the Creative Commons Attribution 4.0 License.
the Creative Commons Attribution 4.0 License.
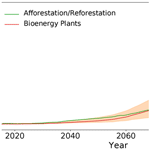
How to measure the efficiency of bioenergy crops compared to forestation
Stefanie Falk
Dorothea Mayer
Tobias Nützel
Wolfgang A. Obermeier
Julia Pongratz
The climate mitigation potential of terrestrial carbon dioxide removal (tCDR) methods depends critically on the timing and magnitude of their implementation. In our study, we introduce different measures of efficiency to evaluate the carbon removal potential of afforestation and reforestation (AR) and bioenergy with carbon capture and storage (BECCS) under the low-emission scenario SSP1-2.6 and in the same area. We define efficiency as the potential to sequester carbon in the biosphere in a specific area or store carbon in geological reservoirs or woody products within a certain time. In addition to carbon capture and storage (CCS), we consider the effects of fossil fuel substitution (FFS) through the usage of bioenergy for energy production, which increases the efficiency through avoided CO2 emissions.
These efficiency measures reflect perspectives regarding climate mitigation, carbon sequestration, land availability, spatiotemporal dynamics, and the technological progress in FFS and CCS. We use the land component JSBACH3.2 of the Max Planck Institute Earth System Model (MPI-ESM) to calculate the carbon sequestration potential in the biosphere using an updated representation of second-generation bioenergy plants such as Miscanthus. Our spatially explicit modeling results reveal that, depending on FFS and CCS levels, BECCS sequesters 24–158 GtC by 2100, whereas AR methods sequester around 53 GtC on a global scale, with BECCS having an advantage in the long term. For our specific setup, BECCS has a higher potential in the South American grasslands and southeast Africa, whereas AR methods are more suitable in southeast China. Our results reveal that the efficiency of BECCS to sequester carbon compared to “nature-based solutions” like AR will depend critically on the upscaling of CCS facilities, replacing fossil fuels with bioenergy in the future, the time frame, and the location of tCDR deployment.
- Article
(8075 KB) - Full-text XML
- BibTeX
- EndNote
Meeting the Paris Agreement's climate targets to limit global warming to well below 2 °C will likely require substantial carbon dioxide removal (CDR) (Azar et al., 2013; Roe et al., 2019; IPCC Working Group III, 2022b). CDR implies sequestering CO2 from the atmosphere and storing it for decades to millennia in the biosphere, long-lived products, geological reservoirs, or the ocean (IPCC Working Group III, 2022b). Various CDR methods exist, from conventional methods applied at large scale for decades to centuries such as afforestation and reforestation (AR) to those only being explored in the laboratory such as artificial photosynthesis (May and Rehfeld, 2022). Nearly all currently deployed CDR depends on terrestrial ecosystems that store carbon in the biosphere (Smith et al., 2023). From 2013 to 2022, bookkeeping models aligned with estimates of the Global Carbon Budget suggest between 1.2 to 2.2 GtCO2 yr−1 removed from the atmosphere through AR (Smith et al., 2024). An additional 2.3 × 10−3 GtCO2 yr−1 comes from novel CDR (Smith et al., 2023) including 1.8 × 10−3 GtCO2 yr−1 from bioenergy with carbon capture and storage (BECCS). Thereby, the CO2 that is emitted upon the combustion of biomass can be captured in geological reservoirs for thousands of years.
Scenario assessments suggest that terrestrial CDR (tCDR) measures will continue to play a major role. In contrast, projections of less-explored options such as direct air carbon capture and storage (DACCS) are more uncertain (IPCC Working Group III, 2022b). Land-based measures, including tCDR and avoided emissions from the land use, land-use change and forestry (LULUCF) sector, equally have the potential to mitigate approximately 10–15 GtCO2 eq. yr−1 by 2050, corresponding to about 20 %–30 % of the mitigation that would be needed to achieve the 1.5 °C temperature target (Griscom et al., 2017; Roe et al., 2019). Among the various tCDR approaches used in Roe et al. (2019), AR and BECCS are implemented on a large scale with the highest carbon removal. They remain the most commonly applied also in future scenarios (Fuss et al., 2014; Meinshausen et al., 2020; IPCC Working Group III, 2022b). Across the scenarios that limit the warming to 2 °C or below, agriculture, forestry, and other land use, mainly AR, remove on average about 2.98 (scenario spread of 0.23–6.38) GtCO2 eq. yr−1 and BECCS removes on average about 2.75 (scenario spread of 0.52–9.45) GtCO2 eq. yr−1 from the atmosphere in 2050 (IPCC Working Group III, 2022c). The large spread in the estimate of BECCS among models and scenarios reflects the high uncertainty regarding carbon capture and storage (CCS) feasibility in the future. Various raw materials, such as energy crops, agricultural and forest residues, and waste fractions, can be used for BECCS (e.g., Borchers et al., 2024). They include woody and herbaceous crops on agriculturally managed plantations of tall and fast-growing grasses for biomass production. Especially second-generation bioenergy crops will gain relevance in the future (Clifton-Brown et al., 2017; Awty-Carroll et al., 2023). The distinguishing characteristics of second-generation biofuels are that they use a non-food feedstock (lignocellulose biomass, field crop residues, forest product residues, or fast-growing dedicated energy crops) compared to first-generation biofuels made from sugar-starch feedstocks (e.g., sugarcane and corn) and edible oil feedstocks (e.g., rapeseed and soybean oil). We will focus here on second-generation herbaceous biomass plantations (HBPs) such as Miscanthus. To compose an efficient and sustainable portfolio of tCDR methods, AR, BECCS, or any other CDR method needs to be carefully evaluated as they differ in risks and side effects.
Despite the large carbon removal potential of AR and BECCS, uncertainties in carbon sequestration rates are high, and side effects on land use, water use, biodiversity, and equity exist (Boysen et al., 2017; Fuss et al., 2018; Cheng et al., 2020). For example, pathways that are limiting warming to 1.5 °C show a mean increase in forest cover of about 322 (−67 to 890) Mha and a mean increase in cropland area to supply biomass for BECCS of around 199 (56 to 482) Mha in 2050 (IPCC Working Group III, 2022c). The extended use of land and water for tCDR might provoke conflicts with nature conservation or agriculture and might cause deforestation, biodiversity loss, and higher food prices and put a larger population at risk of hunger and malnutrition (Creutzig, 2016; Smith et al., 2016; Humpenöder et al., 2018; Roe et al., 2019; Doelman et al., 2020). Thus, not all methods are suitable everywhere globally, and their carbon sequestration potential will evolve differently. Where risks and side effects are not precluding one method or the other, an important question is which method removes CO2 more efficiently from the atmosphere while optimizing the allocation of financial, land, and other resources. This question is surprisingly hard to answer. The carbon sequestration per square meter of forests and bioenergy crops is highly location-specific since it depends on environmental, climate, and soil conditions and will change in the future (e.g., Sharma et al., 2023). Thus, locations of tCDR deployment have to be chosen carefully. But even if the CO2 sequestration per square meter might initially be the same for different tCDR methods, the temporal dynamics differ. BECCS could put similar amounts of carbon into CCS every year if the infrastructure for CCS is available, limited only by the interannual variability in biomass production. By contrast, forests show a distinct evolution of CO2 sequestration with age, which may be altered by wood harvesting in managed forests. Moreover, plant growth, soil respiration, and natural disturbances are influenced by environmental changes (Canadell et al., 2021). The time BECCS needs to take up and store a similar amount of CO2 as forests will further depend on how much of the CO2 is transferred to geological storage or released to the atmosphere beforehand. In addition to CCS, bioenergy crops are typically used for energy production, which enables fossil fuel substitution (FFS), meaning that the energy production from fossil fuels is replaced by bioenergy. However, in practice, biomass production losses and energy conversion reduce the FFS potential of biomass (Chum et al., 2011; Babin et al., 2021), e.g., due to transport emissions and indirect land-use change displacing the prior land use to other regions. Such and other emissions along the process chain can be captured by life cycle assessments (LCAs). The goal of LCAs is to quantify the environmental effects, such as energy use, resource depletion, and emissions, across the entire life cycle of a product or service. The processes considered in LCAs, and thus the emissions avoided through substitution, depend on the choice of system boundaries. They have been found to vary across the literature for BECCS (Terlouw et al., 2021), making a crucial difference for carbon removal potential with its immanent purpose of energy production. Further, it must be considered to what extent bioenergy displaces fossil fuels in practice (Kalt et al., 2019; Cheng et al., 2022).
These remarks reveal that various aspects need to be considered when assessing a certain tCDR target, either in absolute terms or in comparison to another CDR method. However, these aspects are typically not disentangled in studies that evaluate the future deployment of CDR, which limits our ability to understand the levers to deploy CDR methods efficiently. Several studies have assessed the carbon sequestration and climate mitigation potential of AR (Sonntag et al., 2016; Matthews et al., 2022), BECCS (Harper et al., 2018; Muri, 2018), or both (Krause et al., 2017; Melnikova et al., 2023; Cheng et al., 2024) using earth system models (ESMs) and dynamic global vegetation models (DGVMs). However, a direct comparison of the AR and HBP carbon sequestration potential in the same areas was only conducted by Melnikova et al. (2023) within a consistent setup. Many studies use abandoned agricultural areas for AR under different climate scenarios (Sonntag et al., 2016; Jayakrishnan and Bala, 2022). Others build on the output of integrated assessment models (IAMs) to determine the spatial distribution of AR and BECCS in different areas within the same or even different scenarios (Krause et al., 2017; Harper et al., 2018; Cheng et al., 2022, 2024). These different assumptions on the land area used for the CDR methods result in significant differences in the estimated tCDR potential across studies (IPCC Working Group III, 2022c). Krause et al. (2017) find a larger spatial extent needed for avoided deforestation in combination with AR compared to BECCS to reach a similar carbon sequestration potential. However, there is no further exploration of the sensitivity of results concerning the time horizon, the amount of CCS, or the substitution achieved.
In this study, we propose several measures that reflect biogeochemical mitigation efficiency, defined as the combined carbon sequestration, storage, and substitution potential of a tCDR method (hereafter tCDR potential). These measures include the spatiotemporal dynamics, i.e., the change in the tCDR potential over time and space; the level of FFS and CCS needed to achieve a given tCDR potential; and the area required to achieve a given tCDR potential. This is to our knowledge the first study that compares BECCS to AR in the same location within a consistent setup to evaluate different measures of efficiency. In addition, this is the first study to account for FFS together with CCS by a land surface model. We quantify results for BECCS using HBPs and AR in the same area under different assumptions on FFS and CCS over the 21st century. We use the state-of-the-art land surface model JSBACH3.2 extended by a dedicated representation of HBPs and CCS. We use environmental conditions from the low-emission scenario SSP1-2.6, representing a scenario compatible with the 2 °C target. The SSP1-2.6 land-use scenario, which is based on the IAM IMAGE 3.0 (van Vuuren et al., 2017), projects a substantial gain in land for second-generation biofuels (up to 330 Mha), which mainly replaces pasture (Hurtt et al., 2020). We use this area for BECCS, and alternatively for AR, to assess the efficiency of both methods within a consistent setup. As the spatial distribution of bioenergy crops from IAMs is not primarily based on climate-related factors but rather on socioeconomic factors (van Vuuren et al., 2017), forests do not necessarily have a disadvantage in those areas. Evaluating our different proposed measures of efficiency, we provide novel insights into the following research questions:
-
Which of the two tCDR methods, BECCS and AR, has a higher carbon removal potential per area?
-
At which level of FFS and CCS does BECCS become more efficient than AR in removing carbon from the atmosphere?
-
How does the efficiency of the two tCDR methods evolve until the end of the century?
-
How much additional land does BECCS need to reach the efficiency of AR?
In this study, we focus on the carbon sequestration and substitution potential of AR and BECCS and do not assess the side effects of tCDR. Socioeconomic considerations, including side effects, are implicitly accounted for in the IAM land-use scenarios. In addition, a comprehensive assessment covering ecological side effects and impacts of governance and societal acceptance would be needed to evaluate the overall suitability of tCDR methods under certain normative targets.
2.1 Terrestrial carbon dioxide removal (tCDR) methods in JSBACH3.2
We use the land component JSBACH3.2 (Raddatz et al., 2007; Reick et al., 2021) of the Max Planck Institute Earth System Model (MPI-ESM) (Mauritsen et al., 2019). JSBACH3.2 participated in large international intercomparison studies (e.g., LUMIP; Lawrence et al., 2016, within the CMIP6 framework; Eyring et al., 2016), is evaluated against observational data for various ecosystem indicators (e.g., TRENDY; Friedlingstein et al., 2023), and is state of the art concerning land management implementation. We extend JSBACH3.2 by a new plant functional type (PFT) originally implemented by Mayer (2017). This new PFT represents the specific physiology and phenology of highly productive herbaceous biomass plantations (HBPs) such as Miscanthus. We revised several photosynthetic parameters of Mayer (2017) because more recent and accurate data are available now (Li et al., 2018a). The tested parameter values based on observations for the HBP PFT in JSBACH3.2 can be found in Nützel (2024). We connected HBPs with the nitrogen cycle and the latest soil model Yasso in JSBACH3.2 (Goll et al., 2015). These perennial C4 crops grow under most climates and are even frost-tolerant (Naidu and Long, 2004). Their stems grow to 3–4 m in height, allowing them to produce more biomass per area than first-generation bioenergy crops. They produce leaves up to a maximum leaf area index (LAI) of 9 m2 m−2 (LeBauer et al., 2018). The dried stems and leaves provide feedstock for coal power plants or pyrolysis for the production of biofuels. Outside the tropics, plants typically remain in the fields throughout the winter to dry because they are better suited for burning if the moisture content is low and the later harvest enables nutrient translocation back to the rhizome (Clifton-Brown et al., 2010). They are harvested before the new growing season when a specific heat sum is exceeded (Frühwirth et al., 2006), which allows nutrients such as nitrogen to leach back into the soil reducing the need for fertilization (Clifton-Brown et al., 2017). In particular, under a temperate climate, this reduces soil erosion and soil carbon and nutrient loss compared to conventional cultivation of annual crops usually harvested in autumn. Within the tropics, they are harvested at the beginning of the new year within the model. JSBACH3.2 does not explicitly account for crop management such as irrigation or fertilization. Instead, fertilization is simulated by returning the harvested nitrogen of HBPs to the soil over the year, similar to the default treatment for the harvested nitrogen of crops in JSBACH3.2 (Reick et al., 2013). By doing this, we implicitly represent N fertilization as replenishing nutrients removed by annual harvesting, which has been suggested to otherwise limit bioenergy production (Li et al., 2021). HBPs require generally less management and fertilizer input than annual crops (Christian et al., 2008). By affecting water or nutrient supplies, management can influence how much plants must invest in roots. Especially the water availability influences rooting depth and the extent of root networks (Ercoli et al., 1999). These root-to-shoot ratios range from 0.4 to 0.8 (Meyer et al., 2010), corresponding to a shoot fraction between 0.55 and 0.71 of the total biomass, meaning that 55 % to 71 % of the total biomass production (above- and belowground) is harvested (Mayer, 2017). We take the mean of these values and assume a harvested fraction of 63 % in this study. The previous implementation of HBPs in JSBACH has been evaluated against observational data for yields and water use efficiency with satisfactory results (Mayer, 2017). A comparison against observational data of the updated HBP version used in this study is provided in Sect. 2.4 and shown in Fig. 2.
We assume that the type of forest chosen for AR reflects the current preferences of existing forests. To represent AR in JSBACH3.2, we increase the fraction of the existing forest PFTs (tropical broadleaf evergreen trees, tropical broadleaf deciduous trees, extratropical evergreen trees, and extratropical deciduous trees) proportionally to their current fraction in each grid cell. Thus, AR is represented as natural regrowth rather than fast-growing wood plantations in the model. To eliminate confounding effects of carbon sequestration through temporary forest regrowth in shifting cultivation, we use net instead of gross land-use transitions in our simulations (Wilkenskjeld et al., 2014). In the underlying land-use scenario (see Sect. 2.2), the areas used for AR and HBPs increase monotonously over time, and shifting cultivation plays a minor role. We assume that the forests grow in the same areas as HBPs. While the climate, soil, and ecological conditions might not be favorable for forests in these regions, this setup is adequate for the aim of our study, which is a comparison of CDR methods at the same location since our focus is on comparing the different measures of efficiency. The low CDR potential of the forest due to unsuitable conditions would be captured by our model and thereby could find its way into subsequent decision-making processes.
2.2 Land use and climate forcing
The land surface is prescribed by spatial maps of land-use and land cover classes derived from the Land-use Harmonization Project version 2 (LUH2) (Hurtt et al., 2020). LUH2 includes a harmonized set of land-use scenarios that smoothly connects historical reconstructions of land use from HYDE 3.2 and estimates of historical global wood harvest for 850–2015 (Klein Goldewijk et al., 2017) with multiple future scenarios provided by IAMs as spatiotemporal global maps. The future spatial extent of second-generation biofuels of LUH2 is given as a fraction of cropland for every grid cell and year. In the SSP1-2.6 land-use scenario from IMAGE 3.0 (Stehfest et al., 2014; van Vuuren et al., 2017), the plantation of second-generation biofuels begins in 2015. They expand mainly on former pasture land to a global area of 330 Mha by 2095, which decreases slightly afterward until 2100 (Fig. 1b). While second-generation biofuels in IMAGE 3.0 include dedicated herbaceous and woody energy crops (van Vuuren et al., 2017), LUH2 does not differentiate between herbaceous and woody bioenergy. Thus, we consider only HBPs in JSBACH3.2. To assure a consistent comparison, the same areas occupied by HBPs are used for the establishment of new forests in our counterfactual AR simulation.
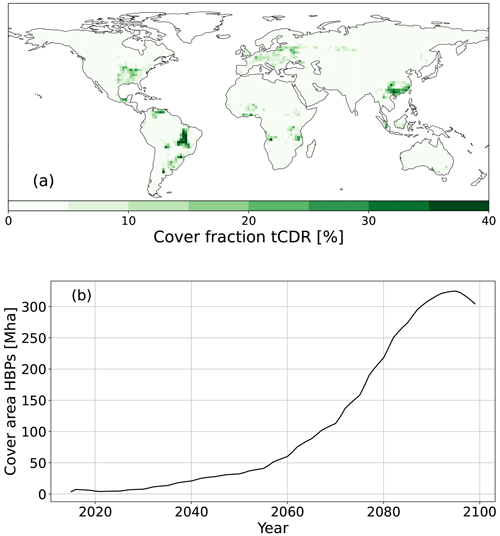
Figure 1(a) Fraction of grid cell that is covered by a tCDR method (HBPs or AR) in 2100 and (b) change in global land area [Mha] covered by HBPs or AR as compared to 2015 according to the implementation of the LUH2 SSP1-2.6 land-use scenario in JSBACH3.2.
We use bias-corrected downscaled climate forcing of MPI-ESM1.2-HR (Gutjahr et al., 2019) for SSP1-2.6 from the Inter-Sectoral Impact Model Intercomparison Project (Hempel et al., 2013). MPI-ESM1.2-HR represents a climate model with low climate sensitivity (Meehl et al., 2020). The Inter-Sectoral Impact Model Intercomparison Project (ISIMIP) climate forcing is provided daily at 0.5° spatial resolution. The climate data are remapped conservatively to T63 resolution using the climate data operators (CDOs; Schulzweida, 2023). The daily climate data are transformed into sub-daily information needed in JSBACH3.2 through an internal weather generator. Climate data from 2015 to 2100 are available in Lange and Büchner (2021). We plot spatial changes in mean temperature and precipitation between 2005–2024 and 2080–2099 in Fig. A1 in Appendix A.
2.3 Simulation setup
We simulate the carbon sequestration potential of AR and HBPs using JSBACH3.2 in its low-resolution configuration with a T63 global grid (corresponding to 1.875° × 1.875° at the Equator). We perform a spinup of 5000 years to equilibrate the carbon and nitrogen pools followed by a historical run from 1700 to 1850 with cyclic climate forcing (1850–1870) and historical land-use change from LUH2 (see Sect. 2.2) (Hurtt et al., 2020). The historical period continues from 1850 to 2015 with transient climate and CO2 forcing and land-use change. Future projections start in 2015 and run until 2100 using LUH2 SSP1-2.6 land use and SSP1-2.6 climate forcing. The simulations include disturbances of forests by wildfires and wind throw (Thonicke et al., 2010; Lasslop et al., 2014) and wood harvest from 1700 onward. We use the default product pool fractions from wood harvest in JSBACH3.2, which are PFT-specific and constant over time. In the HBPs simulation (C1HBP), the future spatial extent of HBPs is derived from the LUH2 layer that indicates the fraction of cropland used for second-generation biofuels in every grid cell and year. We compare the carbon sequestration potential of the newly implemented HBPs and AR by replacing the area occupied by HBPs in C1HBP with forests in the AR simulation (C1AR). Figure 1a shows the spatial extent of second-generation biofuels in 2100 based on the SSP1-2.6 scenario of LUH2 as implemented in JSBACH3.2 (Hurtt et al., 2020).
Additional wood harvest in the afforestation and reforestation scenario
The wood harvest used in JSBACH3.2 is calculated by IAMs based on regional demands for wood products and harmonized by LUH2 (Hurtt et al., 2020). However, LUH2 does not provide a demand-based estimate of additional future carbon removal due to wood harvest in C1AR. Hence, we keep the absolute amount of wood harvest equal in all simulations following LUH2 SSP1-2.6.
We give a rough supply-driven estimate of carbon that might be stored in woody products or used for energy generation due to wood harvest. We did not find any dataset that projects how far a new forest is managed or left to natural regrowth. Thus, we assume that the new forest is managed similarly to the existing forest in the same grid cell. We implement this by increasing the absolute amount of wood harvest following LUH2 SSP1-2.6 by a ratio that reflects the additional vegetation carbon that AR areas provide. We assume that all additional bioenergy from the wood harvest is produced without CCS and FFS. We acknowledge this is just a first-order estimate, ignoring that the carbon balance of wood harvest is time-dependent and impacted by many factors, including the forest age, its use for energy or products, and regional climate and environmental conditions. Future studies could apply our framework to investigate different assumptions on wood harvest in AR areas.
where whAR is the global additional wood harvest of AR [kg], whLUH2 is the global demand-based wood harvest of LUH2 [kg], y is year, and Ctree is the global tree vegetation carbon in the C1AR or C1HBP scenario [GtC].
2.4 Model evaluation with observational yield data
We evaluate the HBP yields of our revised model version against a recent comprehensive global dataset of bioenergy crop yields compiled from scientific literature (Li et al., 2018a). It includes 990 observations of Miscanthus yields with and without irrigation and fertilizer amendment. Note that the observational sites are concentrated in the eastern USA and Europe, and no observations in the tropics exist. For the comparison, we use all available Miscanthus yields, regardless of whether fertilizer or irrigation was applied because the yields do not differ significantly from untreated yields (Li et al., 2018a; Littleton et al., 2020). We run simulations from 1980 to 2010 forced by WATCH–ERA-Interim climate data (Weedon et al., 2014) mapped to T63 spatial resolution using conservative remapping. In our setup, 10 % of the vegetated area in each grid cell is covered with HBPs to account for a more realistic scenario than fully covering the whole grid cell with HBPs. We apply a carbon-to-dry-matter ratio of 0.5 such that 1 t of dry biomass could substitute 0.5 t of carbon (Cannell, 2003). We compare the modeled HBP yields in the grid cell of the specific site to observed Miscanthus yields compiled in Li et al. (2018a) for the respective year or years if multiple (compare Figs. 2 and 3).
We find an observed mean HBP yield of 12.7 t (dry matter) DM ha−1 yr−1 and median of 11.5 t DM ha−1 yr−1 for all sites and a modeled yield mean of 12.1 t DM ha−1 yr−1 and median of 12.7 t DM ha−1 yr−1 across all respective grid cells and respective years. The maximal observed yield is 52.2 t DM ha−1 yr−1 and the maximal modeled yield is 22.3 t DM ha−1 yr−1 (Table 1). Low yields of less than 4 t DM ha−1 yr−1 are much more common in the observations (Fig. 3) (Li et al., 2018a). Lower maximal and higher minimal modeled yields as compared to observations might be caused by the averaging effect within the large extent of the modeled grid cells, which might include other areas with sparse plant growth compared to the observation sites. Higher diversity in observed yields emerges due to different local conditions (soil, micro-climate), different management techniques (irrigation, fertilization) (Mayer, 2017), or different cultivars of Miscanthus (Littleton et al., 2020; Awty-Carroll et al., 2023). Therefore, we also show the spatiotemporal median values of the observations for every grid cell (Fig. 3b). Low and high values are thereby ruled out, and the frequency distribution agrees better with the one of modeled yields.
Compared to other modeling studies (Li et al., 2018b; Littleton et al., 2020), HBP productivity in JSBACH3.2 is similar in the middle and high latitudes but lower in the tropics, where no observations exist. Hence, our estimate of HBP efficiency is rather conservative in the tropics. We find smaller maximal yields compared to other modeling studies (Table 1) that could be due to their higher spatial resolution (0.5° × 0.5°) and generally higher yields in the tropics (Littleton et al., 2020; Li et al., 2018b). While the global mean of the modeled yields agrees well with the observations, there are large regional differences for the single sites (Fig. 3), similar to Littleton et al. (2020) and Li et al. (2018b) due to the low spatial resolution of models.
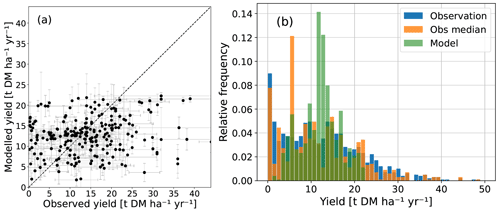
Figure 3Modeled yields with harvest fraction 63 % compared to observed yields of Miscanthus from Li et al. (2018a). In (a), the observed range (horizontal error bars) accounts for variation between sites and fertilizer or irrigation treatment if different sites exist within a grid cell; the modeled range (vertical error bars) reflects interannual variability if several observed yields in different years correspond to the same grid cell. The dashed line represents the 1 : 1 line. In (b), the relative frequencies of observed values, observed median values for every grid cell, and modeled values are shown. This figure is similar to Fig. 3e–f in Li et al. (2018b) and Fig. 5 in Littleton et al. (2020).
2.5 Measures of efficiency
We define the efficiency of a tCDR method for a certain year as the sum of the annual mean carbon sequestered in the biosphere, the emitted carbon avoided by FFS, and the carbon stored in products and geological reservoirs (CCS) since the start year y0 (Mayer, 2017). The carbon sequestered in the biosphere is the change in carbon density in the vegetation, soil, and litter times the spatial extent of the tCDR method. Carbon removal from the atmosphere is achieved by increasing the carbon density; extending the area of AR and HBPs; or increasing the share of FFS, CCS, or carbon storage in long-lived products of harvested biomass. Since we compare AR and HBPs in the same areas, differences in the CDR potential are due to the environmental, climate, and CO2 impact on carbon densities and the level of FFS (fFFS) and CCS (fCCS) in this study. For our main analysis, we use a default value of 50 % for fFFS, which is the mean provided by Gallagher (2008). fCCS is based on a decadal time series of primary energy production from biomass with and without CCS from the CMIP6 AR6 database (Byers et al., 2022) for the SSP1-2.6 scenario calculated with IMAGE 3.0 (Fig. 4 and Eq. 2; van Vuuren et al., 2017; later on referred to as SSP1-2.6 CCS rates).
Note that biomass here includes by definition purpose-grown bioenergy crops, crop and forestry residue bioenergy, municipal solid waste bioenergy, and traditional biomass. However, we assume that fCCS is similar for biomass from second-generation bioenergy. In this scenario, 20 % of primary energy from biomass is produced with CCS in 2050 and around 58 % in 2100. We assume that the share of primary energy production for biomass with and without CCS is equivalent to the share of HBPs with and without CCS and interpolate the time series linearly over time.
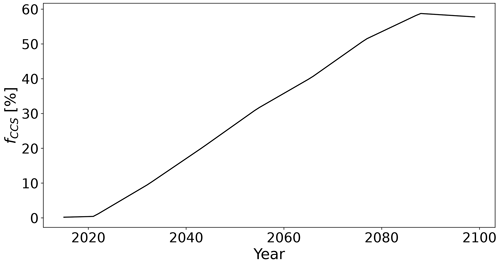
Figure 4Fraction of primary energy from biomass with CCS on primary energy from biomass provided by the CMIP6 AR6 database (Byers et al., 2022) for the SSP1-2.6 scenario calculated with IMAGE 3.0 for every decade and interpolated in between.
The spatial carbon removal potential of AR and HBPs is calculated by the following equations:
where C is the total carbon captured by AR or HBPs [kg], CL is land carbon (vegetation, soil, and litter) of AR or HBPs [kg], is the annual mean change in carbon density of AR or HBPs compared to the start year [kg m−2 (vegetation)], A is the area of AR or HBPs [m2 (vegetation per grid cell)], HHBPs is the harvested carbon of HBPs [kg], fFFS is the efficiency of fossil fuel substitution (FFS) [%], fCCS is the efficiency of carbon capture and storage (CCS) [%], y is year, and y0 is the start year.
Since the levels of FFS and CCS are additive, we can use them simultaneously to analyze their effect on the area-wise CDR potential for AR and HBPs. Overall, we identify the following three measures for CDR efficiency:
-
the level of FFS or CCS needed for HBPs to exceed the efficiency of AR in 2100, which is calculated as
-
the year in which the 5-year running mean of carbon sequestrated by HBPs exceeds the one of AR for the first time, which is calculated as
-
the additional area needed per grid cell for HBPs to reach the efficiency of AR, which is calculated as
where is the combined level of fossil fuel substitution and carbon capture and storage, and aHBPs is the area of HBPs needed to reach a similar efficiency as AR [m2].
All grid cells for which the HBP fraction is smaller than 0.1 % are neglected to avoid numerical artifacts.
In this section, we at first assess the efficiency of carbon removal from the atmosphere by the two tCDR methods in an SSP1-2.6 land-use and climate scenario, which includes carbon sequestration in vegetation, litter, soil, and the proportion of harvested HBP yield used for FFS and CCS. We further evaluate the different measures of efficiency described in Sect. 2.5, i.e., the level of FFS, the temporal dynamics, and the area of cultivation comparing AR and HBPs. We differentiate between HBPs with CCS (equivalent to BECCS) and without CCS.
3.1 Efficiency of AR and HBPs
The amount of CDR realized by HBPs and AR in the same areas in 2100 differs substantially. The key factors determining differences between both are assumptions on levels of CCS and FFS for BECCS (Fig. 5a). For 50 % FFS and SSP1-2.6 CCS rates (Fig. 4), the carbon potential of HBPs outpaces the one of AR after 2071 and is about twice as high towards the end of the century. HBPs become even more efficient in storing carbon than AR in the 100 % FFS and 100 % CCS case (a factor of 3). In contrast, if theoretically no FFS and no CCS are assumed for HBPs – i.e., all bioenergy is used in addition to fossil-fuel-based energy – AR is more efficient towards the end of the 21st century, storing about twice as much carbon as HBPs. Depending on FFS and CCS levels, HBPs sequester 24–158 GtC by 2100, whereas AR methods sequester around 53 GtC. The accumulated harvested HBP yield by 2100 is 67 GtC, reaching levels of about 2.5 GtC harvested per year towards the end of the century (Fig. 5b). The amount of CCS accumulates to 34 GtC by 2100 assuming SSP1-2.6 CCS rates. The difference in tCDR potential only becomes substantial after around 2070, when the land conversion to tCDR increases rapidly in the SSP1-2.6 land-use scenario (Fig. 1). The cumulative global wood harvest of forests without AR estimated by the LUH2 data (whLUH2(2100)) between 2015 and 2100 is 91.6 GtC. Since this is similar in both simulations, it does not impact our results. Our estimate based on Sect. 2.3 reveals an additional cumulative wood harvest of AR whAR of 1.29 GtC between 2015 and 2100. We argue that wood harvesting has a relatively small effect on the carbon cycle compared to the cumulative amount of 53 GtC sequestered by AR (Fig. 5). Hence, we do not further consider it in our global estimates.
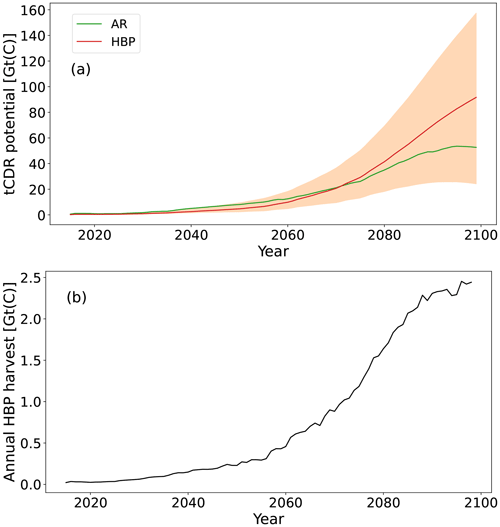
Figure 5(a) tCDR potential of AR and HBPs assuming 50 % FFS and SSP1-2.6 CCS rates from 2015 to 2100 (Fig. 4). The shaded areas indicate the range of tCDR potential without FFS and CCS to 100 % FFS and 100 % CCS. (b) Annual harvest of HBPs [GtC].
The spatial difference in CDR potential in 2100 between C1HBP with 50 % FFS and SSP1-2.6 CCS rates and C1AR is shown in Fig. 6a. The CDR potential of HBPs is almost everywhere higher compared to AR, especially in South American grasslands and southeast Africa. The only exception is southeast China, where even for 50 % FFS and with CCS, AR stores slightly more carbon. Without FFS and CCS for HBPs (Fig. 6b), the potential of AR is higher everywhere, especially in southeast China and the eastern USA. The difference in carbon stored stems mainly from the vegetation pool with its high carbon storage in trees in the AR scenario (Fig. A2a). By contrast, the soil and litter carbon pools show substantially fewer changes between the two CDR methods (Fig. A2b, c).
3.2 Level of fossil fuel substitution (FFS)
Given that for 0 % FFS, HBPs are less efficient in sequestering carbon until 2100 as compared to AR, while for 100 % FFS, HBPs exceed the CDR potential of AR in most regions, there must be an FFS level where the efficiency of HBPs and AR is similar. The lower the level of FFS needed for HBPs to reach a similar efficiency as AR, the more potential the cultivation of HBPs to remove carbon in a region has. We find that, as previously noted, even for 100 % FFS but without CCS, HBPs do not reach the CDR levels of AR in southeast China until 2100, which is one of the hotspots of tCDR deployment in SSP1.2-6 (Fig. 1). The higher CDR efficiency of AR in southeast China is partially due to the late onset of tCDR in this area (Fig. 8). However, compared to areas with a similarly late onset (e.g., the Sahel), there is a much higher level of FFS needed for HBPs, and climate and soil conditions are more favorable for AR. For example, forests benefit more strongly from the precipitation increase in southeast China towards the end of the century (Fig. A1), whereas dryer conditions in the Sahel are likely more favorable for HBPs. In some areas of Eurasia, the east coast of the USA, and South America, the level of FFS without CCS needed for HBPs to exceed the efficiency of AR in 2100 is very high (> 80 %) (Fig. 7a). The FFS level needed for HBPs to exceed the efficiency in AR is at a medium level, between 50 % and 80 %, in Europe, the Congo basin, South American grasslands, and the eastern USA. For areas with a late tCDR onset (eastern USA, Europe), climate and soil conditions are most likely more favorable for HBPs. For sub-Saharan Africa, the Australian coast, and Argentina the FFS level needed is the lowest (< 50 %). Despite the late onset of tCDR, the FFS level in the Sahel is low, showing that dryer climate and soil conditions are more favorable for HBPs compared to forests. With SSP1-2.6 CCS rates, HBPs become more efficient in 2100 than AR even without additional FFS (Fig. 7b). Even in regions where AR is more efficient without CCS and with a late onset of tCDR, such as Eurasia, the east coast of the USA, and southeast China, HBPs become more efficient with CCS and around 50 % FFS.
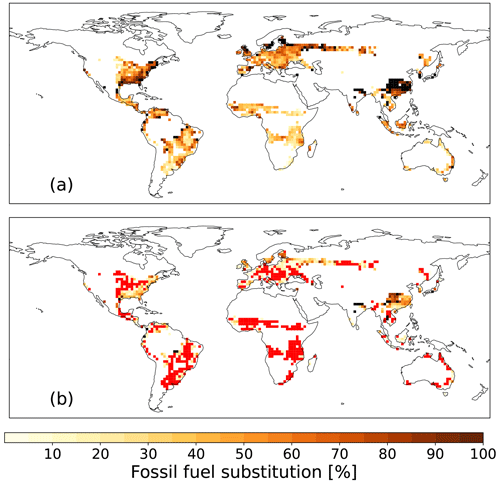
Figure 7Level of FFS [%] needed for HBPs to exceed the efficiency of AR in 2100 (a) without carbon capture and storage (CCS) and (b) for SSP1-2.6 CCS rates. The black color indicates that HBPs do not exceed the effectiveness of AR even with 100 % FFS, while the red color indicates grid cells where HBPs exceed the efficiency of AR without FFS.
3.3 Temporal dynamics in the SSP1-2.6 scenario
In our simulations, bioenergy plants store more carbon in the soil than AR in the vegetation in Eurasia and the eastern USA. In contrast, forests can sequester more carbon in the vegetation and litter over long periods (Fig. A2). If the harvested carbon from HBPs is used for FFS or stored (CCS), HBPs might become more efficient in removing carbon from the atmosphere over time because they regrow quickly and are harvested annually. Additionally, forests tend to absorb less carbon with increasing age (Pugh et al., 2019), compensating/fostering effects of rising/falling CO2 levels, which enhance/reduce net ecosystem productivity even in old-growth forests (Luyssaert et al., 2008, 2021). However, forest age is not represented explicitly in JSBACH3.2. Instead, the impact of forest age on plant productivity is only implicitly represented through structural limits. Assuming 50 % FFS and SSP1-2.6 CCS rates, HBPs become on average more efficient around 2070 (Fig. 5).
We evaluate the time between the onset of tCDR and the year in which the carbon harvested and stored through HBPs will exceed that of forests spatially if 50 % FFS of HBPs is assumed. The year of tCDR onset is defined as the year when the respective tCDR method covers more than 0.1 % of the grid cell. We find that in southeast China and some areas of the eastern USA and Eurasia, HBPs do not reach the potential of AR without CCS until 2100 (Fig. 8a). Note that in these areas the tCDR onset happens late in the century, between 2060 and 2070 (Fig. 8c). Especially in eastern Europe, HBPs become already more efficient than AR shortly after their plantation, whereas in the South American grasslands HBPs need between 20 and 50 years to become more efficient despite in both regions the planting starting in the first half of the 21st century. With CCS (Fig. 8b), the period for HBPs to become more efficient is shorter. Even in those regions with lower efficiency without CCS (eastern USA, Eurasia, southeast China) and where the tCDR onset is late, HBPs become more efficient with CCS within this century.
In this specific SSP1-2.6 land-use and climate scenario, the time until HBPs become more efficient than AR depends very much on the regional climate and soil conditions. For example, although the tCDR onsets in southeast China and the Sahel appear after 2060, the time until HBPs become more efficient is much shorter in the Sahel compared to southeast China due to a drying trend in the former and a wetting trend in the later region (Fig. A1). The additional implementation of CCS will shorten this time or even enable HBPs to become more efficient within the century. The potential of AR to store additional carbon in aboveground biomass decreases over time, whereas the cumulative harvest of HBPs increases steadily. Thus, HBPs have an advantage over time in most regions, especially with CCS in addition to FFS.
In Fig. A3, we show the relative tCDR potential from HBPs compared to AR globally as a function of different levels of FFS for various years. We find that, without CCS, HBPs only become more efficient than AR at a level of FFS above 50 % and never before 2060. With CCS, HBPs become more efficient at any level of FFS by 2100 but also in this case never before 2060. This confirms our finding that HBPs only exceed the efficiency of AR over long periods, independently of the level of FFS and assuming plausible CCS.
3.4 HBP area needed to reach efficiency of forests
The area needed to reach a specific carbon removal target is an important measure of a tCDR method because land-use conflicts with, e.g., agriculture and nature conservation, emerge from the tCDR implementation. Thus, decision-makers need to know how much area is necessary to fulfill a specific carbon sequestration target. We find that more than 4000 km2 per grid cell of bioenergy plantations is necessary in southeast China when no CCS is assumed (Fig. 9a), as compared to AR in our SSP1-2.6 scenario. This area corresponds to roughly 12 % of the land given the size of a grid cell is up to 43 000 km2 in the tropics and about 20 000 km2 in the higher latitudes (62.5° N). In Russia, the east coast of the USA, and southeast China a larger area is needed for HBPs than for AR to reach the same efficiency. In contrast, in Europe, sub-Saharan Africa, the Australian coast, and the eastern USA less area is needed for HBPs compared to AR. In the South American grasslands and southeast Africa, much less area of HBPs is needed to be as efficient as AR. With CCS (Fig. 9b), HBPs need less area to be as efficient as AR in almost all regions, except for southeast China. In the South American grasslands, more than 4000 km2 of additional area is needed for AR to reach the efficiency of HBPs.
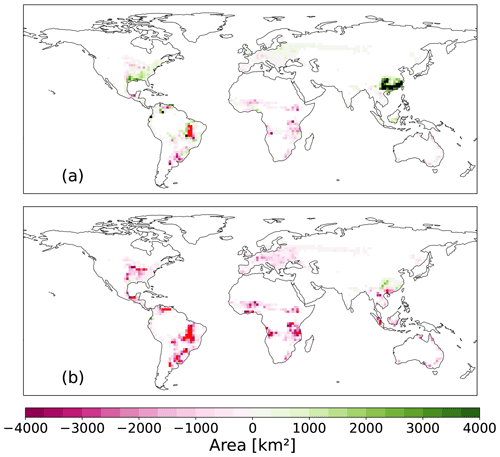
Figure 9Additional HBP area [km2] that is needed per grid cell to reach the efficiency of AR in 2100 assuming 50 % FFS (a) without CCS and (b) with CCS. The red color indicates grid cells where the implementation of HBPs could spare more than 4000 km2 of land area to reach a similar efficiency as AR.
Due to the annual HBP harvest and the saturation of carbon sequestration in forests, HBPs have an advantage over forests in the long term. Without FFS and CCS, HBPs sequester less carbon than AR globally by 2100. In the case of 50 % FFS and SSP1-2.6 CCS rates (Fig. 4), the efficiency of HBPs is higher in most regions compared to AR by the end of the century. There are substantial regional differences in CDR potential in the SSP1-2.6 scenario. In southeast China, the east coast of the USA, and parts of Eurasia, AR is more efficient than cultivating HBPs. In these regions, HBPs reach the efficiency of AR later, at a higher FFS level, or if more area is available. However, the onset of tCDR in these regions happens mostly after 2050 in the SSP1-2.6 scenario, and thus, they have less time to become established. In contrast, in the South American grasslands and southeast Africa, where the onset of tCDR happens before 2050, HBPs have an advantage over AR in that the efficiency is reached earlier and at lower levels of FFS or less area is needed. In other regions, such as the eastern USA, Europe, sub-Saharan Africa, and Central America, it depends mainly on the level of CCS and FFS at which the measure of tCDR is more efficient. Under climate change, forests and bioenergy plants likely take up more carbon from the atmosphere due to the elevated CO2. The CO2 fertilization is usually higher for trees than for the C4 bioenergy plants. This effect strengthens under high-emission scenarios.
4.1 Comparison with previous studies
Table 2 shows the results of earlier DGVM and ESM studies on future tCDR potential. The mean tCDR potential of BECCS per area in our study agrees well with these studies for a similar climate scenario, while the uncertainty is very high. The high uncertainty in BECCS potentials stems from the various CCS and FFS fractions among the studies. Most studies do not consider FFS, while Cheng et al. (2024) show a very high uncertainty in FFS due to different technological and economic scenario assumptions. Several studies assume a fixed level of CCS higher than 50 %, whereas others do not consider CCS. The studies with a higher-emission climate scenario (Mayer, 2017; Melnikova et al., 2022) project a higher tCDR potential per area. BECCS exceeds the climate mitigation potential of AR globally in a fully coupled SSP5-3.4-OS overshoot scenario (Melnikova et al., 2023). In their research, the efficiency varies regionally and temporally and depends critically on the CCS conversion efficiency of bioenergy crops. Although they use a different future scenario and a different model, they also found that BECCS is more efficient on longer timescales compared to AR, similar to Zhao et al. (2024). Most ESMs in CMIP6 do not distinguish second-generation bioenergy crops from others yet (Krause et al., 2018; Harper et al., 2018; Melnikova et al., 2022). This may be a key reason why Harper et al. (2018) find a potential of only 20 to 35 GtC by 2100 to meet the 1.5 °C temperature target compared to 67 GtC in our study using the SSP1-2.6 scenario. However, the area used for bioenergy crops is higher in their study (up to 550 Mha) than in our study (up to 330 Mha). Mayer (2017) uses a previous version of JSBACH and finds a HBP harvest of 293 GtC (mean of 55 % and 71 % HBP harvest) in 560 Mha for RCP4.5. The lower productivity in our model version can be explained by the larger area, later cultivation of HBPs in the SSP1-2.6 land-use scenario, a different climate forcing, the inclusion of nitrogen limitation in our study, and the use of prescribed maps instead of land-use transitions. Harper et al. (2018) identify the land cover transition of bioenergy plants as a critical factor. If bioenergy plants replace high-carbon-content ecosystems, e.g., forests, forest-based mitigation could be more efficient for atmospheric CO2 removal than BECCS (Searchinger et al., 2018; Seo et al., 2024). In our SSP1-2.6 land-use scenario, HBPs mainly replace pasture. The net forest area increases in the future, limiting the danger of deforestation due to HBPs in our scenario. The tCDR potential of AR in our study is lower than in all other studies except Krause et al. (2018). Most of these studies use a higher-emission scenario – a larger area where AR is applied earlier.
Further studies are needed for a robust quantification of CDR potentials for BECCS and AR in all regions of the world, including specific local implementations, and under various future climate projections. Extensively testing the sensitivity of our results to assumptions concerning model implementation or specific characteristics of how a method is implemented with regard to, e.g., biomass usage (see also limitations discussed in Sect. 4.5) is beyond the scope of our study. However, our estimates of tCDR potentials are plausible in comparison to other studies and thus allow us to use them to illustrate the value of applying various measures of efficiency to compare CDR methods to each other.
Cheng et al. (2024)Harper et al. (2018)Krause et al. (2018)Melnikova et al. (2023)Mayer (2017)Wang et al. (2023)Wang et al. (2023)Cheng et al. (2024)Krause et al. (2018)Mayer (2017)Melnikova et al. (2023)Sonntag et al. (2016)4.2 Fossil fuel substitution
The future substitution of fossil fuels with bioenergy depends on several factors: (1) the energy conversion between bioenergy and fossil fuels (i.e., how much of each is needed to produce the same amount of energy) and the type of biofuel and displaced fuels, (2) the carbon content of the bioenergy and the fossil fuel, and (3) to what extent bioenergy is displacing fossil fuels. In addition, the FFS potential of bioenergy reduces through production and transport losses along the process chain (Babin et al., 2021). We do not perform an LCA in our study but include additional emissions through production losses implicitly in the FFS fraction. Cheng et al. (2022) prescribe a conversion factor of up to 234 % assuming that bioenergy is fully displacing fossil fuels and assuming maximal inefficient fossil-fuel-to-energy conversion. As these assumptions are unlikely, we used a more plausible level of FFS between 0 % and 100 % with a default of 50 %, as in Gallagher (2008) (FFS 30 %–70 %) and similar to the assumptions of Kalt et al. (2019). They consider different scenarios, including one with an FFS factor between 0 % and 90 % depending on the energy conversion and type of displaced fuel and one with a dynamic FFS assuming a declining FFS factor over time from 55 %–70 % in 2020 to 25 %–40 % in 2100 due to the upscaling of renewable energy sources. In our simulations, we chose a constant level of FFS because we assume that bioenergy and other renewables replace fossil fuels in a similar way (van Vuuren et al., 2017). In addition, we investigate the impact of different levels of FFS in Fig. A3. The IPCC Special Report on Renewable Energy Sources and Climate Change Mitigation provides FFS factors between −9 % for diesel and 78 % for coal from several local studies (Chum et al., 2011). Because the FFS factor can vary in space and time, we support our study with a sensitivity analysis, where we determine the tCDR potential of HBPs relative to AR over time in an SSP1-2.6 scenario and as a function of the level of FFS (Fig. A3), inspired by Kalt et al. (2019). We find that an increasing level of FFS reduces the time until HBPs become more efficient than AR, but this happens in no case before 2060.
4.3 Carbon capture and storage
The latest IPCC assessment report estimates about 8.7–211 (median 90.3) GtC (1.5 °C temperature target) and 47–177 (median 78.6) GtC (2 °C temperature target) captured from BECCS (IPCC Working Group III, 2022a, Table 3.5). The large spread reflects the high uncertainty of BECCS deployment in the future among the IAM scenarios. The amount of captured carbon through BECCS in our study (34 GtC) is below average compared with the amount projected in the IPCC. Compared to other studies, our assumptions on CCS are rather conservative. While we assume a consecutive rise in CCS of up to 58 % following the CMIP6 scenario database for SSP1-2.6 (van Vuuren et al., 2017), Rose et al. (2014) assume between 50 % and 97 % in 2050 and between 86 % and 100 % in 2100 for different IAMs. However, only a tiny fraction of current CDR results from novel CDR methods, including BECCS (Smith et al., 2023), and future projections of CCS are very uncertain (IPCC Working Group III, 2022a, Table 3.5). Therefore, our assumptions on CCS deployment are rather conservative. Barriers to the upscaling of CCS facilities include the current lack of infrastructure for large-scale power generation from biomass with subsequent CCS, the currently high costs (Budinis et al., 2018), the need for governance and monitoring of CCS facilities, legal constraints, and public perception of geologic storage of CO2 (Vaughan and Gough, 2015; Smith et al., 2023). While the CDR potential is very sensitive to CCS and FFS, the assumptions for either of them are highly uncertain in the literature. Thus, the underlying values should be transparent, and a sensitivity analysis should be provided in future studies.
4.4 Side effects and caveats of tCDR
Several trade-offs and side effects occur in connection with tCDR and might limit their efficiency. Previous studies found that the land, water, and fertilizer, especially for first-generation bioenergy plants, required by BECCS could exacerbate water stress and pose a risk to food security (Creutzig, 2016; Smith et al., 2016; Boysen et al., 2017; Humpenöder et al., 2018; Roe et al., 2019; Cheng et al., 2022). These negative side effects can be alleviated by, e.g., using crop residues for bioenergy production. In addition, increased bioenergy crop production is likely to cause substantial deforestation due to the displacement of food production from other areas (Seo et al., 2024). Furthermore, the extensive cultivation of bioenergy plants, wood plantations, and forest monocultures may harm biodiversity (Veldman et al., 2015; Hanssen et al., 2022; Searchinger et al., 2022), and this has been subjected to societal debates over decades (Jönsson, 2024). Second-generation biofuels had smaller but still significant negative effects on species richness and abundance (Tudge et al., 2021). In our simulation setup, the agricultural production of the LUH2 land use satisfies food demand, and forest area increases in the baseline scenario. Crop yields, irrigation efficiency, and efficiency of livestock production are improved to increase food supply and protect biodiversity, thus limiting side effects (van Vuuren et al., 2017). Replacing first-generation bioenergy fuels, for example, by Miscanthus might save half of the land and one-third of the water use (Zhuang et al., 2013) and may increase soil organic carbon content (Longato et al., 2019; Melnikova et al., 2022). Further, CCS is still at an early technological development stage, it has not been employed on large scales (Reiner, 2016), and legal constraints on CCS still exist in many countries (Melnikova et al., 2022; Smith et al., 2023).
4.5 Limitations
While the main purpose of our study is to demonstrate various ways of how to measure the efficiency of CDR methods, we have also provided new estimates of the CDR potential for BECCS and AR. Unlike the different measures of efficiency per se, which are independent of the exact model implementation, our results of CDR potentials come with several caveats. We did not perform coupled simulations with a global circulation model. Thus, we can not evaluate the climate feedback of tCDR methods. Those include biogeochemical effects through altering the atmospheric CO2 due to land-use change and biogeophysical effects due to changes in surface properties such as albedo and roughness length (Winckler et al., 2019; Pongratz et al., 2021). These biogeophysical effects could counteract up to one-third of the climate effect of carbon emissions due to deforestation (Weber et al., 2024).
Theoretically, CCS can store carbon permanently. However, studies on carbon leakage of CCS are still inconclusive, mainly because they rely on laboratory experiments that are not comparable with the field observations (Gholami et al., 2021). Also, the durability of the carbon sequestration of AR is uncertain. Carbon is either stored in woody products or released back into the atmosphere after trees die or short-lived products decay. In addition, the risk of disturbances from fires, wind throw, droughts, and parasites increases with climate change and might limit the permanent storage of CO2 in trees but also biocrops (Seidl et al., 2017; Anderegg et al., 2020, 2022). These effects are not yet represented well in state-of-the-art land surface models used in CMIP6 projections (Fisher et al., 2018; Anderegg et al., 2022). Hence, the durability of forests is likely overestimated in our study. The current version of the model does not account for forest age. These were implemented in a more recent version of the model (Nabel et al., 2019), showing improved consistency to observation-based products for gross primary production, leaf area index, and aboveground biomass.
We do not assess the sensitivity of our results towards different types of crop management, such as irrigation or fertilization. Instead, the harvested nitrogen is applied as a fertilizer to the soil, which is the standard procedure for crops in JSBACH3.2. However, second-generation bioenergy crops require less fertilizer than first-generation bioenergy crops (Li et al., 2018a; Cheng et al., 2020), and their productivity increases only slightly when using nitrogen fertilization (LeBauer et al., 2018). Still, Li et al. (2021) find that annual harvesting of aboveground biomass of HBPs leads to a loss of nutrients and lower plant productivity if not compensated for by fertilization. We implicitly represent fertilization in the model by adding the harvested nitrogen to the soil. Although fertilizer application likely increases the carbon uptake of bioenergy plants, their use results in additional costs and greenhouse gas emissions, thereby lowering the efficiency (Li et al., 2021). IAMs include agricultural and forest residues and waste fraction for BECCS in addition to HBPs, which we did not consider in our study. Including these additional biomass sources for energy production would likely increase the potential of BECCS.
The study does not account for additional wood harvest in the AR scenario. Even when assuming AR is used for wood harvest in the same intensity as the other, typically older forest in the grid cell, additional global wood harvest from our AR scenario yields a relatively small amount of cumulative sequestered carbon of 1.3 GtC between 2015 and 2100, which does not substantially alter our results (Sect. 2.3). Further, the benefits of storing carbon in woody long-lived products or using wood for bioenergy might be outweighed by decreased carbon sequestration in the forest after harvest and before the re-establishment of the new trees and the increased CO2 emissions to the atmosphere if used for bioenergy (Obermeier et al., 2021; Soimakallio et al., 2021). Note that AR in JSBACH3.2 represents natural forest regrowth rather than fast-growing wood plantations. Implementing forest plantations in the Lund–Potsdam–Jena managed Land (LPJmL) model increases the total carbon uptake by up to 30 % globally by 2100 compared to natural regrowth (Braakhekke et al., 2019).
This study highlights the different measures of efficiency affecting the biogeochemical climate mitigation potential of bioenergy with carbon capture and storage (BECCS) and afforestation and reforestation (AR): the location and spatial extent of the plantations, the level of fossil fuel substitution (FFS) through bioenergy plants, the share of bioenergy that is captured and stored in long-lived products or geological reservoirs (CCS), and the temporal dynamics. While we focus on BECCS and AR in this study, the measures of efficiency are applicable to other, area-based tCDR methods, including soil carbon sequestration, agroforestry, and biochar. Depending on the research question or the climate mitigation target set, different measures for the efficiency of tCDR are meaningful: for reaching our near-term climate goals, the time horizon is key, while for biodiversity and spatial planning, the additional area measure is meaningful and the level of FFS and CCS is a question of technical feasibility.
In our study, the benefit of BECCS to remove carbon only becomes substantial after around 2070, when the areas converted to either AR or HBPs increase rapidly in the SSP1-2.6 land-use scenario. Thus, BECCS has a higher carbon removal potential over longer periods than AR, especially in the South American grasslands and southeast Africa, but will not contribute substantially to reaching short-term climate mitigation targets. However, the temporal dynamics of tCDR methods are scenario-specific. An idealized setup where all tCDR is applied simultaneously and everywhere would help when comparing the CDR efficiency across time and space more precisely. Further, the efficiency of BECCS as compared to “nature-based solutions” like AR will depend critically on the upscaling of CCS facilities; replacing fossil fuels with bioenergy in the future; and the planting of bioenergy crops in suitable locations that do not harm biodiversity, affect water retention, or risk food security. We show, for the first time, how these different measures can be considered simultaneously within a consistent setup as a base for a sensible balancing of land-use interests concerning climate mitigation, food production, and nature conservation.
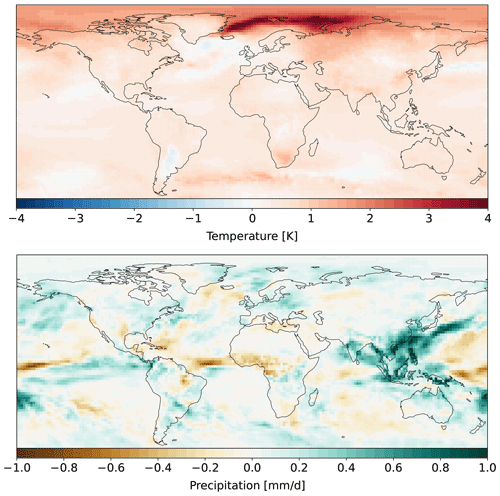
Figure A1Spatial changes between 2005–2024 and 2080–2099 mean temperature and precipitation of bias-corrected downscaled climate forcing of MPI-ESM1.2-HR for SSP1-2.6 mapped to T63 resolution.
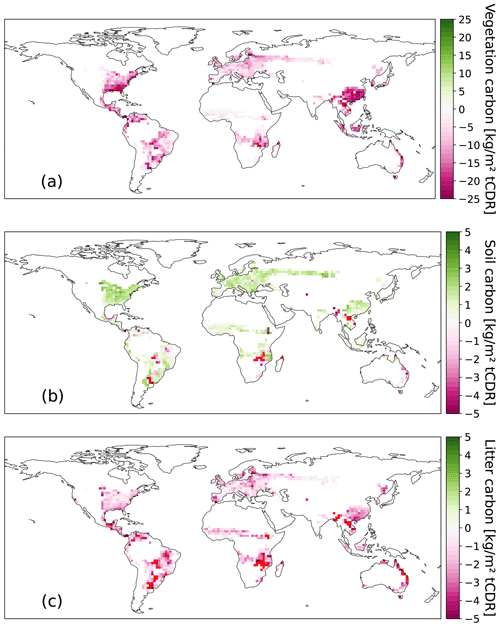
Figure A2Difference in vegetation (a), soil (b), and litter (c) carbon [kg m−2 tCDR] between HBPs and AR in 2100. Note that the scales differ. The red color indicates grid cells with values lower than the minimum value of the scale. Positive values indicate that HBPs store more carbon than AR. Note that this excludes carbon removal through CCS and FFS.
Preprocessing and postprocessing scripts are available at https://doi.org/10.5281/zenodo.13355458 (Egerer, 2024).
ISIMIP climate forcing data can be accessed under https://doi.org/10.48364/ISIMIP.842396.1 (Lange and Büchner, 2021). Miscanthus yield data can be found in Li et al. (2018c, https://doi.org/10.6084/m9.figshare.c.3951967).
JP initialized the project and provided the research idea. SE designed, performed, and analyzed the simulations and wrote the manuscript. DM implemented HBPs in JSBACH in a previous model version. SF, TN, and SE ported and adapted the code to JSBACH3.2 and connected the HBP implementation with the N cycle. TN revised the HBP parameter values. SE and TN evaluated the revised HBP version against observational data. WAO supported shaping the manuscript and engaged actively in discussions, especially on wood harvest. All co-authors proofread and provided input to the model design and the manuscript.
The contact author has declared that none of the authors has any competing interests.
Publisher's note: Copernicus Publications remains neutral with regard to jurisdictional claims made in the text, published maps, institutional affiliations, or any other geographical representation in this paper. While Copernicus Publications makes every effort to include appropriate place names, the final responsibility lies with the authors.
We thank Christian Reick, Mohammad Sadr, Andreas Krause, Wei Li, and Irina Melnikova for valuable discussions and reviews. This work used resources of the Deutsches Klimarechenzentrum (DKRZ) granted by its Scientific Steering Committee (WLA) under project ID bm1241. Sabine Egerer and Tobias Nützel were supported by the German Federal Ministry for Research and Education (BMBF) through the research project STEPSEC (grant number: 01LS2102A).
This research has been supported by the German Federal Ministry for Research and Education (grant no. 01LS2102A).
This paper was edited by Bertrand Guenet and reviewed by Wei Li and Irina Melnikova.
Anderegg, W. R., Trugman, A. T., Badgley, G., Anderson, C. M., Bartuska, A., Ciais, P., Cullenward, D., Field, C. B., Freeman, J., Goetz, S. J., Hicke, J. A., Huntzinger, D., Jackson, R. B., Nickerson, J., Pacala, S., and Randerson, J. T.: Climate-driven risks to the climate mitigation potential of forests, Science, 368, eaaz7005, https://doi.org/10.1126/science.aaz7005, 2020. a
Anderegg, W. R., Chegwidden, O. S., Badgley, G., Trugman, A. T., Cullenward, D., Abatzoglou, J. T., Hicke, J. A., Freeman, J., and Hamman, J. J.: Future climate risks from stress, insects and fire across US forests, Ecol. Lett., 25, 1510–1520, https://doi.org/10.1111/ele.14018, 2022. a, b
Awty-Carroll, D., Magenau, E., Al Hassan, M., Martani, E., Kontek, M., van der Pluijm, P., Ashman, C., de Maupeou, E., McCalmont, J., Petrie, G. J., Davey, C., van der Cruijsen, K., Jurišić, V., Amaducci, S., Lamy, I., Shepherd, A., Kam, J., Hoogendam, A., Croci, M., Dolstra, O., Ferrarini, A., Lewandowski, I., Trindade, L. M., Kiesel, A., and Clifton-Brown, J.: Yield performance of 14 novel inter- and intra-species Miscanthus hybrids across Europe, GCB Bioenergy, 15, 399–423, https://doi.org/10.1111/gcbb.13026, 2023. a, b
Azar, C., Johansson, D. J., and Mattsson, N.: Meeting global temperature targets – The role of bioenergy with carbon capture and storage, Environ. Res. Lett., 8, 034004, https://doi.org/10.1088/1748-9326/8/3/034004, 2013. a
Babin, A., Vaneeckhaute, C., and Iliuta, M. C.: Potential and challenges of bioenergy with carbon capture and storage as a carbon-negative energy source: A review, Biomass Bioenerg., 146, 105968, https://doi.org/10.1016/j.biombioe.2021.105968, 2021. a, b
Borchers, M., Förster, J., Thrän, D., Beck, S., Thoni, T., Korte, K., Gawel, E., Markus, T., Schaller, R., Rhoden, I., Chi, Y., Dahmen, N., Dittmeyer, R., Dolch, T., Dold, C., Herbst, M., Heß, D., Kalhori, A., Jakobsen, K. K., Li, Z., Oschlies, A., Reusch, T. B. H., Sachs, T., Hattenberger, C. S., Stevenson, A., Wu, J., Yeates, C., and Mengis, N.: A Comprehensive Assessment of Carbon Dioxide Removal Options for Germany Earth' s Future, Earth's Future, 12, 1–23, https://doi.org/10.1029/2023EF003986, 2024. a
Boysen, L. R., Lucht, W., Gerten, D., Heck, V., Lenton, T. M., and Schellnhuber, H. J.: The limits to global-warming mitigation by terrestrial carbon removal, Earth's Future, 5, 463–474, https://doi.org/10.1002/2016EF000469, 2017. a, b
Braakhekke, M. C., Doelman, J. C., Baas, P., Müller, C., Schaphoff, S., Stehfest, E., and van Vuuren, D. P.: Modeling forest plantations for carbon uptake with the LPJmL dynamic global vegetation model, Earth Syst. Dynam., 10, 617–630, https://doi.org/10.5194/esd-10-617-2019, 2019. a
Budinis, S., Krevor, S., Dowell, N. M., Brandon, N., and Hawkes, A.: An assessment of CCS costs, barriers and potential, Energy Strateg. Rev., 22, 61–81, https://doi.org/10.1016/j.esr.2018.08.003, 2018. a
Byers, E., Krey, V., Kriegler, E., Riahi, K., Schaeffer, R., Kikstra, J., Lamboll, R., Nicholls, Z., Sandstad, M., Smith, C., van der Wijst, K., Al-Khourdajie, A., Lecocq, F., Portugal-Pereira, J., Saheb, Y., Stromman, A., Winkler, H., Auer, C., Brutschin, E., Gidden, M., Hackstock, P., Harmsen, M., Huppmann, D., Kolp, P., Lepault, C., Lewis, J., Marangoni, G., Müller-Casseres, E., Skeie, R., Werning, M., Calvin, K., Forster, P., Guivarch, C., Hasegawa, T., Meinshausen, M., Peters, G., Rogelj, J., Samset, B., Steinberger, J., Tavoni, M., and van Vuuren, D.: AR6 Scenarios Database, Zenodo, https://doi.org/10.5281/zenodo.7197970, 2022. a, b
Canadell, J., Monteiro, P., Costa, M., da Cunha, L. C., Cox, P., Eliseev, A., Henson, S., Ishii, M., Jaccard, S., Koven, C., Lohila, A., Patra, P., Piao, S., Rogelj, J., Syampungani, S., Zaehle, S., and Zickfeld, K.: Global Carbon and Other Biogeochemical Cycles and Feedbacks, in: Climate Change 2021 – The Physical Science Basiss. Contribution of Working Group I to the Sixth Assessment Report of the Intergovernmental Panel on Climate Change, edited by: Masson-Delmotte, V., Zhai, P., Pirani, A., Connors, S. L., Péan, C., Berger, S., Caud, N., Chen, Y., Goldfarb, L., Gomis, M. I., Huang, M., Leitzell, K., Lonnoy, E., Matthews, J. B. R., Maycock, T. K., Waterfield, T., Yelekçi, O., Yu, R., and Zhou, B., 673–816, Cambridge University Press, Cambridge, United Kingdom and New York, NY, USA, ISBN 9781009157896, https://doi.org/10.1017/9781009157896.007, 2021. a
Cannell, M. G.: Carbon sequestration and biomass energy offset: Theoretical, potential and achievable capacities globally, in Europe and the UK, Biomass Bioenerg., 24, 97–116, https://doi.org/10.1016/S0961-9534(02)00103-4, 2003. a
Cheng, Y., Huang, M., Chen, M., Guan, K., Bernacchi, C., Peng, B., and Tan, Z.: Parameterizing Perennial Bioenergy Crops in Version 5 of the Community Land Model Based on Site-Level Observations in the Central Midwestern United States, J. Adv. Model. Earth Sy., 12, 1–24, https://doi.org/10.1029/2019MS001719, 2020. a, b
Cheng, Y., Huang, M., Lawrence, D. M., Calvin, K., Lombardozzi, D. L., Sinha, E., Pan, M., and He, X.: Future bioenergy expansion could alter carbon sequestration potential and exacerbate water stress in the United States, Sci. Adv., 8, 1–14, https://doi.org/10.1126/sciadv.abm8237, 2022. a, b, c, d
Cheng, Y., Lawrence, D. M., Pan, M., Zhang, B., Graham, N. T., Lawrence, P. J., Liu, Z., and He, X.: A bioenergy- focused versus a reforestation- focused mitigation pathway yields disparate carbon storage and climate responses, P. Natl. Acad. Sci. USA, 121, 1–11, https://doi.org/10.1073/pnas, 2024. a, b, c, d, e
Christian, D. G., Riche, A. B., and Yates, N. E.: Growth, yield and mineral content of Miscanthus x giganteus grown as a biofuel for 14 successive harvests, Industrial Crops and Products, 28, 320–327, https://doi.org/10.1016/j.indcrop.2008.02.009, 2008. a
Chum, H., Faaij, A., Moreira, J., Berndes, G., Dhamija, P., Dong, H., Gabrielle, B., Eng, a. G., Cerutti, O. M., Mcintyre, T., Minowa, T., Pingoud, K., Seyboth, K., Matschoss, P., Kadner, S., Zwickel, T., Eickemeier, P., Hansen, G., and Kingdom, U.: SRREN – Chapter 2 – Bioenergy, in: IPCC Special Report on Renewable Energy Sources and Climate Change Mitigation, edited by: Edenhofer, O., Pichs-Madruga, R., Sokona, Y., Seyboth, K., Matschoss, P., Kadner, S., Zwickel, T., Eickemeier, P., Hansen, G., Schlömer, S., and von Stechow, C., 209–332, Cambridge University Press, Cambridge, United Kingdom and New York, NY, USA, ISBN 9781107607101, 2011. a, b
Clifton-Brown, J., Renvoize, S., Chiang, Y.-C., Ibaragi, Y., Flavell, R., Greef, J., Huang, L., Hsu, T. W., Kim, D.-S., Hastings, A., Schwarz, K., Stampfl, P., Valentine, J., Yamada, T., Xi, Q., and Donnison, I.: Chapter 15: Developing Miscanthus for Bioenergy, in: Energy Crops, edited by: Halford, N. G. and Karp, A., RSC Publishing, https://doi.org/10.1039/9781849732048-00301, 2010. a
Clifton-Brown, J., Hastings, A., Mos, M., McCalmont, J. P., Ashman, C., Awty-Carroll, D., Cerazy, J., Chiang, Y. C., Cosentino, S., Cracroft-Eley, W., Scurlock, J., Donnison, I. S., Glover, C., Goła̧b, I., Greef, J. M., Gwyn, J., Harding, G., Hayes, C., Helios, W., Hsu, T. W., Huang, L. S., Jeżowski, S., Kim, D. S., Kiesel, A., Kotecki, A., Krzyzak, J., Lewandowski, I., Lim, S. H., Liu, J., Loosely, M., Meyer, H., Murphy-Bokern, D., Nelson, W., Pogrzeba, M., Robinson, G., Robson, P., Rogers, C., Scalici, G., Schuele, H., Shafiei, R., Shevchuk, O., Schwarz, K. U., Squance, M., Swaller, T., Thornton, J., Truckses, T., Botnari, V., Vizir, I., Wagner, M., Warren, R., Webster, R., Yamada, T., Youell, S., Xi, Q., Zong, J., and Flavell, R.: Progress in upscaling Miscanthus biomass production for the European bio-economy with seed-based hybrids, GCB Bioenergy, 9, 6–17, https://doi.org/10.1111/gcbb.12357, 2017. a, b
Creutzig, F.: Economic and ecological views on climate change mitigation with bioenergy and negative emissions, GCB Bioenergy, 8, 4–10, https://doi.org/10.1111/gcbb.12235, 2016. a, b
Doelman, J. C., Stehfest, E., van Vuuren, D. P., Tabeau, A., Hof, A. F., Braakhekke, M. C., Gernaat, D. E., van den Berg, M., van Zeist, W. J., Daioglou, V., van Meijl, H., and Lucas, P. L.: Afforestation for climate change mitigation: Potentials, risks and trade-offs, Glob. Change Biol., 26, 1576–1591, https://doi.org/10.1111/gcb.14887, 2020. a
Egerer, S.: Scripts to publication 'How to measure the efficiency of bioenergy crops compared to forestation', Zenodo [code], https://doi.org/10.5281/zenodo.13355458, 2024. a
Ercoli, L., Mariotti, M., Masoni, A., and Bonari, E.: Effect of irrigation and nitrogen fertilization on biomass yield and efficiency of energy use in crop production of Miscanthus, Field Crop Res., 63, 3–11, https://doi.org/10.1016/S0378-4290(99)00022-2, 1999. a
Eyring, V., Bony, S., Meehl, G. A., Senior, C. A., Stevens, B., Stouffer, R. J., and Taylor, K. E.: Overview of the Coupled Model Intercomparison Project Phase 6 (CMIP6) experimental design and organization, Geosci. Model Dev., 9, 1937–1958, https://doi.org/10.5194/gmd-9-1937-2016, 2016. a
Fisher, R. A., Koven, C. D., Anderegg, W. R., Christoffersen, B. O., Dietze, M. C., Farrior, C. E., Holm, J. A., Hurtt, G. C., Knox, R. G., Lawrence, P. J., Lichstein, J. W., Longo, M., Matheny, A. M., Medvigy, D., Muller-Landau, H. C., Powell, T. L., Serbin, S. P., Sato, H., Shuman, J. K., Smith, B., Trugman, A. T., Viskari, T., Verbeeck, H., Weng, E., Xu, C., Xu, X., Zhang, T., and Moorcroft, P. R.: Vegetation demographics in Earth System Models: A review of progress and priorities, Glob. Change Biol., 24, 35–54, https://doi.org/10.1111/gcb.13910, 2018. a
Friedlingstein, P., O'Sullivan, M., Jones, M. W., Andrew, R. M., Bakker, D. C. E., Hauck, J., Landschützer, P., Le Quéré, C., Luijkx, I. T., Peters, G. P., Peters, W., Pongratz, J., Schwingshackl, C., Sitch, S., Canadell, J. G., Ciais, P., Jackson, R. B., Alin, S. R., Anthoni, P., Barbero, L., Bates, N. R., Becker, M., Bellouin, N., Decharme, B., Bopp, L., Brasika, I. B. M., Cadule, P., Chamberlain, M. A., Chandra, N., Chau, T.-T.-T., Chevallier, F., Chini, L. P., Cronin, M., Dou, X., Enyo, K., Evans, W., Falk, S., Feely, R. A., Feng, L., Ford, D. J., Gasser, T., Ghattas, J., Gkritzalis, T., Grassi, G., Gregor, L., Gruber, N., Gürses, Ö., Harris, I., Hefner, M., Heinke, J., Houghton, R. A., Hurtt, G. C., Iida, Y., Ilyina, T., Jacobson, A. R., Jain, A., Jarníková, T., Jersild, A., Jiang, F., Jin, Z., Joos, F., Kato, E., Keeling, R. F., Kennedy, D., Klein Goldewijk, K., Knauer, J., Korsbakken, J. I., Körtzinger, A., Lan, X., Lefèvre, N., Li, H., Liu, J., Liu, Z., Ma, L., Marland, G., Mayot, N., McGuire, P. C., McKinley, G. A., Meyer, G., Morgan, E. J., Munro, D. R., Nakaoka, S.-I., Niwa, Y., O'Brien, K. M., Olsen, A., Omar, A. M., Ono, T., Paulsen, M., Pierrot, D., Pocock, K., Poulter, B., Powis, C. M., Rehder, G., Resplandy, L., Robertson, E., Rödenbeck, C., Rosan, T. M., Schwinger, J., Séférian, R., Smallman, T. L., Smith, S. M., Sospedra-Alfonso, R., Sun, Q., Sutton, A. J., Sweeney, C., Takao, S., Tans, P. P., Tian, H., Tilbrook, B., Tsujino, H., Tubiello, F., van der Werf, G. R., van Ooijen, E., Wanninkhof, R., Watanabe, M., Wimart-Rousseau, C., Yang, D., Yang, X., Yuan, W., Yue, X., Zaehle, S., Zeng, J., and Zheng, B.: Global Carbon Budget 2023, Earth Syst. Sci. Data, 15, 5301–5369, https://doi.org/10.5194/essd-15-5301-2023, 2023. a
Frühwirth, P., Graf, A., Humer, M., Hunger, F., Köppl, H., Liebhard, P., and Thumfart, K.: Miscanthus sinensis, 'Giganteus' – Chinaschilf als nachwachsender Rohstoff, Tech. rep., Landwirtschaftskammer Österreich, ISBN 9783902325020, 2006. a
Fuss, S., Canadell, J. G., Peters, G. P., Tavoni, M., Andrew, R. M., Ciais, P., Jackson, R. B., Jones, C. D., Kraxner, F., Nakicenovic, N., Le Quéré, C., Raupach, M. R., Sharifi, A., Smith, P., and Yamagata, Y.: Betting on negative emissions, Nat. Clim. Change, 4, 850–853, https://doi.org/10.1038/nclimate2392, 2014. a
Fuss, S., Lamb, W. F., Callaghan, M. W., Hilaire, J., Creutzig, F., Amann, T., Beringer, T., De Oliveira Garcia, W., Hartmann, J., Khanna, T., Luderer, G., Nemet, G. F., Rogelj, J., Smith, P., Vicente, J. V., Wilcox, J., Del Mar Zamora Dominguez, M., and Minx, J. C.: Negative emissions – Part 2: Costs, potentials and side effects, Environ. Res. Lett., 13, 063002, https://doi.org/10.1088/1748-9326/aabf9f, 2018. a
Gallagher, E.: The Gallagher review of the indirect effects of biofuels production, Tech. Rep. April, Renewable Fuels Agency, https://www.unido.org/sites/default/files/2009-11/Gallagher_Report_0.pdf (last access: 11 November 2024), 2008. a, b
Gholami, R., Raza, A., and Iglauer, S.: Leakage risk assessment of a CO2 storage site: A review, Earth-Sci. Rev., 223, 103849, https://doi.org/10.1016/j.earscirev.2021.103849, 2021. a
Goll, D. S., Brovkin, V., Liski, J., Raddatz, T., Thum, T., and Todd-Brown, K. E.: Strong dependence of CO2 emissions from anthropogenic land cover change on initial land cover and soil carbon parametrization, Global Biogeochem. Cy., 29, 1511–1523, https://doi.org/10.1002/2014GB004988, 2015. a
Griscom, B. W., Adams, J., Ellis, P. W., Houghton, R. A., Lomax, G., Miteva, D. A., Schlesinger, W. H., Shoch, D., Siikamäki, J. V., Smith, P., Woodbury, P., Zganjar, C., Blackman, A., Campari, J., Conant, R. T., Delgado, C., Gopalakrishna, T., Hamsik, M. R., Herrero, M., Kiesecker, J., Landis, E., Laestadius, L., Leavitt, S. M., Polasky, S., Potapov, P., Putz, F. E., Sanderman, J., Silvius, M., Wollenberg, E., and Fargione, J.: Natural climate solutions, P. Natl. Acad. Sci. USA, 114, 11645–11650, https://doi.org/10.1073/pnas.1710465114, 2017. a
Gutjahr, O., Putrasahan, D., Lohmann, K., Jungclaus, J. H., von Storch, J.-S., Brüggemann, N., Haak, H., and Stössel, A.: Max Planck Institute Earth System Model (MPI-ESM1.2) for the High-Resolution Model Intercomparison Project (HighResMIP), Geosci. Model Dev., 12, 3241–3281, https://doi.org/10.5194/gmd-12-3241-2019, 2019. a
Hanssen, S. V., Steinmann, Z. J., Daioglou, V., Čengić, M., Van Vuuren, D. P., and Huijbregts, M. A.: Global implications of crop-based bioenergy with carbon capture and storage for terrestrial vertebrate biodiversity, GCB Bioenergy, 14, 307–321, https://doi.org/10.1111/gcbb.12911, 2022. a
Harper, A. B., Powell, T., Cox, P. M., House, J., Huntingford, C., Lenton, T. M., Sitch, S., Burke, E., Chadburn, S. E., Collins, W. J., Comyn-Platt, E., Daioglou, V., Doelman, J. C., Hayman, G., Robertson, E., van Vuuren, D., Wiltshire, A., Webber, C. P., Bastos, A., Boysen, L., Ciais, P., Devaraju, N., Jain, A. K., Krause, A., Poulter, B., and Shu, S.: Land-use emissions play a critical role in land-based mitigation for Paris climate targets, Nat. Commun., 9, 2938, https://doi.org/10.1038/s41467-018-05340-z, 2018. a, b, c, d, e, f
Hempel, S., Frieler, K., Warszawski, L., Schewe, J., and Piontek, F.: A trend-preserving bias correction – the ISI-MIP approach, Earth Syst. Dynam., 4, 219–236, https://doi.org/10.5194/esd-4-219-2013, 2013. a
Humpenöder, F., Popp, A., Bodirsky, B. L., Weindl, I., Biewald, A., Lotze-Campen, H., Dietrich, J. P., Klein, D., Kreidenweis, U., Müller, C., Rolinski, S., and Stevanovic, M.: Large-scale bioenergy production: How to resolve sustainability trade-offs?, Environ. Res. Lett., 13, 024011, https://doi.org/10.1088/1748-9326/aa9e3b, 2018. a, b
Hurtt, G. C., Chini, L., Sahajpal, R., Frolking, S., Bodirsky, B. L., Calvin, K., Doelman, J. C., Fisk, J., Fujimori, S., Klein Goldewijk, K., Hasegawa, T., Havlik, P., Heinimann, A., Humpenöder, F., Jungclaus, J., Kaplan, J. O., Kennedy, J., Krisztin, T., Lawrence, D., Lawrence, P., Ma, L., Mertz, O., Pongratz, J., Popp, A., Poulter, B., Riahi, K., Shevliakova, E., Stehfest, E., Thornton, P., Tubiello, F. N., van Vuuren, D. P., and Zhang, X.: Harmonization of global land use change and management for the period 850–2100 (LUH2) for CMIP6, Geosci. Model Dev., 13, 5425–5464, https://doi.org/10.5194/gmd-13-5425-2020, 2020. a, b, c, d, e
IPCC Working Group III: Climate change 2022: Mitigation of Climate Change, Full Report, Tech. Rep. 1, Cambridge, UK and New York, NY, US, ISBN 9789291691609, https://doi.org/10.18356/9789210012973c007, 2022a. a, b
IPCC Working Group III: Climate Change 2022: Mitigation of Climate Change, Summary for Policymakers, Tech. Rep. 1, Cambridge, United Kingdom and New York, NY, USA, ISBN 9781107415416, 2022b. a, b, c, d
IPCC Working Group III: Climate Change 2022 – Mitigation of Climate Change, Technical Summary, Tech. Rep. 1, ISBN 9781107415416, 2022c. a, b, c
Jayakrishnan, K. U. and Bala, G.: A comparison of the climate and carbon cycle effects of carbon removal by afforestation and an equivalent reduction in fossil fuel emissions, Biogeosciences, 20, 1863–1877, https://doi.org/10.5194/bg-20-1863-2023, 2023. a
Jönsson, J.: Historical perspectives on forestry science and monocultures: Ideas of rationality in Sweden during the early twentieth century, Ambio, 53, 933–940, https://doi.org/10.1007/s13280-024-01987-9, 2024. a
Kalt, G., Mayer, A., Theurl, M. C., Lauk, C., Erb, K. H., and Haberl, H.: Natural climate solutions versus bioenergy: Can carbon benefits of natural succession compete with bioenergy from short rotation coppice?, GCB Bioenergy, 11, 1283–1297, https://doi.org/10.1111/gcbb.12626, 2019. a, b, c
Klein Goldewijk, K., Dekker, S. C., and van Zanden, J. L.: Per-capita estimations of long-term historical land use and the consequences for global change research, Journal of Land Use Science, 12, 313–337, https://doi.org/10.1080/1747423X.2017.1354938, 2017. a
Krause, A., Pugh, T. A. M., Bayer, A. D., Doelman, J. C., Humpenöder, F., Anthoni, P., Olin, S., Bodirsky, B. L., Popp, A., Stehfest, E., and Arneth, A.: Global consequences of afforestation and bioenergy cultivation on ecosystem service indicators, Biogeosciences, 14, 4829–4850, https://doi.org/10.5194/bg-14-4829-2017, 2017. a, b, c
Krause, A., Pugh, T. A., Bayer, A. D., Li, W., Leung, F., Bondeau, A., Doelman, J. C., Humpenöder, F., Anthoni, P., Bodirsky, B. L., Ciais, P., Müller, C., Murray-Tortarolo, G., Olin, S., Popp, A., Sitch, S., Stehfest, E., and Arneth, A.: Large uncertainty in carbon uptake potential of land-based climate-change mitigation efforts, Glob. Change Biol., 24, 3025–3038, https://doi.org/10.1111/gcb.14144, 2018. a, b, c, d
Lange, S. and Büchner, M.: ISIMIP3b bias-adjusted atmospheric climate input data, ISIMIP Repository [data set], https://doi.org/10.48364/ISIMIP.842396.1, 2021. a, b
Lasslop, G., Thonicke, K., and Kloster, S.: Journal of Advances in Modeling Earth Systems, J. Adv. Model. Earth Sy., 6, 740–755, https://doi.org/10.1002/2013MS000284, 2014. a
Lawrence, D. M., Hurtt, G. C., Arneth, A., Brovkin, V., Calvin, K. V., Jones, A. D., Jones, C. D., Lawrence, P. J., de Noblet-Ducoudré, N., Pongratz, J., Seneviratne, S. I., and Shevliakova, E.: The Land Use Model Intercomparison Project (LUMIP) contribution to CMIP6: rationale and experimental design, Geosci. Model Dev., 9, 2973–2998, https://doi.org/10.5194/gmd-9-2973-2016, 2016. a
LeBauer, D., Kooper, R., Mulrooney, P., Rohde, S., Wang, D., Long, S. P., and Dietze, M. C.: BETYdb: a yield, trait, and ecosystem service database applied to second-generation bioenergy feedstock production, GCB Bioenergy, 10, 61–71, https://doi.org/10.1111/gcbb.12420, 2018. a, b
Li, W., Ciais, P., Makowski, D., and Peng, S.: Data descriptor: A global yield dataset for major lignocellulosic bioenergy crops based on field measurements, Sci. Data, 5, 1–10, https://doi.org/10.1038/sdata.2018.169, 2018a. a, b, c, d, e, f, g, h, i, j
Li, W., Yue, C., Ciais, P., Chang, J., Goll, D., Zhu, D., Peng, S., and Jornet-Puig, A.: ORCHIDEE-MICT-BIOENERGY: an attempt to represent the production of lignocellulosic crops for bioenergy in a global vegetation model, Geosci. Model Dev., 11, 2249–2272, https://doi.org/10.5194/gmd-11-2249-2018, 2018b. a, b, c, d
Li, W., Ciais, P., Makowski, D., and Peng, S.: A global yield dataset for major lignocellulosic bioenergy crops based on field measurements, Figshare [data set], https://doi.org/10.6084/m9.figshare.c.3951967, 2018c. a, b
Li, W., Ciais, P., Han, M., Zhao, Q., Chang, J., Goll, D. S., Zhu, L., and Wang, J.: Bioenergy Crops for Low Warming Targets Require Half of the Present Agricultural Fertilizer Use, Environ. Sci. Technol., 55, 10654–10661, https://doi.org/10.1021/acs.est.1c02238, 2021. a, b
Littleton, E. W., Harper, A. B., Vaughan, N. E., Oliver, R. J., Duran-Rojas, M. C., and Lenton, T. M.: JULES-BE: representation of bioenergy crops and harvesting in the Joint UK Land Environment Simulator vn5.1, Geosci. Model Dev., 13, 1123–1136, https://doi.org/10.5194/gmd-13-1123-2020, 2020. a, b, c, d, e, f, g
Longato, D., Gaglio, M., Boschetti, M., and Gissi, E.: Bioenergy and ecosystem services trade-offs and synergies in marginal agricultural lands: A remote-sensing-based assessment method, J. Clean. Prod., 237, 117672, https://doi.org/10.1016/j.jclepro.2019.117672, 2019. a
Luyssaert, S., Schulze, E. D., Börner, A., Knohl, A., Hessenmöller, D., Law, B. E., Ciais, P., and Grace, J.: Old-growth forests as global carbon sinks, Nature, 455, 213–215, https://doi.org/10.1038/nature07276, 2008. a
Luyssaert, S., Schulze, E.-D., Knohl, A., Law, B. E., Grace, P. C., and Grace, J.: Reply to: Old-growth forest carbon sinks overestimated, Nature, 591, E24–E25, https://doi.org/10.1038/s41586-021-03267-y, 2021. a
Matthews, H. D., Zickfeld, K., Dickau, M., MacIsaac, A. J., Mathesius, S., Nzotungicimpaye, C. M., and Luers, A.: Temporary nature-based carbon removal can lower peak warming in a well-below 2 °C scenario, Commun. Environ., 3, 1–8, https://doi.org/10.1038/s43247-022-00391-z, 2022. a
Mauritsen, T., Bader, J., Becker, T., Behrens, J., Bittner, M., Brokopf, R., Brovkin, V., Claussen, M., Crueger, T., Esch, M., Fast, I., Fiedler, S., Fläschner, D., Gayler, V., Giorgetta, M., Goll, D. S., Haak, H., Hagemann, S., Hedemann, C., Hohenegger, C., Ilyina, T., Jahns, T., Jimenéz-de-la Cuesta, D., Jungclaus, J., Kleinen, T., Kloster, S., Kracher, D., Kinne, S., Kleberg, D., Lasslop, G., Kornblueh, L., Marotzke, J., Matei, D., Meraner, K., Mikolajewicz, U., Modali, K., Möbis, B., Müller, W. A., Nabel, J. E., Nam, C. C., Notz, D., Nyawira, S. S., Paulsen, H., Peters, K., Pincus, R., Pohlmann, H., Pongratz, J., Popp, M., Raddatz, T. J., Rast, S., Redler, R., Reick, C. H., Rohrschneider, T., Schemann, V., Schmidt, H., Schnur, R., Schulzweida, U., Six, K. D., Stein, L., Stemmler, I., Stevens, B., von Storch, J. S., Tian, F., Voigt, A., Vrese, P., Wieners, K. H., Wilkenskjeld, S., Winkler, A., and Roeckner, E.: Developments in the MPI-M Earth System Model version 1.2 (MPI-ESM1.2) and Its Response to Increasing CO2, J. Adv. Model. Earth Sy., 11, 998–1038, https://doi.org/10.1029/2018MS001400, 2019. a
May, M. M. and Rehfeld, K.: Negative Emissions as the New Frontier of Photoelectrochemical CO2 Reduction, Adv. Energy Mater., 12, 1–6, https://doi.org/10.1002/aenm.202103801, 2022. a
Mayer, D.: Potentials and side-effects of herbaceous biomass plantations for climate change mitigation, PhD thesis, Universität Hamburg, https://doi.org/10.17617/2.2479582, 2017. a, b, c, d, e, f, g, h, i, j
Meehl, G. A., Senior, C. A., Eyring, V., Flato, G., Lamarque, J. F., Stouffer, R. J., Taylor, K. E., and Schlund, M.: Context for interpreting equilibrium climate sensitivity and transient climate response from the CMIP6 Earth system models, Sci. Adv., 6, 1–10, https://doi.org/10.1126/sciadv.aba1981, 2020. a
Meinshausen, M., Nicholls, Z. R. J., Lewis, J., Gidden, M. J., Vogel, E., Freund, M., Beyerle, U., Gessner, C., Nauels, A., Bauer, N., Canadell, J. G., Daniel, J. S., John, A., Krummel, P. B., Luderer, G., Meinshausen, N., Montzka, S. A., Rayner, P. J., Reimann, S., Smith, S. J., van den Berg, M., Velders, G. J. M., Vollmer, M. K., and Wang, R. H. J.: The shared socio-economic pathway (SSP) greenhouse gas concentrations and their extensions to 2500, Geosci. Model Dev., 13, 3571–3605, https://doi.org/10.5194/gmd-13-3571-2020, 2020. a
Melnikova, I., Boucher, O., Cadule, P., Tanaka, K., Gasser, T., Hajima, T., Quilcaille, Y., Shiogama, H., Séférian, R., Tachiiri, K., Vuichard, N., Yokohata, T., and Ciais, P.: Impact of bioenergy crop expansion on climate–carbon cycle feedbacks in overshoot scenarios, Earth Syst. Dynam., 13, 779–794, https://doi.org/10.5194/esd-13-779-2022, 2022. a, b, c, d
Melnikova, I., Ciais, P., Vuichard, N., and Boucher, O.: Relative benefits of allocating land to bioenergy crops and forests vary by region, Commun. Earth Environ., 4, 1–12, https://doi.org/10.1038/s43247-023-00866-7, 2023. a, b, c, d, e
Meyer, M. H., Paul, J., and Anderson, N. O.: Competive ability of invasive Miscanthus biotypes with aggressive switchgrass, Biol. Invasions, 12, 3809–3816, https://doi.org/10.1007/s10530-010-9773-0, 2010. a
Muri, H.: The role of large – Scale BECCS in the pursuit of the 1.5 °C target: An Earth system model perspective, Environ. Res. Lett., 13, 044010, https://doi.org/10.1088/1748-9326/aab324, 2018. a
Nabel, J. E. M. S., Naudts, K., and Pongratz, J.: Accounting for forest age in the tile-based dynamic global vegetation model JSBACH4 (4.20p7; git feature/forests) – a land surface model for the ICON-ESM, Geosci. Model Dev., 13, 185–200, https://doi.org/10.5194/gmd-13-185-2020, 2020. a
Naidu, S. L. and Long, S. P.: Potential mechanisms of low-temperature tolerance of C4 photosynthesis in Miscanthus × giganteus: An in vivo analysis, Planta, 220, 145–155, https://doi.org/10.1007/s00425-004-1322-6, 2004. a
Nützel, T.: Calculation of parameter values based on observations for the herbaceous biomass plantation PFT representing Miscanthus in JSBACH3.2, Zenodo [data set], https://doi.org/10.5281/zenodo.11193881, 2024. a
Obermeier, W. A., Nabel, J. E. M. S., Loughran, T., Hartung, K., Bastos, A., Havermann, F., Anthoni, P., Arneth, A., Goll, D. S., Lienert, S., Lombardozzi, D., Luyssaert, S., McGuire, P. C., Melton, J. R., Poulter, B., Sitch, S., Sullivan, M. O., Tian, H., Walker, A. P., Wiltshire, A. J., Zaehle, S., and Pongratz, J.: Modelled land use and land cover change emissions – a spatio-temporal comparison of different approaches, Earth Syst. Dynam., 12, 635–670, https://doi.org/10.5194/esd-12-635-2021, 2021. a
Pongratz, J., Schwingshackl, C., Bultan, S., Obermeier, W., Havermann, F., and Guo, S.: Land Use Effects on Climate: Current State, Recent Progress, and Emerging Topics, Current Climate Change Reports, 7, 99–120, https://doi.org/10.1007/s40641-021-00178-y, 2021. a
Pugh, T. A., Lindeskog, M., Smith, B., Poulter, B., Arneth, A., Haverd, V., and Calle, L.: Role of forest regrowth in global carbon sink dynamics, P. Natl. Acad. Sci. USA, 116, 4382–4387, https://doi.org/10.1073/pnas.1810512116, 2019. a
Raddatz, T. J., Reick, C. H., Knorr, W., Kattge, J., Roeckner, E., Schnur, R., Schnitzler, K. G., Wetzel, P., and Jungclaus, J.: Will the tropical land biosphere dominate the climate-carbon cycle feedback during the twenty-first century?, Clim. Dynam., 29, 565–574, https://doi.org/10.1007/s00382-007-0247-8, 2007. a
Reick, C. H., Raddatz, T., Brovkin, V., and Gayler, V.: Representation of natural and anthropogenic land cover change in MPI-ESM, J. Adv. Model. Earth Sy., 5, 459–482, https://doi.org/10.1002/jame.20022, 2013. a
Reick, C. H., Gayler, V., Goll, D., Hagemann, S., Heidkamp, M., Nabel, J. E. M. S., Raddatz, T., Roeckner, E., Schnur, R., and Wilkenskjeld, S.: JSBACH 3 – The land component of the MPI Earth System Model: documentation of version 3.2, Berichte zur Erdsystemforschung, 240, https://doi.org/10.17617/2.3279802, 2021. a
Reiner, D. M.: Learning through a portfolio of carbon capture and storage demonstration projects, Nat. Energ., 1, 1–7, https://doi.org/10.1038/nenergy.2015.11, 2016. a
Roe, S., Streck, C., Obersteiner, M., Frank, S., Griscom, B., Drouet, L., Fricko, O., Gusti, M., Harris, N., Hasegawa, T., Hausfather, Z., Havlík, P., House, J., Nabuurs, G. J., Popp, A., Sánchez, M. J. S., Sanderman, J., Smith, P., Stehfest, E., and Lawrence, D.: Contribution of the land sector to a 1.5 °C world, Nat. Clim. Change, 9, 817–828, https://doi.org/10.1038/s41558-019-0591-9, 2019. a, b, c, d, e
Rose, S. K., Kriegler, E., Bibas, R., Calvin, K., Popp, A., van Vuuren, D. P., and Weyant, J.: Bioenergy in energy transformation and climate management, Climatic Change, 123, 477–493, https://doi.org/10.1007/s10584-013-0965-3, 2014. a
Schulzweida, U.: CDO User Guide (2.3.0), Zenodo, Tech. rep., https://doi.org/10.5281/zenodo.10020800, 2023. a
Searchinger, T., James, O., Dumas, P., Kastner, T., and Wirsenius, S.: EU climate plan sacrifices carbon storage and biodiversity for bioenergy, Nature, 612, 27–30, https://doi.org/10.1038/d41586-022-04133-1, 2022. a
Searchinger, T. D., Wirsenius, S., Beringer, T., and Dumas, P.: Assessing the efficiency of changes in land use for mitigating climate change, Nature, 564, 249–253, https://doi.org/10.1038/s41586-018-0757-z, 2018. a
Seidl, R., Thom, D., Kautz, M., Martin-Benito, D., Peltoniemi, M., Vacchiano, G., Wild, J., Ascoli, D., Petr, M., Honkaniemi, J., Lexer, M. J., Trotsiuk, V., Mairota, P., Svoboda, M., Fabrika, M., Nagel, T. A., and Reyer, C. P.: Forest disturbances under climate change, Nat. Clim. Change, 7, 395–402, https://doi.org/10.1038/nclimate3303, 2017. a
Seo, B., Brown, C., Lee, H., and Rounsevell, M.: Bioenergy in Europe is unlikely to make a timely contribution to climate change targets, Environ. Res. Lett., 19, 044004, https://doi.org/10.1088/1748-9326/ad2d11, 2024. a, b
Sharma, B., Kumar, J., Ganguly, A. R., and Hoffman, F. M.: Carbon cycle extremes accelerate weakening of the land carbon sink in the late 21st century, Biogeosciences, 20, 1829–1841, https://doi.org/10.5194/bg-20-1829-2023, 2023. a
Smith, P., Davis, S. J., Creutzig, F., Fuss, S., Minx, J., Gabrielle, B., Kato, E., Jackson, R. B., Cowie, A., Kriegler, E., Van Vuuren, D. P., Rogelj, J., Ciais, P., Milne, J., Canadell, J. G., McCollum, D., Peters, G., Andrew, R., Krey, V., Shrestha, G., Friedlingstein, P., Gasser, T., Grübler, A., Heidug, W. K., Jonas, M., Jones, C. D., Kraxner, F., Littleton, E., Lowe, J., Moreira, J. R., Nakicenovic, N., Obersteiner, M., Patwardhan, A., Rogner, M., Rubin, E., Sharifi, A., Torvanger, A., Yamagata, Y., Edmonds, J., and Yongsung, C.: Biophysical and economic limits to negative CO2 emissions, Nat. Clim. Change, 6, 42–50, https://doi.org/10.1038/nclimate2870, 2016. a, b
Smith, S. M., Geden, O., Minx, J. C., and Nemet, G. F.: The State of Carbon Dioxide Removal, 1st Edn., Tech. rep., OSF, ISBN 9781119130536, https://doi.org/10.17605/OSF.IO/W3B4Z, 2023. a, b, c, d, e
Smith, S. M., Geden, O., Gidden, M. J., Lamb, W. F., Nemet, G. F., Minx, J. C., Buck, H., Burke, J., Cox, E., Edwards, M. R., Fuss, S., Johnstone, I., Müller-Hansen, F., Pongratz, J., Probst, B. S., Roe, S., Schenuit, F., Schulte, I., and Vaughan, N. E. E.: The State of Carbon Dioxide Removal, 2nd Edn., Tech. rep., OSF, https://doi.org/10.17605/OSF.IO/F85QJ, 2024. a
Soimakallio, S., Kalliokoski, T., Lehtonen, A., and Salminen, O.: On the trade-offs and synergies between forest carbon sequestration and substitution, Mitig. Adapt. Strat. Gl., 26, 1–17, https://doi.org/10.1007/s11027-021-09942-9, 2021. a
Sonntag, S., Pongratz, J., Reick, C. H., and Schmidt, H.: Reforestation in a high-CO2 world – Higher mitigation potential than expected, lower adaptation potential than hoped for, Geophys. Res. Lett., 43, 6546–6553, https://doi.org/10.1002/2016GL068824, 2016. a, b, c
Stehfest, E., van Vuuren, D., Kram, T., and Bouwman, L.: Integrated Assessment of Global Environmental Change with IMAGE 3.0: Model description and policy applications, PBL Netherlands Environmental Assessment Agency, ISBN 978-94-91506-71-0, 2014. a
Terlouw, T., Bauer, C., Rosa, L., and Mazzotti, M.: Life cycle assessment of carbon dioxide removal technologies: A critical review, Energy and Environmental Science, 14, 1701–1721, https://doi.org/10.1039/d0ee03757e, 2021. a
Thonicke, K., Spessa, A., Prentice, I. C., Harrison, S. P., Dong, L., and Carmona-Moreno, C.: The influence of vegetation, fire spread and fire behaviour on biomass burning and trace gas emissions: results from a process-based model, Biogeosciences, 7, 1991–2011, https://doi.org/10.5194/bg-7-1991-2010, 2010. a
Tudge, S. J., Purvis, A., and De Palma, A.: The impacts of biofuel crops on local biodiversity: a global synthesis, Biodivers. Conserv., 30, 2863–2883, https://doi.org/10.1007/s10531-021-02232-5, 2021. a
van Vuuren, D. P., Stehfest, E., Gernaat, D. E., Doelman, J. C., van den Berg, M., Harmsen, M., de Boer, H. S., Bouwman, L. F., Daioglou, V., Edelenbosch, O. Y., Girod, B., Kram, T., Lassaletta, L., Lucas, P. L., van Meijl, H., Müller, C., van Ruijven, B. J., van der Sluis, S., and Tabeau, A.: Energy, land-use and greenhouse gas emissions trajectories under a green growth paradigm, Glob. Environ. Change, 42, 237–250, https://doi.org/10.1016/j.gloenvcha.2016.05.008, 2017. a, b, c, d, e, f, g, h
Vaughan, N. E. and Gough, C.: Synthesising existing knowledge on feasibility of BECCS: Workshop Report, Tech. Rep. 1104872, AVOID 2, 2015. a
Veldman, J. W., Overbeck, G. E., Negreiros, D., Mahy, G., Le Stradic, S., Fernandes, G. W., Durigan, G., Buisson, E., Putz, F. E., and Bond, W. J.: Where Tree Planting and Forest Expansion are Bad for Biodiversity and Ecosystem Services, BioScience, 65, 1011–1018, https://doi.org/10.1093/biosci/biv118, 2015. a
Wang, J., Ciais, P., Gasser, T., Chang, J., Tian, H., Zhao, Z., Zhu, L., Li, Z., and Li, W.: Temperature Changes Induced by Biogeochemical and Biophysical Effects of Bioenergy Crop Cultivation, Environ. Sci. Technol., 57, 2474–2483, https://doi.org/10.1021/acs.est.2c05253, 2023. a, b
Weber, J., King, J. A., Abraham, N. L., Grosvenor, D. P., Smith, C. J., Shin, Y. M., Lawrence, P., Roe, S., Beerling, D. J., and Martin, M. V.: Chemistry-albedo feedbacks offset up to a third of forestation's CO2 removal benefits, Science, 383, 860–864, https://doi.org/10.1126/science.adg6196, 2024. a
Weedon, G. P., Balsamo, G., Bellouin, N., Gomes, S., Best, M. J., and Viterbo, P.: Data methodology applied to ERA-Interim reanalysis data, Water Resour. Res., 50, 7505–7514, https://doi.org/10.1002/2014WR015638, 2014. a
Wilkenskjeld, S., Kloster, S., Pongratz, J., Raddatz, T., and Reick, C. H.: Comparing the influence of net and gross anthropogenic land-use and land-cover changes on the carbon cycle in the MPI-ESM, Biogeosciences, 11, 4817–4828, https://doi.org/10.5194/bg-11-4817-2014, 2014. a
Winckler, J., Lejeune, Q., Reick, C. H., and Pongratz, J.: Nonlocal Effects Dominate the Global Mean Surface Temperature Response to the Biogeophysical Effects of Deforestation, Geophys. Res. Lett., 46, 745–755, https://doi.org/10.1029/2018GL080211, 2019. a
Zhao, X., Mignone, B. K., Wise, M. A., and McJeon, H. C.: Trade-offs in land-based carbon removal measures under 1.5 °C and 2 °C futures, Nat. Commun., 15, 2297, https://doi.org/10.1038/s41467-024-46575-3, 2024. a
Zhuang, Q., Qin, Z., and Chen, M.: Biofuel, land and water: Maize, switchgrass or Miscanthus?, Environ. Res. Lett., 8, 015020, https://doi.org/10.1088/1748-9326/8/1/015020, 2013. a