the Creative Commons Attribution 4.0 License.
the Creative Commons Attribution 4.0 License.
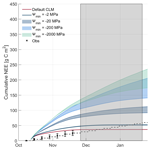
Simulating net ecosystem exchange under seasonal snow cover at an Arctic tundra site
Nick Rutter
Leanne Wake
Oliver Sonnentag
Gabriel Hould Gosselin
Melody Sandells
Chris Derksen
Branden Walker
Gesa Meyer
Richard Essery
Richard Kelly
Phillip Marsh
Julia Boike
Matteo Detto
Estimates of winter (snow-covered non-growing season) CO2 fluxes across the Arctic region vary by a factor of 3.5, with considerable variation between measured and simulated fluxes. Measurements of snow properties, soil temperatures, and net ecosystem exchange (NEE) at Trail Valley Creek, NWT, Canada, allowed for the evaluation of simulated winter NEE in a tundra environment with the Community Land Model (CLM5.0). Default CLM5.0 parameterisations did not adequately simulate winter NEE in this tundra environment, with near-zero NEE (< 0.01 ) simulated between November and mid-May. In contrast, measured NEE was broadly positive (indicating net CO2 release) from snow-cover onset until late April. Changes to the parameterisation of snow thermal conductivity, required to correct for a cold soil temperature bias, reduced the duration for which no NEE was simulated. Parameter sensitivity analysis revealed the critical role of the minimum soil moisture threshold of decomposition (Ψmin) in regulating winter soil respiration. The default value of this parameter (Ψmin) was too high, preventing simulation of soil respiration for the vast majority of the snow-covered season. In addition, the default rate of change of soil respiration with temperature (Q10) was too low, further contributing to poor model performance during winter. As Ψmin and Q10 had opposing effects on the magnitude of simulated winter soil respiration, larger negative values of Ψmin and larger positive values of Q10 are required to simulate wintertime NEE more adequately.
- Article
(924 KB) - Full-text XML
-
Supplement
(684 KB) - BibTeX
- EndNote
Although considerably more attention has been paid to Arctic CO2 fluxes during the growing season, winter (i.e. snow-covered non-growing season) CO2 emissions are now understood to make a significant contribution to annual carbon budgets in Arctic environments (e.g. Campbell, 2019; Natali et al., 2019; Rafat et al., 2021). The cumulative effect of winter emissions may even offset plant uptake of CO2 in the growing season, particularly as the climate warms (Belshe et al., 2013; Christiansen et al., 2012; Jeong et al., 2018), with the magnitude of non-growing season emissions likely to increase under climate change (Box et al., 2019; Commane et al., 2017; Watts et al., 2021). However, understanding of non-growing season CO2 fluxes is limited (Lüers et al., 2014). Cumulative wintertime CO2 fluxes across the Arctic region quantified by either terrestrial biosphere models (TBMs) or empirical estimates vary by a factor of 3.5 (i.e. 377–1301 Tg of carbon; Natali et al., 2019).
Uncertainties in process representation and parameterisation of TBM simulations of carbon fluxes limit our ability to assess and predict future changes (Braghiere et al., 2023; Treharne et al., 2022), particularly shifts in the timing and duration of the non-growing season. The representation of biogeochemical cycles in TBMs is subject to a high degree of parametric uncertainty (Fisher et al., 2019), with non-growing season processes and mechanisms poorly represented (Larson et al., 2021). Model intercomparison studies show large differences between individual predictions, with uncertainty in many aspects of the Arctic carbon cycle greater than the absolute magnitude of carbon fluxes (Fisher et al., 2014). Variability in carbon flux estimates between models is particularly prevalent during the winter (Fisher et al., 2014) and fluxes in the early winter shoulder season are likely underestimated (Commane et al., 2017). Improving (or even just including) the representation and influence of snow, soil, and biogeochemical non-growing season processes in TBMs will potentially improve our understanding of carbon dynamics and projections of Arctic climate change (Campbell and Laudon, 2019).
Mechanisms of non-growing season soil respiration, particularly the impact of environmental controls on heterotrophic respiration in subfreezing soils, are poorly represented in models, leading to large uncertainties (Tao et al., 2021). Here we summarise the limitations of TBMs in simulating CO2 fluxes in the non-growing season under three main groupings. Firstly, poor simulation of early-winter respiration in many TBMs is possibly linked to underestimation of soil temperature (Commane et al., 2017). During the non-growing season, belowground thermal processes become disconnected from the aboveground energy balance due to the insulation provided by nascent snow cover. This problem continues to impact soils throughout the entire snow-covered period. Such cold biases in wintertime soil temperature can be mitigated with a change in the parameterisation of snow thermal conductivity (Dutch et al., 2022; Royer et al., 2021) because the stratigraphic and hence insulative properties of Arctic snowpacks are not well simulated (Barrere et al., 2017; Domine et al., 2019). Decreasing snow thermal conductivity, which increases near-surface soil temperatures, has been found to increase simulated non-growing season net ecosystem exchange (NEE), with winter emissions more than doubling in the TBM LPJ-GUESS after the addition of a multi-layer snow scheme with temporally evolving snow properties (Pongracz et al., 2021).
Secondly, the empirical formulae used by many TBMs to model relationships between soil temperature, moisture, and soil respiration are often derived from data sets which undersample or do not include high-latitude regions (Bonan, 2019). For example, the temperature sensitivity of soil respiration is typically described with the use of a single, globally averaged Q10 value, representing the proportional change in respiration with a 10 ∘C rise in soil temperature (Lloyd and Taylor, 1994). However, Q10 is likely temperature dependent (Hamdi et al., 2013; Lloyd and Taylor, 1994; Kirschbaum, 1995) and may also be influenced by other environmental conditions such as soil moisture, texture, and plant community composition (Chen et al., 2020; Curiel Yuste et al., 2004; Meyer et al., 2018), although Mahecha et al. (2010) suggest otherwise. As a result, observed Q10 values from studies of Arctic ecosystems are typically larger than globally averaged values, with the synthesis of Chen et al. (2020) finding a median Q10 for tundra ecosystems (5.4) that is approximately double that of their global median (2.3). However, differences between Arctic and global Q10 values are not reflected in Arctic climate simulations, with approximately half of the 11 models investigated by Huntzinger et al. (2020) using Q10 values that are only half the size of observed values. Similar limitations also apply to the empirical relationships between soil moisture and respiration used by TBMs. The forms of these relationships (often parameterised using soil water potential, Ψ) used in many TBMs are derived from small-scale studies which do not account for respiration from frozen soils (Andrén and Paustian, 1987; Orchard and Cook, 1983). Relationships between soil moisture and respiration are also likely to be influenced by other soil properties, such as bulk density, texture, and carbon content, with different relationships observed for mineral and organic soils (Moyano et al., 2012). Interactions between temperature, moisture, and respiration suggest that these properties should be considered together when working to improve our understanding of CO2 fluxes.
Finally, biases in NEE, where simulated NEE is lower than measured NEE, have previously been noted in CLM5.0 (Community Land Model) in Arctic environments (Birch et al., 2021; Wieder et al., 2019) and other earth system models due to model underestimates of CO2 uptake by Arctic vegetation (Rogers et al., 2017). While this is particularly pertinent to growing season simulations, this can also impact the “shoulder seasons” of snow-cover onset and snowmelt within the non-growing season as CLM5.0 has limited skill in reproducing the timing of key phenological events, such as leaf onset and senescence (Birch et al., 2021).
As much of the Arctic tundra is covered in snow for up to 10 months of the year (Olsson et al., 2003), it is important to accurately simulate non-growing season carbon emissions under snow-covered conditions to better quantify annual carbon budgets. In our previous study (Dutch et al., 2022) examining the parameterisation of snow thermal conductivity in the Community Land Model version 5 (CLM5.0) at Trail Valley Creek (TVC), NWT, Canada, we found a cold soil temperature bias of ∼ 6 ∘C, and we suggested this bias may impact the simulation of NEE during the snow-covered non-growing season. TVC is an ideal type-site for much of the Arctic tundra, having been intensively studied and used to characterise the hydrology of tundra regions since the mid-1990s (e.g. Marsh et al., 2008; Pomeroy et al., 1993; Quinton and Marsh, 1999). In this study, we assess whether the default parameterisation of CLM5.0 accurately simulates carbon fluxes (NEE) during the snow-covered non-growing season at TVC. We evaluate the impact on the simulation of NEE due to the parameterisation of the following:
-
snow thermal conductivity (Keff),
-
the relationship between soil moisture and soil decomposition (Ψmin),
-
the rate of change of soil respiration as a function of soil temperature (Q10).
The overall aim is to compare simulations of soil respiration and NEE to eddy covariance (EC; Baldocchi, 2003) measurements for three snow-covered non-growing seasons and consider how to parameterise the model better in Arctic tundra environments on both sub-seasonal timescales and cumulatively throughout the snow-covered non-growing season.
2.1 Study site and data
Model evaluation was undertaken with data from Trail Valley Creek (68∘45′ N, 133∘30′ W), a mineral upland tundra site in the Inuvialuit Settlement Region, northeast of Inuvik, NWT, Canada. Mean annual air temperature at TVC was −7.9 ∘C for the period 1999–2018 (Grünberg et al., 2020), with typical maximum snow depths of < 50 cm (King et al., 2018). Precipitation was measured using a Geonor T-200B weighing gauge with an Alter-style wind screen and corrected as per Pan et al. (2016), as gauge undercatch is common in these types of environments (Smith, 2008; Watson et al., 2008; Gray and Male, 1981). Daily precipitation totals were disaggregated to hourly time steps, based on the fraction of daily precipitation at each hourly time step from ERA5 reanalysis data (Hersbach et al., 2020). Air temperature and relative humidity were measured at 2 m using a temperature and humidity sensor (Vaisala HMP35CF, Vaisala Oyj, Helsinki, Finland). Short-wave and long-wave radiation were measured at a height of 4.08 m using Kipp & Zonen CNR1 and CNR4 net radiometers (Kipp & Zonen, Delft, the Netherlands). Wind speed and direction were measured at 6.1 m using an R.M. Young 05103-10 wind monitor (R.M. Young, Traverse City, Michigan, USA). Discontinuous radiation measurements between January 2013 and December 2019 were gap-filled following Essery et al. (2016); gaps of 4 h or less were filled by linear interpolation, whereas longer gaps used ERA5 reanalysis data (Hersbach et al., 2020).
Measurements of NEE from the TVC EC tower (Helbig et al., 2016; Martin et al., 2022) were compared with model simulations. Measured half-hourly CO2 fluxes were calculated from wind speeds measured by a Campbell Scientific CSAT3 sonic anemometer, and CO2 concentrations were measured by an EC150 open-path CO2 H2O infrared gas analyser at a frequency of 10 Hz at a height of 4.08 m above the ground. Net CO2 fluxes are presented as NEE; we follow the micro-meteorological convention where release to the atmosphere is positive NEE and net uptake of CO2 by the land surface is negative NEE.
Non-growing season NEE measurements are presented as a comparison data set to simulated NEE, primarily to assess the direction (positive or negative) of CO2 fluxes and broad seasonal trends rather than absolute magnitudes. A cautious interpretation of measured NEE is prudent due to the difficulties in operation of open-path infrared gas analysers in Arctic winter climates (Amiro, 2010; Goulden et al., 2006; Jentzsch et al., 2021a, 2021b), frequent power failures common to meteorological stations in remote areas without line power, and low signal-to-noise ratios in post-processing flux corrections. Processing of EC measurements followed the pipeline described in Helbig et al. (2017):
-
remove spikes in high-frequency time series (Vickers and Mahrt, 1997),
-
correct sonic temperatures for humidity effects (van Dijk et al., 2004),
-
correct sonic anemometer tilt using a double rotation,
-
calculate half hourly fluxes (EddyPro v6.0+, LI-COR Biosciences),
-
apply the Webb–Pearman–Luening (WPL) correction,
-
fill gaps in the NEE time series where possible (Reichstein et al., 2005).
Data quality, identified using a “QWPL” flag (Jentzsch et al., 2021a), and availability (gap-filled and non-gap-filled) are presented in Sect. 1 of the Supplement (including Figs. S1 and S2 therein). The final processed and gap-filled NEE half-hourly time series are presented as weekly averages throughout the non-growing season with uncertainties calculated as standard deviations of residuals from the gap-filling algorithm (Lasslop et al., 2008).
2.2 Model description
CLM5.0 (Lawrence et al., 2019) is a community-developed land surface model, which includes biogeophysics, the carbon cycle, and vegetation dynamics as a TBM, within the overall framework of the Community Earth System Model (CESM; Danabasoglu et al., 2020). CLM5.0 can be run at a range of spatial scales, from a 1D point to grid cells across the entire earth surface. Recent developments relevant to modelling Arctic biogeochemical cycling include new representations of snow and soil hydrology and changes to carbon allocation schemes (Lawrence et al., 2019).
CLM5.0 describes tundra environments using a C3 arctic grass plant functional type (PFT) (Schädel et al., 2018), with land cover data generated at a 0.5 ∘C resolution (Lawrence and Chase, 2007). However, for 1D simulations at TVC, we prescribed land cover distribution as 60 % C3 arctic grass, 33 % broadleaf deciduous boreal shrub PFTs, and 7 % bare ground in line with ground-based species counts within the TVC EC footprint (Carolina Voigt, personal communication, 2021).
CLM5.0 uses a vertically resolved CENTURY-type soil decomposition scheme as outlined in Koven et al. (2013). Cryoturbation, the mixing of soil material due to freeze–thaw processes, was switched on for these simulations and model spin-up. The maximum depth for cryoturbation was set to 1 m, in line with observations of active layer thickness at this site (Wilcox et al., 2019). The parameterisation of soil freezing in CLM is given in Yang et al. (2018). For each layer (j) of the 20 biogeochemically active soil layers (the upper 8.5 m of the soil column), carbon moves through three soil pools with different default turnover times. The default turnover time (K0) of each of these pools is modified by the rate of decomposition:
where rT, rW, rO, and rZ are rate modifiers applied to each pool in each layer, which scale the rate of decomposition (Kj) depending on the soil layer temperature, soil moisture, oxygen content, and depth, respectively. In this study, we focus on the soil decomposition rate modifiers rT (temperature) and rW (moisture), which are explained in more detail below. The influence of temperature on decomposition is parameterised using a Q10 function for both frozen and unfrozen soils:
where Q10 defines the temperature sensitivity of soil respiration, Tj equals the temperature of soil layer j, and Tref is a reference temperature with a default value of 25 ∘C. By default, CLM5.0 uses a globally constant Q10 of 1.5 (Foereid et al., 2014) for both frozen and unfrozen soils (Lawrence et al., 2018).
The scalar for the impact of soil moisture on decomposition takes the form described by Andrén and Paustian (1987):
where Ψj is the soil water potential in soil layer j, and Ψmin and Ψmax are the upper and lower limits (with default values of −2 and −0.002 MPa, respectively) for soil water potential to impact the rate of soil decomposition. When Ψ is a greater absolute value than Ψmax, a change in the moisture content of the soil has no impact on rates of carbon turnover. When Ψ is smaller than Ψmin, the soil moisture is too low for decomposition to be simulated. This is noted to be a major limitation on the respiration from frozen soils by Lawrence et al. (2018). Respiration of previously decomposed carbon may still occur when Ψ is less than Ψmin, up until the point where labile carbon stocks are depleted (Lawrence et al., 2018).
Parameterisation of effective snow thermal conductivity (Keff) in CLM5.0 is after Jordan (1991). A quadratic equation is used to infer the relationship between the density of the snow (calculated from the masses of ice and interstitial air) and the thermal conductivity of the snowpack. Other parameterisations of this relationship, typically using different constants in the same quadratic equation (Sturm et al., 1997; Calonne et al., 2019; Yen, 1981), were expanded upon for CLM5.0 in Dutch et al. (2022). These different constants have been calculated from snow samples from different environments, with the parameterisation of Sturm et al. (1997) derived from snowpacks in the Alaskan Arctic.
To simulate 1D processes at the TVC EC tower, CLM5.0 was run in point mode adjusting two gridded land surface parameterisations as per Dutch et al. (2022). In order to better represent 1D processes, the snow accumulation factor, a scaling factor which determines the likeliness of a sub-grid-cell area to become covered in snow after a snowfall event, was increased (Swenson and Lawrence, 2012) from 0.1 to 2.0, which is more representative of the binary nature of snow presence or absence at a point. Additionally, the standard deviation of elevation was set to 0.5 m after Malle et al. (2021), as without these changes small parts of the grid cell do not become snow covered until very late in the season. Soil sand, silt, and clay fractions (28 % sand, 36 % silt, and 36 % clay) were taken from the mineral soil texture data set (Bonan et al., 2002), and CLM5.0 default soil organic matter fractions (Hugelius et al., 2013) were also used.
2.3 Experiment setup
The sensitivity of simulated NEE was evaluated in comparison with measured NEE in response to changes in the model parameterisation of (1) snow thermal conductivity, (2) the relationship between soil moisture and soil decomposition (rw; Eq. 3), and (3) the relationship between soil respiration and soil temperature (rT; Eq. 2). Simulation sensitivity was evaluated over snow-cover dates simulated by CLM5.0 (9 October 2016–23 May 2017; 12 October 2017–30 May 2018; 24 September 2018–23 May 2019), which were always within a week of observed snow-cover onset and melt-out (Dutch et al., 2022). We compared two options for the parameterisation of effective snow thermal conductivity: that of Jordan (1991) as used by default in CLM5.0 and that of Sturm et al. (1997) which has been shown to improve soil temperature simulation in both CLM5.0 (Dutch et al., 2022) and other land surface models (Royer et al., 2021). Such an improvement likely occurs as the parameterisation of Sturm et al. (1997) was derived from Arctic snowpack measurements, whereas that of Jordan (1991) was based on the laboratory experiment of Yen (1962), which used sieved snow with a denser and more homogenous structure than observed in Arctic snowpacks.
We also adjusted the soil decomposition rate modifiers (rT and rW in Eq. 1), similar to the approach of Tao et al. (2021), sampling a broad range of values for the parameters Ψmin (for rw; Eq. 3) and Q10 (for rt; Eq. 2), as listed in Table 1. Values for Q10 sampled a wide range of measured Q10 from Arctic soils (based on Chen et al., 2020; Elberling, 2007; Elberling and Brandt, 2003; Grogan and Jonasson, 2005; Mikan et al., 2002; Schmidt et al., 2008), and values of Ψmin were based on Tao et al. (2021). We note that the more negative values (⪅ −200 MPa) of Ψmin used by Tao et al. (2021) and herein are unlikely to be physically representative (Liang et al., 2022).
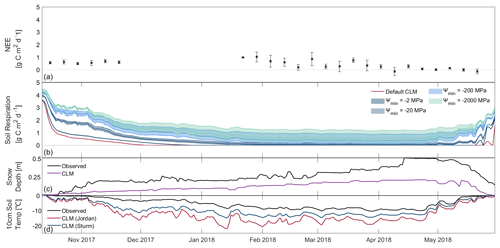
Figure 1(a) Mean (crosses) and uncertainty (as per Lasslop et al. (2008); error bars) of measured NEE at weekly intervals. (b) Simulated soil respiration. The default simulation (red) uses the Jordan (1991) parameterisation of snow thermal conductivity, and blue colours represent simulations using the Sturm et al. (1997) parameterisation of snow thermal conductivity. Darker blue colours represent less-negative Ψmin values and paler blue colours represent more-negative values of Ψmin. Shaded areas in panel (b) represent the range of respiration fluxes for simulations using the Sturm et al. (1997) snow thermal conductivity and the same Ψmin but with different values of Q10 (1.5, 2.5, 5.0, 7.5). (c) Observed (black) and simulated (purple) snow depths. (d) 10 cm soil temperatures, both observed (black) and simulated using both the default Jordan (1991; red) and Sturm et al. (1997; blue) snow thermal conductivity parameterisations.
In total, 32 model simulations were performed, perturbing Keff, Q10, and Ψmin simultaneously. Simultaneous perturbation of parameters avoided the one-at-a-time approach typical of many sensitivity analyses in order to examine the interaction between parameters (Gao et al., 2020) and evaluate their relative importance in improving wintertime carbon flux simulations. Simulations were spun up for 512 years, using 128 concatenated loops of 4 years (2013 to 2016) of meteorological forcing data. Spin-up was achieved once all three soil carbon pools in the decomposition scheme were in a steady state. Steady state was achieved when mean annual changes in the size of all three carbon pools for the last 10 years of the simulation were less than 10 g C m−3 and when the size of the soil carbon pools was within the range of observed values for the Mackenzie Delta region given in Fig. 1 of Schuur et al. (2015). CLM5.0 simulations were run for the period 2013–2019 but only evaluated from the onset of snow cover in 2016 due to the availability of coincident snow, soil, and eddy covariance measurements.
3.1 Measured NEE and soil temperatures
Measured NEE was broadly positive (with weekly NEE averages ranging from −0.1 to 1.1 ) throughout the snow-covered non-growing season, suggesting that CO2 was emitted from the ground at TVC throughout the winter (Figs. 1a and S3 in the Supplement). Measured mean NEE was positive until mid-April, at which point measured NEE followed an increasingly negative trend, indicating potential photosynthetic uptake. Soil freeze-up began with the onset of snowfall in October, with weekly mean 10 cm soil temperatures reaching a minimum value of −10.2 ∘C in early March. Soils began to warm as the snowpack melted, with observed weekly mean soil temperatures becoming positive in the second week of June (Fig. 1d). As considerably more NEE measurements were available for the snow-covered period of 2017–2018 than 2016–17 or 18–19 (Table S1 in the Supplement), we primarily focused on 2017–2018 when presenting measurements or comparing measured and simulated fluxes. However, cumulative simulated fluxes are presented for all three winters.
3.2 Simulated NEE
The default parameter configuration of CLM5.0 simulated negligible, near-zero NEE (all values below 0.01 ) between late November and mid-May in all three winters. CLM5.0 does not simulate gross primary productivity (GPP) during the entirety of the snow-covered season in all 3 winters. Autotrophic respiration is similarly negligible (all values below 0.01 ) in all simulations of the snow-covered non-growing season, regardless of parameter choices. As heterotrophic respiration, other than soil biota, is also not simulated during periods of snow cover, simulated NEE and soil respiration can be considered equivalent for simulations of snow-covered non-growing seasons.
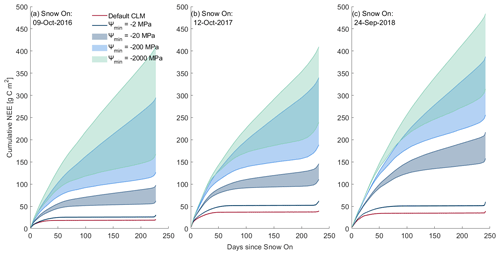
Figure 2Cumulative net ecosystem exchange (NEE) for the simulated snow cover duration of (a) 2016–17 (227 d), (b) 2017–18 (231 d), and (c) 2018–19 (242 d) from the ensemble of simulations. Blue colours represent simulations using the snow thermal conductivity parameterisation of Sturm et al. (1997), with darker colours for less-negative Ψmin. The shaded areas represent the range of Q10 (1.5–7.5) for each Ψmin. The dark red line represents the default CLM snow thermal conductivity parameterisation of Jordan (1991).
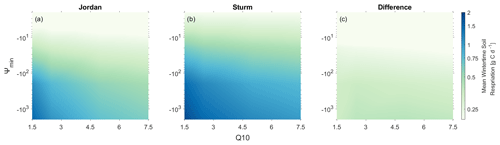
Figure 3Contour plots showing the relative influence of Ψmin and Q10 on the simulations of mean soil respiration for all three snow-covered non-growing seasons using the snow thermal conductivity parameterisations of (a) Jordan (1991) and (b) Sturm et al. (1997). The difference between the two snow thermal conductivity parameterisations is shown in (c)).
Sensitivity analysis of three parameters (Ψmin, Q10 and snow thermal conductivity) resulted in considerable variability in the simulated soil respiration and NEE over all three snow-covered periods (Figs. 1 and S3). Minimum total snow-covered non-growing season NEE was simulated for the default Ψmin (−2 MPa) and the default Jordan (1991) snow thermal conductivity parameterisation. For all years, simulated fluxes were greatest for a Q10 of 1.5, Ψmin of −2000 MPa, and the Sturm et al. (1997) snow thermal conductivity parameterisation. Simulated cumulative NEE varied by 370 g C m−2 across the different sets of parameter values (Fig. 2). This difference in cumulative simulated NEE was greater in years with earlier snow onset date, e.g. 2018–19, as this increased the duration of relatively warmer winter soils with higher respiration rates during freeze-up, in comparison with the total duration of colder soils throughout the non-growing season snow cover. In all three winters, simulations were most sensitive to chosen parameter values during the freeze-up period, with the range of soil respiration fluxes approximately double that in midwinter (Fig. 1). Simulated NEE decreased gradually from snow-cover onset until December–January, and it then remained at that level until late April when NEE increased as soils warm and snow melts.
Changes from Jordan (1991) to Sturm et al. (1997) representations of snow thermal conductivity delayed, by approximately a month (9 to 29 December; Fig. 1b.), the onset of moisture limitation for simulations with the default value of Ψmin, enabling the simulation of more positive NEE during freeze-up. The choice of snow thermal conductivity scheme significantly impacted simulations of mean winter soil respiration when considered throughout the total snow-covered non-growing season in all 3 years (Student's t test: t16–17 = −6.76, t17–18 = −8.01, t18–19 = −8.02, p < 0.001). Compared to the default Jordan (1991) parameterisation of snow thermal conductivity, the Sturm et al. (1997) parameterisation resulted in warmer near-surface soil (Fig. 1d) and hence more positive NEE, provided soil respiration had not become moisture limited. Although the cold soil temperature bias is reduced by two-thirds through the use of the Sturm parameterisation, we note that soil temperatures still remain lower than measured due to model underestimation of snow depth for the winter of 2017–18 (Fig. 1c and d.) Model sensitivity to Ψmin was lower for the Jordan (1991) snow thermal conductivity parameterisation (Fig. 3a) than for Sturm et al. (1997) (Fig. 3b); differences between parameterisations were greatest with a more negative Ψmin (Fig. 3c).
Simulated winter soil moisture potentials (Ψj; Eq. 2) had a typical value of approximately −15 MPa, lower than the default Ψmin (−2 MPa), preventing soil decomposition and respiration for the majority of the winter. Analysis of variance showed significant differences between simulated mean snow season soil respiration ( 19.45, F17–18 = 22.41, F18–19 = 23.80, p < 0.001) and cumulative snow season NEE (F16–17 = 19.47, F17–18 = 22.45, F18–19 = 23.86, p < 0.001; Fig. 2) for Ψmin of −2 and −2000 MPa, though differences between simulations with only 1 order of magnitude between their Ψmin values were not always deemed statistically significant (α = 0.001). Consequently, adjusting Ψmin had the largest impact on simulated fluxes, with larger negative Ψmin resulting in larger NEE.
Changes to Q10 had a smaller impact on simulated NEE than the parameterisation of Keff or Ψmin, with analysis of variance showing no significant difference between the mean snow season soil respiration for different Q10 values (Table 1) in all three winters. Differences in simulated cumulative snow season fluxes were also not statistically significant. Additionally, simulation sensitivity to frozen Q10 values (Schmidt et al., 2008) was tested. An extreme frozen Q10 of 300, after Schmidt et al. (2008), did not reduce the gap between simulated and measured NEE, with no appreciable difference between model runs where all other parameter choices were held constant.
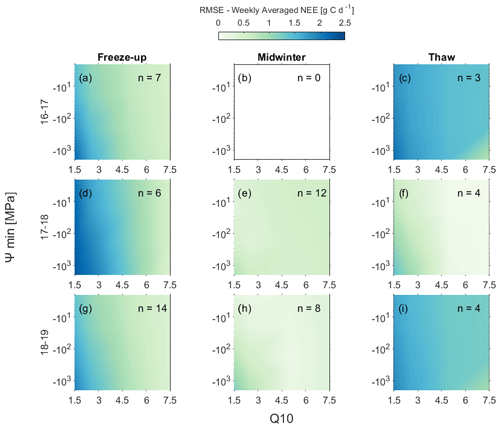
Figure 4Evaluation of the impact of Ψmin and Q10 parameterisations on simulated net ecosystem exchange (NEE) during freeze-up (a, d, and g), midwinter (b, e, and h), and thaw (c, f, and i) periods of each snow-covered season for simulations using the Sturm et al. (1997) snow thermal conductivity parameterisation. The number of weekly averages included in each panel are denoted by n values.
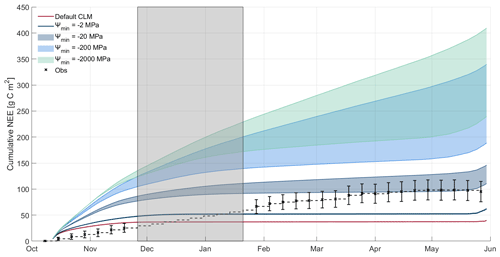
Figure 5Cumulative net ecosystem exchange (NEE) for winter 2017–18. The black crosses show cumulative weekly measured NEE, with error bars representing measurement uncertainty as per Lasslop et al. (2008). The grey area from late November to late January denotes the period when no NEE observations are available. Across this section, an average value for the 6 weeks before and after the gap is used to estimate cumulative NEE. Curves show the simulated cumulative NEE, with blue colours representing simulations using the snow thermal conductivity parameterisation of Sturm et al. (1997), with darker colours for less-negative Ψmin. The shaded areas for these curves represent the range of Q10 (1.5–7.5) for each Ψmin. The dark red line represents the default CLM snow thermal conductivity parameterisation of Jordan (1991).
Simulations with more-negative Ψmin (< −200 MPa) and higher Q10 (≥ 5) tended to have lower RMSE in comparison with measured weekly mean NEE (Fig. 4). As changes to Ψmin and Q10 had opposing impacts on the magnitude of simulated fluxes, different pairs of parameter values gave similar results. This counterbalancing effect strongly influences identification of an appropriate parameter space, e.g. simulations using a wide range of Ψmin with lower Q10 more greatly overestimated measured NEE during freeze-up and thaw than simulations with higher Q10 values (Fig. 4). Overestimation of simulated NEE particularly impacted cumulative NEE during freeze-up in 2017–18 (Fig. 5), with a reduction in December to mid-March NEE compensating for freeze-up overestimations; using mid-range values of Ψmin (−20 MPa) produced similar simulated and measured total cumulative non-growing season NEE.
3.3 Impact of soil properties on simulated respiration
Changes in the soil organic matter and texture profiles have a limited impact on simulated soil respiration and are unlikely to be the cause of the NEE bias seen with default parameterisations of CLM5.0. Table 2 outlines the results of six additional simulations where we changed the soil texture and soil organic matter content as given in Table S2 in the Supplement. All six additional simulations use the Sturm snow thermal conductivity parameterisation, a Ψmin value of −20, and a Q10 value of 2.5. Changes to the soil texture have a greater impact on simulated soil temperature than changes to the soil organic profile (Fig. S4 in the Supplement). The majority of the variability in NEE between simulations with different soil characteristics is restricted to the early snow season, highlighting the importance of the zero curtain period as a control on total seasonal NEE. Different soil organic matter contents lead to changes in NEE as a result of changes in soil insulative properties as opposed to any change to the supply of labile carbon for respiration. However, none of these potential sources of uncertainty lead to a variability in soil respiration or NEE greater than the uncertainty estimates of the eddy covariance observations; the impact of changes to the parameterisation of Ψmin, Q10, and snow thermal conductivity play a greater role in controlling the magnitude of simulated soil respiration during the snow-covered non-growing season.
4.1 NEE variability
The default parameterisation of CLM5.0 prevented simulation of soil respiration for most of the snow-covered non-growing season, leading to negligible simulated NEE, contrary to broadly positive patterns of measured NEE. Application of the Sturm et al. (1997) snow thermal conductivity parameterisation reduced simulated soil temperature biases (Dutch et al., 2022; Royer et al., 2021), which reduced the proportion of the snow-covered non-growing season for which simulated NEE was zero. Other TBMs have shown sensitivity of simulated NEE to snowpack representations, with improvements to the representation of the snowpack (including a multi-layer snowpack with variable, as opposed to prescribed, snow thermal conductivity) in LPJ-GUESS improving the simulation of wintertime NEE (Pongracz et al., 2021).
Cumulative snow-covered non-growing season NEE is not only dependent on parameterisation of snow thermal conductivity, but also on the timing of snow onset at the start of the winter. In 2018–19, when the snow-on date was 3 weeks earlier than the previous year, soils cooled more slowly due to thermal insulation against cold atmospheric air, leading to greater cumulative NEE. This was particularly evident for simulations using the Sturm thermal conductivity parameterisation, which better represents the early winter formation of low thermal conductivity basal snowpack depth hoar layers, providing greater insulation and warmer soil temperatures than the default snow thermal conductivity parameterisation used by CLM5.0. Interannual variability in snow conditions is reflected in simulated fluxes, further substantiating the importance of improving simulations of Arctic snowpacks. Biases and uncertainties in simulated snow mass (e.g. Kim et al., 2021; Mudryk et al., 2020) are likely to influence soil temperature (Dutch et al., 2022), heterotrophic respiration, and CO2 fluxes, particularly on regional scales (Tao et al., 2021). Improving the representation of snow and soil conditions or at least how these relate to respiration at the start of the snow-covered non-growing season is also important as this is likely to be the most biologically active part of the season with comparatively high rates of soil respiration (Commane et al., 2017; Olsson et al., 2003).
Simulated NEE increased considerably after the start of snowmelt, regardless of parameter choices, but was less rapid for simulations with larger negative Ψmin. Simulated NEE was most likely too positive at the end of the winter season due to delayed onset of simulated photosynthesis (Birch et al., 2021) and was not well matched to trends in measured NEE, which decreased from late April through May. Simulated gross primary productivity was zero for the entirety of the snow-covered period, but the pattern of decreasing measured NEE during thaw suggests that photosynthesis could be occurring before snow had completely melted out, which has been observed at similar Arctic locations (Finderup Nielsen et al., 2019; Larsen et al., 2007; Starr and Oberbauer, 2003). Additionally, production and emission of CO2 may become decoupled in frozen soils, with soil cracking with the deeper penetration of the freezing or thawing front potentially releasing stored CO2 produced when levels of respiration were higher earlier in the season; this process is not represented in CLM.
4.2 Parameterisation of soil moisture, temperature, and respiration
Of the three parameters investigated, Ψmin had the largest impact on the simulated snow-covered non-growing season NEE. Without changes to Ψmin, simulated soil moisture limits soil respiration, meaning simulated NEE was near-zero for the majority of the snow-covered non-growing season. Accurate simulation of a moisture threshold to soil respiration is important as moisture acts as a key control on soil respiration (Orchard and Cook, 1983), particularly in the shoulder season before snow onset (Liu et al., 2020) and in frozen soils (Öquist et al., 2009). Consequently, changes to soil moisture content have a strong influence on simulated soil respiration and wider carbon cycling (Chadburn et al., 2017). As soils cool from the surface down and thus become moisture limited from the surface down, a larger proportion of simulated soil respiration is likely to come from deeper soil layers later in the winter. CLM5.0 represents the limitation of respiration in frozen soils by an unavailability of liquid water (Lawrence et al., 2018), as shown by the strong dependence of simulated fluxes on Ψmin. However, CLM5.0 has known deficiencies in simulating soil moisture in high-altitude and high-latitude environments (Deng et al., 2020; 2021; Schädel et al., 2018), overestimating soil moisture when soils are frozen (Deng et al., 2020) and with soil heating leading to increased soil dryness, as opposed to observed increases in soil wetness (Schädel et al., 2018). Soil moisture biases may even have been exacerbated by model development, with Deng et al. (2020) finding a greater difference between simulations and observations for CLM5.0 than CLM4.5.
Even in frozen soils, liquid water can be present within the soil matrix (Hayashi, 2013), sustaining microbial activity and enabling respiration at temperatures well below 0 ∘C (Henry, 2007; Elberling & Brandt, 2003). Respiration is thought to continue down to −18 ∘C (Elberling and Brandt, 2003); however, in CLM5.0 respiration ceases when Ψmin exceeds Ψ, preventing respiration from being simulated at temperatures warmer than −18 ∘C. Recent findings from Liang et al. (2022) suggest that mineral soils should be able to respire below a Ψ value of −10 MPa, suggesting a Ψmin value below −10 MPa would be more physically representative than the current CLM5.0 default of −2 MPa. Tao et al. (2021) found that the E3SM default Ψmin of −10 MPa (a value 5 times greater in magnitude than the CLM5.0 default) prevented respiration from being simulated when soil temperatures were sub-zero and failed to allow for the accurate simulation of wintertime respiration in permafrost tundra environments, further highlighting the unsuitability of such a high Ψmin. A simpler approach, e.g. Yan et al. (2018), where respiration increases linearly from zero as soon as soil moisture is not zero (Chadburn et al., 2022) may produce more appropriate simulations of soil respiration in tundra environments than the commonly used thresholding approach of CLM5.0. The use of a Ψmin threshold may still be appropriate if decomposition does not automatically drop to zero when the threshold is reached; for example, the rw scalar in JULES drops to 0.2, not zero, when Ψ is lower than Ψmin (Burke et al., 2017), allowing for wintertime decomposition.
The impact of changing Q10 in CLM5.0 was lower than in other TBMs; smaller changes to Q10 had a larger influence on E3SM-simulated fluxes at similar Arctic tundra sites (Tao et al., 2021). At most negative Ψmin values, higher Q10 values were required to simulate soil respiration more accurately, similar to Tao et al. (2021), who found that a Q10 that was 66 % larger than the default of 1.5 led to improved simulations of wintertime soil respiration for sites in the Alaskan tundra. As observed Q10 changes with temperature, it may be more appropriate to generate Q10 at each time step as a function of soil temperature, an approach already undertaken in other TBMs such as CLASSIC (Melton and Arora, 2016; Wu et al., 2016). By using both soil moisture and soil temperature to parameterise Q10, Kim et al. (2019) found an improvement to negative ecosystem respiration biases compared to the use of a Q10 of 1.5 in global CLM4 simulations. However, Byun et al. (2021) state that standard Q10 functions fail when describing the relationship between temperature and CO2 production of frozen soils, and so the use of a Q10 function may not be the most appropriate way to model the relationship between soil respiration and temperature at sites such as TVC. Alternative parameterisations of rT (such as RothC; Jenkinson, 1990) may provide a more appropriate description of the relationship between temperature and soil respiration, as has been suggested for other TBMs such as JULES (Burke et al., 2017). This may not lead to improved model performance; Tao et al. (2021) tested non-Q10 parameterisations of the soil temperature–respiration relationship in the CLM-based E3SM and found that a Q10 parameterisation gave the best result for three of their four Alaskan Arctic sites. Although limited observational data of soil respiration limits the assessment of the suitability of Q10 functions (Kim et al., 2019), testing additional parameterisations (as opposed to just Q10) may give insight that could improve the simulation of NEE at Arctic tundra sites in CLM5.0.
The default parameterisation of CLM5.0 did not reproduce the broadly positive measured NEE during snow-covered non-growing seasons at our Arctic tundra site, despite widely documented midwinter CO2 emission at numerous sites across the Arctic tundra (Natali et al., 2019; Virkkala et al., 2021). An overly conservative moisture threshold limiting soil respiration in frozen soils was the most likely explanation for the lack of simulated soil respiration for the majority of the snow-covered non-growing season. Furthermore, the default parameterisation of CLM5.0 did not capture sub-seasonal patterns of measured NEE. Simulated NEE was too high towards the start of the snow-covered non-growing season, regardless of parameter values tested. Initial conditions at freeze-up are important in determining the magnitude of cumulative NEE for the entire snow-covered non-growing season, with changes to all parameters tested having the greatest impact at this time as the insulative capacity of the snow has not yet been reached.
Reducing soil temperature biases in CLM5.0 through a change to the parameterisation of snow thermal conductivity from Jordan (1991) to Sturm et al. (1997) increased the magnitude of simulated NEE during the snow-covered period. However, without improvement to the minimum soil moisture threshold, other parameter changes had very little impact on simulated NEE. The default Ψmin of −2 MPa was not appropriate for Arctic environments, with a 5 times larger negative Ψmin producing snow-covered non-growing season NEE more similar to measured NEE. Larger negative values of Ψmin were also tested but are likely to be physically implausible. Not only did the default parameterisation of Ψmin prevent wintertime respiration (poorly representing seasonal and annual carbon budgets and dynamics), but it may also have longer-term implications for the simulation of soil carbon turnover and the state of permafrost, limiting the reliability of longer-term climate simulations. Larger positive Q10 had an opposite impact on simulations than larger negative Ψmin, with larger Q10 depressing the magnitude of simulated NEE. Adjustments to both parameters in tandem provided the greatest improvement to simulated NEE, with larger negative Ψmin and larger positive Q10 simulating greater NEE during the snow-covered non-growing season.
Code and data to produce figures are available at https://github.com/V-Dutch/CLMWinterFlux_TVC (V-Dutch/CLMWinterFlux_TVC, 2024).
The supplement related to this article is available online at: https://doi.org/10.5194/bg-21-825-2024-supplement.
VRD, NR, LW, OS: investigation, formal analysis, writing (original draft preparation). NR, LW, MS, CD, RK: supervision. OS, GHG, BW, JB, MD: data acquisition. PM: data planning. LW: software. NR, OS, PM: funding acquisition. All authors were involved in reviewing and editing prior to submission.
The contact author has declared that none of the authors has any competing interests.
Publisher's note: Copernicus Publications remains neutral with regard to jurisdictional claims made in the text, published maps, institutional affiliations, or any other geographical representation in this paper. While Copernicus Publications makes every effort to include appropriate place names, the final responsibility lies with the authors.
Victoria R. Dutch was funded by an RDF Studentship from Northumbria University and the Northern Water Futures project. The eddy covariance and supporting measurements at Trail Valley Creek were funded through the Canada Foundation for Innovation, the Canada Research Chairs Program, and a Natural Sciences and Engineering Research Council of Canada Discovery Grant awarded to Oliver Sonnentag and Phillip Marsh.
This research has been supported by the Natural Environment Research Council (Carbon Emissions under Arctic Snow – grant no. NE/W003686/1).
This paper was edited by Kirsten Thonicke and reviewed by William Wieder and one anonymous referee.
Amiro, B.: Estimating annual carbon dioxide eddy fluxes using open-path analysers for cold forest sites, Agr. Forest Meteorol., 150, 1366–1372, https://doi.org/10.1016/j.agrformet.2010.06.007, 2010.
Andrén, O. and Paustian, K.: Barley Straw Decomposition in the Field: A Comparison of Models, Ecology, 68, 1190–1200, https://doi.org/10.2307/1939203, 1987.
Baldocchi, D. D.: Assessing the eddy covariance technique for evaluating carbon dioxide exchange rates of ecosystems: past, present and future, Glob. Change Biol., 9, 479–492, https://doi.org/10.1046/j.1365-2486.2003.00629.x, 2003.
Barrere, M., Domine, F., Decharme, B., Morin, S., Vionnet, V., and Lafaysse, M.: Evaluating the performance of coupled snow–soil models in SURFEXv8 to simulate the permafrost thermal regime at a high Arctic site, Geosci. Model Dev., 10, 3461–3479, https://doi.org/10.5194/gmd-10-3461-2017, 2017.
Belshe, E. F., Schuur, E. A., and Bolker, B. M.: Tundra ecosystems observed to be CO2 sources due to differential amplification of the carbon cycle, Ecol. Lett., 16, 1307–1315, https://doi.org/10.1111/ele.12164, 2013.
Birch, L., Schwalm, C. R., Natali, S., Lombardozzi, D., Keppel-Aleks, G., Watts, J., Lin, X., Zona, D., Oechel, W., Sachs, T., Black, T. A., and Rogers, B. M.: Addressing biases in Arctic–boreal carbon cycling in the Community Land Model Version 5, Geosci. Model Dev., 14, 3361–3382, https://doi.org/10.5194/gmd-14-3361-2021, 2021.
Bonan, G.: Climate Change and Terrestrial Ecosystem Modelling, Cambridge University Press, Cambridge, UK, , 437 pp., ISBN: 9-781107-619074, 2019.
Bonan, G. B., Levis, S., Kergoat, L., and Oleson, K. W.: Landscapes as patches of plant functional types: An integrating concept for climate and ecosystem models, Global Biogeochem. Cy., 16, 5-1–5-23, https://doi.org/10.1029/2000gb001360, 2002.
Box, J. E., Colgan, W. T., Christensen, T. R., Schmidt, N. M., Lund, M., Parmentier, F.-J. W., Brown, R., Bhatt, U. S., Euskirchen, E. S., Romanovsky, V. E., Walsh, J. E., Overland, J. E., Wang, M., Corell, R. W., Meier, W. N., Wouters, B., Mernild, S., Mård, J., Pawlak, J., and Olsen, M. S.: Key indicators of Arctic climate change: 1971–2017, Environ. Res. Lett., 14, 045010, https://doi.org/10.1088/1748-9326/aafc1b, 2019.
Braghiere, R. K., Fisher, J. B., Miner, K. R., Miller, C. E., Worden, J. R., Schimel, D. S., and Frankenberg, C.: Tipping point in North American Arctic-Boreal carbon sink persists in new generation Earth system models despite reduced uncertainty, Environ. Res. Lett., 18, 025008, https://doi.org/10.1088/1748-9326/acb226, 2023.
Burke, E. J., Chadburn, S. E., and Ekici, A.: A vertical representation of soil carbon in the JULES land surface scheme (vn4.3_permafrost) with a focus on permafrost regions, Geosci. Model Dev., 10, 959–975, https://doi.org/10.5194/gmd-10-959-2017, 2017.
Byun, E., Rezanezhad, F., Fairbairn, L., Slowinski, S., Basiliko, N., Price, J. S., Quinton, W. L., Roy-Leveillee, P., Webster, K., and Van Cappellen, P.: Temperature, moisture and freeze-thaw controls on CO2 production in soil incubations from northern peatlands, Sci. Rep.-UK, 11, 23219, https://doi.org/10.1038/s41598-021-02606-3, 2021.
Calonne, N., Milliancourt, L., Burr, A., Philip, A., Martin, C. L., Flin, F., and Geindreau, C.: Thermal Conductivity of Snow, Firn, and Porous Ice From 3-D Image-Based Computations, Geophys. Res. Lett., 46, 13079–13089, https://doi.org/10.1029/2019gl085228, 2019.
Campbell, J. L.: Arctic Loses Carbon as Winters Wane, Nat. Clim. Change, 9, 806–807, https://doi.org/10.1038/s41558-019-0606-6, 2019.
Campbell, J. L. and Laudon, H.: Carbon response to changing winter conditions in northern regions: current understanding and emerging research needs, Environ. Rev., 27, 545–566, https://doi.org/10.1139/er-2018-0097, 2019.
Chadburn, S. E., Krinner, G., Porada, P., Bartsch, A., Beer, C., Belelli Marchesini, L., Boike, J., Ekici, A., Elberling, B., Friborg, T., Hugelius, G., Johansson, M., Kuhry, P., Kutzbach, L., Langer, M., Lund, M., Parmentier, F.-J. W., Peng, S., Van Huissteden, K., Wang, T., Westermann, S., Zhu, D., and Burke, E. J.: Carbon stocks and fluxes in the high latitudes: using site-level data to evaluate Earth system models, Biogeosciences, 14, 5143–5169, https://doi.org/10.5194/bg-14-5143-2017, 2017.
Chadburn, S. E., Burke, E. J., Gallego-Sala, A. V., Smith, N. D., Bret-Harte, M. S., Charman, D. J., Drewer, J., Edgar, C. W., Euskirchen, E. S., Fortuniak, K., Gao, Y., Nakhavali, M., Pawlak, W., Schuur, E. A. G., and Westermann, S.: A new approach to simulate peat accumulation, degradation and stability in a global land surface scheme (JULES vn5.8_accumulate_soil) for northern and temperate peatlands, Geosci. Model Dev., 15, 1633–1657, https://doi.org/10.5194/gmd-15-1633-2022, 2022.
Chen, S., Wang, J., Zhang, T., and Hu, Z.: Climatic, soil, and vegetation controls of the temperature sensitivity (Q10) of soil respiration across terrestrial biomes, Global Ecology and Conservation, 22, e00955, https://doi.org/10.1016/j.gecco.2020.e00955, 2020.
Christiansen, C. T., Schmidt, N. M., and Michelsen, A.: High Arctic Dry Heath CO2 Exchange During the Early Cold Season, Ecosystems, 15, 1083–1092, https://doi.org/10.1007/s10021-012-9569-4, 2012.
Commane, R., Lindaas, J., Benmergui, J., Luus, K. A., Chang, R. Y., Daube, B. C., Euskirchen, E. S., Henderson, J. M., Karion, A., Miller, J. B., Miller, S. M., Parazoo, N. C., Randerson, J. T., Sweeney, C., Tans, P., Thoning, K., Veraverbeke, S., Miller, C. E., and Wofsy, S. C.: Carbon dioxide sources from Alaska driven by increasing early winter respiration from Arctic tundra, P. Natl. Acad. Sci. USA, 114, 5361–5366, https://doi.org/10.1073/pnas.1618567114, 2017.
Curiel Yuste, J., Janssens, I. A., Carrara, A., and Ceulemans, R.: Annual Q10 of soil respiration reflects plant phenological patterns as well as temperature sensitivity, Glob. Change Biol., 10, 161–169, https://doi.org/10.1111/j.1529-8817.2003.00727.x, 2004.
Danabasoglu, G., Lamarque, J. F., Bacmeister, J., Bailey, D. A., DuVivier, A. K., Edwards, J., Emmons, L. K., Fasullo, J., Garcia, R., Gettelman, A., Hannay, C., Holland, M. M., Large, W. G., Lauritzen, P. H., Lawrence, D. M., Lenaerts, J. T. M., Lindsay, K., Lipscomb, W. H., Mills, M. J., Neale, R., Oleson, K. W., Otto-Bliesner, B., Phillips, A. S., Sacks, W., Tilmes, S., Kampenhout, L., Vertenstein, M., Bertini, A., Dennis, J., Deser, C., Fischer, C., Fox-Kemper, B., Kay, J. E., Kinnison, D., Kushner, P. J., Larson, V. E., Long, M. C., Mickelson, S., Moore, J. K., Nienhouse, E., Polvani, L., Rasch, P. J., and Strand, W. G.: The Community Earth System Model Version 2 (CESM2), J. Adv. Model. Earth Sy., 12, e2019MS001916, https://doi.org/10.1029/2019ms001916, 2020.
Deng, M., Meng, X., Lyv, Y., Zhao, L., Li, Z., Jing, H., and Hu, Z.: Comparison of Soil Water and Heat Transfer Modeling Over the Tibetan Plateau Using Two Community Land Surface Model (CLM) Versions, J. Adv. Model. Earth Sy., 12, e2020MS002189, https://doi.org/10.1029/2020MS002189, 2020.
Deng, M., Meng, X., Lu, Y., Li, Z., Zhao, L., Hu, Z., Chen, H., Shang, L., Wang, S., and Li, Q.: Impact and Sensitivity Analysis of Soil Water and Heat Transfer Parameterizations in Community Land Surface Model on the Tibetan Plateau, J. Adv. Model. Earth Sy., 13, e2021MS002670, https://doi.org/10.1029/2021ms002670, 2021.
Domine, F., Picard, G., Morin, S., Barrere, M., Madore, J.-B., and Langlois, A.: Major Issues in Simulating Some Arctic Snowpack Properties Using Current Detailed Snow Physics Models: Consequences for the Thermal Regime and Water Budget of Permafrost, J. Adv. Model. Earth Sy., 11, 34–44, https://doi.org/10.1029/2018ms001445, 2019.
Dutch, V. R., Rutter, N., Wake, L., Sandells, M., Derksen, C., Walker, B., Hould Gosselin, G., Sonnentag, O., Essery, R., Kelly, R., Marsh, P., King, J., and Boike, J.: Impact of measured and simulated tundra snowpack properties on heat transfer, The Cryosphere, 16, 4201–4222, https://doi.org/10.5194/tc-16-4201-2022, 2022.
Elberling, B.: Annual soil CO2 effluxes in the High Arctic: The role of snow thickness and vegetation type, Soil Biol. Biochem., 39, 646–654, https://doi.org/10.1016/j.soilbio.2006.09.017, 2007.
Elberling, B. and Brandt, K. K.: Uncoupling of microbial CO2 production and release in frozen soil and its implications for field studies of arctic C cycling, Soil Biol. Biochem., 35, 263–272, https://doi.org/10.1016/s0038-0717(02)00258-4, 2003.
Essery, R., Kontu, A., Lemmetyinen, J., Dumont, M., and Ménard, C. B.: A 7-year dataset for driving and evaluating snow models at an Arctic site (Sodankylä, Finland), Geosci. Instrum. Method. Data Syst., 5, 219–227, https://doi.org/10.5194/gi-5-219-2016, 2016.
Finderup Nielsen, T., Ravn, N. R., and Michelsen, A.: Increased CO2 efflux due to long-term experimental summer warming and litter input in subarctic tundra – CO2 fluxes at snowmelt, in growing season, fall and winter, Plant Soil, 444, 365–382, https://doi.org/10.1007/s11104-019-04282-9, 2019.
Fisher, J. B., Sikka, M., Oechel, W. C., Huntzinger, D. N., Melton, J. R., Koven, C. D., Ahlström, A., Arain, M. A., Baker, I., Chen, J. M., Ciais, P., Davidson, C., Dietze, M., El-Masri, B., Hayes, D., Huntingford, C., Jain, A. K., Levy, P. E., Lomas, M. R., Poulter, B., Price, D., Sahoo, A. K., Schaefer, K., Tian, H., Tomelleri, E., Verbeeck, H., Viovy, N., Wania, R., Zeng, N., and Miller, C. E.: Carbon cycle uncertainty in the Alaskan Arctic, Biogeosciences, 11, 4271–4288, https://doi.org/10.5194/bg-11-4271-2014, 2014.
Fisher, R. A., Wieder, W. R., Sanderson, B. M., Koven, C. D., Oleson, K. W., Xu, C., Fisher, J. B., Shi, M., Walker, A. P., and Lawrence, D. M.: Parametric Controls on Vegetation Responses to Biogeochemical Forcing in the CLM5, J. Adv. Model. Earth Sy., 11, 2879–2895, https://doi.org/10.1029/2019ms001609, 2019.
Foereid, B., Ward, D. S., Mahowald, N., Paterson, E., and Lehmann, J.: The sensitivity of carbon turnover in the Community Land Model to modified assumptions about soil processes, Earth Syst. Dynam., 5, 211–221, https://doi.org/10.5194/esd-5-211-2014, 2014.
Gao, X., Avramov, A., Saikawa, E., and Schlosser, C. A.: Emulation of Community Land Model Version 5 (CLM5) to Quantify Sensitivity of Soil Moisture to Uncertain Parameters, https://globalchange.mit.edu/sites/default/files/MITJPSPGC_Rpt341.pdf (last access: 1 February 2024), 2020.
Goulden, M. L., Winston, G. C., McMillan, A. M. S., Litvak, M. E., Read, E. L., Rocha, A. V., and Rob Elliot, J.: An eddy covariance mesonet to measure the effect of forest age on land-atmosphere exchange, Glob. Change Biol., 12, 2146–2162, https://doi.org/10.1111/j.1365-2486.2006.01251.x, 2006.
Gray, D. M. and Male, D. H.: Handbook of Snow: Principles, Processes, Management & Use, Blackburn Press, Caldwell, New Jersey, ISBN: 0-08-025374-1, 1981.
Grogan, P. and Jonasson, S.: Temperature and substrate controls on intra-annual variation in ecosystem respiration in two subarctic vegetation types, Glob. Change Biol., 11, 465–475, https://doi.org/10.1111/j.1365-2486.2005.00912.x, 2005.
Grünberg, I., Wilcox, E. J., Zwieback, S., Marsh, P., and Boike, J.: Linking tundra vegetation, snow, soil temperature, and permafrost, Biogeosciences, 17, 4261–4279, https://doi.org/10.5194/bg-17-4261-2020, 2020.
Hamdi, S., Moyano, F., Sall, S., Bernoux, M., and Chevallier, T.: Synthesis analysis of the temperature sensitivity of soil respiration from laboratory studies in relation to incubation methods and soil conditions, Soil Biol. Biochem., 58, 115–126, https://doi.org/10.1016/j.soilbio.2012.11.012, 2013.
Hayashi, M.: The Cold Vadose Zone: Hydrological and Ecological Significance of Frozen-Soil Processes, Vadose Zone J., 12, 1–8, https://doi.org/10.2136/vzj2013.03.0064, 2013.
Helbig, M., Wischnewski, K., Gosselin, G. H., Biraud, S. C., Bogoev, I., Chan, W. S., Euskirchen, E. S., Glenn, A. J., Marsh, P. M., Quinton, W. L., and Sonnentag, O.: Addressing a systematic bias in carbon dioxide flux measurements with the EC150 and the IRGASON open-path gas analyzers, Agr. Forest Meteorol., 228–229, 349–359, https://doi.org/10.1016/j.agrformet.2016.07.018, 2016.
Helbig, M., Chasmer, L. E., Kljun, N., Quinton, W. L., Treat, C. C., and Sonnentag, O.: The positive net radiative greenhouse gas forcing of increasing methane emissions from a thawing boreal forest-wetland landscape, Glob. Chang. Biol., 23, 2413–2427, https://doi.org/10.1111/gcb.13520, 2017.
Henry, H. A. L.: Soil freeze–thaw cycle experiments: Trends, methodological weaknesses and suggested improvements, Soil Biol. Biochem., 39, 977–986, https://doi.org/10.1016/j.soilbio.2006.11.017, 2007.
Hersbach, H., Bell, B., Berrisford, P., Hirahara, S., Horányi, A., Muñoz-Sabater, J., Nicolas, J., Peubey, C., Radu, R., Schepers, D., Simmons, A., Soci, C., Abdalla, S., Abellan, X., Balsamo, G., Bechtold, P., Biavati, G., Bidlot, J., Bonavita, M., Chiara, G., Dahlgren, P., Dee, D., Diamantakis, M., Dragani, R., Flemming, J., Forbes, R., Fuentes, M., Geer, A., Haimberger, L., Healy, S., Hogan, R. J., Hólm, E., Janisková, M., Keeley, S., Laloyaux, P., Lopez, P., Lupu, C., Radnoti, G., Rosnay, P., Rozum, I., Vamborg, F., Villaume, S., and Thépaut, J. N.: The ERA5 global reanalysis, Q. J. Roy. Meteor. Soc., 146, 1999–2049, https://doi.org/10.1002/qj.3803, 2020.
Hugelius, G., Tarnocai, C., Broll, G., Canadell, J. G., Kuhry, P., and Swanson, D. K.: The Northern Circumpolar Soil Carbon Database: spatially distributed datasets of soil coverage and soil carbon storage in the northern permafrost regions, Earth Syst. Sci. Data, 5, 3–13, https://doi.org/10.5194/essd-5-3-2013, 2013.
Huntzinger, D. N., Schaefer, K., Schwalm, C., Fisher, J. B., Hayes, D., Stofferahn, E., Carey, J., Michalak, A. M., Wei, Y., Jain, A. K., Kolus, H., Mao, J., Poulter, B., Shi, X., Tang, J., and Tian, H.: Evaluation of simulated soil carbon dynamics in Arctic-Boreal ecosystems, Environ. Res. Lett., 15, 025005, https://doi.org/10.1088/1748-9326/ab6784, 2020.
Jenkinson, D. S.: The turnover of organic carbon and nitrogen in soil, Philos. T. R. Soc. B, 329, 361–368, https://doi.org/10.1098/rstb.1990.0177, 1990.
Jentzsch, K., Boike, J., and Foken, T.: Importance of the Webb, Pearman, and Leuning (WPL) correction for the measurement of small CO2 fluxes, Atmos. Meas. Tech., 14, 7291–7296, https://doi.org/10.5194/amt-14-7291-2021, 2021a.
Jentzsch, K., Schulz, A., Pirk, N., Foken, T., Crewell, S., and Boike, J.: High Levels of CO2 Exchange During Synoptic-Scale Events Introduce Large Uncertainty Into the Arctic Carbon Budget, Geophys. Res. Lett., 48, e2020GL092256, https://doi.org/10.1029/2020gl092256, 2021b.
Jeong, S.-J., Bloom, A. A., Schimel, D., Sweeney, C., Parazoo, N. C., Medvigy, D., Schaepman-Strub, G., Zheng, C., Schwalm, C. R., Huntzinger, D. N., Michalak, A. M., and Miller, C. E.: Accelerating rates of Arctic carbon cycling revealed by long-term atmospheric CO2 measurements, Science Advances, 4, eaao1167, https://doi.org/10.1126/sciadv.aao1167, 2018.
Jordan, R.: A One-Dimensional Temperature Model for a Snow Cover – Technical Documentation for SNTHERM.89, U.S Army Cold Regions Research and Engineering Laboratory, Hanover, New Hampshire, https://hdl.handle.net/11681/11677 (last access: 1 February 2024), 1991.
Kim, D., Lee, M.-I., and Seo, E.: Improvement of Soil Respiration Parameterization in a Dynamic Global Vegetation Model and Its Impact on the Simulation of Terrestrial Carbon Fluxes, J. Climate, 32, 127–143, https://doi.org/10.1175/JCLI-D-18-0018.1, 2019.
Kim, R. S., Kumar, S., Vuyovich, C., Houser, P., Lundquist, J., Mudryk, L., Durand, M., Barros, A., Kim, E. J., Forman, B. A., Gutmann, E. D., Wrzesien, M. L., Garnaud, C., Sandells, M., Marshall, H.-P., Cristea, N., Pflug, J. M., Johnston, J., Cao, Y., Mocko, D., and Wang, S.: Snow Ensemble Uncertainty Project (SEUP): quantification of snow water equivalent uncertainty across North America via ensemble land surface modeling, The Cryosphere, 15, 771–791, https://doi.org/10.5194/tc-15-771-2021, 2021.
King, J., Derksen, C., Toose, P., Langlois, A., Larsen, C., Lemmetyinen, J., Marsh, P., Montpetit, B., Roy, A., Rutter, N., and Sturm, M.: The influence of snow microstructure on dual-frequency radar measurements in a tundra environment, Remote Sens. Environ., 215, 242–254, https://doi.org/10.1016/j.rse.2018.05.028, 2018.
Kirschbaum, M. U. F.: The Temperature Dependance of Soil Organic Matter Decomposition, and the Effect of Global Warming on Soil Organic C Storage, Soil Biol. Biochem., 27, 753–760, https://doi.org/10.1016/0038-0717(94)00242-S, 1995.
Koven, C. D., Riley, W. J., Subin, Z. M., Tang, J. Y., Torn, M. S., Collins, W. D., Bonan, G. B., Lawrence, D. M., and Swenson, S. C.: The effect of vertically resolved soil biogeochemistry and alternate soil C and N models on C dynamics of CLM4, Biogeosciences, 10, 7109–7131, https://doi.org/10.5194/bg-10-7109-2013, 2013.
Larsen, K. S., Ibrom, A., Jonasson, S., Michelsen, A., and Beier, C.: Significance of cold-season respiration and photosynthesis in a subarctic heath ecosystem in Northern Sweden, Glob. Change Biol., 13, 1498–1508, https://doi.org/10.1111/j.1365-2486.2007.01370.x, 2007.
Larson, E. J. L., Schiferl, L. D., Commane, R., Munger, J. W., Trugman, A. T., Ise, T., Euskirchen, E. S., Wofsy, S., and Moorcroft, P. M.: The changing carbon balance of tundra ecosystems: results from a vertically-resolved peatland biosphere model, Environ. Res. Lett., 17, 014019, https://doi.org/10.1088/1748-9326/ac4070, 2021.
Lasslop, G., Reichstein, M., Kattge, J., and Papale, D.: Influences of observation errors in eddy flux data on inverse model parameter estimation, Biogeosciences, 5, 1311–1324, https://doi.org/10.5194/bg-5-1311-2008, 2008.
Lawrence, D. M., Fisher, R. A., Koven, C., Oleson, K., Swenson, S., Vertenstein, M., Andre, B., Bonan, G., Ghimire, B., van Kampenhout, L., Kennedy, D., Kluzek, E., Knox, R., Lawrence, P., Li, F., Li, H., Lombardozzi, D., Lu, Y., Perket, J., Riley, W. J., Sacks, W. J., Shi, M., Wieder, W. R., Xu, C., Ali, A. A., Badger, A. M., Bisht, G., Broxton, P. D., Brunke, M. A., Buzan, J., Clark, M., Craig, T., Dahlin, K., Drewniak, B., Emmons, L., Fisher, J. B., Flanner, M., Gentine, P., Lenaerts, J., Levis, S., Leung, L. R., Lipscomb, W. H., Pelletier, J. D., Ricciuto, D. M., Sanderson, B. M., Shuman, J., Slater, A., Subin, Z. M., Tang, J., Tawfik, A., Thomas, Q., Tilmes, S., Vitt, F., and Zeng, X.: Technical Description of version 5.0 of the Community Land Model (CLM), National Centre for Atmospheric Research, Boulder, Colorado, 2018.
Lawrence, D. M., Fisher, R. A., Koven, C. D., Oleson, K. W., Swenson, S. C., Bonan, G., Collier, N., Ghimire, B., Kampenhout, L., Kennedy, D., Kluzek, E., Lawrence, P. J., Li, F., Li, H., Lombardozzi, D., Riley, W. J., Sacks, W. J., Shi, M., Vertenstein, M., Wieder, W. R., Xu, C., Ali, A. A., Badger, A. M., Bisht, G., Broeke, M., Brunke, M. A., Burns, S. P., Buzan, J., Clark, M., Craig, A., Dahlin, K., Drewniak, B., Fisher, J. B., Flanner, M., Fox, A. M., Gentine, P., Hoffman, F., Keppel-Aleks, G., Knox, R., Kumar, S., Lenaerts, J., Leung, L. R., Lipscomb, W. H., Lu, Y., Pandey, A., Pelletier, J. D., Perket, J., Randerson, J. T., Ricciuto, D. M., Sanderson, B. M., Slater, A., Subin, Z. M., Tang, J., Thomas, R. Q., Val Martin, M., and Zeng, X.: The Community Land Model Version 5: Description of New Features, Benchmarking, and Impact of Forcing Uncertainty, J. Adv. Model. Earth Sy., 11, 4245–4287, https://doi.org/10.1029/2018ms001583, 2019.
Lawrence, P. J. and Chase, T. N.: Representing a new MODIS consistent land surface in the Community Land Model (CLM 3.0), J. Geophys. Res., 112, G01023, https://doi.org/10.1029/2006jg000168, 2007.
Liang, J., Chen, K., Siqintana, Huo, T., Zhang, Y., Jing, J., and Feng, W.: Towards improved modeling of SOC decomposition: soil water potential beyond the wilting point, Glob. Chang. Biol., 28, 3665–3673, https://doi.org/10.1111/gcb.16127, 2022.
Liu, Z., Kimball, J. S., Parazoo, N. C., Ballantyne, A. P., Wang, W. J., Madani, N., Pan, C. G., Watts, J. D., Reichle, R. H., Sonnentag, O., Marsh, P., Hurkuck, M., Helbig, M., Quinton, W. L., Zona, D., Ueyama, M., Kobayashi, H., and Euskirchen, E. S.: Increased high-latitude photosynthetic carbon gain offset by respiration carbon loss during an anomalous warm winter to spring transition, Glob. Chang. Biol., 26, 682–696, https://doi.org/10.1111/gcb.14863, 2020.
Lloyd, J. and Taylor, J. A.: On the temperature dependence of soil respiration, Funct. Ecol., 8, 315–323, 1994.
Lüers, J., Westermann, S., Piel, K., and Boike, J.: Annual CO2 budget and seasonal CO2 exchange signals at a high Arctic permafrost site on Spitsbergen, Svalbard archipelago, Biogeosciences, 11, 6307–6322, https://doi.org/10.5194/bg-11-6307-2014, 2014.
Mahecha, M. D., Reichstein, M., Carvalhais, N., Lasslop, G., Lange, H., Seneviratne, S. I., Vargas, R., Ammann, C., Arain, M. A., Cescatti, A., Janssens, I. A., Migliavacca, M., Montagnani, L., and Richardson, A. D.: Global convergence in the temperature sensitivity of respiration at ecosystem level, Science, 329, 838–840, https://doi.org/10.1126/science.1189587, 2010.
Malle, J., Rutter, N., Webster, C., Mazzotti, G., Wake, L., and Jonas, T.: Effect of Forest Canopy Structure on Wintertime Land Surface Albedo: Evaluating CLM5 Simulations With In-Situ Measurements, J. Geophys. Res.-Atmos., 126, e2020JD034118, https://doi.org/10.1029/2020jd034118, 2021.
Marsh, P., Pomeroy, J., Pohl, S., Quinton, W., Onclin, C., Russell, M., Neumann, N., Pietroniro, A., Davison, B., and McCartney, S.: Snowmelt Processes and Runoff at the Arctic Treeline: Ten Years of MAGS Research, in: Cold Region Atmospheric and Hydrologic Studies. The Mackenzie GEWEX Experience, edited by: Woo, M.-K., 2, Springer Berlin, Heidelberg, 97–123, https://doi.org/10.1007/978-3-540-75136-6_6, 2008.
Martin, M. R., Kumar, P., Sonnentag, O., and Marsh, P.: Thermodynamic basis for the demarcation of Arctic and alpine treelines, Sci. Rep.-UK, 12, 12565, https://doi.org/10.1038/s41598-022-16462-2, 2022.
Melton, J. R. and Arora, V. K.: Competition between plant functional types in the Canadian Terrestrial Ecosystem Model (CTEM) v. 2.0, Geosci. Model Dev., 9, 323–361, https://doi.org/10.5194/gmd-9-323-2016, 2016.
Meyer, N., Welp, G., and Amelung, W.: The Temperature Sensitivity (Q10) of Soil Respiration: Controlling Factors and Spatial Prediction at Regional Scale Based on Environmental Soil Classes, Global Biogeochem. Cy., 32, 306–323, https://doi.org/10.1002/2017gb005644, 2018.
Mikan, C. J., Schimel, J. P., and Doyle, A. P.: Temperature controls of microbial respiration in arctic tundra soils above and below freezing, Soil Biol. Biochem., 34, 1785–1795, https://doi.org/10.1016/s0038-0717(02)00168-2, 2002.
Moyano, F. E., Vasilyeva, N., Bouckaert, L., Cook, F., Craine, J., Curiel Yuste, J., Don, A., Epron, D., Formanek, P., Franzluebbers, A., Ilstedt, U., Kätterer, T., Orchard, V., Reichstein, M., Rey, A., Ruamps, L., Subke, J.-A., Thomsen, I. K., and Chenu, C.: The moisture response of soil heterotrophic respiration: interaction with soil properties, Biogeosciences, 9, 1173–1182, https://doi.org/10.5194/bg-9-1173-2012, 2012.
Mudryk, L., Santolaria-Otín, M., Krinner, G., Ménégoz, M., Derksen, C., Brutel-Vuilmet, C., Brady, M., and Essery, R.: Historical Northern Hemisphere snow cover trends and projected changes in the CMIP6 multi-model ensemble, The Cryosphere, 14, 2495–2514, https://doi.org/10.5194/tc-14-2495-2020, 2020.
Natali, S. M., Watts, J. D., Rogers, B. M., Potter, S., Ludwig, S. M., Selbmann, A.-K., Sullivan, P. F., Abbott, B. W., Arndt, K. A., Birch, L., Björkman, M. P., Bloom, A. A., Celis, G., Christensen, T. R., Christiansen, C. T., Commane, R., Cooper, E. J., Crill, P., Czimczik, C., Davydov, S., Du, J., Egan, J. E., Elberling, B., Euskirchen, E. S., Friborg, T., Genet, H., Göckede, M., Goodrich, J. P., Grogan, P., Helbig, M., Jafarov, E. E., Jastrow, J. D., Kalhori, A. A. M., Kim, Y., Kimball, J. S., Kutzbach, L., Lara, M. J., Larsen, K. S., Lee, B.-Y., Liu, Z., Loranty, M. M., Lund, M., Lupascu, M., Madani, N., Malhotra, A., Matamala, R., McFarland, J., McGuire, A. D., Michelsen, A., Minions, C., Oechel, W. C., Olefeldt, D., Parmentier, F.-J. W., Pirk, N., Poulter, B., Quinton, W., Rezanezhad, F., Risk, D., Sachs, T., Schaefer, K., Schmidt, N. M., Schuur, E. A. G., Semenchuk, P. R., Shaver, G., Sonnentag, O., Starr, G., Treat, C. C., Waldrop, M. P., Wang, Y., Welker, J., Wille, C., Xu, X., Zhang, Z., Zhuang, Q., and Zona, D.: Large loss of CO2 in winter observed across the northern permafrost region, Nat. Clim. Change, 9, 852–857, https://doi.org/10.1038/s41558-019-0592-8, 2019.
Olsson, P. Q., Sturm, M., Racine, C. H., Romanovsky, V., and Liston, G. E.: Five Stages of the Alaskan Arctic Cold Season with Ecosystem Implications, Arct. Antarct. Alp. Res., 35, 74–81, https://doi.org/10.1657/1523-0430(2003)035[0074:Fsotaa]2.0.Co;2, 2003.
Öquist, M. G., Sparrman, T., Klemedtsson, L., Drotz, S. H., Grip, H., Schleucher, J., and Nilsson, M.: Water availability controls microbial temperature responses in frozen soil CO2 production, Glob. Change Biol., 15, 2715–2722, https://doi.org/10.1111/j.1365-2486.2009.01898.x, 2009.
Orchard, V. A. and Cook, F. J.: Relationship Between Soil Respriation and Soil Moisture, Soil Biol. Biochem., 15, 447–453, https://doi.org/10.1016/0038-0717(83)90010-X, 1983.
Pan, X., Yang, D., Li, Y., Barr, A., Helgason, W., Hayashi, M., Marsh, P., Pomeroy, J., and Janowicz, R. J.: Bias corrections of precipitation measurements across experimental sites in different ecoclimatic regions of western Canada, The Cryosphere, 10, 2347–2360, https://doi.org/10.5194/tc-10-2347-2016, 2016.
Pomeroy, J., Marsh, P., and Lesack, L.: Relocation of Major Ions in Snow along the Tundra-Taiga Ecotone, Nord. Hydrol., 24, 151–168, https://doi.org/10.2166/nh.1993.0019, 1993.
Pongracz, A., Wårlind, D., Miller, P. A., and Parmentier, F.-J. W.: Model simulations of arctic biogeochemistry and permafrost extent are highly sensitive to the implemented snow scheme in LPJ-GUESS, Biogeosciences, 18, 5767–5787, https://doi.org/10.5194/bg-18-5767-2021, 2021.
Quinton, W. L. and Marsh, P.: A Conceptual Framework for Runoff Generation in a Permafrost Environment, Hydrol. Process., 13, 2563–2581, https://doi.org/10.1002/(SICI)1099-1085(199911)13:16<2563::AID-HYP942>3.0.CO;2-D, 1999.
Rafat, A., Rezanezhad, F., Quinton, W. L., Humphreys, E. R., Webster, K., and Van Cappellen, P.: Non-growing season carbon emissions in a northern peatland are projected to increase under global warming, Communications Earth & Environment, 2, 111, https://doi.org/10.1038/s43247-021-00184-w, 2021.
Reichstein, M., Falge, E., Baldocchi, D., Papale, D., Aubinet, M., Berbigier, P., Bernhofer, C., Buchmann, N., Gilmanov, T., Granier, A., Grunwald, T., Havrankova, K., Ilvesniemi, H., Janous, D., Knohl, A., Laurila, T., Lohila, A., Loustau, D., Matteucci, G., Meyers, T., Miglietta, F., Ourcival, J.-M., Pumpanen, J., Rambal, S., Rotenberg, E., Sanz, M., Tenhunen, J., Seufert, G., Vaccari, F., Vesala, T., Yakir, D., and Valentini, R.: On the separation of net ecosystem exchange into assimilation and ecosystem respiration: review and improved algorithm, Glob. Change Biol., 11, 1424–1439, https://doi.org/10.1111/j.1365-2486.2005.001002.x, 2005.
Rogers, A., Serbin, S. P., Ely, K. S., Sloan, V. L., and Wullschleger, S. D.: Terrestrial biosphere models underestimate photosynthetic capacity and CO2 assimilation in the Arctic, New Phytol., 216, 1090–1103, https://doi.org/10.1111/nph.14740, 2017.
Royer, A., Picard, G., Vargel, C., Langlois, A., Gouttevin, I., and Dumont, M.: Improved Simulation of Arctic Circumpolar Land Area Snow Properties and Soil Temperatures, Front. Earth Sci., 9, 658140, https://doi.org/10.3389/feart.2021.685140, 2021.
Schädel, C., Koven, C. D., Lawrence, D. M., Celis, G., Garnello, A. J., Hutchings, J., Mauritz, M., Natali, S. M., Pegoraro, E., Rodenhizer, H., Salmon, V. G., Taylor, M. A., Webb, E. E., Wieder, W. R., and Schuur, E. A. G.: Divergent patterns of experimental and model-derived permafrost ecosystem carbon dynamics in response to Arctic warming, Environ. Res. Lett., 13, 105002, https://doi.org/10.1088/1748-9326/aae0ff, 2018.
Schmidt, S. K., Wilson, K. L., Monson, R. K., and Lipson, D. A.: Exponential growth of “snow molds” at sub-zero temperatures: an explanation for high beneath-snow respiration rates and Q10 values, Biogeochemistry, 95, 13–21, https://doi.org/10.1007/s10533-008-9247-y, 2008.
Schuur, E. A., McGuire, A. D., Schadel, C., Grosse, G., Harden, J. W., Hayes, D. J., Hugelius, G., Koven, C. D., Kuhry, P., Lawrence, D. M., Natali, S. M., Olefeldt, D., Romanovsky, V. E., Schaefer, K., Turetsky, M. R., Treat, C. C., and Vonk, J. E.: Climate change and the permafrost carbon feedback, Nature, 520, 171–179, https://doi.org/10.1038/nature14338, 2015.
Smith, C. D.: Correcting the Wind Bias in Snowfall Measurements Made with a Geonor T-200B Precipitation Gauge and Alter Wind Shield, Bulletin of the Canadian and Oceanographic Meteorological Society, 36, 162–167, 2008.
Starr, G. and Oberbauer, S. F.: Photosynthesis of Arctic Evergreens under Snow: Implications for Tundra Ecosystem Carbon Balance, Ecology, 84, 1415–1420, https://doi.org/10.1890/02-3154, 2003.
Sturm, M., Holmgren, J., Konig, M., and Morris, K.: The thermal conductivity of seasonal snow, J. Glaciol., 43, 26–41, https://doi.org/10.3189/s0022143000002781, 1997.
Swenson, S. C. and Lawrence, D. M.: A new fractional snow-covered area parameterization for the Community Land Model and its effect on the surface energy balance, J. Geophys. Res.-Atmos., 117, D21107, https://doi.org/10.1029/2012jd018178, 2012.
Tao, J., Zhu, Q., Riley, W. J., and Neumann, R. B.: Improved ELMv1-ECA simulations of zero-curtain periods and cold-season CH4 and CO2 emissions at Alaskan Arctic tundra sites, The Cryosphere, 15, 5281–5307, https://doi.org/10.5194/tc-15-5281-2021, 2021.
Treharne, R., Rogers, B. M., Gasser, T., MacDonald, E., and Natali, S.: Identifying Barriers to Estimating Carbon Release From Interacting Feedbacks in a Warming Arctic, Frontiers in Climate, 3, 716464, https://doi.org/10.3389/fclim.2021.716464, 2022.
V-Dutch/CLMWinterFlux_TVC: V-Dutch/CLMWinterFlux_TVC, Zenodo [code and data set], https://doi.org/10.5281/zenodo.10605127, 2024.
van Dijk, A., Moene, A. F., and de Bruin, H. A. R.: The principles of surface flux physics: Theory, practice and description of the ECPACK library, Wageningen University, Wageningen, the Netherlands, 2004.
Vickers, D. and Mahrt, L.: Quality Control and Flux Sampling Problems for Tower and Aircraft Data, J. Atmos. Ocean. Tech., 14, 512–526, https://doi.org/10.1175/1520-0426(1997)014<0512:Qcafsp>2.0.Co;2, 1997.
Virkkala, A. M., Aalto, J., Rogers, B. M., Tagesson, T., Treat, C. C., Natali, S. M., Watts, J. D., Potter, S., Lehtonen, A., Mauritz, M., Schuur, E. A. G., Kochendorfer, J., Zona, D., Oechel, W., Kobayashi, H., Humphreys, E., Goeckede, M., Iwata, H., Lafleur, P. M., Euskirchen, E. S., Bokhorst, S., Marushchak, M., Martikainen, P. J., Elberling, B., Voigt, C., Biasi, C., Sonnentag, O., Parmentier, F. W., Ueyama, M., Celis, G., St Louis, V. L., Emmerton, C. A., Peichl, M., Chi, J., Jarveoja, J., Nilsson, M. B., Oberbauer, S. F., Torn, M. S., Park, S. J., Dolman, H., Mammarella, I., Chae, N., Poyatos, R., Lopez-Blanco, E., Christensen, T. R., Kwon, M. J., Sachs, T., Holl, D., and Luoto, M.: Statistical upscaling of ecosystem CO2 fluxes across the terrestrial tundra and boreal domain: Regional patterns and uncertainties, Glob. Change Biol., 27, 4040–4059, https://doi.org/10.1111/gcb.15659, 2021.
Watson, S., Smith, C. D., Lassi, M., and Misfeldt, J.: An Evaluation of the Effectiveness of the Double Alter Wind Shield for Increasing the Catch Efficiency of the Geonor T-200B Precipitation Gauge, Bulletin of the Canadian and Oceanographic Meteorological Society, 36, 168–175, 2008.
Watts, J., Natali, S. M., Minions, C., Risk, D., Arndt, K. A., Zona, D., Euskirchen, E. S., Rocha, A. V., Sonnentag, O., Helbig, M., Kalhori, A., Oechel, W. C., Ikawa, H., Ueyama, M., Suzuki, R., Kobayashi, H., Celis, G., Schuur, E. A. G., Humphreys, E. R., Kim, Y., Lee, B.-Y., Goetz, S. J., Madani, N., Schiferl, L., Commane, R., Kimball, J. S., Liu, Z., Torn, M. S., Potter, S., Wang, J. A., Jorgenson, T., Xiao, J., Li, X., and Edgar, C.: Soil respiration strongly offsets carbon uptake in Alaska and Northwest Canada, Environ. Res. Lett., 16, 084051, https://doi.org/10.1088/1748-9326/ac1222, 2021.
Wieder, W. R., Lawrence, D. M., Fisher, R. A., Bonan, G. B., Cheng, S. J., Goodale, C. L., Grandy, A. S., Koven, C. D., Lombardozzi, D. L., Oleson, K. W., and Thomas, R. Q.: Beyond Static Benchmarking: Using Experimental Manipulations to Evaluate Land Model Assumptions, Global Biogeochem. Cy., 33, 1289–1309, https://doi.org/10.1029/2018GB006141, 2019.
Wilcox, E. J., Keim, D., de Jong, T., Walker, B., Sonnentag, O., Sniderhan, A. E., Mann, P., and Marsh, P.: Tundra shrub expansion may amplify permafrost thaw by advancing snowmelt timing, Arctic Science, 5, 202–217, https://doi.org/10.1139/as-2018-0028, 2019.
Wu, Y., Verseghy, D. L., and Melton, J. R.: Integrating peatlands into the coupled Canadian Land Surface Scheme (CLASS) v3.6 and the Canadian Terrestrial Ecosystem Model (CTEM) v2.0, Geosci. Model Dev., 9, 2639–2663, https://doi.org/10.5194/gmd-9-2639-2016, 2016.
Yan, Z., Bond-Lamberty, B., Todd-Brown, K. E., Bailey, V. L., Li, S., Liu, C., and Liu, C.: A moisture function of soil heterotrophic respiration that incorporates microscale processes, Nat. Commun., 9, 2562, https://doi.org/10.1038/s41467-018-04971-6, 2018.
Yang, K., Wang, C., and Li, S.: Improved Simulation of Frozen-Thawing Process in Land Surface Model (CLM4.5), J. Geophys. Res.-Atmos., 123, 13238–13258, https://doi.org/10.1029/2017jd028260, 2018.
Yen, Y.-C.: Effective Thermal Conductivity of Ventilated Snow, J. Geophys. Res., 67, 1091–1098, https://doi.org/10.1029/JZ067i003p01091, 1962.
Yen, Y.-C.: Review of thermal properties of snow, ice and sea ice, U.S. Army Cold Regions Research and Engineering Laboratory, Hanover, New Hampshire, https://usace.contentdm.oclc.org/digital/api/collection/p266001coll1/id/7366/download (last access: 1 February 2024), 1981.