the Creative Commons Attribution 4.0 License.
the Creative Commons Attribution 4.0 License.
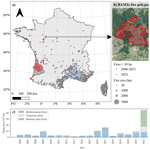
High-resolution data reveal a surge of biomass loss from temperate and Atlantic pine forests, contextualizing the 2022 fire season distinctiveness in France
Martin Schwartz
Philippe Ciais
Dave van Wees
Aurelien de Truchis
Florent Mouillot
The frequency and intensity of summer droughts and heat waves in Western Europe have been increasing, raising concerns about the emergence of fire hazard in less fire-prone areas. This exposure of old-growth forests hosting unadapted tree species may cause disproportionately large biomass losses compared to those observed in frequently burned Mediterranean ecosystems. Therefore, analyzing fire seasons from the perspective of exposed burned areas alone is insufficient; we must also consider impacts on biomass loss. In this study, we focus on the exceptional 2022 summer fire season in France and use very high-resolution (10 m) satellite data to calculate the burned area, tree height at the national level, and subsequent ecological impact based on biomass loss during fires. Our high-resolution semi-automated detection estimated 42 520 ha of burned area, compared to the 66 393 ha estimated by the European automated remote sensing detection system (EFFIS), including 48 330 ha actually occurring in forests. We show that Mediterranean forests had a lower biomass loss than in previous years, whereas there was a drastic increase in burned area and biomass loss over the Atlantic pine forests and temperate forests. High biomass losses in the Atlantic pine forests were driven by the large burned area (28 600 ha in 2022 vs. 494 ha yr−1 in 2006–2021 period) but mitigated by a low exposed tree biomass mostly located on intensive management areas. Conversely, biomass loss in temperate forests was abnormally high due to both a 15-fold increase in burned area compared to previous years (3300 ha in 2022 vs. 216 ha in the 2006–2021 period) and a high tree biomass of the forests which burned. Overall, the biomass loss (i.e., wood biomass dry weight) was 0.25 Mt in Mediterranean forests and shrublands, 1.74 Mt in the Atlantic pine forest, and 0.57 Mt in temperate forests, amounting to a total loss of 2.553 Mt, equivalent to a 17 % increase of the average natural mortality of all French forests, as reported by the national inventory. A comparison of biomass loss between our estimates and global biomass/burned areas data indicates that higher resolution improves the identification of small fire patches, reduces the commission errors with a more accurate delineation of the perimeter of each fire, and increases the biomass affected. This study paves the way for the development of low-latency, high-accuracy assessment of biomass losses and fire patch contours to deliver a more informative impact-based characterization of each fire year.
- Article
(12793 KB) - Full-text XML
- BibTeX
- EndNote
Wildfires are a recurrent disturbance across Europe, with an average annual burned area of 475 000 ha since 1980 (European Commission. Joint Research Center, 2022). This represents on average of 0.05 % of wildland area each year, with the majority (95 %) occurring in Mediterranean ecosystems which experience drier and warmer summers. In contrast, temperate forests in Europe are less prone to fires due to their humid and mild climate conditions and, consequently, receiving less attention by the fire science community (Zin et al., 2022). However, the 2022 fire season in Europe was particularly out of the norm compared to previous decades, characterized by a severe drought and heat waves leading to numerous extreme fire events and widespread burned areas throughout the western part of the continent (Rodrigues et al., 2023). For instance, in Spain, the burned area in 2022 was estimated to be over 300 000 ha compared to an average of 64 000 ha yr−1 over the 2008–2021 period according to the European Forest Fire Information System (EFFIS). The impacts on local populations and firefighting capacities were significant, drawing media attention. While the way society perceives these fires and their economic impacts on infrastructure and populations are crucial, it is also necessary to accurately evaluate their immediate ecological impacts to provide valuable information to societies and stakeholders. This evaluation could reveal unexplored aspects, potentially challenging the characterization of distinctiveness granted to extreme fire seasons as in 2022 solely based on burned areas, which may overstate or oversimplify wildfire issues “to garner attention in a competitive media ecosystem” (Jones et al., 2022).
In 2022, France has indeed experienced an unusual surge in wildfires during the summer, reaching a burned area of 66 393 ha according to automated remote sensing estimates from EFFIS when considering all fire types. This represents a significant increase from the average of 10 900 ha yr−1 recorded over the 2008–2021 period by the same source. Notably, the burned areas shifted to regions outside the traditionally most fire-prone Mediterranean part of the country. The French forests can actually be divided into three main regions based on their historical fire regimes (Barbero et al., 2019): (i) Mediterranean forests and shrublands in the southeast, frequently exposed to fires; (ii) Atlantic maritime pine forests in the southwest, affected by infrequent but large fires in recent decades, representing an epicenter of large fires in the middle of the 20th Century; and (iii) the rest of the French territory that is predominantly agricultural and hosts temperate forests with varying management intensities. Although this part of France is typically much less affected by fires due to its wetter climate and high landscape fragmentation, several fire events occurred during the summer of 2022, raising concerns about their environmental consequences.
In recent decades, studies that qualify disturbance factors like fire have increasingly accounted for the ecological impact. Rather than solely focusing on the extent of the disturbance, a more scientifically based holistic vulnerability framework, which combines the concepts of ecological loss and resilience, is employed (Forzieri et al., 2021; Arrogante-Funes et al., 2022; Chuvieco et al., 2023). The vulnerability of an ecosystem, in conjunction with its recovery rate, is highly dependent on the loss experienced by the system during the disturbance, assessed by its pre-fire state. As forest ecosystems contribute to several ecosystem services (Ninan and Inoue, 2014; Mori et al., 2017), including regulating (carbon sequestration), provisioning (timber and non-timber products), cultural (recreational, aesthetic), and supporting (decomposition, nutrient cycling) purposes, defining a pre-fire state is topic specific. Tree aboveground biomass is a crucial spatial variable used to evaluate the impact of fires and the resilience of ecosystem services to fires (Díaz et al., 2018; Martínez-Batlle, 2022; Powell et al., 2014; Sirin et al., 2021; Tyukavina et al., 2022; Volkova and Weston, 2015; Wu et al., 2020), as it serves as a proxy for wood resources and habitat for wildlife and biodiversity (Fusco et al., 2021; Basile et al., 2021; Cazzolla Gatti et al., 2017).
The accurate estimation of the aboveground biomass loss (AGB-L) by fires is challenging due to its spatiotemporal variability, which requires high-resolution data on burned areas and the corresponding spatially varying biomass within each fire patch. These two pieces of information are not yet available in an operational near-real-time impact assessment tool, although recently initiated for Amazonia by Andela et al. (2022). However, they could constitute keystone knowledge for an accurate comparison of the AGB lost across forest regions and fire events. For instance, a large fire affecting a low biomass plantation may have less impact than a small fire burning an old-growth forest. The challenge is therefore to combine data on the location of burned areas and the impacted forest biomass. Until recently, combining coarse estimates of burned area with the mean forest biomass of a region was the standard method to assess AGB-L when remote sensing information was not available (Chiriacò et al., 2013; Leenhouts, 1998). Statistical distribution (Prichard et al., 2019) or spatial interpolations (Keith et al., 2014) of biomass using plot data from forest inventories could bring an improved description of the spatial variation of biomass losses but still lack precise locations to be crossed with the actual burned area location. Integrative models of fire emissions combining burned area datasets, land cover, seasonal ecosystem functioning, and a simulation of the biomass carbon pools affected by fires have been applied globally at 0.25∘ resolution in the GFED model (Randerson et al., 2017). A finer resolution of 500 m was recently achieved with this method and constitutes key information for global studies (van Wees et al., 2022). However, this resolution is still too coarse to capture small fires in Europe. Recent advances in satellite imagery now allow for finer resolution in the burned area detection, down to 10 m with Sentinel-2 (Roteta et al., 2019). In parallel, a high-resolution description of forest height and biomass can be obtained from refined land cover and new space-borne lidar observations of tree height and canopy structure, as pioneered, e.g., in new global maps of forest height at a 30 m resolution (Potapov et al., 2021a). Combining very high-resolution and high-accuracy maps of burned area and biomass thus opens new perspectives for assessing AGB-L over large areas. High-resolution 20 m burned area detection using the Sentinel-2 MSI (MultiSpectral Instrument) sensor already demonstrated an 80 % increase in the area burned in Africa compared to the 500 m MODIS sensors, but such data remain to be combined with high-resolution biomass data to assess AGB-L (Ramo et al., 2021).
Hence, we propose in this study to combine a high-resolution exhaustive dataset of fire contours from remote sensing and a new map of tree height at a high resolution (10 m) converted to biomass using local forest inventory plot data to assess the biomass loss in French forests during the fire seasons of 2020 to 2022. We discuss the uncertainties of our approach and assess the benefits of high-resolution burned area and high-resolution biomass maps compared to existing approaches obtained at coarser resolution. By adopting a multifaceted approach that includes a detailed description of the fire season's distinctiveness, we will revisit the conclusions drawn from the 2022 fire season in France.
2.1 Study area
In this study, we employed the study of Barbero et al. (2019) and the classification of the French national forest inventory (NFI) to categorize France into three major regions (Fig. 1).
-
The Mediterranean forest and shrubland region (sylvoecoregions J and K of the NFI; IFN, 2023) encompasses the southeast portion of the country and surrounds the Mediterranean basin. This region is composed of low, dense forests that are dominated by Quercus species (Q. ilex, Q. pubescens, Q. suber) and Pinus halepensis. These species show strategies of resistance (cork for Q. suber) and tolerance (resprouting for Q. ilex and serotiny for P. halepensis) to cope with the frequent fire regime that occurs in this region. In addition, sclerophyllous non-forest vegetation, called maquis and garrigue, is widely distributed and predominantly affected by fire disturbances (Mouillot et al., 2003).
-
The Atlantic maritime pine forest region (sylvoecoregion F21 and F22 of the NFI; IFN, 2023) is almost exclusively composed of intensively managed forests for timber production. The cultivation of P. pinaster (maritime pine) has a rotation time of 20 to 30 years, resulting in a landscape characterized by a mosaic of plots at different growth levels (Petucco and Andrés-Domenech, 2018; Salas-Gonzalez et al., 2001). This region is less frequently affected by fire than the Mediterranean region, but fires can spread over large areas and lead to dramatic fire events in the past (Papy, 1950). Despite the serotinous fire tolerance of maritime pines (i.e., prolonged canopy storage of seeds protected in cones retained on the plant), forest management practices tend to favor replanting after a patch is affected by fire (Lamont et al., 2020).
-
The temperate forest region corresponds to the rest of the French territory. This zone features a diversity of forest communities dominated by deciduous and/or coniferous trees and ranging from no to intensive management. Being weakly affected by fires and due to the absence of a common evolutionary history to this type of disturbance, tree species show a lack of adapted strategy. Agricultural, pastoral, and other herbaceous vegetation areas comprise a significant portion of this region and can be susceptible to prescribed spring fires, such as stubble burning, particularly in the Pyrenean mountains area. Nevertheless, this study will not consider prescribed fires that primarily impact understory or non-forest lands and that are largely determined by local decisions rather than climate.
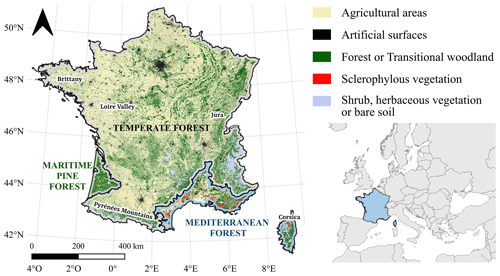
Figure 1Forest and vegetation cover in France from Corine Land Cover 2018 (SDES et al., 2019), with delimited major forest regions used in the study (Mediterranean forest, maritime pine forest, and temperate forest). Locations discussed in the following sections (Brittany, Loire Valley, Jura, and Pyrénées Mountains) are specified in black.
2.2 Fire polygons – BAMTs method
Due to the lack of a reliable and available fire contour dataset over the country, we developed our own fire polygon dataset with BAMTs (Burned Area Mapping Tools), a semi-automated method of fire contouring at high resolution (Bastarrika et al., 2014; Roteta et al., 2021). We processed atmospherically corrected and orthorectified images from the L2A product of ESA's Sentinel-2 mission between 2020 and 2022. The BAMTs method involves the calculation of three spectral indices: Normalized Differential Vegetation Index (NDVI) (Rouse et al., 1974), Normalized Burn Ratio (NBR) (Key and Benson, 1999), and NBR2 (García and Caselles, 1991). Each fire was first spatially and temporally located using NASA's Fire Information for Resource Management System (FIRMS) or national registration from the French Base de Données des Incendies de Forêt en France BDIFF official fire registration (BDIFF, 2023) to target a BAMTs processing zone. Subsequently, we defined the date of burning to determine a pre- and post-burn period, which enabled us to represent the pre-/post-differences in the three indices on an RGB color scale composite. The date of the fire is provided by the first hotspot detected by FIRMS. The pre-fire period thus runs from 1 January of the year of interest to the fire date, and the post-fire period lasts from the fire date to a user-defined final date. The final date can vary from a few weeks to several months after the fire, to guarantee a sufficient number of satellite images without cloud cover.
From the visual observation of the pre-/post-fire composite difference, the user manually draws a burned and unburned training zone further used in a random forest classifier (Belgiu and Drăguţ, 2016), based on 500 trees and unlimited maximum tree depth. This supervised classification was used to detect changes in the NDVI, NBR, and NBR2 composites. Finally, each produced fire polygon was visually evaluated and manually tuned until the desired visual accuracy was achieved. This process allowed us to remove commission errors (e.g., erroneous fire polygons being detected in agricultural lands) and to reprocess, if needed, for better capturing omitted burned areas by enlarging the training zones. We decided to capture the low-/mid-severity fires affecting the reflectance composite but still allowing unburned islands to remain classified as “unburned”. Understory fires are not captured, as they do not affect the upper canopy reflectances. BAMTs finally generates a burned area probability map, and the shapefile of the fire polygon is generated from pixels having a burn probability >50 %. A visual inspection follows this semi-supervised procedure to confirm that the detected perimeters were indeed burned areas. This key step is hardly provided by automated methods and helps to reach the international standard recommended by the CEOS (Committee for Earth Observation Satellites) Working Group on Calibration and Validation of remote sensing datasets (Franquesa et al., 2020). By focusing on forest fires larger than 30 ha, a total of 113 fire polygons were obtained over the 2020–2022 period of analysis (Fig. 2 shows three examples of a BAMTs fire polygons). We selected only fire polygons that dominantly occurred in forests and shrublands, in turn removing fires occurring on pastures and grasslands, and thus matching the French forest fire database BDIFF.
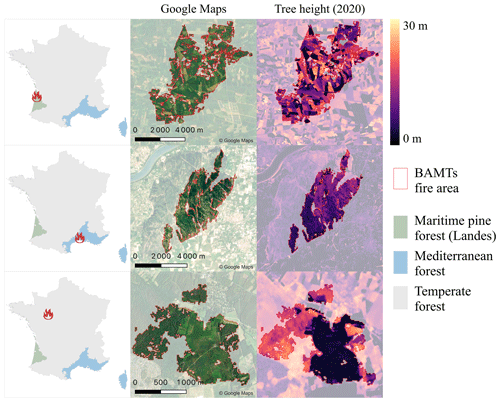
Figure 2Three examples of height prediction within fire polygons in Atlantic Maritime Pine forests (green), Mediterranean forests (blue), and temperate forests (gray). The fire locations are indicated by the red fire icon in the first column. The second column shows Google Maps imagery and the BAMTs fire polygons perimeter in red. The third column shows the 10 m canopy height, predicted with the method of Schwartz et al. (2022). Brighter colors indicate higher heights.
2.3 Tree height
To obtain canopy height within each fire patch, we followed the methodology described in Schwartz et al. (2022, 2023) that yielded accurate results (MAE = 2.67 m when compared to in situ forest inventory measurements) over the Les Landes maritime pine forests in France. The method to map tree height at 10 m resolution in 2020 combines optical (Sentinel-2), SAR (Sentinel-1), and spaceborne lidar data (Global Ecosystem Dynamics Investigation (GEDI)) with deep learning methods (U-Net model). Sentinel-1 (S1) is a C-band Synthetic Aperture Radar (SAR) mission launched in 2014 by the European Space Agency (ESA). Here, we used the Ground Range Detected (GRD) scenes with dual-band cross-polarization (vertical–vertical plus vertical–horizontal bands at 10 m resolution) preprocessed in Google Earth Engine. We computed a single median composite image of France based on all S1 images from the leaf-on season (1 May to 1 October 2020) separated into ascending and descending orbits, thus creating a single composite image of France with four layers: VV_ascending, VH_ascending, VV_descending, and VH_descending at 10 m resolution. The Sentinel-2 (S2) mission provides multispectral images from the Earth's surface reflectance with a revisit interval of ∼5 d. Here, we used 10 bands of the L2A product (bottom of the atmosphere reflectance) resampled at 10 m when necessary (nearest neighbors method): B2 – blue, B3– green, B4 – red, B5-B6-B7 – red edge, B8 – near infrared (NIR), B8 A – ”narrow” NIR, B11-B12 – short-wave infrared (SWIR). Similarly to S1, we computed the median of all S2 images with less than 50 % of clouds for the same time period after applying QA60 cloud mask.
The Global Ecosystem Dynamics Investigation (GEDI) mission is a spaceborne infrared lidar on the International Space Station (ISS). It provides energy return waveforms (L1B product) and derived metrics such as canopy relative height (RH) (L2A product) that describe the vertical forest structure within 25 m diameter circular footprints. In this case, we used RH95 height metrics as the reference height in order to train our model. We downloaded all GEDI footprints available for France since the beginning of the GEDI mission and filtered them using the quality flag provided by NASA. U-Net is a fully convolutional network (FCN) widely used in deep learning for image segmentation tasks. Here, we adapted this model for pixel-wise height regression, with GEDI data as the reference height and S1/S2 images as predictors. With a series of linear operations (convolutions) and non-linear “activation” functions, these types of models can learn multiscale image features, such as image texture, that are then used to carry out height predictions. More specifically, U-Nets (Ronneberger et al., 2015) are divided into a contracting path (left) and an expansive path (right) which gives it its “U” shape and enables the model to extract relevant information at different spatial scales. In total, the network has 18 convolutional layers and ∼17 million trainable weights.
We trained the model following the method described in Schwartz et al. (2022) and obtained a canopy height map for 2020 in France at 10 m resolution (See Fig. 2). Considering that this map has been designed only on the data of the year 2020, we evaluate it to be reliable for the 2020–2022 period only, particularly in the intensively managed Landes region (Petucco and Andrés-Domenech, 2018), covered by the fast-growing P. pinaster species (Serrano-León et al., 2021). Schwartz's innovative high-resolution tree height map is the basis for our method of estimating forest aboveground biomass in the remainder of this study. Note that due to GEDI RH95 properties, the heights below ∼3 m cannot be separated from bare soil or crops.
2.4 Aboveground biomass loss (AGB-L)
The workflow in Fig. 3 describes how the aboveground biomass losses (AGB-L) were computed for each fire patch using the 10 m resolution tree height map of Schwartz et al. (2022), the fire polygons obtained with BAMTs, and the French NFI plot data. AGB-L is defined as all direct and indirect potential biomass losses due to fire. These potential losses then include all the biomass exposed to fire, leading to either the combustion of the plant material during the fire, resulting in the release of gases and aerosols, or the formation of standing and ground dead wood, which is then decomposed or harvested by forest managers. This definition refers to the concept of potential loss used in fire risk assessments (Chuvieco et al., 2023). The calculation of AGB-L at 10 m resolution within each fire patch is performed according to the following successive steps (Fig. 3).
-
Step 1: tree height within each fire patch. We cropped the tree height map at 10 m resolution from Schwartz et al. (2022) within the BAMTs fire polygons, keeping only the 10 m pixels with height values higher than 3 m.
-
Step 2: NFI plots around the fire patch. Data collected since 2005 on more than 100 000 plots were used. The morphological characterization of more than 1.7 million trees allowed the establishment of allometric relationships for each species. For each fire patch, the NFI plots located at a maximum distance of 5 km outside the fire perimeter were selected. This buffer made it possible to rely on a sufficient number of plots ranging from a minimum of 5 plots to 278 (median = 41).
-
Step 3: DBH (diameter at breast height) ∼ height allometry. We established an allometric relationship between the diameter at breast height (DBHNFI) and the tree height (HNFI) from NFI measurements for each tree of the dominant species in each plot (Eq. 1). The parameter p is species dependent and affects the relationship between height and diameter for the dominant species considered. We computed p for each fire patch based on all surrounding NFI plots.
-
Step 4: DBH map. We applied Eq. (2) to the 10 m×10 m resolution tree height values from Schwartz et al. (2022) (Hx) to compute the DBH of the highest tree for each 10 m pixel (DBHx).
-
Step 5: height ∼ biomass allometry. To estimate the aboveground biomass of the highest tree in each pixel (AGBx) from its DBH, we used allometric relationships from the R package allodb (Gonzalez-Akre et al., 2022) for each species. This tool compares the allometric relations of different studies and builds a new relationship according to the taxonomic and geographical information provided. By considering only the dominant species, we obtained an estimation of the AGB of the highest tree within each 10 m resolution pixel (AGBtx) as the result of the allometry function fp relative to the fire patch, applied to the values of each pixel (DBHx).
-
Step 6: tree density estimation. To obtain the aboveground biomass over the whole 10 m×10 m pixel (AGBfx) we need the stand density D which is not provided by remote sensing. We assumed that most of the forests that burned were closed forests so that the aboveground biomass of a tree is influenced by the density according to the “self-thinning” rule commonly used in forestry (Eq. 4) (Yoda, 1963; Puntieri, 1993). Equation (4) relates the forest density at the pixel level (Dx) to the biomass of an average tree at the pixel level (AGBtx).
In Eq. (4), the parameter kp has a specific value for each fire patch and was estimated by fitting the equation to all the trees in NFI plots surrounding each fire patch (see Appendix A for more details). Finally, we obtained the aboveground biomass of a forest pixel (AGBfx) by multiplying the density Dx of the pixel by the biomass of the highest tree of this pixel (AGBtx) as shown in Eq. (5). We assume here that our high-resolution 10 m dataset highly limits tree height variability within the pixel so that the difference between the average tree height and maximum tree height is below the uncertainty of the maximum tree height (2.75 m) extracted from Schwartz et al. (2022).
which led to Eq. (6) expressing the forest biomass of a 10 m×10 m pixel as a function of the pixel height (Hx) and other fire-patch related parameters (αp and kp) (Chan et al., 2021).
-
Step 7: correction for complex topography. The GEDI-based method tends to overestimate values in areas with complex topography, especially in mountain regions. The slope of each pixel of a fire patch was calculated based on a digital terrain model (NASA/METI/AIST/Japan Space Systems and US/Japan ASTER Science Team, 2019). Topography was considered complex when the average slope of all the 10 m pixels belonging to the same patch was greater than 3∘. In these patches, we used a tree cover mask produced in 2018 (Copernicus Land Monitoring Service, 2023) to remove from the AGBfx estimation all the pixels marked with a tree cover value of zero, where our 10 m AGB values can be considered as unreliable. This correction applies to 12 of the 113 fires considered, mostly located in Corsica and in the Pyrenees.
-
Step 8: biomass of short sclerophyllous vegetation. The Mediterranean vegetation composed largely of sclerophyllous shrubs (mostly maquis and garrigues) was identified by the CORINE land cover dataset (SDES et al., 2019) as the sclerophyllous vegetation class. Within this CORINE class, all burned 10 m pixels with vegetation higher than 3 m were treated like forests to calculate AGB-L. The pixels with vegetation shorter than 3 m were considered to be non-forests. In those pixels, our GEDI-based tree height map is not reliable for estimating vegetation height and biomass because the height of short vegetation cannot be separated from bare soil. We thus assigned a fixed value of 10 t ha−1 to all those pixels, based on allometric equations of Mediterranean shrublands from De Cáceres et al. (2019).
-
Step 9: herbaceous vegetation. Although we focus on forest fires, there can be a fraction of non-woody herbaceous vegetation in each patch, after excluding non-forest sclerophyllous in the previous step. This fraction was estimated to correspond with herbaceous vegetation burning on which was assigned a biomass density of 4 t C ha−1, based on the national grassland biomass assessment from Graux et al. (2020).
2.5 Comparison with other burned area and biomass datasets
The calculation of AGB-L described in Sect. 2.4 was applied on the 113 fire patches from BAMTs. The same calculation has been realized for the whole region outside the burned areas (see Sect. 2.1) to get a rough estimation of living biomass compared to burned one. We also computed AGB-L using two global burned area products and three global height or biomass products to compare with our high-resolution results (Table 1). The 113 fire polygons from BAMTs were compared, when available, with the national fire polygons estimates from the EFFIS (San-Miguel-Ayanz et al., 2012) and MCD64A1 burned area (Key and Benson, 2005; Giglio et al., 2018). Because the BAMTs fire dataset focused on forests and shrublands, a large amount of fires and total burned area occurring on pastures and grasslands were removed from the EFFIS and MCD64A1 data. While BAMTs relies exclusively on image analysis from the Sentinel-2 sensors, the EFFIS product relies on both hotspots obtained by the MODIS sensor and Sentinel-2 images crossover. MCD64A1 (500 m resolution) relies solely on the MODIS sensor.
Table 1Summary of the datasets used in this study. Fire area datasets are given in the first three rows, and datasets used to estimate AGB are given in the last four rows.
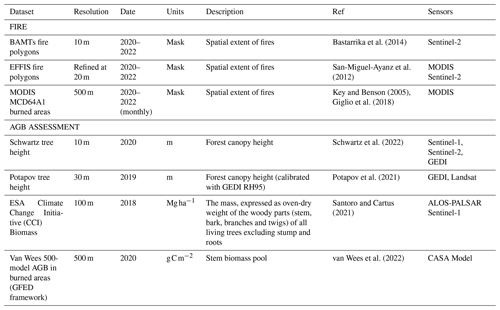
The AGB-L from each patch from our study was compared with AGB-L calculated from global tree height maps at 30 m resolution delivered globally by Potapov et al. (2021) and processed according to the same method as presented in Sect. 2.4 for the Schwartz tree height map. We also used AGB-L directly sampled within each patch from the ESA CCI Biomass dataset at 100 m resolution for the year 2018 (Santoro and Cartus, 2021). It should be noted that this AGB product considers all the biomass compartments, including foliage and understory vegetation, whereas our AGB product considers only woody tree biomass. Finally, AGB-L obtained in this study was compared to the (burned) wood carbon pool of the global 500 m resolution fire emissions dataset provided by Van Wees et al. (2022), based on the GFED framework and with biomass cycling simulated by the CASA model (van Wees et al., 2022). All these alternative products have a coarser resolution than our burned area and AGB-L maps (Table 1). Overall, we compared our results with an ensemble of three burned areas maps times four AGB maps, thus 12 different estimates of AGB-L for each of the three forest regions.
2.6 Statistical test
To establish whether a difference exists between two distributions, we used the Kolmogorov–Smirnov test (KS test). This test compares the parameters of two distributions (mean and variances) to conclude whether their difference is significant. A p value below the 0.05 threshold indicates a significant difference between the two distributions. To perform this, we used the ks.test function in the “stat” set of the R program.
3.1 Fire season 2022
During the 2022 fire season, our method estimated a burned area of 42 520 ha, which is lower than the 48 330 ha estimated by EFFIS for the same selected fires >30 ha. Our estimation excluded the spring prescribed fires, leading to a total of 66 393 ha for the whole year as estimated by EFFIS. In addition to the significantly higher burned area, this fire season was characterized by an unusual distribution outside the highly fire-prone Mediterranean region. This southeastern region of France, composed of Mediterranean forest and sclerophyllous short vegetation (shrubland, maquis, and garrigues) has been the most affected by fires each year since 2006. According to BDIFF (2006–2019) and our BAMTs estimates (2020–2022), this region (blue area in Fig. 4a) has an average annual burned area of 4812 ha yr−1 from 2006 to 2021 (standard deviation (SD) = 3855 ha), with high fire years observed in 2009 (8403 ha), 2016 (9800 ha), and 2017 (15 660 ha). Over the same period, this fire-prone part of the French territory accounted for 73.5 % (SD = 18.4 %) of the burned surface. Unlike most western European regions, the southern region of France did not experience an extreme fire season in 2022, with a burned area of 7971 ha, moderately above the mean but representing only 18.7 % of the total area burned in France that year. In contrast, the rest of the French territory, separated into two types of forests in Fig. 4a (temperate and Atlantic maritime pine), experienced a significantly different burned area. The burned area in the maritime pine forest reached 26 858 ha in 2022 (Fig. 4c) compared to an average of 494 ha yr−1 (SD = 379 ha) over the 2006–2021 period. In 2022, the total number of fires larger than 30 ha detected was 15, compared to an average of one to five fire events per year observed during the previous years. In this region, the average burned surface per fire event reached 3357 ha in 2022, compared to 216 (SD = 229) over the 2006–2021 period. Another keystone result was the abnormally large area of temperate forests that burned in 2022, reaching a total of 7813 ha, which is 2.6 times larger than the maximum of all the previous years (2019, burned area = 3035 ha). The most important temperate forest fire events occurred in Brittany, the Loire valley, and the Jura regions (Fig. 4a). Although the burned area in this region was significantly higher than the previous years, it was composed of only a few small fires, with an average fire size of 174 ha and a maximum fire size of 1730 ha. We conclude here that the 2022 fire season differs significantly from previous years' fire seasons since 2006 in many aspects, including the total burned area, the fire sizes, and the region affected. Consequently, the impact on biomass loss over the territory cannot be derived from simple correspondence rules based on previous years' observations. Therefore, further investigation was conducted on the biomass affected.
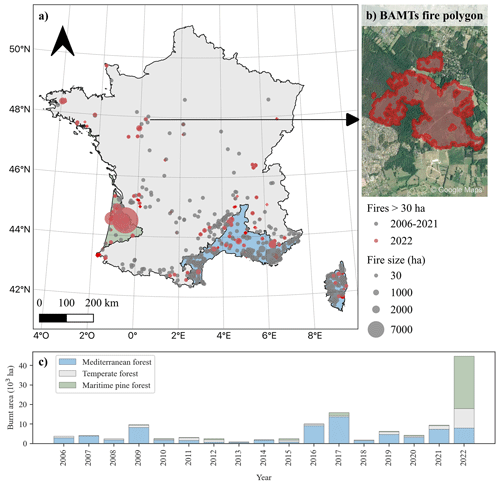
Figure 4(a) Location of fires >30 ha detected in France for 2006–2021 (gray) and 2022 (red) from BDIFF and BAMTs. The background colors indicate the main forest type. (b) Example of a fire polygon determined with BAMTs based on S2 imagery. The background image is from Google Maps. (c) Evolution of the burned area since 2006, colored by forest region. The data come from BDIFF for 2006–2019 and from BAMTs for 2020–2022.
3.2 Tree height and biomass assessment on fire patches
The accuracy of AGB-L assessment mostly depends on the accuracy of the 10 m tree height map sampled within fire patches. This tree height product was previously validated in Schwartz et al. (2022) against NFI plot data over the maritime pine forest of the Landes, showing a MAE of 2.67 m. Here, we extended this validation effort to other forest regions using a larger set of NFI plot measurements collected during 2019–2021. Only the plot locations within a 5 km area around each fire patch were considered (n=451). The validation results presented in Fig. 5 show a good agreement between our 10 m resolution canopy height map and the NFI height values, with mean absolute errors of 2.38, 2.85, and 2.59 m for the Mediterranean (n=118 plots), maritime pine (n=86 plots), and temperate forests respectively (n=247 plots). Outliers in Fig. 5, where the predicted height is close to zero, are likely to be forests that were measured by a former NFI census and were then clear-cut during the observation period for which our height map is established, especially in intensively managed forests such as Les Landes (maritime pine forest). Removing these outliers (when the predicted height <4 m) results in an MAE of 2.08 m.
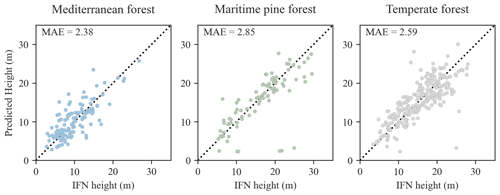
Figure 5Comparison of the French NFI height (2019–2021) from the plots within a 5 km buffer around fire locations and predicted values with the method developed in Schwartz et al. (2022). The dotted black lines indicate the x=y axis.
As a second step, we examined the relationship between tree height in 10 m burned pixels and biomass, calculated as described in 3.4 (Fig. 6). We observe that, depending on the region considered and even within one region, tree height and biomass are not correlated in the same way. In Mediterranean forests, trees are relatively smaller, but biomass increases faster with height due to a high tree density and hardwood species. Conversely, in the Atlantic maritime pine forest, trees can reach upper canopy heights of up to 25 m but with lower biomass due to high forest management limiting tree density and lower wood density for this species. Interestingly, for the old unmanaged forest of La Teste-de-Buch (tree height ∼25 m), we observe a different tree height/biomass correlation, with a higher biomass of highest trees compared to the neighboring managed forest. Temperate forests show an intermediate tree height/biomass relationship, with more biomass at a given tree height than in the maritime pine forest but less than the Mediterranean forests, with a bimodal relationship between needle leaf low wood density and hardwood forest with high wood density co-occurring in the region (Fig. 6).
3.3 Aboveground biomass loss by fires
When crossing our fire patches with our biomass map, our results reveal a marked increase in the amount of aboveground biomass loss (AGB-L) between the 2022 fire season (2.553 Mt) and the 2 preceding years (2020: 0.361 Mt, 2021: 0.805 Mt). Although the burned area in 2022 was significantly larger than the 2 previous years, being 10.3 and 4.5 times larger than in 2020 and 2021, the increase in biomass loss was proportionally lower, being only 7.1 and 3.2 times larger respectively. Between 2020 and 2021, the increase in biomass loss (times 2.21) was almost proportional to the burned area increase (times 2.38).
The 2022 fire season showed a major contribution of biomass loss from the maritime pine forests in Les Landes (68.2 %; 1.74 Mt) and by temperate forests (22.3 %; 0.57 Mt), associated with a lower relative contribution of 63.0 % and 18.3 % in burned area. The lower impact of fires on biomass loss in 2022 compared to the burned area affected is mainly due to the low contribution of biomass loss observed over Mediterranean forests, which represents only 9.8 % (0.25 Mt) of the total loss during the year 2022. In this latter region, the AGB-L was surprisingly 74 % lower than in 2021 and 42 % lower than in 2020. This decrease in AGB-L cannot be attributed to a reduction in burned area alone because in 2022 (7971 ha) about as much area was burned as in 2021 (7275 ha) and more than in 2020 (3141 ha). This difference in the Mediterranean biomass/burned area balance requires a more comprehensive analysis of biomass distribution within regions.
3.4 Biomass loss distribution in burned areas
To investigate how the spatial heterogeneity of biomass within and across fire patches affects AGB-L, we conducted a study on the biomass distribution within burned areas. The distribution of the AGB-L density (t ha−1) over burned areas during the period of 2020–2022 is shown in Fig. 8. In 2022, the distribution of AGB-L in Mediterranean forests has a left-hand skewed distribution, with a mode at 10 t ha−1. This value corresponds to the sclerophyllous biomass density that we fixed and could not be derived from tree height data. This burned biomass distribution is actually different from the available biomass distribution (red in Fig. 8) over the whole Mediterranean region (KS test p value<0.001). Our findings indicate that the 2022 fires in the Mediterranean region primarily impacted low biomass vegetation, with 88.6 % of the burned area affecting biomass values under 100 t ha−1. Notably, sclerophyllous short vegetation, which represents 26 % of the woody land cover, was found to be proportionally much more affected by fires (Fig. 8a for years 2020 and 2021). This vegetation type has a biomass of only 14 % of the median biomass of the entire region. Consequently, the higher propensity of shrublands to fire reduces the impact on biomass loss. This skewed distribution towards low biomass vegetation was even more prevalent in 2022 than during previous years.
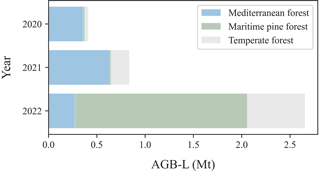
Figure 7Aboveground biomass loss (AGB-L, in dry weight) by the fires in France in 2020, 2021, and 2022.
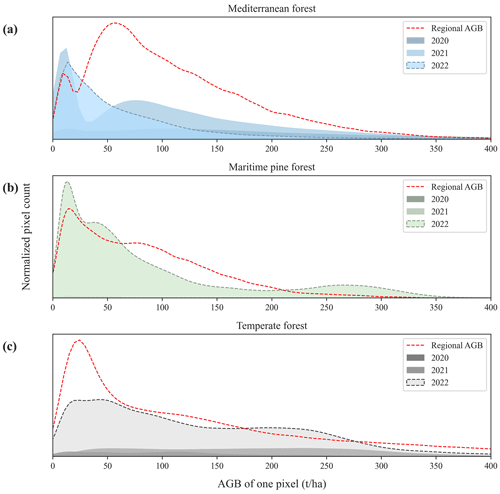
Figure 8Distribution of AGB-L density (t ha−1) in Mediterranean forests (a), maritime pine forests (b), and temperate forests (c) for 2020, 2021, and 2022. The y axis is a normalized count of the pixels with a given value of AGB. The same scale has been used for the three regions. The dotted red line represents the AGB distribution of the whole region. The y axis has been normalized for each region.
Our study reveals that the distribution of AGB-L in maritime pine forests exposed to fire in 2022 shows a binomial distribution. The first and main peak from 20 to 80 t ha−1 (35.5 % of the distribution) in Fig. 8b corresponds to the intensively managed mono-specific stands with heterogeneous ages. The area occupied by young stands with a low biomass is larger than in other regions due to shorter rotations and a larger harvested fraction. Notably, the main part of the burned distribution is actually close to the biomass distribution across the entire region. The second peak occurs over a very high biomass density (around 270 t ha−1, 10.2 % of distribution) representing the old-growth, unmanaged forest that burned during the extreme fire of La Teste on the coast. The La Teste fire AGB-L outlier illustrates one of the distinctive facets of the 2022 fire season.
Furthermore, the distribution of AGB-L in burned temperate forests in 2022 shows a rather homogeneous distribution between 20 and 250 t ha−1. The high biomass AGB-L range of 150–250 t ha−1 corresponds to 14.3 % of the distribution. This distribution of AGB-L contrasts with the distribution over the entire region, indicating that the 2022 fires affected a relatively higher proportion of old-growth forests than were available to burn (KS test p value<0.001). This impact on carbon loss was greater than expected and corresponds to another distinctive facet of the 2022 fire season.
3.5 Comparison of burned area and biomass loss datasets
The refined BAMTs method (see Sect. 2.2) shows differences in the detection rate and total area of fire polygons compared to the EFFIS and MCD64A1 products (Table 2). In contrast to BAMTs, EFFIS detected only 89 % (101 fires) of the 113 fires due to a lower detection capacity of small fires (<100 ha), as only 51 of 63 fires were recorded (81 %). However, all fires >100 ha were detected. Despite its limited capability to detect small fires, EFFIS reported a much higher total burned area of 62 002 ha over the period of 2020–2022 compared to 56 053 ha in the BAMTs method. This 10 % overestimation by EFFIS was primarily due to the overestimation of large fires (>500 ha), with the total burned area of the 16 largest fires of 2020–2022 being 4649 ha (+10 %) higher. On the other hand, the MCD64A1 product presents a higher burned area in the same sample of 113 fires detected by BAMTs, despite its lower detection rate of only 39 % (44 fires), which is much lower than EFFIS. Although MCD64A1 was capable of detecting almost all large fires (>500 ha), it was unable to achieve the same accuracy on intermediate fires (100–500 ha), with a detection rate of 68 % (23 fires), and even less on small fires (<100 ha), with a detection capability of only 10 % (6 fires). Over the matching fire events, we observe that MCD64A1 tends to overestimate the burned area for fires larger than 500 ha by 16 % (Fig. 9).
Table 2Area (ha) and count of fires detected between 2020 and 2022 with the method used in this study (BAMTs) and two other methods (EFFIS and MCD64A1). Fires are divided into small (<100 ha), medium (100–500 ha), and large areas (>500 ha).
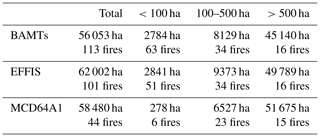
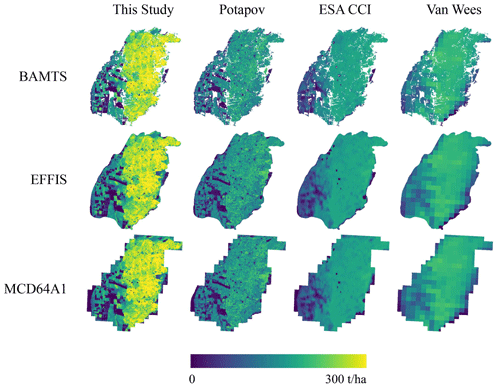
Figure 9Example of fire polygons obtained from BAMTs, EFFIS, and MCD64A1 for the fire of La Teste in an old-growth maritime pine forest with AGB-L estimations from different methods described in Sect. 2.5. The color map shows the pixel-wise estimation of AGB-L where brighter colors indicate higher AGB-L (t ha−1). The figures on top of each column indicate the total AGB-L estimation for France for 2020–2022.
Regarding our estimate of AGB-L (Sect. 2.4), we found higher values than the one obtained from global products (Fig. 10). When our biomass estimation method is applied to the tree height data from Potapov et al. (2021), we estimate that the AGB-L is 8 % lower. This difference reflects the propensity of our 10 m resolution tree height map to capture higher trees and their spatial variability within forest patches, including forest edges. Secondly, although the ESA CCI Biomass data take into account the fine elements of the trees (branches, leaves), it provides a much lower estimate of AGB-L (−32 %). This is particularly related to the underestimation of the biomass of Mediterranean ecosystems. Finally, the Van Wees et al. (2022) AGB-L seems relatively close to our estimates (−9 %) despite a much lower resolution. The major difference also appears in the Mediterranean region, for similar reasons as for ESA CCI Biomass. In conclusion, the lower-resolution products tend to estimate a higher burned area but a lower biomass being affected.
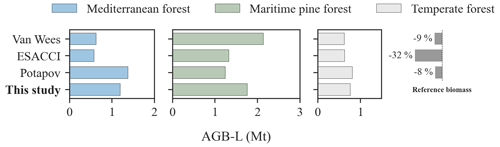
Figure 10Comparison of aboveground biomass loss (AGB-L, in Mt of dry weight) by all considered fires over the 2020–2022 period estimated by our AGB-L assessment method (this study), by our method applied on Potapov tree height, and by the ESA CCI Biomass and Van Wees 500 m model. The color represents each of the three main regions of France. The bars on the right correspond with the difference in AGB-L over all three regions (in percentage) between our method and the other data sources.
4.1 Uncertainties in burned area and biomass estimates
Our study represents a significant step towards the development of a national monitoring system for burned area polygons at high resolution for fires larger than 30 ha. This type of dataset is not yet available either for burned areas estimations delivered by forest services and previously used in scientific studies (Ruffault and Mouillot, 2017) or for regional/landscape applications relying on coarse-resolution global datasets (Barbero et al., 2019) or hand-drawn fire contours (Mouillot et al., 2003; Ganteaume and Barbero, 2019). To ensure the reliability of our dataset, we visually checked and adjusted each fire contour following international standards (Franquesa et al., 2020). We add a reminder here that pixel reflectances are mostly driven by the upper canopy so that NBR and other indices, used in BAMTs, have been shown to hardly capture understory fires (Roy et al., 2006; Morton et al., 2013). This “weakness” is shared by all global burned area datasets, as they use the dNBR (difference normalized burn ratio) index in a similar approach. Then, the mismatches we observed in burned areas across BA datasets is mostly a consequence of pixel resolution and algorithm construction. Recent advances using lidar may be of use in the future to better consider understory fires (East et al., 2023). We also set the seed of observation from registered fire events by French forest services (BDIFF), potentially non-exhaustive, thus completed by thermal anomalies (this latter source being the only source for 2022, before fire events are published by official statistics) following Majdalani et al. (2022). Our estimate of a forest burned area of 42 520 ha was lower than the official 66 363 ha provided by EFFIS for 2022, as we focused our study on forest and shrubland fires. This is a major limitation when comparing the registered national burned area's statistics with remote sensing data not filtered by land cover, as pointed out by Turco et al. (2019). EFFIS provides a total burned area assembling all sources of fires without filtering out fire types, information that is largely directly used by the media. To ensure consistency with BDIFF, the French fire observation system, we focused on forest or shrubland fires, voluntarily omitting pasture and agricultural fires where uncertainty is still high (Hall et al., 2021). We warn here about the use of raw data provided by remote sensing services without mentioning a proper description of vegetation types affected. When filtering out pastoral fires, covering roughly 15 000 ha and occurring between February and April when prescribed burning is allowed in the Pyrenees and central France, EFFIS detected 48 328 ha, much closer to our estimates, with a 10.6 % overestimation, a result actually consistent with Llorens et al. (2021). Mostly, EFFIS tends to produce a smooth external envelope of the burned polygons and ignores unburned patches within the fire patch. Still, EFFIS remains a reliable dataset when this limitation is known. We observed a much greater uncertainty on burned areas with global remote sensing datasets at coarse spatial resolution (500 m). Mostly, small fires <100 ha were not observed by MCD64A1, fires between 100 and 500 ha were underestimated, and fires larger than 500 ha were overestimated by 10 % with the same reason as for EFFIS, as they provide an external envelope omitting unburned internal patches. This caveat and the fire size threshold for reliability are consistent with Nogueira et al. (2016), or Katagis and Gitas (2022) and Galizia et al. (2021) in the Mediterranean area. To prevent misinformation to stakeholders in the future, we suggest here to increase efforts in developing a long-term nationwide observation system quickly collected and analyzed during emergency events as the 2022 fire season.
Regarding biomass, we estimated higher values than other automated global biomass information by 8 % to 32 %, mostly as a consequence of varying spatial resolution among datasets, where coarse resolution tends to underestimate higher biomass values as previously reported (Yu et al., 2022). Our AGB-L estimates rely on assumptions on tree allometry and tree density. We derived AGB only from tree height provided by remote sensing data, while standard allometry equations rely on both tree height and diameter at breast height (DBH). Field-based generic allometric equations are acknowledged to be locally variable (Henry et al., 2015) with some significant, potentially high (up to 97 %) uncertainty and impacts on C stock estimates (Vorster et al., 2020). Lidar-based biomass estimates, capturing the whole tree architecture, highly recently improved the quantification of carbon stocks in standing trees (Xu et al., 2021), fuel load for fire hazard (Fares et al., 2017), and CO2 emissions (Domingo et al., 2017) when compared to multispectral images. This latter method has been mostly based on foliage information as a proxy to tree structure and, thus, is only partially informative in logged areas where crown development saturates while tree height continues to increase (Jubanski et al., 2013). Our method, using tree height of the tallest tree at high resolution, then provides a step forward for a spatially continuous estimation of forest biomass in the highly managed French forests beside the plot-based forest inventory. The uncertainty reached in our method includes the tree height uncertainty from lidar (Schwartz et al., 2022) with an MAE varying between 2.38 and 2.85 m for tree height varying between 3 and 30 m, leading to a 10 % error. The additional error associated with the conversion of tree height to biomass is a major bottleneck in the current biomass density maps at the European or global level. Saito et al. (2022), for example, pointed out a 35 % difference between two reference global biomass datasets (GlobBiomass and GEOCARBON), and Avitabile and Camia (2018) report an error reaching up to 58 % to 67 % in Europe for biomass maps elaborated from multispectral remote sensing between 2000 and 2010 so that harmonized global AGB maps still report a 10 % to 26 % variation over Europe (Spawn et al., 2020). Using the recent methods of lidar and GEDI data, Duncanson et al. (2022) report a 40 % to 50 % RMSE from a generic method of European biomass estimates. They however also report local studies with lower errors, around 13 % to 25 %. Indeed, for example, Fassnacht et al. (2021) actually reach a 9.1 % to 15.7 % RMSE for biomass estimates from lidar in Mediterranean Chile, and Simonson et al. (2016) obtained a 10 % error in estimating Mediterranean woodland carbon stock increment from lidar compared to forest inventories. Our method then uses the benefits of the fine-resolution GEDI data with locally fitted allometric conversion rules to minimize errors in biomass estimates.
Our method also used the tallest tree height detected from GEDI data as a proxy for the forest structure at the 10 m pixel level, as suggested by Meyer et al. (2018). This constitutes a potential source of uncertainty in our study, as we had to assume a tree density function decreasing with tree height according to the generic “self-thinning” rule used in forestry in the absence of any tree density information. Tree crown delimitation from fine-resolution lidar should deliver new information to capture tree density and then reduce biomass estimate uncertainties in the future (Weinstein et al., 2021). With an average tree crown of 3 to 5 m diameter, we expect four to nine trees over the pixels, close to the average of 500 trees per hectare observed in France. We expect the high resolution used in our study to reduce the error associated with the tree density function on such a low number of trees. Ultimately, it should be noted that we chose to ignore our biomass estimates for tree heights lower than 3 m and replaced it by an average shrub and grass biomass from the literature, as lidar data remain highly uncertain (>100 % error) for this forest class of tree height (Urbazaev et al., 2018; Durante et al., 2019). Shrub (Li et al., 2022) and grassland (Graux et al., 2020) biomass can be more related to foliage proxy detected by lidar or multispectral images and should be further explored for fire impact assessments. Biomass in the understory vegetation, yet hardly provided by remote sensing (Ferrara et al., 2023), would refine carbon stocks for fire impact or fire hazard assessments from this highly flammable and fire conductive vegetation layer. We also acknowledge that our study only covers a short period from 2020 to 2022, as we chose to analyze the fire impact close to the GEDI image acquisition. Tree height information is actually highly dynamic as a result of tree cover change due to disturbances or management plans (Hansen et al., 2013; Senf and Seidl, 2020), particularly in the highly managed Landes region, preventing the use of our database backward in time without increasing bias. Further, routinely updated biomass estimates would pave the way for recurrent and accurate impact assessment, as we could show the benefits of quantifying biomass loss at fine resolution for this 2022 fire season.
4.2 Biomass-based vulnerability assessment redefines the 2022 distinctiveness
From our refined burned area and AGB-L estimates, we conclude a total biomass affected by fire of 2.553 Mt in 2022. This loss of biomass corresponds to 8.94 % of the biomass harvested each year (28.5 Mt) and further used for the energy (13.5 Mt), construction (9.5 Mt), and industrial (5.5 Mt) sectors (EFESE, 2023). More generally, fires impacted an amount of biomass corresponding to 3.86 % of the annual gross forest production (i.e., forest wood growth), which is 66 Mt yr−1. This fire affected biomass then contributed to a 17 % increase of the average natural mortality of all French forests, as reported by the national inventory.
We will note that most of the anomaly in 2022 comes from rarely observed fires in the Atlantic maritime pine and temperate forests. The high biomass lost in Les Landes is explained more by the large abnormal burned area. This large burned area had a lower-than-expected impact on AGB-L in the maritime pine forests because fires mostly affected managed forests with a year exploitation return interval. The media actually thoroughly reported images of burnings in the old-growth forest of La Teste-de-Buch with 5 times more biomass and located behind the emblematic and touristic Pyla sand dune. This old-growth forest represented, however, only 10 % of the burned area in Les Landes and 30 % of the biomass loss.
Still yet poorly reported information is the low-burned area in the Mediterranean region in 2022 (Rodrigues et al., 2023). In addition, we could show in our results the low biomass affected (mostly shrublands) compared to all available burnable biomass in the region. Fire return intervals in Mediterranean ecosystems vary between 15–25 years for shrublands and 70–120 years for forests (Mouillot et al., 2002) so that 4 to 5 times more burned area in shrublands is expected, while we experience 6 times more burned area in shrublands than forests in 2022.
The most concerning impact is then the high biomass lost in temperate forests due to high biomass densities. Unmanaged and low-fire-prone temperate forests have been accumulating large amounts of biomass across the last decades and thus were highly impacted when the fire occurred. The burned area remains low and represents only 0.056 % of the temperate forest surface. Accounting for biomass loss appears as a substantial informative variable to characterize a fire season and its ecological impacts, rather than burned area alone, which is potentially misleading when affecting contrasted ecosystems, sylvoecoregions, and forest structures.
As a first attempt, we focused on estimating the AGB affected by fires without separating surviving trees, dead trees, and gaseous/aerosol emissions into the atmosphere. Future development of fire impact assessment should dig further into total biomass, including foliage and fine fuel such as understory and litter (Li et al., 2022). This biomass fraction is economically less valuable but highly combustible and contributes to carbon and pollutant emissions to the atmosphere. Mortality in Quercus species is actually low, and previous research could show the high mortality rate in pine forests (Garcia-Gonzalo et al., 2011). The high crown architecture of P. pinaster could induce a low combustion rate and lower carbon emissions compared to other species. Combustion efficiency, defined as the fraction of affected biomass emitted to the atmosphere as CO2, is usually assumed to not exceed 20 % for the woody compartment of forests (Mouillot et al., 2006), with even lower values of 0.5 % to 3.5 % in Mediterranean forests (Harmon et al., 2022), leaving a large amount of standing biomass for decomposition (Campbell et al., 2016) or harvesting. Post-disturbance harvesting is actually a dominant strategy in Les Landes to collect timber and biomass for low-value wood products or the paper industry. For 2022, the carbon impact may be minor, while the landscape value of the touristic Landes forest might have suffered more regarding its attractiveness and emotional aspects to society (Tribot et al., 2018). One final major, yet hardly assessed, impact might be soil carbon combustion. This neglected aspect in most ecosystems in Mediterranean Europe due to low carbon stock is a major concern in boreal forests and peatlands (Wiggins et al., 2021). The soil in Les Landes pine forest is actually slowly decomposed due to the chemical properties of the needles growing on a well-drained sandy soil, thus accumulating more carbon in the soil than in Mediterranean forests as suggested by the upper soil carbon stocks inventory of France (Martin et al., 2021). Rough estimates of forest soil carbon deeper than the upper 20 cm do not exist at the national or European level, and smoldering combustion is hardly detected by remote sensing as yet. We might suspect high smoldering combustion after the 2022 fire season in Les Landes as identified by local firefighters when the Landiras fire started again in a second large fire from soil combustion lasting more than 2 weeks, a phenomenon previously observed in Mediterranean pine forests (Xifré-Salvadó et al., 2020). This should be further investigated in terms of soil carbon loss and combustion impact on atmospheric emissions.
An additional step to further account for in the holistic evaluation of fire ecological impacts for the year 2022 would be the resilience capacities of the exposed species, as Qin et al. (2022) showed how the high fire season of 2019–2020 in Australia rapidly recovered from the efficient regenerating strategies of the affected vegetation. Mediterranean shrublands and Quercus species are efficient resprouters with high resistance (low combustion or mortality) and rapid recovery rates due to basal or tree bud regeneration, reducing the ecological impact of the 7971 ha affecting this region. P. pinaster regenerates from seeds and should recover from this disturbance, as the previous large fire in the region occurred more than 15 years before, thus allowing tree maturity and seed production. In addition, this highly managed forest was rapidly harvested after the fire to collect partially burned stems and will be rapidly planted for a fast and efficient recovery with a growth rate of 0.2–0.7 m yr−1 (Lemoine, 1991). We might be more concerned about the temperate forests affected in 2022 with poor resistance strategies and low growth rates. This should be included in a fully holistic vulnerability framework to better drive communication with societies and political decisions (Forzieri et al., 2021). Post-fire recovery rates in each region could be evaluated from historical fire polygons and the current biomass map produced in this study for 2020, as performed by Berner et al. (2012) in the Euro–Siberian boreal forest.
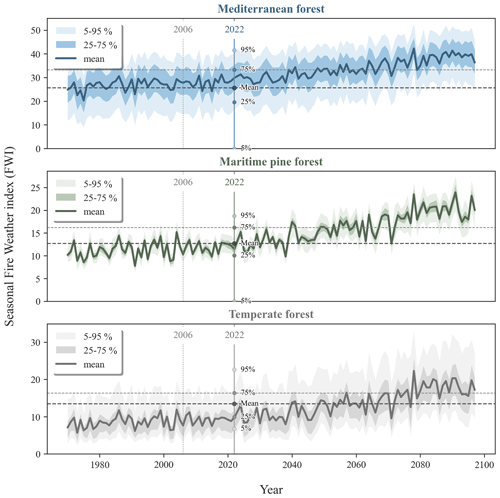
Figure 11Seasonal Fire Weather Index (FWI; June–September) obtained over the period of 1970–2097 for the three regions considered in France: Mediterranean forest, maritime pine forest, and temperate forest (Copernicus Climate Change Service, 2020). The 1970–2005 data correspond with a multi-model analysis of historical data, while the 2006–2097 data correspond with the RCP8.5 scenario, corresponding with a constant increase of carbon emissions. The dark line corresponds with the average seasonal FWI over all pixels in the region. The seasonal FWI is the average of the daily FWI values from 1 June to 30 September. The values for the year 2022 have been recalculated from the near-real-time calculation by Field et al. (2015). The value intervals of 5 %–95 % and 25 %–75 % are also provided.
4.3 The 2022 fire season with regard to historical and future fire regimes
We focused our study on the 2022 fire season, referenced as extreme for France and the overall European context, and compared this year to the 2 previous years. An extended comparison to the previous 6 years shows that 2022 was an exceptional fire year compared to preceding years, particularly outside the Mediterranean region. The extremely hot and long drought of 2022 can thus be viewed as a “natural experiment” to assess the biomass loss by new fire regimes that never or merely happened in the recent past. This conclusion that the fire season of 2022 never happened before was highly reported in the media. However, this conclusion was based on the raw EFFIS dataset, covering only the last 12 years and including pastoral fires. In the last decades, the Mediterranean region experienced way more total burned area and larger fire sizes, such as in the year 2003 when a heat wave covered most of Western Europe (Ciais et al., 2005), and even earlier in 1989, when fire prevention was less effective than nowadays (Ruffault and Mouillot, 2015). Regarding the Atlantic maritime pine forests, this region is historically less affected than the Mediterranean, but rare large fires actually occurred in the late 1940s, e.g., in 1949 when 130 000 ha were burned (Papy, 1950). We could argue that fire fighting services back in the 1940s were less efficient, but the burned area covered 5 times the burned area of 2022 with climatic conditions close to or less extreme than in 2022. For the temperate forest fires, little information has been registered for France, but the year 1976 was marked by a severe heat wave and drought and experienced large fires in northwestern France (Boulbin, 1978; Jean and Larue, 1999). If 2022 is part of the most extreme years over the recent decades, it cannot be claimed as “never observed before”. In the absence of any reference historical database for France and other European countries covering a longer period than the remote sensing era (a few decades), misleading short-term views may continue to spread out. We encourage historical data gathering, although incomplete (Pausas, 2004; Koutsias et al., 2013; Mouillot and Field, 2005), to better characterize high fire years as exceptional, unique, or novel. Still, the year 2022, with all the information available on biomass affected and precise fire contours (yet hardly available before), could be used as a reference fire year that could potentially more regularly happen in the future.
Actually, future climate projections show how warmer temperatures combined with more frequent and more extreme droughts in Europe could potentially increase the risk of fires in the future (Mouillot et al., 2002; Ruffault et al., 2020; Fargeon et al., 2020). As a result, fire indicators such as the Fire Weather Index (FWI) are expected to increase strongly in the coming decades when considering scenarios where carbon emissions are constantly increasing (RCP8.5) (Fig. 11). The high average seasonal FWI values currently observed in the Mediterranean region of France (26.75 in 1970–2005) are expected to continue to increase until reaching an extreme danger level during the whole summer period (38.7 in 2080–2097). Despite a lower-than-usual mean FWI level in this region in 2022 compared to the previous decade, the burned area was higher than normal (7971 ha compared to 4812 ha yr−1 in 2006–2021) and could tend to increase with a prolonged fire season and repeated heat waves. In the maritime pine forest, the average seasonal FWI is expected to almost double, from an average of 11.23 over the period of 1970–2005 to 20.24 over the period 2070–2097. The year 2022 was marked by an average FWI value in the normal range (12.69) of the previous decade but reaching very high local extremes (95th percentile = 18.75). This high margin of the distribution of FWI, closely linked to the extremely large fires this year, is projected to become the norm in the years 2050–2080 and could even become the low margin in the period of 2080–2097. The temperate forest experienced abnormal FWI in 2022. Projections estimate that the mean seasonal FWI should more than double between the historical period (8.59 in 1970–2005) and the end of the century (18.01 in 2080–2097). The year 2022, with an average seasonal FWI value of 13.46, already gives us a glimpse of what may be the norm in the years 2050–2070. But the fire risk is expected to be even higher at the end of the century, exceeding the values obtained for this year, which was marked by many large fires in the north of the country. Impacts on fire-induced CO2 emissions might in addition shift toward more emitting fire events (Carnicer et al., 2022).
In French NFI plot data, trees are categorized into three wood diameter classes: small wood (s) between 7.5 and 27.5 cm excluded, medium wood (m) between 27.5 and 47.5 cm excluded, and large wood (l) greater than 47.5 cm. The enumeration (n) of each tree is carried out according to three circumscribed circles as follows: (1) in a radius of 6 m, all the small (ns1), medium (nm1), and large (nl1) woods; (2) in a radius of 9 m only the medium (nm2) and large (nl2) woods; and (3) in a radius of 15 m, only the large woods (nl3). Thus, small woods are sampled on an area of As=62113.10 m2, medium woods on an area of Am=92254.47 m2, and large woods on an area of Al=152706.86 m2. To calculate the stand density of the plot (D, in nb m−2) we summed the density of each diameter class as follows:
We then used this density D, along with the AGB of each tree calculated with the allodb package, in order to fit the self-thinning rule and find the coefficient k, described in Sect. 3.3.3.
Fire polygons and their AGB-L assessments are available through the OSU OREME website (https://doi.org/10.15148/3DB37FDF-46B1-4E7A-BD86-CA4FB93307E1, Vallet et al., 2023).
Forest inventory data can be downloaded from the French National Inventory platform (https://inventaire-forestier.ign.fr/dataifn/, IFN, 2022).
Other Fire polygon data can be downloaded from the Copernicus EFFIS website (https://effis.jrc.ec.europa.eu/applications/data-and-services, EFFIS, 2022) and from the NASA MCD64A1 website (https://doi.org/10.5067/MODIS/MCD64A1.006, Giglio et al., 2015).
Other biomass data can be accessed through the GLAD website for Potapov tree height (https://glad.umd.edu/dataset/gedi, Potapov et al., 2020a), the ESA CCI Biomass web page (https://climate.esa.int/en/projects/biomass/, ESA CCI, 2022), and the supplementary materials of Van Wees et al. (2022).
LV and MS performed data curation and analysis and wrote the paper. FM and PC wrote the paper and supervised the project. AT and DvW revised the paper. DvW provided GFED framework data.
The contact author has declared that none of the authors has any competing interests.
Publisher's note: Copernicus Publications remains neutral with regard to jurisdictional claims in published maps and institutional affiliations.
The fine-resolution long-term burned area observation from remote sensing over France is supported by OSU OREME. The fire data and biomass estimation are available at https://doi.org/10.15148/3DB37FDF-46B1-4E7A-BD86-CA4FB93307E1 (Vallet et al., 2023).
We are also grateful to Cedric Vega (IGN) who made possible the validation of our height map with the French NFI plot data.
This work was supported by the French Environment and Energy Management Agency (ADEME) and the FirEUrisk H2020 project. The FirEUrisk project has been granted funding from the European Union's Horizon 2020 research and innovation program under grant agreement no. 101003890. This work was also supported by the Climate Change Initiative (CCI) Fire_cci Project (contract no. 4000126706/19/I-NB).
This paper was edited by David McLagan and reviewed by two anonymous referees.
Andela, N., Morton, D. C., Schroeder, W., Chen, Y., Brando, P. M., and Randerson, J. T.: Tracking and classifying Amazon fire events in near real time, Sci. Adv., 8, eabd2713, https://doi.org/10.1126/sciadv.abd2713, 2022.
Arrogante-Funes, F., Aguado, I., and Chuvieco, E.: Global assessment and mapping of ecological vulnerability to wildfires, Nat. Hazards Earth Syst. Sci., 22, 2981–3003, https://doi.org/10.5194/nhess-22-2981-2022, 2022.
Avitabile, V. and Camia, A.: An assessment of forest biomass maps in Europe using harmonized national statistics and inventory plots, Forest Ecol. Manag., 409, 489–498, https://doi.org/10.1016/j.foreco.2017.11.047, 2018.
Barbero, R., Curt, T., Ganteaume, A., Maillé, E., Jappiot, M., and Bellet, A.: Simulating the effects of weather and climate on large wildfires in France, Nat. Hazards Earth Syst. Sci., 19, 441–454, https://doi.org/10.5194/nhess-19-441-2019, 2019.
Basile, M., Storch, I., and Mikusiński, G.: Abundance, species richness and diversity of forest bird assemblages – The relative importance of habitat structures and landscape context, Ecol. Indic., 133, 108402, https://doi.org/10.1016/j.ecolind.2021.108402, 2021.
Bastarrika, A., Alvarado, M., Artano, K., Martinez, M., Mesanza, A., Torre, L., Ramo, R., and Chuvieco, E.: BAMS: A Tool for Supervised Burned Area Mapping Using Landsat Data, Remote Sens.-Basel, 6, 12360–12380, https://doi.org/10.3390/rs61212360, 2014.
BDIFF: https://bdiff.agriculture.gouv.fr/, last access: 8 March 2023.
Belgiu, M. and Drăguţ, L.: Random forest in remote sensing: A review of applications and future directions, ISPRS J. Photogramm., 114, 24–31, https://doi.org/10.1016/j.isprsjprs.2016.01.011, 2016.
Berner, L. T., Beck, P. S. A., Loranty, M. M., Alexander, H. D., Mack, M. C., and Goetz, S. J.: Cajander larch (Larix cajanderi) biomass distribution, fire regime and post-fire recovery in northeastern Siberia, Biogeosciences, 9, 3943–3959, https://doi.org/10.5194/bg-9-3943-2012, 2012.
Boulbin, M.: Les incendies de forêt en Bretagne, M. Boulbin in Penn ar Bed, no. 95, 25ème année, fasc. 4, 1978.
Campbell, J. L., Fontaine, J. B., and Donato, D. C.: Carbon emissions from decomposition of fire-killed trees following a large wildfire in Oregon, United States: Decomposition Following Wildfire, J. Geophys. Res.-Biogeo., 121, 718–730, https://doi.org/10.1002/2015JG003165, 2016.
Carnicer, J., Alegria, A., Giannakopoulos, C., Di Giuseppe, F., Karali, A., Koutsias, N., Lionello, P., Parrington, M., and Vitolo, C.: Global warming is shifting the relationships between fire weather and realized fire-induced CO2 emissions in Europe, Sci. Rep.-UK, 12, 10365, https://doi.org/10.1038/s41598-022-14480-8, 2022.
Cazzolla Gatti, R., Di Paola, A., Bombelli, A., Noce, S., and Valentini, R.: Exploring the relationship between canopy height and terrestrial plant diversity, Plant Ecol., 218, 899–908, https://doi.org/10.1007/s11258-017-0738-6, 2017.
Chan, E. P. Y., Fung, T., and Wong, F. K. K.: Estimating above-ground biomass of subtropical forest using airborne LiDAR in Hong Kong, Sci. Rep.-UK, 11, 1751, https://doi.org/10.1038/s41598-021-81267-8, 2021.
Chiriacò, M. V., Perugini, L., Cimini, D., D'Amato, E., Valentini, R., Bovio, G., Corona, P., and Barbati, A.: Comparison of approaches for reporting forest fire-related biomass loss and greenhouse gas emissions in southern Europe, Int. J. Wildland Fire, 22, 730, https://doi.org/10.1071/WF12011, 2013.
Chuvieco, E., Yebra, M., Martino, S., Thonicke, K., Gómez-Giménez, M., San-Miguel, J., Oom, D., Velea, R., Mouillot, F., Molina, J. R., Miranda, A. I., Lopes, D., Salis, M., Bugaric, M., Sofiev, M., Kadantsev, E., Gitas, I. Z., Stavrakoudis, D., Eftychidis, G., Bar-Massada, A., Neidermeier, A., Pampanoni, V., Pettinari, M. L., Arrogante-Funes, F., Ochoa, C., Moreira, B., and Viegas, D.: Towards an Integrated Approach to Wildfire Risk Assessment: When, Where, What and How May the Landscapes Burn, Fire, 6, 215, https://doi.org/10.3390/fire6050215, 2023.
Ciais, P., Reichstein, M., Viovy, N., Granier, A., Ogée, J., Allard, V., Aubinet, M., Buchmann, N., Bernhofer, C., Carrara, A., Chevallier, F., De Noblet, N., Friend, A. D., Friedlingstein, P., Grünwald, T., Heinesch, B., Keronen, P., Knohl, A., Krinner, G., Loustau, D., Manca, G., Matteucci, G., Miglietta, F., Ourcival, J. M., Papale, D., Pilegaard, K., Rambal, S., Seufert, G., Soussana, J. F., Sanz, M. J., Schulze, E. D., Vesala, T., and Valentini, R.: Europe-wide reduction in primary productivity caused by the heat and drought in 2003, Nature, 437, 529–533, https://doi.org/10.1038/nature03972, 2005.
Copernicus Climate Change Service: Fire danger indicators for Europe from 1970 to 2098 derived from climate projections, Copernicus Climate Change Service (C3S) Climate Data Store (CDS) [data set], https://doi.org/10.24381/CDS.CA755DE7, 2020.
Copernicus Land Monitoring Service: https://land.copernicus.eu/pan-european/high-resolution-layers/forests/tree-cover-density/status-maps/tree-cover-density-2018, last access: 15 March 2023.
De Cáceres, M., Casals, P., Gabriel, E., and Castro, X.: Scaling-up individual-level allometric equations to predict stand-level fuel loading in Mediterranean shrublands, Ann. For. Sci., 76, 87, https://doi.org/10.1007/s13595-019-0873-4, 2019.
Díaz, M. E., Figueroa, R., Vidal-Abarca, M. D. R., Suárez, M. L., and Climent, M. J.: CO2 emission and biomass loss, associated to the occurrence of forest fires in the Biobío Region, Chile: An approach from Ecosystem Services (ES), Gayana Bot., 75, 482–493, https://doi.org/10.4067/S0717-66432018000100482, 2018.
Domingo, D., Lamelas-Gracia, M. T., Montealegre-Gracia, A. L., and de la Riva-Fernández, J.: Comparison of regression models to estimate biomass losses and CO2 emissions using low-density airborne laser scanning data in a burnt Aleppo pine forest, Eur. J. Remote Sens., 50, 384–396, https://doi.org/10.1080/22797254.2017.1336067, 2017.
Duncanson, L., Kellner, J. R., Armston, J., Dubayah, R., Minor, D. M., Hancock, S., Healey, S. P., Patterson, P. L., Saarela, S., Marselis, S., Silva, C. E., Bruening, J., Goetz, S. J., Tang, H., Hofton, M., Blair, B., Luthcke, S., Fatoyinbo, L., Abernethy, K., Alonso, A., Andersen, H.-E., Aplin, P., Baker, T. R., Barbier, N., Bastin, J. F., Biber, P., Boeckx, P., Bogaert, J., Boschetti, L., Boucher, P. B., Boyd, D. S., Burslem, D. F. R. P., Calvo-Rodriguez, S., Chave, J., Chazdon, R. L., Clark, D. B., Clark, D. A., Cohen, W. B., Coomes, D. A., Corona, P., Cushman, K. C., Cutler, M. E. J., Dalling, J. W., Dalponte, M., Dash, J., de-Miguel, S., Deng, S., Ellis, P. W., Erasmus, B., Fekety, P. A., Fernandez-Landa, A., Ferraz, A., Fischer, R., Fisher, A. G., García-Abril, A., Gobakken, T., Hacker, J. M., Heurich, M., Hill, R. A., Hopkinson, C., Huang, H., Hubbell, S. P., Hudak, A. T., Huth, A., Imbach, B., Jeffery, K. J., Katoh, M., Kearsley, E., Kenfack, D., Kljun, N., Knapp, N., Král, K., Krůček, M., Labričre, N., Lewis, S. L., Longo, M., Lucas, R. M., Main, R., Manzanera, J. A., Martínez, R. V., Mathieu, R., Memiaghe, H., Meyer, V., Mendoza, A. M., Monerris, A., Montesano, P., Morsdorf, F., Nćsset, E., Naidoo, L., Nilus, R., O'Brien, M., Orwig, D. A., Papathanassiou, K., Parker, G., Philipson, C., Phillips, O. L., Pisek, J., Poulsen, J. R., Pretzsch, H., et al.: Aboveground biomass density models for NASA's Global Ecosystem Dynamics Investigation (GEDI) lidar mission, Remote Sens. Environ., 270, 112845, https://doi.org/10.1016/j.rse.2021.112845, 2022.
Durante, P., Martín-Alcón, S., Gil-Tena, A., Algeet, N., Tomé, J., Recuero, L., Palacios-Orueta, A., and Oyonarte, C.: Improving Aboveground Forest Biomass Maps: From High-Resolution to National Scale, Remote Sens.-Basel, 11, 795, https://doi.org/10.3390/rs11070795, 2019.
East, A., Hansen, A., Armenteras, D., Jantz, P., and Roberts, D. W.: Measuring Understory Fire Effects from Space: Canopy Change in Response to Tropical Understory Fire and What This Means for Applications of GEDI to Tropical Forest Fire, Remote Sens.-Basel, 15, 696, https://doi.org/10.3390/rs15030696, 2023.
EFESE: https://www.ecologie.gouv.fr/levaluation-francaise-des- ecosystemes-et-des-services-ecosystemiques, last access: 20 March 2023.
EFFIS: European Forest Fire Information System EFFIS, https://effis.jrc.ec.europa.eu/, last access: 12 December 2022.
ESA CCI: ESA’s Climate Change Initiative Biomass project, https://climate.esa.int/en/projects/biomass/, last access: 12 December 2022.
European Commission. Joint Research Centre: Forest Fires in Europe, Middle East and North Africa 2021, Publications Office, LU, https://doi.org/10.2760/34094, 2022.
Fares, S., Bajocco, S., Salvati, L., Camarretta, N., Dupuy, J.-L., Xanthopoulos, G., Guijarro, M., Madrigal, J., Hernando, C., and Corona, P.: Characterizing potential wildland fire fuel in live vegetation in the Mediterranean region, Ann. For. Sci., 74, 1, https://doi.org/10.1007/s13595-016-0599-5, 2017.
Fargeon, H., Pimont, F., Martin-StPaul, N., De Caceres, M., Ruffault, J., Barbero, R., and Dupuy, J.-L.: Projections of fire danger under climate change over France: where do the greatest uncertainties lie?, Climatic Change, 160, 479–493, https://doi.org/10.1007/s10584-019-02629-w, 2020.
Fassnacht, F. E., Poblete-Olivares, J., Rivero, L., Lopatin, J., Ceballos-Comisso, A., and Galleguillos, M.: Using Sentinel-2 and canopy height models to derive a landscape-level biomass map covering multiple vegetation types, Int. J. Appl. Earth Obs., 94, 102236, https://doi.org/10.1016/j.jag.2020.102236, 2021.
Ferrara, C., Puletti, N., Guasti, M., and Scotti, R.: Mapping Understory Vegetation Density in Mediterranean Forests: Insights from Airborne and Terrestrial Laser Scanning Integration, Sensors, 23, 511, https://doi.org/10.3390/s23010511, 2023.
Field, R. D., Spessa, A. C., Aziz, N. A., Camia, A., Cantin, A., Carr, R., de Groot, W. J., Dowdy, A. J., Flannigan, M. D., Manomaiphiboon, K., Pappenberger, F., Tanpipat, V., and Wang, X.: Development of a Global Fire Weather Database, Nat. Hazards Earth Syst. Sci., 15, 1407–1423, https://doi.org/10.5194/nhess-15-1407-2015, 2015.
Forzieri, G., Girardello, M., Ceccherini, G., Spinoni, J., Feyen, L., Hartmann, H., Beck, P. S. A., Camps-Valls, G., Chirici, G., Mauri, A., and Cescatti, A.: Emergent vulnerability to climate-driven disturbances in European forests, Nat. Commun., 12, 1081, https://doi.org/10.1038/s41467-021-21399-7, 2021.
Franquesa, M., Vanderhoof, M. K., Stavrakoudis, D., Gitas, I. Z., Roteta, E., Padilla, M., and Chuvieco, E.: Development of a standard database of reference sites for validating global burned area products, Earth Syst. Sci. Data, 12, 3229–3246, https://doi.org/10.5194/essd-12-3229-2020, 2020.
Fusco, J., Walker, E., Papaïx, J., Debolini, M., Bondeau, A., and Barnagaud, J.-Y.: Land Use Changes Threaten Bird Taxonomic and Functional Diversity Across the Mediterranean Basin: A Spatial Analysis to Prioritize Monitoring for Conservation, Front. Ecol. Evol., 9, 612356, https://doi.org/10.3389/fevo.2021.612356, 2021.
Galizia, L. F., Curt, T., Barbero, R., and Rodrigues, M.: Assessing the accuracy of remotely sensed fire datasets across the southwestern Mediterranean Basin, Nat. Hazards Earth Syst. Sci., 21, 73–86, https://doi.org/10.5194/nhess-21-73-2021, 2021.
Ganteaume, A. and Barbero, R.: Contrasting large fire activity in the French Mediterranean, Nat. Hazards Earth Syst. Sci., 19, 1055–1066, https://doi.org/10.5194/nhess-19-1055-2019, 2019.
García, M. J. L. and Caselles, V.: Mapping burns and natural reforestation using thematic Mapper data, Geocarto Int., 6, 31–37, https://doi.org/10.1080/10106049109354290, 1991.
Garcia-Gonzalo, J., Marques, S., Borges, J. G., Botequim, B., Oliveira, M. M., Tome, J., and Tome, M.: A three-step approach to post-fire mortality modelling in maritime pine (Pinus pinaster Ait) stands for enhanced forest planning in Portugal, Forestry, 84, 197–206, https://doi.org/10.1093/forestry/cpr006, 2011.
Giglio, L., Justice, C., Boschetti, L., and Roy, D.: MCD64A1 MODIS/Terra+Aqua Burned Area Monthly L3 Global 500m SIN Grid V006, NASA EOSDIS Land Processes Distributed Active Archive Center [data set], https://doi.org/10.5067/MODIS/MCD64A1.006, 2015.
Giglio, L., Boschetti, L., Roy, D. P., Humber, M. L., and Justice, C. O.: The Collection 6 MODIS burned area mapping algorithm and product, Remote Sens. Environ., 217, 72–85, https://doi.org/10.1016/j.rse.2018.08.005, 2018.
Gonzalez-Akre, E., Piponiot, C., Lepore, M., Herrmann, V., Lutz, J. A., Baltzer, J. L., Dick, C. W., Gilbert, G. S., He, F., Heym, M., Huerta, A. I., Jansen, P. A., Johnson, D. J., Knapp, N., Král, K., Lin, D., Malhi, Y., McMahon, S. M., Myers, J. A., Orwig, D., Rodríguez-Hernández, D. I., Russo, S. E., Shue, J., Wang, X., Wolf, A., Yang, T., Davies, S. J., and Anderson-Teixeira, K. J.: allodb: An R package for biomass estimation at globally distributed extratropical forest plots, Methods Ecol. Evol., 13, 330–338, https://doi.org/10.1111/2041-210X.13756, 2022.
Graux, A.-I., Resmond, R., Casellas, E., Delaby, L., Faverdin, P., Le Bas, C., Ripoche, D., Ruget, F., Thérond, O., Vertès, F., and Peyraud, J.-L.: High-resolution assessment of French grassland dry matter and nitrogen yields, Eur. J. Agron., 112, 125952, https://doi.org/10.1016/j.eja.2019.125952, 2020.
Hall, J. V., Zibtsev, S. V., Giglio, L., Skakun, S., Myroniuk, V., Zhuravel, O., Goldammer, J. G., and Kussul, N.: Environmental and political implications of underestimated cropland burning in Ukraine, Environ. Res. Lett., 16, 064019, https://doi.org/10.1088/1748-9326/abfc04, 2021.
Hansen, M. C., Potapov, P. V., Moore, R., Hancher, M., Turubanova, S. A., Tyukavina, A., Thau, D., Stehman, S. V., Goetz, S. J., Loveland, T. R., Kommareddy, A., Egorov, A., Chini, L., Justice, C. O., and Townshend, J. R. G.: High-Resolution Global Maps of 21st-Century Forest Cover Change, Science, 342, 850–853, https://doi.org/10.1126/science.1244693, 2013.
Harmon, M. E., Hanson, C. T., and DellaSala, D. A.: Combustion of Aboveground Wood from Live Trees in Megafires, CA, USA, Forests, 13, 391, https://doi.org/10.3390/f13030391, 2022.
Henry, M., Cifuentes Jara, M., Réjou-Méchain, M., Piotto, D., Michel Fuentes, J. M., Wayson, C., Alice Guier, F., Castañeda Lombis, H., Castellanos López, E., Cuenca Lara, R., Cueva Rojas, K., Del Águila Pasquel, J., Duque Montoya, Á., Fernández Vega, J., Jiménez Galo, A., López, O. R., Marklund, L. G., Milla, F., de Jesús Návar Cahidez, J., Malavassi, E. O., Pérez, J., Ramírez Zea, C., Rangel García, L., Rubilar Pons, R., Sanquetta, C., Scott, C., Westfall, J., Zapata-Cuartas, M., and Saint-André, L.: Recommendations for the use of tree models to estimate national forest biomass and assess their uncertainty, Ann. For. Sci., 72, 769–777, https://doi.org/10.1007/s13595-015-0465-x, 2015.
IFN: https://inventaire-forestier.ign.fr/spip.php?article773, last access: 9 March 2023.
Jean, G. and Larue, J.-P.: La dégradation des forêts par le feu en milieu océanique, l'exemple de la Sarthe, Norois, 184, 617–628, https://doi.org/10.3406/noroi.1999.6982, 1999.
Jones, G. M., Vraga, E. K., Hessburg, P. F., Hurteau, M. D., Allen, C. D., Keane, R. E., Spies, T. A., North, M. P., Collins, B. M., Finney, M. A., Lydersen, J. M., and Westerling, A. L.: Counteracting wildfire misinformation, Front. Ecol. Environ., 20, 392–393, https://doi.org/10.1002/fee.2553, 2022.
Jubanski, J., Ballhorn, U., Kronseder, K., J Franke, and Siegert, F.: Detection of large above-ground biomass variability in lowland forest ecosystems by airborne LiDAR, Biogeosciences, 10, 3917–3930, https://doi.org/10.5194/bg-10-3917-2013, 2013.
Katagis, T. and Gitas, I. Z.: Assessing the Accuracy of MODIS MCD64A1 C6 and FireCCI51 Burned Area Products in Mediterranean Ecosystems, Remote Sens.-Basel, 14, 602, https://doi.org/10.3390/rs14030602, 2022.
Keith, H., Lindenmayer, D. B., Mackey, B. G., Blair, D., Carter, L., McBurney, L., Okada, S., and Konishi-Nagano, T.: Accounting for Biomass Carbon Stock Change Due to Wildfire in Temperate Forest Landscapes in Australia, PLoS ONE, 9, e107126, https://doi.org/10.1371/journal.pone.0107126, 2014.
Key, C. and Benson, N.: Landscape assessment: ground measure of severity, the Composite Burn Index; and remote sensing of severity, the Normalized Burn Ratio, FIREMON Fire Eff. Monit. Inventory Syst., Vol. 2004, 2005.
Key, C. H. and Benson, N. C.: The normalized burn ratio (NBR): A landsat TM radiometric measure of burn severity, U. S. Geol. Surv. North. Rocky Mt. Sci. Cent., Bozeman MT, USA, 1999.
Koutsias, N., Xanthopoulos, G., Founda, D., Xystrakis, F., Nioti, F., Pleniou, M., Mallinis, G., and Arianoutsou, M.: On the relationships between forest fires and weather conditions in Greece from long-term national observations (1894–2010), Int. J. Wildland Fire, 22, 493, https://doi.org/10.1071/WF12003, 2013.
Lamont, B. B., Pausas, J. G., He, T., Witkowski, E. T. F., and Hanley, M. E.: Fire as a Selective Agent for both Serotiny and Nonserotiny Over Space and Time, Crit. Rev. Plant Sci., 39, 140–172, https://doi.org/10.1080/07352689.2020.1768465, 2020.
Leenhouts, B.: Assessment of Biomass Burning in the Conterminous United States, Conserv. Ecol., 2, https://doi.org/10.5751/ES-00035-020101, 1998.
Lemoine, B.: Growth and yield of maritime pine (Pinus pinaster Ait): the average dominant tree of the stand, Ann. Sci. For., 48, 593, https://doi.org/10.1051/forest:19910508 , 1991.
Li, Y., Chen, R., He, B., and Veraverbeke, S.: Forest foliage fuel load estimation from multi-sensor spatiotemporal features, Int. J. Appl. Earth Obs., 115, 103101, https://doi.org/10.1016/j.jag.2022.103101, 2022.
Llorens, R., Sobrino, J. A., Fernández, C., Fernández-Alonso, J. M., and Vega, J. A.: A methodology to estimate forest fires burned areas and burn severity degrees using Sentinel-2 data. Application to the October 2017 fires in the Iberian Peninsula, Int. J. Appl. Earth Obs., 95, 102243, https://doi.org/10.1016/j.jag.2020.102243, 2021.
Majdalani, G., Koutsias, N., Faour, G., Adjizian-Gerard, J., and Mouillot, F.: Fire Regime Analysis in Lebanon (2001–2020): Combining Remote Sensing Data in a Scarcely Documented Area, Fire, 5, 141, https://doi.org/10.3390/fire5050141, 2022.
Martin, M. P., Dimassi, B., Román Dobarco, M., Guenet, B., Arrouays, D., Angers, D. A., Blache, F., Huard, F., Soussana, J., and Pellerin, S.: Feasibility of the 4 per 1000 aspirational target for soil carbon: A case study for France, Glob. Change Biol., 27, 2458–2477, https://doi.org/10.1111/gcb.15547, 2021.
Martínez-Batlle, J.-R.: Fire and forest loss in the Dominican Republic during the 21st Century, Peer Community J., 2, e47, https://doi.org/10.24072/pcjournal.157, 2022.
Meyer, V., Saatchi, S., Clark, D. B., Keller, M., Vincent, G., Ferraz, A., Espírito-Santo, F., d'Oliveira, M. V. N., Kaki, D., and Chave, J.: Canopy area of large trees explains aboveground biomass variations across neotropical forest landscapes, Biogeosciences, 15, 3377–3390, https://doi.org/10.5194/bg-15-3377-2018, 2018.
Mori, A. S., Lertzman, K. P., and Gustafsson, L.: Biodiversity and ecosystem services in forest ecosystems: a research agenda for applied forest ecology, J. Appl. Ecol., 54, 12–27, https://doi.org/10.1111/1365-2664.12669, 2017.
Morton, D. C., Le Page, Y., DeFries, R., Collatz, G. J., and Hurtt, G. C.: Understorey fire frequency and the fate of burned forests in southern Amazonia, Philos. T. Roy. Soc. B, 368, 20120163, https://doi.org/10.1098/rstb.2012.0163, 2013.
Mouillot, F. and Field, C. B.: Fire history and the global carbon budget: a fire history reconstruction for the 20th century, Glob. Change Biol., 11, 398–420, https://doi.org/10.1111/j.1365-2486.2005.00920.x, 2005.
Mouillot, F., Rambal, S., and Joffre, R.: Simulating climate change impacts on fire frequency and vegetation dynamics in a Mediterranean-type ecosystem: A mediterranean-type ecosystem under a changing climate, Glob. Change Biol., 8, 423–437, https://doi.org/10.1046/j.1365-2486.2002.00494.x, 2002.
Mouillot, F., Ratte, J.-P., Joffre, R., Moreno, J. M., and Rambal, S.: Some determinants of the spatio-temporal fire cycle in a mediterranean landscape (Corsica, France), Landsc. Ecol., 18, 665–674, https://doi.org/10.1023/B:LAND.0000004182.22525.a9, 2003.
Mouillot, F., Narasimha, A., Balkanski, Y., Lamarque, J.-F., and Field, C. B.: Global carbon emissions from biomass burning in the 20th century: Global carbon emissions from biomass burning, Geophys. Res. Lett., 33, L01801, https://doi.org/10.1029/2005GL024707, 2006.
NASA/METI/AIST/Japan Spacesystems and U. S./Japan ASTER Science Team: ASTER Global Digital Elevation Model V003, https://doi.org/10.5067/ASTER/ASTGTM.003, 2019.
Ninan, K. N. and Inoue, M.: Valuing forest ecosystem services: what we know and what we don't, in: Valuing Ecosystem Services, Edward Elgar Publishing, 189–226, https://doi.org/10.4337/9781781955161.00021, 2014.
Nogueira, J., Ruffault, J., Chuvieco, E., and Mouillot, F.: Can We Go Beyond Burned Area in the Assessment of Global Remote Sensing Products with Fire Patch Metrics?, Remote Sens.-Basel, 9, 7, https://doi.org/10.3390/rs9010007, 2016.
Papy, L.: Le problème de la restauration des Landes de Gascogne, Cah. O.-m., 3, 231–279, https://doi.org/10.3406/caoum.1950.1688, 1950.
Pausas, J. G.: Changes in Fire and Climate in the Eastern Iberian Peninsula (Mediterranean Basin), Climatic Change, 63, 337–350, https://doi.org/10.1023/B:CLIM.0000018508.94901.9c, 2004.
Petucco, C. and Andrés-Domenech, P.: Land expectation value and optimal rotation age of maritime pine plantations under multiple risks, J. Forest Econ., 30, 58–70, https://doi.org/10.1016/j.jfe.2018.01.001, 2018.
Potapov, P., Li, X., Hernandez-Serna, A., Tyukavina, A., Hansen, M. C., Kommareddy, A., Pickens, A., Turubanova, S., Tang, H., Silva, C. E., Armston, J., Dubayah, R., Blair, J. B., and Hofton, M.: Mapping global forest canopy height through integration of GEDI and Landsat data, Remote Sens. Environ., 253, 112165, https://doi.org/10.1016/j.rse.2020.112165, 2021a.
Powell, S. L., Cohen, W. B., Kennedy, R. E., Healey, S. P., and Huang, C.: Observation of Trends in Biomass Loss as a Result of Disturbance in the Conterminous U. S.: 1986–2004, Ecosystems, 17, 142–157, https://doi.org/10.1007/s10021-013-9713-9, 2014.
Prichard, S. J., Kennedy, M. C., Andreu, A. G., Eagle, P. C., French, N. H., and Billmire, M.: Next-Generation Biomass Mapping for Regional Emissions and Carbon Inventories: Incorporating Uncertainty in Wildland Fuel Characterization, J. Geophys. Res.-Biogeo., 124, 3699–3716, https://doi.org/10.1029/2019JG005083, 2019.
Puntieri, J. G.: The self-thinning rule: bibliography revision, Preslia, 65, 243–267, 1993.
Qin, Y., Xiao, X., Wigneron, J.-P., Ciais, P., Canadell, J. G., Brandt, M., Li, X., Fan, L., Wu, X., Tang, H., Dubayah, R., Doughty, R., Crowell, S., Zheng, B., and Moore, B.: Large loss and rapid recovery of vegetation cover and aboveground biomass over forest areas in Australia during 2019–2020, Remote Sens. Environ., 278, 113087, https://doi.org/10.1016/j.rse.2022.113087, 2022.
Ramo, R., Roteta, E., Bistinas, I., van Wees, D., Bastarrika, A., Chuvieco, E., and van der Werf, G. R.: African burned area and fire carbon emissions are strongly impacted by small fires undetected by coarse resolution satellite data, P. Natl. Acad. Sci. USA, 118, e2011160118, https://doi.org/10.1073/pnas.2011160118, 2021.
Randerson, J. T., Van der Werf, G. R., Giglio, L., Collatz, G. J., and Kasibhatla, P. S.: Global Fire Emissions Database, Version 4.1 (GFEDv4), 1925.7122549999906 MB, https://doi.org/10.3334/ORNLDAAC/1293, 2017.
Rodrigues, M., Cunill Camprubí, À., Balaguer-Romano, R., Coco Megía, C. J., Castañares, F., Ruffault, J., Fernandes, P. M., and Resco de Dios, V.: Drivers and implications of the extreme 2022 wildfire season in Southwest Europe, Sci. Total Environ., 859, 160320, https://doi.org/10.1016/j.scitotenv.2022.160320, 2023.
Ronneberger, O., Fischer, P., and Brox, T.: U-Net: Convolutional Networks for Biomedical Image Segmentation, in: Medical Image Computing and Computer-Assisted Intervention – MICCAI 2015, vol. 9351, edited by: Navab, N., Hornegger, J., Wells, W. M., and Frangi, A. F., Springer International Publishing, Cham, 234–241, https://doi.org/10.1007/978-3-319-24574-4_28, 2015.
Roteta, E., Bastarrika, A., Padilla, M., Storm, T., and Chuvieco, E.: Development of a Sentinel-2 burned area algorithm: Generation of a small fire database for sub-Saharan Africa, Remote Sens. Environ., 222, 1–17, https://doi.org/10.1016/j.rse.2018.12.011, 2019.
Roteta, E., Bastarrika, A., Franquesa, M., and Chuvieco, E.: Landsat and Sentinel-2 Based Burned Area Mapping Tools in Google Earth Engine, Remote Sens.-Basel, 13, 816, https://doi.org/10.3390/rs13040816, 2021.
Rouse, J. W., Haas, R. H., Deering, D. W., Schell, J. A., and Harlan, J. C.: Monitoring the Vernal Advancement and Retrogradation (Green Wave Effect) of Natural Vegetation, 1974.
Roy, D. P., Boschetti, L., and Trigg, S. N.: Remote Sensing of Fire Severity: Assessing the Performance of the Normalized Burn Ratio, IEEE Geosci. Remote S., 3, 112–116, https://doi.org/10.1109/LGRS.2005.858485, 2006.
Ruffault, J. and Mouillot, F.: How a new fire-suppression policy can abruptly reshape the fire-weather relationship, Ecosphere, 6, art199, https://doi.org/10.1890/ES15-00182.1, 2015.
Ruffault, J. and Mouillot, F.: Contribution of human and biophysical factors to the spatial distribution of forest fire ignitions and large wildfires in a French Mediterranean region, Int. J. Wildland Fire, 26, 498, https://doi.org/10.1071/WF16181, 2017.
Ruffault, J., Curt, T., Moron, V., Trigo, R. M., Mouillot, F., Koutsias, N., Pimont, F., Martin-StPaul, N., Barbero, R., Dupuy, J.-L., Russo, A., and Belhadj-Khedher, C.: Increased likelihood of heat-induced large wildfires in the Mediterranean Basin, Sci. Rep.-UK, 10, 13790, https://doi.org/10.1038/s41598-020-70069-z, 2020.
Saito, M., Shiraishi, T., Hirata, R., Niwa, Y., Saito, K., Steinbacher, M., Worthy, D., and Matsunaga, T.: Sensitivity of biomass burning emissions estimates to land surface information, Biogeosciences, 19, 2059–2078, https://doi.org/10.5194/bg-19-2059-2022, 2022.
Salas-Gonzalez, R., Houllier, F., Lemoine, B., and Pignard, G.: Forecasting wood resources on the basis of national forest inventory data. Application to Pinus pinaster Ait. in southwestern France, Ann. For. Sci., 58, 785–802, https://doi.org/10.1051/forest:2001163, 2001.
San-Miguel-Ayanz, J., Schulte, E., Schmuck, G., Camia, A., Strobl, P., Liberta, G., Giovando, C., Boca, R., Sedano, F., Kempeneers, P., McInerney, D., Withmore, C., de Oliveira, S. S., Rodrigues, M., Durrant, T., Corti, P., Oehler, F., Vilar, L., and Amatulli, G.: Comprehensive Monitoring of Wildfires in Europe: The European Forest Fire Information System (EFFIS), IntechOpen, https://doi.org/10.5772/28441, 2012.
Santoro, M. and Cartus, O.: ESA Biomass Climate Change Initiative (Biomass_cci): Global datasets of forest above-ground biomass for the years 2010, 2017 and 2018, v2, https:// doi.org/10.5285/84403D09CEF3485883158F4DF2989B0C, 2021.
Schwartz, M., Ciais, P., Ottlé, C., De Truchis, A., Vega, C., Fayad, I., Brandt, M., Fensholt, R., Baghdadi, N., Morneau, F., Morin, D., Guyon, D., Dayau, S., and Wigneron, J.-P.: High-resolution canopy height map in the Landes forest (France) based on GEDI, Sentinel-1, and Sentinel-2 data with a deep learning approach, arXiv, https://doi.org/10.48550/arXiv.2212.10265, 20 December 2022.
Schwartz, M., Ciais, P., De Truchis, A., Chave, J., Ottlé, C., Vega, C., Wigneron, J.-P., Nicolas, M., Jouaber, S., Liu, S., Brandt, M., and Fayad, I.: FORMS: Forest Multiple Source height, wood volume, and biomass maps in France at 10 to 30 m resolution based on Sentinel-1, Sentinel-2, and GEDI data with a deep learning approach, Earth Syst. Sci. Data Discuss. [preprint], https://doi.org/10.5194/essd-2023-196, in review, 2023.
SDES, IGN, and European Environment Agency: Occupation du sol – Corine Land Cover (2018), https://doi.org/10.12770/AC797A9E-60B5-4E22-BD4C-DF2F7A6FE217, 2019.
Senf, C. and Seidl, R.: Mapping the forest disturbance regimes of Europe, Nat. Sustain., 4, 63–70, https://doi.org/10.1038/s41893-020-00609-y, 2020.
Serrano-León, H., Ahtikoski, A., Sonesson, J., Fady, B., Lindner, M., Meredieu, C., Raffin, A., Perret, S., Perot, T., and Orazio, C.: From genetic gain to economic gain: simulated growth and financial performance of genetically improved Pinus sylvestris and Pinus pinaster planted stands in France, Finland and Sweden, Forestry, 94, 512–525, https://doi.org/10.1093/forestry/cpab004, 2021.
Simonson, W., Ruiz-Benito, P., Valladares, F., and Coomes, D.: Modelling above-ground carbon dynamics using multi-temporal airborne lidar: insights from a Mediterranean woodland, Biogeosciences, 13, 961–973, https://doi.org/10.5194/bg-13-961-2016, 2016.
Sirin, A., Maslov, A., Makarov, D., Gulbe, Y., and Joosten, H.: Assessing Wood and Soil Carbon Losses from a Forest-Peat Fire in the Boreo-Nemoral Zone, Forests, 12, 880, https://doi.org/10.3390/f12070880, 2021.
Spawn, S. A., Sullivan, C. C., Lark, T. J., and Gibbs, H. K.: Harmonized global maps of above and belowground biomass carbon density in the year 2010, Sci. Data, 7, 112, https://doi.org/10.1038/s41597-020-0444-4, 2020.
Tribot, A.-S., Deter, J., and Mouquet, N.: Integrating the aesthetic value of landscapes and biological diversity, P. Roy. Soc. B-Biol. Sci., 285, 20180971, https://doi.org/10.1098/rspb.2018.0971, 2018.
Turco, M., Herrera, S., Tourigny, E., Chuvieco, E., and Provenzale, A.: A comparison of remotely-sensed and inventory datasets for burned area in Mediterranean Europe, Int. J. Appl. Earth Obs., 82, 101887, https://doi.org/10.1016/j.jag.2019.05.020, 2019.
Tyukavina, A., Potapov, P., Hansen, M. C., Pickens, A. H., Stehman, S. V., Turubanova, S., Parker, D., Zalles, V., Lima, A., Kommareddy, I., Song, X.-P., Wang, L., and Harris, N.: Global Trends of Forest Loss Due to Fire From 2001 to 2019, Front. Remote Sens., 3, 825190, https://doi.org/10.3389/frsen.2022.825190, 2022.
Urbazaev, M., Thiel, C., Cremer, F., Dubayah, R., Migliavacca, M., Reichstein, M., and Schmullius, C.: Estimation of forest aboveground biomass and uncertainties by integration of field measurements, airborne LiDAR, and SAR and optical satellite data in Mexico, Carbon Balance Manag., 13, 5, https://doi.org/10.1186/s13021-018-0093-5, 2018.
Vallet, L., Ciais, P., van Wees, D., de Truchis, A., and Mouillot, F.: Forest biomass loss by fire 2020–2022 in France, OSU OREME [data set], https://doi.org/10.15148/3DB37FDF-46B1-4E7A-BD86-CA4FB93307E1, 2023.
van Wees, D., van der Werf, G. R., Randerson, J. T., Rogers, B. M., Chen, Y., Veraverbeke, S., Giglio, L., and Morton, D. C.: Global biomass burning fuel consumption and emissions at 500 m spatial resolution based on the Global Fire Emissions Database (GFED), Geosci. Model Dev., 15, 8411–8437, https://doi.org/10.5194/gmd-15-8411-2022, 2022.
Volkova, L. and Weston, C. J.: Carbon loss from planned fires in southeastern Australian dry Eucalyptus forests, Forest Ecol. Manag., 336, 91–98, https://doi.org/10.1016/j.foreco.2014.10.018, 2015.
Vorster, A. G., Evangelista, P. H., Stovall, A. E. L., and Ex, S.: Variability and uncertainty in forest biomass estimates from the tree to landscape scale: the role of allometric equations, Carbon Balance Manag., 15, 8, https://doi.org/10.1186/s13021-020-00143-6, 2020.
Weinstein, B. G., Marconi, S., Bohlman, S. A., Zare, A., Singh, A., Graves, S. J., and White, E. P.: A remote sensing derived data set of 100 million individual tree crowns for the National Ecological Observatory Network, eLife, 10, e62922, https://doi.org/10.7554/eLife.62922, 2021.
Wiggins, E. B., Andrews, A., Sweeney, C., Miller, J. B., Miller, C. E., Veraverbeke, S., Commane, R., Wofsy, S., Henderson, J. M., and Randerson, J. T.: Boreal forest fire CO and CH4 emission factors derived from tower observations in Alaska during the extreme fire season of 2015, Atmos. Chem. Phys., 21, 8557–8574, https://doi.org/10.5194/acp-21-8557-2021, 2021.
Wu, Z., Yan, S., He, L., and Shan, Y.: Spatiotemporal changes in forest loss and its linkage to burned areas in China, J. For. Res., 31, 2525–2536, https://doi.org/10.1007/s11676-019-01062-0, 2020.
Xifré-Salvadó, M. À., Prat-Guitart, N., Francos, M., Úbeda, X., and Castellnou, M.: Smouldering Combustion Dynamics of a Soil from a Pinus halepensis Mill. Forest. A Case Study of the Rocallaura Fires in Northeastern Spain, Appl. Sci., 10, 3449, https://doi.org/10.3390/app10103449, 2020.
Xu, D., Wang, H., Xu, W., Luan, Z., and Xu, X.: LiDAR Applications to Estimate Forest Biomass at Individual Tree Scale: Opportunities, Challenges and Future Perspectives, Forests, 12, 550, https://doi.org/10.3390/f12050550, 2021.
Yoda, K.: Self-thinning in overcrowded pure stands under cultivated and natural conditions (Intraspecific competition among higher plants. XI), J. Inst. Polytech. Osaka City Univ. Ser. D, 14, 107–129, 1963.
Yu, Y., Pan, Y., Yang, X., and Fan, W.: Spatial Scale Effect and Correction of Forest Aboveground Biomass Estimation Using Remote Sensing, Remote Sens.-Basel, 14, 2828, https://doi.org/10.3390/rs14122828, 2022.
Zin, E., Kuberski, Ł., Drobyshev, I., and Niklasson, M.: First Spatial Reconstruction of Past Fires in Temperate Europe Suggests Large Variability of Fire Sizes and an Important Role of Human-Related Ignitions, Front. Ecol. Evol., 10, 768464, https://doi.org/10.3389/fevo.2022.768464, 2022.