the Creative Commons Attribution 4.0 License.
the Creative Commons Attribution 4.0 License.
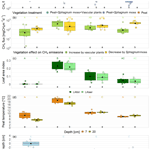
Shoulder season controls on methane emissions from a boreal peatland
Katharina Jentzsch
Elisa Männistö
Maija E. Marushchak
Aino Korrensalo
Lona van Delden
Eeva-Stiina Tuittila
Christian Knoblauch
Claire C. Treat
Thirdly, accumulation of methane in the pore water partly delayed the emission of methane produced in summer and winter to the shoulder seasons.
Cold-season emissions substantially contribute to the annual methane budget of northern wetlands, yet they remain underestimated by process-based models. Models show significant uncertainty in their parameterization of processes, particularly during the transitional phases of freezing and thawing temperatures in the shoulder seasons. Our aim was to identify the environmental controls on the components of the methane fluxes – methane production, oxidation, and transport – from a boreal peatland during the shoulder seasons. We partitioned net methane emissions into their components by combining manual chamber flux measurements on vegetation removal treatments with pore water sampling for concentrations and stable carbon isotope ratios of dissolved methane in the wet hollows of Siikaneva bog in southern Finland during seasonal field campaigns in 2021 and 2022.
The results suggest that the decrease in methane emissions due to decreasing production rates with decreasing peat temperatures in the shoulder seasons was dampened by several processes. Firstly, highly efficient transport of methane through the aerenchyma of peatland sedges continued outside of the growing season after plant senescence. Secondly, decaying vascular plants provided additional substrate for methane production at the end of the growing season. Thirdly, accumulation of methane in the pore water partly delayed the emission of methane produced in summer and winter to the shoulder seasons. Substrate-limited oxidation rates, however, largely compensated for the higher diffusion rates related to high pore water concentrations in fall. Accounting for these processes specific to the shoulder seasons by separately modeling the components of methane fluxes will likely work against the underestimation of cold-season methane emissions from northern peatlands.
-
Please read the editorial note first before accessing the article.
-
Article
(1832 KB)
-
Please read the editorial note first before accessing the article.
- Article
(1832 KB) - Full-text XML
- BibTeX
- EndNote
Wetlands are the largest natural source of atmospheric methane (CH4) (Saunois et al., 2020), a greenhouse gas with 45 times the global warming potential of carbon dioxide (CO2) (Neubauer, 2021). Wetland emissions account for 22 %–30 % of global CH4 emissions and for 60 % of total CH4 emissions from boreal regions (Saunois et al., 2020), where peatlands are the dominant wetland type. At the same time, emissions from wetlands are a major source of uncertainty for estimates of the global CH4 budget (Kirschke et al., 2013; Saunois et al., 2020). About two-thirds of the uncertainty in estimates of wetland CH4 emissions can be attributed to uncertainties in model structures and parameters related to an incomplete understanding of the processes involved in the wetland CH4 cycle (Melton et al., 2013; Saunois et al., 2020; Poulter et al., 2017). Process-based models particularly differ in their estimates of CH4 emissions at freezing and thawing temperatures and generally underestimate winter and shoulder season emissions from northern wetlands (Ito et al., 2023), thereby obscuring the high contribution of non-growing-season emissions to the annual CH4 budget (Treat et al., 2018).
In peatlands, CH4 is produced by methanogenic archaea in the anaerobic peat zone below the water table (catotelm). A part of the CH4 is converted to CO2 by methane-oxidizing archaea (methanotrophs) mostly under aerobic conditions above the water table in the surface peat layer (acrotelm) (Hanson and Hanson, 1996). The amount of CH4 emitted to the atmosphere furthermore depends on the pathway of CH4 transport (Lai, 2009). CH4 following the concentration gradient to the atmosphere via diffusion through the peat is most prone to oxidation in the acrotelm, while CH4 emitted through aerenchyma of peatland sedges or in the form of gas bubbles (ebullition) passes by the oxidation layer. All three components of CH4 fluxes – production, oxidation, and transport – are sensitive to changes in environmental and ecological conditions. Peat temperatures and water level affect the rates of CH4 production and oxidation by controlling the microbial activity and the thickness of the aerobic peat layer, respectively (Dunfield et al., 1993; Dise et al., 1993; Ström and Christensen, 2007). Peatland vegetation can affect all three components of CH4 fluxes with in part opposing effects on net CH4 emissions. Large areas of peatlands and especially of ombrotrophic bogs are typically covered by a layer of Sphagnum moss, which can actively enhance CH4 oxidation rates through a symbiotic relation – methanotrophs provide the moss with CO2 and in turn receive the oxygen released from moss photosynthesis (Larmola et al., 2010; Kip et al., 2010). Peatland sedges are adapted to high water levels by gas transport through the spongy tissue in their leaves, stems, and roots (aerenchyma). On the one hand, this gas transport can enhance CH4 emissions by allowing the CH4 to escape to the atmosphere without passing through the aerobic oxidation layer. On the other hand, oxygen can leak into the rhizosphere of aerenchymatous plants and allow for additional CH4 oxidation in the otherwise anaerobic peat zone, thereby reducing net CH4 emissions. Additionally, vascular plants can enhance CH4 emissions by providing additional substrate for CH4 production in the form of plant litter or root exudates (Joabsson et al., 1999). The magnitude and relative importance of each of these plant effects are strongly species specific (Dorodnikov et al., 2011; Korrensalo et al., 2022; Schimel, 1995; Ström et al., 2005).
Each component of CH4 fluxes has its own set of environmental and ecological controls. In order to explain the variation in net CH4 fluxes, measured CH4 emissions therefore need to be split into their components. In previous studies, the rates and pathways of CH4 production, oxidation, and transport have been quantified using chemical inhibitors for CH4 oxidation (e.g., Frenzel and Karofeld, 2000; Chan and Parkin, 2000; Bu et al., 2019) and stable carbon isotope modeling (e.g., Blanc-Betes et al., 2016; Dorodnikov et al., 2013; Knoblauch et al., 2015). Stable carbon isotope models make use of the characteristic trace that CH4 production, oxidation, and transport leave in the stable carbon isotope ratios of CH4 and CO2 through their specific preferential use of molecules containing the lighter 12C isotope. Vegetation effects on peatland CH4 emissions have been investigated in plant removal experiments, showing that vascular plants generally enhance CH4 emissions through plant-mediated CH4 transport (Frenzel and Karofeld, 2000; Riutta et al., 2020; Galera et al., 2023; Noyce et al., 2014), while oxidation in the living layer of Sphagnum moss has a decreasing effect on the CH4 emissions (Frenzel and Karofeld, 2000). Despite the previous efforts to partition net CH4 fluxes, environmental and ecological controls have rarely been studied separately for the individual flux components.
We expect a seasonal variation in the response of net CH4 emissions to changes in environmental conditions because the same environmental or ecological variable can control the strength of several, in part counteracting, flux components. Higher peat temperatures, for instance, have been shown to increase the rates of both CH4 production and oxidation, but production is much more strongly inhibited by low temperatures than oxidation (Dunfield et al., 1993). A nonlinear reaction of CH4 emissions to changes in environmental and ecological variables could furthermore be supported by interactions between the effects of individual environmental and ecological variables. For example, the effects of both peat temperature and plant-mediated CH4 transport have been shown to depend on the water level (Kutzbach et al., 2004), and the water level effect intensifies with rising temperatures (Taylor et al., 2023). Despite these indications of seasonally changing controls on CH4 emissions, previous studies of boreal peatlands have often been limited to the growing season.
In this study, we aimed to identify the processes controlling shoulder season CH4 emissions from wet hollows, i.e., typically high-emitting microtopographical features of a boreal bog (Turetsky et al., 2014) that are highly sensitive to changes in environmental conditions (Kotiaho et al., 2013). Our objectives were to quantify seasonal differences in (1) net CH4 emissions, (2) CH4 oxidation, and (3) plant-mediated CH4 transport and to relate these to seasonal changes in environmental and ecological conditions. We achieved this by isolating the seasonal effects of vascular plants and Sphagnum moss on CH4 emissions using vegetation removal experiments and relating the plant effects to CH4 production, oxidation, and transport using pore water data, including the concentrations and stable carbon isotope ratios of dissolved CH4. We considered the water level, the leaf area of vascular plants, and the peat temperatures in acrotelm and catotelm as potential environmental and ecological controls on the components of CH4 fluxes.
2.1 Study site
The study was carried out in 2021 and 2022 in an ombrotrophic bog, which is part of the Siikaneva peatland complex located in southern Finland (61°50′ N, 24°12′ E; 160 m a.s.l.), within the southern boreal vegetation zone (Ahti et al., 1968) (Fig. 1a). The average annual temperature in the area is 4.1 °C, and average temperatures in January and July are −6.5 and 16.4 °C, respectively, according to the 30-year averages (1993–2022) from the Juupajoki–Hyytiälä weather station that is located 6.3 km east of the bog site (Finnish Meteorological Institute, 2023a). The mean annual precipitation sum is 688 mm of which about one-third falls as snow (Riutta et al., 2020). The region is typically snow-covered for 190 d between 24 October and 30 April. In 2021, the annual mean temperature was similar to the 30-year average but 0.7 °C higher in 2022. Mean temperatures in January were 0.1 °C lower in 2021 and 1.2 °C higher in 2022. Mean temperatures in July were 2.4 °C higher in 2021 but similar to the 30-year average in 2022 (Fig. 2a).
Siikaneva bog has a pronounced microtopography ranging from open-water pools and low-lying bare peat surfaces to wet hollows and intermediate lawns to drier and higher hummocks. Each microtopography type has a characteristic plant community (Korrensalo et al., 2018b) and nutrient concentration in the surface peat (Korrensalo et al., 2018a). In this study, we focused on the wet hollows, which cover about 20 % of Siikaneva bog, making it the second largest microtopography type in Siikaneva bog after the lawns (Alekseychik et al., 2021). The hollow vegetation typically consists of a moss layer formed by Sphagnum cuspidatum and Sphagnum majus as well as of the aerenchymatous vascular sedges Carex limosa, Rhynchospora alba, and Scheuchzeria palustris (Korrensalo et al., 2018b). The soil in the hollows was classified as Histosol, consisting of slightly decomposed peat with a pH of 4.4 measured down to 30 cm depth.
2.2 Experimental design
We used a vegetation removal experiment, established in 2016, with one control plot and two treatments that allowed us to isolate the effects of vascular vegetation and moss on CH4 emissions (Fig. 1c). The control plot had intact natural vegetation including Sphagnum mosses and vascular plants (peat–Sphagnum–vascular, or PSV); one treatment had all vascular plants removed and only the Sphagnum moss layer remaining (PS), and another treatment had all vegetation removed, leaving behind a bare peat surface (P). For the plant removal treatments, all vascular plants had been clipped from an area of 0.5 m2 (50×100 cm), and the area had been surrounded by polypropylene root barrier fabric 70 cm deep in the ground to keep roots from growing back into the area from the sides. Ever since, any newly growing vascular plants have been gently pulled out with their roots. We assume that the disturbance caused by establishing the plant removal plots, including the gradual death and decomposition of the belowground parts of the clipped plants, was negligible in our study, which was 5 years after the experiment was installed (Riutta et al., 2020). To create the P treatment, within the vegetation removal area, about 40×40 cm of the 4 to 5 cm thick living layer of the Sphagnum moss carpet had been cut out and placed on net fabric in a frame that could be lifted aside exposing the bare peat. Circular aluminum collars (inner diameter: 30.7 cm) for chamber measurements were permanently installed at the PSV and PS plots, while at the P plots the moss layer was lifted aside and a collar was placed underneath only for the time of chamber measurements. There were five spatial replicate plot clusters within the hollow microtopography type placed along a boardwalk in Siikaneva bog, each comprising one control plot and one of each vegetation treatments (Fig. 1b, c). The data for this study were collected during seven field campaigns that took place in July, August, and October 2021 and in May, July, September, and October 2022 (Fig. 2).
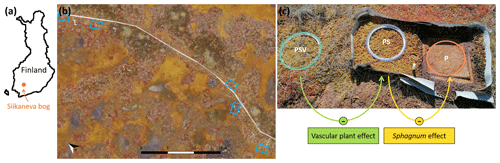
Figure 1Location of (a) Siikaneva bog in Finland, (b) the five spatial replicates of chamber measurement plot clusters (blue rectangles) within Siikaneva bog, and (c) the control plot and the two vegetation treatments within one plot cluster (PSV: intact vegetation plot including Sphagnum mosses and vascular plants; PS: Sphagnum moss plot with vascular plants removed; P: peat plot with all vegetation removed). The vascular plant effect and the Sphagnum effect on CH4 emissions were calculated as the difference between emissions from the PSV and the PS plots and from the PS and the P plots, respectively. The drone image in (b) was taken and processed in August 2022 by Lion Golde and Tabea Rettelbach (AWI).
2.3 Quantifying CH4 fluxes
2.3.1 Manual chamber measurements
During each field campaign, we measured the CH4 flux from each of the 15 plots using a transparent manual chamber. Each plot was usually measured twice – once under natural light conditions and once under dark conditions, with blackout fabric covering the chamber. In July 2021, measurements were additionally performed at two different levels of incomplete shading using one or two layers of net fabric, respectively. The different light levels were chosen to partition the CO2 fluxes that were measured alongside the CH4 fluxes, but those data are not part of this study. Since the CH4 fluxes did not differ significantly between the light levels (t(64)=1.178; p=0.2432), we treated light and dark measurements of CH4 as temporal replicates in the data analysis.
For the flux measurements, we placed a transparent cylindrical chamber with a volume of 36 L (inner height of 39.0 cm and inner diameter of 34.4 cm) on the collars at the plots (inner diameter: 30.7 cm, surrounding an area of 0.074 m2). Since the chamber was larger in diameter than the collar, we attached a rubber seal at the bottom of the chamber in 2021. In 2022, we used a 3D-printed adapter (added height: 8 cm) to connect the collar and the chamber. Both the collars and the adapter had a rim at the top that we filled with water to seal the connections. For each measurement, we kept the chamber closed for 3 min (2021) or 5 min (2022) and continuously recorded the CH4 and CO2 concentrations inside the chamber at a frequency of 1 Hz using an inline gas analyzer (LI-COR LI-7810 in summer 2021 and additionally an LGR microportable greenhouse gas analyzer (MGGA) in fall 2021 and in 2022). Prior to each measurement, we ventilated the chamber until the CH4 and CO2 concentrations inside the chamber were back to ambient conditions. Two fans with Peltier elements continuously mixed and cooled the air inside the chamber. The temperature inside the chamber was measured with a HOBO temperature sensor at a frequency of 1 Hz. Despite the cooling, the temperature inside the chamber increased by more than 1 °C in 20 % and by more than 2 °C in 10 % of the measurements between May and August. In September and October, the temperature increase inside the chamber remained within 0.5 °C of the ambient air temperature during 90 % of the measurements.
2.3.2 Flux calculations
We removed the first 25 s of each measurement to account for potential initial disturbances caused by the chamber placement. We then applied three steps of quality control. First, we discarded the measurements (14 %) showing obvious instrument failures, temporary decreases in gas concentrations indicating chamber leakage, or excessive CH4 ebullition with less than 30 s between individual ebullition events based on visual inspection of the change in CH4 concentration over time. As ebullition event, we classified every obvious sudden step increase in CH4 concentrations if, after the increase, the CH4 concentration did not return to a level similar to before the increase. Second, we visually identified the measurements showing individual episodic ebullition events (20 %) and split them into time periods of diffusive and ebullitive CH4 emissions (modified from Hoffmann et al., 2017). For this, we marked as ebullition events all consecutive rows of three or more data points that showed a concentration change from its predecessor of more than the 75th percentile + 0.7 × IQR (interquartile range) or less than the 25th percentile – 0.7×IQR of the measurement. We then extracted the longest series of consecutive data points that were not classified as ebullition for calculation of the diffusive CH4 flux. We visually controlled the performance of the algorithm and manually adjusted the time periods of diffusive CH4 emissions where needed. Third, we visually identified measurements that showed an initial exponential increase in CH4 concentrations (20 %). Some studies suggest that the change in chamber CH4 concentrations decreases over time due to a weakening concentration gradient between peat and chamber headspace and that thus the higher initial slope in CH4 concentrations is best suited to estimate the CH4 flux (e.g., Hutchinson and Mosier, 1981; Pedersen et al., 2010; Forbrich et al., 2010). Efforts to evaluate the performance of different models for flux calculations indicate that process-based models should only be applied with much caution to assure that model assumptions are met, and additional information like soil gas concentrations should be considered to identify the reason for the observed nonlinear behavior (Forbrich et al., 2010; Pirk et al., 2016). In our study, we observed an exponential increase in chamber concentrations mainly at sites with high pore water concentrations and despite short chamber closures and relatively low headspace CH4 concentrations in the comparatively large chamber. This points towards a steady ebullition of microbubbles caused by the chamber placement rather than a saturation effect. We therefore manually extracted the time periods of linear concentration change towards the end of the measurements for flux calculation. We then determined the CH4 fluxes as the slope of linear fits to all time periods extracted for calculation of the diffusive flux. To convert the mole fractions of CH4 in dry air, as measured by the gas analyzer, to molar concentrations, we used the ideal gas law with the mean temperature recorded inside the chamber during the measurement and assuming standard atmospheric pressure.
We quantified the effects of vascular plants () and of Sphagnum moss () on the CH4 fluxes by subtracting the CH4 fluxes measured at the moss plots () from the fluxes measured at the control plots () (Eq. 1) and by subtracting the fluxes measured at the bare peat plots () from the fluxes at the moss plots (Eq. 2), respectively. We subtracted pairs of fluxes measured on the same day at the same spatial replicate and light level (transparent chamber, complete, single, or double shading of the chamber). In cases where the flux measurement at the bare peat plot was only available for one light level, we used this same flux value for calculation with all light levels applied at the respective moss plot.
We discarded negative values of and when the respective other was either also negative or missing as an additional quality indicator (10 %). We assume that these unexpected observations of higher emissions from the moss plots compared to the control and/or bare peat plots were caused by processes other than the direct vegetation effects, such as spatial or temporal variation in CH4 emissions between the treatment plots or steady ebullition of microbubbles from the moss plots.
2.4 Carbon stable isotope signatures of emitted and pore water CH4 and concentrations of CH4, organic carbon, and total nitrogen dissolved in the pore water
In addition to the CH4 fluxes, we measured the δ13C values of emitted and pore water CH4 and CO2 and the concentrations of CH4, CO2, organic carbon (DOC), and total nitrogen (TDN) dissolved in the pore water at each measurement plot during the measurement campaigns in 2022. All samples for one measurement plot were taken on the same day. Only at one plot in May and in September 2022 had samples for emitted and for pore-water-dissolved CH4 to be taken on consecutive days due to bad weather conditions.
To determine the δ13C values of emitted CH4, we took 30 mL manual gas samples from the chamber headspace every 5 min during 25 min chamber closures at all measurement plots under natural light conditions. We transferred 25 mL of each gas sample into evacuated 12 mL glass vials (Labco Exetainer). To measure DOC, TDN, and the concentrations as well as the δ13C values of the CH4 dissolved in the pore water, we took 20 mL as well as 30 mL water samples in 60 mL syringes from three depths, representing conditions in the acrotelm (7 cm), as well as within (20 cm) and below (50 cm) the main root zone in the catotelm (Korrensalo et al., 2018a). We sampled once next to each control plot and once from the vegetation removal area. Since the bare peat plots were still covered with the removed moss layer sitting on net fabric apart for the short periods of flux measurements, we assumed that the investigated pore water properties below the moss layer were similar between the moss and bare peat treatments. To extract the water samples from the peat, we used a metal sampling probe with a small hole at the end that we inserted into the peat up to the desired sampling depth.
The water samples for DOC and TDN were filtered with glass fiber filters of 0.7 µm pore size, acidified with HCl, and stored under cool (4 °C) and dark conditions until DOC was quantified as non-purgeable organic carbon (NPOC) together with TDN using a Shimadzu TOC-L analyzer. The water samples for analysis of dissolved CH4 were kept cool until the evening of the same day. Then we added 30 mL of N2 to the syringes containing the water samples, shook them for 2 min to equilibrate the gas concentrations in water and gas volume, and transferred the gas phase into evacuated 12 mL glass vials (Labco Exetainer). To derive the actual pore water gas concentrations from the concentrations measured after equilibrating pore water and headspace gas concentrations, we used Henry's law, considering the temperature dependence of gas solubility (Lide and Frederikse, 1996). The glass vials were sealed with hot glue, and the samples were analyzed for concentrations and δ13C values of CH4 and CO2 by cavity ring-down spectroscopy (CRDS; Picarro G2201-I isotopic analyzer plus autosampler SAM) within 1 month after sampling. The soil gas samples had to be diluted by up to with CO2- and CH4-free synthetic air (purity ≥ 99 999 %) to obtain the optimal concentration range for the isotopic analyzer (CH4: 2–200 ppm, CO2: 400–7000 ppm). Due to different dilutions, sometimes several subsamples of the same gas sample were measured. For further data analysis, we used the gas concentrations measured in the least diluted sample and the δ13C values obtained from the dilutions that produced gas concentrations within the optimal range for the isotopic analyzer.
After the sample analysis, three corrections were applied to the measurement data. (1) The concentrations of CO2 and CH4 had to be corrected for dilution. This correction was based on the measurement of a dilution series of a standard gas (100 ppm CH4, 1 % CO2) with nine levels of dilution ratios between 1 and within each sample batch of up to 150 samples. A linear regression was performed between the measured gas concentrations and the theoretical gas concentrations calculated for the standard gas concentrations using the respective dilution factors. This regression was then used to correct the measured gas concentrations of the soil gas samples for their actual dilutions. (2) The δ13C values were corrected for the day-to-day drift. For this, samples of a reference gas (gas mixture purchased from Oy Linde Gas Ab with 10 ppm CH4 concentration, 2000 ppm CO2 concentration, −41.5 ‰ δ13C-CH4, and −35.6 ‰ δ13C-CO2; δ13C values of the reference gas were determined by calibrating it against four licensed standards from Air Liquide with −60 ‰ and −20 ‰ δ13C-CH4 and −30 ‰ and −5 ‰ δ13C-CO2) were added at the beginning and at the end of each sample batch as well as after every 15 samples within the sample batch. The offset of the average of measured δ13C values per sample batch from the actual δ13C values of the reference gas was used to correct the δ13C values of the gas samples. (3) The δ13C values were corrected for nonlinearity as a function of the gas concentration since we observed nonlinearity of the δ13C values in the low-concentration range. First, we determined the default δ13C values of the standard gas as the average δ13C value of the standard gas at dilutions that produced gas concentrations similar to the reference gas concentrations (dilution of 0.08 for CH4 and 0.2 for CO2). For each standard gas sample, the offset of the δ13C value from the default δ13C value was determined. Next, we fitted a quadratic model to this offset for each sample batch with the inverse of the gas concentration as an independent variable. Depending on the measured gas concentration, a correction factor was then calculated and applied for each measured δ13C value. We estimate an analytical uncertainty of 0.4 ‰ and 0.2 ‰ for the δ13C values and of 0.2 and 46 ppm for the concentrations of CH4 and CO2, respectively, based on the standard deviation of the reference gas values after all three corrections. We used the gas samples taken from the chamber headspace to estimate the δ13C values of the CO2 and CH4 emitted from the soil as the intercept of a linear regression function describing the δ13C values as a function of the inverse of the gas concentration (Keeling estimate) (Keeling, 1958, 1961).
For quality control of the emission δ13C calculated from the δ13C values in the chamber gas samples, we visually inspected the simultaneous high-frequency continuous CH4 concentration measurements of the portable gas analyzer. We excluded individual δ13C measurements that were separated by ebullition events from our δ13C estimates of CH4 emissions. In cases where ebullition occurred between every manual sample, the entire measurement was discarded. Measurements were also discarded if the portable gas analyzer showed a concentration change that obviously deviated from a linear or exponential form and if the gas concentrations in the manual samples deviated irregularly from the portable gas analyzer measurements (7 % of the chamber measurements). We furthermore discarded all Keeling estimates with R2 values below 0.8 (another 53 % of the chamber measurements). Low R2 values particularly occurred at low gas fluxes. Due to the generally low fluxes, all but one Keeling estimate from the PS plots had to be discarded (94 %). Keeling estimates were removed for 22 % of the PSV plots and 42 % of the P plots.
2.5 Stable carbon isotope modeling
We estimated the fraction of CH4 lost from the peat through CH4 oxidation or transport using the stable carbon isotope mass balance model proposed by Corbett et al. (2013). First, we calculated the fraction of CO2 produced by methanogenesis at each sampling depth based on the measured concentrations and δ13C values of CH4 and CO2 dissolved in the pore water and using a δ13C value of −26 ‰ for the organic starting material (Corbett et al., 2013). Assuming that methanogenesis produces equal amounts of CO2 and CH4, we next inferred the potential concentrations of CH4 dissolved in the pore water in the absence of CH4 oxidation or transport. We then derived the fraction of CH4 lost from each sampling depth based on the difference between the modeled potential and the measured CH4 concentrations in the pore water. The estimated fractions of CH4 lost from the peat represent lower limits due to the model assumption that, different from CH4, no CO2 is lost from the peat so that measured CO2 concentrations in the pore water directly result from the rate of CO2 production (Corbett et al., 2015).
To obtain a second estimate of CH4 oxidation and transport rates, independent from the rates derived from the flux measurements on the vegetation removal experiment, we attempted to split the fraction of CH4 lost from the peat into the fractions lost through oxidation and through transport, following Blanc-Betes et al. (2016) and Liptay et al. (1998). However, we abandoned the attempt when we found unrealistic negative fractions of CH4 oxidized in the surface peat of the control plots, similar to Dorodnikov et al. (2013), which were probably related to uncertainties in the assumed isotopic fractionation by oxidation and plant transport (Appendix B).
2.6 Collecting environmental data
2.6.1 Environmental controls on CH4 fluxes
As potential environmental controls on diffusive CH4 fluxes and their components, we considered peat temperatures, water table depth, and the green leaf area of all vascular plants and of aerenchymatous sedges. Peat temperatures at 7 and 20 cm depths were measured manually with a rod thermometer next to each control plot and within the vegetation removal area right after the pore water sampling. The water table depth was measured manually on the days of flux measurements and pore water sampling in perforated plastic tubes that were permanently installed in the peat at average surface elevation once per plot cluster.
We determined the leaf area index (LAI) inside each control plot following Wilson et al. (2007). We estimated the average number of leaves per square meter for each vascular plant species by counting their leaves within each control plot on 3 d in 2021 and on 5 d in 2022. To determine the average leaf sizes, we collected samples of each species from the measurement site on the day of leaf counting and measured their leaf area with a LI-3000 portable area meter (LI-COR, Lincoln, Nebraska). We applied correction factors to the measured average leaf areas to account for the typical leaf shape of each vascular plant species (Op de Beeck et al., 2017). We then calculated the LAI on the sampling days for each vascular plant species present in each control plot by multiplying the respective leaf number with the average leaf area per square meter. To reconstructed the LAI of each vascular plant species for each day in 2022, we used the log-normal curve version of the model presented by Wilson et al. (2007). For 2021, the curve could not be fitted because of too few sampling days. We therefore linearly interpolated the LAI between the sampling days. We calculated the total LAI of each control plot as the sum of the LAI of all vascular plants present at the measurement plot (LAItot). The LAI of aerenchymatous plants (LAIaer) was determined as the sum of the LAI of all aerenchymatous species present in the hollows, namely C. limosa, Scheuchzeria palustris, R. alba, and Eriophorum vaginatum.
2.6.2 Meteorological conditions
To characterize the meteorological conditions at the study site in 2021 and 2022, we used air temperature, water table depth, and snow depth measured at the weather station at Siikaneva fen (Alekseychik et al., 2023), about 1.3 km southeast of Siikaneva bog. We corrected air temperature and water table depth measurements for conditions at the bog site based on a linear regression between bog and fen data between 2011 and 2016 when measurements were still being performed at both sites (Figs. 2a, b, A1, A2 in Appendix A).
Additionally, we used the water table depth and the peat temperatures at 2 and 10 cm depths, recorded four times per day at four spatial replicates within the hollow microtopography type at Siikaneva bog starting in July 2021 (Fig. 2a). To verify the timing of onset and complete thaw of the snow cover, we used the photos from a PhenoCam installed at Siikaneva bog and overlooking a hollow area (https://phenocam.nau.edu/webcam/sites/siikanevabog/, last access: 13 August 2024).
To separate the measurement years into seasons, we used the thresholds in daily mean temperatures of below 0 °C in winter, between 0 and 10 °C in spring and fall, and above 10 °C in summer, given by the Finnish Meteorological Institute (2023b). We modified this definition by only recognizing a change between seasons when daily average air temperatures were above the lower threshold (0 °C for spring, 10 °C for summer) or below the upper threshold (10 °C for fall, 0 °C for winter) for at least 3 consecutive days and when periods of consecutive days with average temperatures below the lower or above the upper threshold did not exceed 3 d. We defined the growing season as the snow-free time period where soil temperatures at 2 cm depth were continuously above 0 °C (Fig. 2).
2.7 Applying statistical analyses
Due to better data coverage and the availability of concentration and isotopic data from the pore water, we limited our statistical analyses to the data collected in 2022. We used linear mixed-effects models to test whether the measured CH4 fluxes, pore water CH4 concentrations, and δ13C-CH4 values differed significantly between measurement campaigns, vegetation treatments, and sampling depths. We furthermore applied linear mixed-effects models to identify environmental variables controlling the CH4 fluxes from the control plots and from both vegetation treatments as well as the vegetation effects on CH4 fluxes. As potential environmental controls, we considered peat temperatures at 7 and 20 cm depths, water table depth, LAItot, and LAIaer. As expected, we found a strong positive correlation (r>0.8) between LAItot, LAIaer, and the peat temperatures at 7 and 20 cm. We used the function lme of the package nlme to construct the models and the stepAIC function of the package MASS to identify the best combination of fixed effects. The stepAIC function uses the AIC value (Akaike information criterion) to evaluate whether the addition of a fixed predictor significantly improves the model compared to the simpler one. We then recomputed the model parameters for the best model including the spatial replicates as a random effect to account for the randomized block design with repeated measures. Univariate models best explained the variation in all but one flux data set. Only for the fluxes from the bare peat treatments did a multivariate model perform better. To achieve normality of the residuals, which we tested using the Shapiro–Wilk test with the function shapiro.test, the CH4 fluxes as well as the vegetation effects had to be logarithmically transformed prior to statistical analyses. We applied the post hoc Tukey HSD (honestly significant difference) test to identify significant differences (p<0.05) between combinations of vegetation treatment, measurement campaign, and sampling depth in the model results using the glht function of the package multcomp. All statistical analyses were done in the R environment (version 4.3.0).
3.1 Environmental and ecological conditions
The green leaf area of vascular plants, the peat temperatures, and the water table depth showed a clear seasonal trend with values increasing between spring and summer and then decreasing again towards late fall (Fig. 3c–e).
Aerenchymatous plants accounted for 91±12 % of the LAI of vascular vegetation during all measurement campaigns. Both LAItot and LAIaer were close to zero in spring, reached their maximum in summer, and decreased again after but still remained above zero in late fall. Peat temperatures at 7 cm depth were significantly higher than at 20 cm depth in spring and summer, reaching peak summer values of 18.5±1.8 °C. Around early fall, the temperature profile started to reverse, showing slightly higher temperatures at 20 cm depth than at 7 cm depth in late fall. While peat temperatures at 7 cm depth were similar in spring and late fall (9.2±1.9 to 7.7±0.5 °C), temperatures at 20 cm depth were significantly higher in late fall than in spring (8.3±0.6 °C vs. 5.4±0.5 °C). The water table depth followed a seasonal trend similar to the ones of LAI and peat temperatures with the water table being close to the surface in spring, then decreasing until reaching its annual minimum of 7±2 cm below the surface in summer and then increasing again towards late fall but still remaining significantly below the spring levels.
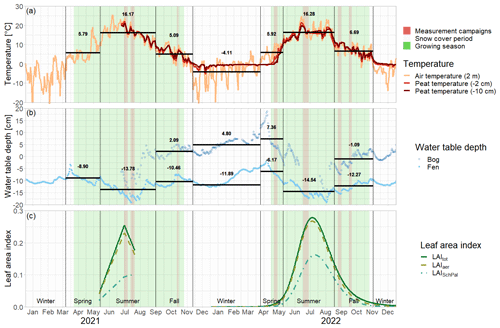
Figure 2Daily mean air and peat temperatures (a), daily mean water table depth (b), and daily leaf area index of the total green vascular vegetation (LAItot), aerenchymatous plants (LAIaer), and Scheuchzeria Palustris (LAISchPal) (interpolated and modeled based on field measurements for 2021 and 2022, respectively) (c) at Siikaneva bog in 2021 and 2022. The snow cover period is the time period between the first and the last day of snow cover even if interrupted by snow-free days. Water table depth at the nearby Siikaneva fen site is given to show the general course of the water table over the year at times where no water table measurements were available for Siikaneva bog. Seasonal mean air temperatures and water table depths are given as horizontal lines and noted in the figures.
3.2 CH4 fluxes
3.2.1 Seasonal variation in CH4 fluxes
Mean CH4 emissions from the control plots with intact vegetation (PSV plots) showed a clear seasonal trend with a significant increase between spring (177 ± 221 mg CH4 m−2 d−1) and summer (342 ± 273 mg CH4 m−2 d−1) and a subsequent significant decrease between summer and late fall back to spring levels (136 ± 175 mg CH4 m−2 d−1) (Fig. 3a). Emission rates ranged between a minimum of 34 mg CH4 m−2 d−1 measured in spring and a maximum of 1025 mg CH4 m−2 d−1 in summer.
The seasonal trend in CH4 emissions from the control plots was similar to the seasonal variations in all of the considered environmental and ecological variables. Higher LAItot, LAIaer, peat temperatures at 7 and 20 cm depths, and water table depth all resulted in higher CH4 emissions from the control plots (Fig. 3, Table A1 in Appendix A). The increase in CH4 emissions with increasing LAIaer explained most of the variation in the fluxes at the control plots.
3.2.2 Vegetation effects on CH4 fluxes
The presence of sphagna (PS treatment) decreased the CH4 emissions by 30 to 1502 mg CH4 m−2 d−1 compared to the bare peat (P treatment) during all measurement campaigns. The additional presence of vascular plants increased the CH4 emissions by 2 to 960 mg CH4 m−2 d−1, but they still remained below the emissions from the bare peat in spring and in fall (Fig. 3a). Both the decreasing effect of the Sphagnum moss and the increasing effect of the vascular plants on the CH4 emissions were significant during the fall campaigns.
The effect of Sphagnum moss on CH4 emissions showed a seasonal trend similar to the one of the total CH4 emissions from the bare peat. The moss layer decreased the CH4 emissions significantly more in late fall (493 ± 234 mg CH4 m−2 d−1) than in spring (106 ± 73 mg CH4 m−2 d−1) both due to significantly higher emissions from the bare peat plots (P treatment) and significantly lower emissions from the moss plots (PS treatments) in fall than in spring (Fig. 3a, b). The relative effect of the moss layer was weakest in summer, decreasing the CH4 emissions from the bare peat plots by 76 ± 29 %, and highest in late fall with a decrease by 98 ± 1 % (Fig. A4). The effect of the Sphagnum layer on CH4 fluxes was independent of peat temperatures and water table depth, when considered separately (Table A5). Similar to the CH4 emissions from the bare peat plots, the moss effect was best described by a combination of its increase with increasing peat temperature at 20 cm depth and its increase with decreasing peat temperature at 7 cm (Tables A3, A5). Additionally, the moss effect was stronger at higher water tables.
The effect of vascular plants on CH4 emissions showed a seasonal trend similar to the one of the CH4 emissions from the control plots (Fig. 3b), accounting for between 55 ± 31 % of the CH4 emitted from the control plots in spring and 94 ± 3 % in summer (Fig. A4). The absolute increase in CH4 emissions in the presence of vascular plants increased between spring and summer and then decreased again until late fall to reach values similar to the spring increase. Similar to the CH4 emissions from the control plots, the effect of vascular plants was stronger at higher LAItot, LAIaer, peat temperatures at 7 and 20 cm depths, and water table depth (Table A4). Vascular plants particularly led to a stronger increase in CH4 emissions at higher peat temperatures at 20 cm depth.
The decreasing effect of the Sphagnum moss on CH4 emissions canceled out the enhancing effect of the vascular plants in spring, summer, and early fall, leading to CH4 emissions from the control plots similar to those from the bare peat (177 ± 221 vs. 152 ± 101 mg CH4 m−2 d−1 in spring, 342 ± 273 vs. 377 ± 413 mg CH4 m−2 d−1 in summer, and 189 ± 134 vs. 470 ± 588 mg CH4 m−2 d−1 in early fall). In late fall, the Sphagnum effect was significantly higher than the vascular plant effect, showing as higher CH4 emissions from the bare peat compared to the control plots (505 ± 257 vs. 136 ± 175 mg CH4 m−2 d−1; Fig. 3a).
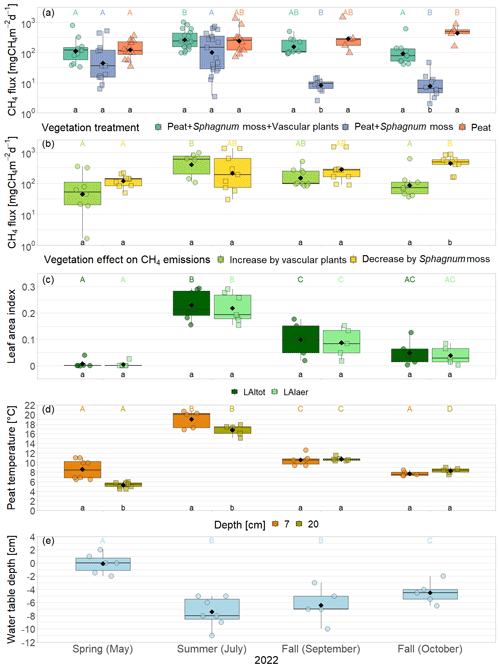
Figure 3CH4 emissions from the vegetation removal experiment (a) and effects of vascular plants and Sphagnum layer on CH4 emissions (b) by measurement campaign, displayed on logarithmic axes. Leaf area index of green vascular plants for total vascular vegetation (LAItot) and aerenchymatous plants only (LAIaer) (c), peat temperatures (d), and water table depth (e). Markers show the individual values; the boxplot shows the median (horizontal line), 25th percentile, and 75th percentile (hinges), as well as smallest and largest values, no more than 1.5 times the interquartile range from the hinges (whiskers). Values above/below the whiskers are classified as outliers. Mean values are given as black diamonds. Letters indicate significant differences (p<0.05) with different capital letters above the boxes indicating significant differences between seasons within one category (a – treatment, b – plant type, c – vascular plant type, d – measurement depth) and different small letters below the boxes indicating significant differences between these categories within one season. The significant differences displayed in (a), (b), and (c) were derived from the logarithmically transformed data.
3.3 Pore water properties
3.3.1 CH4 pore water concentrations
The concentrations of CH4 dissolved in the pore water underneath the control plots ranged from 26 µmol L−1 at 7 cm depth in summer to 444 µmol L−1 at 50 cm in spring (Fig. 4a). Mean pore water concentrations were higher underneath the vegetation removal treatments (PS and P plots) than under the control plots at all depths in summer and particularly in fall (72 ± 31 %), but the differences were not significant during any campaign or at any sampling depth (Table A6). Mean pore water concentrations increased by 58 ± 17 % between 7 and 20 cm depths across all treatments and campaigns, but the difference was only significant at the vegetation removal treatments in May. Mean pore water concentrations at the control plots were highest in spring and late fall at all depths. At the vegetation removal treatments, the concentrations were similarly highest during the shoulder seasons (spring and fall), particularly at 20 cm depth, with higher concentrations even in early fall than in summer.
3.3.2 δ13C values of CH4 emitted and dissolved in the pore water
Pore water δ13C-CH4 values underneath the control plots ranged from −72.7 ‰ at 20 cm depth in spring to −47.1 ‰ at 7 cm depth in summer. In spring, the δ13C values of dissolved CH4 were similar between the control plots (PSV) and the vegetation removal treatments (PS and P) and constant with depth. At the vegetation removal treatments, δ13C-CH4 values remained similar for the rest of the year, showing only a slight enrichment in 13C at 7 cm depth in summer and early fall (−65.9 ± 2.4 ‰ in spring and late fall vs. −63.1 ± 4.7 ‰ in summer and early fall) and at 20 cm depth in summer and both fall campaigns (−69.3 ± 2.0 ‰ in spring vs. −67.9 ± 2.6 ‰ in summer and fall). Dissolved CH4 at the control plots became more enriched in 13C compared to the vegetation removal treatments at 7 and 20 cm depths in summer and fall (−67.6 ± 1.6 and −68.7 ± 3.3 ‰ in spring vs. −58.2 ± 4.7 and −62.5 ± 3.3 ‰ in summer and fall at 7 and 20 cm, respectively). This enrichment in 13C at the control plots after spring resulted in significantly less negative δ13C values in summer and fall than in spring at 7 cm depth (−58.2 ± 4.7 vs. −67.6 ± 1.6 ‰) and significantly less negative values at 7 cm than at 50 cm depth in summer and fall (−58.2 ± 4.7 vs. −67.0 ± 2.1 ‰) (Table A7). The differences in δ13C values between the control plots and the vegetation removal treatments, however, were only significant at 7 cm depth in July (−56.4 ± 7.5 vs. −63.0 ± 2.8 ‰). While CH4 generally became more enriched in 13C, pore water CO2 became more depleted towards the peat surface (Fig. A8).
The range of δ13C values differed between the CH4 emitted and dissolved in the pore water. CH4 emitted from the control plots was significantly more depleted in 13C than the dissolved CH4 at 7 cm depth during all measurement campaigns, ranging from −83.9 ‰ to −69.1 ‰. CH4 emitted from the bare peat plots was significantly enriched in 13C compared to the CH4 emitted from the control plots in spring, summer, and late fall. In fall, δ13C values of the CH4 emitted from the bare peat plots were similar to the values of the CH4 dissolved at 7 cm depth (−65.2 ± 4.8 vs. −64.6 ± 4.6 ‰), while emissions were more enriched in 13C compared to pore water CH4 in spring and summer (−56.7 ± 11.4 vs. −64.3 ± 3.1 ‰). This enrichment of CH4 in 13C upon emission from the bare peat plots was significant in summer. For the moss plots (PS treatment), all but one δ13C value for emitted CH4 had to be discarded due to low accuracy of the Keeling estimates, mostly related to low emission rates.
3.3.3 Modeled CH4 loss through oxidation and transport
Modeled potential CH4 concentrations in the absence of oxidation and transport increased with depth at both control and vegetation removal plots during all field campaigns. This depth increase was significant except for the vegetation removal treatments in summer (Table A8). Differences between treatments or measurement campaigns were not significant but modeled potential CH4 concentrations slightly increased after spring at 7 and 20 cm depth. At the control plots, potential CH4 concentrations slightly increased between early and late fall at all depths, while the concentrations at the vegetation removal treatments decreased so that potential concentrations at the control plots slightly exceeded the concentrations at the vegetation removal plots at all depths in late fall.
A large fraction of the produced CH4 was lost from the peat through oxidation and transport. CH4 loss from 7 cm depth at the control plots was significantly higher in summer and fall than in spring (90 ± 5 % vs. 70 ± 6 %). The fraction of CH4 lost from the vegetation removal plots was generally lower than at the control plots. This treatment difference increased after summer with decreasing loss rates from the vegetation removal plots and became significant at 7 and 20 cm depths in late fall. A significantly higher fraction of CH4 was then lost from 7 cm than from 50 cm depth at the vegetation removal plots (Table A9).
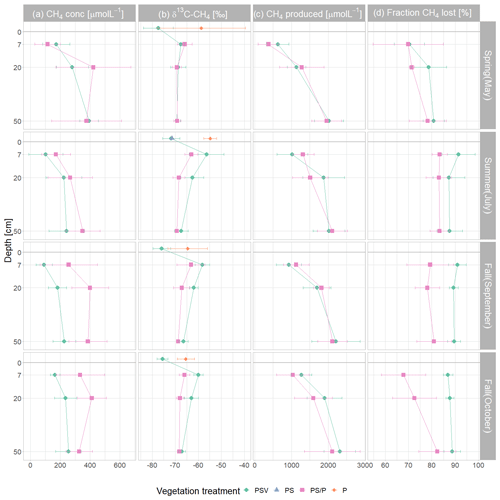
Figure 4Mean and standard deviation of dissolved pore water CH4 concentrations (a); δ13C-CH4 values (b); modeled potential CH4 concentrations if no CH4 was lost through oxidation or transport (c); and fraction of CH4 lost through oxidation or transport (d) by measurement campaign, vegetation treatment, and sampling depth. The δ13C values of emitted CH4 are displayed above the peat surface (depth of 0 cm). Control plots and vegetation treatments were the following. PSV: intact vegetation including Sphagnum mosses and vascular plants; PS: Sphagnum moss with vascular plants removed; P: peat with all vegetation removed. Pore water data were combined for the PS and P plots because the vegetation removal treatments were collocated. Significant differences between measurement campaigns, vegetation treatments, and sampling depths are given in Tables A6 to A9.
In our study, we combined measurements of CH4 emissions from vegetation removal experiments in the wet hollows of a boreal bog with pore water CH4 concentration and isotopic data. We aimed to quantify and explain seasonal differences in the components of CH4 emissions – production, oxidation, and transport – considering their environmental and ecological controls.
The CH4 emissions measured in this study were higher than most chamber measurements of CH4 emissions reported for other non-permafrost bogs but similar to the emissions previously found at Siikaneva bog. According to our study, on average, 287 mg CH4 m−2 d−1 was emitted from the control plots with intact vegetation in the hollows of Siikaneva bog between May and October in 2021 and 2022, while the mean emissions from non-permafrost bogs with sedges during the same time of year that are included in the BAWLD data set were 52 ± 66 mg CH4 m−2 d−1 (Kuhn et al., 2021). The mean CH4 emissions in our study were, however, similar to the ones found for Siikaneva bog by Korrensalo et al. (2018b) of 200, 250, and 300 mg CH4 m−2 d−1 in 2012, 2013, and 2014, respectively. This indicates that CH4 emissions from Siikaneva bog are high compared to the emissions from other boreal bogs. The emissions found in our study might also be higher than most mean emissions reported in the BAWLD data set, because we focused on hollows which have been shown to be high-emitting features of patterned boreal bogs (Frenzel and Karofeld, 2000; Moore and Knowles, 1990; Waddington and Roulet, 1996; Laine et al., 2007).
4.1 Vegetation effects on CH4 production, oxidation, and transport
We quantified the effects of vascular plants and of Sphagnum moss on CH4 emissions as the difference in emissions between vegetation removal treatments, and we used pore water data to relate them to the processes involved in the CH4 cycle (CH4 production, oxidation, and transport). We found that CH4 oxidation in the Sphagnum moss layer significantly reduced net CH4 emissions, while the presence of vascular plants increased CH4 emissions predominantly through plant-mediated CH4 transport.
4.1.1 Sphagnum moss layer decreases CH4 emissions
A significant decrease in CH4 emissions in the presence of sphagna indicated significant CH4 oxidation in the moss layer (Fig. 3a). The presence of a Sphagnum moss layer decreased net CH4 emissions by 83 ± 27 % across all measurement campaigns, which is in line with the fivefold increase in CH4 emissions upon removal of the moss layer in Sphagnum-dominated hollows of ombrotrophic peat bogs found by Kip et al. (2010). The decrease in CH4 emissions related to moss layer effects agrees with the high mean value and the high variability of oxidation rates previously reported for wetlands (Segers, 1998; Roslev and King, 1996).
The main effect of the Sphagnum moss layer on CH4 fluxes was to reduce emissions by providing conditions conducive to CH4 oxidation. CH4 oxidation in the Sphagnum moss layer was supported by (1) aerobic conditions as well as by (2) a loose symbiosis between Sphagnum species and methanotrophs (Larmola et al., 2010; Kip et al., 2010). (1) The living moss layer of about 4 to 5 cm thickness was at least partly above the water table for all but four measurements in spring (Fig. 3e). Oxic conditions thus prevailed in the Sphagnum moss layer during most of our measurement campaigns, allowing for aerobic CH4 oxidation. (2) In a symbiosis between sphagna and methanotrophs, the methanotrophs benefit from the oxygen supplied by the mosses through photosynthesis while the mosses use the CO2 released from CH4 oxidation by methanotrophs (Liebner et al., 2011). In contrast to previous findings on the link between CH4 oxidation and moss-associated photosynthesis, net CH4 emissions in our study did not change with changing light exposure (Liebner et al., 2011). The stronger decreasing effect of the Sphagnum moss on CH4 emissions at higher water tables (Table A5), however, is in line with the higher oxidation rates found in submerged Sphagnum moss (Larmola et al., 2010; Kip et al., 2010).
4.1.2 Vascular plants increase CH4 emissions
The main function of vascular plants in our study was to provide a direct pathway for CH4 transport to the atmosphere, passing by the aerobic peat layer and thus avoiding oxidation. Plant-mediated CH4 transport in the presence of vascular plants showed as (1) higher CH4 emissions, (2) lower concentrations of CH4 in the pore water, and (3) an accumulation of the heavier 13CH4 molecules in the rhizosphere due to a preferential emission of the lighter 12CH molecules. During plant senescence in fall, decaying vascular plants furthermore provided additional substrate for CH4 production.
(1) Vascular plant effects that increase CH4 emissions, i.e., plant-mediated CH4 transport and/or enhanced substrate supply for methanogenesis, dominated over the decreasing effect of rhizospheric oxidation (Joabsson et al., 1999), as previously found by Whiting and Chanton (1992), Frenzel and Rudolph (1998), Ström et al. (2012), Henneberg et al. (2016), and Noyce et al. (2014) (Fig. 3a, b). The high summer contributions of vascular plant effects to total CH4 emissions of 94 ± 3 % in 2022 (Fig. A4) are in line with previously reported proportions of plant transport between 70 % and more than 90 % of the total CH4 emissions (Whiting and Chanton, 1992; Schimel, 1995; Riutta et al., 2020; Knoblauch et al., 2015), indicating that plant transport is the primary pathway for CH4 emissions in the presence of aerenchymatous plants (Van Der Nat and Middelburg, 1998). The high mean vascular plant effect found in our study can be explained by the dominance of aerenchymatous plants and in particular of Scheuchzeria palustris (Fig. 2c), which transports the most CH4 of all studied aerenchymatous bog plant species (Dorodnikov et al., 2011; Korrensalo et al., 2022). The large range of positive vascular plant effects, accounting for 1 % to 99 % of the CH4 emissions, furthermore matches the proportions of plant-mediated CH4 transport of 6 % to 90 % reported for Siikaneva bog between May and October by Korrensalo et al. (2022).
(2) Effective CH4 transport to the atmosphere through aerenchymatous plants decreased the concentrations of CH4 dissolved in the pore water. The pore water concentrations of 242 ± 118 µmol L−1 that we measured at 50 cm depth underneath the control plots in summer are lower than the concentration of around 600 µmol L−1 reported for an unvegetated mud bottom hollow in an Estonian bog by Frenzel and Karofeld (2000), which is more similar to the concentrations of 350 ± 117 µmol L−1, reaching individual values of up to 541 µmol L−1, that we found underneath the plots where all vascular plants had been removed. Concentrations underneath the control plots were similar to the concentrations of 150 to 250 µmol L−1 found for the sedge-dominated hollows of a Finnish fen by Dorodnikov et al. (2013). Between the vegetation treatments in our study, pore water CH4 concentrations were 43 ± 24 % lower when vascular plants were present (Fig. 4a), which is in line with the about 50 % lower pore water CH4 concentrations in the presence of vascular plants reported in previous studies (Wilson et al., 1989; Chanton et al., 1989; Chanton, 1991).
Whiting and Chanton (1992), on the contrary, found that clipping of aboveground vegetation reduced pore water CH4 concentrations and related their observation to root exudates, senescence, and decay of vascular plants providing additional substrates for CH4 production. This indicates that vascular plants in our study increased CH4 emissions through plant-mediated CH4 transport rather than through additional substrate supply. Efficient CH4 transport through aerenchymatous plants also shows in the high rates of CH4 lost from the peat in the presence of vascular plants (Fig. 4d). The missing difference in DOC values between plots with and without vascular plants (Fig. A6a) similarly suggests that the presence of vascular plants did not significantly affect the substrate availability for CH4 production. However, this does not rule out the possibility that certain more specific plant root exudates such as acetate could have been better associated with CH4 production (Ström et al., 2003). Additionally, higher modeled potential CH4 concentrations in the presence of vascular plants in late fall, when CH4 oxidation and transport are excluded (Fig. 4c), suggest that decaying vascular plants might increase CH4 production rates in times of leaf senescence.
(3) Plant-mediated CH4 transport showed in a preferential emission of lighter 12CH4 molecules from areas with vascular plants (Fig. 4b). Similar δ13C-CH4 values at 50 cm depth across all measurement campaigns indicate that the stable carbon isotope ratio of CH4 below the main root zone was mainly controlled by the pathway of methane production. As expected for a bog, below the rhizosphere, hydrogenotrophic methanogenesis, using H2 and CO2 to produce CH4, dominated year-round over acetoclastic methanogenesis, using acetate as an electron acceptor. This is indicated by the low δ13C-CH4 values and the high δ13C-CO2 values at 50 cm depth, which result in a carbon isotope separation between CO2 and CH4 (ϵc) of 60 to 75 compared to the values for acetoclastic methanogenesis of 24 to 29, for hydrogenotrophic methanogenesis of 49 to 95, and for CH4 oxidation of 4 to 30 (Whiticar, 1999) (Fig. A7). The accumulation of heavier 13CH4 molecules within the rhizosphere of vascular plants (at 7 and 20 cm sampling depth) compared to pore water CH4 below the rhizosphere or in the absence of vascular plants (Fig. 4b) could have been caused by different processes associated with vascular plants, such as plant transport, rhizospheric oxidation, and acetoclastic CH4 production from root exudates (Chanton, 2005; Popp et al., 1999). The strong 13C depletion of the CH4 emitted from areas with vascular plants, however, is in line with the preferential transport of lighter 12CH4 molecules through aerenchymatous plants (Chanton, 2005; Popp et al., 1999). CH4 oxidation, on the contrary, usually leads to a preferential conversion of lighter 12CH4 molecules to CO2 (Popp et al., 1999) and should thus result in higher emissions of the remaining heavier 13CH4. Therefore, the 13C depletion of emitted CH4 suggests that rhizospheric oxidation did not play a major role in our study (Chanton, 2005).
4.2 Seasonal variation in environmental and ecological controls on CH4 production, oxidation, and transport
CH4 fluxes depend on the net balance of CH4 production and CH4 oxidation. The pathways of CH4 transport further affect CH4 fluxes by influencing the percentage of produced CH4 that is either stored in the pore water, oxidized, or directly emitted to the atmosphere. It is therefore important to know how temperature, water table, and plant phenology interact to control the components of CH4 fluxes (production, oxidation, and transport) over the year.
4.2.1 CH4 production and storage
The rates of CH4 production and the CH4 dissolution and storage in the pore water interact to control the amount of CH4 that is theoretically available for CH4 emission. We hypothesize that in our study this interaction is best represented by the CH4 emissions measured at the bare peat plots (P treatment), which are directly driven by the gradient in CH4 concentrations between pore water and atmosphere in the absence of CH4 oxidation in the moss layer and plant-mediated CH4 transport.
CH4 production was mainly controlled by the peat temperature in the catotelm (Dunfield et al., 1993). CH4 emissions from the bare peat plots increased with increasing temperatures at 20 cm depth (Table A3). Higher production rates due to significantly higher peat temperatures in the catotelm likely contributed to the significantly higher CH4 emissions from the bare peat plots in late fall compared to spring (Fig. 3a, d).
At plots with intact vegetation, additional substrate supply for CH4 production from decaying vascular plants potentially dampened the decrease in CH4 emissions at the end of the growing season. An increase in CH4 production rates with leave senescence is supported by higher potential pore water concentrations at the control plots than at the vegetation removal treatments in late fall (Fig. 4c). In spring, on the contrary, potential pore water concentrations were generally lower than in fall and similar between all vegetation treatments, indicating that the additional substrate supplied by decaying vascular plants was depleted after the winter.
The release of CH4 stored in the pore water might have further obscured the temperature dependency of CH4 production during the shoulder seasons. While a temporal decoupling between the production and emission of CH4 was most obvious at the plant removal treatments, delayed emission of the CH4 produced in summer and winter likely also enhanced the shoulder seasons emissions from areas with vascular plants. The absence of aerenchymatous plants together with decreasing peat temperatures led to a buildup of high CH4 concentrations in the pore water of the vegetation removal plots, following the high production rates in the summer (Fig. 4a). A similar trend of increasing pore water concentrations also becomes visible at the control plots with progressing plant senescence in late fall. Missing or reduced plant transport lowered the efficiency with which the produced CH4 could be released to the atmosphere. At the same time, decreasing peat temperatures increased the solubility of CH4 in the pore water (Docherty et al., 2007; Guo and Rodger, 2013). The latter is supported by the decreasing rates of CH4 lost from the vegetation removal plots between summer and late fall (Fig. 4d) as well as by the increase in CH4 emissions from the bare peat with decreasing peat temperatures at 7 cm depth (Table A3). Higher diffusion rates driven by the increasing concentration gradient between pore water and atmosphere are therefore one possible explanation for the increase in CH4 emissions from the bare peat plots between summer and late fall despite a significant decrease in peat temperatures at 20 cm depth (Fig. 3a, d). High pore water concentrations in spring might furthermore indicate that CH4 that is produced in the deeper, unfrozen peat over the winter, accumulated underneath a frozen surface layer until it could be released upon spring thaw (Zona et al., 2016; Friborg et al., 1997; Alm et al., 1999; Tokida et al., 2007). The emission of a substantial part of the CH4 produced in summer and winter might be delayed by increasing solubility and decreasing transport efficiency, leading to higher CH4 emissions during the shoulder seasons than suggested by the temperature relationship of CH4 production.
4.2.2 CH4 oxidation
CH4 oxidation occurred both in the lower parts of the acrotelm as well as in the layer of living Sphagnum moss. While oxidation in the lower acrotelm mainly depended on the availability of oxygen and thus decreased with increasing water level, oxidation rates in the Sphagnum layer were mainly controlled by the substrate availability and increased with increasing water level.
CH4 oxidation in the lower acrotelm was higher at higher peat temperatures in the acrotelm and at lower water levels. The water table fell below the 4 to 5 cm thick living moss layer in summer and fall (Fig. 3e), thereby exposing up to 7 cm of the peat below the living moss to oxygen. A decrease in CH4 oxidation with rising water table (Roslev and King, 1996; Perryman et al., 2023) and with decreasing peat temperatures in the acrotelm (Whalen and Reeburgh, 1996; Zhang et al., 2020) (Table A3), therefore, is another possible explanation for the increasing CH4 emissions from the bare peat plots between summer and late fall in addition to delayed emission of produced CH4. Lower pore water concentrations as well as higher δ13C-CH4 values at 7 cm compared to 20 cm depth (Fig. 4a, b) give additional proof of CH4 oxidation in the lower acrotelm. The preferential use of the lighter 12CH4 for the conversion to CO2 by methanotrophs enriched the CH4 remaining in the pore water in 13C (Popp et al., 1999; Whiticar, 1999). In the absence of plant-mediated transport at the bare peat plots, the low isotopic fractionation by diffusive CH4 transport (Chanton, 2005) allowed us to isolate the isotopic effect of CH4 oxidation, showing as similar or higher δ13C values of emitted CH4 compared to the CH4 dissolved in the pore water of the lower acrotelm.
CH4 oxidation in the Sphagnum layer was mainly controlled by the concentration of CH4 in the pore water, similar to the CH4 emissions from the bare peat plots. Oxidation rates showed a seasonal trend similar to the one of the CH4 emissions from the bare peat plots in both 2021 and 2022 (Figs. 3a, b, A3a, b). Similar to the CH4 emissions from the bare peat plots, CH4 oxidation rates were higher at lower peat temperatures in the acrotelm and at higher temperatures in the catotelm (Tables A3, A5). This temperature dependence indicates that oxidation in the Sphagnum layer was limited mainly by the amount of CH4 available in the pore water which increases with increasing CH4 production at higher peat temperatures in the catotelm (Dunfield et al., 1993) and decreases with increasing oxidation rates at higher peat temperatures in the lower acrotelm below the moss layer (Whalen and Reeburgh, 1996; Zhang et al., 2020).
Unlike the oxidation in the lower acrotelm, oxidation rates within the living Sphagnum moss layer increased with rising water table (Fig. 2b and e, Table A5). This unexpected finding might support the stronger dependence of oxidation rates on substrate availability compared to previous studies which showed an increase in oxidation rates with increasing depth of the water table (Roslev and King, 1996; Perryman et al., 2023). Higher oxidation rates in the living moss layer at higher water levels might also be related to the symbiotic relationship between the Sphagnum moss and methanotrophs (Larmola et al., 2010; Kip et al., 2010).
The reversal of the temperature profile over the year with peat temperatures decreasing with depth in summer and increasing with depth in winter (Figs. 3d, 2a) might have further affected the balance between CH4 production and oxidation. Besides acting as a physical barrier to CH4 transport to the atmosphere when frozen, a cold surface peat layer might strongly restrict CH4 oxidation in winter, while CH4 production can continue in the warmer deeper peat layers. This might have added to the accumulation of CH4 in the pore water over winter, showing as high pore water concentrations in spring (Fig. 4a). The seasonal change in the temperature profile might thus have outweighed the stronger inhibition of CH4 production than CH4 oxidation by low temperatures (Dunfield et al., 1993).
4.2.3 CH4 transport
Plant-mediated transport of CH4 enhanced CH4 emissions even after leaf senescence. Plant transport followed a seasonal trend which was strongly controlled by the green leaf area of aerenchymatous plants (Fig. 3b, c, Table A4). Even in spring, when the LAIaer was close to zero, plant transport did, however, not cease completely but still accounted for 55 ± 31 % of diffusive CH4 emissions (Figs. 3a, b, A5). Together with the higher rates of CH4 lost from the control compared to the vegetation removal plots during the shoulder seasons (Fig. 4d), this indicates that diffusion through aerenchymatous plants continues outside of the growing season through completely senesced leaves (Roslev and King, 1996; Korrensalo et al., 2022).
Plant transport was higher at lower water levels (Fig. 3b, e, Table A4), which contradicts previous findings of a decrease in plant transport rates with decreasing water levels (Kutzbach et al., 2004; Waddington et al., 1996). However, water levels in the hollows were generally high and did not drop below the main root zone of the sedges between about 10 and 30 cm depth (Korrensalo et al., 2018a) even in summer. Any separate effect of the small variations in water levels might therefore be concealed by the covariation of the water table depth with peat temperatures and leaf area of aerenchymatous plants.
The seasonal variation in plant transport rates was best explained by the variation in peat temperatures in the catotelm (Table A4). Significant 13C depletion of the CH4 emitted from the control plots as well as similar CH4 emissions and δ13C values between light conditions indicate that gas transport through the present aerenchymatous plants was dominated by passive diffusion instead of active convective throughflow (Popp et al., 1999; Whiting and Chanton, 1996; Van Der Nat et al., 1998). Since diffusion is driven by the concentration gradient between peat and atmosphere, this might indicate a direct dependence of plant transport on pore water concentrations in the catotelm and thus on CH4 production rates, in addition to the high correlation between peat temperatures and the green leaf area of aerenchymatous plants. Continued plant transport after leaf senescence raises the question as to which environmental variables control the rates of plant transport outside of the growing season. Higher plant transport at higher availability of CH4 in the root zone provides one possible answer to this question.
By reducing pore water CH4 concentrations (Fig. 4a), plant-mediated CH4 transport affects the rates of the other emission pathways for CH4, i.e., diffusion and ebullition. At lower pore water concentrations, there is a lower concentration gradient between peat and atmosphere, reducing diffusive CH4 transport (Chanton, 2005). Lower pore water concentrations due to efficient plant transport might similarly decrease CH4 ebullition by preventing gas bubbles in the peat from becoming sufficiently large to move to the surface (e.g., van den Berg et al., 2020). This shows in the higher number of ebullition events occurring at the vegetation removal treatments compared to the intact vegetation (Fig. A8). Most ebullition events occurred from bare peat (P treatment), and their frequency followed the seasonal change in water table. This shows that ebullition is particularly important at nonvegetated plots where we expect pore water CH4 concentrations to be even higher due to the missing oxidation in the Sphagnum layer and where water tables are highest (Männistö et al., 2019).
Plant transport accounted for 83 ± 22 % of the total diffusive emission of CH4 from the control plots during all measurements in 2022 (Fig. A5). This percentage of plant-mediated CH4 transport is based on the assumption that diffusion rates are unaffected by the presence of vascular plants. The actual contribution of plant transport to the total diffusive CH4 emissions might be even higher because plant transport decreases the pore water concentrations of CH4 (Fig. 4a), reducing the concentration gradient between peat and atmosphere and thus the diffusion rates (Chanton, 2005).
4.2.4 Net CH4 emissions
CH4 emissions to the atmosphere resulted from the complex interaction of CH4 production, oxidation, and transport, each of which were in turn controlled by a set of sometimes interacting environmental and ecological variables. Net emissions from the control plots increased with increasing LAItot and LAIaer as well as with increasing peat temperatures at 7 and 20 cm depths (Table A1), which is in line with Korrensalo et al. (2018b). Contrary to Korrensalo et al. (2018b), water table depth had a significant effect on CH4 emissions with higher CH4 emission occurring at lower water tables.
Substrate-limited oxidation, to some extent, led to a self-regulating balance between CH4 production and oxidation. The strong effect of CH4 production on net CH4 emissions shows as increasing emissions with increasing peat temperatures in the catotelm (Table A1). This positive relationship was, however, weakened by the substrate limitation of CH4 oxidation. Despite significantly higher production rates related to higher temperatures in late fall, CH4 emissions from the control plots were similar between spring and late fall. This is due to significantly higher oxidation rates in late fall than in spring due to the higher substrate supply. Higher rates of CH4 oxidation thus compensated for higher CH4 production, resulting in similar net emissions of CH4. With the ratio between CH4 production and oxidation remaining close to constant over the study period, the seasonal variation in CH4 emissions was mainly controlled by the rate of plant-mediated CH4 transport.
Higher CH4 emissions at lower water levels in this study are unexpected and are most likely related to the covariation of the water table depth with peat temperatures and the leaf area of aerenchymatous plants, which exerted a stronger effect on CH4 emissions than the small variations in water table depth. Higher oxidation rates in submerged Sphagnum moss due to the symbiosis between sphagna and methanotrophs (Liebner et al., 2011) could have further contributed to higher emissions at lower water levels. An alternative explanation for the counterintuitive effect of the water table on CH4 emissions could be the degassing of CH4 that is trapped in the soil pores (even below the water table the peat is usually not fully water saturated) upon a drop in the water table (Moore et al., 1990; Moore and Roulet, 1993; Dinsmore et al., 2009). The number of chamber measurements showing episodic ebullition events, however, indicates less ebullition from the control plots following the decrease in water table between spring and summer in 2021 (Fig. A8).
The total vegetation present at the site led to a net reduction in CH4 emissions both in summer and during the shoulder seasons. Actual oxidation rates in the moss layer were probably lower in the presence of aerenchymatous plants than the rates estimated from the moss plots (PS treatment). Aerenchymatous plants reduced the pore water concentrations of CH4 (Fig. 4a) and thus the available substrate for the strongly substrate-limited CH4 oxidation in the moss layer. Despite the likely overestimation of actual oxidation rates, the decreasing effect of CH4 oxidation in the moss layer generally outweighed the increasing effect of plant transport, leading to lower mean emissions from the control plots compared to the mean emissions from the bare peat plots during all measurement campaigns in 2021 and 2022, except for July 2022 (Fig. A3a, b).
This study investigated the environmental and ecological controls on the seasonal dynamics of CH4 emissions from the wet hollows of a boreal bog, with a particular focus on shoulder season processes. Seasonal variations in CH4 emissions resulted from complex interactions between CH4 production, oxidation, and transport, which in turn were controlled by combinations of peat temperatures, vegetation properties, and water table depth. During the shoulder seasons, several processes dampened the effect of decreasing CH4 production with decreasing peat temperatures on net CH4 emissions, including continued plant-mediated CH4 transport through senesced leaves, substrate supply for CH4 production from decaying vascular plants, delayed emission of a part of the CH4 produced in summer and winter, and substrate-limited CH4 oxidation in the Sphagnum moss. The temporal decoupling between CH4 production and emission highlights the importance of year-round flux measurements to reliably capture annual CH4 budgets. High rates of CH4 oxidation in the Sphagnum layer and of CH4 transport through aerenchymatous plants in summer and shoulder seasons underline the crucial role of vegetation in controlling net CH4 fluxes. Our study points towards the high need to refine the current parameterization of seasonal dynamics in CH4 emissions in process-based models. Replacing simple temperature dependencies of CH4 emissions by the interaction of separately modeled components of CH4 fluxes (CH4 production, oxidation, and transport) will greatly improve our estimates of CH4 emissions from boreal peatlands, particularly in the shoulder seasons, and will thus work against an underestimation of cold-season CH4 emissions.
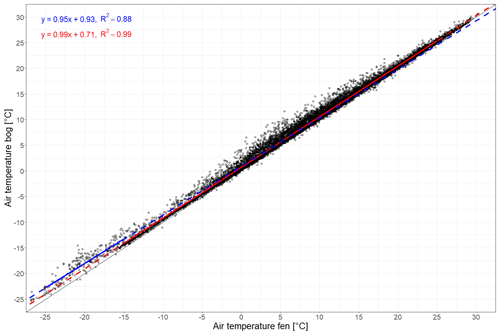
Figure A1Linear regression between air temperatures recorded hourly at Siikaneva bog and at Siikaneva fen (https://smear.avaa.csc.fi/download, last access: 13 August 2024; Station SMEAR II Siikaneva 1 (fen) and 2 (bog) wetland) between 2012 and 2016. The air temperature was fit using two linear regressions with an inflection point at −15 °C at the fen site. The linear regressions for temperatures below −15 °C and equal to or above −15 °C are given in blue and red, respectively.
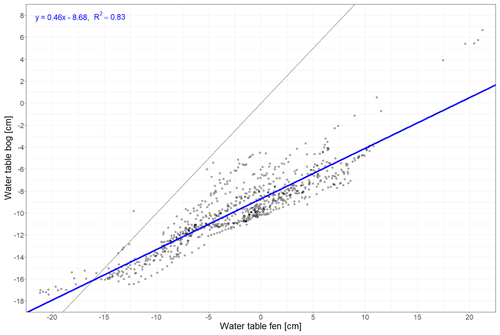
Figure A2Linear regression between daily water table depths recorded at Siikaneva bog and Siikaneva fen (https://smear.avaa.csc.fi/download; Station SMEAR II Siikaneva 1 (fen) and 2 (bog) wetland) between 2012 and 2016.
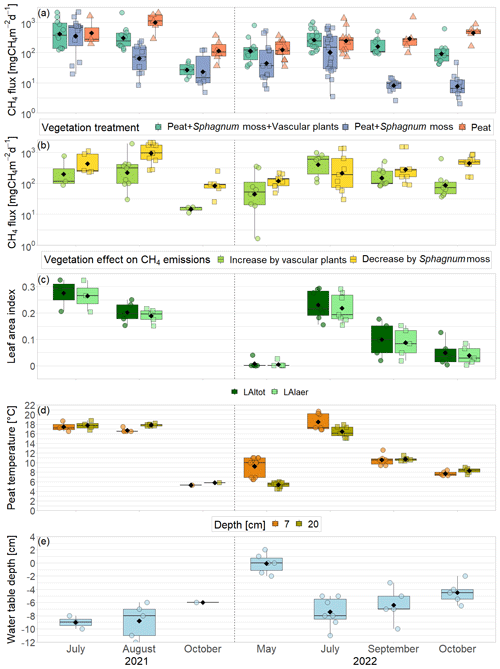
Figure A3CH4 emissions from the vegetation removal experiment (a), vascular plant effects and Sphagnum layer effects on CH4 emissions (b) by measurement campaigns in 2021 and 2022, displayed on logarithmic axes. Five negative values for vascular plant effects in 2021 ranging from −7 to −401 mg CH4 m−2 d−1 at simultaneous positive values of the Sphagnum effect are not shown. Leaf area index of green vascular plants for total vascular vegetation (LAItot) and aerenchymatous plants only (LAIaer) (c), peat temperatures (d), and water table depth (e). Markers show the individual values; the boxplot shows the median (horizontal line), 25th percentile, and 75th percentile (hinges), as well as the smallest and largest values, no more than 1.5 times the interquartile range from the hinges (whiskers). Values above/below the whiskers are classified as outliers. Mean values are given as black diamonds.
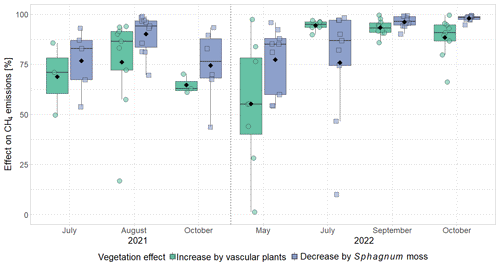
Figure A4Relative enhancing effect of vascular plants and decreasing effect of Sphagnum moss on CH4 emissions by measurement campaign in 2021 and 2022. Cases where emissions from the control plots were lower than from the moss plots (negative vascular plant effect) were excluded from this figure (five values in 2021).
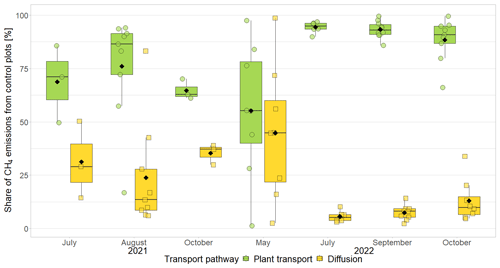
Figure A5Percentage of CH4 emitted via diffusion (emissions from the moss-only (PS) plots) and via plant transport (emissions from the control (PSV) plots minus emissions from the moss-only plots) of the total CH4 emissions (from the control plots) after ebullition events were excluded. Cases where emissions from the control plots were lower than from the moss plots (negative plant transport) were excluded from this figure (five values in 2021).
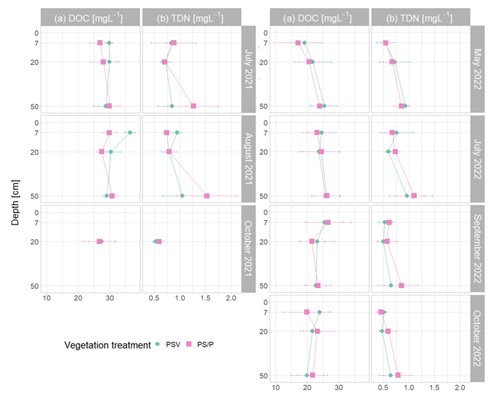
Figure A6Mean and standard deviation of dissolved organic carbon (DOC) (a) and total dissolved nitrogen (TDN) (b) by sampling depth, measurement campaign, and vegetation treatment. Control plots and vegetation treatments are the following. PSV: intact vegetation including Sphagnum mosses and vascular plants; PS: Sphagnum moss with vascular plants removed; P: peat with all vegetation removed. Pore water data are combined for the PS and P plots because the vegetation removal treatments were collocated.
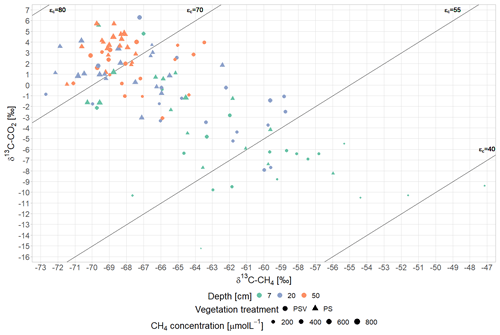
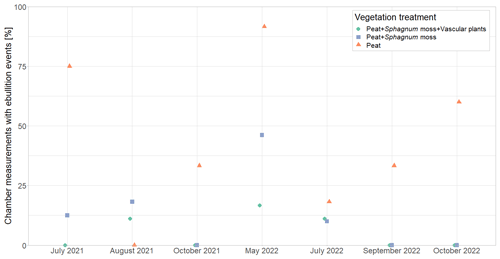
Figure A8Number of flux measurements during which one or more ebullition events were visually detected, normalized by the total number of measurements. Measurements that were discarded from flux calculation due to excessive ebullition are included in this figure.
Table A1Parameter estimates for linear models for CH4 fluxes from the control plots (PSV). Estimate values, standard error (SE), degrees of freedom (DOF), test statistics (t, p, and significance level (signif.)) are given to the fixed predictors of the model as well as conditional pseudo-R-squared for generalized mixed effects models (R2). The model that best explained the variation in the data is highlighted in bold font. The significance level of the effects of total leaf area index (LAItot), leaf area index of aerenchymatous plants (LAIaer), peat temperatures at 7 (Tpeat (7 cm)) and 20 cm depth (Tpeat (20 cm)), and water table depth (WTD) on the CH4 fluxes is indicated by the number of asterisks as follows. : , : , *: .
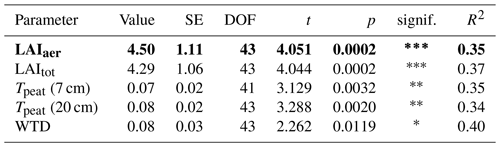
Table A2Parameter estimates for linear models for CH4 fluxes from the moss treatment (PS). Estimate values, standard error (SE), degrees of freedom (DOF), test statistics (t, p, and significance level (signif.)) are given to the fixed predictors of the model as well as conditional pseudo-R-squared for generalized mixed effects models (R2). The model that best explained the variation in the data is highlighted in bold font. The significance level of the effects of peat temperatures at 7 (Tpeat (7 cm)) and 20 cm depth (Tpeat (20 cm)), and water table depth (WTD) on the CH4 fluxes is indicated by the number of asterisks as follows. : , : , *: .
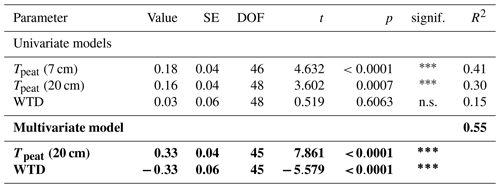
n.s. – not significant.
Table A3Parameter estimates for linear models for CH4 fluxes from the bare peat treatment (P). Estimate values, standard error (SE), degrees of freedom (DOF), test statistics (t, p, and significance level (signif.)) are given to the fixed predictors of the model as well as conditional pseudo-R-squared for generalized mixed effects models (R2). The model that best explained the variation in the data is highlighted in bold font. The significance level of the effects of peat temperatures at 7 (Tpeat (7 cm)) and 20 cm depth (Tpeat (20 cm)), and water table depth (WTD) on the CH4 fluxes is indicated by the number of asterisks as follows. : , : , *: .
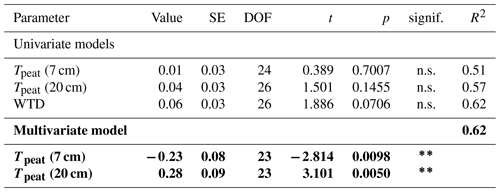
n.s. – not significant.
Table A4Parameter estimates for linear models for the effect of vascular plants on CH4 fluxes. Estimate values, standard error (SE), degrees of freedom (DOF), test statistics (t, p, and significance level (signif.)) are given to the fixed predictors of the model as well as conditional pseudo-R-squared for generalized mixed effects models (R2). The model that best explained the variation in the data is highlighted in bold font. The significance level of the effects of total leaf area index (LAItot), leaf area index of aerenchymatous plants (LAIaer), peat temperatures at 7 (Tpeat (7 cm)) and 20 cm depth (Tpeat (20 cm)), and water table depth (WTD) on the vascular plant effect is indicated by the number of asterisks as follows. : , : , *: .
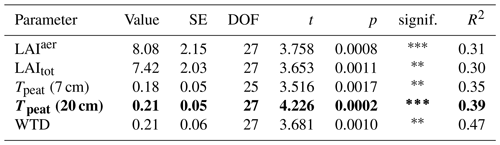
Table A5Parameter estimates for linear models for the effect of Sphagnum moss on CH4 fluxes. Estimate values, standard error (SE), degrees of freedom (DOF), test statistics (t, p, and significance level (signif.)) are given to the fixed predictors of the model as well as conditional pseudo-R-squared for generalized mixed effects models (R2). The model that best explained the variation in the data is highlighted in bold font. The significance level of the effects of peat temperatures at 7 (Tpeat (7 cm)) and 20 cm depth (Tpeat (20 cm)), and water table depth (WTD) on the Sphagnum effect is indicated by the number of asterisks as follows. : , : , *: .
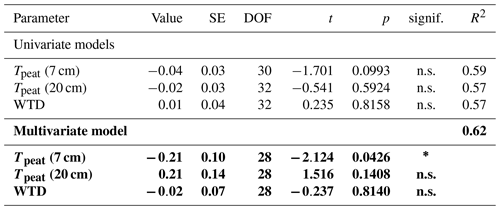
n.s. – not significant.
Table A6Significant differences in measured concentrations of CH4 dissolved in the pore water (CH4 conc.) between the categories of measurement campaign, vegetation treatment, or sampling depth while the remaining categories are constant. Estimate values, standard error (SE), and test statistics (z, adjusted p, and significance level (signif.)) are given as resulting from Tukey's HSD test. The significance level of the differences is indicated by the number of asterisks as follows. : , : , *: .

Table A7Significant differences in δ13C values of the CH4 dissolved in the pore water (diss.) and emitted from the peat (em.) between the categories of measurement campaign, vegetation treatment, or sampling depth while the remaining categories are constant. Estimate values, standard error (SE), and test statistics (z, adjusted p, and significance level (signif.)) are given as resulting from Tukey's HSD test. The significance level of the differences is indicated by the number of asterisks as follows. ***: , : , *: .
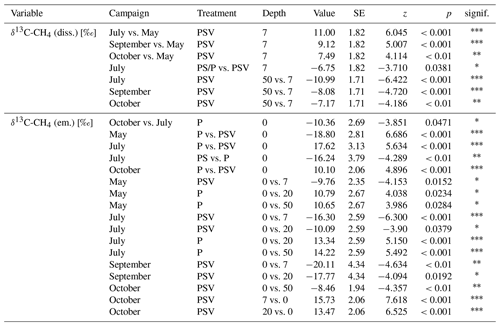
Table A8Significant differences in modeled potential concentrations of CH4 dissolved in the pore water in the absence of CH4 oxidation and transport (pot CH4 conc.) between the categories of measurement campaign, vegetation treatment, or sampling depth while the remaining categories are constant. Estimate values, standard error (SE), and test statistics (z, adjusted p, and significance level (signif.)) are given as resulting from Tukey's HSD test. The significance level of the differences is indicated by the number of asterisks as follows. : , : , *: .
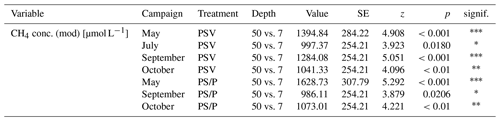
Table A9Significant differences in the fraction of CH4 lost from the peat through oxidation or transport (fCH4 lost) between the categories of measurement campaign, vegetation treatment, or sampling depth while the remaining categories are constant. Estimate values, standard error (SE), and test statistics (z, adjusted p, and significance level (signif.)) are given as resulting from Tukey's HSD test. The significance level of the differences is indicated by the number of asterisks as follows. : , : , *: .
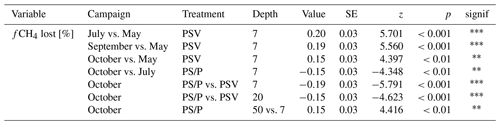
Similar to Dorodnikov et al. (2013), stable carbon isotope modeling resulted in unrealistic negative fractions of CH4 oxidation in the surface peat of the control plots. This was probably due to a high sensitivity of the fraction of CH4 oxidized to the choice of isotopic fractionation factors for oxidation and plant transport, αox and αtrans. Due to this high sensitivity as well as the high variability between ecosystems, temperature, and moisture conditions, large uncertainties can be introduced into estimates of oxidation rates when literature values are used for αox (Cabral et al., 2010; Gebert and Streese-Kleeberg, 2017). Instead, αox should be determined specifically for each research site and corrected for its temperature dependency (Chanton et al., 2008). This can be done using headspace samples from incubations or chamber measurements at sites with net CH4 uptake (King et al., 1989). Since none of our measurement plots showed a net uptake of CH4, we could not determine αox specifically for our research site from our chamber measurements. Furthermore, CH4 emissions from the moss plots (PS treatments) were generally low so that most estimates for stable isotope carbon ratios of emitted CH4 were not reliable. We therefore could not identify the fractionating effect of oxidation processes directly from the flux measurements on this treatment. Besides the αox value being problematic, the results from the control plots showing negative fractions of CH4 oxidization probably indicate an underestimation of the isotopic fractionation of CH4 during to plant transport (αtrans) at our measurement plots; that is, plant transport seems to be strongly fractionating at the measurement site. Given the high uncertainty in the two key model parameters, αox and αtrans, we ran into the problem of not being able to constrain the model. From this, we decided that using the isotope model to estimate fractions of CH4 oxidation and transport was not feasible.
The data sets used in this paper are available at https://doi.org/10.1594/PANGAEA.965402 (Jentzsch et al., 2024).
EST, AK, and EM designed and installed the experimental setup. CCT, AK, LvD, and KJ planned the measurements and sampling performed during the field campaigns. KJ conducted the flux measurements and the pore water sampling and processed the flux data. EM performed the field sampling, measurements, and processing of the LAI data. MEM and KJ analyzed the pore water gas samples. MEM wrote the R scripts to process and correct the gas concentrations and stable carbon isotope ratios of the pore water gas samples. CCT, LvD, and KJ processed the meteorological data. KJ performed the data analysis. The manuscript was written by KJ and commented on by all authors. CCT and CK supervised the project.
The contact author has declared that none of the authors has any competing interests.
Publisher’s note: Copernicus Publications remains neutral with regard to jurisdictional claims made in the text, published maps, institutional affiliations, or any other geographical representation in this paper. While Copernicus Publications makes every effort to include appropriate place names, the final responsibility lies with the authors.
John M. Zobitz is acknowledged for assisting with the R script used for Keeling plot calculations. We would like to thank Hyytiälä Forest Research Station and its staff for research facilities and for the support and logistics during the fieldwork. Special thanks to Mélissa Laurent, Mackenzie Baysinger, Jonas Vollmer, Lion Golde, Finn Overduin, Jakob Reif, Johanna Schwarzer, and Sarah Wocheslander for assistance in the fieldwork. We also thank the researchers and lab technicians at Alfred Wegener Institute, Potsdam, and at the University of Eastern Finland, Kuopio, who assisted with the laboratory analyses.
The contribution of Katharina Jentzsch, Lona van Delden, and Claire C. Treat is part of the FluxWIN project, funded with a Starting Grant by the European Research Council (ERC) (ID 851181). The work of Maija E. Marushchak was supported by the Academy of Finland funded projects PANDA (no. 317054) and Thaw-N (no. 349503).
The article processing charges for this open-access publication were covered by the Alfred-Wegener-Institut Helmholtz-Zentrum für Polar- und Meeresforschung.
This paper was edited by Steven Bouillon and reviewed by Pierre Taillardat and one anonymous referee.
Ahti, T., Hämet-Ahti, L., and Jalas, J.: Vegetation zones and their sections in northwestern Europe, Ann. Bot. Fenn., 5, 169–211, http://www.jstor.org/stable/23724233 (last access: 14 August 2024), 1968. a
Alekseychik, P., Korrensalo, A., Mammarella, I., Launiainen, S., Tuittila, E.-S., Korpela, I., and Vesala, T.: Carbon balance of a Finnish bog: temporal variability and limiting factors based on 6 years of eddy-covariance data, Biogeosciences, 18, 4681–4704, https://doi.org/10.5194/bg-18-4681-2021, 2021. a
Alekseychik, P., Kolari, P., Rinne, J., Haapanala, S., Laakso, H., Taipale, R., Matilainen, T., Salminen, T., Levula, J., and Tuittila, E.-S.: SMEAR II Siikaneva 1 wetland meteorology and soil, University of Helsinki, Institute for Atmospheric and Earth System Research, https://doi.org/10.23729/08d89ada-d152-4c8b-8db4-ae8a8f17f825, 2023. a
Alm, J., Saarnio, S., Nykänen, H., Silvola, J., and Martikainen, P.: Winter CO2, CH4 and N2O fluxes on some natural and drained boreal peatlands, Biogeochemistry, 44, 163–186, https://doi.org/10.1007/BF00992977, 1999. a
Blanc-Betes, E., Welker, J. M., Sturchio, N. C., Chanton, J. P., and Gonzalez-Meler, M. A.: Winter precipitation and snow accumulation drive the methane sink or source strength of Arctic tussock tundra, Glob. Change Biol., 22, 2818–2833, https://doi.org/10.1111/gcb.13242, 2016. a, b
Bu, X., Krause, S. M., Gu, X., Tian, J., and Zhou, X.: Ethylene rather than acetylene inhibits soil methane oxidation rates in a subtropical evergreen forest, Soil Biol. Biochem., 135, 10–12, https://doi.org/10.1016/j.soilbio.2019.04.001, 2019. a
Cabral, A. R., Capanema, M. A., Gebert, J., Moreira, J. F., and Jugnia, L. B.: Quantifying microbial methane oxidation efficiencies in two experimental landfill biocovers using stable isotopes, Water Air Soil Poll., 209, 157–172, https://doi.org/10.1007/s11270-009-0188-4, 2010. a
Chan, A. and Parkin, T.: Evaluation of potential inhibitors of methanogenesis and methane oxidation in a landfill cover soil, Soil Biol. Biochem., 32, 1581–1590, https://doi.org/10.1016/S0038-0717(00)00071-7, 2000. a
Chanton, J. P.: Effects of vegetation on methane flux, reservoir, and isotopic composition, in: Trace Gas Emissions by Plants, edited by: Sharkey, T. D., Holland, E. A., and Mooney, H. A., Academic Press, San Diego, USA (CA), 65–92, https://doi.org/10.1016/B978-0-12-639010-0.50008-X, 1991. a
Chanton, J. P.: The effect of gas transport on the isotope signature of methane in wetlands, Org. Geochem., 36, 753–768, https://doi.org/10.1016/j.orggeochem.2004.10.007, 2005. a, b, c, d, e, f
Chanton, J. P., Martens, C. S., and Kelley, C. A.: Gas transport from methane-saturated, tidal freshwater and wetland sediments, Limnol. Oceanogr., 34, 807–819, https://doi.org/10.4319/lo.1989.34.5.0807, 1989. a
Chanton, J. P., Powelson, D. K., Abichou, T., Fields, D., and Green, R.: Effect of temperature and oxidation rate on carbon-isotope fractionation during methane oxidation by landfill cover materials, Environ. Sci. Technol., 42, 7818–7823, https://doi.org/10.1021/es801221y, 2008. a
Corbett, J. E., Tfaily, M. M., Burdige, D. J., Cooper, W. T., Glaser, P. H., and Chanton, J. P.: Partitioning pathways of CO2 production in peatlands with stable carbon isotopes, Biogeochemistry, 114, 327–340, https://doi.org/10.1007/s10533-012-9813-1, 2013. a, b
Corbett, J. E., Tfaily, M. M., Burdige, D. J., Glaser, P. H., and Chanton, J. P.: The relative importance of methanogenesis in the decomposition of organic matter in northern peatlands, J. Geophys. Res.-Biogeosci., 120, 280–293, https://doi.org/10.1002/2014JG002797, 2015. a
Dinsmore, K. J., Skiba, U. M., Billett, M. F., and Rees, R. M.: Effect of water table on greenhouse gas emissions from peatland mesocosms, Plant Soil, 318, 229–242, https://doi.org/10.1007/s11104-008-9832-9, 2009. a
Dise, N. B., Gorham, E., and Verry, E. S.: Environmental factors controlling methane emissions from peatlands in northern Minnesota, J. Geophys. Res.-Atmos., 98, 10583–10594, 1993. a
Docherty, H., Galindo, A., Sanz, E., and Vega, C.: Investigation of the salting out of methane from aqueous electrolyte solutions using computer simulations, J. Phys. Chem. B, 111, 8993–9000, https://doi.org/10.1021/jp0678249, 2007. a
Dorodnikov, M., Knorr, K.-H., Kuzyakov, Y., and Wilmking, M.: Plant-mediated CH4 transport and contribution of photosynthates to methanogenesis at a boreal mire: a 14C pulse-labeling study, Biogeosciences, 8, 2365–2375, https://doi.org/10.5194/bg-8-2365-2011, 2011. a, b
Dorodnikov, M., Marushchak, M., Biasi, C., and Wilmking, M.: Effect of microtopography on isotopic composition of methane in porewater and efflux at a boreal peatland, Boreal Environ. Res., 18, 269–279, 2013. a, b, c, d
Dunfield, P., Dumont, R., Moore, T. R., et al.: Methane production and consumption in temperate and subarctic peat soils: response to temperature and pH, Soil Biol. Biochem., 25, 321–326, https://doi.org/10.1016/0038-0717(93)90130-4, 1993. a, b, c, d, e
Finnish Meteorological Institute: Download observations, https://en.ilmatieteenlaitos.fi/download-observations (last access: 15 August 2023), 2023a. a
Finnish Meteorological Institute: Seasons in Finland, https://en.ilmatieteenlaitos.fi/seasons-in-finland (last access: 16 August 2023), 2023b. a
Forbrich, I., Kutzbach, L., Hormann, A., and Wilmking, M.: A comparison of linear and exponential regression for estimating diffusive CH4 fluxes by closed-chambers in peatlands, Soil Biol. Biochem., 42, 507–515, https://doi.org/10.1016/j.soilbio.2009.12.004, 2010. a, b
Frenzel, P. and Karofeld, E.: CH4 emission from a hollow-ridge complex in a raised bog: The role of CH4 production and oxidation, Biogeochemistry, 51, 91–112, https://doi.org/10.1023/A:1006351118347, 2000. a, b, c, d, e
Frenzel, P. and Rudolph, J.: Methane emission from a wetland plant: the role of CH4 oxidation in Eriophorum, Plant Soil, 202, 27–32, https://doi.org/10.1023/A:1004348929219, 1998. a
Friborg, T., Christensen, T., and Søgaard, H.: Rapid response of greenhouse gas emission to early spring thaw in a subarctic mire as shown by micrometeorological techniques, Geophys. Res. Lett., 24, 3061–3064, https://doi.org/10.1029/97GL03024, 1997. a
Galera, L. d. A., Eckhardt, T., Beer, C., Pfeiffer, E.-M., and Knoblauch, C.: Ratio of in situ CO2 to CH4 production and its environmental controls in polygonal tundra soils of Samoylov Island, Northeastern Siberia, J. Geophys. Res.-Biogeo., 128, e2022JG006956, https://doi.org/10.1029/2022JG006956, 2023. a
Gebert, J. and Streese-Kleeberg, J.: Coupling Stable Isotope Analysis with Gas Push-Pull Tests to Derive In Situ Values for the Fractionation Factor αox Associated with the Microbial Oxidation of Methane in Soils, Soil Sci. Soc. Am. J., 81, 1107–1114, https://doi.org/10.2136/sssaj2016.11.0387, 2017. a
Guo, G.-J. and Rodger, P. M.: Solubility of aqueous methane under metastable conditions: Implications for gas hydrate nucleation, J. Phys. Chem. B, 117, 6498–6504, https://doi.org/10.1021/jp3117215, 2013. a
Hanson, R. S. and Hanson, T. E.: Methanotrophic bacteria, Microbiol. Rev., 60, 439–471, https://doi.org/10.1128/mr.60.2.439-471.1996, 1996. a
Henneberg, A., Brix, H., and Sorrell, B. K.: The interactive effect of Juncus effusus and water table position on mesocosm methanogenesis and methane emissions, Plant Soil, 400, 45–54, https://doi.org/10.1007/s11104-015-2707-y, 2016. a
Hoffmann, M., Schulz-Hanke, M., Garcia Alba, J., Jurisch, N., Hagemann, U., Sachs, T., Sommer, M., and Augustin, J.: A simple calculation algorithm to separate high-resolution CH4 flux measurements into ebullition- and diffusion-derived components, Atmos. Meas. Tech., 10, 109–118, https://doi.org/10.5194/amt-10-109-2017, 2017. a
Hutchinson, G. and Mosier, A.: Improved soil cover method for field measurement of nitrous oxide fluxes, Soil Sci. Soc. Am. J., 45, 311–316, https://doi.org/10.2136/sssaj1981.03615995004500020017x, 1981. a
Ito, A., Li, T., Qin, Z., Melton, J., Tian, H., Kleinen, T., Zhang, W., Zhang, Z., Joos, F., Ciais, P., Hopcroft, P. O., Beerling, D. J., Liu, X., Zhuang, Q., Zhu, Q., Peng, C., Chang, K.-Y., Fluet-Chouinard, E., McNicol, G., Patra, P., Poulter, B., Sitch, S., Riley, W., and Zhu, Q.: Cold-season methane fluxes simulated by GCP-CH4 Models, Geophys. Res. Lett., 50, e2023GL103037, https://doi.org/10.1029/2023GL103037, 2023. a
Jentzsch, K., Männistö, E., Marushchak, M. E., Korrensalo, A., van Delden, L., Tuittila, E.-S., and Treat, C. C.: Seasonal chamber measurements of CH4 fluxes and their isotopic composition along with environmental conditions at vegetation removal experiments in hollows at Siikaneva bog, Finland, in 2021 and 2022, PANGAEA [data set], https://doi.org/10.1594/PANGAEA.965402, 2024. a
Joabsson, A., Christensen, T. R., and Wallén, B.: Vascular plant controls on methane emissions from northern peatforming wetlands, Trend. Ecol. Evol., 14, 385–388, https://doi.org/10.1016/S0169-5347(99)01649-3, 1999. a, b
Keeling, C. D.: The concentration and isotopic abundances of atmospheric carbon dioxide in rural areas, Geochim. Cosmochim. Ac., 13, 322–334, https://doi.org/10.1016/0016-7037(58)90033-4, 1958. a
Keeling, C. D.: The concentration and isotopic abundances of carbon dioxide in rural and marine air, Geochim. Cosmochim. Ac., 24, 277–298, https://doi.org/10.1016/0016-7037(61)90023-0, 1961. a
King, S. L., Quay, P. D., and Lansdown, J. M.: The 13C/12C kinetic isotope effect for soil oxidation of methane at ambient atmospheric concentrations, J. Geophys. Res.-Atmos., 94, 18273–18277, https://doi.org/10.1029/JD094iD15p18273, 1989. a
Kip, N., Van Winden, J. F., Pan, Y., Bodrossy, L., Reichart, G.-J., Smolders, A. J., Jetten, M. S., Damsté, J. S. S., and Op den Camp, H. J.: Global prevalence of methane oxidation by symbiotic bacteria in peat-moss ecosystems, Nat. Geosci., 3, 617–621, https://doi.org/10.1038/ngeo939, 2010. a, b, c, d, e
Kirschke, S., Bousquet, P., Ciais, P., Saunois, M., Canadell, J. G., Dlugokencky, E. J., Bergamaschi, P., Bergmann, D., Blake, D. R., Bruhwiler, L., Cameron-Smith, P., Castaldi, S., Chevallier, F., Feng, L., Fraser, A., Heimann, M., Hodson, E. L., Houweling, S., Josse, B., Fraser, P. J., Krummel, P. B., Lamarque, J.-F., Langenfeld, R. L., Le Quéré, C., Naik, V., O'Doherty, S., Palmer, P. I., Pison, I., Plummer, D., Poulter, B., Prinn, R. G., Rigby, M., Ringeval, B., Santini, M., Schmidt, M., Shindell, D. T., Simpson, I. J., Spahni, R., Steele, L. P., Strode, S. A., Sudo, K., Szopa, S., van der Werf, G. R., Voulgarakis, A., van Weele, M., Weiss, R. F., Williams, J. E., and Zeng, G.: Three decades of global methane sources and sinks, Nat. Geosci., 6, 813–823, https://doi.org/10.1038/NGEO1955, 2013. a
Knoblauch, C., Spott, O., Evgrafova, S., Kutzbach, L., and Pfeiffer, E.-M.: Regulation of methane production, oxidation, and emission by vascular plants and bryophytes in ponds of the northeast Siberian polygonal tundra, J. Geophys. Res.-Biogeo., 120, 2525–2541, https://doi.org/10.1002/2015JG003053, 2015. a, b
Korrensalo, A., Kettunen, L., Laiho, R., Alekseychik, P., Vesala, T., Mammarella, I., and Tuittila, E.-S.: Boreal bog plant communities along a water table gradient differ in their standing biomass but not their biomass production, J. Veg. Sci., 29, 136–146, https://doi.org/10.1111/jvs.12602, 2018a. a, b, c
Korrensalo, A., Männistö, E., Alekseychik, P., Mammarella, I., Rinne, J., Vesala, T., and Tuittila, E.-S.: Small spatial variability in methane emission measured from a wet patterned boreal bog, Biogeosciences, 15, 1749–1761, https://doi.org/10.5194/bg-15-1749-2018, 2018b. a, b, c, d, e
Korrensalo, A., Mammarella, I., Alekseychik, P., Vesala, T., and Tuittila, E.: Plant mediated methane efflux from a boreal peatland complex, Plant Soil, 471, 375–392, https://doi.org/10.1007/s11104-021-05180-9, 2022. a, b, c, d
Kotiaho, M., Fritze, H., Merilä, P., Tuomivirta, T., Väliranta, M., Korhola, A., Karofeld, E., and Tuittila, E.-S.: Actinobacteria community structure in the peat profile of boreal bogs follows a variation in the microtopographical gradient similar to vegetation, Plant Soil, 369, 103–114, https://doi.org/10.1007/s11104-012-1546-3, 2013. a
Kuhn, M., Varner, R., Bastviken, D., Crill, P., MacIntyre, S., Turetsky, M., Anthony, K., McGuire, D., and Olefeldt, D.: BAWLD-CH4: Methane fluxes from boreal and arctic ecosystems, Arctic Data Center, https://doi.org/10.18739/A2DN3ZX1R [data set], 2021. a
Kutzbach, L., Wagner, D., and Pfeiffer, E.-M.: Effect of microrelief and vegetation on methane emission from wet polygonal tundra, Lena Delta, Northern Siberia, Biogeochemistry, 69, 341–362, https://doi.org/10.1023/B:BIOG.0000031053.81520.db, 2004. a, b
Lai, D.: Methane dynamics in northern peatlands: a review, Pedosphere, 19, 409–421, https://doi.org/10.1016/S1002-0160(09)00003-4, 2009. a
Laine, A., Wilson, D., Kiely, G., and Byrne, K. A.: Methane flux dynamics in an Irish lowland blanket bog, Plant Soil, 299, 181–193, https://doi.org/10.1007/s11104-007-9374-6, 2007. a
Larmola, T., Tuittila, E.-S., Tiirola, M., Nykänen, H., Martikainen, P. J., Yrjälä, K., Tuomivirta, T., and Fritze, H.: The role of Sphagnum mosses in the methane cycling of a boreal mire, Ecology, 91, 2356–2365, https://doi.org/10.1890/09-1343.1, 2010. a, b, c, d
Lide, D. R. and Frederikse, H. P. R. (Eds.): CRC Handbook of Chemistry and Physics, 76th Edn., CRC press Inc., Boca Raton, USA (FL), ISBN 978-0849304767, 1995. a
Liebner, S., Zeyer, J., Wagner, D., Schubert, C., Pfeiffer, E.-M., and Knoblauch, C.: Methane oxidation associated with submerged brown mosses reduces methane emissions from Siberian polygonal tundra, J. Ecol., 99, 914–922, https://doi.org/10.1111/j.1365-2745.2011.01823.x, 2011. a, b, c
Liptay, K., Chanton, J., Czepiel, P., and Mosher, B.: Use of stable isotopes to determine methane oxidation in landfill cover soils, J. Geophys. Res.-Atmos., 103, 8243–8250, https://doi.org/10.1029/97JD02630, 1998. a
Männistö, E., Korrensalo, A., Alekseychik, P., Mammarella, I., Peltola, O., Vesala, T., and Tuittila, E.-S.: Multi-year methane ebullition measurements from water and bare peat surfaces of a patterned boreal bog, Biogeosciences, 16, 2409–2421, https://doi.org/10.5194/bg-16-2409-2019, 2019. a
Melton, J. R., Wania, R., Hodson, E. L., Poulter, B., Ringeval, B., Spahni, R., Bohn, T., Avis, C. A., Beerling, D. J., Chen, G., Eliseev, A. V., Denisov, S. N., Hopcroft, P. O., Lettenmaier, D. P., Riley, W. J., Singarayer, J. S., Subin, Z. M., Tian, H., Zürcher, S., Brovkin, V., van Bodegom, P. M., Kleinen, T., Yu, Z. C., and Kaplan, J. O.: Present state of global wetland extent and wetland methane modelling: conclusions from a model inter-comparison project (WETCHIMP), Biogeosciences, 10, 753–788, https://doi.org/10.5194/bg-10-753-2013, 2013. a
Moore, T. and Knowles, R.: Methane emissions from fen, bog and swamp peatlands in Quebec, Biogeochemistry, 11, 45–61, https://doi.org/10.1007/BF00000851, 1990. a
Moore, T. and Roulet, N.: Methane flux: water table relations in northern wetlands, Geophys. Res. Lett., 20, 587–590, https://doi.org/10.1029/93GL00208, 1993. a
Moore, T., Roulet, N., and Knowles, R.: Spatial and temporal variations of methane flux from subarctic/northern boreal fens, Global Biogeochem. Cyc., 4, 29–46, https://doi.org/10.1029/GB004i001p00029, 1990. a
Neubauer, S. C.: Global warming potential is not an ecosystem property, Ecosystems, 24, 2079–2089, https://doi.org/10.1007/s10021-021-00631-x, 2021. a
Noyce, G. L., Varner, R. K., Bubier, J. L., and Frolking, S.: Effect of Carex rostrata on seasonal and interannual variability in peatland methane emissions, J. Geophys. Res.-Biogeo., 119, 24–34, https://doi.org/10.1002/2013JG002474, 2014. a, b
Op de Beeck, M., Sabbatini, S., and Papale, D.: ICOS Ecosystem Instructions for Ancillary Vegetation Measurements in Mires (Version 20200316), ICOS Ecosystem Thematic Centre, https://doi.org/10.18160/6mkw-3s2r, 2017. a
Pedersen, A., Petersen, S., and Schelde, K.: A comprehensive approach to soil-atmosphere trace-gas flux estimation with static chambers, Eur. J. Soil Sci., 61, 888–902, https://doi.org/10.1111/j.1365-2389.2010.01291.x, 2010. a
Perryman, C. R., McCalley, C. K., Shorter, J. H., Perry, A. L., White, N., Dziurzynski, A., and Varner, R. K.: Effect of Drought and Heavy Precipitation on CH4 Emissions and δ13C–CH4 in a Northern Temperate Peatland, Ecosystems, 27, 1–18, https://doi.org/10.1007/s10021-023-00868-8, 2023. a, b
Pirk, N., Mastepanov, M., Parmentier, F.-J. W., Lund, M., Crill, P., and Christensen, T. R.: Calculations of automatic chamber flux measurements of methane and carbon dioxide using short time series of concentrations, Biogeosciences, 13, 903–912, https://doi.org/10.5194/bg-13-903-2016, 2016. a
Popp, T. J., Chanton, J. P., Whiting, G. J., and Grant, N.: Methane stable isotope distribution at a Carex dominated fen in north central Alberta, Global Biogeochem. Cy., 13, 1063–1077, https://doi.org/10.1029/1999GB900060, 1999. a, b, c, d, e
Poulter, B., Bousquet, P., Canadell, J. G., Ciais, P., Peregon, A., Saunois, M., Arora, V. K., Beerling, D. J., Brovkin, V., Jones, C. D., Joos, F., Gedney, N., Ito, A., Kleinen, T., Koven, C. D., McDonald, K., Melton, J. R., Peng, C., Peng, S., Prigent, C., Schroeder, R., Riley, W. J., Saito, M., Spahni, R., Tian, H., Taylor, L., Viovy, N., Wilton, D., Wiltshire, A., Xu, X., Zhang, B., Zhang, Z., and Zhu, Q.: Global wetland contribution to 2000–2012 atmospheric methane growth rate dynamics, Environ. Res. Lett., 12, 094013, https://doi.org/10.1088/1748-9326/aa8391, 2017. a
Riutta, T., Korrensalo, A., Laine, A. M., Laine, J., and Tuittila, E.-S.: Interacting effects of vegetation components and water level on methane dynamics in a boreal fen, Biogeosciences, 17, 727–740, https://doi.org/10.5194/bg-17-727-2020, 2020. a, b, c, d
Roslev, P. and King, G. M.: Regulation of methane oxidation in a freshwater wetland by water table changes and anoxia, FEMS Microbiol. Ecol., 19, 105–115, https://doi.org/10.1016/0168-6496(95)00084-4, 1996. a, b, c, d
Saunois, M., Stavert, A. R., Poulter, B., Bousquet, P., Canadell, J. G., Jackson, R. B., Raymond, P. A., Dlugokencky, E. J., Houweling, S., Patra, P. K., Ciais, P., Arora, V. K., Bastviken, D., Bergamaschi, P., Blake, D. R., Brailsford, G., Bruhwiler, L., Carlson, K. M., Carrol, M., Castaldi, S., Chandra, N., Crevoisier, C., Crill, P. M., Covey, K., Curry, C. L., Etiope, G., Frankenberg, C., Gedney, N., Hegglin, M. I., Höglund-Isaksson, L., Hugelius, G., Ishizawa, M., Ito, A., Janssens-Maenhout, G., Jensen, K. M., Joos, F., Kleinen, T., Krummel, P. B., Langenfelds, R. L., Laruelle, G. G., Liu, L., Machida, T., Maksyutov, S., McDonald, K. C., McNorton, J., Miller, P. A., Melton, J. R., Morino, I., Müller, J., Murguia-Flores, F., Naik, V., Niwa, Y., Noce, S., O'Doherty, S., Parker, R. J., Peng, C., Peng, S., Peters, G. P., Prigent, C., Prinn, R., Ramonet, M., Regnier, P., Riley, W. J., Rosentreter, J. A., Segers, A., Simpson, I. J., Shi, H., Smith, S. J., Steele, L. P., Thornton, B. F., Tian, H., Tohjima, Y., Tubiello, F. N., Tsuruta, A., Viovy, N., Voulgarakis, A., Weber, T. S., van Weele, M., van der Werf, G. R., Weiss, R. F., Worthy, D., Wunch, D., Yin, Y., Yoshida, Y., Zhang, W., Zhang, Z., Zhao, Y., Zheng, B., Zhu, Q., Zhu, Q., and Zhuang, Q.: The Global Methane Budget 2000–2017, Earth Syst. Sci. Data, 12, 1561–1623, https://doi.org/10.5194/essd-12-1561-2020, 2020. a, b, c, d
Schimel, J. P.: Plant transport and methane production as controls on methane flux from arctic wet meadow tundra, Biogeochemistry, 28, 183–200, https://doi.org/10.1007/BF02186458, 1995. a, b
Segers, R.: Methane production and methane consumption: a review of processes underlying wetland methane fluxes, Biogeochemistry, 41, 23–51, https://doi.org/10.1023/A:1005929032764, 1998. a
Ström, L. and Christensen, T. R.: Below ground carbon turnover and greenhouse gas exchanges in a sub-arctic wetland, Soil Biol. Biochem., 39, 1689–1698, https://doi.org/10.1016/j.soilbio.2007.01.019, 2007. a
Ström, L., Ekberg, A., Mastepanov, M., and Røjle Christensen, T.: The effect of vascular plants on carbon turnover and methane emissions from a tundra wetland, Glob. Change Biol., 9, 1185–1192, https://doi.org/10.1046/j.1365-2486.2003.00655.x, 2003. a
Ström, L., Mastepanov, M., and Christensen, T. R.: Species-specific effects of vascular plants on carbon turnover and methane emissions from wetlands, Biogeochemistry, 75, 65–82, https://doi.org/10.1007/s10533-004-6124-1, 2005. a
Ström, L., Tagesson, T., Mastepanov, M., and Christensen, T. R.: Presence of Eriophorum scheuchzeri enhances substrate availability and methane emission in an Arctic wetland, Soil Biol. Biochem., 45, 61–70, https://doi.org/10.1016/j.soilbio.2011.09.005, 2012. a
Taylor, M., Bradford, M., Arnold, W., Takahashi, D., Colgan, T., Davis, V., Losos, D., Peccia, J., and Raymond, P.: Quantifying the effects sizes of common controls on methane emissions from an ombrotrophic peat bog, J. Geophys. Res.-Biogeo., 128, e2022JG007271, https://doi.org/10.1029/2022JG007271, 2023. a
Tokida, T., Mizoguchi, M., Miyazaki, T., Kagemoto, A., Nagata, O., and Hatano, R.: Episodic release of methane bubbles from peatland during spring thaw, Chemosphere, 70, 165–171, https://doi.org/10.1016/j.chemosphere.2007.06.042, 2007. a
Treat, C. C., Bloom, A. A., and Marushchak, M. E.: Nongrowing season methane emissions – a significant component of annual emissions across northern ecosystems, Glob. Change Biol., 24, 3331–3343, https://doi.org/10.1111/gcb.14137, 2018. a
Turetsky, M. R., Kotowska, A., Bubier, J., Dise, N. B., Crill, P., Hornibrook, E. R., Minkkinen, K., Moore, T. R., Myers-Smith, I. H., Nykänen, H., Olefeldt, D., Rinne, J., Saarnio, S., Shurpali, N., Tuittila, E.-S., Waddington, J. M., White, J. R., Wickland, K. P., and Wilmking, M.: A synthesis of methane emissions from 71 northern, temperate, and subtropical wetlands, Glob. Change Biol., 20, 2183–2197, https://doi.org/10.1111/gcb.12580, 2014. a
van den Berg, M., van den Elzen, E., Ingwersen, J., Kosten, S., Lamers, L. P., and Streck, T.: Contribution of plant-induced pressurized flow to CH4 emission from a Phragmites fen, Sci. Rep., 10, 12304, https://doi.org/10.1038/s41598-020-69034-7, 2020. a
Van Der Nat, F.-J. W. and Middelburg, J. J.: Effects of two common macrophytes on methane dynamics in freshwater sediments, Biogeochemistry, 43, 79–104, https://doi.org/10.1023/A:1006076527187, 1998. a
Van Der Nat, F.-F. W., Middelburg, J. J., Van Meteren, D., and Wielemakers, A.: Diel methane emission patterns from Scirpus lacustris and Phragmites australis, Biogeochemistry, 41, 1–22, https://doi.org/10.1023/A:1005933100905, 1998. a
Waddington, J. and Roulet, N.: Atmosphere-wetland carbon exchanges: Scale dependency of CO2 and CH4 exchange on the developmental topography of a peatland, Global Biogeochem. Cy., 10, 233–245, https://doi.org/10.1029/95GB03871, 1996. a
Waddington, J., Roulet, N., and Swanson, R.: Water table control of CH4 emission enhancement by vascular plants in boreal peatlands, J. Geophys. Res.-Atmos., 101, 22775–22785, https://doi.org/10.1029/96JD02014, 1996. a
Whalen, S. and Reeburgh, W.: Moisture and temperature sensitivity of CH4 oxidation in boreal soils, Soil Biol. Biochem., 28, 1271–1281, https://doi.org/10.1016/S0038-0717(96)00139-3, 1996. a, b
Whiticar, M. J.: Carbon and hydrogen isotope systematics of bacterial formation and oxidation of methane, Chem. Geol., 161, 291–314, https://doi.org/10.1016/S0009-2541(99)00092-3, 1999. a, b, c
Whiting, G. J. and Chanton, J. P.: Plant-dependent CH4 emission in a subarctic Canadian fen, Global Biogeochem. Cy., 6, 225–231, https://doi.org/10.1029/92GB00710, 1992. a, b, c
Whiting, G. J. and Chanton, J. P.: Control of the diurnal pattern of methane emission from emergent aquatic macrophytes by gas transport mechanisms, Aquat. Bot., 54, 237–253, https://doi.org/10.1016/0304-3770(96)01048-0, 1996. a
Wilson, D., Alm, J., Riutta, T., Laine, J., Byrne, K. A., Farrell, E. P., and Tuittila, E.-S.: A high resolution green area index for modelling the seasonal dynamics of CO2 exchange in peatland vascular plant communities, Plant Ecol., 190, 37–51, https://doi.org/10.1007/s11258-006-9189-1, 2007. a, b
Wilson, J. O., Crill, P. M., Bartlett, K. B., Sebacher, D. I., Harriss, R. C., and Sass, R. L.: Seasonal variation of methane emissions from a temperate swamp, Biogeochemistry, 8, 55–71, https://doi.org/10.1007/BF02180167, 1989. a
Zhang, L., Dumont, M. G., Bodelier, P. L., Adams, J. M., He, D., and Chu, H.: DNA stable-isotope probing highlights the effects of temperature on functionally active methanotrophs in natural wetlands, Soil Biol. Biochem., 149, 107954, https://doi.org/10.1016/j.soilbio.2020.107954, 2020. a, b
Zona, D., Gioli, B., Commane, R., Lindaas, J., Wofsy, S. C., Miller, C. E., Dinardo, S. J., Dengel, S., Sweeney, C., Karion, A., Chang, R. Y.-W., Henderson, J. M., Murphy, P. C., Goodrich, J. P., Moreaux, V., Liljedahl, A., Watts, J. D., Kimball, J. S., Lipson, D. A., and Oechel, W. C.: Cold season emissions dominate the Arctic tundra methane budget, P. Natl. Acad. Sci. USA, 113, 40–45, https://doi.org/10.1073/pnas.1516017113, 2016. a
Please read the editorial note first before accessing the article.
- Article
(1832 KB) - Full-text XML