Neill, P., Dunbar, R. S., Dang, L., Kimball, J., Jackson, T., Al-Jassar, H., Asanuma, J., Bhattacharya, B., Berg, A., Bosch, D., Bourgeau-Chevez, L., Caldwell, T., Calvert, J.-C., Collins, C. H., Jenson, K., Livingston, S., Lopez-Baeza, E., Martínez-Fernández, J., McNairn, H., Moghaddam, M., Montzka, C., Notarnicola, C., Pellarin, T., Greimeister-Pfeil, I., Pulliainen, J., Gpe, J., Hernández, R., Seyfried, M., Starks, P., Su, Z., van der Velde, R., Zeng, Y., Thibeault, M., Vreugdenhil, M., Walker, J., Zribi, M., Entekhabi, D., and Yueh, S.: Validation of soil moisture data products from the NASA SMAP mission, IEEE J. Sel. Top. Appl., 15, 364–392, https://doi.org/10.1109/JSTARS.2021.3124743, 2022.
Cui, Q., Shi, J., Du, J., Zhao, T., and Xiong, C.: An approach for monitoring global vegetation based on multiangular observations from SMOS, IEEE J. Sel. Top. Appl., 8, 604–616, https://doi.org/10.1109/JSTARS.2015.2388698, 2015.
Das, B., Bordoloi, R., Deka, S., Paul, A., Pandey, P. K., Singha, L. B., Tripathi, O. P., Mishra, B. P., and Mishra, M.: Above ground biomass carbon assessment using field, satellite data and model based integrated approach to predict the carbon sequestration potential of major land use sector of Arunachal Himalaya, India, Carbon Manag., 12, 201–214, https://doi.org/10.1080/17583004.2021.1899753, 2021.
Das, K. and Paul, P.: Present status of soil moisture estimation by microwave, Remote Sens., Cogent Geoscience, 1, 1084669, https://doi.org/10.1080/23312041.2015.1084669, 2015.
Das, N., Entekhabi, D., Kim, S., Yueh, S. Dunbar, R. S., and Colliander, A.: SMAP/Sentinel-1 L2 Radiometer/Radar 30-Second Scene 3 km EASE-Grid Soil Moisture, Version 1. Boulder, Colorado USA, NASA National Snow and Ice Data Center Distributed Active Archive Center [data set], https://doi.org/10.5067/9UWR1WTHW1WN, 2017.
Derksen, C., Xu, X., Scott Dunbar, R., Colliander, A., Kim, Y., Kimball, J. S., Black, T. A., Euskirchen, E., Langlois, A., Loranty, M. M., Marsh, P., Rautiainen, K., Roy, A., Royer, A., and Stephens, J.: Retrieving landscape freeze/thaw state from Soil Moisture Active Passive (SMAP) radar and radiometer measurements, Remote Sens. Environ., 194, 48–62, https://doi.org/10.1016/j.rse.2017.03.007, 2017.
Derksen, C., Burgess, D., Duguay, C., Howell, S., Mudryk, L., Smith, S., Thackeray, C., and Kirchmeier-Young, M.: Changes in snow, ice, and permafrost across Canada. Canada's Changing Climate Report – Chap. 5, Government of Canada, Ottawa, Ontario, Canada, 194–260, 2019.
Dimitrov, D. D., Lafleur, P., Sonnentag, O., Talbot, J., and Quinton, W. L.: Hydrology of peat estimated fron near-surface water contents, Hydrolog. Sci. J., 67, 1702–1721, 2022.
Dobson, M., Ulaby, F., Hallikainen, M., and El-Rayes, M.: Microwave dielectric behavior of wet soil – Part II: Dielectric mixing models, IEEE T. Geosci. Remote Sens., 23, 35–46, https://doi.org/10.1109/TGRS.1985.289498, 1985.
Dobson, M., Ulaby, F., and Pierce, L.: Land-cover classification and estimation of terrain attributes using synthetic aperture radar, Remote Sens. Environ., 51, 199–214, https://doi.org/10.1016/0034-4257(94)00075-X, 1995.
Dolant, C., Langlois, A., Brucker, L., Royer, A., Roy, A., and Montpetit, B. L.: Meteorological inventory of rain-on-snow events in the Canadian Arctic Archipelago and satellite detection assessment using passive microwave data, Phys. Geogr., 39, 428–444, https://doi.org/10.1080/02723646.2017.1400339, 2018.
Dou, Y., Tian, F., Wigneron, J. P., Tagesson, T., Du, J., Brandt, M., Liu, Y., Zou, L., Kimball, J. S., and Fensholt, R.: Reliability of using vegetation optical depth for estimating decadal and interannual carbon dynamics, Remote Sens. Environ., 285, 113390, https://doi.org/10.1016/j.rse.2022.113390, 2023.
Du, J., Kimball, J. S., Jones, L. A., Kim, Y., Glassy, J., and Watts, J. D.: A global satellite environmental data record derived from AMSR-E and AMSR2 microwave Earth observations, Earth Syst. Sci. Data, 9, 791–808, https://doi.org/10.5194/essd-9-791-2017, 2017.
Du, J., Watts, J., Jiang, L., Lu, H., Cheng, X., Duguay, C., Farina, M., Qiu, Y., Kim, Y., Kimball, J., and Tarolli, P.: Remote sensing of environmental changes in cold regions: methods, achievements and challenges, Remote Sens., 11, 1952, https://doi.org/10.3390/rs11161952, 2019.
Du, J., Kimball, J. S., Bindlish, R., Walker, J. P., and Watts, J. D.: Local Scale (3-m) Soil Moisture Mapping Using SMAP and Planet SuperDove, Remote Sens., 14, 3812, https://doi.org/10.3390/rs14153812, 2022.
Du, S., Liu, L., Liu, X., Guo, J., Hu, J., Wang, S., and Zhang, Y.: SIFSpec: Measuring solar-induced chlorophyll fluorescence observations for remote sensing of photosynthesis, Sensors, 19, 3009, https://doi.org/10.3390/s19133009, 2019.
Dubock, D., Spoto, F., Simpson, J., Spencer, D., Schutte, E., and Sontag, H.: The Envisat satellite and its integration, ESA Bull., 106, 26–45, 2001.
Duan, S.-B., Han, X.-J., Huang, C., Li, Z.-L., Wu, H., Qian, Y., Gao, M., Leng, P.: Land surface temperature retrieval from passive microwave satellite observations: state-of-the-art and future directions, Remote Sens., 12, 2573, https://doi.org/10.3390/rs12162573, 2020.
Edokossi, K., Calabia, A., Jin, S., and Molina, I.: GNSS-Reflectometry and Remote Sensing of Soil Moisture: A Review of Measurement Techniques, Methods, and Applications, Remote Sens., 12, 614, https://doi.org/10.3390/rs12040614, 2020.
El-Amine, M., Roy, A., Koebsch, F., Baltzer, J., Barr, A., Black, A., Ikawa, H., Iwata, H., Kobayashi, H., Ueyama, M., and Sonnentag, O.: What explains the year-to-year variation in thestart and end of the photosynthetic growing season of boreal black spruceforests?, Agr. Forest Meteorol., 324, 109113, https://doi.org/10.1016/j.agrformet.2022.109113, 2022.
Elberling, B.: Annual soil CO2 effluxes in the High Arctic: The role of snow thickness and vegetation type, Soil Biol. Biochem., 39, 646–654, https://doi.org/10.1016/j.soilbio.2006.09.017, 2007.
El Hajj, M., Baghdadi, N., Zribi, M., and Bazzi, H.: Synergic Use of Sentinel-1 and Sentinel-2 Images for operational soil moisture mapping at high spatial resolution over agricultural areas, Remote Sens., 9, 1292, https://doi.org/10.3390/rs9121292, 2017.
El-Rayes, M. and Ulaby, F.: Microwave dielectric spectrum of vegetation-Part I: Experimental observations, IEEE T. Geosci. Remote, 25, 541–549, https://doi.org/10.1109/TGRS.1987.289832, 1987.
Engman, E.: Applications of microwave remote sensing of soil moisture for water resources and agriculture, Remote Sens. Environ., 35, 213-2-26, https://doi.org/10.1016/0034-4257(91)90013-V, 1991.
Entekhabi, D., Njoku, E., O'Neill, P., Kellogg, K., Crow, W., Edelstein, W., Entin, J., Goodman, S., Jackson, T., Jackson, J., Kimball, J., Piepmeier, J., Koster, R., Martin, N., McDonald, K., Moghaddam, M., Moran, S., Reichle, R., Shi, J., Spencer, M., Thurman, S., Tsang, L., and Van Zyl, J.: The Soil Moisture Active Passive (SMAP) mission, P. IEEE, 98, 704–716, https://doi.org/10.1109/JPROC.2010.2043918, 2010.
Euskirchen, E., McGuire, A., Kicklighter, D., Zhuang, Q., Clein, J., Dargaville, R., Dye, D., Kimball, J., McDonald, K., Melilli, J., Romanovsky, V., and Smith, N.: Importance of recent shifts in soil thermal dynamics on growing season length, productivity, and carbon sequestration in terrestrial high-latitude ecosystems, Glob. Change Biol., 12, 731–750, https://doi.org/10.1111/j.1365-2486.2006.01113.x, 2006.
Fahnestock, J., Jones, M., Brooks, P., Walker, D., and Welker, J.: Winter and early spring CO2 efflux from tundra communities of northern Alaska, J. Geophys. Res., 103, 29023–29027, https://doi.org/10.1029/98JD00805, 1998.
Fahnestock, J., Jones, M., and Welker, J.: Wintertime CO2 efflux from arctic soils: implications for annual carbon budgets, Gobal Biogeochem. Cy., 13, 775–779, https://doi.org/10.1029/1999gb900006, 1999.
FAO – Food and Agriculture Organization of the United Nations: Global forest resources assessment 2000: main report, FAO Forestry Paper 140, United Nations, Rome, Italy, 479 pp., https://www.fao.org/3/Y1997E/Y1997E00.htm (last access: 16 July 2023), 2001.
Figa-Saldaña, J., Wilson, J., Attema, E., Gelsthorpe, R., Drinkwater, M., and Stoffelen, A.: The advanced scatterometer (ASCAT) on the meteorological operational (MetOp) platform: A follow on for European wind scatterometers, Can. J. Remote Sens., 28, 404–412, https://doi.org/10.5589/m02-035, 2002.
Fily, M., Royer, A., Goïta, K., and Prigent, C.: A simple retrieval method for land surface temperature and fraction of water surface determination from satellite microwave brightness temperatures in sub-arctic areas, Remote Sens. Environ., 85, 328–338, https://doi.org/10.1016/S0034-4257(03)00011-7, 2003.
Fisher, J., Hayes, D., Schwalm, C., Huntzinger, D., Stofferahn, E., Schaefer, K., Luo, Y., Wullschleger, S., Goetz, S., Miller, C., Griffith, P., Chadburn, S., Chatterjee, A., Ciais, P., Douglas, T., Genet, H., Ito, A., Neigh, C., Poulter, B., Rogers, B., Sonnentag, O., Tian, H., Wang, W., Xue, Y., Yang, Z.-L., Zeng, N., and Zhang, Z.: Missing pieces to modeling the Arctic-Boreal puzzle, Environ. Res. Lett., 13, 020202, https://doi.org/10.1088/1748-9326/aa9d9a, 2018.
Forster, R., Long, D., Jezel, K., Brobot, S., and Anderson, M.: The onset of Arctic sea-ice snowmelt as detected with passive- and active-microwave, Ann. Glaciol., 33, 85–93, https://doi.org/10.3189/172756401781818428, 2001.
Foster, A. C., Shuman, J. K., Rogers, B. M., Walker, X. J., Mack, M. C., Bourgeau-Chavez, L. L., Veraverbeke, S., and Goetz, S. J.: Bottom-up drivers of future fire regimes in western boreal North America, Environ. Res. Lett., 17, 025006, https://doi.org/10.1088/1748-9326/ac4c1e, 2022.
Frolking, S., Goulden, M.,Wofsy, S., Fan, S.-M., Sutton, D., Munger, J., Bazzaz, A., Daube, B., Crill, P., Aber, J., Band, L., Wang, X., Savage K., Moore, T., and Harriss, R.: Modeling temporal variability in the carbon balance of a spruce/moss boreal forest, Glob. Change Biol., 2, 343–366, https://doi.org/10.1111/j.1365-2486.1996.tb00086.x, 1996.
Fu, Z., Stoy, P., Luo, Y., Chen, J., Sun, J., Montagnani, L., Wohlfahrt, G., Rahman, A., Rambal, S., Bernhofer, C., Wang, J., Shirkey, G., and Niu, S.: Climate controls over the net carbon uptake period and amplitude of net ecosystem production in temperate and boreal ecosystems., Agr. Forest Meteorol., 243, 9–18, https://doi.org/10.1016/j.agrformet.2017.05.009, 2017.
Gaiser, P., St. Germain, K., Twarog, E., Poe, G., Purdy, W., Richardson, D., Grossman, W., Jones, W., L., Spencer, D., Golba, G., Cleveland, J., Choy, L., Bevilacqua, R., and Chang, P.: The WindSat spaceborne polarimetric microwave radiometer: sensor description and early orbit performance, IEEE T. Geosci. Remote Sens., 42, 2347–2361, https://doi.org/10.1109/TGRS.2004.836867, 2004.
Gasser, T., Crepin, L., Quilcaille, Y., Houghton, R. A., Ciais, P., and Obersteiner, M.: Historical CO2 emissions from land use and land cover change and their uncertainty, Biogeosciences, 17, 4075–4101, https://doi.org/10.5194/bg-17-4075-2020, 2020.
Gauthier, S., Bernier, P., Kuuluvainen, T., Shvidenko, A., and Schepaschenko, D.: Boreal forest health and global change, Science, 349, 819–822, https://doi.org/10.1126/science.aaa9092, 2015.
Gloersen, P. and Barath, F.: A scanning multichannel microwave radiometer for Nimbus-G and SeaSat-A, IEEE J. Ocean. Eng., 2, 172–178, https://doi.org/10.1109/JOE.1977.1145331, 1977.
Gough, C. M.: Terrestrial primary production: Fuel for life, Nat. Educ. Knowl., 3, p. 28, 2011.
Grasso, M., Renga, A., Fasano, G., Graziano, M., Grassi, M., and Moccia, A.: Design of an end-to-end demonstration mission of a Formation-Flying Synthetic Aperture Radar (FF-SAR) based on microsatellites, Adv. Space Res., 67, 3909–3923, https://doi.org/10.1016/j.asr.2020.05.051, 2021.
Grosse, G., Harden, J., Turetsky, M., McGuire, D., Camill, P., Tarnocai, C., Frolking, S., Schuur, E., Jorgenson, T., Marchenko, S., Romanovsky, V., Wickland, K., French, N., Waldrop, M., Bourgeau-Chavez, L., and Striegl, R.: Vulnerability of high-latitude soil organic carbonin North America to disturbance, J. Geophys. Res., 116, G00K06, https://doi.org/10.1029/2010JG001507, 2011.
Gruber, A., Scanlon, T., van der Schalie, R., Wagner, W., and Dorigo, W.: Evolution of the ESA CCI Soil Moisture climate data records and their underlying merging methodology, Earth Syst. Sci. Data, 11, 717–739, https://doi.org/10.5194/essd-11-717-2019, 2019.
Harrison, J., Sanders-DeMott, R., Reinmann, A., Sorensen, P., Phillips, N., and Templer, P.: Growing-season warming and winter soil freeze/thaw cycles increase transpiration in a northern hardwood forest, Ecology, 101, e03173, https://doi.org/10.1002/ecy.3173, 2020.
Hayes, J., McGuire, A., Kicklighter, D., Gurney, K., Burnside, T., and Melillo, J.: Is the northern high-latitude land-based CO2 sink weakening?. Gobal Biogeochem. Cy., 25, GB3018, https://doi.org/10.1029/2010GB003813, 2011.
Hollinger, J., Peirce, J., and Poe, G.: SSM/I instrument evaluation, IEEE T. Geosci. Remote Sens., 28, 781–790, https://doi.org/10.1109/36.58964, 1990.
Holtzman, N. M., Anderegg, L. D. L., Kraatz, S., Mavrovic, A., Sonnentag, O., Pappas, C., Cosh, M. H., Langlois, A., Lakhankar, T., Tesser, D., Steiner, N., Colliander, A., Roy, A., and Konings, A. G.: L-band vegetation optical depth as an indicator of plant water potential in a temperate deciduous forest stand, Biogeosciences, 18, 739–753, https://doi.org/10.5194/bg-18-739-2021, 2021.
Hori, M., Sugiura, K., Kobayashi, K., Aoki, T, Tanikawa, T., Kuchiki, K., Niwano, M., and Enomoto, H.: A 38-year (1978–2015) Northern Hemisphere daily snow cover extent product derived using consistent objective criteria from satellite-borne optical sensors, Remote Sens. Environ., 191, 402–418, https://doi.org/10.1016/j.rse.2017.01.023, 2017.
Houghton, R.: Aboveground Forest Biomass and the Global Carbon Balance, Glob. Change Biol., 11, 945–958, https://doi.org/10.1111/j.1365-2486.2005.00955.x, 2005.
Huang, H., Tsang, L., Njoku, E., Colliander, A., Liao, T.-H., and Ding, K.-H.: Propagation and Scattering by a Layer of Randomly Distributed Dielectric Cylinders Using Monte Carlo Simulations of 3D Maxwell Equations With Applications in Microwave Interactions With Vegetation, IEEE Access, 5, 11985–12003, https://doi.org/10.1109/ACCESS.2017.2714620, 2017.
Huber, S., Villano, M., Younis, M., Krieger, G., Moreira, A., Grafmueller, B., and Wolters, R.: Tandem-L: Design Concepts for a Next-Generation Spaceborne SAR System, in: Proceedings of the EUSAR 2016: 11th European Conference on Synthetic Aperture Radar, 6–9 June 2016, Hamburg, Germany, 1–5, 2016.
Huntzinger, D., Schaefer, K., Schwalm, C., Fisher, J., Hayes, D., Stofferahn, E., Carey, J., Michalak, A., Wei, Y., Jain, A., Kolus, H., Mao, J., Poulter, B., Shi, X., Tang, J., and Tian, H.: Evaluation of simulated soil carbon dynamics in Arctic-Boreal ecosystems, Environ. Res. Lett., 15, 025005, https://doi.org/10.1088/1748-9326/ab6784, 2020.
IPCC (Intergovernmental Panel on Climate Change): Special Report on the Ocean and Cryosphere in a Changing Climate, edited by: Pörtner, H.-O. Roberts, D., Masson Delmotte, V., Zhai, P., Tignor, M., Poloczanska, E., Mintenbeck, K., Alegría, A., Nicolai, M., Okem, A., Petzold, J., Rama, B., and Weyer, N., Cambridge University Press, Cambridge, UK and New York, NY, USA, 755 pp., https://doi.org/10.1017/9781009157964, 2019.
Jackson, T. and Schmugge, T.: Vegetation effects on the microwave emission of soils, Remote Sens. Environ., 36, 203–212, https://doi.org/10.1016/0034-4257(91)90057-D, 1991.
Jarvis, P. and Linder, S.: Constraints to growth of boreal forests, Nature, 405, 904–905, https://doi.org/10.1038/35016154, 2000.
Jenson, J.: Remote sensing of the Environment: An Earth Resource Perspective, 2nd Edn., Pearson Prentice Hall, Upper Saddle River, New Jersey, United States, 656 pp., ISBN 978-1-29202-170-6, 2006.
Jiménez-Muñoz, G. and Sobrino, J.: Error sources on the land surface temperature retrieved from thermal infrared single channel remote sensing data, Int. J. Remote Sens., 27, 999–1014, https://doi.org/10.1080/01431160500075907, 2006.
Jones, L., Kimball, J., McDonald, K., Chan, S., Njoku, E., and Oechel, W.: Satellite microwave remote sensing of boreal and Arctic soil temperatures from AMSR-E, IEEE T. Geosci. Remote Sens., 45, 2004–2018, https://doi.org/10.1109/TGRS.2007.898436, 2007.
Jones, L., Kimball, J., Reichle, R., Madani, N., Glassy, J., Ardizzone, J., Colliander, A., Cleverly, J., Desai, A., Eamus, D., Euskirchen, E., Hutley, L., Macfarlane, C., and Scott, R.: The SMAP Level 4 Carbon Product for Monitoring Ecosystem Land–Atmosphere CO2 Exchange, IEEE T. Geosci. Remote Sens., 55, 6517–6532, https://doi.org/10.1109/TGRS.2017.2729343, 2017.
Jones, L. A., Ferguson, C. R., Kimball, J. S., Zhang, K., Chan, S. T. K., McDonald, K. C., Njoku, E. G., and Wood, E. F.: Satellite Microwave Remote Sensing of Daily Land Surface Air Temperature Minima and Maxima From AMSR-E, IEEE J. Sel. Top. Appl., 3, 111–123, https://doi.org/10.1109/jstars.2010.2041530, 2010.
Jones, M., Jones, L., Kimball, J., and McDonald, K.: Satellite passive microwave remote sensing for monitoring global land surface phenology, Remote Sens. Environ., 115, 1102–1114, https://doi.org/10.1016/j.rse.2010.12.015, 2011.
Kawanishi, T., Sezai, T., Ito, Y., Imaoka, K., Takashima, T., Ishido, Y., Shibata, A., Miura, M., Inahata, H., and Spencer, R.: The advanced scanning microwave radiometer for the EarthObserving System (AMSR-E): NASDA’s contribution to the EOS for global energy and water cycle studies, IEEE T. Geosci. Remote Sens., 41, 184–194, https://doi.org/10.1109/TGRS.2002.808331, 2003.
Kerr, Y., Waldteufel, P., Wigneron, J.-P., Delwart, S., Cabot, F., Boutin, J., Escorihuela, M., Font, J., Reul, N., Gruhier, C., and Juglea, S.: The SMOS mission: New tool for monitoring key elements of the global water cycle, IEEE T. Geosci. Remote, 98, 666–687, https://doi.org/10.1109/JPROC.2010.2043032, 2010.
Kerr, Y., Waldteufel, P., Richaume, P., Wigneron, J., Ferrazzoli, P., Mahmoodi, A., Al Bitar, A., Cabot, F., Gruhier, C., Juglea, S., Leroux, D., Mialon, A., and Delwart, S.: The SMOS soil moisture retrieval algorithm, IEEE T. Geosci. Remote. Sens., 50, 1384–1403, https://doi.org/10.1109/TGRS.2012.2184548, 2012.
Kilic, L., Prigent, C., Aires, F., Boutin, J., Heygster, G., Tonboe, R., Roquet, H., Jimenez, C., and Donlon, C.: Expected Performances of the Copernicus Imaging Microwave Radiometer (CIMR) for an All-Weather and High Spatial Resolution Estimation of Ocean and Sea Ice Parameters, J. Geophys. Res.-Oceans, 123, 7564–7580, https://doi.org/10.1029/2018JC014408, 2018.
Kim, S.-B., van Zyl, J., Johnson, J., Moghaddam, M., Tsang, L., Colliander, A., Dunbar, R., Jackson, T., Jaruwatanadilok, S., West, R., Berg, A., Caldwell, T., Cosh, M., Goodrich, D., Livingston, S., López-Baeza, E., Rowlandson, T., Thibeault, M., Walker, J., Entekhabi, D., Njoku, E., O'Neill, P., and Yueh, S.: Surface Soil Moisture Retrieval Using the L-Band Synthetic Aperture Radar Onboard the Soil Moisture Active–Passive Satellite and Evaluation at Core Validation Sites, IEEE T. Geosci. Remote Sens., 55, 1897–1914, https://doi.org/10.1109/TGRS.2016.2631126, 2017.
Kim, Y., Kimball, J., Zhang, K., and McDonald, K.: Satellite detection of increasing Northern Hemisphere non-frozen seasons from 1979 to 2008: Implications for regional vegetation growth, Remote Sens. Environ., 121, 472–487, https://doi.org/10.1016/j.rse.2012.02.014, 2012.
Kim, Y., Kimball, J., Xu, X., Dunbar, S., Colliander, A., and Derksen, C.: Global Assessment of the SMAP Freeze/Thaw Data Record and Regional Applications for Detecting Spring Onset and Frost Events, Remote Sens., 11, 1317, https://doi.org/10.3390/rs11111317, 2019.
Kimball, J., McDonald, K., Keyser, A. R., Frolking, S., and Running, S.: Application of the NASA Scatterometer (NSCAT) for determining the Daily Frozen and Nonfrozen Landscape of Alaska, Remote Sens. Environ., 75, 113–126, https://doi.org/10.1016/S0034-4257(00)00160-7, 2001.
Kimball, J., Zhao, M., McDonald, K., Heinsch, F. A., and Running, S.: Satellite observations of annual variability in terrestrial carbon cycles and seasonal growing seasons at high northern latitudes, Proc. Spie, Microwave Remote Sensing of the Atmosphere and Environment IV, 5654, https://doi.org/10.1117/12.578815, 2004a.
Kimball, J., McDonald, K., Running, S., and Frolking, S.: Satellite radar Remote sensingof seasonal growing seasons for boreal and subalpine evergreen forests, Remote Sens. Environ., 90, 243–258, https://doi.org/10.1016/j.rse.2004.01.002, 2004b.
Kimball, J., Jones, L., Zhang, K., Heinsch, F. A., McDonald, K., and Oechel, W.: A Satellite Approach to Estimate Land-Atmosphere CO2 Exchange for Boreal and Arctic Biomes Using MODIS and AMSR-E, IEEE T. Geosci. Remote Sens., 47, 569–587, https://doi.org/10.1109/TGRS.2008.2003248, 2009.
Kimball, J., Jones, L., Glassy, J., Stavros, N., Madani, N., Reichle, R., Jackson, T., and Colliander, A.: Soil Moisture Active Passive Mission L4_C Data Product Assessment (Version 2 Validated Release), MAO Office Note No. 13 (Version 1.0), NASA Goddard Space Flight Center, Greenbelt, Maryland, United States, 37 pp., https://gmao.gsfc.nasa.gov/pubs/docs/Kimball852.pdf (last access: 19 July 2023), 2017.
Köcher, P., Horna, V., and Leuschner, C.: Stem water storage in five coexisting temperate broad-leaved tree species: significance, temporal dynamics and dependence on tree functional traits, Tree Physiol., 33, 817–832, https://doi.org/10.1093/treephys/tpt055, 2013.
Kohn, J. and Royer, A.: AMSR-E data inversion for soil temperature estimation under snow cover, Remote Sens. Environ., 114, 2951–2961, https://doi.org/10.1016/j.rse.2010.08.002, 2010.
Konings, A., Piles, M., Das N., and Entekhabi, D.: L-band vegetation visible depth and effective scattering albedo estimation from SMAP, Remote Sens. Environ., 198, 460–470, https://doi.org/10.1016/j.rse.2017.06.037, 2017.
Konings, A., Rao, K., and Steele-Dunne, S.: Macro to micro: microwave Remote sensing of plant water content for physiology and ecology, New Phytol., 223, 1166–1172, https://doi.org/10.1111/nph.15808, 2019.
Krieger, G., Moreira, A., Zink, M., Hajnsek, I., Huber, S., Villano, M., Papathanassiou, K., Younis, M., Lopez Dekker, P., Pardini, M., Schulze, D., Bachmann, M., Borla Tridon, D., Reimann, J., Bräutigam, B., Steinbrecher, U., Tiendra, C., Sanjuan Ferrer, M., Zonno, M., Eineder, M., De Zan, F., Parizzi, A., Fritz, T., Diedrich, E., Maurer, E., Münzenmayer, R., Grafmüller, B., Wolters, R., te Hennepe, F., Ernst, R., and Bewick, C.: Tandem-L: Main results of the phase a feasibility study,” 2016 IEEE International Geoscience and Remote Sensing Symposium (IGARSS), 10–15 July 2016, Beijing, China, 2116–2119, https://doi.org/10.1109/IGARSS.2016.7729546, 2016.
Krishnan, P., Meyers, T., Hook, S., Heuer, M., Senn, D., and Dumas, E.: Intercomparison of In Situ Sensors for Ground-Based Land Surface Temperature Measurements, Sensors, 20, 5268, https://doi.org/10.3390/s20185268, 2020.
Lai, D.: Methane Dynamics in Northern Peatlands: A Review, Pedosphere, 19, 409–421, https://doi.org/10.1016/S1002-0160(09)00003-4, 2009.
Lakhankar, T., Krakauer, N., and Khanbilvardi, R.: Applications of microwave Remote sensing of soil moisture for agricultural applications, Int. J. Terraspace Sci. Eng., 2, 81–91, 2009.
Larue, F., Royer, A., De Sève, D., Langlois, A., Roy, A., and Brucker, L.: Validation of GlobSnow-2 snow water equivalent over Eastern Canada, Remote Sens. Environ., 194, 264–277, https://doi.org/10.1016/j.rse.2017.03.027, 2017.
Larue, F., Royer, A., De Sève, D., Roy, A., and Cosme, E.: Assimilation of passive microwave AMSR-2 satellite observations in a snowpack evolution model over northeastern Canada, Hydrol. Earth Syst. Sci., 22, 5711–5734, https://doi.org/10.5194/hess-22-5711-2018, 2018.
Lawrence, H., Wigneron, J.-P., Richaume, P., Novello, N., Grant, J., Mialon, A., Al Bitar, A., Merlin, O., Guyon, D., Leroux, D., Bircher, S., and Kerr, Y.: Comparison between SMOS Vegetation Visible Depth products and MODIS vegetation indices over crop zones of the USA, Remote Sens. Environ., 140, 396–406, https://doi.org/10.1016/j.rse.2013.07.021, 2014.
Leanza, A., Manzoni, M., Monti-Guarnieri, A., and di Clemente, M.: LEO to GEO-SAR Interferences: Modelling and performance evaluation, Remote Sens., 11, 1720, https://doi.org/10.3390/rs11141720, 2019.
Lee, J.-S., Grunes, M., and Pottier, E.: Quantitative comparison of classification capability: fully polarimetric versus dual and single-polarization SAR, IEEE T. Geosci. Remote Sens., 39, 2343–2351, https://doi.org/10.1109/36.964970, 2001.
Lees, K., Quaife, T., Artz, R., Khomik, M., and Clarl, J.: Potential for using remote sensing to estimate carbon fluxes across northern peatlands – A review. Sci. Total Environ., 615, 857–874, https://doi.org/10.1016/j.scitotenv.2017.09.103, 2018.
Le Toan, T., Quegan, S., Davidson, M., Balzter, H., Paillou, P., Papathanassiou, K., Plummer, S., Rocca, F., Saatchi, S., Shugart, H., and Ilander, L.: The BIOMASS mission: Mapping global forest biomass to better understand the terrestrial carbon cycle, Remote Sens. Environ., 115, 2850–2860, https://doi.org/10.1016/j.rse.2011.03.020, 2011.
Li, Q., Kelly, R., Leppanen, L., Vehvilainen, J., Kontu, A., Lemmetyinen, J., and Pulliainen, J.: The influence of thermal properties and canopy-intercepted snow on passive microwave transmissivity of a scots pine. IEEE T. Geosci. Remote Sens., 57, 5424–5433, https://doi.org/10.1109/TGRS.2019.2899345, 2019.
Li, W., Cardellach, E., Ribó, S., Oliveras, S., and Rius, A.: Exploration of Multi-Mission Spaceborne GNSS-R Raw IF Data Sets: Processing, Data Products and Potential Applications, Remote Sens., 14, 1344, https://doi.org/10.3390/rs14061344, 2022.
Li, X., Wigneron, J. P., Fan, L., Frappart, F., Simon, H., Colliander, A., Ebtehaj, A., Gao, L., Fernandez-Moran, R., Liu, X. Z., Wang, M. J., Ma, H. L., Moisy, C., and Ciais, P.: A new SMAP soil moisture and vegetation optical depth product (SMAP-IB): Algorithm, assessment and inter-comparison, Remote Sens. Environ., 271, 112921, https://doi.org/10.1016/j.rse.2022.112921, 2022.
Lieffers, V. and Rothwell, R.: Rooting of peatland black spruce and tamarack in relation to depth of water table, Can. J. Bot., 65, 817–821, https://doi.org/10.1139/b87-111, 1987.
Lievens, H., Demuzere, M., Marshall, H.-P., Reichle, R., Brucker, L., Brangers, I., de Rosnay, P., Dumont, M., Girotto, M., Immerzeel, W., Jonas, T., Kim, E., Koch, I., Marty, C., Saloranta, T., Schöber, J., and De Lannoy, G.: Snow depth variability in the Northern Hemisphere mountains observed from space, Nat. Commun., 10, 4629, https://doi.org/10.1038/s41467-019-12566-y, 2019.
Liljedahl, A., Boike, J., Daanen, R., Fedorov, A., Frost, G., Grosse, G., Hinzman, L., Iijma, Y., Jorgenson, J., Matveyeva, N., Necsoiu, M., Raynolds, M., Romanovsky, V., Schulla, J., Tape, K., Walker, D., Wilson, C., Yabuki, H., and Zona, D.: Pan-Arctic ice-wedge degradation in warming permafrost and its influence on tundra hydrology, Nat. Geosci., 9, 312–318, https://doi.org/10.1038/ngeo2674, 2016.
Liu, X., Wigneron, J.-P., Fan, L., Frappart, F., Ciais, P., Baghdadi, N., Zribi, M., Jaghuber, T., Li, X., Wang, M., Bai, X., and Moisy, C.: ASCAT IB: A radar-based vegetation optical depth retrieved from the ASCAT scatterometer satellite, Remote Sens. Environ., 264, 112587, https://doi.org/10.1016/j.rse.2021.112587, 2021.
Liu Y., van Dijk, A., de Jeu, R., Canadell, J., McCabe, M., Evans, J., and Wang, G.: Recent reversal in loss of global terrestrial biomass, Nat. Clim. Change, 5, 470–474, https://doi.org/10.1038/nclimate2581, 2011a.
Liu, Y. A., de Jeu, R. J., McCabe, M., Evans, J., and van Dijk, A.: Global long-term passive microwave satellite-based retrievals of vegetation visible depth, Geophys. Res. Lett., 38, L18402, https://doi.org/10.1029/2011GL048684, 2011b.
Liu, Y., Holtzman, N. M., and Konings, A. G.: Global ecosystem-scale plant hydraulic traits retrieved using model–data fusion, Hydrol. Earth Syst. Sci., 25, 2399–2417, https://doi.org/10.5194/hess-25-2399-2021, 2021.
Loisel, J., Gallego-Sala, A. V., Amesbury, M. J., Magnan, G., Anshari, G., Beilman, D. W., Benavides, J. C., Blewett, J., Camill, P., Charman, D. J., Chawchai, S., Hedgpeth, A., Kleinen, T., Korhola, A., Large, D., Mansilla, C. A., Müller, J., van Bellen, S., West, J. B., Yu, Z., Bubier, J. L., Garneau, M., Moore, T., Sannel, A. B. K., Page, S., Väliranta, M., Bechtold, M., Brovkin, V., Cole, L. E. S., Chanton, J. P., Christensen, T. R., Davies, M. A., De Vleeschouwer, F., Finkelstein, S. A., Frolking, S., Galka, M., Gandois, L., Girkin, N., Harris, L. I., Heinemeyer, A., Hoyt, A. M., Jones, M. C., Joos, F., Juutinen, S., Kaiser, K., Lacourse, T., Lamentowicz, M., Larmola, T., Leifeld, J., Lohila, A., Milner, A. M., Minkkinen, K., Moss, P., Naafs, B. D. A., Nichols, J., O'Donnel, J., Payne, R., Philben, M., Piilo, S., Quillet, A., Ratnayake, A. S., Roland, T. P., Sjögersten, S., Sonnentag, O., Swindles, G. T., Swinnen, W., Talbot, J., Treat, C., Valach, A. C., and Wu, J.: Expert assessment of future vulnerability of the global peatland carbon sink, Nat. Clim. Change, 11, 70–77, 2021.
Lönnqvist, A., Rauste, Y., Molinier, M., and Häme, T.: Polarimetric SAR Data in Land Cover Mapping in Boreal Zone, IEEE T. Geosci. Remote Sens., 48, 3652–3662, https://doi.org/10.1109/TGRS.2010.2048115, 2010.
Lorente, A., Borsdorff, T., Butz, A., Hasekamp, O., aan de Brugh, J., Schneider, A., Wu, L., Hase, F., Kivi, R., Wunch, D., Pollard, D. F., Shiomi, K., Deutscher, N. M., Velazco, V. A., Roehl, C. M., Wennberg, P. O., Warneke, T., and Landgraf, J.: Methane retrieved from TROPOMI: improvement of the data product and validation of the first 2 years of measurements, Atmos. Meas. Tech., 14, 665–684, https://doi.org/10.5194/amt-14-665-2021, 2021.
Luojus, K., Pulliainen, J., Takala, M., Lemmetyinen, J., Mortimer, C., Derksen, C., Mudryk, L., Moisander, M., Hiltunen, M., Smolander, T., Ikonen, J., Cohen, J., Salminen, M., Norberg, J., Veijola, K., and Venäläinen, P.: GlobSnow v3.0 Northern Hemisphere snow water equivalent dataset, Sci. Data, 8, 163, https://doi.org/10.1038/s41597-021-00939-2, 2021.
Maeda, T., Taniguchi, Y., and Imaoka, K.: GCOM-W1 AMSR2 Level 1R Product: Dataset of brightness temperature modified using the antenna pattern matching technique, IEEE T. Geosci. Remote Sens., 54, 770–782, https://doi.org/10.1109/TGRS.2015.2465170, 2016.
Magney, T., Bowling, D., Logan, B., Grossmann, K., Stutz, J., Blanken, P., Burns, S., Cheng, R., Garcia, M., Köhler, P., Lopez, S., Parazoo, N., Raczka, B., Schimel, D., and Frankenberg, C.: Mechanistic evidence for tracking the seasonality of photosynthesis with solar-induced fluorescence, P. Natl. Acad. Sci. USA, 116, 11640–11645, https://doi.org/10.1073/pnas.1900278116, 2019.
Mao, J., Ribes, A., Yan, B., Shi, X., Thornton, P., Séférian, R., Ciais, P., Myneni, R., Douville, H., Piao, S., Zhu, Z., Dickinson, R., Dai, Y., Ricciuto, D., Jin, M., Hoffman, F., Wang, B., Huang, M., and Lian, X.: Human-induced greening of the northern extratropical land surface, Nat. Clim. Change, 6, 959–963, https://doi.org/10.1038/nclimate3056, 2016.
Mao, K., Zuo, Z., Shen, X., Xu, T., Gao, C., and Liu, G.: Retrieval of land-surface temperature from AMSR2 data using a deep dynamic learning neural network, Chinese Geogr. Sci., 28, 1–11, https://doi.org/10.1007/s11769-018-0930-1, 2018.
Marchand, N., Royer, A., Krinner, G., Roy, A., Langlois, A., and Vargel, C.: Snow-covered soil temperature retrieval in Canadian Arctic permafrost areas, using a land surface scheme informed with satellite remote sensing data, Remote Sens., 10, 1703, https://doi.org/10.3390/rs10111703, 2018.
Marghany, M.: Principle theories of synthetic aperture radar. Synthetic aperture radar imaging mechanism for oil spills, 127–150, Gulf Professional Publishing, United States, 322 pp., ISBN 9780128181119, 2019.
Matheny, A., Bohrer, G., Garrity, S., Morin, T., Howard, C., and Vogel, C.: Observations of stem water storage in trees of opposing hydraulic strategies, Ecosphere, 6, 1–13, https://doi.org/10.1890/ES15-00170.1, 2015.
Matthews, E., Johnson, M. S., Genovese, V., Du, J., and Bastviken, D.: Methane emission from high latitude lakes: methane-centric lake classification and satellite-driven annual cycle of emissions, Sci. Rep., 10, 12465, https://doi.org/10.1038/s41598-020-68246-1, 2020.
McDonald, K., Kimball, J., Njoku, E., Zimmermann, R., and Zhao, M.: Variability in Springtime Thaw in the Terrestrial High Latitudes: Monitoring a Major Control on the Biospheric Assimilation of Atmospheric CO2 with Spaceborne Microwave Remote Sensing, Earth Interact., 8, 1–23, https://doi.org/10.1175/1087-3562(2004)8<1:VISTIT>2.0.CO;2, 2004.
McMahon, S., Parker, G., and Miller, D.: Evidence for a recent increase in forest growth, P. Natl. Acad. Sci. USA, 107, 3611–3615, https://doi.org/10.1073/pnas.0912376107, 2010.
Meloche, J., Langlois, A., Rutter, N., Royer, A., King, J., Walker, B., Marsh, P., and Wilcox, E. J.: Characterizing tundra snow sub-pixel variability to improve brightness temperature estimation in satellite SWE retrievals, The Cryosphere, 16, 87–101, https://doi.org/10.5194/tc-16-87-2022, 2022.
Melton, J. R., Arora, V. K., Wisernig-Cojoc, E., Seiler, C., Fortier, M., Chan, E., and Teckentrup, L.: CLASSIC v1.0: the open-source community successor to the Canadian Land Surface Scheme (CLASS) and the Canadian Terrestrial Ecosystem Model (CTEM) – Part 1: Model framework and site-level performance, Geosci. Model Dev., 13, 2825–2850, https://doi.org/10.5194/gmd-13-2825-2020, 2020.
Merchant, M., Adams, J., Berg, A., Baltzer, J., Quinton, W., and Chasmer, L.: Contributions of C-Band SAR data and polarimetric decompositions to subarctic boreal peatland mapping, IEEE J. Sel. Top. Appl., 10, 1467–1482, https://doi.org/10.1109/JSTARS.2016.2621043, 2017.
Merchant, M., Warren, R., Edwards, R., and Kenyon, J.: An object-based assessment of multi-wavelength SAR, optical imagery and topographical datasets for operational wetland mapping in boreal Yukon, Canada, Can. J. Remote Sens., 45, 308–332, https://doi.org/10.1080/07038992.2019.1605500, 2019.
Merchant, M., Obadia, M., Brisco, B., DeVries, B., and Berg, A.: Applying machine learning and time-series analysis on Sentinel-1A SAR/InSAR for characterizing arctic tundra hydro-ecological condition, Remote Sens., 14, 1123, https://doi.org/10.3390/rs14051123, 2022.
Merzouki, A., McNairn, H., and Pacheco, A.: Mapping soil moisture using RADARSAT-2 data and local autocorrelation statistics, IEEE J. Sel. Top. Appl., 4, 128–137, https://doi.org/10.1109/JSTARS.2011.2116769, 2011.
Mialon, A., Royer, A., Fily, M., and Picard, G.: Daily microwave-derived surface temperature over Canada/Alaska, J. Appl. Meteorol. Clim., 46, 591–604, https://doi.org/10.1175/JAM2485.1, 2007.
Mialon, A., Rodríguez-Fernández, N., Santoro, M., Saatchi, S., Mermoz, S., Bousquet, E., and Kerr, Y.: Evaluation of the sensitivity of SMOS L-VOD to forest above-ground biomass at global scale, Remote Sens., 12, 1450, https://doi.org/10.3390/rs12091450, 2020.
Mikan, C., Schimel, J., and Doyle, A.: Temperature controls of microbial respiration above and below freezing in Arctic tundra soils, Soil Biol. Biochem., 34, 1785–1795, https://doi.org/10.3390/rs12091450, 2002.
Miner, K. R., Turesky, M. R., Malina, E., Bartsch, A., Tamminen, J., McGuire, A. D., Fix, A., Sweeney, C., Elder, C. D., and Miller, C. E.: Permafrost carbon emissions in a changing Arctic, Nat. Rev. Earth Environ., 3, 55–67, https://doi.org/10.1038/s43017-021-00230-3, 2022.
Mironov, V. and Savin, I.: A temperature-dependent multi-relaxation spectroscopic dielectric model for thawed and frozen organic soil at 0.05–15 GHz, Phys. Chem. Earth, 83–84, 57–64, https://doi.org/10.1016/j.pce.2015.02.011, 2015.
Misra, T., Jha, A., Putrevu, D., Rao, J., Dave, D., and Rana, S.: Ground calibration of multifrequency ScanningMicrowave radiometer (MSMR), IEEE T. Geosci. Remote Sens., 40, 504–508, https://doi.org/10.1109/36.992823, 2002.
Mo, T., Choudhury, B., Schmugge, T., Wang, J., and Jackson, T.: A model for microwave emission from vegetation-covered fields, J. Geophys. Res., 87, 11229–11237, https://doi.org/10.1029/JC087iC13p11229, 1982.
Moreira, A., Bachmann, M., Balzer, W., Tridon, D., Diedrich, E., Fritz, T., Grigorov, C., Kahle, R., Krieger, G., Hajnsek, I., Huber, S., Jörg, H., Klenk, P., Lachaise, M., Maier, M., Maurer, E., Papathanassiou, K., Parizzi, A., Prats, P., Reimann, J., Rodriguez, M., Schättler, B., Schwinger, M., Schulze, D., Steinbrecher, U., Villano, M., Younis, M., De Zan, F., Zink, M., and Zonno, M.: Tandem-L: Project Status and Main Findings of the Phase Bl Study, IGARSS 2018 – 2018 IEEE International Geoscience and Remote sensing Symposium, 22–27 July 2018, Valencia, Spain, 8667–8670, https://doi.org/10.1109/IGARSS.2018.8518591, 2018.
Morrissey, L., Durden, S., Livingston, G., Steam, J., and Guild, L.: Differentiating methane source areas in Arctic environments with multitemporal ERS-1 SAR data, IEEE T. Geosci. Remote Sens., 34, 667–673, https://doi.org/10.1109/36.499746, 1996.
Mortimer, C., Mudryk, L., Derksen, C., Luojus, K., Brown, R., Kelly, R., and Tedesco, M.: Evaluation of long-term Northern Hemisphere snow water equivalent products, The Cryosphere, 14, 1579–1594, https://doi.org/10.5194/tc-14-1579-2020, 2020.
Mortin, J., Schrøder, T., Walløe Hansen, A., Holt, B., and McDonald, K.: Mapping of seasonal freeze-thaw transitions across the pan-Arctic land and sea ice domains with satellite radar, J. Geophys. Res.-Oceans, 117, C08004, https://doi.org/10.1029/2012JC008001, 2012.
Mu, Q., Zhao, M., Heinsch, F. A., Liu, M., Tian, H., and Running, S.: Evaluating water stress controls on primary production in biogeochemical and remote sensing based models, J. Geophys. Res.-Biogeo., 112, G01012, https://doi.org/10.1029/2006JG000179, 2007.
Murfitt, J. and Duguay, C.: 50 years of lake ice research from active microwave remote sensing: Progress and prospects, Remote Sens. Environ., 264, 112616, https://doi.org/10.1016/j.rse.2021.112616, 2021.
Myers-Smith, I. H., Forbes, B., Wilmking, M., Hallinger, M., Lantz, T., Blok, D., Tape, K., Macias-Fauria, M., Sass-Klaassen, U., Lévesque, E., Boudreau, S., Ropars, P., Hermanutz, L., Trant, A., Collier, L., Weijers, S., Rozema, J., Rayback, S., Schmidt, N., Schaepman-Strub, G., Wipf, S., Rixen, C., Ménard, C., Venn, S., Goetz, S., Andreu-Hayles, L., Elmondorf, S., Ravolainen, V., Welker, J., Grogan, P., Epstein, H., and Hik, D.: Shrub expansion in tundra ecosystems: dynamics, impacts and research priorities, Environ. Res. Lett., 6, 045509, https://doi.org/10.1088/1748-9326/6/4/045509, 2011.
Myers-Smith, I. H., Kerby, J., Phoenix, G., Bjerke, J., Epstein, H., Assmann, J., John, C., Andreu-Hayles, L., Angers-Blondin, S., Beck, P., Berner, L., Bhatt, U., Bjorkman, A., Blok, C., Bryn, A., Christiansen, C., Cornelissen, J. H. C., Cunliffe, A., Elmendorf, S., Forbes, B., Goetz, S., Hollister, R., de Jong, R., Loranty, M., Macias-Fauria, M., Maseyk, K., Normand, S., Olofsson, J., Parker, T., Parmentier, F.-J., Post. E., Schaepman-Strub, G., Stordal, F., Sullivan, P., Thomas, H., Tømmervik, H., Treharne, R., Tweedie, C., Walker, D., Wilmking, M., and Wipf, S.: Complexity revealed in the greening of the Arctic, Nat. Clim. Change, 10, 106–117, https://doi.org/10.1038/s41558-019-0688-1, 2020.
Naeimi, V., Scipal, K., Bartalis, Z., Hasenauer, S., and Wagner, W.: An Improved Soil Moisture Retrieval Algorithm for ERS and METOP Scatterometer Observations, IEEE T. Geosci. Remote Sens., 47, 1999–2013, https://doi.org/10.1109/TGRS.2008.2011617, 2009.
Naeimi, V., Paulik, C., Bartsch, A., Wagner, W., Kidd, R., Park, S.-E. Elger, K., and Boike, J.: ASCAT Surface State Flag (SSF): Extracting Information on Surface Freeze/Thaw Conditions From Backscatter Data Using an Empirical Threshold-Analysis Algorithm, IEEE T. Geosci. Remote Sens., 50, 2566–2582, https://doi.org/10.1109/TGRS.2011.2177667, 2012.
Nagler, T. and Rott, H.: Retrieval of wet snow by means of multitemporal SAR data, IEEE T. Geosci. Remote Sens., 38, 754–765, https://doi.org/10.1109/36.842004, 2000.
Natali, S.,Watts, J., Rogers, B., Potter, S., Ludwig, S., Selbmann, A.-K., Sullivan, P., Abbott, B., Arndt, K., Birch, L., Björkman, M., Bloom, A., Celis, G., Christensen, T., Christiansen, C., Commane, R., Cooper, E., Crill, P., Czimczik, C., Davydov, S., Du, J., Egan, J., Elberling, B., Euskirchen, E., Friborg, T., Genet, H., Göckede, M., Goodrich, J., Grogan, P., Helbig, M., Jafarov, E., Jastrow, J., Kalhori, A., Kim, Y., Kimball, J., Kutzbach, L., Lara, M., Larsen, K., Lee, B.-Y., Liu, Z., Loranty, M., Lund, M., Lupascu, M., Madani, N., Malhotra, A., Matamala, R., McFarland, J., McGuire, A., Michelsen, A., Minions, C., Oechel, W., Olefeldt, D., Parmentier, F.-J., Pirk, N., Poulter, B., Quinton, W., Rezanezhad, F., Risk, D., Sachs, T., Schaefer, K., Schmidt, N., Schuur, E., Semenchuk, P., Shaver, G., Sonnentag, O., Starr, G., Treat, C., Waldrop, M., Wang, Y., Welker, J., Wille, C., Xu, X., Zhang, Z., Zhuang, Q., and Zona, D.: Large loss of CO2 in winter observed across the northern permafrost region, Nat. Clim. Change, 9, 852–857, https://doi.org/10.1038/s41558-019-0592-8, 2019.
Neumann, M., Saatchi, S., Ulander, L., and Fransson, J.: Assessing performance of L- and P-Band polarimetric interferometric SAR data in estimating boreal forest above-ground biomass, IEEE T. Geosci. Remote, 50, 714–726, https://doi.org/10.1109/TGRS.2011.2176133, 2012.
Osińska-Skotak, K.: Studies of soil temperature on the basis of satellite data, Int. Agrophys., 21, 275–284, 2007.
Pallandt, M. M. T. A., Kumar, J., Mauritz, M., Schuur, E. A. G., Virkkala, A.-M., Celis, G., Hoffman, F. M., and Göckede, M.: Representativeness assessment of the pan-Arctic eddy covariance site network and optimized future enhancements, Biogeosciences, 19, 559–583, https://doi.org/10.5194/bg-19-559-2022, 2022.
Pan, Y., Birdsey, R., Fang, J., Houghton, R., Kauppi, P., Kurz, W., Phillips, O., Shvidenko, A., Lewis, S., Canadell, J., Ciais, P., Jackson, R., Pacala, S., McGuire, A., Piao, S., Rautiainen, A., Sitch, S., and Hayes, D.: A large and persistent carbon sink in the world's forests, Science, 333, 988–993, https://doi.org/10.1126/science.1201609, 2011.
Pan, Y., Birdsey, R., Phillips, O., and Jackson, R.: The structure, distribution, and biomass of the world's forests, Annu. Rev. Ecol. Syst., 44, 593–622, https://doi.org/10.1146/annurev-ecolsys-110512-135914, 2013.
Panikov, N., Flanagan, P., Oechel, W., Mastepanov, M., and Christensen, T.: Microbial activity in soils frozen to below −39 ∘C, Soil Biol. Biochem., 38, 785–794, https://doi.org/10.1016/j.soilbio.2005.07.004, 2006.
Pappas, C., Maillet, J., Rakowski, S., Baltzer, J., Barr, A., Black, A., Fatichi, S., Laroque, C., Matheny, A., Roy, A., Sonnentag, O., and Zha, T.: Aboveground tree growth is a minor and decoupled fraction of boreal forest carbon input, Agr. Forest Meteorol., 290, 108030, https://doi.org/10.1016/j.agrformet.2020.108030, 2020.
Parinussa, R., Holmes, T., and de Jeu, R.: Soil moisture retrievals from the WindSat spaceborne polarimetric microwave radiometer, IEEE T. Geosci. Remote Sens., 50, 2683–2694, https://doi.org/10.1109/TGRS.2011.2174643, 2012.
Pastorello, G., Trotta, C., Canfora, E., et al.: The FLUXNET2015 dataset and the ONEFlux processing pipeline for eddy covariance data, Sci. Data, 7, 225, https://doi.org/10.1038/s41597-020-0534-3, 2020.
Peng, C., Ma, Z., Lei, X., Zhu, Q., Chen, H., Wang, W., Liu, S., Li, W., Fang, X., and Zhou, X.: A drought-induced pervasive increase in tree mortality across Canada's boreal forests, Nat. Clim. Change, 1, 467–471, https://doi.org/10.1038/nclimate1293, 2011.
Piao, S., Ciais, P., Friedlingstein, P., Peylin, P., Reichstein, M., Luyssaert, S., Margolis, H., Fang, J., Barr, A., Chen, A., Grelle, A., Hollinger, D., Laurila, T., Lindroth, A., Richardson, A., and Vesala, T.: Net carbon dioxide losses of northern ecosystems in response to autumn warming, Nature, 451, 49–52, https://doi.org/10.1038/nature06444, 2008.
Picard, G., Sandells, M., and Löwe, H.: SMRT: an active–passive microwave radiative transfer model for snow with multiple microstructure and scattering formulations (v1.0), Geosci. Model Dev., 11, 2763–2788, https://doi.org/10.5194/gmd-11-2763-2018, 2018.
Pierce, L., Ulaby, F., Sarabandi, K., and Dobson, M.: Knowledge-based classification of polarimetric SAR images, IEEE T. Geosci. Remote Sens., 31, 1081–1086, https://doi.org/10.1109/36.312896, 1994.
Pierdicca, N., Davidson, M., Chini, M., Dierking, W., Djavidnia, S., Haarpaintner, J., Hajduch, G., Laurin, G., Lavalle, M., López-Martínez, C., Nagler, T., and Su, B.: The Copernicus L-band SAR mission ROSE-L (Radar Observing System for Europe, Proc. Spie, Microwave Remote sensing for Environmental Monitoring III, 111540E, https://doi.org/10.1117/12.2534743, 2019.
Pierrat, Z., Nehemy, M. F., Roy, A., Magney, T., Parazoo, N., Laroque, C., Pappas, C., Sonnentang, O., Grossman, K., Bowling, D. R., Seibt, U., Ramirez, A., Johnson, B., Helgason, W., Barr, A., and Stutz, J.: Tower-based Remote sensing reveals mechanisms behind a two-phased spring transition in a mixed species boreal forest, J. Geophys. Res.-Biogeo., 126, e2020JG006191, https://doi.org/10.1029/2020JG006191, 2021.
Potapov, P., Hansen, M., Stehman, S., Loveland, T., and Pittman, K.: Combining MODIS and Landsat imagery to estimate and map boreal forest cover loss, Remote Sens. Environ., 112, 3708–3719, https://doi.org/10.1016/j.rse.2008.05.006, 2008.
Prince, M., Roy, A., Brucker, L., Royer, A., Kim, Y., and Zhao, T.: Northern Hemisphere surface freeze–thaw product from Aquarius L-band radiometers, Earth Syst. Sci. Data, 10, 2055–2067, https://doi.org/10.5194/essd-10-2055-2018, 2018.
Prince, M., Roy, A., Royer, A., and Langlois, A.: Timing and spatial variability of fall soil freezing in boreal forest and its effect on SMAP L-band radiometer measurements, Remote Sens. Environ., 231, 111230, https://doi.org/10.1016/j.rse.2019.111230, 2019.
Pulliainen, J., Grandell, J., and Hallikainen, M.: Retrieval of surface temperature in boreal forest zone from SSM/I data, IEEE T. Geosci. Remote Sens., 35, 1188–1200, https://doi.org/10.1109/36.628786, 1997.
Pulliainen, J.: Mapping of snow water equivalent and snow depth in boreal and sub-arctic zones by assimilating space-borne microwave radiometer data and ground-based observations, Remote Sens. Environ., 101, 257–269, https://doi.org/10.1016/j.rse.2006.01.002, 2006.
Pulliainen, J., Aurela, M., Laurila, T., Aalto, T., Takala, M., Salminen, M., Kulmala, M., Barr, A., Heimann, M., Lindroth, A., Laaksonen, A., Derksen, C., Mäkelä, A., Markkanen, T., Lemmetyinen, J., Susiluoto, J., Dengel, S., Mammarella, I., Tuovinen, J.-P., and Vesala, T.: Early snowmelt significantly enhances boreal springtime carbon uptake, P. Natl. Acad. Sci. USA, 114, 11081–11086, https://doi.org/10.1073/pnas.1707889114, 2017.
Pulliainen, J., Luojus, K., Derksen, C., Mudryk, L., Lemmetyinen, J., Salminen, M., Ikonen, J., Takala, M., Cohen, J., Smolander, T., and Norberg, J.: Patterns and trends of Northern Hemisphere snow mass from 1980 to 2018, Nature, 581, 294–298, https://doi.org/10.1038/s41586-020-2258-0, 2020.
Quegan, S., Le Toan, T., Chave, J., Dall, J., Exbrayat, J.-F., Minh, D., Lomas, M., Mariotti D'Alessandro, M. , Paillou, P., Papathanassiou, K., Rocca, F., Saatchi, S., Scipal, K., Shugart, H., Smallman, L., Soja, M., Tebaldini, S., Ulander, L., Vllard, L., and Williams, M.: The European Space Agency BIOMASS mission: Measuring forest above-ground biomass from space, Remote Sens. Environ., 227, 44–60, https://doi.org/10.1016/j.rse.2019.03.032, 2019.
Rafat, A., Rezanezhad, F., Quinton, W. L., Humphreys, E. R., Webster, K., and Van Cappellen, P.: Non-growing season carbon emissions in a northern peatland are projected to increase under global warming, Commun. Earth Environ., 2, 111, https://doi.org/10.1038/s43247-021-00184-w, 2021.
Ranson, K. and Sun, G.: Effects of environmental conditions on boreal forest classification and biomass estimates with SAR, IEEE T. Geosci. Remote Sens., 38, 1242–1252, https://doi.org/10.1109/36.843016, 2000.
Rantanen, M., Karpechko, A. Y., Lipponen, A., Nordling, K., Hyvärinen, O., Ruosteenoja, K., Vihma, T., and Laaksonen, A.: The Arctic has warmed nearly four times faster than the globe since 1979, Commun. Earth Environ., 3, 168, https://doi.org/10.1038/s43247-022-00498-3, 2022.
Rautiainen, K., Lemmetyinen, J., Pulliainen, J., Vehviläinen, J., Drusch, M., Kontu, A., Kainulainen, J., and Seppanen, J.: L-band radiometer observations of soil processes at boreal and sub- Arctic environments, IEEE T. Geosci. Remote Sens., 50, 1483–1497, https://doi.org/10.1109/TGRS.2011.2167755, 2012.
Rautiainen, K., Parkkinen, T., Lemmetyinen, J., Schwank, M., Wiesmann, A., Ikonen, J., Derksen, C., Davydov, S., Davydova, A., Boike, J., and Langer, M.: SMOS prototype algorithm for detecting autumn soil freezing, Remote Sens. Environ., 180, 346–360, https://doi.org/10.1016/j.rse.2016.01.012, 2016.
Rautiainen, K., Comite, D., Cohen, J., Cardellach, E., Unwin, M., and Pierdicca, N.: Freeze–Thaw Detection Over High-Latitude Regions by Means of GNSS-R Data, IEEE T. Geosci. Remote Sens., 60, 4302713, https://doi.org/10.1109/TGRS.2021.3125315, 2022.
Ravn, N., Elberling, B., and Michelsen, A.: Arctic soil carbon turnover controlled by experimental snow addition, summer warming and shrub removal, Soil Biol. Biochem., 142, 107698, https://doi.org/10.1016/j.soilbio.2019.107698, 2020.
Rodríguez-Fernández, N. J., Mialon, A., Mermoz, S., Bouvet, A., Richaume, P., Al Bitar, A., Al-Yaari, A., Brandt, M., Kaminski, T., Le Toan, T., Kerr, Y. H., and Wigneron, J.-P.: An evaluation of SMOS L-band vegetation optical depth (L-VOD) data sets: high sensitivity of L-VOD to above-ground biomass in Africa, Biogeosciences, 15, 4627–4645, https://doi.org/10.5194/bg-15-4627-2018, 2018.
Rodríguez-Fernández, N., Al Bitar, A., Colliander, A., and Zhao, T.: Soil moisture remote sensing across scales, Remote Sens., 11, 190, https://doi.org/10.3390/rs11020190, 2019a.
Rodríguez-Fernández, N., Mialon, A., Merlin, O., Suere, C., Cabot, F., Khazaal, A., Costeraste, J., Palacin, B., Rodriguez-Suquet, R., Tournier, T., Decoopman, T., Colom, M., Morel, J.-M., and Kerr, Y.: SMOS-HR: A high resolution L-Band passive radiometer for earth science and applications, IGARSS 2019 – 2019 IEEE International Geoscience and Remote sensing Symposium, 28 July–2 August 2019, Yokohama, Japan, 8392–8395, https://doi.org/10.1109/IGARSS.2019.8897815, 2019b.
Rogers, M., Sullivan, P., and Welker, J.: Evidence of nonlinearity in the response of net ecosystem CO2 exchange to increasing levels of winter snow depth in the high Arctic of Northwest Greenland, Arct. Antarct. Alp. Res., 43, 95–106, https://doi.org/10.1657/1938-4246-43.1.95, 2010.
Rosen, P., Hensley, S., Shaffer, S., Veilleux, L., Chakraborty, M., Misra, T., Bhan, R., Sagi, R., and Satish, R.: The NASA-ISRO SAR mission – An international space partnership for science and societal benefit, 2015 IEEE Radar Conference (RadarCon), 10–15 May 2015, Arlington, United States, 1610–1613, https://doi.org/10.1109/RADAR.2015.7131255, 2015.
Rosen, P., Hensley, S., Shaffer, S., Edelstein, W., Kim, Y., Kumar, R., Misra, T., Bhan, R., Satish, R., and Sagi, R.: An update on the NASA-ISRO dual-frequency DBF SAR (NISAR) mission, 2016 IEEE International Geoscience and Remote sensing Symposium (IGARSS), 10–15 July 2016, Beijing, China, 2106–2108, https://doi.org/10.1109/IGARSS.2016.7729543, 2016.
Roy, A., Royer, A., Wigneron, J.-P., Langlois, A., Bergeron, J., and Cliche, P.: A simple parameterization for a boreal forest radiative transfer model at microwave frequencies, Remote Sens. Environ., 124, 371–383, https://doi.org/10.1016/j.rse.2012.05.020, 2012.
Roy, A., Royer, A., and Hall, R.: Relationship between forest microwave transmissivity and structural parameters for the Canadian boreal forest, IEEE Geosci. Remote Sens., 11, 1802–1806, https://doi.org/10.1109/LGRS.2014.2309941, 2014.
Roy, A., Royer, A., Derksen, C., Brucker, L., Langlois, A., Mialon, A., and Kerr, Y.: Evaluation of spaceborne L-Band radiometer measurements for terrestrial freeze/thaw retrievals in Canada, IEEE J. Sel. Top. Appl., 8, 4442–4459, https://doi.org/10.1109/JSTARS.2015.2476358, 2015.
Roy, A., Toose, P., Williamson, M., Rowlandson, T., Derksen, C., Royer, A., Berg, A., Lemmetyinen, J., and Arnold, L.: Response of L-Band brightness temperatures to freeze/thaw and snow dynamics in a prairie environment from ground-based radiometer measurements, Remote Sens. Environ., 191, 67–80, https://doi.org/10.1016/j.rse.2017.01.017, 2017a.
Roy, A., Toose, P., Derksen, C., Rowlandson, T., Berg, A., Lemmetyinen, J., Royer, A., Tetlock, E., Helgason, W., and Sonnentag, O.: Spatial Variability of L-Band Brightness Temperature during Freeze/Thaw Events over a Prairie Environment, Remote Sens., 9, 894, https://doi.org/10.3390/rs9090894, 2017b.
Roy, A., Toose, P., Mavrovic, A., Pappas, C., Royer, C., Derksen, C., Berg, A., Rowlandson, T., El-Amine, M., Barr, A., Black, A., Langlois, A., and Sonnentag, O.: L-Band response to freeze/thaw in a boreal forest stand from ground- and tower-based radiometer observations, Remote Sens. Environ., 237, 111542, https://doi.org/10.1016/j.rse.2019.111542, 2020.
Royer, A. and Poirier, S.: Surface temperature spatial and temporal variations in North America from homogenized satellite SMMR-SSM/I microwave measurements and reanalysis for 1979–2008, J. Geophys. Res., 115, D08110, https://doi.org/10.1029/2009JD012760, 2010.
Royer, A., Roy, A., Jutras, S., and Langlois, A.: Review article: Performance assessment of radiation-based field sensors for monitoring the water equivalent of snow cover (SWE), Cryosphere, 15, 5079–5098, https://doi.org/10.5194/tc-15-5079-2021, 2021.
Ruiz-Pérez, G. and Vico, G.: Effects of Temperature and Water Availability on Northern European Boreal Forests, Front. For. Glob. Change, 3, 34, https://doi.org/10.3389/ffgc.2020.00034, 2020.
Saatchi, S. and Rignot, E.: Classification of boreal forest cover types using SAR images, Remote Sens. Environ., 60, 270–281, https://doi.org/10.1016/S0034-4257(96)00181-2, 1997.
Saberi, N., Kelly, R., Flemming, M., and Li, Q.: Review of snow water equivalent retrieval methods using spaceborne passive microwave radiometry, Int. J. Remote Sens., 41, 996–1018, https://doi.org/10.1080/01431161.2019.1654144, 2020.
Santoro, M. and Cartus, O.: Research pathways of forest above-ground biomass estimation based on SAR backscatter and interferometric SAR observations, Remote Sens., 10, 608, https://doi.org/10.3390/rs10040608, 2018.
Santoro, M., Cartus, O., Mermoz, S., Bouvet, A., Le Toan, T., Carvalhais, N., Rozendaal, D., Herold, M., Avitabile, V., Shaun, Q., Carreiras, J., Rauste, Y., Balzter, H., Schmullius, C., and Seifert, F.: A detailed portrait of the forest aboveground biomass pool for the year 2010 obtained from multiple Remote sensing observations, Geophys. Res. Abstr., EGU2018-18932, EGU General Assembly 2018, Vienna, Austria, 2018.
Schär, C., Fuhrer, O., Arteaga, A., Ban, N., Charpilloz, C., Di Girolamo, S., Hentgen, L., Hoefler, T., Lapillonne, X., Leutwyler, D., Osterried, K., Panosetti, D., Rüdisühli, S., Schlemmer, L., Schulthess, T., Sprenger, M., Ubbiali, S., and Wernli, H.: Kilometer-scale climate models: prospects and challenges, B. Am. Meteorol. Soc., 101, E567–E587, https://doi.org/10.1175/BAMS-D-18-0167.1, 2020.
Schädel, C., Bader, M., Schuur, E., Biasi, C., Bracho, R., Čapek, P., De Baets, S., Diáková, K., Ernakovich, J., Estop-Aragones, C., Graham, D., Hartley, I., Iversen, C., Kane, E., Knoblauch, C., Lupascu, M., Martikainen, P., Natali, S., Norby, R., O'Donnell, J., Chowdhury, T., Šantrůčková, H., Shaver, G., Sloan, V., Treat, C., Turetsky, M., Waldrop, M., and Wickland, K.: Potential carbon emissions dominated by carbon dioxide from thawed permafrost soils, Nat. Clim. Change, 6, 950–953, https://doi.org/10.1038/nclimate3054, 2016.
Schlund, M., Scipal, K., and Quegan, S.: Assessment of a power law relationship between P-band SAR backscatter and aboveground biomass and its implications for BIOMASS mission performance, IEEE J. Sel. Top. Appl., 11, 3538–3547, https://doi.org/10.1109/JSTARS.2018.2866868, 2018.
Schuur, E., McGuire, A., Schädel, C., Grosse, G., Harden, J., Hayes, D., Hugelius, G., Koven, C., Kuhry, P., Lawrence, D., Natali, S., Olefeldt, D., Romanovsky, V., Schaefer, K., Turetsky, M., Treat, C., and Vonk, J.: Climate change and the permafrost carbon feedback, Nature, 520, 171–179, https://doi.org/10.1038/nature14338, 2015.
Seiler, C., Melton, J., Arora, V., Sitch, S., Friedlingstein, P., Anthoni, P., Goll, D., Jain, A., Joetzjer, E., Lienert, S., Lombardozzi, D., Luyssaert, S., Nabel, J., Tian, H., Vuichard, N., Walker, A., Yuan, W., and Zaehle, S.: Are terrestrial biosphere models fit for simulating the global land carbon sink?, J. Adv. Model Earth Sy., 14, e2021MS002946, https://doi.org/10.1029/2021MS002946, 2022.
Shi, J., Xiong, C., and Jiang, L.: Review of snow water equivalent microwave remote Sensing, Sci. China Earth Sci., 59, 731–745, https://doi.org/10.1007/s11430-015-5225-0, 2016.
Sitch, S., McGuire, D., Kimball, J., Gedney, N., Gamon, J., Engstrom, R., Wolf, A., Zhuang, Q., Clein, J., and McDonald, K.: Assessing the carbon balance of circumpolar Arctic tundra using Remote sensing and process modelling, Ecol. Appl., 17, 213–234, https://doi.org/10.1890/1051-0761(2007)017[0213:ATCBOC]2.0.CO;2, 2007.
Sniderhan, A., Mamet, S., and Baltzer, J.: Non-uniform growth dynamics of a dominant boreal tree species (Picea mariana) in the face of rapid climate change, Can. J. Forest Res., 51, 565–572, https://doi.org/10.1139/cjfr-2020-0188, 2021.
Stefan, V.-G., Indrio, G., Escorihuela, M.-J., Quintana-Sehuì, P., and Villar, J., M.: High-resolution SMAP-derived root-zone soil moisture using an exponential filter model calibrated per land cover type, Remote Sens., 13, 1112, https://doi.org/10.3390/rs13061112, 2021.
Stocker, B., Zscheischler, J., Keenan, T., Prentice, C., Peñuelas, J., and Seneviratne, S.: Quantifying soil moisture impacts on light use efficiency across biomes, New Phytol., 218, 1430–1449, https://doi.org/10.1111/nph.15123, 2018.
Sturm, M., Holmgren, J., König, M., and Morris, K.: The thermal conductivity of seasonal snow, J. Glaciol., 43, 26–41, https://doi.org/10.3189/s0022143000002781, 1997.
Sturm, M., Schimel, J., Michaelson, G., Welker, J., Oberbauer, S., Liston, G., Fahnestock, J., and Romanovsky, V.: Winter biological processes could help convert arctic tundra to shrubland, Bioscience, 55, 17–26, https://doi.org/10.1641/0006-3568(2005)055[0017:WBPCHC]2.0.CO;2, 2005.
Sulla-Menashe, D., Woodcock, C., and Friedl, M.: Canadian boreal forest greening and browning trends: an analysis of biogeographic patterns and the relative roles of disturbance versus climate drivers, Environ. Res. Lett., 13, 014007, https://doi.org/10.1088/1748-9326/aa9b88, 2018.
Takala, M., Luojus, K., Pulliainen, J., Derksen, C., Lemmetyinen, J., Kärnä, J.-P., Koskinen, J., and Bojkov, B.: Estimating northern hemisphere snow water equivalent for climate research through assimilation of space-borne radiometer data and ground-based measurements, Remote Sens. Environ., 115, 3517–3529, https://doi.org/10.1016/j.rse.2011.08.014, 2011.
Tanja, S., Berninger, F., Vesala, T., Markkanen, T., Hari, P., Mäkelä, A., Ilvesniemi, H., Hänninen, H., Nikinmaa, E., Huttula, T., Laurila, T., Aurela, M., Grelle, A., Lindroth, A., Arneth, A., Shibistova, O., and Lloyd, J.: Air temperature triggers the commencement of evergreen boreal forest photosynthesis in spring, Glob. Change Biol., 9, 1410–1426, https://doi.org/10.1046/j.1365-2486.2003.00597.x, 2003.
Tarnocai, C., Canadell, J., Schuur, E., Kuhry, P., Mazhitova, G., and Zimov, S.: Soil organic carbon pools in the northern circumpolar permafrost region, Gobal Biogeochem. Cy., 23, GB2023, https://doi.org/10.1029/2008GB003327, 2009.
Tebaldini, S., Ho Tong Minh, D., Mariotti d'Alessandro, M., Villard, L., Le Toan, T., and Chave, J.: The status of technologies to measure forest biomass and structural properties: state of the art in SAR tomography of tropical forests, Surv. Geophys., 40, 779–801, https://doi.org/10.1007/s10712-019-09539-7, 2019.
Tedesco, M. and Jeyaratnam, J.: A new operational snow retrieval algorithm applied to historical AMSR-E brightness temperatures, Remote Sens., 8, 1037, https://doi.org/10.3390/rs8121037, 2016.
Tei, S. and Sugimoto, A.: Excessive positive response of model-simulated land net primary production to climate changes over circumboreal forests, Plant-Environment Interactions, 1, 102–121, https://doi.org/10.1002/pei3.10025, 2020.
Tenkanen, M., Tsuruta, A., Rautiainen, K., Kangasaho, V., Ellul, R., and Aalto, T.: Utilizing earth observations of soil freeze/thaw data and atmospheric concentrations to estimate cold season methane emissions in the Northern high latitudes, Remote Sens., 13, 5059, https://doi.org/10.3390/rs13245059, 2021.
Teubner, I., Forkel, M., Jung, M., Liu, Y., Miralles, D., Parinussa, R., van der Schalie, R., Vreugdenhil, M., Schwalm, C., Tramontana, G., Camps-Valls, G., and Drigo, W.: Assessing the relationship between microwave vegetation visible depth and gross primary production, Int. J. Appl. Earth Obs., 65, 79–91, https://doi.org/10.1016/j.jag.2017.10.006, 2018.
Teubner, I., Forkel, M., Camps-Valls, G., Jung, M., Miralles, D., Tramontana, G., van der Schalie, R., Vreugdenhil, Mösinger, L., and Dorigo, W.: A carbon sink-driven approach to estimate gross primary production from microwave satellite observations, Remote Sens. Environ., 229, 100–113, https://doi.org/10.1016/j.rse.2019.04.022, 2019.
Tian, F., Brandt, M., Liu, Y., Verger, A., Tagesson, T., Diouf, A., Rasmussen, K., Mbow, C., Wang, Y., and Fensholt, R.: Remote sensing of vegetation dynamics in drylands: Evaluating vegetation visible depth (VOD) using AVHRR NDVI and in situ green biomass data over West African Sahel, Remote Sens. Environ., 177, 265–276, https://doi.org/10.1016/j.rse.2016.02.056, 2016.
Tomiyasu, K.: Tutorial Review of Synthetic-Aperture Radar (SAR) with Applications to Imaging of Ocean Surface, P. IEEE, 66, 563–583, https://doi.org/10.1109/PROC.1978.10961, 1978.
Touati, C., Ratsimbazafy, T., Ludwig, R., and Bernier, M.: New approaches for removing the effect of water damping on SMAP freeze/thaw mapping, Can. J. Remote Sens., 45, 405–422, https://doi.org/10.1080/07038992.2019.1638236, 2019.
Töyrä, J., Pietroniro, A., and Martz, L.: Multisensor hydrologic assessment of a freshwater wetland, Remote Sens. Environ., 75, 162–173, https://doi.org/10.1016/s0034-4257(00)00164-4, 2001.
Tu, Q., Hase, F., Blumenstock, T., Kivi, R., Heikkinen, P., Sha, M. K., Raffalski, U., Landgraf, J., Lorente, A., Borsdorff, T., Chen, H., Dietrich, F., and Chen, J.: Intercomparison of atmospheric CO2 and CH4 abundances on regional scales in boreal areas using Copernicus Atmosphere Monitoring Service (CAMS) analysis, COllaborative Carbon Column Observing Network (COCCON) spectrometers, and Sentinel-5 Precursor satellite observations, Atmos. Meas. Tech., 13, 4751–4771, https://doi.org/10.5194/amt-13-4751-2020, 2020.
Tucker, C. J.: Red and photographic infrared linear combinations for monitoring vegetation, Remote Sens. Environ., 8, 127–150, https://doi.org/10.1016/0034-4257(79)90013-0, 1979.
Turner, D., Ollinger, S., and Kimball, J.: Integrating remote sensing and ecosystem process models for landscape- to regional-scale analysis of the carbon cycle, Bioscience, 54, 573–584, https://doi.org/10.1641/0006-3568(2004)054[0573:IRSAEP]2.0.CO;2, 2004.
Ulaby, F., Moore, R., and Fung, A.: Microwave Remote Sens.-Basel: Active and Passive, Vol. II – Radar remote sensing and surface scattering and emission theory, Addison-Wesley Publishing Company, Advanced Book Program/World Science Division, Norwood, Massachusetts, United-States, ISBN 9780201107609, 1982.
Ulaby, F., Allen, C., and Fung, A.: Method for Retrieving the True Backscattering Coefficient from Measurements with a Real Antenna, IEEE T. Geosci. Remote Sens., GE-21, 308–313, https://doi.org/10.1109/TGRS.1983.350558, 1983.
Ulaby, F., Moore, R., and Fung, A.: Microwave Remote Sens.: Active and Passive. Vol. III. From theory to applications, Artech House Publishers, Norwood, Massachusetts, United-States, https://doi.org/10.1017/S0016756800015831, 1986.
Ulaby, F., Sarabandi, K., McDonald, K., Whitt, M., and Dobson, M. C.: Michigan microwave canopy scattering model, Int. J. Remote Sens., 11, 1223–1253, https://doi.org/10.1080/01431169008955090, 1990.
Ullmann, T., Schmitt, A., Roth, A., Duffe, J., Dech, S., Hubberten, H.-W., and Baumhauer, R.: Land cover characterization and classification of arctic tundra environments by means of polarized synthetic aperture X- and C-Band radar (PolSAR) and Landsat 8 multispectral imagery – Richards Island, Canada, Remote Sens., 6, 8565–8593, https://doi.org/10.3390/rs6098565, 2014.
van Huissteden, J. and Dolman, A.: Soil carbon in the Arctic and the permafrost carbon feedback, Curr. Opin. Env. Sust., 4, 545–551, https://doi.org/10.1016/j.cosust.2012.09.008, 2012.
Virkkala, A.-M., Aalto, J., Rogers, B., Tagesson, T., Treat, C., Natali, S., Watts, J., Potter, S., Lehtonen, A., Mauritz, M., Schuur, E., Kochendorfer, J., Zona, D., Oechel, W., Kobayashi, H., Humphreys, E., Goeckede, M., Iwata, H., Lafleur, P., Euskirchen, E., Bokhorst, S., Marushchak, M., Martikainen, P., Elberling, B., Voigt, C., Biasi, C., Sonnentag, O., Parmentier, F.-J., Ueyama, M., Celis, G., St.Louis, V., Emmerton, C., Peichl, M., Chi, J., Järveoja, J., Nilsson, M., Oberbauer, S., Torn, M., Park, S.-J., Dolman, H., Mammarella, I., Chae, N., Poyatos, R., López-Blanco, E., Christensen, T., Kwon, M., Sachs, T., Holl, D., and Luoto, M.: Statistical upscaling of ecosystem CO2 fluxes across the terrestrial tundra and boreal domain: Regional patterns and uncertainties, Glob. Change Biol., 27, 4040–4059, https://doi.org/10.1111/gcb.15659, 2021.
Vittucci, C., Vaglio Laurin, G., Tramontana, G., Ferrazzoli, P., Guerriero, L., and Papale, D.: Vegetation visible depth at L-band and above ground biomass in the tropical range: Evaluating their relationships at continental and regional scales, Int. J. Appl. Earth Obs., 77, 151–161, https://doi.org/10.1016/j.jag.2019.01.006, 2019.
Wagner, W., Hahn, S., Kidd, R., Melzer, T., Bartalis, Z., Hasenauer, S., Figa-Saldaña, J., de Rosnay, P., Jann, A., Schneider, S., Komma, J., Kubu, G., Brugger, K., Aubrecht, C., Züger, J., Gangkofner, U., Kienberger, S., Brocca, L., Wang, Y., Blöschl, G., Eitzinger, J., and Steinnocher, K.: The ASCAT soil moisture product: A review of its specifications, validation results, and emerging applications, Meteorol. Z., 22, 5–33, https://doi.org/10.1127/0941-2948/2013/0399, 2013.
Walker, X. and Johnstone, J.: Widespread negative correlations between black spruce growth and temperature across topographic moisture gradients in the boreal forest, Environ. Res. Lett., 9, 064016, https://doi.org/10.1088/1748-9326/9/6/064016, 2014.
Walker, X., Rogers, B., Veraverbeke, S., Johnstone, J., Baltzer, J., Barrett, K., Bourgeau-Chavez, L., Day, N., de Groot, W., Dieleman, C., Goetz, S., Hoy, E., Jenkins, L., Kane, E., Parisien, M.-A., Potter, S., Schuur, E., Turetsky, M., Whitman, E., and Mack, M.: Fuel availability not fire weather controls boreal wildfire severity and carbon emissions, Nat. Clim. Change, 10, 1130–1136, https://doi.org/10.1038/s41558-020-00920-8, 2020.
Wang, J., Sulla-Menashe, D., Woodcock, C., Sonnentag, O., Keeling, R., and Friedl, M.: Extensive land cover change across Arctic-Boreal Northwestern North America from disturbance and climate forcing, Glob. Change Biol., 26, 807–822, https://doi.org/10.1111/gcb.14804, 2019.
Wang, J., Sulla-Menashe, D., Woodcock, C., Sonnentag, O., Keeling, R., and Friedl, M.: Extensive land cover change across Arctic–Boreal Northwestern North America from disturbance and climate forcing, Glob. Change Biol., 26, 807–822, https://doi.org/10.1111/gcb.14804, 2020.
Washington, W., Buja, L., and Craig, A.: The computational future for climate and Earth system models: on the path to petaflop and beyond, Philos. T. R. Soc. A., 367, 833–846, https://doi.org/10.1098/rsta.2008.0219, 2009.
Watts, J., Kimball, J., Bartsch, A., and McDonald, K.: Surface water inundation in the boreal-Arctic: potential impacts on regional methane emissions, Environ. Res. Lett., 9, 075001, https://doi.org/10.1088/1748-9326/9/7/075001, 2014.
Webb, E., Schuur, E., Natali, S., Oken, K., Bracho, R., Krapek, J., Risk, D., and Nickerson, N.: Increased wintertime CO2 loss as a result of sustained tundra warming, J. Geophys. Res.-Biogeo., 121, 249–265, https://doi.org/10.1002/2014JG002795, 2016.
Welker, J., Fahnestock, J., and Jones, M.: Annual CO2 flux in dry and moist Arctic tundra: field responses to increases in summer temperatures and winter snow depth, Climatic Change, 44, 139–150, https://doi.org/10.1023/A:1005555012742, 2000.
Whitcomb, J., Moghaddam, M., McDonald, K., Kellndorfer, J., and Podest, E.: Mapping vegetated wetlands of Alaska using L-band radar satellite imagery, Can. J. Remote Sens., 35, 54–72, https://doi.org/10.5589/m08-080, 2009.
Wigneron, J.-P., Kerr, Y., Waldteufel, P., Saleh, K., Escorihuela, M.-J., Richaume, P., Ferrazzoli, P., de Rosnay, P., Gurneye, R., Calvet, J.-C., Grant, J., Guglielmetti, M., Hornbuckle, B., Mätzler, C., Pellarin, T., and Schwank, M.: L-band Microwave Emission of the Biosphere (L-MEB) Model: Description and calibration against experimental data sets over crop fields, Remote Sens. Environ., 107, 639–655, https://doi.org/10.1016/j.rse.2006.10.014, 2007.
Wigneron, J.-P., Li, X., Frappart, F., Fan, L., Al-Yaari, A., De Lannoy, G., Liu, X., Wang, M., Le Masson, E., and Moisy, C.: Overview of the SMOS-IC data recordofsoil moisture and L-VOD: Historic development, applications and perspectives, Remote Sens. Environ., 254, 112238, https://doi.org/10.1016/j.rse.2020.112238, 2021.
Wohlfahrt, G., Gerdel, K., Migliavacca, M., Rotenberg, E., Tatarinov, F., Müller, J., Hammerle, A., Julitta, T., Spielmann, F., and Yakir, D.: Sun-induced fluorescence and gross primary productivity during a heat wave, Sci. Rep.-UK, 8, 14169, https://doi.org/10.1038/s41598-018-32602-z, 2018.
Wu, M., Scholze, M., Kaminski, T., Voßbeck, M., and Tagesson, T.: Using SMOS soil moisture data combining CO2 flask samples to constrain carbon fluxes during 2010–2015 within a Carbon Cycle Data Assimilation System (CCDAS), Remote Sens. Environ., 240, 111719, https://doi.org/10.1016/j.rse.2020.111719, 2020.
Xian, D., Zhang, P., Gao, L., Sun, R., Zhang, H., and Jia, X.: Fengyun Meteorological Satellite Products for Earth System Science Applications, Adv. Atmos. Sci., 38, 1267–1284, https://doi.org/10.1007/s00376-021-0425-3, 2021.
Xiao, J., Chevallier, F., Gomez, C., Guanter, L., Hicke, J., Huete, A., Ichii, K., Nih, W., Pang, Y., Rahman, A., Sun, G., Yuan, W., Zhang, L., and Zhang, X.: Remote sensing of the terrestrial carbon cycle: A review of advances over 50 years, Remote Sens. Environ., 233, 111383, https://doi.org/10.1016/j.rse.2019.111383, 2019.
Xu, X., Derksen, C., Yueh, S. Dunbar, R., and Colliander, A.: Freeze/thaw detection and validation using Aquarius' L-Band backscattering data, IEEE J. Sel. Top. Appl., 9, 1370–1381, https://doi.org/10.1109/JSTARS.2016.2519347, 2016.
Yang, W., Meng, H., Ferraro, R., Moradi, I., and Devaraj, C.: Cross-Scan asymmetry of AMSU-A window channels: characterization, correction, and verification, IEEE T. Geosci. Remote, 51, 1514–1530, https://doi.org/10.1109/TGRS.2012.2211884, 2013.
Yi, Y., Kimball, J., Jones, L., Reichle, R., Nemani, R., and Margolis, H.: Recent climate and fire disturbance impacts on boreal and arctic ecosystem productivity estimated using a satellite-based terrestrial carbon flux model, J. Geophys. Res.-Biogeo., 118, 606–622, https://doi.org/10.1002/jgrg.20053, 2013.
Yi, Y., Chen, R., Kimball, J., Moghaddam, M., Xu, X., Euskirchen, E., Das, N., and Miller, C.: Potential satellite monitoring of surface organic soil properties in arctic tundra from SMAP, Water Resour. Res., 58, e2021WR030957, https://doi.org/10.1029/2021WR030957, 2022.
Yu, K., Han, S., Bu, J., An, Y., Zhou, Z., Wang, C., Tabibi, S., and Cheong, J. W.: Spaceborne GNSS Reflectometry, Remote Sens., 14, 1605, https://doi.org/10.3390/rs14071605, 2022.
Zhang, Q. and Cheng, J.: An empirical algorithm for retrieving land surface temperature from AMSR-E data considering the comprehensive effects of environmental variables, Earth Space Sci., 7, e2019EA001006, https://doi.org/10.1029/2019EA001006, 2020.
Zhang, Y. Song, C., Sun, G., Band, L., Noormets, A. and Zhang, Q.: Understanding moisture stress on light use efficiency across terrestrial ecosystems based on global flux and remote-sensing data, J. Geophys. Res.-Biogeo., 120, 2053–2066, https://doi.org/10.1002/2015JG003023, 2015.
Zhou, Z., Li, Z., Waldron, S., and Tanaka, A.: InSAR time series analysis of L-Band data for understanding tropical peatland degradation and restoration, Remote Sens., 11, 2592, https://doi.org/10.3390/rs11212592, 2019.
Zona, D., Gioli, B., Commane, R., and Oechel, W. C.: Cold season emissions dominate the Arctic tundra methane budget, P. Natl. Acad. Sci. USA, 113, 40–45, https://doi.org/10.1073/pnas.1516017113, 2015.
van Zyl, J.: Unsupervised classification of scattering behavior using radar polarimetry data, IEEE T. Geosci. Remote Sens., 27, 36–45, https://doi.org/10.1109/36.20273, 1989.
- Abstract
- Introduction
- Principles of microwave remote sensing
- Microwave remote sensing for retrieval of surface variables
- Assimilating microwave data in terrestrial biosphere models in Arctic–boreal regions
- Challenges and opportunities in microwave remote sensing for supporting carbon cycle science
- Conclusions
- Appendix A
- Appendix B: Acronyms and abbreviations
- Data availability
- Author contributions
- Competing interests
- Disclaimer
- Acknowledgements
- Financial support
- Review statement
- References
- Abstract
- Introduction
- Principles of microwave remote sensing
- Microwave remote sensing for retrieval of surface variables
- Assimilating microwave data in terrestrial biosphere models in Arctic–boreal regions
- Challenges and opportunities in microwave remote sensing for supporting carbon cycle science
- Conclusions
- Appendix A
- Appendix B: Acronyms and abbreviations
- Data availability
- Author contributions
- Competing interests
- Disclaimer
- Acknowledgements
- Financial support
- Review statement
- References