the Creative Commons Attribution 4.0 License.
the Creative Commons Attribution 4.0 License.
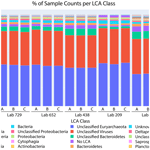
Results from a multi-laboratory ocean metaproteomic intercomparison: effects of LC-MS acquisition and data analysis procedures
Jaclyn K. Saunders
Matthew R. McIlvin
Erin M. Bertrand
John A. Breier
Margaret Mars Brisbin
Sophie M. Colston
Jaimee R. Compton
Tim J. Griffin
W. Judson Hervey
Robert L. Hettich
Pratik D. Jagtap
Michael Janech
Rod Johnson
Rick Keil
Hugo Kleikamp
Dagmar Leary
Lennart Martens
J. Scott P. McCain
Eli Moore
Subina Mehta
Dawn M. Moran
Jaqui Neibauer
Benjamin A. Neely
Michael V. Jakuba
Jim Johnson
Megan Duffy
Gerhard J. Herndl
Richard Giannone
Ryan Mueller
Brook L. Nunn
Martin Pabst
Samantha Peters
Andrew Rajczewski
Elden Rowland
Brian Searle
Tim Van Den Bossche
Gary J. Vora
Jacob R. Waldbauer
Haiyan Zheng
Zihao Zhao
Metaproteomics is an increasingly popular methodology that provides information regarding the metabolic functions of specific microbial taxa and has potential for contributing to ocean ecology and biogeochemical studies. A blinded multi-laboratory intercomparison was conducted to assess comparability and reproducibility of taxonomic and functional results and their sensitivity to methodological variables. Euphotic zone samples from the Bermuda Atlantic Time-series Study (BATS) in the North Atlantic Ocean collected by in situ pumps and the autonomous underwater vehicle (AUV) Clio were distributed with a paired metagenome, and one-dimensional (1D) liquid chromatographic data-dependent acquisition mass spectrometry analysis was stipulated. Analysis of mass spectra from seven laboratories through a common bioinformatic pipeline identified a shared set of 1056 proteins from 1395 shared peptide constituents. Quantitative analyses showed good reproducibility: pairwise regressions of spectral counts between laboratories yielded R2 values averaged 0.62±0.11, and a Sørensen similarity analysis of the top 1000 proteins revealed 70 %–80 % similarity between laboratory groups. Taxonomic and functional assignments showed good coherence between technical replicates and different laboratories. A bioinformatic intercomparison study, involving 10 laboratories using eight software packages, successfully identified thousands of peptides within the complex metaproteomic datasets, demonstrating the utility of these software tools for ocean metaproteomic research. Lessons learned and potential improvements in methods were described. Future efforts could examine reproducibility in deeper metaproteomes, examine accuracy in targeted absolute quantitation analyses, and develop standards for data output formats to improve data interoperability. Together, these results demonstrate the reproducibility of metaproteomic analyses and their suitability for microbial oceanography research, including integration into global-scale ocean surveys and ocean biogeochemical models.
- Article
(4909 KB) - Full-text XML
-
Supplement
(1040 KB) - BibTeX
- EndNote
Microorganisms within the oceans are major contributors to global biogeochemical cycles, influencing the cycling of carbon, nitrogen, phosphorus, sulfur, iron, cobalt, and other elements (Falkowski et al., 2008; Moran et al., 2022; Worden et al., 2015). Omic methodologies can provide an expansive window into these communities, with genomic approaches characterizing the diversity and potential metabolisms and with transcriptomic and proteomic methods providing insights into the expression and function of that potential. Similarly to other omic approaches, proteomics is increasingly being applied to natural ocean environments and the diverse microbial communities within them. When proteomics is applied to such mixed communities, it is generally referred to as metaproteomics (Wilmes and Bond, 2006). Metaproteomic samples contain an extraordinary level of complexity relative to single-organism proteomes (at least 1–2 orders of magnitude) due to the simultaneous presence of many different organisms in widely varying abundances (McCain and Bertrand, 2019). In particular, ocean metaproteome samples are significantly more complex than the human proteome, the latter of which is itself considered to be a highly complex sample (Saito et al., 2019). Proteomics (including metaproteomics) provides a perspective distinct from other omic methods: as a direct measurement of cellular functions, it can be used to examine the diversity of ecosystem biogeochemical capabilities, to determine the extent of specific nutrient stressors by measurement of transporters or regulatory systems, to determine cellular resource allocation strategies in situ, to estimate biomass contributions from specific microbial groups, and even to estimate potential enzyme activity (Bender et al., 2018; Bergauer et al., 2018; Cohen et al., 2021; Fuchsman et al., 2019; Georges et al., 2014; Hawley et al., 2014; Held et al., 2021; Leary et al., 2014; McCain et al., 2022; Mikan et al., 2020; Moore et al., 2012; Morris et al., 2010; Saito et al., 2020; Sowell et al., 2009; Williams et al., 2012). The functional perspective that metaproteomics allows is often complementary to metagenomic and metatranscriptomic analyses and can provide biological insights that are distinct from organisms studied in the laboratory (Kleiner, 2019). Moreover, the measurement of microbial proteins in environmental samples has improved greatly in recent years, due to the advancements in nanospray liquid chromatography and high-resolution mass spectrometry approaches (Mueller and Pan, 2013; Ram et al., 2005; McIlvin and Saito, 2021).
With increasing interest in the measurement of proteins and their biogeochemical functions within the oceans, the metaproteomic data are being established as a valuable research and monitoring tool. However, given rapid changes in technology and methods and the overall youth of the metaproteomic field, demonstrating the reproducibility and robustness of metaproteomic measurements to microbial ecology and oceanographic communities is an important goal. This is particularly true as applications for metaproteomics expand in research and monitoring of the changing ocean environment, for example, in global-scale efforts such as the developing BioGeoSCAPES program (https://www.biogeoscapes.org, last access: 18 October 2024; Tagliabue, 2023), which aims to characterize the ocean metabolism and nutrient cycles on a changing planet. As a result, there is a pressing need to assess interlaboratory consistency and to understand the impacts of sampling, extraction, mass spectrometry, and bioinformatic analyses on the biological inferences that can be drawn from the data.
There have been efforts to conduct intercomparisons of metaproteomic analyses in both biomedical and environmental sample types in recent years that provide a precedent for this study. A recent community best-practice effort in ocean metaproteomic data sharing also identified major challenges in ocean metaproteomic research, including sampling, extraction, sample analysis, bioinformatic pipelines, and data sharing, and conducted a quantitative assessment of sample complexity in ocean metaproteome samples (Saito et al., 2019). A previous benchmark study, driven by the Metaproteomics Initiative (Van Den Bossche et al., 2021), was the Critical Assessment of Metaproteome Investigation (CAMPI) study that employed a laboratory-assembled microbiome and human fecal microbiome sample to successfully demonstrate the reproducibility of results between laboratories. CAMPI found robustness in results across datasets while also observing variability in peptide identifications largely attributed to sample preparation. This observation was consistent with prior findings on single-organism samples that determined >70 % of the variability was due to sample processing rather than chromatography and mass spectrometry (Piehowski et al., 2013). Finally, the Proteome Informatics Research Group (iPRG) from the Association of Biomolecular Resource Facilities (ABRF) conducted a study examining the influence of informatic pipelines on metaproteomic analyses that found consistency among research groups in taxonomic attributions (Jagtap et al., 2023), and previous research has demonstrated the impact of database choices on final functional annotations and biological implications (Timmins-Schiffman et al., 2017).
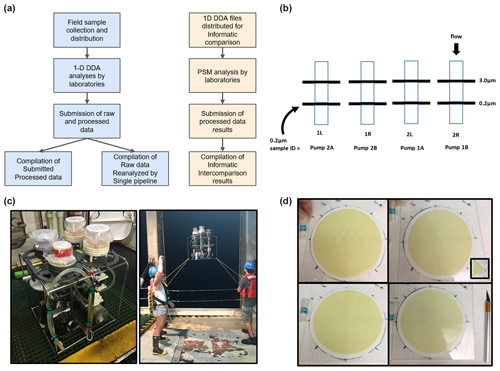
Figure 1Ocean metaproteomic intercomparison experimental design and sample collection. (a) The laboratory component (left) consisted of the collection of field samples, one-dimensional (1D) chromatographic separation followed by data-dependent analysis (DDA) uniformly employing Orbitrap mass spectrometer analyses by participating laboratories, and the submission of raw and processed data. The bioinformatic (right) component consisted of the distribution of two 1D DDA files, peptide-spectrum matches (PSMs), and the submission and compilation of results. (b) Size-fractionated sample collection on a filter of 3.0 µm pore size followed by a Supor filter of 0.2 µm pore size, and the 0.2–3.0 µm size fraction was used for the intercomparison study. (c) Two horizontal in situ McLane pumps were bracketed together with two Mini-MULVS filter head units each and deployed on a synthetic line. (d) The four 142 mm filters were sliced into eighths (inset), and two slices were distributed to each participating laboratory.
Here we describe the results from the first ocean metaproteomic intercomparison. In this study, environmental ocean samples were collected from the euphotic zone of the North Atlantic Ocean and partitioned into subsamples and distributed to an international group of laboratories (Fig. 1). The study was designed to examine interlaboratory consistency rather than maximal capabilities, stipulating one-dimensional (1D) chromatographic analyses from each laboratory (with optional deeper analysis). Users were invited to use their preferred extraction, analytical, and bioinformatic procedures. The effort focused on the data-dependent analysis (DDA) methods, also known as global proteomics, where the targets are unknown; hence there is a discovery element to the approach. DDA is currently common in ocean and other environmental and biomedical metaproteomics, and its spectral abundance units of relative quantitation have been shown to be reproducible in metaproteomics (Kleiner et al., 2017; Pietilä et al., 2022). Blinded results were submitted, compared, and discussed at a virtual community workshop in September 2021. An additional bioinformatic pipeline comparison study was also conducted, where participants were provided metaproteomic raw data and associated metagenomic sequence database files and were encouraged to use the bioinformatic pipeline of their choice.
2.1 Sample collection and metadata
Ocean metaproteome filter samples for the wet lab comparison (Fig. 1) were collected at the Bermuda Atlantic Time-series Study (BATS; 31°40′ N, 64°10′ W) on expedition BATS 348 on 16 June 2018, between 01:00 and 05:00 am local time. In situ (underwater) large-volume filtration was conducted using submersible pumps to produce replicate biomass samples at a single depth in the water column for intercomparisons. All filter subsamples are matched for location, time, and depth. To collect the samples, two horizontal McLane pumps were clamped together (Fig. 1c) and attached at the same depth (80 m) with two filter heads (Mini-MULVS design) on each pump and a flow meter downstream of each filter head. This depth was chosen to correspond to a depth with abundant chlorophyll and photosynthetic organisms. Each filter head contained a 142 mm diameter Supor filter of 0.2 µm pore size (Pall Inc.) with an upstream 142 mm diameter Supor filter of 3.0 µm pore size (Fig. 1b, d). Only the 0.2–3.0 µm size fraction was used in this study. The pumps were set to run for 240 min at 3 L min−1. Volume-filtered was measured by three gauges on each pump, one downstream of each pump head, and one on the total outflow (Table S2). Individual pump head gauges summed to the total gauge for pump 1 (within 1: 447 and 446.2 L) but deviated by 89 L on pump 2 (478 and 388.9 L). Given that the total gauge is further downstream, we report the pump head gauges as being more accurate.
The pump heads were removed from the McLane pumps immediately upon retrieval, decanted of excess seawater by vacuum, placed in coolers with ice packs, and brought into a fabricated clean-room environment on board the ship. The filters of 0.2 µm pore size were cut into eight equivalent pieces and frozen at −80 °C in 2 mL cryovials, creating 16 samples per pump that were co-collected temporally and in very close proximity (<1 m) to each other for a total of 32 samples used in this study (Fig. 1d). The filters of 3.0 µm pore size are not included in this study but are archived for future efforts. The sample-naming scheme associated with the different pumps and pump heads is described in Table S2. Note that pump samples 1A and 1B accidentally had two 3.0 µm filters superimposed above the 0.2 µm filter and that 1B had a small puncture in it, although neither of these issues seemed to affect the biomass collected; presumably the puncture occurred after sampling was completed.
Samples for the bioinformatic component were collected by the autonomous underwater vehicle (AUV) Clio. The vehicle and its sampling characteristics were used as previously described (Breier et al., 2020; Cohen et al., 2023). Specifically, samples Ocean-8 and Ocean-11 were also in the northwestern Atlantic Ocean on R/V Atlantic Explorer with the expedition identifier AE1913 (also described as BATS validation track BV55; Station 2 at 32.75834° N, 65.7374° W). The samples were collected by the AUV Clio on 19 June 2019, dive Clio020, with samples collected at 20 m (Ocean-11) and 120 m (Ocean-8) with 66.6 and 92.6 L, respectively, filtered for this study. These depths were chosen to reflect the near-surface (high-light) and deep chlorophyll maximum (low-light) communities present in the stratified summer conditions. These samples were analyzed by 1D DDA analysis using extraction and mass spectrometry for laboratory 438 within their laboratory (Tables S5–S7). Sample metadata for both arms of this intercomparison study and the corresponding repository information are provided in Table S3, and repository links are in the Code and data availability statement.
2.2 Metagenomic extraction, sequencing, and assembly
A metagenomic (reference sequence) database was created for peptide-spectrum matches (PSMs) for the metaproteomic studies using a one-eighth sample split from the exact sample used in the intercomparison as described above. Samples were shipped on dry ice to the Naval Research Laboratory in Washington, D.C. (USA), where DNA was extracted and sequenced. Preserved filters were cut into smaller pieces using a sterile blade and placed into a PowerBead tube with a mixture of zirconium beads and lysis buffer (CD1) from the Dneasy PowerSoil Pro Kit (Qiagen, Hilden, Germany). The bead tube with filter sample was heated at 65 °C for 10 min then placed on a vortex adapter and vortexed at maximum speed for 10 min. After sample homogenization/lysis, the bead tube was centrifuged at 16 k×g for 2 min. The supernatant was transferred to a DNA LoBind tube and processed using the manufacturer's recommendations. The purified DNA was further concentrated by adding 10 µL3 M NaCl and 100 µL cold 100 % ethanol. The sample was incubated at −30 °C for 1 h, followed by centrifugation at 16 k×g for 10 min. The supernatant was removed, and precipitated DNA was air-dried and resuspended in 10 mM Tris. DNA concentration was quantified with the Qubit dsDNA High-Sensitivity Assay Kit (Thermo Fisher Scientific, Waltham, MA, USA), and DNA quality was assessed using the NanoDrop (Thermo Fisher) and gel electrophoresis. Processing controls included reagent-only and blank filter samples.
Sequencing libraries were created from purified sample DNA using the Ion Xpress Plus gDNA Fragment Library Kit (Thermo Fisher) for a 200 bp library insert size. No amplification of the library was required, as determined by qPCR using the Ion Library TaqMan Quantitation Kit. A starting library concentration of 100 pM was used in template generation and chip loading with the Ion 540 Chip Kit on the Ion Chef instrument prior to single-end sequencing on the S5 benchtop sequencer.
Sequencing used a mix of Ion Torrent and Oxford Nanopore sequencing, and resulting sequencing reads were assembled using SPAdes v.3.13.1 with Python v.3.6.8. Following metagenome assembly, contigs smaller than 500 bases were discarded. Open reading frame (ORF) calling was performed on contigs 500 bps or longer using Prodigal v.2.6.3 (Hyatt et al., 2010) run with metagenomic settings and MetaGeneMark by submitting to the MetaGeneMark server (http://exon.gatech.edu/meta_gmhmmp.cgi, last access: 2 January 2024) using the GeneMark.hmm prokaryotic program v.3.25 on 11 August 2019. ORFs called from both programs were combined and made non-redundant using in-house Python scripts that utilize Biopython v.1.73. Non-redundant ORFs were annotated using the sequence alignment program DIAMOND (v.0.9.29) with the NCBI nr database (downloaded 17 December 2019). ORFs were also annotated with InterProScan (v.5.29) and with GhostKOALA (Kanehisa et al., 2016) (submitted to server 2 January 2020). Taxonomy lineages were generated by using the best DIAMOND (Buchfink et al., 2015) hit and pulling lineage information from the NCBI Taxonomy database using Biopython v.1.73.
2.3 Proteomic methodologies: extraction, instrumentation, and bioinformatics
Some basic protocol stipulations were provided to study participants regarding analytical conditions to set a uniformity of experimental design. While users were encouraged to use the extraction method of their preference, constraints on chromatography and mass spectrometry conditions were set, limiting the number of chromatographic dimensions (1D), the total length of the chromatographic run, and the amount of protein injected (as proteolytic digests), as well as enforcing a single mass spectrometry injection rather than gas-phase fraction approaches (Table S4). Each laboratory group's specific approach is summarized in the Supplement, with extraction in Table S5 and with chromatography and mass spectrometry equipment and parameters in Tables S6 and S7. While there are more sophisticated methods, such as two-dimensional (2D) chromatography and gas-phase fractionations, that have been demonstrated to provide deeper metaproteomes (McIlvin and Saito, 2021), these often require specialized equipment and/or additional instrument time. As a result, the study constraints were provided to ensure a single simple method that all labs could utilize. Laboratories were invited to submit additional data from more complex analytical setups if they first completed the 1D analyses.
2.4 Compilation, analysis, and re-analysis of laboratory data submissions
Results from individual laboratories' data submissions were analyzed in two ways, as shown in the flowchart of Fig. 1a. Firstly, submitted processed data reports (i.e., PSMs, taxonomic, functional annotations) were compiled and interpreted. Secondly, raw data files (i.e., spectra directly from instruments) from each group were put through a single bioinformatic pipeline using SEQUEST HT/Percolator within Proteome Discoverer v.2.2.0.388 (Thermo Scientific) and Scaffold v.5.2.1 (Proteome Software) to isolate variability associated with bioinformatic processing. Note that Scaffold ignores the Percolator output from Proteome Discoverer when re-running in Scaffold. This re-analysis (single-pipeline re-analysis hereon) allowed detailed cross-comparisons of laboratory practices to assess the influence of the extraction and mass spectrometry components. Specific parameters of the latter included the following: parent tolerances of 10 ppm were used on all instruments (all Orbitraps), and fragment tolerances of 0.02 and 0.6 Da were used for Orbitrap MS2 instruments and for ion trap MS2 instruments, respectively. Fixed and variable modifications of +57 on C (fixed), +16 on M (variable), and +42 on the peptide N-terminal (variable) were used. Peptide and protein false discovery rates (FDRs) were set to lower than 1.0 % using a decoy database, with one minimum peptide per protein, and the resulting peptide FDR was 0.1 %. The database used for PSMs was Intercal_ORFs_prodigal_metagenemark.fasta based on the metagenomic sequencing described above with 197 824 protein entries. The re-analysis was conducted within Scaffold using total spectral counts and allowing single peptides to be attributed to proteins. In addition to the total number of protein identifications, the number of protein groups identified by Scaffold was also provided. Each protein group represented proteins identified with identical peptides, collapsed into a single protein entry with the highest probability and number of spectral counts.
2.5 Data analysis methods
Several analyses were conducted using data from the single-pipeline re-analysis. Firstly, pairwise comparisons of protein identifications were conducted using spectral abundance reports produced in Scaffold and were loaded, analyzed, and visualized in MATLAB (MathWorks Inc). Two-way (independent) linear regressions were conducted using the script linfit.m. The R2 on the seven datasets was averaged, and the standard deviation was calculated for shared proteins in each dataset. Secondly, a Sørensen similarity (Sørensen, 1948) was calculated where a matrix was generated that consisted of the unique proteins or peptides identified across all technical replicates from the various labs with the relative abundance per replicate (percentage contribution of each protein/peptide per technical replicate total). The Bray–Curtis dissimilarity pairwise distance was calculated on this matrix using Python and the SciPy library (v.1.4.1; Virtanen et al., 2020) and then 1; the Bray–Curtis dissimilarity was calculated across the matrix to generate the Sørensen pairwise similarity across all replicates. The resulting similarities per replicate were clustered and visualized using the clustermap function in the Seaborn library (v.0.10.0; Waskom, 2021). Thirdly, shared peptides and proteins were visualized using UpSet plots and using the R package UpSetR (Conway et al., 2017) to determine the number of unique peptide sequences and annotated proteins in intersecting sets between all labs, all permutations of lab subsets, and all lab pairs.
2.6 Bioinformatic intercomparison methods
The methods used for the bioinformatic intercomparison study are described by each laboratory using their unique three-digit identifier code. All laboratories used the metagenomic database generated in the laboratory study (see Sect. 2.2). In Lab 109, the raw files were searched against the metagenomic database employing a two-round search using PEAKS Studio X. The initial database search was performed to focus the metagenomic database for protein sequences with peptide sequence matches at 5 % FDR. The focused database was further used for a second-round search, which allowed a parent mass error tolerance of 10.0 ppm and a fragment mass error tolerance of 0.6 Da. The search considered up to three missed cleavages, with carbamidomethylation as fixed and with methionine oxidation and N-terminal acetylation as variable modifications. The cRAP protein sequences (http://ftp.thegpm.org/fasta/cRAP./, last access: 1 October 2020) were included as a contaminant database. Finally, PSMs were filtered for 1 % FDR and annotated with taxonomic lineages (obtained from the metagenomic experiments). Non-unique peptide matches were annotated with the LCA of the respective lineages.
In Lab 321, SearchGUI (Galaxy v.3.3.10.1) was used to search using multiple search algorithms (X!Tandem, MS-GF+, and Comet). For each search algorithm, a precursor tolerance of 10.0 ppm was used, a fragment ion tolerance of 0.6 Da was used, and trypsin was used as an enzyme for proteolytic cleavage. Searches were performed allowing two missed cleavages: fixed modification of carbamidomethylation at cysteine and variable modifications of acetylation of protein N-term and oxidation of methionine. PeptideShaker (v.1.16.36) was used to filter peptides with a length of 8–50 aas and a precursor tolerance of 10.0 ppm. Detected peptide-spectrum matches, peptides, and proteins were reported at 1 % global FDR. The entire analysis was performed within the Galaxy platform.
In Lab 321, MaxQuant (Galaxy v.1.6.17.0+galaxy3) was used to search the datasets. A fixed modification of carbamidomethylation at cysteine and variable modifications of acetylation of protein N-term and oxidation of methionine were applied along with allowing two missed cleavages. The detection peptides and proteins were reported at 1 % FDR.
In Lab 362, the raw files were converted using ThermoRawFileParserGUI (v.1.4.1) to peak lists (.mgf files) using “native Thermo library peak picking” as the peak picking option and “Ignore missing instrument properties” as the error option. The peak lists (.mgf files) obtained from MS/MS spectra were identified using X! Tandem (Vengeance V.2015.12.1) using SearchGUI v.4.1.0. Here, the parameters provided and suggested by the study were used: tolerances of 10 ppm for MS1 and 0.6 Da for MS/MS, dynamic modifications of oxidation of M and acetyl on the N-terminus, and a static modification of carbamidomethylation of C. Identification was conducted against a concatenated target/decoy database of the provided database.
The X!Tandem files were used as input in MS2Rescore (https://github.com/compomics/ms2rescore, last access: 1 October 2020), a machine-learning-based post-processing tool that improves upon Percolator rescoring of peptide-spectrum matches (PSMs). Here, the search-engine-dependent features of Percolator were appended with MS2 peak intensity features by comparing the PSM with the corresponding MS2 PIP-predicted spectrum. All reported MS2Rescore PSM identifications have a q value <0.01. No protein grouping algorithm was applied, and all identified taxa and functions are extracted from the provided database.
In Lab 458, the Proteome Discoverer 2.5 platform was used (SequestHT + Percolator (MPS)). Fully tryptic peptides with a minimum length of six peptides and a maximum of two missed cleavages were required. A precursor tolerance of 10.0 ppm and a fragment ion tolerance of 0.6 Da were used, carbamidomethylation was fixed, and methionine oxidation was set as a variable modification. Filtering was performed at a 1 % PSM- and peptide-level FDR. The MaxQuant contaminant list was used as a contaminant database.
In Lab 501, we first appended the database with a set of common contaminants (Global Proteome Machine Organization common Repository of Adventitious Proteins). Then, we used MSGF+ (Kim and Pevzner, 2014) to match mass spectra with peptide sequences, with cysteine carbamidomethylation as a fixed modification and with methionine oxidation, glutamine modified to pyroglutamic acid, deamidated asparagine, and deamidated glutamine as variable modifications. Peptides were searched for with a target-decoy approach, with a 1 % false discovery rate at the peptide-spectrum match level. For spectral counts, we summed MS2 spectra that identified a peptide and normalized all spectral counts to the total spectral counts per sample. Proteins were quantified using the median spectral count for all proteotypic peptides (those peptides which uniquely correspond to a protein), specifically using the OpenMS tool ProteinQuantifier. This approach requires at least one proteotypic peptide, but, if more are identified, those peptides are also used for quantification.
In Lab 828, the raw files were analyzed using Proteome Discoverer. MS/MS spectra were searched against the provided database using the SEQUEST-HT engine. MS/MS spectrum searches were performed with a precursor ion tolerance of 10.0 ppm and a fragment ion tolerance of 0.6 Da. Carbamidomethyl cysteine was specified as a fixed modification, whereas oxidation (M), deamidation (N/Q), and N-terminal protein acetylation were set as variable modifications. Trypsin was specified as the proteolytic enzyme, allowing two missed cleavages. Percolator-based scoring was chosen to improve the discrimination between correct and incorrect spectrum identifications, learning from the results of a decoy and a target database. Settings were as follows: maximum delta Cn of 0.05, strict false-discovery rate of 0.01, and validation based on q values.
In Lab 902, SEQUEST-HT was used within Proteome Discoverer 2.2 using the following settings: two maximum missed cleavages, a minimum peptide length of 6 and a maximum peptide length of 122, a precursor mass tolerance of 10 ppm, and a fragment mass tolerance of 0.6 Da. The dynamic modifications were M oxidation and acetyl on N-terminus, and the static modification was C carbamidomethyl. A Percolator PSM validator (within Proteome Discoverer) was used with following settings: maximum delta Cn 0.05, target FDR strict 0.01, target FDR relaxed 0.05, and validation based on PEP. Scaffold 5.0 was used to analyze files generated with Proteome Discoverer with following settings: a scoring system in prefiltered mode, standard experiment-wide protein grouping, a protein threshold of 1.0 % FDR, a peptide threshold of 0.1 % FDR, and a minimum of one peptide.
In Lab 932, mass spectrometry data were transformed from Thermo RAW format (v.66) to mzML and Mascot Generic (MGF) formats using ThermoRawFileParser (v.1.2.0; Hulstaert et al., 2019). Experimental metadata were extracted from mass spectrometry data using the MARMoSET program (Kiweler et al., 2019). Mascot Server (v.2.6.2; Matrix Science, LTD) software performed peptide-spectrum matching between experimental data and a reference sequence database. Reference sequences included a total of 197 824 predicted protein-coding ORFs from a metagenome assembly. Peptides matching an in-house curated inventory of contaminant protein sequences, mass standards, and proteolytic enzyme sequences were removed from the results. Mascot search parameters included the following settings: +10.0 ppm monoisotopic precursor mass tolerance, +0.6 Da monoisotopic fragment ion tolerance, one fixed modification (+57 to C residues), two variable modifications (+16 to M residues and +42 to peptide amino-termini), the digestion enzyme trypsin, two missed cleavages, peptide charges , and electrospray ionization coupled to Fourier-transform ion cyclotron resonance (ESI-FTICR). Mascot search results containing peptide-spectrum matches (PSMs) were exported for downstream data analysis. Scaffold Q+S (v.4.8.9) was used to validate MS/MS-based peptide- and protein-level peptide-spectrum matches with the PeptideProphet algorithm. Mascot PSM data were imported into Scaffold Q+S with the following settings specified: a quantitative metric of spectrum counting, legacy PeptideProphet scoring (high-mass accuracy), and standard experiment-wide protein grouping. Optional loading steps were to pre-compute false discovery rate (FDR) thresholds and to use local gene ontology (GO) annotations (UniProt GO annotation data retrieved 25 June 2020). Scaffold Q+S identification criteria were set at greater than or equal to 99.9 % probability by the PeptideProphet algorithm (Keller et al., 2002.) and >99.9 % probability by the ProteinProphet algorithm (Nesvizhskii et al., 2003) with more than two peptides at the protein level.
In Lab 957, MSFragger 3.3 searches were performed with FragPipe 16.0 and Philosopher 4.0.0. A concatenated target/reverse database was searched with a 50 PPM precursor and a 0.4 Da fragment mass tolerance. Automatic mass calibration and parameter optimization were enabled, and precursor mass errors for up to +2 neutrons were considered. Peptide candidates were generated from database protein sequences assuming tryptic digestion, allowing up to one missed cleavage. Peptides were required to have between 8–50 amino acids and range from 500 to 5000 . Cysteines were assumed to be fully carbamidomethylated, and peptides were searched considering variable n-terminal pyroglutamic acid formation and methionine oxidation. PeptideProphet was used for FDR validation with the following default options: “–decoy probs”, “–ppm”, “–accmass”, “–nonparam”, and “–expectscore”, which allow additional high-mass accuracy analysis and non-parametric distribution fitting. ProteinProphet was used for protein-level FDR validation with the following default option: “–maxppmdiff 2000000”. Filtering was performed using a 1 % peptide-level and a 1 % protein-level FDR threshold.
3.1 Experimental design
This ocean metaproteomic intercomparison consisted of two major components: a laboratory component, where independent labs processed identical ocean samples simultaneously collected from the North Atlantic Ocean (Fig. 1a; see Sect. 2.1), and a subsequent bioinformatic component. Participating institutions and persons at those institutions are listed in Table S1 in the Supplement, with all participants also listed as co-authors. Both arms of the study were conducted under blinded conditions, where correspondence with participants was conducted by an individual not involved in either study and where submitted results and data were anonymized prior to sharing with the consortium. Within both arms of the study, participants were provided the location of the study site and metadata about the sampling locations, time, and depth at the onset of the study. The laboratory study involved two biomass-laden filter slices collected from the North Atlantic Ocean Bermuda Atlantic Time-series Study site at 80 m depth being sent to each participating group for protein extraction, mass spectrometry, and bioinformatic analyses (see Sect. 2.1). This depth was chosen to correspond to a depth with abundant chlorophyll and associated photosynthetic organisms. The bioinformatic effort was independent of the laboratory effort and involved the distribution and bioinformatic analysis of two metaproteomic raw data files generated from samples also from the North Atlantic Ocean upper-water-column BATS station (20 and 120 m depths; see Sect. 2.1). These depth were chosen to reflect the near-surface (high-light) and deep chlorophyll maximum (low-light) communities present in the stratified summer conditions. These files were distributed after labs had submitted their laboratory-extracted raw data files. The raw files from the bioinformatic study were distinct from the samples used in the laboratory intercomparison study to avoid any biases from groups that analyzed those samples previously. Submitted results from both components were anonymized and assigned three-digit lab identifiers generated randomly, with laboratory and bioinformatic results from the same lab being assigned distinct identifiers.
We report results for two study components: part 1 (Sect. 3.2) involves the data generation intercomparison of distributed subsamples from the North Atlantic Ocean (Fig. 1, Sect. 2.1). Part 2 (Sect. 3.3) was a bioinformatic intercomparison, where metaproteomic raw files were shared with participants and processed results were submitted. Both components were conducted as blinded studies, where each dataset was assigned a three-digit randomly generated identifier, with those identifiers used throughout the Results and Discussion.
3.2 Mass spectrometry data generation intercomparison
Nine laboratories submitted raw and processed datasets from the analysis of the distributed Atlantic Ocean field samples (Table S1). The processed data submissions were heterogeneous in output format, statistical approach, and parameter definition. Because of the challenges of comparing data derived from different types of statistical approaches used for peptide and protein identification and inference and of the varying output formats from various software packages, the user-generated data submissions were difficult to compile and compare, resulting in variability in the number of identifications depending on the statistical approaches and thresholds applied. These results are further discussed in the Supplement (Fig. S1, Table S8). Despite these challenges, an average of 7142±2074 peptides was identified across the pairwise comparisons (Fig. S1c), representing 20 % of the 35 715 total unique peptides detected across all labs. Together, these findings implied a consistency in peptide identification across participants. The variability in proteome depth reflected the combination of differing parameters employed by software and laboratory approaches.
To remove this variability associated with user-selected bioinformatic pipelines, a single-pipeline re-analysis of the submitted raw mass spectral data was conducted. Raw data files were processed together within a single bioinformatic pipeline consisting of SEQUEST-HT, Percolator, and Scaffold software and evaluated to a false discovery rate threshold of <0.1 % for peptides and 1.0 % for proteins (see Sect. 2.4). Two datasets were found to have had issues during extraction and analysis that affected the results in both processed and raw data (labs 593 and 811; Table S8). Notably, these two laboratories differed from the others in that they did not use SDS as a protein-solubilizing detergent (Table S5). This likely resulted in inefficient extraction of the bacteria that dominated the sample biomass (e.g., picocyanobacteria and Pelagibacter) embedded within the membrane filter slices. Further examination showed polyethylene glycol contamination of one dataset (Lab 811) and low yield from sample processing and extraction from the other (Lab 593). As a result, those datasets were not included in the single-pipeline re-analysis. The standardized pipeline included calculations of shared peptides and proteins, quantitative comparisons, and consistency of taxonomic and functional results.
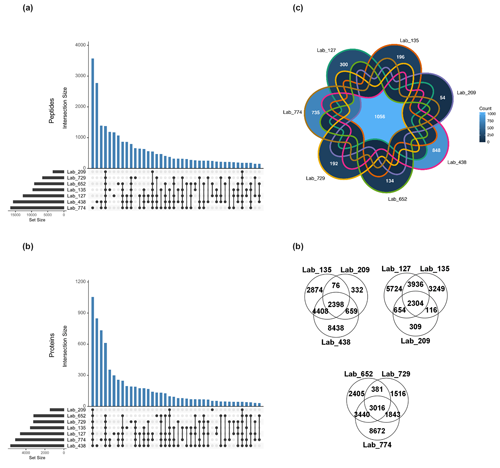
Figure 2Shared peptides and proteins between laboratory groups using laboratory submissions processed through a single bioinformatics re-analysis pipeline. (a) The total number of discovered unique peptides varied by more than 3-fold among seven laboratory groups (horizontal bars) due to varying extraction and analytical schemes (FDR 0.1 %). The number of intersections between datasets across all seven datasets was 1395 (fourth blue bar from left), and various sets of intersections of peptides were observed amongst the data. (b) The total number of discovered proteins (FDR <1 %) varied more than 4-fold from 1586 to 6221 among labs (horizontal bars). The number of intersections between datasets across all seven laboratories was 1056, with various sets of intersections of proteins observed, similar to the peptides. (c) Seven-way Venn diagrams of shared unique peptides between laboratories showed 1056 shared peptides between the seven laboratories. (d) Three-way Venn diagrams showed 2398, 2304, and 3016 shared unique peptides between laboratories.
The total number of peptide and protein identifications and PSMs in the single-bioinformatic-pipeline analysis varied by laboratory (Table S9), with unique peptides ranging by more than a factor of 3 from 3354 to 16 500 and with 27 346 total unique peptides identified across laboratories. This variability was likely due to different extraction, chromatographic, and mass spectrometry hardware and parameters employed used by each laboratory, resulting in a varying depth of metaproteomic results. However, as with the user-submitted results, there was considerable overlap in identifications between all datasets. An intersection analysis found the numerous shared peptides between all combinations of laboratories, with 1395 peptides shared between all seven laboratory datasets (Fig. 2a). Laboratories with deeper proteomes shared numerous peptides; for example, the two laboratories with the most discovered unique peptides shared ∼3000 peptides between them, implying that shared peptides is a useful metric for intercomparability. They also had the largest numbers of peptides that were not found by any other labs (3617 and 2819, respectively). The fourth-largest intersection size (1395) represented the unique peptides discovered by all labs. Beyond that, there were 12 different groupings of peptides that were shared among at least four laboratories. Consistent with this, three-way Venn diagrams of labs 135, 209, and 438 had an intersection of 2398 peptides; labs 652, 729, and 774 shared 3016 peptides; and labs 127, 135, and 309 shared 2304 peptides (Fig. 2d).
A similar analysis was conducted at the protein level (see Sect. 2, Methods), where 8043 unique proteins in total were identified across all laboratories, with 1056 of those observed in all seven labs (see seven-way Venn diagram in Fig. 2c). Three-way Venn diagram comparisons among labs 135, 209, and 438 had an intersection of 1254 proteins, and labs 652, 729, and 774 shared 1925 proteins (data not shown).
Optional deeper metaproteome results were submitted by three laboratories using either a long gradient of 12 h or two-dimensional chromatographic methods (Table S10). The number of discovered peptide and protein identifications was higher in each case, with as many as 18 477 unique peptides and 7765 protein identifications from an online two-dimensional chromatographic analysis of a 5 µg single injection.
The mapping of identified peptides to protein sequences forms the basis for protein identification in the form of DDA bottom-up proteomics employed here. The relationship between peptides and protein identification was explored in Fig. 3 and found to be correlated by two-way linear regression with R2 values of 0.97 and 0.98 for total protein identifications and protein groups, respectively. Together, the fact that there is a linear relationship between peptides and proteins across all laboratories (including labs employing deeper methods) could imply that the number of protein identifications has not begun to plateau and has reached “saturation”, likely due to the immense biological diversity and lower abundance of peptides within these samples. This approach has some similarities to rarefaction curves used in metagenomic sequencing to determine if the majority of species diversity has been sampled, although, in this case, the number of peptides is used as a metric for sampling depth instead of the additional number of DNA sequencing samples typically used for rarefaction curves. This indicated that, with a greater depth of analysis by some laboratories, there was no fall-off in the increase in protein identification that might be attributed to additional peptides mapping to already-discovered protein sequences. In addition, the 2D and long-gradient additional analyses conducted by several laboratories fell upon this line, consistent with the “more peptides, more proteins” observation, implying more room for improvement in the depth of metaproteomic analyses.
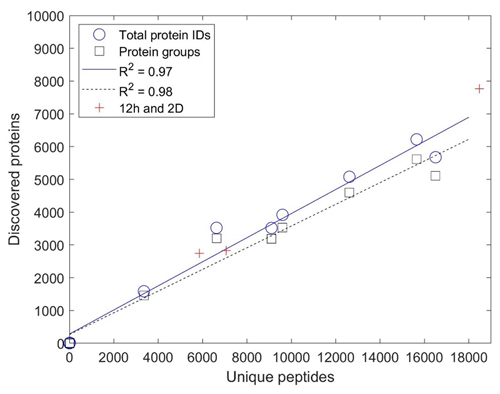
Figure 3Comparison of unique peptides and discovered proteins. Comparison as total protein identifications and protein groups from the single-pipeline re-analysis based on submissions from nine laboratories. Increasing sample depth is linear with mapping to proteins (R2 of 0.97 and 0.98 for total protein IDs and protein groups, respectively, with slopes of 0.37 and 33), implying that additional peptide discovery leads to proportionally more protein discovery and that protein discovery has not yet begun to saturate with more peptides mapping to each protein. Because simple 1D analyses were stipulated in the intercomparison experimental design, peptide and protein discovery were correspondingly limited in depth.
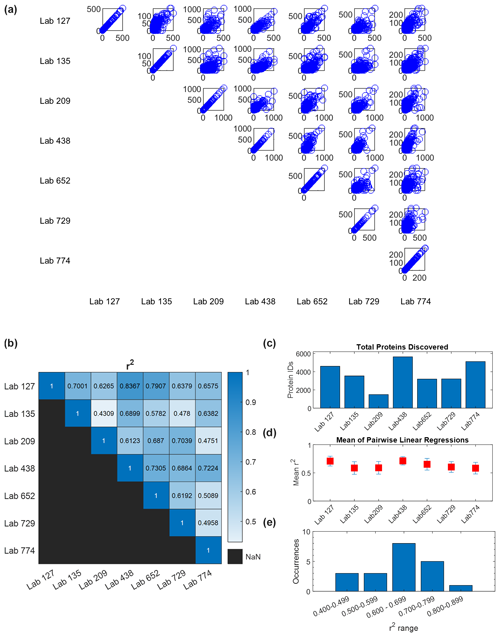
Figure 4Quantitative comparison of intercomparison results. (a) Pairwise comparisons of quantitative abundance across six laboratories in units of spectral counts (the comparison of each lab with itself shows unison diagonals). (b) R2 values from pairwise linear regressions. (c) Total proteins identified in each laboratory. (d) Average of each laboratory's R2 values from pairwise regression with the other six laboratories (error bars are standard deviation). In all cases, the average R2 value is higher than 0.5. (e) Occurrences of R2 values in pairwise comparisons spanning 0.4 to 0.9. Potential causes of this range are outlined in the Discussion.
A quantitative analysis of spectral counts from the wet-lab re-analysis showed broad coherence among the seven laboratories. Pairwise comparisons of protein spectral counts were conducted for each of the seven labs against the other six (visualized in a 7×7 matrix, with duplicate comparisons removed (e.g., A vs. B and B vs. A)), where each data point reflects the spectral counts for a protein shared between laboratories (Fig. 4a). When a dataset was compared with itself, a unity line of data points was observed along the diagonal axis as expected. Two-way linear regressions were conducted on each of these pairwise comparisons. The slopes ranged from 0.33 to 5.5 (Fig. S2), implying a varying dynamic range in spectral counts across laboratories, likely due to variations in instrument parameterizations selected by each laboratory, and consistency with the lack of normalization between laboratories. The coefficient of determination R2 values from 0.43 to 0.84 with an average of 0.63±0.11 show coherence among results for these large metaproteomic datasets (Fig. 4b, Table S12). To provide a sense of coherence between each laboratory and the others, the R2 values of a lab against the other six laboratories were averaged and the standard deviation was calculated. All of these average R2 values were higher than 0.5, which showed overall quantitative consistency despite the size and complexity of these datasets (Fig. 4d).
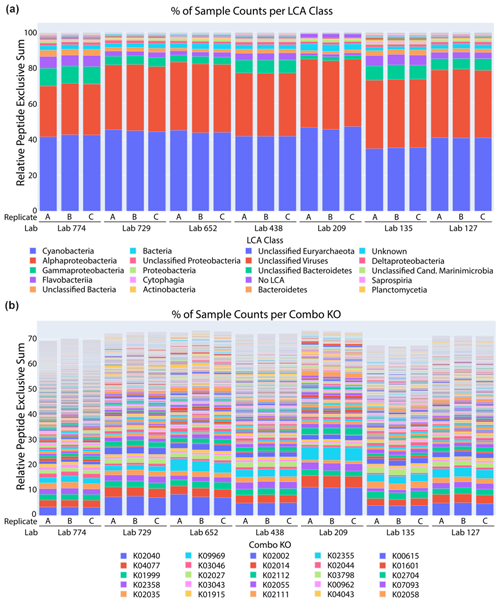
Figure 5Taxonomic and functional analysis of metaproteomic intercomparison. (a) The percentage of spectral counts by taxonomy was similar across laboratories and technical replicates within laboratories. The sample was dominated by cyanobacteria and alphaproteobacteria, corresponding primarily to Prochlorococcus and Pelagibacter, respectively. (b) The percentage of spectral counts per KEGG Orthology group showed the functional diversity of the sample.
A comparative taxonomic and functional analysis was also conducted using a single bioinformatic pipeline (see metagenomic sequencing methods for annotation pipeline). A lowest common ancestor (LCA) analysis of peptides identified from datasets from seven laboratories showed consistent patterns of taxonomic distribution using the METATRYP package (Fig. 5a; Saunders et al., 2020). Cyanobacteria and alphaproteobacteria were the top two taxonomic groups in all laboratory submissions, consistent with the abundant picocyanobacteria Prochlorococcus and the heterotrophic bacterium Pelagibacter ubique known to be dominant components of the Sargasso Sea ecosystem (Sowell et al., 2009; Malmstrom et al., 2010). For example, Prochlorococcus is consistently present between 104 and 105 cells per milliliter in this region and has been observed to contribute to carbon export from the euphotic zone (Casey et al., 2007). Pelagibacter cells can also be in excess of 105 cells per milliliter at the BATS North Atlantic location (Carlson et al., 2009). These results are broadly similar to the representation of phyla within the metagenome annotations, where Proteobacteria (including Pelagibacter) and Cyanobacteria (including Prochlorococcus and Synechococcus) were major components, although Bacteriodetes (including Flavobacteria) are more prevalent in the metagenome annotations than in the metaproteome. Some differences may also be due to the incorporation of protein abundances in Fig. 5a versus the simple taxonomic attribution of non-redundant assembled open reading frames in the metagenome analysis and to the use of multiple sequencing platforms and gene-calling algorithms (Sect. 2.2, Fig. S4).
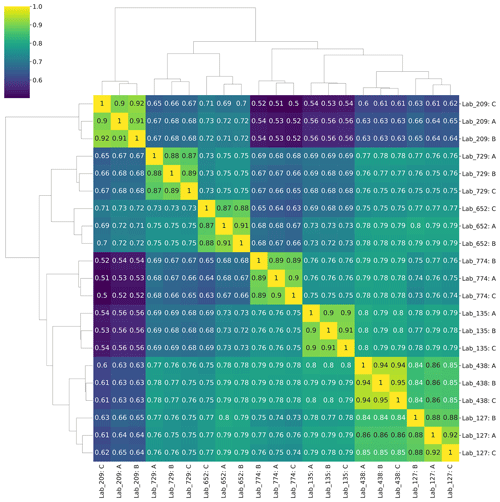
Figure 6Quantitative Sørensen similarity analysis. Analysis of the top 1000 proteins (∼75 % of all proteins) showed 70 %–80 % similarity between most laboratory groups. Technical triplicates for each laboratory group are shown.
Similarly, KEGG Orthology (KO) group analysis of those datasets also showed highly similar patterns of protein functional distributions across laboratories (Fig. 5b). Notably, the PstS phosphate transporter protein from Prochlorococcus was the most abundant protein in all datasets, consistent with observations of phosphorus stress in the North Atlantic oligotrophic gyre and its biosynthesis in marine cyanobacteria (Scanlan et al., 1997; Coleman and Chisholm, 2010; Ustick et al., 2021). These findings demonstrate the reproducibility in the primary functional and taxonomic conclusions from the metaproteome datasets. Finally, a Sørensen similarity analysis of the 1000 proteins with highest spectral counts revealed 70 %–80 % similarities between most laboratory groups in the data re-analysis (Fig. 6). When conducted on the full dataset with all peptides and proteins, the Sørensen similarity analysis showed peptides had lower similarity than proteins, implying variability is ameliorated when aggregated to the protein level (Fig. S3).
3.3 Bioinformatic data analysis intercomparison
Two metaproteomic raw files were provided to intercomparison participants and were searched, with each laboratory's preferred database searching the bioinformatic pipeline. The samples that generated the data for these files were collected by AUV Clio during a single dive at the Bermuda Atlantic Time-series Study station (Breier et al., 2020) and were distinct from the samples associated with the laboratory intercomparison component. However, they were also from the North Atlantic Ocean, allowing the same metagenomic database to be used. This database was not collected simultaneously with the bioinformatics samples, so it was not as representative as that used in the laboratory intercomparison. However, the BATS region is known to maintain similar major taxonomic composition throughout the year (e.g., Prochlorococcus and SAR11; see discussion in Sect. 3.2), hence enabling many protein identifications. This bioinformatic study component was not launched until after the laboratory-based intercomparison submission deadline to avoid influencing that part of the study by sharing similar raw data. Samples were named Ocean 8 and Ocean 11 and were taken from 120 and 20 m depths, respectively.
The bioinformatic intercomparison involved 10 laboratories utilizing eight different software pipelines, including the PSM search engines SEQUEST, X!Tandem, MaxQuant, MSGF+, Mascot, MSFragger, and PEAKS (Table S11; see Methods Sect. 2.6). As with the user-supplied laboratory results, the results were challenging to compile due to different types of data outputs, approaches used in protein inference, and statistical approaches applied within each pipeline. Unique peptide discoveries served as a useful base unit of comparison, as they were less subject to these comparison challenges. The number of peptides ranged from 1724 to 6369 in Ocean 8 and 3019 to 8288 in Ocean 11 (Fig. 7, Table S11). The difference in the number of peptides was likely due to parameters used in software; for example, Lab 932 had the lowest number of peptides identified in both samples but also used a highly stringent 99.9 % probability cutoff that likely influenced this result.
4.1 Assessment of ocean metaproteomic reproducibility
Given the recent establishment of complex metaproteomic techniques, intercomparisons are valuable in demonstrating their suitability for ocean ecological and biogeochemistry studies. Synthesizing the results of the laboratory and mass spectrometry blinded intercomparison study (Sect. 3.2) processed with a single bioinformatic pipeline (Sect. 2.4), we observed consistent reproducibility with regard to three attributes of ocean metaproteomics analyses: (1) the identity of discovered peptides and proteins (Fig. 2), (2) their relative quantitative abundances (Figs. 4 and 6), and (3) the taxonomic and functional assignments within intercompared samples (Fig. 5). With over 1000 proteins identified across seven laboratories and Sørensen similarity indexes typically higher than 70 %–80 % (Fig. 6), the results demonstrate consistent detection and quantitation of major proteins in the sample. These results provide confidence that multiple laboratories can generate reproducible results describing the major proteome composition of ocean microbiome samples to assess their functional and biogeochemical activity.
While there is good agreement, this congregation of data allows further exploration of the influence of methods on the results. In particular, as mentioned above, the range of pairwise comparisons had correlation coefficients ranging from 0.43 to 0.84, with most values falling between 0.6 and 0.8 (Fig. 4b and e, Table S12). This average of all correlation coefficients described above (0.63±0.11) implied good reproducibility between laboratories in general. We can explore what might have influenced the variability and lower range of coefficients. The correlation coefficients of Lab 209 had two of the three R2 values below 0.499 in pairwise comparisons (0.431 and 0.475) yet also had values that ranged from 0.61 to 0.70. Why would this variability exist? Lab 209's methods differed from other labs in several ways: it used the oldest and slowest instrument of the group (Thermo Orbitrap Elite), used CID instead of HCD for fragmentation and rapid scan mode, and used an unusually long column of 200 cm to compensate for the older instrument (Table S6). As a result, Lab 209 had the lowest number of peptide (3354) and protein (1586) IDs of the seven labs (Table S9), which was several-fold lower than the lab with the highest number and reduced the number of shared peptides across all laboratories. In pairwise comparisons, Lab 209 had the lowest number of shared peptides at an average of 1304. Interestingly, however, Lab 209 did not have the lowest number of total spectral counts (63 198) but was close to the average (70 843±27 455), implying that more abundant peptides were detected relative to rarer ones.
We initially suspected that the lower R2 values in pairwise comparisons with Lab 209 may have been related to comparisons to laboratories with similarly lesser peptide depth, but this was not the case: the two lowest correlation coefficients for Lab 209 were with labs 135 and 774 (the 0.431 and 0.475 values), the latter of which had the highest number of peptide identifications. The answer for this difference in quantitative values may be within the selection of parameters used to sample peptide peaks: both Lab 135 and Lab 774 used 60 s dynamic exclusion, whereas the other five labs used dynamic exclusions between 10 and 30 s in length (Table S7). This higher dynamic exclusion likely contributed to providing greater peptide discovery depth but at the cost of quantitative consistency with other laboratories, since this parameter selects against repeat counting of abundant peaks and would reduce spectral counts of the more abundant peptides that Lab 209 was detecting. This result demonstrates the influence of the mass spectrometer parameters in quantitative reproducibility when using global proteomic DDA mode.
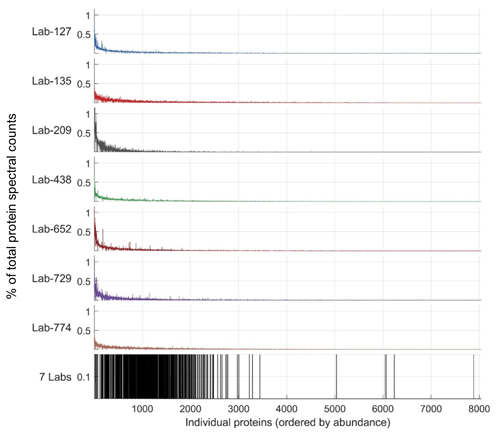
Figure 8Variability in discovered proteins between laboratories occurs in lower-abundance proteins. Top seven panels: abundance of proteins as a percentage of total protein spectral counts within each laboratory (y axis is percentage), with proteins on the x axis shown by ranked abundance as the sum of spectral counts across all laboratories. Almost all proteins fall below 1 % of spectral counts within the sample, and deeper proteomes have lower percentages due to the sharing of percent spectral counts across more discovered proteins. Bottom panel: shared proteins were found early within the long tail of discovered proteins; the 1056 proteins shared between all laboratory groups are almost all found on the left-hand side, indicating their higher abundance in all seven datasets. Scale is binary in the seventh panel, indicating presence or lack thereof in the seven labs.
4.2 Metrics in metaproteomics: core versus rare “long-tail“ proteins
While abundant proteins were consistently detected across the seven laboratories' submissions, there was substantial variability in the less abundant proteins (Fig. 2). This is evident in Fig. 8, where most of the 1063 proteins across the seven laboratories in the re-analysis were in the upper half of proteins when ranked by abundance. This simultaneous consistency in abundant proteins and diversity in rare proteins (and their respective peptide constituents) was likely a result of several factors. Firstly, the intercomparison experimental design stipulated 1D chromatography in order to provide straightforward comparisons that all laboratories could accomplish. This contributed to study consistency but also resulted in lesser proteome depth compared to more elaborate methods commonly in use, such as 2D chromatography and gas-phase fractionation. Secondly, the sample complexity of ocean metaproteomes has been shown to be enormous, with a far greater number of low-abundance peptides present than HeLa human cell lines (Saito et al., 2019). The combined effect of these factors meant that, while laboratories were able to detect abundant proteins consistently, there was considerable stochasticity associated with the detection of less abundant peptides resulting in a long tail of lower-abundance proteins discovered.
Mass spectrometer settings, such as dynamic exclusion, chromatography conditions, and variation in sample preparation methods, all likely contributed to this stochastic variability in rare peptide detection among laboratories. Moreover, while all participating laboratories used Thermo Orbitrap mass spectrometers, there were seven variants of instrument model, including some with Tribrid multiple detector capability (Table S6). While testing other mass spectrometry platforms is of interest, the trend of community Orbitrap usage in this study is consistent with the broader proteomic community, where 9 of the top 10 instruments currently used in ProteomeXchange consortium repository data submissions utilize Orbitraps as of the article submission date (Deutsch et al., 2019). When conducting analysis of environmental samples, choices can be made about instrument setup and parameters based on the scientific objectives, for example, if maximal proteome depth or robust quantitation while using a discovery approach is desired. Future intercalibration efforts enlisting more sensitive metaproteomic methods such as 2D chromatography (McIlvin and Saito, 2021), more sensitive instruments (Stewart et al., 2023), and other emerging methods can greatly improve the detection and quantitation of rarer proteins in metaproteomes, allowing exploration of the depths of state-of-the-art capabilities rather than our present emphasis on interlaboratory consistency. Moreover, the development and adoption of best practices in sample collection, extraction, chromatographic separation, mass spectrometry analyses, and bioinformatic approaches will contribute to interlaboratory consistency.
Despite the interlaboratory variability in the detected sets of rarer peptides and proteins, we interpret these to be largely robust identifications. The stringent 0.1 % peptide-level FDR threshold we use here is determined by scoring decoys: reverse-sequenced peptides that are not in our samples. Peptide assignments to these decoys model the score distribution of all incorrect peptide-spectrum matches (PSMs) in our study such that FDRs can be estimated in an unbiased way for each laboratory. However, these estimates are complicated by subtle sequence diversity within a population's proteome, which is typically not considered by proteomic software designed to analyze a single species (Schiebenhoefer et al., 2019). This diversity within metaproteomic samples results in the presence of highly similar peptides with nearly identical precursor masses that produce many of the same b- and y-ions, and this similarity is not well modeled by decoy peptides. The influence of microdiversity on metaproteomic FDR estimation using strain-specific proteogenomic databases is an important area of future exploration (Wilmes et al., 2008).
4.3 Bioinformatic intercomparison assessment
The discovery of peptide constituents of proteins within a complex ocean metaproteomic matrix was successful across all software packages tested (Fig. 7), where the metric for success is a comparable number of peptide identifications. This is a notable finding due to the highly complex mass spectra, large number of chimeric peaks present (Saito et al., 2019), and large database sizes involved in ocean metaproteomes. To our knowledge, some of these software packages had not yet been applied to ocean metaproteomes. There was also variability associated with the stringency of statistical parameters employed, which points to the challenges in assembling datasets from multiple laboratories with different depths of proteome identification.
Despite the success of this intercomparison component across software packages, there is likely considerable room for improvement in the future. As mentioned previously, ocean samples are highly complex, and there are likely additional peptides that remain unidentified using current technology, due to low-intensity peaks and co-elution with other peptides resulting in the chimeric spectra. Significant improvements in depth of analysis can be achieved through increased chromatographic sample separation and optimized (or alternative) mass spectrometry data acquisition strategies. However, there is also room for bioinformatic improvements: most DDA database-searching algorithms are unable to identify multiple peptides within a single fragmentation spectrum. Moreover, when in DDA collection mode, mass spectrometry software typically does not isolate and fragment peptides that cannot be assigned a charge state, which is a common occurrence for the low-abundance peaks within ocean samples. As a result, there is considerable room for improvement in bioinformatic pipelines to discover additional peptides. Although the application of data-independent approaches (DIAs) to oceanographic metaproteomic analysis has been limited (e.g., Morris et al., 2010), the systematic nature of ion selection and fragmentation allows a greater number of low-abundance peptides to be quantified when enough ions can be isolated to produce robust MS2 spectra.
4.4 Lessons learned and future efforts in ocean metaproteomic intercomparisons and intercalibrations
As the first interlaboratory ocean metaproteomic study, we chose to describe this study as an intercomparison rather than an intercalibration, and it served as a vehicle with which to assess the extent of reproducibility. There were several lessons learned that can be summarized here. These include the efficacy of an SDS detergent and heat treatment in lysing and solubilizing marine microbial cells embedded on membrane filters; the significant problem of data intercomparability between PSM software outputs and the need for data output standardization; and the influence of different hardware capabilities (Orbitrap generation) and their parameter settings, such as dynamic exclusion on proteome depth and quantitative comparisons of spectral counts. The development of best practices associated with sample collection, extraction, and analysis would be valuable, while also encouraging methodological improvements and backward compatibility through the use of reference samples.
Future intercalibration efforts could aim to further assess and improve upon the level of accuracy, reproducibility, and standardization in ocean metaproteome measurements. In particular, alternative modes of data collection and quantitation could also be tested in future interlaboratory comparisons, including parallel reaction monitoring (PRM), multiple reaction monitoring (MRM), quantification using isotopic labeling or tagging, and DIA methods. PRM and MRM methods allow sensitive targeted measurements of absolute quantities of peptides (e.g., copies per liter of seawater in the ocean context). As many omic methodologies applied in environmental settings operate in relative abundance modes, adding the ability to measure absolute quantities would be particularly valuable for comparisons of environments across space and time. Targeted metaproteomic methods have been deployed in marine studies using stable-isotope-labeled peptides for calibration, achieving femtomoles per liter of seawater estimates of transporters, regulatory proteins, and enzymes (Saito et al., 2014, 2015, 2020; Bertrand et al., 2013; Joy-Warren et al., 2022; Wu et al., 2019). These methods are not yet widely adopted, but, with growing interest, they could be deployed to other laboratories and incorporated into future iterations of intercomparison and intercalibration studies. DIA also has great potential in ocean metaproteome studies and is increasingly being deployed in laboratory and field studies of marine systems. Similar to this DDA intercomparison, the methodological and bioinformatic challenges of DIA could be explored during intercomparisons of analyses of ocean samples. Finally, as mentioned above, all participants of this study used Orbitrap mass spectrometers for DDA submissions, but new instrumentation, such as trapped ion mobility spectrometry time-of-flight (timsTOF) mass spectrometers, may be applied to ocean metaproteome analyses and would be important to intercompare with Orbitrap platforms.
As noted above, there were also challenges in collating and comparing data outputs from various software, along with variation in how those programs conducted protein inference. For example, peptide-level data from different research groups were reported as either unmodified peptide sequences or various peptide analytes (where modifications and charge states were included with the peptide sequence), making compilation of peptide reports difficult. Similarly, at the protein level, reported proteins could be counted either before or after protein grouping, e.g., applying Occam's razor logic to peptide groupings into proteins, with the former reflecting the set of all proteins in the database that could be in the sample and the latter reflecting the minimum set required to explain the peptide data. Such issues will also contribute to challenges in the integration and assembly of data from different laboratories for large ocean datasets. While best practices for metadata and data types have been described by the community, including specific attributes important for environmental and ocean samples such as geospatial location and sample collection information (Saito et al., 2019) similar to the metadata standard recently put forward in the human proteome field (Dai et al., 2021), this study also demonstrated that there is a need for standardization of data output formats for metaproteomic results.
4.5 Metaproteomics in global ocean surveys
Understanding how the oceans are responding to the rapid changes driven by human alteration of ecosystems is a high priority. Ocean and environmental sciences have a long history of chemical measurements that are critical to assessing ecosystems and climatic change. Such measurements have been straightforward for discrete measurements, such as temperature, pH, chlorophyll, phosphate, dissolved iron, and numerous other variables. When collected over large spatial (ocean basin) or temporal (seasonal or decadal spans) scales, these datasets have been powerful in identifying major (both cyclical and secular) changes. Omic measurements represent a more complex data type, where each discrete sample can generate thousands (if not more) of units of information. This study demonstrates the power and potential for collaborative metaproteomic studies to identify key functional molecules and relate them to their taxonomic microbial sources within the microbiome from multiple lab groups. Moreover, multi-lab metaproteomics results in vastly enhanced identification of low-abundance proteins that are not identified by all research groups. Such low-abundance proteins can be more likely to change in abundance with changing environmental conditions and nutrient limitations, resulting in a more nuanced and richer investigation of marine microbial ecology and biogeochemistry with collaborative metaproteomic research. The implementation of such voluminous data is beginning to be applied on larger scales and holds great promise in improving not only our understanding of the functioning of the current system, but also the way we assess how environments are changing with continued human perturbations.
Intercomparison and intercalibration are critical activities to undertake in order to allow the comparison of omic results across time and space dimensions. With major programs underway and being envisioned, such as the BioGEOTRACES, AtlantECO, Bio-GO-SHIP, and BioGeoSCAPES efforts, the imperative for such intercalibration has grown and the need for best practices is urgent. This ocean metaproteomic intercomparison study is a valuable step in assessing metaproteomic capabilities across a number of international laboratories, demonstrating a clear consistency in measurement capability while also pointing to the potential for continued community development of metaproteomic capacity and technology.
The raw files, metagenome database (Intercal_ORFs_prodigal_metagenemark.fasta), and associated annotations (Intercal_assembly_annotations.csv) for this project summarized in Table S3 are available in the ProteomeXchange and PRIDE repository with the dataset identifiers PXD043218 (https://doi.org/10.6019/PXD043218, Participants of the Ocean Metaproteome Intercomparison Consortium, 2024) and PXD044234 (https://doi.org/10.6019/PXD044234, McIlvin and Saito, 2024). Co-located information about these datasets is available at the Biological and Chemical Oceanography Data Management Office under project 765945 (https://www.bco-dmo.org/project/765945, last access: 5 November 2024 and https://doi.org/10.26008/1912/bco-dmo.934706.1, Saito and Cohen, 2024) and on the BATS page (https://doi.org/10.26008/1912/bco-dmo.3782.6, Johnson et al., 2024). The metagenomic reads are listed under BioProject accession number PRJNA932835 in the NCBI BioProject database (https://www.ncbi.nlm.nih.gov/bioproject/, last access: 5 November 2024), and SRA accession numbers SRX19315480 and SRX19315479.
Methods for the bioinformatic intercomparison study are available in the Supplemental Methods. Supplemental Information is available as Tables S1–S11 and Figs. S1–S3. The supplement related to this article is available online at: https://doi.org/10.5194/bg-21-4889-2024-supplement.
MAS and MRM obtained OCB workshop support and drafted the experimental design with feedback from BAN, MJ, and DL acting as the Advisory Committee. SMC, WJH, DL, GJV, and JKS conducted the metagenomic analyses and assembly. JKS, MAS, MMB, MRM, and RM conducted data analysis and visualization. MRM, MAS, JAB, MVJ, and RJ conducted sample collection and/or AUV Clio operations. MAS, JKS, MRM, EMB, SMC, JRC, TJG, WJH, RLH, PJ, MJ, RK, HK, DL, JSPM, EM, SM, DMM, JN, BN, JJ, MD, GJH, RG, RM, BLN, MP, SP, AR, ER, BS, TVDB, JRW, HZ, and ZZ contributed mass spectrometry data and/or bioinformatics data for the intercomparison. JKS anonymized data submissions and conducted follow-up correspondence about methods. The article was drafted by MAS, and all authors contributed to the writing and editing.
The contact author has declared that none of the authors has any competing interests.
Identification of certain commercial equipment, instruments, software, or materials does not imply recommendation or endorsement by the National Institute of Standards and Technology, nor does it imply that the products identified are necessarily the best available for this purpose.
Publisher’s note: Copernicus Publications remains neutral with regard to jurisdictional claims made in the text, published maps, institutional affiliations, or any other geographical representation in this paper. While Copernicus Publications makes every effort to include appropriate place names, the final responsibility lies with the authors.
We thank the R/V Atlantic Explorer and the Bermuda Atlantic Time-series Study team for assistance at sea. We thank Mary Zawoysky for editorial and study anonymization assistance. We thank Magnus Palmblad, John Kucklick, and an anonymous reviewer for comments on the pre-submission version of the article. We also thank the two anonymous reviewers for their constructive comments during the review of this article.
This article is a product of the sustained efforts of a small group activity supported by the Ocean Carbon and Biogeochemistry (OCB) Project Office (NSF OCE-1850983 and NASA NNX17AB17G), based on a proposal written by Mak A. Saito and Matthew R. McIlvin. The research expedition where samples were collected was supported by the NSF Biological Oceanography and Chemical Oceanography. AUV Clio sample collection was supported by NSF OCE 1658030 and 1924554. Analyses by participating laboratories acknowledge support from the following: NSERC Discovery Grant RGPIN-2015-05009 and Simons Foundation grant no. 504183 to Erin M. Bertrand; the Austrian Science Fund (FWF) DEPOCA (project no. AP3558721) to Gerhard J. Herndl; Simons Foundation grant no. 402971 to Jacob R. Waldbauer; National Institute of Health grant no. 1R21ES034337-01 to Brook L. Nunn; the Norwegian Centennial Chair Program at the University of Minnesota for funding to Pratik D. Jagtap, Subina Mehta, and Tim J. Griffin (grant nos. NIH R01 GM135709, NSF OCE-1924554, and OCE-2019589); and Simons Foundation grant no. 1038971 to Mak A. Saito
This paper was edited by Cindy De Jonge and reviewed by two anonymous referees.
Bender, S. J., Moran, D. M., McIlvin, M. R., Zheng, H., McCrow, J. P., Badger, J., DiTullio, G. R., Allen, A. E., and Saito, M. A.: Colony formation in Phaeocystis antarctica: connecting molecular mechanisms with iron biogeochemistry, Biogeosciences, 15, 4923–4942, https://doi.org/10.5194/bg-15-4923-2018, 2018.
Bergauer, K., Fernandez-Guerra, A., Garcia, J. A., Sprenger, R. R., Stepanauskas, R., Pachiadaki, M. G., Jensen, O. N., and Herndl, G. J.: Organic matter processing by microbial communities throughout the Atlantic water column as revealed by metaproteomics, P. Natl. Acad. Sci. USA, 115, E400–E408, https://doi.org/10.1073/pnas.1708779115, 2018.
Bertrand, E. M., Moran, D. M., McIlvin, M. R., Hoffman, J. M., Allen, A. E., and Saito, M. A.: Methionine synthase interreplacement in diatom cultures and communities: Implications for the persistence of B12 use by eukaryotic phytoplankton, Limnol. Oceanogr., 58, 1431–1450, 2013.
Breier, J. A., Jakuba, M. V., Saito, M. A., Dick, G. J., Grim, S. L., Chan, E. W., McIlvin, M. R., Moran, D. M., Alanis, B. A., and Allen, A. E.: Revealing ocean-scale biochemical structure with a deep-diving vertical profiling autonomous vehicle, Science Robotics, 5, eabc7104, https://doi.org/10.1126/scirobotics.abc7104, 2020.
Buchfink, B., Xie, C., and Huson, D. H.: Fast and sensitive protein alignment using DIAMOND, Nat. Methods, 12, 59–60, 2015.
Carlson, C. A., Morris, R., Parsons, R., Treusch, A. H., Giovannoni, S. J., and Vergin, K.: Seasonal dynamics of SAR11 populations in the euphotic and mesopelagic zones of the northwestern Sargasso Sea, ISME J., 3, 283–295, 2009.
Casey, J. R., Lomas, M. W., Mandecki, J., and Walker, D. E.: Prochlorococcus contributes to new production in the Sargasso Sea deep chlorophyll maximum, Geophys. Res. Lett., 34, 2007.
Cohen, N. R., McIlvin, M. R., Moran, D. M., Held, N. A., Saunders, J. K., Hawco, N. J., Brosnahan, M., DiTullio, G. R., Lamborg, C., and McCrow, J. P.: Dinoflagellates alter their carbon and nutrient metabolic strategies across environmental gradients in the central Pacific Ocean, Nat. Microbiol., 6, 173–186, 2021.
Cohen, N. R., Krinos, A. I., Kell, R. M., Chmiel, R. J., Moran, D. M., McIlvin, M. R., Lopez, P. Z., Barth, A., Stone, J., Alanis, B. A., Chan, E. W., Breier, J. A., Jakuba, M. V., Johnson, R., Alexander, H., and Saito, M. A.: Microeukaryote metabolism across the western North Atlantic Ocean revealed through autonomous underwater profiling, Nat. Commun., 15, 7325, https://doi.org/10.1038/s41467-024-51583-4, 2023.
Coleman, M. L. and Chisholm, S. W.: Ecosystem-specific selection pressures revealed through comparative population genomics, P. Natl. Acad. Sci. USA, 107, 18634–18639, 2010.
Conway, J. R., Lex, A., and Gehlenborg, N.: UpSetR: an R package for the visualization of intersecting sets and their properties, Bioinformatics, 33, 2938–2940, https://doi.org/10.1093/bioinformatics/btx364, 2017.
Dai, C., Füllgrabe, A., Pfeuffer, J., Solovyeva, E. M., Deng, J., Moreno, P., Kamatchinathan, S., Kundu, D. J., George, N., and Fexova, S.: A proteomics sample metadata representation for multiomics integration and big data analysis, Nat. Commun., 12, 5854, https://doi.org/10.1038/s41467-021-26111-3, 2021.
Deutsch, E. W., Bandeira, N., Sharma, V., Perez-Riverol, Y., Carver, J. J., Kundu, D. J., García-Seisdedos, D., Jarnuczak, A. F., Hewapathirana, S., Pullman, B. S., Wertz, J., Sun, Z., Kawano, S., Okuda, S., Watanabe, Y., Hermjakob, H., MacLean, B., MacCoss, M. J., Zhu, Y., Ishihama, Y., and Vizcaíno, J. A.: The ProteomeXchange consortium in 2020: enabling “big data” approaches in proteomics, Nucleic Acids Res., 48, D1145–D1152, https://doi.org/10.1093/nar/gkz984, 2019.
Falkowski, P. G., Fenchel, T., and Delong, E. F.: The microbial engines that drive Earth's biogeochemical cycles, Science, 320, 1034–1039, 2008.
Fuchsman, C. A., Palevsky, H. I., Widner, B., Duffy, M., Carlson, M. C., Neibauer, J. A., Mulholland, M. R., Keil, R. G., Devol, A. H., and Rocap, G.: Cyanobacteria and cyanophage contributions to carbon and nitrogen cycling in an oligotrophic oxygen-deficient zone, ISME J., 13, 2714–2726, 2019.
Georges, A. A., El-Swais, H., Craig, S. E., Li, W. K., and Walsh, D. A.: Metaproteomic analysis of a winter to spring succession in coastal northwest Atlantic Ocean microbial plankton, ISME J., 8, 1301–1313, 2014.
Hawley, A. K., Brewer, H. M., Norbeck, A. D., Paša-Tolić, L., and Hallam, S. J.: Metaproteomics reveals differential modes of metabolic coupling among ubiquitous oxygen minimum zone microbes, P. Natl. Acad. Sci. USA, 111, 11395–11400, 2014.
Held, N. A., Sutherland, K. M., Webb, E. A., McIlvin, M. R., Cohen, N. R., Devaux, A. J., Hutchins, D. A., Waterbury, J. B., Hansel, C. M., and Saito, M. A.: Mechanisms and heterogeneity of in situ mineral processing by the marine nitrogen fixer Trichodesmium revealed by single-colony metaproteomics, ISME Communications, 1, 1–9, 2021.
Hulstaert, N., Shofstahl, J., Sachsenberg, T., Walzer, M., Barsnes, H., Martens, L., and Perez-Riverol, Y.: ThermoRawFileParser: modular, scalable, and cross-platform RAW file conversion, J. Proteome Res., 19, 537–542, 2019.
Hyatt, D., Chen, G.-L., LoCascio, P. F., Land, M. L., Larimer, F. W., and Hauser, L. J.: Prodigal: prokaryotic gene recognition and translation initiation site identification, BMC Bioinformatics, 11, 1–11, 2010.
Jagtap, P. D., Hoopmann, M. R., Neely, B. A., Harvey, A., Käll, L., Perez-Riverol, Y., Abajorga, M. K., Thomas, J. A., Weintraub, S. T., and Palmblad, M.: The Association of Biomolecular Resource FacilitiesProteome Informatics Research Group Study on Metaproteomics(iPRG-2020), J. Biomol. Tech., 34, 3fc1f5fe.a058bad4, https://doi.org/10.7171/3fc1f5fe.a058bad4, 2023.
Johnson, R. J., Bates, N., Lethaby, P. J., Smith, D., and Lomas, M. W.: Discrete bottle samples collected at the Bermuda Atlantic Time-series Study (BATS) site in the Sargasso Sea from October 1988 through December 2023, Biological and Chemical Oceanography Data Management Office (BCO-DMO) [data set], https://doi.org/10.26008/1912/bco-dmo.3782.6, 2024.
Joy-Warren, H. L., Alderkamp, A.-C., van Dijken, G. L., J Jabre, L., Bertrand, E. M., Baldonado, E. N., Glickman, M. W., Lewis, K. M., Middag, R., and Seyitmuhammedov, K.: Springtime phytoplankton responses to light and iron availability along the western Antarctic Peninsula, Limnol. Oceanogr., 67, 800–815, 2022.
Kanehisa, M., Sato, Y., and Morishima, K.: BlastKOALA and GhostKOALA: KEGG tools for functional characterization of genome and metagenome sequences, J. Mol. Biol., 428, 726–731, 2016.
Keller, A., Nesvizhskii, A. I., Kolker, E., and Aebersold, R.: An explanation of the Peptide Prophet algorithm developed, Anal. Chem, 74, 5383–5392, 2002.
Kim, S. and Pevzner, P. A.: MS-GF+ makes progress towards a universal database search tool for proteomics, Nat. Commun., 5, 5277, https://doi.org/10.1038/ncomms6277, 2014.
Kiweler, M., Looso, M., and Graumann, J.: MARMoSET–extracting publication-ready mass spectrometry metadata from RAW files, Molecular & Cellular Proteomics, 18, 1700–1702, 2019.
Kleiner, M.: Metaproteomics: much more than measuring gene expression in microbial communities, Msystems, 4, e00115-19, https://doi.org/10.1128/mSystems.00115-19, 2019.
Kleiner, M., Thorson, E., Sharp, C. E., Dong, X., Liu, D., Li, C., and Strous, M.: Assessing species biomass contributions in microbial communities via metaproteomics, Nat. Commun., 8, 1558, https://doi.org/10.1038/s41467-017-01544-x, 2017.
Leary, D. H., Li, R. W., Hamdan, L. J., Hervey IV, W. J., Lebedev, N., Wang, Z., Deschamps, J. R., Kusterbeck, A. W., and Vora, G. J.: Integrated metagenomic and metaproteomic analyses of marine biofilm communities, Biofouling, 30, 1211–1223, 2014.
Malmstrom, R. R., Coe, A., Kettler, G. C., Martiny, A. C., Frias-Lopez, J., Zinser, E. R., and Chisholm, S. W.: Temporal dynamics of Prochlorococcus ecotypes in the Atlantic and Pacific oceans, ISME J., 4, 1252–1264, 2010.
McCain, J. S. P. and Bertrand, E. M.: Prediction and consequences of cofragmentation in metaproteomics, J. Proteome Res., 18, 3555–3566, 2019.
McCain, J. S. P., Allen, A. E., and Bertrand, E. M.: Proteomic traits vary across taxa in a coastal Antarctic phytoplankton bloom, ISME J., 16, 569–579, 2022.
McIlvin, M. R. and Saito, M. A.: Online Nanoflow Two-Dimension Comprehensive Active Modulation Reversed Phase–Reversed Phase Liquid Chromatography High-Resolution Mass Spectrometry for Metaproteomics of Environmental and Microbiome Samples, J. Proteome Res., 20, 4589–4597, 2021.
McIlvin, M. and Saito, M. A.: Informatics Component: Results from a Multi-Laboratory Ocean Metaproteomic Intercomparison: Effects of LC-MS Acquisition and Data Analysis Procedures, Pride PXD044234 [data set], https://doi.org/10.6019/PXD044234, 2024.
Mikan, M. P., Harvey, H. R., Timmins-Schiffman, E., Riffle, M., May, D. H., Salter, I., Noble, W. S., and Nunn, B. L.: Metaproteomics reveal that rapid perturbations in organic matter prioritize functional restructuring over taxonomy in western Arctic Ocean microbiomes, ISME J., 14, 39–52, 2020.
Moore, E. K., Nunn, B. L., Goodlett, D. R., and Harvey, H. R.: Identifying and tracking proteins through the marine water column: Insights into the inputs and preservation mechanisms of protein in sediments, Geochim. Cosmochim. Ac., 83, 324–359, 2012.
Moran, M. A., Kujawinski, E. B., Schroer, W. F., Amin, S. A., Bates, N. R., Bertrand, E. M., Braakman, R., Brown, C. T., Covert, M. W., Doney, S. C., and Dyhrman, S. T.: Microbial metabolites in the marine carbon cycle, Nat. Microbiol., 7, 508–523, 2022.
Morris, R. M., Nunn, B. L., Frazar, C., Goodlett, D. R., Ting, Y. S., and Rocap, G.: Comparative metaproteomics reveals ocean-scale shifts in microbial nutrient utilization and energy transduction, ISME J., 4, 673–685, 2010.
Mueller, R. S. and Pan, C.: Sample handling and mass spectrometry for microbial metaproteomic analyses, in: Methods in Enzymology, vol. 531, Elsevier, 289–303, https://doi.org/10.1016/B978-0-12-407863-5.00015-0, 2013.
Nesvizhskii, A. I., Keller, A., Kolker, E., and Aebersold, R.: A statistical model for identifying proteins by tandem mass spectrometry, Anal. Chem., 75, 4646–4658, 2003.
Participants of the Ocean Metaproteome Intercomparison Consortium: Results from a Multi-Laboratory Ocean Metaproteomic Intercomparison: Effects of LC-MS Acquisition and Data Analysis Procedures, Pride PXD043218 [data set], https://doi.org/10.6019/PXD043218, 2024.
Piehowski, P. D., Petyuk, V. A., Orton, D. J., Xie, F., Moore, R. J., Ramirez-Restrepo, M., Engel, A., Lieberman, A. P., Albin, R. L., and Camp, D. G.: Sources of technical variability in quantitative LC–MS proteomics: human brain tissue sample analysis, J. Proteome Res., 12, 2128–2137, 2013.
Pietilä, S., Suomi, T., and Elo, L. L.: Introducing untargeted data-independent acquisition for metaproteomics of complex microbial samples, ISME Commun., 12, 7305, https://doi.org/10.1038/s43705-022-00137-0, 2022.
Ram, R. J., VerBerkmoes, N. C., Thelen, M. P., Tyson, G. W., Baker, B. J., Blake, R. C., Shah, M., Hettich, R. L., and Banfield, J. F.: Community proteomics of a natural microbial biofilm, Science, 308, 1915–1920, 2005.
Saito, M. A. and Cohen, N.: Scaffold-derived metaproteomic exclusive and total spectral counts associated with proteins from samples taken during R/V Atlantic Explorer cruise AE1913 from the Sargasso Sea to Northeast US shelf waters in June of 2019, MBLWHOI Library [data set], https://doi.org/10.26008/1912/bco-dmo.934706.1, 2024.
Saito, M. A., McIlvin, M. R., Moran, D. M., Goepfert, T. J., DiTullio, G. R., Post, A. F., and Lamborg, C. H.: Multiple nutrient stresses at intersecting Pacific Ocean biomes detected by protein biomarkers, Science, 345, 1173–1177, 2014.
Saito, M. A., Dorsk, A., Post, A. F., McIlvin, M. R., Rappé, M. S., DiTullio, G. R., and Moran, D. M.: Needles in the blue sea: Sub-species specificity in targeted protein biomarker analyses within the vast oceanic microbial metaproteome, Proteomics, 15, 3521–3531, 2015.
Saito, M. A., Bertrand, E. M., Duffy, M. E., Gaylord, D. A., Held, N. A., Hervey IV, W. J., Hettich, R. L., Jagtap, P. D., Janech, M. G., and Kinkade, D. B.: Progress and challenges in ocean metaproteomics and proposed best practices for data sharing, J. Proteome Res., 18, 1461–1476, 2019.
Saito, M. A., McIlvin, M. R., Moran, D. M., Santoro, A. E., Dupont, C. L., Rafter, P. A., Saunders, J. K., Kaul, D., Lamborg, C. H., and Westley, M.: Abundant nitrite-oxidizing metalloenzymes in the mesopelagic zone of the tropical Pacific Ocean, Nat. Geosci., 13, 355–362, 2020.
Saunders, J. K., Gaylord, D. A., Held, N. A., Symmonds, N., Dupont, C. L., Shepherd, A., Kinkade, D. B., and Saito, M. A.: METATRYP v 2.0: Metaproteomic least common ancestor analysis for taxonomic inference using specialized sequence assemblies–standalone software and web servers for marine microorganisms and coronaviruses, J. Proteome Res., 19, 4718–4729, 2020.
Scanlan, D. J., Silman, N. J., Donald, K. M., Wilson, W. H., Carr, N. G., Joint, I., and Mann, N. H.: An immunological approach to detect phosphate stress in populations and single cells of photosynthetic picoplankton, Appl. Environ. Microbiol., 63, 2411–2420, 1997.
Schiebenhoefer, H., Van Den Bossche, T., Fuchs, S., Renard, B. Y., Muth, T., and Martens, L.: Challenges and promise at the interface of metaproteomics and genomics: an overview of recent progress in metaproteogenomic data analysis, Expert Rev. Proteomic., 16, 375–390, 2019.
Sørensen, T.: A method of establishing groups of equal amplitude in plant sociology based on similarity of species and its application to analyses of the vegetation on Danish common, Kongelige Danske Videnskabernes Selskab, 5, 1–34, 1948.
Sowell, S. M., Wilhelm, L. J., Norbeck, A. D., Lipton, M. S., Nicora, C. D., Barofsky, D. F., Carlson, C. A., Smith, R. D., and Giovanonni, S. J.: Transport functions dominate the SAR11 metaproteome at low-nutrient extremes in the Sargasso Sea, ISME J., 3, 93–105, 2009.
Stewart, H. I., Grinfeld, D., Giannakopulos, A., Petzoldt, J., Shanley, T., Garland, M., Denisov, E., Peterson, A. C., Damoc, E., Zeller, M., Arrey, T. N., Pashkova, A., Renuse, S., Hakimi, A., Kühn, A., Biel, M., Kreutzmann, A., Hagedorn, B., Colonius, I., Schütz, A., Stefes, A., Dwivedi, A., Mourad, D., Hoek, M., Reitemeier, B., Cochems, P., Kholomeev, A., Ostermann, R., Quiring, G., Ochmann, M., Möhring, S., Wagner, A., Petker, A., Kanngiesser, S., Wiedemeyer, M., Balschun, W., Hermanson, D., Zabrouskov, V., Makarov, A. A., and Hock, C.: Parallelized Acquisition of Orbitrap and Astral Analyzers Enables High-Throughput Quantitative Analysis, Anal. Chem., 95, 15656–15664, https://doi.org/10.1021/acs.analchem.3c02856, 2023.
Tagliabue, A.: “Oceans are hugely complex';: modelling marine microbes is key to climate forecasts, Nature, 623, 250–252, https://doi.org/10.1038/d41586-023-03425-4, 2023.
Timmins-Schiffman, E., May, D. H., Mikan, M., Riffle, M., Frazar, C., Harvey, H. R., Noble, W. S., and Nunn, B. L.: Critical decisions in metaproteomics: achieving high confidence protein annotations in a sea of unknowns, ISME J., 11, 309–314, 2017.
Ustick, L. J., Larkin, A. A., Garcia, C. A., Garcia, N. S., Brock, M. L., Lee, J. A., Wiseman, N. A., Moore, J. K., and Martiny, A. C.: Metagenomic analysis reveals global-scale patterns of ocean nutrient limitation, Science, 372, 287–291, 2021.
Van Den Bossche, T., Kunath, B. J., Schallert, K., Schäpe, S. S., Abraham, P. E., Armengaud, J., Arntzen, M. Ø., Bassignani, A., Benndorf, D., and Fuchs, S.: Critical Assessment of MetaProteome Investigation (CAMPI): a multi-laboratory comparison of established workflows, Nat. Commun., 12, 1–15, https://doi.org/10.1038/s41467-021-27542-8, 2021.
Virtanen, P., Gommers, R., Oliphant, T. E., Haberland, M., Reddy, T., Cournapeau, D., Burovski, E., Peterson, P., Weckesser, W., and Bright, J.: SciPy 1.0: fundamental algorithms for scientific computing in Python, Nat. Methods, 17, 261–272, 2020.
Waskom, M. L.: Seaborn: statistical data visualization, J. Open Source Softw., 6, 3021, https://doi.org/10.21105/joss, 2021.
Williams, T. J., Long, E., Evans, F., DeMaere, M. Z., Lauro, F. M., Raftery, M. J., Ducklow, H., Grzymski, J. J., Murray, A. E., and Cavicchioli, R.: A metaproteomic assessment of winter and summer bacterioplankton from Antarctic Peninsula coastal surface waters, ISME J., 6, 1883–1900, 2012.
Wilmes, P. and Bond, P. L.: Metaproteomics: studying functional gene expression in microbial ecosystems, Trends Microbiol., 14, 92–97, 2006.
Wilmes, P., Andersson, A. F., Lefsrud, M. G., Wexler, M., Shah, M., Zhang, B., Hettich, R. L., Bond, P. L., VerBerkmoes, N. C., and Banfield, J. F.: Community proteogenomics highlights microbial strain-variant protein expression within activated sludge performing enhanced biological phosphorus removal, ISME J., 2, 853–864, 2008.
Worden, A. Z., Follows, M. J., Giovannoni, S. J., Wilken, S., Zimmerman, A. E., and Keeling, P. J.: Rethinking the marine carbon cycle: factoring in the multifarious lifestyles of microbes, Science, 347, 1257594, https://doi.org/10.1126/science.1257594, 2015.
Wu, M., McCain, J. S. P., Rowland, E., Middag, R., Sandgren, M., Allen, A. E., and Bertrand, E. M.: Manganese and iron deficiency in Southern Ocean Phaeocystis antarctica populations revealed through taxon-specific protein indicators, Nat. Commun., 10, 3582, https://doi.org/10.1038/s41467-019-11426-z, 2019.