the Creative Commons Attribution 4.0 License.
the Creative Commons Attribution 4.0 License.
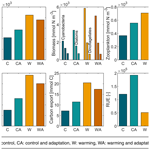
Phytoplankton adaptation to steady or changing environments affects marine ecosystem functioning
Isabell Hochfeld
Jana Hinners
Global warming poses a major threat to marine ecosystems, which fulfill important functions for humans and the climate. Ecosystem models are therefore increasingly used to estimate future changes in the functioning of marine ecosystems. However, projections differ notably between models. We propose that a major uncertainty factor in current models is that they ignore the high adaptive potential of phytoplankton, key players in marine ecosystems. Here, we use a zero-dimensional evolutionary ecosystem model to study how phytoplankton adaptation can affect estimates of future ecosystem-level changes. We found that phytoplankton adaptation can notably change simulated ecosystem dynamics, with the effect depending on environmental conditions. In a steady environment, adaptation allows for a more efficient use of resources, which enhances primary production and related ecosystem functions. In a warming environment, on the contrary, adaptation mitigates dominance changes among functionally different taxa and consequently leads to weaker changes in related ecosystem functions. Our results demonstrate that by neglecting phytoplankton adaptation, models may systematically overestimate future changes in the functioning of marine ecosystems. Future work can build on our results and include evolutionary processes into more complex model environments.
- Article
(4281 KB) - Full-text XML
- BibTeX
- EndNote
Global warming leads to a rapid reorganization of marine ecosystems, which poses a major threat to their functioning (Pecl et al., 2017). Since changes in the functioning of marine ecosystems directly impact humans and even feed back on the climate, understanding them is crucial (Pecl et al., 2017; Prentice et al., 2015). Ecosystem models have proven to be a valuable tool in this regard, but projections differ notably between models (Laufkötter et al., 2015, 2016). Current models largely ignore the high adaptive potential of phytoplankton (Laufkötter et al., 2015, 2016; Munkes et al., 2021), which are key players in marine ecosystems (Litchman et al., 2015). Here, we fill this gap by using an evolutionary ecosystem model to study the effect of phytoplankton adaptation to global warming on projected changes in ecosystem functioning. We apply the model to the Baltic Sea, which is impacted by above-average levels of multiple stressors (Reusch et al., 2018).
Marine ecosystems are vital to human societies. Primary production by phytoplankton accounts for about half of global photosynthesis (Field et al., 1998) and drives the biological carbon pump, which involves the fixation and export of atmospheric carbon to the deep ocean (Basu and Mackey, 2018). Cyanobacteria, on the contrary, pose a threat to marine ecosystems due to their toxicity and ability to fix atmospheric nitrogen, which can shift the nutrient balance toward eutrophication (Backer and McGillicuddy, 2006; Gustafsson et al., 2012, 2017; Schindler et al., 2008). Finally, marine food webs, from phytoplankton at the base to zooplankton as secondary producers to fish as top predators, represent commercially relevant resources of great importance to coastal regions around the globe (Atkinson et al., 2004; Everson, 2000; Lomartire et al., 2021; Weatherdon et al., 2016).
By forming the basis of the marine food web and driving biogeochemical cycles, phytoplankton play a key role in the functioning of marine ecosystems. Climate-change-related alterations in phytoplankton dynamics can therefore have far-reaching repercussions for marine ecosystem functioning. Phytoplankton respond to global warming by changing their phenology, which has led to an earlier and prolonged blooming season in the Baltic Sea, for example (Wasmund et al., 2019). The resulting mismatches with higher trophic levels like zooplankton and fish alter food web structures and may eventually lead to ecosystem-level changes (Asch et al., 2019; Edwards and Richardson, 2004; Winder and Schindler, 2004a). In addition, warming and eutrophication promote harmful algal blooms, which pose a threat to animal and human health (Glibert et al., 2014; Gobler et al., 2017; Paerl et al., 2015). Since ecosystem-level changes like these are expected to have a direct impact on human well-being and the climate (Pecl et al., 2017; Prentice et al., 2015), predicting them is of great importance.
Ecosystem models offer the possibility to assess future ecosystem-level changes. For example, ecosystem models can be integrated into global ocean circulation models to simulate future changes in net primary production on a global scale, but models do not even agree on the direction of change (Laufkötter et al., 2015). Similarly, regional models for the Baltic Sea cannot agree on the future development of cyanobacteria blooms regarding timing, concentration, and nitrogen fixation (Hense et al., 2013; Meier et al., 2011; Neumann, 2010). These uncertainties can notably affect estimates of future ocean deoxygenation (Long et al., 2021), nutrient load (Reusch et al., 2018; Wasmund et al., 2001), and harmful algal bloom dynamics (Hallegraeff, 2010; Paerl et al., 2015). Since model projections form the base of political decision-making (IPCC, 2022; Meier et al., 2014), there is an urgent need to improve their informative value. A first step could be to identify the key processes that affect ecosystem functioning. One key process that is lacking in most ecosystem models is the evolutionary adaptation of phytoplankton.
Their large population sizes and short generation times allow phytoplankton to quickly adapt to environmental changes. Evolution experiments, observations, and resurrection experiments showed that phytoplankton adaptation can be relevant on perennial or even shorter timescales (Hattich et al., 2024; Irwin et al., 2015; Jin and Agustí, 2018). Due to the crucial role of phytoplankton in marine ecosystems, considering phytoplankton adaptation in models may notably alter projected changes in ecosystem functioning (Ward et al., 2019). Some ecosystem models have already considered evolutionary processes in phytoplankton, such as natural selection from a diverse standing stock (Banas, 2011; Bruggeman and Kooijman, 2007; Dutkiewicz et al., 2020; Follows et al., 2007; Merico et al., 2009; Ward et al., 2012), the combination of selection and immigration (Acevedo-Trejos et al., 2018; Terseleer et al., 2014), the instantaneous acclimation of cellular resource allocation and metabolism (Kerimoglu et al., 2017; Smith et al., 2016a), or evolutionary adaptation in the form of mutation and selection. Models including the latter, from here on called evolutionary ecosystem models, have been used as a strategy to reduce model complexity (Pahlow et al., 2008), to identify the drivers of phytoplankton diversity (Wirtz, 2013), to analyze evolutionary mechanisms under idealized (laboratory) conditions (Beckmann et al., 2019; Clark et al., 2011; Collins, 2016), or to study the spatial distribution and/or temporal evolution of different functional traits in more realistic environments (Grimaud et al., 2015; Hinners et al., 2019; Le Gland et al., 2021; Sauterey et al., 2017). However, only a few evolutionary ecosystem models have already addressed questions related to ecosystem functioning. For example, Smith et al. (2016b) and Chen et al. (2019) studied the relationships between phytoplankton size diversity and productivity. Toseland et al. (2013), Daines et al. (2014), and Sauterey and Ward (2022) investigated drivers of and future changes in phytoplankton stoichiometry, which affects biogeochemical cycling. Finally, Cherabier and Ferrière (2022) analyzed the effect of bacterial adaptation to global warming on the microbial loop and the resulting impact on primary production.
So far, however, no model has explicitly addressed the question of how phytoplankton adaptation to global warming could affect the functioning of a marine ecosystem. A first step can be to estimate the effect of adaptation on warming-related changes in phytoplankton community composition. Different phytoplankton functional groups fulfill different functions in the ecosystem, for example, by contributing differently to the biological carbon pump (sinking speed), the nitrogen cycle (nitrogen fixation), and the energy transfer to higher trophic levels (food quality, susceptibility to predation) (Litchman et al., 2015). To our knowledge, there is only one model to date that considers competition between multiple phytoplankton functional groups and their adaptation to global warming simultaneously (Hochfeld and Hinners, 2024). Using this model, Hochfeld and Hinners (2024) demonstrated that adaptation can significantly reduce simulated phytoplankton responses to global warming in terms of changes in bloom timing and relative taxa abundance. However, how adaptation-related changes in phytoplankton responses may affect ecosystem functioning has not been studied yet.
Here, we use the Hochfeld and Hinners (2024) model to estimate for the first time how phytoplankton adaptation may affect warming-related changes in different ecosystem functions, including primary production, secondary production, carbon export, nitrogen fixation, and resource use efficiency (RUE). We apply the model to the Baltic Sea, which is already impacted by above-average levels of warming, nutrient load, and deoxygenation (Reusch et al., 2018). Due to the zero-dimensional setup of the model, we do not evaluate absolute changes in the above-mentioned ecosystem functions. Instead, we focus on how phytoplankton adaptation may change the future contribution of primary production to these ecosystem functions. Our study is a first step toward improving model projections of ecosystem-level changes that future work can build upon.
2.1 Model description
To study how phytoplankton adaptation to global warming may affect predicted changes in ecosystem functioning, we use the model from Hochfeld and Hinners (2024). A detailed description of the model is available in Hochfeld and Hinners (2024) and the associated supplementary material. In summary, the model simulates the dynamics of phytoplankton, zooplankton, dissolved inorganic nitrogen, and dead organic matter (detritus) in a zero-dimensional framework (Fig. B1). Three different phytoplankton functional groups common to the Baltic Sea are resolved: dinoflagellates, diatoms, and diazotrophic cyanobacteria. Each functional group is represented by a common taxon or by a complex of common taxa. For dinoflagellates and diatoms, the model simulates two cold-water species of the genera Apocalathium and Thalassiosira, respectively. For cyanobacteria, the model considers a complex that represents the dominant nitrogen-fixing genera in the Baltic Sea, Nodularia, Aphanizomenon, and Anabaena (Karlsson et al., 2005; Stal et al., 2003). Like in other modeling studies (Hense and Beckmann, 2006; Hinners et al., 2015; Lee et al., 2018), cyanobacteria are assumed to be non-grazeable due to toxicity, while dinoflagellates and diatoms are equally grazed by zooplankton. To ensure an accurate representation of phytoplankton phenology under ongoing global warming, the model additionally resolves phytoplankton life cycle dynamics, including growing and resting stages.
The key feature of the model is the flexibility in two temperature-dependent functional traits. The first flexible trait, the optimum temperature for growth, adapts through random mutations. Mutations occur during reproduction and lead to a randomized change in the optimum temperature, with small changes being much more likely than large changes. Cell size, on the contrary, does not respond randomly to temperature but plastically, with the cell size decreasing linearly with increasing temperature (Atkinson et al., 2003). For further details on the implementation of mutations and plasticity, see Hochfeld and Hinners (2024). The model additionally considers that changes in cell size affect metabolic cell properties (Litchman et al., 2007; Marañón et al., 2013; Ward et al., 2017), which in turn determine the nitrogen-limited growth rate (Grover, 1991). Since trait changes such as those described above affect individual cells, the model uses an agent-based approach after Beckmann et al. (2019) to simulate the dynamics of agents (super-individuals) with their individual phenotypic trait values. Zooplankton, dissolved inorganic nitrogen, and detritus, on the contrary, are represented by compartments, i.e., collections of cells or molecules described by their averaged properties and their concentration.
2.2 Model modifications
We slightly extended the Hochfeld and Hinners (2024) model described above by adding the explicit calculation of different ecosystem functions, including carbon export, cyanobacterial nitrogen fixation, and resource use efficiency (RUE).
We calculate carbon export from the carbon content of buried phytoplankton resting cells and the carbon that is exported through sinking of detritus. Detritus contains the dead phytoplankton and zooplankton cells, as well as the remains from unassimilated feeding. Following Ward et al. (2012), we divide detritus into dissolved organic matter (DOM) and particulate organic matter (POM) in a 50:50 ratio, with only POM being exported to deeper water layers. The distinction between POM and DOM represents a modification of the previous publication (Hochfeld and Hinners, 2024), in which we assumed that the entire detritus pool sinks. Since the sinking of detritus depends quadratically on the detritus concentration, we increased the original sinking rate by a factor of 4, from 0.097 to 0.388 d−1, to keep the mass loss per time step similar to the original version of the model. The sinking rate represents the only parameter value that was changed in comparison to the version published in Hochfeld and Hinners (2024).
To determine the amount of fixed atmospheric nitrogen, we assume that all the fixed nitrogen is converted into biomass. Thus, we define nitrogen fixation as the biomass built up by the diazotrophic cyanobacteria life cycle stage during each time step.
Finally, following Ptacnik et al. (2008), we calculate resource use efficiency (RUE) as the ratio of phytoplankton biomass to dissolved inorganic nitrogen. Since the cyanobacteria in our model can fix atmospheric nitrogen, we use simulations without cyanobacteria to derive RUE. Hence, we only consider the RUE of dinoflagellates and diatoms. Both dinoflagellates and diatoms are grazed by zooplankton; to avoid grazing-related biases in RUE, we additionally exclude zooplankton from RUE simulations.
2.3 Model scenarios
To understand how the adaptation of phytoplankton to different environments affects model estimates of associated ecosystem functions, we evaluate four different model scenarios, which are adopted from Hochfeld and Hinners (2024) (Table 1). Since our simulations are affected by random processes, e.g., during mortality and mutation, we perform seven simulations for each scenario and average the output to ensure robust results. Each simulation is run over 100 years.
Table 1Overview of the four model scenarios that we evaluate in this paper. All scenarios are adopted from Hochfeld and Hinners (2024). For each scenario, we run seven different simulations over 100 years and average the output to ensure robust results. Control represents a present-day seasonal temperature forcing for the Gulf of Finland. Warming adds a constant increase in temperature of 0.3 °C per decade to the control forcing (IPCC scenario SSP3-7.0, IPCC, 2021).
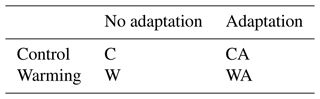
The first two model scenarios C (control) and CA (control and adaptation) represent control scenarios, which are forced with a steady seasonal temperature and irradiance forcing for present-day conditions in the Gulf of Finland. The two control scenarios C and CA serve as spin-up for two global warming scenarios W (warming) and WA (warming and adaptation). Global warming is simulated by adding a steady temperature increase of 0.3 °C per decade to the seasonal temperature forcing, which corresponds to the IPCC scenario SSP3-7.0 (IPCC, 2021). While adaptation in the optimum temperature is disabled in C and W, it is enabled in CA and WA. In this way, we can study how the (in)ability of phytoplankton to adapt to their environment may affect ecosystem functioning.
3.1 Model validation
The simulated seasonal phytoplankton dynamics are described in detail in Hochfeld and Hinners (2024). In summary, the two control scenarios C and CA produce a spring bloom of dinoflagellates and diatoms, a summer bloom of cyanobacteria, and a second but weaker bloom of diatoms in autumn (Fig. B2). The simulated bloom succession agrees reasonably well with recent monitoring data from the Baltic Sea, despite slight differences in spring bloom timing (Hjerne et al., 2019). As discussed in Hochfeld and Hinners (2024), the simulated bloom succession is realistic for the focal phytoplankton taxa.
In contrast to phytoplankton, Hochfeld and Hinners (2024) did not validate the seasonal dynamics of zooplankton against monitoring data. Here, we find that zooplankton biomass peaks during phytoplankton spring bloom following the peak in phytoplankton biomass (Fig. B3); remember that the model simulates cyanobacteria as the only summer-blooming phytoplankton taxon, which is assumed to be non-grazeable due to toxicity. Despite these simplifications in the model, the simulated seasonal pattern is indeed reasonable for some of the common zooplankton taxa in the Baltic Sea (Dutz et al., 2010; Feike et al., 2007).
3.2 Annual balances
The annual balances of our simulated ecosystem functions are shown in Fig. 1 for the last simulation year of all four model scenarios. Figure 1 reveals that phytoplankton produce ∼ 10 times more biomass than zooplankton per year and hence dominate biomass production in our simulations. Primary production, in turn, is dominated by cyanobacteria, while dinoflagellates account for the smallest amount of primary production per year.
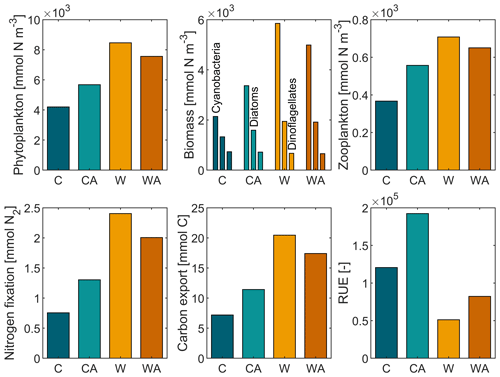
Figure 1Annual balances of our simulated ecosystem functions for the last simulation year of the four model scenarios (C – control, CA – control and adaptation, W – warming, WA – warming and adaptation). For each scenario, annual balances were averaged from seven different simulations. The differences between the four scenarios are statistically significant at the 0.05 level, except for dinoflagellates in W and WA (Table A1).
Annual primary production, i.e., total phytoplankton biomass, increases under global warming, with the increase being more than halved if phytoplankton thermal adaptation is enabled. Under control conditions, on the contrary, total phytoplankton biomass is ∼ 35 % higher with thermal adaptation. The observed development of annual primary production is predominantly driven by cyanobacteria and, to a lesser extent, diatoms, while dinoflagellates show a contrasting development (Fig. B4). This finding is underlined by strong positive correlations between total phytoplankton biomass, cyanobacteria, and diatoms, while dinoflagellates correlate negatively with all three (Fig. 2). In all four model scenarios, total phytoplankton biomass is most strongly correlated with cyanobacteria (1) and least strongly correlated with dinoflagellates ().
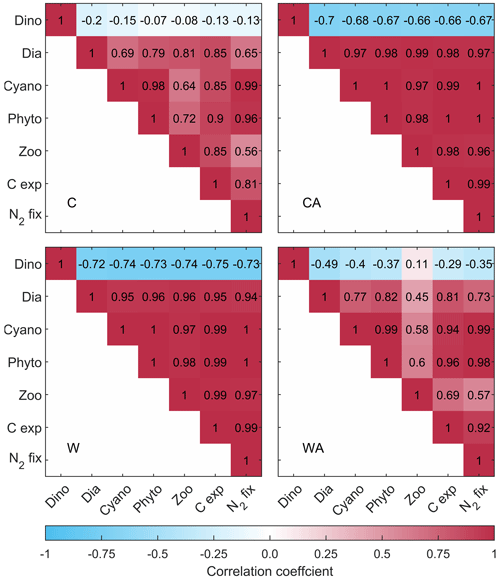
Figure 2Correlation matrices showing the correlation coefficients between the simulated ecosystem functions for the four different model scenarios (C – control, CA – control and adaptation, W – warming, WA – warming and adaptation). For C and CA, we calculated correlation coefficients using the annual balances from the last 95 years of seven different simulations. For W and WA, however, we only used the last 40 years to capture warming-related changes. All correlations shown here, except for those with dinoflagellates, are significant at the 0.05 level according to a t test (see Fig. B5). Note that resource use efficiency (RUE) is not included since we derived RUE from simulations without cyanobacteria and zooplankton.
Annual secondary production, i.e., zooplankton biomass, correlates positively with annual primary production. Under control conditions, the correlation is strongest with diatoms, whereas under global warming, zooplankton biomass is most strongly correlated with total phytoplankton biomass. In addition, zooplankton biomass production is notably affected by phytoplankton adaptation. Under control conditions, zooplankton produce ∼ 52 % more biomass if phytoplankton can adapt. Under global warming, zooplankton biomass increases, with the increase being ∼ 73 % weaker when phytoplankton adaptation is enabled.
The annual amount of fixed atmospheric nitrogen mirrors the annual biomass of cyanobacteria, which is confirmed by a strong positive correlation in all four model scenarios with r≥0.99. Under control conditions, cyanobacteria fix ∼ 72 % more nitrogen when adaptation is enabled. Global warming leads to an increase in nitrogen fixation by ∼ 218 % in W and ∼ 54 % in WA.
Carbon export correlates positively with both phytoplankton and zooplankton biomass, with the correlation being stronger with phytoplankton, which dominate biomass production (r≥0.90 vs. r≥0.69). Among phytoplankton, carbon export is most strongly correlated with cyanobacteria, which dominate primary production. In addition, carbon export is notably affected by phytoplankton adaptation. Under present-day conditions, carbon export is ∼ 59 % higher in CA than in C. Global warming leads to an increase in carbon export by ∼ 184 % in W and ∼ 52 % in WA.
Finally, resource use efficiency (RUE) decreases under global warming in our simulations, with the decrease being similar with and without phytoplankton adaptation (∼ 57 % and ∼ 58 %, respectively). Independent of the climate scenario, RUE is always higher if phytoplankton can adapt. Phytoplankton adaptation leads to an increase in RUE by ∼ 59 % and ∼ 61 % under control and warming conditions, respectively.
In conclusion, all ecosystem functions that we investigate in this study, except for dinoflagellates and RUE, show similar developments in the four model scenarios. This is underlined by strong positive correlations, which are significant at the 0.05 level (Fig. B5). While RUE is excluded from correlations as it was derived from simulations without cyanobacteria and zooplankton, dinoflagellates correlate (mostly) negatively with all other ecosystem functions. Independent of their direction, all correlations notably change their strength between the four model scenarios. Under control conditions, correlations are stronger if phytoplankton adaptation is enabled. This pattern reverses under global warming, where correlations are weaker with adaptation. This weakening is particularly strong for zooplankton, for which the negative correlation with dinoflagellates turns slightly positive in WA.
In this study, we used an evolutionary ecosystem model to analyze how ecosystem functioning may change in response to global warming and how these changes may be affected by phytoplankton adaptation. Our results show that warming-induced changes in primary production and associated ecosystem functions are generally less pronounced if phytoplankton adaptation is enabled. In addition, we found that most ecosystem functions are significantly positively correlated and that the strength of these correlations differs between model scenarios.
4.1 Primary production
The model projects an increase in annual primary production in response to global warming. This increase is predominantly driven by cyanobacteria, which are pre-adapted to high temperatures (Collins and Boylen, 1982; Lehtimäki et al., 1997; Nalewajko and Murphy, 2001). This finding agrees with observations showing a strong increase in Baltic Sea cyanobacteria over the past few decades (Suikkanen et al., 2007), as well as with a modeling study projecting future increases in cyanobacteria biomass and primary production for several areas of the Baltic Sea (Meier et al., 2011).
Our simulations further suggest that phytoplankton adaptation can lead to a weakened increase in cyanobacteria biomass, and hence primary production, under global warming. In Hochfeld and Hinners (2024), we discuss extensively how adaptation can influence the competition between different phytoplankton groups. In summary, under steady temperature conditions, adaptation results in increased primary production across phytoplankton taxa due to niche separation. Under global warming, the adaptation of spring-blooming diatoms to higher temperatures leads to a stronger competition with the summer-blooming cyanobacteria, weakening the bloom of the latter. However, even when phytoplankton adaptation is taken into account, our simulations still suggest an increase in cyanobacteria biomass under global warming. A further increase in cyanobacteria in the future can have severe consequences for the ecosystem, for example, due to their toxicity for higher trophic levels (Chorus and Welker, 2021; Quesada et al., 2006; Repavich et al., 1990) and their ability to fix atmospheric nitrogen.
4.2 Nitrogen fixation
Our model results suggest a strong warming-related increase in nitrogen fixation in the future, which is a direct result of the projected increase in cyanobacterial summer biomass. Today, the Baltic Sea is already impacted by above-average levels of nutrient load (Reusch et al., 2018). For example, nitrogen-driven eutrophication turned the Baltic Sea into one of the most hypoxic ocean areas worldwide, with severe consequences for productivity, biodiversity, and biogeochemical cycling (Breitburg et al., 2018). In the future, global warming is expected to further increase the vulnerability of coastal systems to nutrient loading as harmful algal bloom events become more likely and pose an increasing threat to animal and human health (Glibert et al., 2014; Gobler et al., 2017; Paerl et al., 2015).
Since the 1970s, nutrient management strategies have been applied to the Baltic Sea catchment area, resulting in a reduction in anthropogenic nitrogen load by ∼ 25 % (Reusch et al., 2018). At the same time, however, nitrogen load by fixation increased notably (Gustafsson et al., 2017). Model simulations have demonstrated that the contribution of nitrogen fixation to the total nitrogen load in the Baltic Sea increased from almost 20 % in the 1980s to almost 35 % in the 2000s such that the total nitrogen load decreased by only ∼ 9 % (Gustafsson et al., 2017). For the future, our results suggest that the extent of nitrogen fixation in the Baltic Sea will further increase with rising temperatures. Even though this increase may be limited by adaptation and the resulting stronger competition between cyanobacteria and other phytoplankton taxa, higher temperatures will still have a positive effect on nitrogen fixation. Thus, the importance of nitrogen fixation for the nitrogen budget of the Baltic Sea will most likely continue to increase and further mitigate the success of nutrient management strategies. Therefore, nutrient management strategies urgently need to account for nitrogen load by fixation to be successful in the future. Since our projected increase in nitrogen fixation is significantly reduced if we consider phytoplankton adaptation, we strongly recommend that models used for assessment consider phytoplankton adaptation to realistically estimate future nitrogen load by fixation.
4.3 Secondary production
Our simulated changes in secondary production in response to global warming qualitatively agree with our simulated changes in total primary production. In our warming scenarios, both phytoplankton and zooplankton increase in abundance. A study by Richardson and Shoeman (2004) demonstrated that the abundance of herbivorous zooplankton significantly depends on their phytoplankton prey (bottom-up control), meaning that a warming-related increase in phytoplankton will most likely lead to an increase in zooplankton abundance. Similar to our findings for total primary production and nitrogen fixation, we observe that accounting for phytoplankton adaptation leads to a weaker increase in zooplankton biomass under global warming. These results indicate that future efforts to model ecosystems in terms of trophic transfer should consider phytoplankton adaptation as a crucial factor.
In addition, our simulations show a warming-related earlier bloom timing for phytoplankton and zooplankton, with the shift being stronger for zooplankton (Table A2). The resulting decrease in the time lag between primary producers and grazers stands in contrast to findings from other studies reporting a warming-related increase in time lag (Edwards and Richardson, 2004; Winder and Schindler, 2004a, b; Adrian et al., 2006). However, observations have revealed that some phytoplankton and zooplankton taxa indeed show synchronous shifts in bloom timing, for example diatoms and Daphnia (Adrian et al., 2006). Some studies even suggest a warming-related decrease in the time lag between phytoplankton and zooplankton (Aberle et al., 2012; Almén and Tamelander, 2020). Consequently, the reduced time lag produced by our model seems realistic for fast-growing zooplankton taxa like Daphnia, which can quickly respond to phenological changes in their phytoplankton prey.
4.4 Carbon export
Our simulations project a warming-related increase in carbon export in the future, which is significantly reduced if phytoplankton adaptation is enabled. The projected changes in carbon export correlate notably with projected changes in biomass production, which are dominated by a strong increase in cyanobacterial summer biomass. In the Baltic Sea, cyanobacteria blooms have intensified over the last century of global warming (Finni et al., 2001), especially during the last few decades (Suikkanen et al., 2007). This development is reflected by sediment records, which show a simultaneous increase in cyanobacteria pigments and carbon content during the same period (Poutanen and Nikkilä, 2001). In the future, warming is expected to further increase summer primary production with a positive feedback on carbon export in several areas of the Baltic Sea (Tamelander et al., 2017).
The ocean is a major sink for atmospheric carbon; the biological carbon pump is of similar magnitude to current carbon emissions from fossil fuels (Giering et al., 2020). Our results demonstrate that phytoplankton adaptation can have a significant impact on the amount of carbon exported and therefore needs to be taken into account for predictions of the global carbon cycle.
4.5 Resource use efficiency (RUE)
We furthermore analyzed how adaptation may influence RUE under global warming. Since we had to exclude nitrogen-fixing cyanobacteria and zooplankton grazing from RUE simulations, our assessments on potential effects of warming and adaptation on RUE are only valid for a two-species ecosystem including dinoflagellates and diatoms. For this species configuration, we found that adaptation increases resource use efficiency under both control and warming conditions. For both climate scenarios, adaptation is driven by competition for nitrogen, allowing dinoflagellates and diatoms to use the available nitrogen optimally within their means.
RUE is lower under global warming than under control conditions, both with and without adaptation. The warming-related decrease in RUE is predominantly driven by a fixed temperature-dependent life cycle trait of dinoflagellates (for details, see Hochfeld and Hinners, 2024), which terminates the dinoflagellate spring bloom at temperatures around 6 °C and thus restricts dinoflagellate adaptation to the increasing temperatures. With adaptation, however, RUE is still higher than without adaptation because diatoms are not restricted by their life cycle and can therefore optimize their nitrogen uptake even under global warming.
Our simulations show that adaptation generally allows for a more efficient use of resources and thus higher RUE. Models that ignore adaptation may hence systematically underestimate RUE under both present-day and future conditions. In addition, our results demonstrate that future models should consider not only adaptation, but also possible species-specific constraints on adaptation, such as life cycle dynamics.
4.6 Control factors and feedbacks in our model ecosystem
We found that all ecosystem functions are positively correlated in our simulations, except for dinoflagellate annual biomass (and RUE). Under control conditions, all correlations (regardless of their direction) are stronger when phytoplankton adaptation is considered. Dinoflagellates and diatoms adapt to individual temperature niches to reduce competition for nitrogen, with the reduced competition between diatoms and cyanobacteria allowing for a stronger cyanobacterial summer bloom and hence increased nitrogen fixation (see Fig. B2 and Hochfeld and Hinners, 2024). Zooplankton peak during dinoflagellate spring bloom (Figs. B2 and B3), meaning that dinoflagellates constitute the main food source for zooplankton. Hence, the stronger dinoflagellates grow due to increased nitrogen fixation, the more they are grazed by zooplankton. Thus, the increased cyanobacterial nitrogen fixation indirectly fuels zooplankton growth, while it directly fuels the growth of diatoms. The result is an overall increase in biomass production, which in turn increases carbon export. Dinoflagellates are the only losers in this scenario due to the strong grazing pressure by zooplankton.
Under global warming, however, adaptation leads to an overall weakening of correlations, even though cyanobacteria are stronger than under control conditions. While diatoms benefit from the increased nitrogen fixation, grazing pressure on them increases, weakening the positive correlation with zooplankton. In addition, as demonstrated by Hochfeld and Hinners (2024), the presence of cyanobacteria in summer restricts diatom adaptation to the increasing temperatures, causing a weaker positive correlation between diatoms and cyanobacteria. Due to the stronger grazing on diatoms, zooplankton are also less positively impacted by cyanobacteria. The weaker positive effect of cyanobacteria on diatoms and zooplankton is reflected in a slight weakening of the remaining positive correlations and a notable weakening of the negative correlations with dinoflagellates. Furthermore, the reduced relative grazing pressure on dinoflagellates reverses the negative correlation with zooplankton, meaning that an increase in zooplankton biomass no longer implies a decrease in dinoflagellate biomass.
To conclude, cyanobacteria are the most important control factor in our model ecosystem, which is also confirmed by a principal component analysis (Fig. B6). First, cyanobacteria produce the highest amount of biomass per year. Second, through their ability to fix atmospheric nitrogen, they directly control the biomass production of dinoflagellates and diatoms and indirectly that of zooplankton. Cyanobacteria are therefore the main factor for carbon export in our simulations, which also agrees with observations as discussed above (see Sect. 4.4). However, the interdependencies between cyanobacteria and the other taxa may change depending on the climate scenario and the presence or absence of phytoplankton adaptation. These results demonstrate that by neglecting adaptation, we may be missing adaptation-related changes in taxa interactions, especially in changing environments, which can affect the entire ecosystem and hence its functioning.
4.7 Model biases and outlook
In the following, we discuss the simplifications and assumptions of our model that may bias our predicted changes in ecosystem functioning. Based on this discussion, we give suggestions for future modeling studies on climate-related ecosystem changes.
First, our model lacks quantitative validation against observational data. For the Baltic Sea, phytoplankton observations at the species level are sparse and insufficient in temporal resolution and/or temporal coverage to allow extensive quantitative model validation. Data that provide sufficient temporal resolution and coverage are usually at the functional group level and thus show the signal of the focal species superimposed on the signal of other species from the same functional group. Thus, such data are not suitable for quantitative model validation, but they can still narrow down the seasonality of the focal species and hence provide information on qualitative differences between the model taxa. Here, we used functional-group-level data from Hjerne et al. (2019) to validate our ecosystem model qualitatively (Sect. 3.1 and Fig. B2). Due to the lack of extensive quantitative validation, however, we only evaluate our results qualitatively and focus on identifying fundamental relationships between phytoplankton adaptation and ecosystem functioning.
Second, simulating at the species level may limit the generality of our results. For example, our projected warming-related decrease in RUE results from a fixed temperature threshold in the life cycle of the modeled dinoflagellate and hence only applies to the species configuration in our model. We cannot make statements about future changes in RUE in other ecosystems with a different set of species. Future work can build on our results and investigate RUE in more complex ecosystems to make more general statements about future warming-related changes. Nevertheless, we think that our simulated adaptation-related increase in RUE is robust and independent of the species configuration in our model.
In addition to biases in phytoplankton, further biases may be introduced by our simplistic representation of zooplankton. We assume that zooplankton grazing depends exclusively on phytoplankton biomass and do not consider potential effects of irradiance and temperature. Moreover, we neglect both zooplankton life cycle dynamics and zooplankton adaptation. Thus, the zooplankton in our model is entirely controlled by prey availability, which may be reasonable for fast-growing taxa that were found to respond rapidly to changes in their phytoplankton prey (Adrian et al., 2006). However, our representation of zooplankton is inappropriate for simulating slow-growing taxa with longer and more complex life cycles (Adrian et al., 2006). Future work can build on our model and study how a more complex representation of zooplankton, including both fast- and slow-growing taxa, and higher trophic levels may be affected by phytoplankton adaptation.
Furthermore, we use a zero-dimensional model setup, which may lead to biases in all predicted ecosystem functions, particularly in carbon export. For example, we cannot explicitly simulate physical processes in the ocean like vertical mixing, including seasonal and future changes in stratification and mixed layer depth. Multiple studies suggest a future increase in ocean surface stratification, which may reduce vertical nutrient fluxes and hence affect primary production, marine food web dynamics, and carbon export (Capotondi et al., 2012; Hordoir and Meier, 2012; Sallée et al., 2021). Thus, our projected increase in primary production might not be repeated if our model accounted for future decreases in nutrient supply in a more stratified system. However, an increase in primary production indeed seems likely under current anthropogenic nutrient loads for several areas of the Baltic Sea, as predicted by a three-dimensional coupled biogeochemical–physical model (Meier et al., 2011). Considering carbon export specifically, other crucial processes like gravitational particle sinking and fragmentation are only included implicitly in our model, while we neglect vertical migration of zooplankton and nekton (Henson et al., 2022). In addition, in semi-enclosed ecosystems like the Baltic Sea, carbon export is not predominantly fueled by phytoplankton primary production but also by benthic primary production and riverine and terrestrial inputs (Goñi et al., 2000; Renaud et al., 2015; Tallberg and Heiskanen, 1998). Since these key processes (and maybe others) are lacking in our model, we cannot interpret our results as projections of future carbon export. Instead, we interpret them as projections of the future contribution of primary production to carbon export.
Finally, our results may be influenced by our implementation of evolutionary adaptation. We use an agent-based approach to simulate evolutionary trait changes in super-individuals (agents) due to random mutations. Since the mutated trait value is sampled from a normal distribution centered at the parental trait value, we need information about the standard deviation of this distribution (i.e., the mutational step size), which cannot be measured directly in the laboratory. However, simulated trait changes over time can be compared to observed trait changes. As shown by Hochfeld and Hinners (2024), the model used here agrees well with rates of trait change observed in the laboratory and in nature (Irwin et al., 2015; Jin and Agustí, 2018). In addition to parameter-related biases, our results are sensitive to resolution (i.e., the number of cells per agent). To ensure robust results without increasing computation time by orders of magnitude, we consider the results of seven different simulations per model scenario. Despite these biases, the agent-based approach used here has notable advantages over population-level approaches that do not track the evolutionary history of individuals. Population models based on fitness gradients, which assume that trait change is proportional to change in population fitness (Grimaud et al., 2015; Norberg et al., 2012; Pahlow et al., 2008; Wirtz, 2013), are inapplicable to complex fitness landscapes with thresholds. So-called trait diffusion models, on the other hand, represent mutations as non-random biomass fluxes in trait space, with both discrete (Beckmann et al., 2019; Hinners et al., 2019; Sauterey et al., 2017) and continuous versions (Chen et al., 2019; Le Gland et al., 2021; Merico et al., 2014; Smith et al., 2016b). Since continuous trait diffusion models require the shape of the trait distribution to be prescribed, such models cannot account for multimodality or evolutionary branching. In conclusion, the agent-based approach used here provides the most realistic representation of evolution among current approaches. To date, agent-based approaches have been used to investigate evolutionary mechanisms under laboratory conditions (Beckmann et al., 2019; Clark et al., 2011; Collins, 2016), to derive biogeographic patterns (Daines et al., 2014; Sauterey and Ward, 2022), or to infer the effects of global warming on biogeochemical cycling based on stoichiometric changes (Toseland et al., 2013). To our knowledge, our model is the first agent-based model, and indeed the first ecosystem model, to explicitly simulate the effects of phytoplankton adaptation to global warming on ecosystem functioning.
Our study demonstrates that phytoplankton adaptation affects simulated ecosystem functions through bottom-up control. The effect of phytoplankton adaptation on simulated ecosystem functions depends on environmental conditions.
In a steady environment, phytoplankton adaptation allows for a more efficient use of resources through niche separation, which in turn increases primary production. An increase in primary production may enhance secondary production, nitrogen fixation, carbon export, and maybe even other ecosystem functions not included in this study. Thus, by neglecting adaptation, models can systematically underestimate resource use efficiency in a steady environment and hence ecosystem functions that are directly related to primary production. In a warming environment, however, adaptation has the opposite effect. With the ability to adapt to the increasing temperatures, non-pre-adapted taxa can mitigate the dominance of superior pre-adapted taxa. Since different taxa fulfill different functions in the ecosystem, weaker changes in their abundance lead to weaker changes in associated ecosystem functions. By neglecting phytoplankton adaptation, models may therefore systematically underestimate the resilience of phytoplankton communities to environmental change, which may lead to a systematic overestimation of warming-induced changes in ecosystem functioning. Thus, to realistically simulate ecosystem functioning in both steady and changing environments, future models should consider not only multiple phytoplankton functional groups due to their different roles in the ecosystem but also their potential to adapt to their environment.
Our study is a first step toward improving model projections of future ecosystem-level changes. Future work can build on our results, for example by expanding on our model ecosystem to include multiple nutrients, a higher diversity of phytoplankton functional groups, a more complex representation of zooplankton, and higher trophic levels. Another next step would be to couple our or a similar evolutionary ecosystem model to a one-dimensional or three-dimensional physical environment to allow for a more realistic representation of physically driven processes, e.g., biogeochemical cycling. The performance of such an evolutionary biogeochemical–physical model could then be tested against long-term evolutionary data (e.g., from sediment archives). Using such a validated model for climate projections could notably improve estimates of future ecosystem-level changes.
Table A1Results of a series of t tests comparing all model scenarios (C – control, CA – control and adaptation, W – warming, WA – warming and adaptation) with regard to annual balances. The table presents the value of the test statistic (t), the degrees of freedom (df's), and the p value (p). Note that we used a paired-sample t test when comparing control and warming simulations, since these were performed pairwise, and a two-sample t test otherwise.
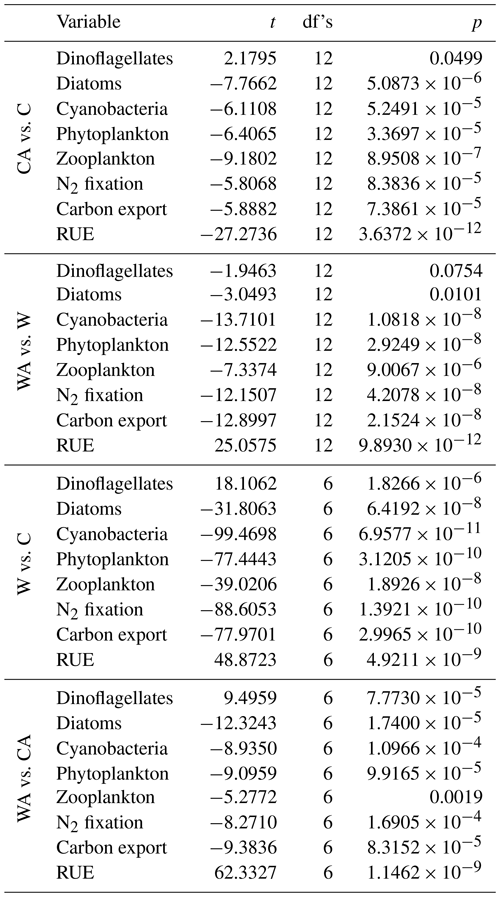
Table A2Average timing of phytoplankton and zooplankton blooms in spring, as well as the time lag between phytoplankton and zooplankton for the two control scenarios C (control) and CA (control and adaptation), along with the associated standard deviations. Also shown are the corresponding average warming-related changes in W (warming) and WA (warming and adaptation), including propagated errors. For each scenario, we calculated average values from the last simulation year of seven different simulations.

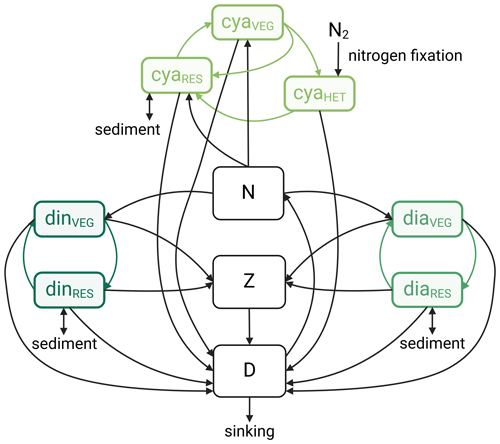
Figure B1Components of the Hochfeld and Hinners (2024) ecosystem model, including compartments for dissolved inorganic nitrogen (N), detritus (D), and zooplankton (Z), along with agent-based life cycles of dinoflagellates (din), diatoms (dia), and cyanobacteria (cya). Each life cycle is represented by a resting stage (RES) and a vegetative growing stage (vegetative cells, VEG). For cyanobacteria, the model simulates a second, nitrogen-fixing growing stage (vegetative cells with heterocysts, HET). The figure additionally shows the nitrogen fluxes between the different ecosystem components, as well as the sinks and sources of nitrogen (sinking of detritus, burial of phytoplankton resting cells, resuspension of phytoplankton resting cells (not part of this study and therefore disabled), and cyanobacterial nitrogen fixation). The figure was adapted from Hochfeld and Hinners (2024) under the terms of the Creative Commons Attribution 4.0 International License (https://creativecommons.org/licenses/by/4.0/, last access: 26 November 2024) and created with BioRender.com.
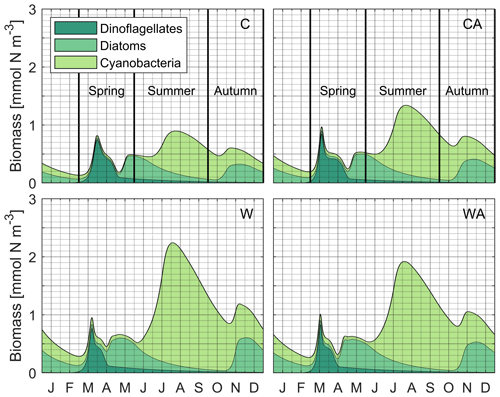
Figure B2Accumulated phytoplankton biomass during the last simulation year of the four different model scenarios (C – control, CA – control and adaptation, W – warming, WA – warming and adaptation). For each scenario, the output of seven different simulations was averaged. The colors indicate the share of dinoflagellates, diatoms, and cyanobacteria in the total phytoplankton biomass. In the two control scenarios, the vertical black lines show the observed timing of the Baltic Sea spring bloom, the cyanobacterial summer bloom, and the diatom autumn bloom (Hjerne et al., 2019; bloom periods were derived from Fig. 2c). Note that the accumulated phytoplankton biomass shown in this figure includes both growing and resting stages integrated over the entire water column due to the zero-dimensional model setup, while the monitoring data by Hjerne et al. (2019) only cover the first 20 m. Thus, the figure most likely overestimates resting stage biomass compared to the monitoring data, meaning that the simulated biomass peak(s) of a taxon, rather than the general presence of the taxon, are most relevant for model validation.
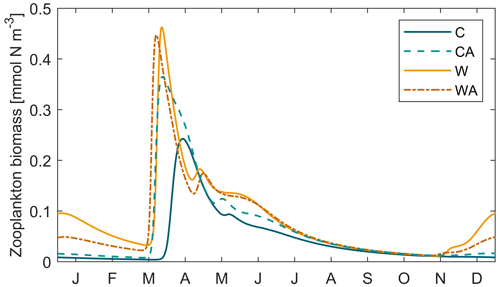
Figure B3Zooplankton biomass during the last simulation year of the four different model scenarios (C – control, CA – control and adaptation, W – warming, WA – warming and adaptation). For each scenario, we averaged the output of seven different simulations.
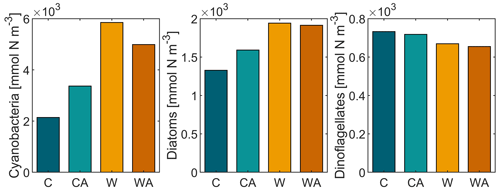
Figure B4Annual balances of cyanobacteria, diatoms, and dinoflagellates for the four different model scenarios (C – control, CA – control and adaptation, W – warming, WA – warming and adaptation). This figure has been recomposed from Fig. 1.
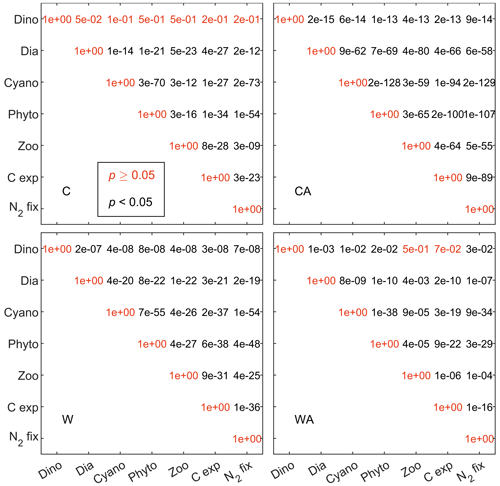
Figure B5Matrices showing the p values for the correlations in Fig. 2 (Sect. 3.2). Model scenario abbreviations: C – control, CA – control and adaptation, W – warming, WA – warming and adaptation. The black numbers indicate that the corresponding correlations are statistically significant at the 0.05 level, while the orange numbers indicate the opposite.
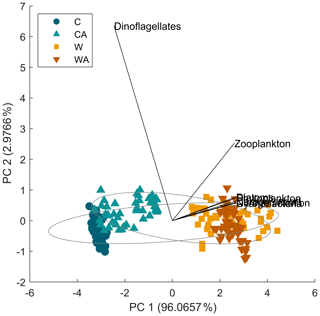
Figure B6Results for a principal component analysis (PCA). The PCA shows that most variability in our model ecosystem can be explained by the first principal component (PC 1), which is associated with all model variables that are positively impacted by cyanobacteria. Zooplankton and especially dinoflagellates can be clearly identified as outliers.
The model code and the scripts for evaluating the model output and creating the figures are available on GitHub at https://github.com/Isabell-Hochfeld/Adaptive-Phytoplankton-Community-Model (last access: 23 April 2024) and on Zenodo at https://doi.org/10.5281/zenodo.10693812 (version 1.1.0, Hochfeld, 2024). All code is written in MATLAB (version R2022a).
The data used for model validation are published in Hjerne et al. (2019), Dutz et al. (2010), and Feike et al. (2007).
JH and IH designed the study. IH modified the model, performed the model simulations, and analyzed the model output. Both authors contributed to writing the paper.
The contact author has declared that neither of the authors has any competing interests.
Publisher's note: Copernicus Publications remains neutral with regard to jurisdictional claims made in the text, published maps, institutional affiliations, or any other geographical representation in this paper. While Copernicus Publications makes every effort to include appropriate place names, the final responsibility lies with the authors.
We would like to thank the editorial board of Biogeosciences and the two anonymous reviewers for their constructive feedback on our paper.
This research has been supported by the Leibniz-Gemeinschaft (grant no. K314/2020).
This paper was edited by Emilio Marañón and reviewed by two anonymous referees.
Aberle, N., Bauer, B., Lewandowska, A., Gaedke, U., and Sommer, U.: Warming induces shifts in microzooplankton phenology and reduces time-lags between phytoplankton and protozoan production, Mar. Biol., 159, 2441–2453, https://doi.org/10.1007/s00227-012-1947-0, 2012.
Acevedo-Trejos, E., Marañón, E., and Merico, A.: Phytoplankton size diversity and ecosystem function relationships across oceanic regions, Proc. Roy. Soc. B, 285, 20180621, https://doi.org/10.1098/rspb.2018.0621, 2018.
Adrian, R., Wilhelm, S., and Gerten, D.: Life-history traits of lake plankton species may govern their phenological response to climate warming, Glob. Change Biol., 12, 652–661, https://doi.org/10.1111/j.1365-2486.2006.01125.x, 2006.
Almén, A.-K. and Tamelander, T.: Temperature-related timing of the spring bloom and match between phytoplankton and zooplankton, Mar. Biol. Res., 16, 674–682, https://doi.org/10.1080/17451000.2020.1846201, 2020.
Asch, R. G., Stock, C. A., and Sarmiento, J. L.: Climate change impacts on mismatches between phytoplankton blooms and fish spawning phenology, Glob. Change Biol., 25, 2544–2559, https://doi.org/10.1111/gcb.14650, 2019.
Atkinson, A., Siegel, V., Pakhomov, E., and Rothery, P.: Long-term decline in krill stock and increase in salps within the Southern Ocean, Nature, 432, 100–103, https://doi.org/10.1038/nature02996, 2004.
Atkinson, D., Ciotti, B. J., and Montagnes, D. J. S.: Protists decrease in size linearly with temperature: ca. 2.5 % °C−1, Proc. Roy. Soc. Lond. Ser. B, 270, 2605–2611, https://doi.org/10.1098/rspb.2003.2538, 2003.
Backer, L. C. and McGillicuddy, D. J.: Harmful Algal Blooms: At the Interface Between Coastal Oceanography and Human Health, Oceanography, 19, 94–106, https://doi.org/10.5670/oceanog.2006.72, 2006.
Banas, N. S.: Adding complex trophic interactions to a size-spectral plankton model: Emergent diversity patterns and limits on predictability, Ecol. Model., 222, 2663–2675, https://doi.org/10.1016/j.ecolmodel.2011.05.018, 2011.
Basu, S. and Mackey, K. R. M.: Phytoplankton as Key Mediators of the Biological Carbon Pump: Their Responses to a Changing Climate, Sustainability, 10, 869, https://doi.org/10.3390/su10030869, 2018.
Beckmann, A., Schaum, C.-E., and Hense, I.: Phytoplankton adaptation in ecosystem models, J. Theor. Biol., 468, 60–71, https://doi.org/10.1016/j.jtbi.2019.01.041, 2019.
Breitburg, D., Levin, L. A., Oschlies, A., Grégoire, M., Chavez, F. P., Conley, D. J., Garçon, V., Gilbert, D., Gutiérrez, D., Isensee, K., Jacinto, G. S., Limburg, K. E., Montes, I., Naqvi, S. W. A., Pitcher, G. C., Rabalais, N. N., Roman, M. R., Rose, K. A., Seibel, B. A., Telszewski, M., Yasuhara, M., and Zhang, J.: Declining oxygen in the global ocean and coastal waters, Science, 359, eaam7240, https://doi.org/10.1126/science.aam7240, 2018.
Bruggeman, J. and Kooijman, S. A. L. M.: A biodiversity-inspired approach to aquatic ecosystem modeling, Limnol. Oceanogr., 52, 1533–1544, https://doi.org/10.4319/lo.2007.52.4.1533, 2007.
Capotondi, A., Alexander, M. A., Bond, N. A., Curchitser, E. N., and Scott, J. D.: Enhanced upper ocean stratification with climate change in the CMIP3 models, J. Geophys. Res.-Ocean., 117, C04031, https://doi.org/10.1029/2011JC007409, 2012.
Chen, B., Smith, S. L., and Wirtz, K. W.: Effect of phytoplankton size diversity on primary productivity in the North Pacific: trait distributions under environmental variability, Ecol. Lett., 22, 56–66, https://doi.org/10.1111/ele.13167, 2019.
Cherabier, P. and Ferrière, R.: Eco-evolutionary responses of the microbial loop to surface ocean warming and consequences for primary production, ISME J., 16, 1130–1139, https://doi.org/10.1038/s41396-021-01166-8, 2022.
Chorus, I. and Welker, M. (Eds.): Toxic Cyanobacteria in Water, 2nd Edn., CRC Press, Boca Raton (FL), 859 pp., https://doi.org/10.1201/9781003081449, 2021.
Clark, J. R., Daines, S. J., Lenton, T. M., Watson, A. J., and Williams, H. T. P.: Individual-based modelling of adaptation in marine microbial populations using genetically defined physiological parameters, Ecol. Model., 222, 3823–3837, https://doi.org/10.1016/j.ecolmodel.2011.10.001, 2011.
Collins, C. D. and Boylen, C. W.: Physiological Responses of Anabaena variabilis (cyanophyceae) to Instantaneous Exposure to Various Combinations of Light Intensity and Temperature, J. Phycol., 18, 206–211, https://doi.org/10.1111/j.1529-8817.1982.tb03175.x, 1982.
Collins, S.: Growth rate evolution in improved environments under Prodigal Son dynamics, Evol. Appl., 9, 1179–1188, https://doi.org/10.1111/eva.12403, 2016.
Daines, S. J., Clark, J. R., and Lenton, T. M.: Multiple environmental controls on phytoplankton growth strategies determine adaptive responses of the N:P ratio, Ecol. Lett., 17, 414–425, https://doi.org/10.1111/ele.12239, 2014.
Dutkiewicz, S., Cermeno, P., Jahn, O., Follows, M. J., Hickman, A. E., Taniguchi, D. A. A., and Ward, B. A.: Dimensions of marine phytoplankton diversity, Biogeosciences, 17, 609–634, https://doi.org/10.5194/bg-17-609-2020, 2020.
Dutz, J., Mohrholz, V., and van Beusekom, J. E. E.: Life cycle and spring phenology of Temora longicornis in the Baltic Sea, Mar. Ecol. Prog. Ser., 406, 223–238, https://doi.org/10.3354/meps08545, 2010.
Edwards, M. and Richardson, A. J.: Impact of climate change on marine pelagic phenology and trophic mismatch, Nature, 430, 881–884, https://doi.org/10.1038/nature02808, 2004.
Everson, I. (Ed.): Krill: Biology, Ecology, and Fisheries, Blackwell Science, 372 pp., https://doi.org/10.1002/9780470999493, 2000.
Feike, M., Heerkloss, R., Rieling, T., and Schubert, H.: Studies on the zooplankton community of a shallow lagoon of the Southern Baltic Sea: long-term trends, seasonal changes, and relations with physical and chemical parameters, Hydrobiologia, 577, 95–106, https://doi.org/10.1007/s10750-006-0420-9, 2007.
Field, C. B., Behrenfeld, M. J., Randerson, J. T., and Falkowski, P.: Primary Production of the Biosphere: Integrating Terrestrial and Oceanic Components, Science, 281, 237–240, https://doi.org/10.1126/science.281.5374.237, 1998.
Finni, T., Kononen, K., Olsonen, R., and Wallström, K.: The History of Cyanobacterial Blooms in the Baltic Sea, Ambi, 30, 172–178, https://doi.org/10.1579/0044-7447-30.4.172, 2001.
Follows, M. J., Dutkiewicz, S., Grant, S., and Chisholm, S. W.: Emergent Biogeography of Microbial Communities in a Model Ocean, Science, 315, 1843–1846, https://doi.org/10.1126/science.1138544, 2007.
Giering, S. L. C., Cavan, E. L., Basedow, S. L., Briggs, N., Burd, A. B., Darroch, L. J., Guidi, L., Irisson, J.-O., Iversen, M. H., Kiko, R., Lindsay, D., Marcolin, C. R., McDonnell, A. M. P., Möller, K. O., Passow, U., Thomalla, S., Trull, T. W., and Waite, A. M.: Sinking Organic Particles in the Ocean – Flux Estimates From in situ Optical Devices, Front. Mar. Sci., 6, 834, https://doi.org/10.3389/fmars.2019.00834, 2020.
Glibert, P. M., Icarus Allen, J., Artioli, Y., Beusen, A., Bouwman, L., Harle, J., Holmes, R., and Holt, J.: Vulnerability of coastal ecosystems to changes in harmful algal bloom distribution in response to climate change: projections based on model analysis, Glob. Change Biol., 20, 3845–3858, https://doi.org/10.1111/gcb.12662, 2014.
Gobler, C. J., Doherty, O. M., Hattenrath-Lehmann, T. K., Griffith, A. W., Kang, Y., and Litaker, R. W.: Ocean warming since 1982 has expanded the niche of toxic algal blooms in the North Atlantic and North Pacific oceans, P. Natl. Acad. Sci. USA, 114, 4975–4980, https://doi.org/10.1073/pnas.1619575114, 2017.
Goñi, M. A., Yunker, M. B., Macdonald, R. W., and Eglinton, T. I.: Distribution and sources of organic biomarkers in arctic sediments from the Mackenzie River and Beaufort Shelf, Mar. Chem., 71, 23–51, https://doi.org/10.1016/S0304-4203(00)00037-2, 2000.
Grimaud, G. M., Le Guennec, V., Ayata, S.-D., Mairet, F., Sciandra, A., and Bernard, O.: Modelling the effect of temperature on phytoplankton growth across the global ocean, IFAC-PapersOnLine, 48, 228–233, https://doi.org/10.1016/j.ifacol.2015.05.059, 2015.
Grover, J. P.: Resource Competition in a Variable Environment: Phytoplankton Growing According to the Variable-Internal-Stores Model, Am. Nat., 138, 811–835, https://doi.org/10.1086/285254, 1991.
Gustafsson, B. G., Schenk, F., Blenckner, T., Eilola, K., Meier, H. E. M., Müller-Karulis, B., Neumann, T., Ruoho-Airola, T., Savchuk, O. P., and Zorita, E.: Reconstructing the Development of Baltic Sea Eutrophication 1850–2006, AMBIO, 41, 534–548, https://doi.org/10.1007/s13280-012-0318-x, 2012.
Gustafsson, E., Savchuk, O. P., Gustafsson, B. G., and Müller-Karulis, B.: Key processes in the coupled carbon, nitrogen, and phosphorus cycling of the Baltic Sea, Biogeochemistry, 134, 301–317, https://doi.org/10.1007/s10533-017-0361-6, 2017.
Hallegraeff, G. M.: Ocean Climate Change, Phytoplankton Community Responses, and Harmful Algal Blooms: A Formidable Predictive Challenge, J. Phycol., 46, 220–235, https://doi.org/10.1111/j.1529-8817.2010.00815.x, 2010.
Hattich, G. S. I., Jokinen, S., Sildever, S., Gareis, M., Heikkinen, J., Junghardt, N., Segovia, M., Machado, M., and Sjöqvist, C.: Temperature optima of a natural diatom population increases as global warming proceeds, Nat. Clim. Change, 14, 518–525, https://doi.org/10.1038/s41558-024-01981-9, 2024.
Hense, I. and Beckmann, A.: Towards a model of cyanobacteria life cycle – effects of growing and resting stages on bloom formation of N2-fixing species, Ecol. Model., 195, 205–218, https://doi.org/10.1016/j.ecolmodel.2005.11.018, 2006.
Hense, I., Meier, H. E. M., and Sonntag, S.: Projected climate change impact on Baltic Sea cyanobacteria, Climatic Change, 119, 391–406, https://doi.org/10.1007/s10584-013-0702-y, 2013.
Henson, S. A., Laufkötter, C., Leung, S., Giering, S. L. C., Palevsky, H. I., and Cavan, E. L.: Uncertain response of ocean biological carbon export in a changing world, Nat. Geosci., 15, 248–254, https://doi.org/10.1038/s41561-022-00927-0, 2022.
Hinners, J., Hofmeister, R., and Hense, I.: Modeling the Role of pH on Baltic Sea Cyanobacteria, Life, 5, 1204–1217, https://doi.org/10.3390/life5021204, 2015.
Hinners, J., Hense, I., and Kremp, A.: Modelling phytoplankton adaptation to global warming based on resurrection experiments, Ecol. Model., 400, 27–33, https://doi.org/10.1016/j.ecolmodel.2019.03.006, 2019.
Hjerne, O., Hajdu, S., Larsson, U., Downing, A. S., and Winder, M.: Climate Driven Changes in Timing, Composition and Magnitude of the Baltic Sea Phytoplankton Spring Bloom, Front. Mar. Sci., 6, 482, https://doi.org/10.3389/fmars.2019.00482, 2019.
Hochfeld, I.: Adaptive Phytoplankton Community Model (version 1.1.0), Zenodo [code], https://doi.org/10.5281/zenodo.10693812, 2024.
Hochfeld, I. and Hinners, J.: Evolutionary adaptation to steady or changing environments affects competitive outcomes in marine phytoplankton, Limnol. Oceanogr., 69, 1172–1186, https://doi.org/10.1002/lno.12559, 2024.
Hordoir, R. and Meier, H. E. M.: Effect of climate change on the thermal stratification of the baltic sea: a sensitivity experiment, Clim. Dynam., 38, 1703–1713, https://doi.org/10.1007/s00382-011-1036-y, 2012.
IPCC: Summary for Policymakers, in: Climate Change 2021: The Physical Science Basis. Contribution of Working Group I to the Sixth Assessment Report of the Intergovernmental Panel on Climate Change, edited by: Masson-Delmotte, V., Zhai, P., Pirani, A., Connors, S. L., Péan, C., Berger, S., Caud, N., Chen, Y., Goldfarb, L., Gomis, M. I., Huang, M., Leitzell, K., Lonnoy, E., Matthews, J. B. R., Maycock, T. K., Waterfield, T., Yelekçi, O., Yu, R., and Zhou, B., Cambridge University Press, Cambridge, United Kingdom and New York, NY, USA, 3–32, https://doi.org/10.1017/9781009157896.001, 2021.
IPCC: Climate Change 2022: Impacts, Adaptation and Vulnerability. Contribution of Working Group II to the Sixth Assessment Report of the Intergovernmental Panel on Climate Change, edited by: Pörtner, H.-O., Roberts, D. C., Tignor, M., Poloczanska, E. S., Mintenbeck, K., Alegría, A., Craig, M., Langsdorf, S., Löschke, S., Möller, V., Okem, A., and Rama, B., Cambridge University Press, Cambridge, UK and New York, NY, USA, 3056 pp., https://doi.org/10.1017/9781009325844, 2022.
Irwin, A. J., Finkel, Z. V., Müller-Karger, F. E., and Troccoli Ghinaglia, L.: Phytoplankton adapt to changing ocean environments, P. Natl. Acad. Sci. USA, 112, 5762–5766, https://doi.org/10.1073/pnas.1414752112, 2015.
Jin, P. and Agustí, S.: Fast adaptation of tropical diatoms to increased warming with trade-offs, Sci. Rep., 8, 17771, https://doi.org/10.1038/s41598-018-36091-y, 2018.
Karlsson, K. M., Kankaanpää, H., Huttunen, M., and Meriluoto, J.: First observation of microcystin-LR in pelagic cyanobacterial blooms in the northern Baltic Sea, Harmful Algae, 4, 163–166, https://doi.org/10.1016/j.hal.2004.02.002, 2005.
Kerimoglu, O., Hofmeister, R., Maerz, J., Riethmüller, R., and Wirtz, K. W.: The acclimative biogeochemical model of the southern North Sea, Biogeosciences, 14, 4499–4531, https://doi.org/10.5194/bg-14-4499-2017, 2017.
Laufkötter, C., Vogt, M., Gruber, N., Aita-Noguchi, M., Aumont, O., Bopp, L., Buitenhuis, E., Doney, S. C., Dunne, J., Hashioka, T., Hauck, J., Hirata, T., John, J., Le Quéré, C., Lima, I. D., Nakano, H., Seferian, R., Totterdell, I., Vichi, M., and Völker, C.: Drivers and uncertainties of future global marine primary production in marine ecosystem models, Biogeosciences, 12, 6955–6984, https://doi.org/10.5194/bg-12-6955-2015, 2015.
Laufkötter, C., Vogt, M., Gruber, N., Aumont, O., Bopp, L., Doney, S. C., Dunne, J. P., Hauck, J., John, J. G., Lima, I. D., Seferian, R., and Völker, C.: Projected decreases in future marine export production: the role of the carbon flux through the upper ocean ecosystem, Biogeosciences, 13, 4023–4047, https://doi.org/10.5194/bg-13-4023-2016, 2016.
Le Gland, G., Vallina, S. M., Smith, S. L., and Cermeño, P.: SPEAD 1.0 – Simulating Plankton Evolution with Adaptive Dynamics in a two-trait continuous fitness landscape applied to the Sargasso Sea, Geosci. Model Dev., 14, 1949–1985, https://doi.org/10.5194/gmd-14-1949-2021, 2021.
Lee, S., Hofmeister, R., and Hense, I.: The role of life cycle processes on phytoplankton spring bloom composition: a modelling study applied to the Gulf of Finland, J. Mar. Syst., 178, 75–85, https://doi.org/10.1016/j.jmarsys.2017.10.010, 2018.
Lehtimäki, J., Moisander, P., Sivonen, K., and Kononen, K.: Growth, nitrogen fixation, and nodularin production by two Baltic Sea cyanobacteria, Appl. Environ. Microbiol., 63, 1647–1656, https://doi.org/10.1128/aem.63.5.1647-1656.1997, 1997.
Litchman, E., Klausmeier, C. A., Schofield, O. M., and Falkowski, P. G.: The role of functional traits and trade-offs in structuring phytoplankton communities: scaling from cellular to ecosystem level, Ecol. Lett., 10, 1170–1181, https://doi.org/10.1111/j.1461-0248.2007.01117.x, 2007.
Litchman, E., de Tezanos Pinto, P., Edwards, K. F., Klausmeier, C. A., Kremer, C. T., and Thomas, M. K.: Global biogeochemical impacts of phytoplankton: a trait-based perspective, J. Ecol., 103, 1384–1396, https://doi.org/10.1111/1365-2745.12438, 2015.
Lomartire, S., Marques, J. C., and Gonçalves, A. M. M.: The key role of zooplankton in ecosystem services: A perspective of interaction between zooplankton and fish recruitment, Ecol. Indic., 129, 107867, https://doi.org/10.1016/j.ecolind.2021.107867, 2021.
Long, A. M., Jurgensen, S. K., Petchel, A. R., Savoie, E. R., and Brum, J. R.: Microbial Ecology of Oxygen Minimum Zones Amidst Ocean Deoxygenation, Front. Microbiol., 12, 748961, https://doi.org/10.3389/fmicb.2021.748961, 2021.
Marañón, E., Cermeño, P., López-Sandoval, D. C., Rodríguez-Ramos, T., Sobrino, C., Huete-Ortega, M., Blanco, J. M., and Rodríguez, J.: Unimodal size scaling of phytoplankton growth and the size dependence of nutrient uptake and use, Ecol. Lett., 16, 371–379, https://doi.org/10.1111/ele.12052, 2013.
Meier, H. E. M., Eilola, K., and Almroth, E.: Climate-related changes in marine ecosystems simulated with a 3-dimensional coupled physical-biogeochemical model of the Baltic Sea, Clim. Res., 48, 31–55, https://doi.org/10.3354/cr00968, 2011.
Meier, H. E. M., Andersson, H. C., Arheimer, B., Donnelly, C., Eilola, K., Gustafsson, B. G., Kotwicki, L., Neset, T.-S., Niiranen, S., Piwowarczyk, J., Savchuk, O. P., Schenk, F., Węsławski, J. M., and Zorita, E.: Ensemble Modeling of the Baltic Sea Ecosystem to Provide Scenarios for Management, AMBIO, 43, 37–48, https://doi.org/10.1007/s13280-013-0475-6, 2014.
Merico, A., Bruggeman, J., and Wirtz, K.: A trait-based approach for downscaling complexity in plankton ecosystem models, Ecol. Model., 220, 3001–3010, https://doi.org/10.1016/j.ecolmodel.2009.05.005, 2009.
Merico, A., Brandt, G., Smith, S. L., and Oliver, M.: Sustaining diversity in trait-based models of phytoplankton communities, Front. Ecol. Evol., 2, 59, https://doi.org/10.3389/fevo.2014.00059, 2014.
Munkes, B., Löptien, U., and Dietze, H.: Cyanobacteria blooms in the Baltic Sea: a review of models and facts, Biogeosciences, 18, 2347–2378, https://doi.org/10.5194/bg-18-2347-2021, 2021.
Nalewajko, C. and Murphy, T. P.: Effects of temperature, and availability of nitrogen and phosphorus on the abundance of Anabaena and Microcystis in Lake Biwa, Japan: an experimental approach, Limnology, 2, 45–48, https://doi.org/10.1007/s102010170015, 2001.
Neumann, T.: Climate-change effects on the Baltic Sea ecosystem: A model study, J. Mar. Syst., 81, 213–224, https://doi.org/10.1016/j.jmarsys.2009.12.001, 2010.
Norberg, J., Urban, M. C., Vellend, M., Klausmeier, C. A., and Loeuille, N.: Eco-evolutionary responses of biodiversity to climate change, Nat. Clim. Change, 2, 747–751, https://doi.org/10.1038/nclimate1588, 2012.
Paerl, H. W., Xu, H., Hall, N. S., Rossignol, K. L., Joyner, A. R., Zhu, G., and Qin, B.: Nutrient limitation dynamics examined on a multi-annual scale in Lake Taihu, China: implications for controlling eutrophication and harmful algal blooms, J. Freshwater Ecol., 30, 5–24, https://doi.org/10.1080/02705060.2014.994047, 2015.
Pahlow, M., Vézina, A. F., Casault, B., Maass, H., Malloch, L., Wright, D. G., and Lu, Y.: Adaptive model of plankton dynamics for the North Atlantic, Prog. Oceanogr., 76, 151–191, https://doi.org/10.1016/j.pocean.2007.11.001, 2008.
Pecl, G. T., Araújo, M. B., Bell, J. D., Blanchard, J., Bonebrake, T. C., Chen, I.-C., Clark, T. D., Colwell, R. K., Danielsen, F., Evengård, B., Falconi, L., Ferrier, S., Frusher, S., Garcia, R. A., Griffis, R. B., Hobday, A. J., Janion-Scheepers, C., Jarzyna, M. A., Jennings, S., Lenoir, J., Linnetved, H. I., Martin, V. Y., McCormack, P. C., McDonald, J., Mitchell, N. J., Mustonen, T., Pandolfi, J. M., Pettorelli, N., Popova, E., Robinson, S. A., Scheffers, B. R., Shaw, J. D., Sorte, C. J. B., Strugnell, J. M., Sunday, J. M., Tuanmu, M.-N., Vergés, A., Villanueva, C., Wernberg, T., Wapstra, E., and Williams, S. E.: Biodiversity redistribution under climate change: Impacts on ecosystems and human well-being, Science, 355, eaai9214, https://doi.org/10.1126/science.aai9214, 2017.
Poutanen, E.-L. and Nikkilä, K.: Carotenoid Pigments as Tracers of Cyanobacterial Blooms in Recent and Post-glacial Sediments of the Baltic Sea, Ambi, 30, 179–183, https://doi.org/10.1579/0044-7447-30.4.179, 2001.
Prentice, I. C., Williams, S., and Friedlingstein, P.: Biosphere feedbacks and climate change, Grantham Institute Briefing paper no. 12, Imperial College London, 1–20, 2015.
Ptacnik, R., Solimini, A. G., Andersen, T., Tamminen, T., Brettum, P., Lepistö, L., Willén, E., and Rekolainen, S.: Diversity predicts stability and resource use efficiency in natural phytoplankton communities, P. Natl. Acad. Sci. USA, 105, 5134–5138, https://doi.org/10.1073/pnas.0708328105, 2008.
Quesada, A., Moreno, E., Carrasco, D., Paniagua, T., Wormer, L., Hoyos, C. de, and Sukenik, A.: Toxicity of Aphanizomenon ovalisporum (Cyanobacteria) in a Spanish water reservoir, Eur. J. Phycol., 41, 39–45, https://doi.org/10.1080/09670260500480926, 2006.
Renaud, P. E., Løkken, T. S., Jørgensen, L. L., Berge, J., and Johnson, B. J.: Macroalgal detritus and food-web subsidies along an Arctic fjord depth-gradient, Front. Mar. Sci., 2, 31, https://doi.org/10.3389/fmars.2015.00031, 2015.
Repavich, W. M., Sonzogni, W. C., Standridge, J. H., Wedepohl, R. E., and Meisner, L. F.: Cyanobacteria (blue-green algae) in Wisconsin waters: acute and chronic toxicity, Water Res., 24, 225–231, https://doi.org/10.1016/0043-1354(90)90107-H, 1990.
Reusch, T. B. H., Dierking, J., Andersson, H. C., Bonsdorff, E., Carstensen, J., Casini, M., Czajkowski, M., Hasler, B., Hinsby, K., Hyytiäinen, K., Johannesson, K., Jomaa, S., Jormalainen, V., Kuosa, H., Kurland, S., Laikre, L., MacKenzie, B. R., Margonski, P., Melzner, F., Oesterwind, D., Ojaveer, H., Refsgaard, J. C., Sandström, A., Schwarz, G., Tonderski, K., Winder, M., and Zandersen, M.: The Baltic Sea as a time machine for the future coastal ocean, Sci. Adv., 4, eaar8195, https://doi.org/10.1126/sciadv.aar8195, 2018.
Richardson, A. J. and Schoeman, D. S.: Climate Impact on Plankton Ecosystems in the Northeast Atlantic, Science, 305, 1609–1612, https://doi.org/10.1126/science.1100958, 2004.
Sallée, J.-B., Pellichero, V., Akhoudas, C., Pauthenet, E., Vignes, L., Schmidtko, S., Garabato, A. N., Sutherland, P., and Kuusela, M.: Summertime increases in upper-ocean stratification and mixed-layer depth, Nature, 591, 592–598, https://doi.org/10.1038/s41586-021-03303-x, 2021.
Sauterey, B. and Ward, B. A.: Environmental control of marine phytoplankton stoichiometry in the North Atlantic Ocean, P. Natl. Acad. Sci. USA, 119, e2114602118, https://doi.org/10.1073/pnas.2114602118, 2022.
Sauterey, B., Ward, B., Rault, J., Bowler, C., and Claessen, D.: The Implications of Eco-Evolutionary Processes for the Emergence of Marine Plankton Community Biogeography, Am. Nat., 190, 116–130, https://doi.org/10.1086/692067, 2017.
Schindler, D. W., Hecky, R. E., Findlay, D. L., Stainton, M. P., Parker, B. R., Paterson, M. J., Beaty, K. G., Lyng, M., and Kasian, S. E. M.: Eutrophication of lakes cannot be controlled by reducing nitrogen input: Results of a 37-year whole-ecosystem experiment, P. Natl. Acad. Sci. USA, 105, 11254–11258, https://doi.org/10.1073/pnas.0805108105, 2008.
Smith, S. L., Pahlow, M., Merico, A., Acevedo-Trejos, E., Sasai, Y., Yoshikawa, C., Sasaoka, K., Fujiki, T., Matsumoto, K., and Honda, M. C.: Flexible phytoplankton functional type (FlexPFT) model: size-scaling of traits and optimal growth, J. Plankton Res., 38, 977–992, https://doi.org/10.1093/plankt/fbv038, 2016a.
Smith, S. L., Vallina, S. M., and Merico, A.: Phytoplankton size-diversity mediates an emergent trade-off in ecosystem functioning for rare versus frequent disturbances, Sci. Rep., 6, 34170, https://doi.org/10.1038/srep34170, 2016b.
Stal, L. J., Albertano, P., Bergman, B., Bröckel, K. von, Gallon, J. R., Hayes, P. K., Sivonen, K., and Walsby, A. E.: BASIC: Baltic Sea cyanobacteria. An investigation of the structure and dynamics of water blooms of cyanobacteria in the Baltic Sea – responses to a changing environment, Cont. Shelf Res., 23, 1695–1714, https://doi.org/10.1016/j.csr.2003.06.001, 2003.
Suikkanen, S., Laamanen, M., and Huttunen, M.: Long-term changes in summer phytoplankton communities of the open northern Baltic Sea, Estuar. Coast. Shelf Sci., 71, 580–592, https://doi.org/10.1016/j.ecss.2006.09.004, 2007.
Tallberg, P. and Heiskanen, A.-S.: Species-specific phytoplankton sedimentation in relation to primary production along an inshore – offshore gradient in the Baltic Sea, J. Plankton Res., 20, 2053–2070, https://doi.org/10.1093/plankt/20.11.2053, 1998.
Tamelander, T., Spilling, K., and Winder, M.: Organic matter export to the seafloor in the Baltic Sea: Drivers of change and future projections, Ambio, 46, 842–851, https://doi.org/10.1007/s13280-017-0930-x, 2017.
Terseleer, N., Bruggeman, J., Lancelot, C., and Gypens, N.: Trait-based representation of diatom functional diversity in a plankton functional type model of the eutrophied southern North Sea, Limnol. Oceanogr., 59, 1958–1972, https://doi.org/10.4319/lo.2014.59.6.1958, 2014.
Toseland, A., Daines, S. J., Clark, J. R., Kirkham, A., Strauss, J., Uhlig, C., Lenton, T. M., Valentin, K., Pearson, G. A., Moulton, V., and Mock, T.: The impact of temperature on marine phytoplankton resource allocation and metabolism, Nat. Clim. Change, 3, 979–984, https://doi.org/10.1038/nclimate1989, 2013.
Ward, B. A., Dutkiewicz, S., Jahn, O., and Follows, M. J.: A size-structured food-web model for the global ocean, Limnol. Oceanogr., 57, 1877–1891, https://doi.org/10.4319/lo.2012.57.6.1877, 2012.
Ward, B. A., Marañón, E., Sauterey, B., Rault, J., and Claessen, D.: The Size Dependence of Phytoplankton Growth Rates: A Trade-Off between Nutrient Uptake and Metabolism, Am. Nat., 189, 170–177, https://doi.org/10.1086/689992, 2017.
Ward, B. A., Collins, S., Dutkiewicz, S., Gibbs, S., Bown, P., Ridgwell, A., Sauterey, B., Wilson, J. D., and Oschlies, A.: Considering the Role of Adaptive Evolution in Models of the Ocean and Climate System, J. Adv. Model. Earth Sy., 11, 3343–3361, https://doi.org/10.1029/2018MS001452, 2019.
Wasmund, N., Voss, M., and Lochte, K.: Evidence of nitrogen fixation by non-heterocystous cyanobacteria in the Baltic Sea and re-calculation of a budget of nitrogen fixation, Mar. Ecol. Prog. Ser., 214, 1–14, https://doi.org/10.3354/meps214001, 2001.
Wasmund, N., Nausch, G., Gerth, M., Busch, S., Burmeister, C., Hansen, R., and Sadkowiak, B.: Extension of the growing season of phytoplankton in the western Baltic Sea in response to climate change, Mar. Ecol. Prog. Ser., 622, 1–16, https://doi.org/10.3354/meps12994, 2019.
Weatherdon, L. V., Magnan, A. K., Rogers, A. D., Sumaila, U. R., and Cheung, W. W. L.: Observed and Projected Impacts of Climate Change on Marine Fisheries, Aquaculture, Coastal Tourism, and Human Health: An Update, Front. Mar. Sci., 3, 48, https://doi.org/10.3389/fmars.2016.00048, 2016.
Winder, M. and Schindler, D. E.: Climate Change Uncouples Trophic Interactions in an Aquatic Ecosystem, Ecology, 85, 2100–2106, https://doi.org/10.1890/04-0151, 2004a.
Winder, M. and Schindler, D. E.: Climatic effects on the phenology of lake processes, Glob. Change Biol., 10, 1844–1856, https://doi.org/10.1111/j.1365-2486.2004.00849.x, 2004b.
Wirtz, K. W.: Mechanistic origins of variability in phytoplankton dynamics: Part I: niche formation revealed by a size-based model, Mar. Biol., 160, 2319–2335, https://doi.org/10.1007/s00227-012-2163-7, 2013.