the Creative Commons Attribution 4.0 License.
the Creative Commons Attribution 4.0 License.
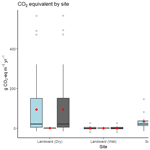
Dynamics of CO2 and CH4 fluxes in Red Sea mangrove soils
Jessica Breavington
Alexandra Steckbauer
Chuancheng Fu
Mongi Ennasri
Carlos M. Duarte
Red Sea mangroves have a lower carbon burial rate than the global average, whereby small greenhouse gas fluxes may offset a large proportion of carbon burial. Monthly soil core sampling was conducted across 2 years at two sites within a central eastern Red Sea mangrove stand to examine carbon dioxide (CO2) and methane (CH4) fluxes under dry and inundated conditions. Fluxes were highly variable, characterized by a prevalence of low emissions punctuated by bursts of high emissions. At the landward site, average ± SE (median) flux from the soil–air interface was 3111 ± 929 (811) µmol CO2 m−2 d−1 and 1.68 ± 0.63 (0.26) µmol CH4 m−2 d−1 under light conditions and 8657 ± 2269 (1615) µmol CO2 m−2 d−1 and 0.84 ± 0.79 (0.59) µmol CH4 m−2 d−1 under dark conditions. Average ± SE (median) sea–air fluxes were −55 ± 165 (−79) µmol CO2 m−2 d−1 and 0.12 ± 0.23 (0.08) µmol CH4 m−2 d−1 under light conditions and 27 ± 48 (53) µmol CO2 m−2 d−1 and 0.16 ± 0.13 (0.09) µmol CH4 m−2 d−1 in dark conditions. The seaward site recorded a higher CH4 flux, averaging 18.7 ± 8.18 (1.7) and 17.1 ± 4.55 (7.7) µmol CH4 m−2 d−1 in light and dark conditions. Mean fluxes offset 94.5 % of carbon burial, with a median of 4.9 % skewed by extreme variability. However, reported CO2 removal by total alkalinity emission from carbonate dissolution greatly exceeded both processes and drives the role of these ecosystems as intense CO2 sinks.
- Article
(1172 KB) - Full-text XML
-
Supplement
(654 KB) - BibTeX
- EndNote
Mangrove forests thrive in estuarine and intertidal zones within latitudes of 40° N to 40° S (Rosentreter et al., 2018a), storing a significant amount of organic carbon and providing numerous ecosystem services, including coastal protection and biodiversity enhancement (Curran et al., 2002; Howard et al., 2014). Mangroves offer a promising nature-based solution to mitigate global warming due to their high sequestration of soil organic carbon (Corg) while offering coastal protection to sea level rise (Duarte et al., 2013). Carbon preservation in mangrove soils is facilitated by waterlogged, anoxic conditions that limit the decay of organic matter (OM). However, as mangroves exist at the boundary between terrestrial and marine environments, the capacity for carbon sequestration varies depending on multiple factors such as the tidal range, sediment, and nutrient inputs.
Mangroves in the Red Sea are subject to extreme environmental conditions that restrict their growth and productivity. The Red Sea is one of the warmest and most saline seas globally, characterized by oligotrophic and nutrient-limited conditions. Moreover, central Saudi Arabia experiences extreme aridity, with an average annual precipitation of 60 mm (Gabr et al., 2017). Consequently, Avicennia marina is the dominant mangrove species in the Red Sea, existing at the threshold of its physiological tolerance. It is one of the most highly adapted mangrove species to the high salinity and aridity and found predominantly as monospecific mangrove stands (Khalil, 2015). Rhizophora mucronata is also found within the Red Sea but predominantly in southern regions where there is lower salinity (Khalil, 2015). Due to the absence of permanent rivers, mangroves in the Saudi Arabian Red Sea typically form narrow fringing bands along the coastline. In the central Red Sea, the distribution of mangroves is also constrained by the small tidal range, which is typically less than 1.5 m (Blanco-Sacristán et al., 2022). The conditions in the Red Sea result in reduced growth of A. marina with trees only reaching 2–3 m compared to over 16 m in Australia (Mackey, 1993). The oligotrophic conditions prevalent in the Red Sea result in stunted growth and dwarf forms of mangroves due to nutrient limitation (Almahasheer et al., 2016b). As a result, mangroves in the Red Sea have one of the lowest carbon sequestration rates, approximately 15 ± 1 g Corg m−2 yr−1, compared to a global average estimated at 163 g Corg m−2 yr−1 (Almahasheer et al., 2017; Breithaupt et al., 2012; Sanderman et al., 2018).
Greenhouse gas (GHG) fluxes, involving the release of carbon dioxide (CO2) or methane (CH4), in mangrove soils partially offset their role in removing atmospheric CO2, which is at its highest of the past 800 000 years (Tripati et al., 2009), contributing to radiative heating of the atmosphere and a global temperature increase at a rate of 1.7 °C per century since the beginning of the industrial revolution (Allen et al., 2014; Marcott et al., 2013). CH4 is the second-most-important GHG associated with climate change (Forster et al., 2007) and substantially more potent than CO2, with a global warming potential (GWP) approximately 28 times greater (Myhre et al., 2013). The low carbon sequestration rates of Red Sea mangroves may be offset by GHG fluxes. However, a lack of dynamic estimates or GHG fluxes from arid mangrove soils in the Red Sea precludes such an assessment. To date and to the best of our knowledge, only one other known study has provided estimates of GHG fluxes from mangrove soil in the Red Sea. These fluxes ranged from −3452 to 7500 µmol CO2 m−2 d−1 and from 0.9 to 13.3 µmol CH4 m−2 d−1 across different locations in the Red Sea (Sea et al., 2018). Therefore, it is difficult to reliably quantify the role of GHG emissions in offsetting CO2 removal by carbon sequestration in Red Sea mangrove soils, which is important for creating accurate carbon budgets for arid mangroves. Furthermore, GHG flux estimations exhibit wide variation due to factors such as location, environmental conditions, and study design.
Intertidal conditions in mangrove forests allow for flux measurements directly from the soil to the air (soil–air interface) or through the sea–air interface, with different transfer velocity equations introducing variability in the flux estimates (Akhand et al., 2021; Call et al., 2015). Additionally, flux measurements can be measured in situ or through controlled ex situ laboratory studies, with variations in chamber design, that can be closed or open with circulating air. Recent advancements in measurement technology, particularly with the growing use of cavity ring-down spectroscopy (CRDS), enable high-accuracy measurements even at low gas concentrations, but accurate comparison with other methods, such as eddy flux covariance, can be challenging (Brannon et al., 2016). Furthermore, environmental variables and physicochemical properties should be considered to comprehensively understand the variability of GHG emissions from mangrove soils. A comprehensive understanding of carbon stores and emissions in mangrove ecosystems is required to accurately determine the net climate benefits from mangrove coverage and restoration efforts (Lovelock et al., 2022). The Red Sea is one of the few regions where mangrove coverage has been steadily increasing over the past 4 decades, underscoring the importance of accurate carbon budgets for Red Sea mangroves (Almahasheer et al., 2016a).
Here, we quantify the dynamics of CO2 and CH4 fluxes from mangrove soils in a mangrove stand in the highly arid central Red Sea to assess the scale of soil carbon burial offset by GHG flux. We also test the effect of various physical and chemical soil properties on GHG fluxes. This study represents the first effort to simultaneously measure CO2 and CH4 fluxes from both the sea–air and soil–air interfaces in Red Sea mangroves over a time series relevant to providing needed insights into the dynamics of carbon cycling in this unique ecosystem.
2.1 Sampling locations
Sampling was conducted at two adjacent monospecific Avicenna marina mangrove stands in Thuwal, on the eastern coast of the central Red Sea (22.340787° N, 39.087991° E) (Fig. 1). Soil cores for CO2 and CH4 flux were collected over 2 years, from April 2021 to May 2023, on a monthly basis, except when this was prevented by logistical challenges. The first sampling location was approximately 150 m inland from the coast, referred to as the landward site, with an elevation approximately 0.75 m above sea level. This landward site was characterized by a strong seasonal microtidal influence, with a tidal range of less than 0.5 m, resulting in a scarce tidal inundation during the summer months and a more regular inundation during winter. The second site was located approximately 200 m from the landward site, referred to as the seaward site. Sampling of this second site was conducted over a narrower time window between September and October 2022: weekly sampling for 2 consecutive weeks, followed by a 2-week break to minimize disturbance to the site and allow for a greater range in temperature, and then resumed for another 2 weeks of weekly sampling, resulting in a total of four sampling events. This seaward site experienced continuous water coverage across the sampling period and, resultingly, was subject to fewer environmental extremes than the landward site.
2.2 Core collection
Two sets of cores were collected each month. The first set of cores comprised four large clear PVC cylinders (height: 30 cm, diameter: 9.6 cm) inserted into the soil to a depth of 10 cm and retrieved without disturbing the soil layers. During sampling, roots and undecomposed organic matter were avoided as the aim was to estimate GHG emissions from the soil rather than the mangrove trees. If water was present during sampling, it was retained within the cylinder up to a maximum depth of 10 cm to ensure a minimum of 10 cm of air for incubation and without disturbing the soil–water interface. Initially, in April, May, and July (2021), collection of the soil cores was conducted in the early morning hours allowing for sufficient time to transport and process soil cores with a stabilization period of 1 h between sealing the core and taking the T0 gas sample at 07:00 LT (following the protocol of Sea et al., 2018). Subsequent sampling events, until the study conclusion, were conducted late afternoon on the day before for logistical reasons, with the cores left unsealed in the incubator under darkness to mirror night-time conditions. Leaving the cores unsealed and undisturbed was to allow for regular gas exchange following disturbance of the soil caused by collection in the field and to avoid the creation of anoxic conditions in the sediment and water overnight before the start of the experiment. If water was present at the time of sample collection, extra water was sampled with the soil cores and also placed in the incubator to keep the temperature stable. On the morning of the start of the experiment, water was exchanged, and the air–water interface of the sealed cores was allowed to stabilize for 1 h before the collection of the T0 gas measurement at 07:00 LT (Sea et al., 2018). The 1 h stabilization was not required for cores without the water phase: there was no water to exchange and no water–soil interface to influence gas exchange dynamics. There were no significant differences in T0 concentrations with or without water.
The second set of cores (height: 9 cm, diameter: 2.5 cm) was collected immediately next to the large cores and used to assess the physical and chemical properties of the soil, including conductivity, total carbon (TC), total organic carbon (TOC), total nitrogen (TN), bulk density (BD), and water content (WC). Both sets of cores were transferred into a cooler and transported to the lab for processing within an hour after sampling. Temperature and tidal inundation at the site were continuously recorded via in situ temperature and water level loggers (U22-001 v2 and U20L-04, Onset Computer Corp., Massachusetts, USA).
2.3 Flux measurements
GHG flux was measured from the soil–air interface or the sea–air interface, depending on the presence of water at the time of sampling. The four replicate cores were immediately transported to the laboratory and placed in an incubator (I-30L, Percival, Geneva Scientific LLC, Fontana, Wisconsin, USA). Temperature was set to the average temperature in the field as determined by readings from in situ temperature loggers (U22-001 v2, Onset Computer Corp., Massachusetts, USA) over the past 72 h from the time of sampling. After sampling, an airtight lid was fitted to the bottom of the core, and opaque tape was wrapped around the outside of the core to cover the soil phase, to avoid light exposure to the sides of the soil. The top of each core was left unsealed and kept in the incubator overnight to equilibrate. Immediately before the onset of sampling, the tops of the cores were sealed with airtight lids. Three gas samples of 25 mL per core were taken starting at 07:00 LT (T0), after 12 h of light (T1), and the final sample (T2) after 12 h of darkness. For the duration of the light condition, incubator lights were set to 100 % intensity at 125 µmol m−2 s−1 irradiance (I-30L, Percival, Geneva Scientific LLC, Fontana, Wisconsin, USA). Gas samples were taken using a syringe and valve system. The syringes with gas samples were connected to a G2201-i CRDS analyser (Picarro, Santa Clara, California, USA), coupled to a Small Sample Introduction Module (SSIM2), to measure CO2, CH4, δ13C–CO2, and δ13C–CH4. CO2 and CH4 concentrations were converted from dry mole fractions in parts per million (ppm) to µmol m−2 d−1 (24 h) using the linear change in concentration between the 12 h sampling periods (Brannon et al., 2016; Tete et al., 2015) (Eq. 1).
where F is flux of CO2 or CH4 (µmol m−2); is the linear concentration change of CO2 or CH4 over 12 h from T0 to T1 to measure light fluxes or T1 to T2 to measure fluxes under dark conditions; P is the pressure (Pa) calculated using Boyle's law, which was used to correct the pressure in the headspace after taking 25 mL air at each sampling point; V is the volume of gas (m3) in the cylinder headspace; R is the ideal gas constant (8.314 J mol−1 K−1); A is the area of soil (m2); and T is temperature (K).
The CO2 equivalent (g CO2-eq m−2 yr−1) of the combined CO2 and CH4 fluxes was calculated for the flux across the sea–air and soil–air interfaces (Eqs. 2 and 3). Mangrove carbon storage was calculated using estimates from previous studies in the Red Sea, using 55 g CO2-eq m−2 yr−1 for the soil carbon burial rate (Almahasheer et al., 2017) and 1266 g CO2-eq m−2 yr−1 for CO2 uptake from total alkalinity (TA) enhancement determined at this site (Saderne et al., 2021).
where CO2 = 44 g mol−1, CH4 = 16 g mol−1, and CH4 is global warming potential (GWP) over a 100-year horizon = 28 (Allen et al., 2014).
2.4 Soil chemical and physical variables
The soil from the 10 cm incubated cores were dried at 60 °C to a constant weight to determine bulk density (BD 10 cm) and water content (WC 10 cm). The soil from the small cores was also dried at 60 °C to a constant weight to determine bulk density (BD 3 cm) and water content (WC 3 cm). After drying, the small cores were ground using an agate pestle and mortar for analysis of total carbon (TC), total organic carbon (TOC), total nitrogen (TN), and soil electrical conductivity (EC 1 : 5). For TOC, a 10 ± 1 mg sample was acidified with 5 µL of 3 mol HCL L−1 in silver capsules. Samples were dried for 30 min at 60 °C, and acidification was repeated a minimum of three times or until no bubbles were observed during the addition of HCL to remove all carbonates before being fully dried and wrapped in tin capsules for organic elemental analysis (Flash 2000, Thermo Fisher Scientific, Massachusetts, USA). Soil organic carbon (Corg) and inorganic carbon (Cinorg) for 0–3 cm soil depth were calculated using bulk density (Howard et al., 2014) and with the following formulas (Eqs. 4–6):
Conductivity was measured using an electrical conductivity (EC) sensor (InLab® 738 ISM, Mettler Toledo, Schwerzenbach, Switzerland). Prior to measurement the sensor was calibrated with 12.88 mS cm−1 potassium chloride as produced by the manufacturer (Mettler Toledo). For the surface soil, 5 ± 0.01 g of soil was used with 25 mL water for a 1 : 5 ratio of one part soil to five parts Milli-Q water. The samples were mixed on an orbital shaker (VWR©) following a typical protocol for the EC 1 : 5 method for high-salinity soils (Hardie and Doyle, 2012; Kargas et al., 2018).
2.5 Data analysis
Differences in soil properties and GHG flux between sampling sites and wet and dry conditions were evaluated for significance by means of a Mann–Whitney U test in RStudio (v.4.1.2). A correlation matrix showing significance between GHG fluxes, isotope signatures, soil properties, and environmental variables using Spearman rank correlation coefficient was created with the use of the “SciPy” package (v1.11.1) in Python (v3.11.5). In addition, a random forest algorithm was used to model the influence of environmental and temporal variables on CO2 flux in light and dark conditions through the use of regression trees utilizing bootstrapping techniques (Breiman, 2001). The models were built in Python v.3.9.13 and Jupyter Notebook v.6.4.12 using the RandomForestRegressor from the scikit-learn package v.1.0.2. Only data from the landward site were used in the models due to the greater number of observations and longer sampling period. A total of 80 % of data were randomly selected and used for training, with the remaining 20 % used for validation.
To optimize model accuracy and minimize overfitting, we utilized the R2 metric, which is an easy-to-interpret standardized measure of linear association (Fox et al., 2017), and implemented a 5-fold cross-validation to assess how the model generalizes to unseen data and reduces the risk of overfitting. Hyperparameter tuning for the number of trees, maximum depth, minimum sample split, and minimum sample leaf was utilized to maximize the R2 metric. Furthermore, a baseline accuracy threshold was defined for feature selection, where R2 ≥ 0.6 and the average 5-fold cross-validation (CV) score ≥ 0.4. Backward elimination of variables based on these performance metrics was systematically performed to maximize the number of variables included within each model without sacrificing model performance to ensure the maximum predictive power without overfitting (Genuer et al., 2010; Speiser et al., 2019). These models were used to map feature importance of the variables retained from the feature selection stage. All other figures were made with the use of “ggplot” in RStudio (v.4.1.2).
3.1 Soil properties
The most pronounced variation in soil characteristics between wet and dry sampling conditions at the landward site was observed in conductivity (EC), averaging 22.6 mS cm−1 under dry conditions compared to 9.25 mS cm−1 under wet conditions (Table 1), although all locations were classified under the “extreme” salinity class (Hardie and Doyle, 2012). EC and WC were the only soil properties to demonstrate significant differences (p < 0.001) under wet and dry sampling conditions at the landward site. The largest contrast between the two sampling sites was evident in the Cinorg concentration, with the seaward site exhibiting a significantly higher (p < 0.001) mean Cinorg (94.51 ± 3.37 mg C cm−3) compared to the landward site (66.64 ± 1.16 mg C cm−3) under wet and dry sampling conditions. Additionally, the seaward site had a lower Corg concentration, averaging 5.53 mg Corg cm−3 (0.34 % ± 0.017 %) compared to an average of 9.52 mg Corg cm−3 (0.72 % ± 0.021 %) at the landward site throughout the entire sampling period. Corg was significantly greater (p < 0.001) at the landward site under dry conditions, averaging 2.43 mg Corg cm−3 more than the seaward site. Under wet conditions, there was a smaller but still significant difference (p < 0.05) of 2.29 mg Corg cm−3 between the landward and seaward sites.
Table 1Average soil properties (± SE) for the top 3 cm of soil at the landward site in dry and wet sampling conditions and at the continually inundated seaward site. C : N denotes the C : N (molar ratio), Corg the organic carbon, Cinorg the inorganic carbon, WC the water content, and EC1:5 the electrical conductivity (1 : 5 soil : water ratio). Superscript letters indicate compact letter display (CLD). Different letters within individual columns indicate a significant difference in the mean between sampling sites or conditions (Mann–Whitney U test, p < 0.05). Conditions that share common letters demonstrate no significant difference.

3.2 Highly variable CO2 and CH4 fluxes
Between April 2021 to May 2023, 20 months were sampled at the landward site. Nine were under inundated conditions measuring flux from the sea–air interface, and 11 months of sampling were under dry conditions, measuring fluxes from the soil–air interface. Five months could not due sampled due to logistical issues. At the landward site the CO2 flux varied from −3136 µmol CO2 m−2 d−1 in the light condition to 37 644 µmol CO2 m−2 d−1 in the dark condition (Fig. 2). The average fluxes combined across the soil–air and sea–air interfaces were 1686 ± 546 µmol CO2 m−2 d−1 under the light conditions and 3 times larger, 4774 ± 1337 µmol CO2 m−2 d−1, under dark conditions (Table 2). The net daily flux over the full incubation period combining light and dark fluxes was 3178 ± 806 µmol CO2 m−2 d−1 (range: −811 to 28 048 µmol CO2 m−2 d−1). On average, the soil was a net source of CO2 to the atmosphere in all conditions except the light CO2 flux from the sea–air interface at the landward site (−55 µmol CO2 m−2 d−1).
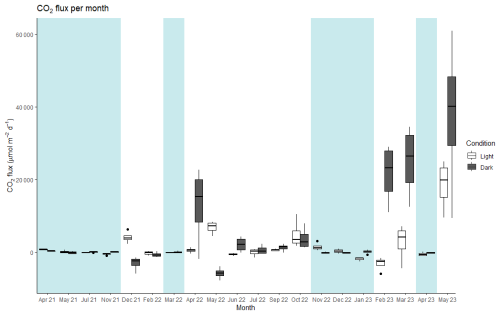
Figure 2Median values of CO2 flux for each month and condition (light and dark) at the landward site. The thick line inside the box represents the median value of the data, and 25th and 75th percentiles are denoted by the box ends. The whiskers extend to the minimum and maximum values within 1.5 times the interquartile range, and outliers are marked by black points. Blue shading: periods of net flux from the sea–air interface. No shading: periods of net flux from the soil air interface. Note that the axis label for the timescale is non-continuous, as months without sampling are not shown.
Table 2Summary of CO2 and CH4 fluxes and combined CO2-eq flux offset (using the total flux over the 24 h incubation) of carbon burial (C burial) and total alkalinity enhancement (TA) for the landward and seaward study sites (carbon burial data adapted from Almahasheer et al., 2017, and Saderne et al., 2021). Superscript letters indicate compact letter display (CLD). Different letters within individual columns indicate a significant difference in the mean between sampling sites or conditions (Mann–Whitney U test, p < 0.05). Conditions that share common letters demonstrate no significant difference. For example, in light CO2 flux, the soil-air mean (“bc”) is significantly different from the sea-air mean (“a”) but not significantly different from the combined mean (sharing “b”) or the seaward mean (sharing “c”).
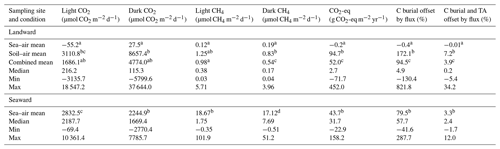
The average CH4 flux at the landward site was 0.98 ± 0.37 µmol CH4 m−2 d−1 under light conditions and 0.54 ± 0.44 µmol CH4 m−2 d−1 under dark conditions (Fig. 3). The net daily flux over the 24 h incubation period was 0.74 ± 0.23 µmol CH4 m−2 d−1 (range: −1.47 to 5.71 µmol CH4 m−2 d−1).
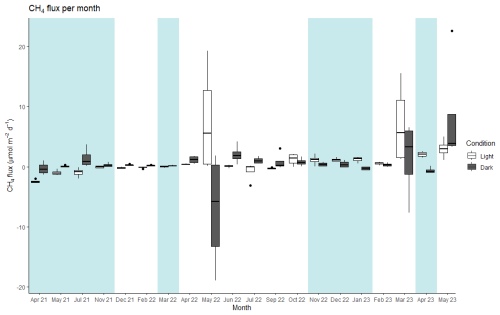
Figure 3Median values of CH4 flux for each month and condition (light and dark) at the landward site. The thick line inside the box represents the median value of the data, and 25th and 75th percentiles are denoted by the box ends. The whiskers extend to the minimum and maximum values within 1.5 times the interquartile range, and outliers are marked by black points. Blue shading: periods of net flux from the sea–air interface. No shading: periods of net flux from the soil air interface. Note that the axis label for the timescale is non-continuous, as months without sampling are not shown.
At the seaward site, only sea–air flux was measured given the constant inundation, and there was a lower CO2 flux compared to the overall mean CO2 flux from landward site (Table 2). However, there was a higher mean and median sea–air CO2 flux when compared with only the sea–air fluxes from the landward site (Fig. 4). CH4 flux was also significantly higher than that at the landward site (Table 2). The average flux was 18.67 and 17.12 µmol CH4 m−2 d−1 in light and dark conditions, respectively.
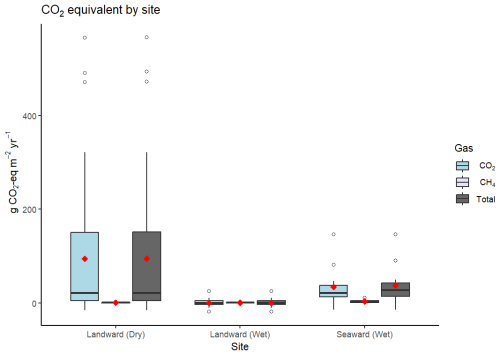
Figure 4Boxplot comparison of mean (red diamond), interquartile range (boxes), median (black line), and outliers (white circles) of CO2-eq flux across sites, with landward sites separated by dry and wet sampling conditions. “Total” shows the combined g CO2-eq m−2 yr−1 for both CO2 and CH4.
The isotopic signature of CO2 averaged −12.02 ± 0.14 ‰ at the landward site and −11.75 ± 0.46 ‰ at the seaward site. Despite the lighter isotope at the landward site, there was no significant difference in δ13C–CO2 between sites (Mann–Whitney U test, p = 0.0795). The isotopic signature of the CH4 averaged −46.24 ± 0.58 ‰ at the landward site and −48.18 ± 0.67 ‰ at the seaward site, with no significant difference in δ13C–CH4 between sites (Mann–Whitney U test, p = 0.3684). The δ13C signature of CO2 and CH4 did not change significantly across seasons. However, significant correlations (p > 0.05) were observed between core replicates and inorganic carbon (Cinorg) with δ13C–CO2, and well as between electrical conductivity (EC1:5) and δ13C–CH4 (Fig. S1 in the Supplement).
Fluxes were generally a net source of CO2-eq to the atmosphere (Table 2). Using a mean estimate, 95 % of soil carbon burial was offset by GHG flux at the landward site. However, the estimates were highly skewed so that the mean value does not represent the central tendency, which was best represented by the median flux. Median CO2-eq fluxes only offset 4.9 % of the carbon burial rate at the same site. When incorporating the CO2 drawdown of TA enhancement, 3.9 % (mean) and 0.2 % (median) of carbon sequestration potential was offset by the GHG fluxes measured in this study at the landward site (Table 2). At the seaward site, the greater flux and GWP of CH4 resulted in a greater median offset of carbon burial compared to the landward site, but the mean offset at the landward site remained higher due to the very large upper-range CO2 fluxes. Generally, the CO2-eq offset was significantly higher when fluxes were measured between the soil–air interface, compared to measurements between the sea–air interface (Fig. 4).
3.3 Drivers of flux variation
There were several significant correlations relating to GHG flux with environmental and soil properties. CO2 flux demonstrated a significant correlation with water volume under both conditions (light condition, p = 0.008; dark condition, p = 0.032) (Fig. S1). Light CH4 flux significantly correlated with temperature (p = 0.007) and water content (p = 0.009), while dark CH4 flux correlated with water content (p = 0.043) and electrical conductivity (p = 0.018) (Fig. S1).
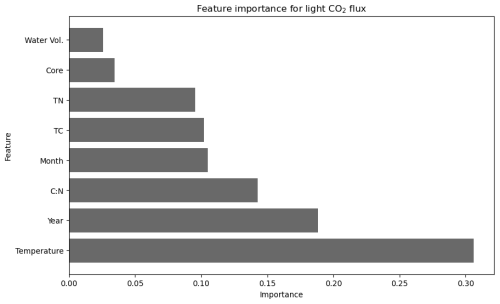
Figure 5Importance of environmental, temporal, and physicochemical variables in predicting CO2 flux under light conditions at the landward sampling site (R2 = 0.62, cross-validation average (n = 5) of 0.48 after feature engineering).
Random forest modelling for CO2 flux under light conditions yielded the maximum predictive power, with an R2 value of 0.62, when using only eight variables. Inclusion of any additional variables resulted in a model performance below the baseline threshold (R2 ≥ 0.6, CV score ≥ 0.4). Of the eight variables, temperature is the most important single variable in correctly predicting CO2 flux under light conditions with the feature importance of temperature exceeding 0.3, compared to all other variables in the model which have a feature importance below 0.2 (Fig. 5). Temporal variables (year and month) featured among the eight selected variables, with the year of sampling being the second-most-important variable in predicting CO2 flux under light conditions (0.19 importance).
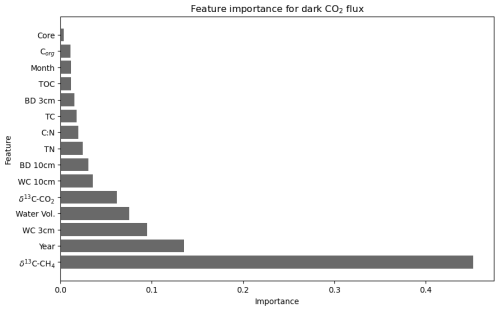
Figure 6Importance of environmental, temporal, and physicochemical variables in predicting CO2 flux under dark conditions at the landward sampling site (R2 = 0.63, cross-validation average (n = 5) of 0.43 after feature engineering).
CO2 flux under dark conditions was accurately predicted with the inclusion of 15 out of the 17 possible variables (Fig. 6). The most important single variable in predicting CO2 flux under dark conditions was δ13C–CH4 (0.46 importance) by a large margin. δ13C–CH4 averaged −47.5 ± 0.25 ‰ in dry conditions and −44.75 ± 1.2 ‰ under wet conditions, with a large range from −54.84 ‰ to −21.12 ‰. As with the model for light CO2 flux, the year of sampling was also the second-most-important feature in predicting dark CO2 flux (0.14 importance). Although the remaining 13 variables all had a feature importance below 0.1, this combination contributed towards an R2 score of 0.63.
In both models the core replicate was of minor importance (Figs. 5 and 6). The season during sampling was not included in the random forest model due to its nature as a categorical variable and high collinearity with other variables. Instead, Kruskal–Wallis ANOVA showed that the season had significant relationships with temperature (p < 0.001), water volume in the cores during incubation (p < 0.05), dark CH4 flux (p < 0.05), and light CH4 flux (p < 0.05). However, GHG flux and soil water content (WC) were not significantly influenced by seasonality.
4.1 Small but highly variable GHG fluxes
The CO2 and CH4 fluxes reported in this study are, in general, a small source of GHG emissions but include episodic events of high flux. The results fall within the lower range of fluxes previously reported, with CO2 flux from −16 900 to 629 200 µmol CO2 m−2 d−1 and CH4 flux ranging from −2.1 to 25 974 µmol CH4 m−2 d−1 (Sea et al., 2018). A review of 140 mangrove studies reported the global average CO2 flux of 56 800 ± 890 µmol CO2 m−2 d−1 (Rosentreter et al., 2018b), while a CH4 flux of 4557.0 ± 1102.1 µmol CH4 m−2 d−1 was found across 54 mangrove studies, with a total of 110 flux observations (Al-Haj and Fulweiler, 2020). Our results for sea–air fluxes in particular are many orders of magnitude smaller than other studies with similar methodologies (Jacotot et al., 2018). Two defining characteristics of the soil in this study are the low Corg and high salinity, which may reduce CO2 and CH4, respectively (Ouyang et al., 2017; Poffenbarger et al., 2011).
While comparisons can, and should, be drawn across different studies, the methodology of the respective study should be considered when interpreting results. For example, in situ studies have the advantage of natural conditions with minimal disturbance caused by sampling, whereas ex situ studies, such as incubation techniques, allow for greater control of variables but typically cannot entirely replicate in situ conditions such as diel temperature variation, changes in light intensity, and meteorological conditions (Toczydlowski et al., 2020; Sjögersten et al., 2018). For example, one study found a mangrove ecosystem flux of CH4 was the most variable on a daily basis due to meteorological variables and plant activities, both of which were excluded in this study (Liu et al., 2022). However, our study utilized incubations to maintain stringent control of environmental variables during the measurement period. The caveat of this approach is that it limits applicability to field conditions, but it is useful in separating the effects of individual drivers of GHG flux variation from mangrove soil and minimizing the number of confounding variables (Bond-Lamberty et al., 2016). An additional element of variation comes from different measurement techniques, as results can differ markedly between laser-based spectrometers, chamber-based systems, and eddy covariance measurements (Brannon et al., 2016; Podgrajsek et al., 2014). All studies compared in Table 3 are of in situ design, but there are a range of techniques and calculations used. These elements of variability complicate comparison across studies. There is often a large variation in GHG flux across studies, and it should be considered whether this variation is due to environmental conditions or different study designs. For example, in the same study site, CH4 fluxes from eddy covariance measurements have been lower than closed static chamber designs (Gnanamoorthy et al., 2022).
Table 3Comparative assessments of average mangrove fluxes under light conditions and standardized to µmol m−2 d−1 (± SE where data are available), unless otherwise specified. Fluxes for this study are calculated using data from both sites. More comprehensive review papers can be found for CO2 (Rosentreter et al., 2018b) and CH4 (Al-Haj and Fulweiler, 2020). Note: n/a – not applicable, NA – not available.
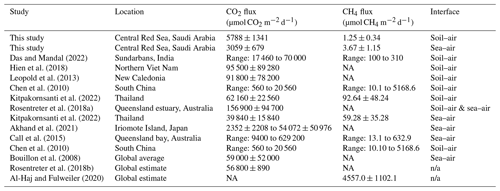
4.2 Drivers of flux variation
Although the landward and seaward study sites were within the same mangrove stand, there were considerably higher fluxes of CO2 and CH4 at the sea–air interface of the seaward site (43.7 g CO2-eq m−2 yr−1), compared to the sea–air interface at the landward site (−0.2 g CO2-eq m−2 yr−1). The main distinguishing environmental factor between the two sites appears to be the frequency and magnitude of tidal inundation as the landward site was microtidal, with long periods without tidal inundation. There is a strong semi-annual seasonal influence on tides in the central Red Sea. Extremely hot summer months coincide with low mean sea level states (Sultan et al., 1995), and in winter, the normally prevailing northwest winds are met by southeast winds, forming the Red Sea convergence zone in the centre of the Red Sea, resulting in higher mean sea levels (Langodan et al., 2017). This is supported by our analysis, showing the significance (p < 0.05) of season on the water volume captured in the soil cores during sampling. There was also a statistically significant seasonal influence on light and dark CH4 flux. This seasonal effect is likely modulated by temperature variation, which proved to be an important element of light CO2 flux in our random forest model. Additionally, higher temperatures in summer increase subsurface soil temperature, which can increase CH4 emissions due to the temperature dependency of microbial methanogens (Liu et al., 2020). The frequent absence of tidal inundation in summer exacerbates this effect as the high latent heat capacity of water could otherwise help regulate soil temperatures. Therefore, seasonality may exert a dual impact on methane emissions, explaining the significance.
A second important factor in CH4 flux is salinity, measured by electrical conductivity in this study, which demonstrated a lower mean in the seaward location. This may explain the higher CH4 emissions from this site as salinity is reported to have a negative influence on CH4 flux (Liu et al., 2020). Hypersaline mangrove environments are associated with low methane emissions (Cotovicz et al., 2024; Sea et al., 2018), because high salinity suppresses microbial activity and biogeochemical processes, reducing GHG cycling (Zhu et al., 2021). There is a proposed salinity threshold of 18 ppt, where CH4 flux may become negligible, which is significantly below the salinity found in the Red Sea (Alhassan and Aljahdali, 2021; Poffenbarger et al., 2011). The causes of the large differences in GHG flux between sites within the same mangrove stand are not fully resolved, although it is likely that there is microscale variation due, in part, to different gas transport processes. The release of CH4 from the soil via ebullition has particularly high spatial variability within sampling sites (Baulch et al., 2011; Chuang et al., 2017). Furthermore, the episodic nature of ebullition events may distort the flux calculation, which assumes a linear concentration change over time, as is the case with diffusive flux (Jacotot et al., 2018). The possibility of active ebullition in saline, undisturbed mangrove ecosystems requires further investigation, as to date, no study has found ebullition to be a significant pathway of CH4 release under these conditions (Cotovicz et al., 2024). Considering this small-scale variability, it is important to emphasize the need for comprehensive assessments in individual mangrove ecosystems as GHG flux is highly site-specific. Commonly, spatial variation in GHG fluxes is inferred from a few plots within the study site (Castillo et al., 2017). However, this method is likely to result in larger errors in estimates without attempting to determine factors driving this variation.
As evidenced by the monthly and site-specific flux variation, environmental and soil physicochemical factors are important in regulating mangrove soil GHG fluxes. In the literature, there are a multitude of variables suggested to influence CO2 and CH4 flux from mangrove soils. The variables reported to affect CO2 fluxes include soil organic carbon (SOC), nitrogen, phosphate, iron, ammonium, porosity, and tidal range (Chen et al., 2010; Jacotot et al., 2018; Sugiana et al., 2023; Wang et al., 2016). The variables reported to affect CH4 flux include SOC, ammonium, porewater salinity, redox potential, soil temperature, air temperature, and tidal range (Allen et al., 2007; Chen et al., 2010; Jacotot et al., 2018; Sugiana et al., 2023; Wang et al., 2016). Furthermore, additional factors have been suggested as general influences on overall mangrove GHG flux such as temperature fluctuations, soil moisture content, soil grain size, and tidal patterns (Hien et al., 2018; Ouyang et al., 2017). Many of these factors are inferred by a correlational relationship with GHG flux, with many variables likely to be co-linear, making causality difficult to determine.
An advantage of the random forest algorithm is that it allows many variables to be taken into account, with the ability to uncover non-linear relationships, its resistance to outliers, and the ability to test the model on other datasets (Smorkalov, 2022). However, there were variables mentioned above that were found to be important in GHG flux in other studies but were not measured in this study, for example, ammonium, iron, and soil grain size. There are limitations on the number of variables relative to the fairly small number of observations in this study (Kiers and Smilde, 2007), along with practical limitations of time and resources. There is substantial scope in future research to comprehensively investigate more variables than those reported here over a longer sampling period or with more frequent observations. An analysis of a greater number of chemical and physical characteristics of the soil beyond carbon and nitrogen would be particularly relevant for GHG flux (Nóbrega et al., 2014; Chen et al., 2010). This limitation must be acknowledged when interpreting our results as there may have been significantly important factors which were not measured and thus not considered in our analysis of the most important drivers of GHG flux.
The random forest modelling we conducted suggested temperature to be the most important factor in predicting light CO2 flux and δ13C–CH4 to be the most important factor in accurately predicting dark CO2 flux. To the best of our knowledge, the isotopic signature of methane is a variable that has not been previously suggested as an important predictor of mangrove CO2 flux. Notably, there was no statistically significant correlation to suggest a link between δ13C–CH4 and dark CO2 flux, contrary to the random forest model, suggesting this finding may be a result of overfitting from the random forest model, or there may be more complex non-linear relationships uncovered by machine learning, which was not detected by simple correlation or previous studies. The mean δ13C–CH4 at the landward and seaward sites (−47.2 ‰ and −48.1 ‰) were considerably less enriched than the −80.6 ‰ δ13C–CH4 found in a similar study on Red Sea mangrove GHG flux (Sea et al., 2018). The difference may be due to a number of factors including methanogenesis or oxidization, although both factors are unlikely to directly influence CO2 emissions. A previous study has found mangroves with the lightest δ13C–CO2 and δ13C–CH4 to have the lowest CO2 flux, further suggesting there may be a link between δ13C–CH4 and CO2 flux (Sea et al., 2018). Variations in δ13C–CH4 are highly likely to be driven by microbial processes, for example, methanotrophic bacteria which oxidize a fraction of total CH4 production, resulting in a more positive δ13C–CH4. A range of −65 ‰ to −50 ‰, similar to this study, found that aceticlastic methanogenesis (produced from acetate) dominates (Ouyang et al., 2024; Teh et al., 2005). Additionally, in a previous study on mangrove forests in Mexico, it was found that 30 %–70 % of the total CO2 measured was produced by methanogenesis (Sanchez-Carrillo et al., 2021). Anaerobic oxidation can also form CO2 (Shukla et al., 2013). These are possible explanations for our results demonstrating high importance of δ13C–CH4 as a predictor of CO2 flux. However, to better understand the origin and fate of CH4 from mangrove soils, methanogenesis should be studied directly through the determination of δ13C of the methyl group of acetate (Goevert and Conrad, 2009) or an isotope mass-balance approach (Sánchez-Carrillo et al., 2021), along with an investigation of the soil microbial community.
From our random forest models, the most important soil variables for CO2 flux were C : N and TC for light conditions and soil water content (WC 3 cm) and water volume for dark conditions. All factors have previously been documented to play a role in CO2 emissions (Chen et al., 2010). Preservation of TC is related to factors such as water level and inundation time and where low OC burial efficiency increases soil respiration (Breithaupt et al., 2019). C : N is a good predictor of soil microbial respiration (Fang and Moncrieff, 2005) and has previously been found to have a significant positive correlation with mangrove CO2 flux (Hien et al., 2018). Furthermore, soil respiration exhibits diurnal patterns, which may explain the high importance of carbon and nitrogen concentrations in predicting light CO2 flux but not dark CO2 flux (Jin et al., 2013). C : N may also be a good predictor for CO2 flux variability because of its relationship with the labile carbon pool, influenced by microbial biomass, which will vary by month and season, depending on the suitability of conditions for microbial growth (Padhy et al., 2020). Secondly, soil water content has been found to exert a negative influence on CO2 flux but has a positive relationship with Corg (Ouyang et al., 2017). However, there is also likely to be covariation among water content and variables not measured in this study, such as soil porosity, grain size, and density of crab burrows, which can increase CO2 flux (Booth et al., 2019; Ouyang et al., 2017). This implies that the interpretation of GHG flux variability should be carefully considered to ensure that non-linear relationships between multiple interrelated variables are accounted for.
In both models, the year had the second-highest predictive importance. There are a few theories for the importance of this factor. The growth and flowering cycles of A. marina mangroves in the Red Sea are not annual (Almahasheer et al., 2016c). In theory, increased growth over a given year may result in increased soil carbon pools for microbial respiration, directly impacting GHG flux. However, this cannot be tested as mangrove growth was not measured in the present study. Alternatively, the importance of the year of sampling may be artificially inflated in our models due to the presence of water during 4 of the 5 months sampled in 2021, while subsequent years were dominated by dry sampling conditions. However, there were also climatic variables and extreme weather patterns for the region across the 3-year period. Central Saudi Arabia experienced widespread greening in 2023 due to higher-than-average rainfall (Van Dijk et al., 2023), potentially also facilitating mangrove growth. It is likely that a combination of these three factors explain the predictive importance of the sampling year and emphasize the importance of long-term flux measurements to capture variations resulting from climatic changes and perennial life cycles of mangroves. Also, in both models, the core was of minor importance in predicting CO2 flux, which shows good replicability across the four cores sampled each month. This is supported by the correlation analysis, where the core replicate had no significant relationships with CO2 or CH4 flux under any conditions. However, there were significant relationships between the core and soil physicochemical properties such as Corg, TN, and δ13C–CO2 (Fig. S1). This is likely due to microscale differences in the deposition of organic matter and microbial communities, which is an element of natural variation in response to environmental conditions (Padhy et al., 2020).
The random forest model for the CO2 dark condition had a R2 score comparable to models previously used to predict SOC stock (Moreno Muñoz et al., 2024) while maintaining the majority of variables (15 of 17). However, based on the R2 metric, the model for light CO2 flux performed poorly on a higher number of variables, suggesting that many of these variables simply added “noise” to the predictions, without adding predictive power (Fox et al., 2017). It is likely the models' performance, particularly for light CO2, could be improved with the addition of other unmeasured factors such as clay or sulfur content, which were found to be important predictors of soil CO2 flux in sugarcane with random forest modelling (Tavares et al., 2018). The CH4 flux was not modelled due to the importance of microbial activities in CH4 cycling, which would not be accurately captured by the variables measured in this study (Das et al., 2018; Liu et al., 2020; Yu et al., 2020).
4.3 Implications for mangrove carbon budgets
Despite the small magnitude of fluxes reported in this study compared to global estimates, they deserve consideration in the net carbon sequestration of Red Sea mangroves, given their low carbon burial rate (Almahasheer et al., 2017). The carbon sequestration offset by the CO2-eq of the combined CO2 and CH4 fluxes measured in this study ranged between −130 % and 822 %. A negative CO2-eq implies net GHG removal from the atmosphere. There was an important difference between the mean and median offset of carbon sequestration by the combined CO2 and CH4 fluxes, which were 94.5 % and 4.9 %, respectively. The median estimate is less affected by extreme values and is, therefore, more representative of the central tendency of the offset, while the mean estimate fully captures the large variability in the long-term dataset of this study. Previous studies have also highlighted extreme variability where global mean emissions of CH4 flux were ∼ 16 times higher than the median estimate (Al-Haj and Fulweiler, 2020). Highly skewed data are appropriate to use only if they accurately reflect the true distribution of fluxes and not sampling bias (Rosentreter and Williamson, 2020). In this study, averages are likely to be an accurate statistic, given the controls on sampling location and consistent samples times each month over the full study period. This means that whereas the combined CO2 and CH4 fluxes were relatively small compared to reported mean organic carbon sequestration by the Red Sea mangrove stands studied, these are subject to occasionally large emissions that offset much of the carbon removed.
Prior studies have found GHG emissions to offset between 9.3 % and 32.7 % of the organic carbon sequestration of mangrove forests (Chen et al., 2016). A large component of this variability is dependent on whether fluxes are measured between the sea–air or soil–air interface (Table 3). CO2 emissions, which are the biggest contributor to CO2-eq emissions, are greatly affected when measured between the sea–air or soil–air interface. When CO2 is released from the soil into the water column, it enters the carbonate system and can be converted to bicarbonate or carbonate ions (Zeebe and Wolf-Gladrow, 2001). As a result, the majority of CO2 emitted from the soil undergoes dissolution in the water column before it is released to the air. This explains the lower CO2-eq from the sea–air interface of −0.4 g CO2-eq m−2 yr−1 compared to 172.1 g CO2-eq m−2 yr−1 for the soil–air interface when soils are directly exposed to air. Typically, when fluxes are measured from the sea–air interface, equilibration equations are used to account for the changes in carbonate chemistry in the seawater (Akhand et al., 2021; Call et al., 2015). However, the aim of this study was to compare GHG flux to the air between interfaces, so the calculations used here only consider linear changes in concentration across time points emphasizing diffusive fluxes to the atmosphere over other methods of gas transfer such as bubble ebullition (Jacotot et al., 2018). Overall, the CO2-eq released to the atmosphere is a significant offset to carbon burial, given the carbon burial rate of Red Sea mangroves is just 55 g CO2-eq m−2 yr−1 (15 g Corg m−2 yr−1), over 10-fold lower than the global average of 598 g CO2-eq m−2 yr−1 (163 g Corg m−2 yr−1) (Almahasheer et al., 2017; Breithaupt et al., 2012).
However, in the Red Sea region mangrove soils have a high carbonate content; our estimates of Cinorg fall within the upper range of previously reported figures for Red Sea mangroves, which are higher than global average estimates (Garcias-Bonet et al., 2019; Saderne et al., 2019). Furthermore, mangrove soil in the same location as the present study has 76 % ± 2 % (dry weight) CaCO3, which is attributed to their growth on underlying carbonate platforms formed by Pleistocene coral reefs (Saderne et al., 2018). As a result, there is an additional factor to consider: the role of total alkalinity (TA) enhancement from carbonate dissolution in the mangrove soils, which increases the capacity for seawater to absorb CO2 from the atmosphere (Alongi, 2022; Saderne et al., 2019). Mangroves in the Red Sea are characterized as important TA sources (Saderne et al., 2019), which are driven by high metabolic activity in their soil and multi-stage biogeochemical processes such as carbonate dissolution, denitrification, sulfate reduction, and ammonification (Baldry et al., 2020; Saderne et al., 2021; Sippo et al., 2016).
CaCO3 dissolution is particularly relevant to the central Red Sea, as 1 mol of dissolved CaCO3 results in the uptake of 0.6 mol of atmospheric CO2 (Frankignoulle et al., 1995). The dissolution of the large CaCO3 pool in the soils of Red Sea mangroves presents a substantial additional carbon sink, exceeding 23-fold the Corg burial rate for the central Red Sea (Almahasheer et al., 2017; Saderne et al., 2021). Although the lower carbon burial in soil means GHG fluxes are a large offset to the soil carbon burial, TA enhancement brings the carbon sink value of the mangrove stand in this study to 360 g C m−2 yr−1, which is 2.2-fold above global mean mangrove Corg (Saderne et al., 2021). In the present study the CO2-eq of GHG fluxes represents a small offset (3.9 % on average) to the combined carbon sequestration of this mangrove stand when accounting for carbon burial and TA enhancement combined.
The long-term flux variability captured in this study provides valuable insights into the role of GHG flux in offsetting carbon burial in Red Sea mangrove soils. Our study involved an improved temporal resolution, in terms of the overall duration and frequency of assessments, beyond most previous assessments. This is important because our results show that CO2 and CH4 fluxes are typically a small carbon offset compared to carbon burial in soils, but they are punctuated with episodic GHG emission bursts that suffice to offset a large fraction of carbon burial. This aspect of GHG flux dynamics may be missed by studies with poorer temporal coverage.
When considering the carbon budget of the central Red Sea mangrove stand considered in this study, our results show the overriding importance of TA enhancement from carbonate dissolution, which is emerging as a major component of mangrove CO2 removal, not yet captured in blue-carbon projects. Our results also showed that the direct exposure of mangrove soils to the atmosphere drastically enhances GHG emissions compared to emissions during tidal flooding. Environmental conditions helped explain variability in CO2 emissions, whereas those in CH4 emissions seem to be dominated by the dynamics of the microbial community responsible for methanogenesis and methane oxidation.
All data to support the findings of this study are available in Figshare. Raw data for the landward site are available at https://doi.org/10.6084/m9.figshare.26085898 (Breavington et al., 2024a). Combined site data across sea–air and soil–air interfaces are available at https://doi.org/10.6084/m9.figshare.26085928 (Breavington et al., 2024b). Code and associated data for random forest algorithms are available at https://doi.org/10.6084/m9.figshare.26085940 (Breavington, 2024).
The supplement related to this article is available online at: https://doi.org/10.5194/bg-22-117-2025-supplement.
CMD conceived the research. AS, CF, JB, and CMD designed the study. AS, CF, and JB performed the field sampling. AS, CF, JB, and ME performed laboratory work. ME performed gas measurements. AS, CF, and JB conducted data analysis. JB produced the displayed figures and wrote the manuscript. All authors contributed and approved the manuscript.
The contact author has declared that none of the authors has any competing interests.
Publisher's note: Copernicus Publications remains neutral with regard to jurisdictional claims made in the text, published maps, institutional affiliations, or any other geographical representation in this paper. While Copernicus Publications makes every effort to include appropriate place names, the final responsibility lies with the authors.
We extend thanks to Asalata Kotikalapudi for help with sample processing and Reny P. Devassy, Jennifer Thompson, Naira Pluma, Elisa Laiolo, and Anastasiia Martynova for their assistance with fieldwork. We thank Larissa Frühe for her assistance with R plots.
This research has been supported by the King Abdullah University of Science and Technology through baseline (grant no. BAS/1/1071-01-01) funding for Carlos M. Duarte.
This paper was edited by Edzo Veldkamp and reviewed by Stephan Glatzel and Andre Rovai.
Akhand, A., Watanabe, K., Chanda, A., Tokoro, T., Chakraborty, K., Moki, H., Tanaya, T., Ghosh, J., and Kuwae, T.: Lateral carbon fluxes and CO2 evasion from a subtropical mangrove-seagrass-coral continuum, Sci. Total Environ., 752, 142190, https://doi.org/10.1016/j.scitotenv.2020.142190, 2021.
Al-Haj, A. N. and Fulweiler, R. W.: A synthesis of methane emissions from shallow vegetated coastal ecosystems, Glob. Change Biol., 26, 2988–3005, https://doi.org/10.1111/gcb.15046, 2020.
Alhassan, A. B. and Aljahdali, M. O.: Nutrient and physicochemical properties as potential causes of stress in mangroves of the central Red Sea, PLOS ONE, 16, e0261620, https://doi.org/10.1371/journal.pone.0261620, 2021.
Allen, D. E., Dalal, R. C., Rennenberg, H., Meyer, R. L., Reeves, S., and Schmidt, S.: Spatial and temporal variation of nitrous oxide and methane flux between subtropical mangrove sediments and the atmosphere, Soil Biol. Biochem., 39, 622–631, https://doi.org/10.1016/j.soilbio.2006.09.013, 2007.
Allen, M. R., Barros, V. R., Broome, J., Cramer, W., Christ, R., Church, J. A., Clarke, L., Dahe, Q., Dasgupta, P., Dubash, N. K., Edenhofer, O., Elgizouli, I., Field, C. B., Forster, P., Friedlingstein, P., Fuglestvedt, J., Gomez-Echeverri, L., Hallegatte, S., Hegerl, G., Howden, M., Jiang, K., Jimenez Cisneroz, B., Kattsov, V., Lee, H., Mach, K. J., Marotzke, J., Mastrandrea, M. D., Meyer, L., Minx, J., Mulugetta, Y., O'Brien, K., Oppenheimer, M., Pereira, J. J., Pichs-Madruga, R., Plattner, G.-K., Pörtner, H.-O., Power, S. B., Preston, B., Ravindranath, N. H., Reisinger, A., Riahi, K., Rusticucci, M., Scholes, R., Seyboth, K., Sokona, Y., Stavins, R., Stocker, T. F., Tschakert, P., van Vuuren, D., and van Ypserle, J.-P.: IPCC: Climate Change 2014: Synthesis Report. Contribution of Working Groups I, II and III to the Fifth Assessment Report of the Intergovernmental Panel on Climate Change, edited by: Pachauri, R. K. and Meyer, L., IPCC, Geneva, Switzerland, 151 pp., ISBN 9789291691432, 2014.
Almahasheer, H., Aljowair, A., Duarte, C. M., and Irigoien, X.: Decadal stability of Red Sea mangroves, Estuar. Coast. Shelf S., 169, 164–172, https://doi.org/10.1016/j.ecss.2015.11.027, 2016a.
Almahasheer, H., Duarte, C. M., and Irigoien, X.: Nutrient Limitation in Central Red Sea Mangroves, Front. Mar. Sci., 3, 271, https://doi.org/10.3389/fmars.2016.00271, 2016b.
Almahasheer, H., Duarte, C., and Irigoien, X.: Phenology and Growth dynamics of Avicennia marina in the Central Red Sea, Sci. Rep., 6, 37785, https://doi.org/10.1038/srep37785, 2016c.
Almahasheer, H., Serrano, O., Duarte, C. M., Arias-Ortiz, A., Masque, P., and Irigoien, X.: Low Carbon sink capacity of Red Sea mangroves, Sci. Rep., 7, 9700, https://doi.org/10.1038/s41598-017-10424-9, 2017.
Alongi, D.: Impacts of Climate Change on Blue Carbon Stocks and Fluxes in Mangrove Forests, FORESTS, 13, 149, https://doi.org/10.3390/f13020149, 2022.
Baldry, K., Saderne, V., McCorkle, D. C., Churchill, J. H., Agusti, S., and Duarte, C. M.: Anomalies in the carbonate system of Red Sea coastal habitats, Biogeosciences, 17, 423–439, https://doi.org/10.5194/bg-17-423-2020, 2020.
Baulch, H. M., Dillon, P. J., Maranger, R., and Schiff, S. L.: Diffusive and ebullitive transport of methane and nitrous oxide from streams: Are bubble-mediated fluxes important?, J. Geophys. Res.-Biogeo., 116, G04028, https://doi.org/10.1029/2011JG001656, 2011.
Blanco-Sacristán, J., Johansen, K., Duarte, C. M., Daffonchio, D., Hoteit, I., and McCabe, M. F.: Mangrove distribution and afforestation potential in the Red Sea, Sci. Total Environ., 843, 157098, https://doi.org/10.1016/j.scitotenv.2022.157098, 2022.
Bond-Lamberty, B., Smith, A. P., and Bailey, V.: Temperature and moisture effects on greenhouse gas emissions from deep active-layer boreal soils, Biogeosciences, 13, 6669–6681, https://doi.org/10.5194/bg-13-6669-2016, 2016.
Booth, J. M., Fusi, M., Marasco, R., Mbobo, T., and Daffonchio, D.: Fiddler crab bioturbation determines consistent changes in bacterial communities across contrasting environmental conditions, Sci. Rep., 9, 3749, https://doi.org/10.1038/s41598-019-40315-0, 2019.
Bouillon, S., Borges, A. V., Castañeda-Moya, E., Diele, K., Dittmar, T., Duke, N. C., Kristensen, E., Lee, S. Y., Marchand, C., Middelburg, J. J., Rivera-Monroy, V. H., Smith III, T. J., and Twilley, R. R.: Mangrove production and carbon sinks: A revision of global budget estimates, Global Biogeochem. Cy., 22, GB2013, https://doi.org/10.1029/2007GB003052, 2008.
Brannon, E. Q., Moseman-Valtierra, S. M., Rella, C. W., Martin, R. M., Chen, X., and Tang, J.: Evaluation of laser-based spectrometers for greenhouse gas flux measurements in coastal marshes, Limnol. Oceanogr.-Meth., 14, 466–476, https://doi.org/10.1002/lom3.10105, 2016.
Breavington, J.: Random forest variable importance, figshare [code], https://doi.org/10.6084/m9.figshare.26085940, 2024.
Breavington, J., Steckbauer, A., Fu, C., Ennasri, M., and Duarte, C. M.: Landward site raw data, figshare [data set], https://doi.org/10.6084/m9.figshare.26085898, 2024a.
Breavington, J., Steckbauer, A., Fu, C., Ennasri, M., and Duarte, C. M.: Landward and seaward fluxes and soil properties, figshare [data set], https://doi.org/10.6084/m9.figshare.26085928, 2024b.
Breiman, L.: Random Forests, Mach. Learn., 45, 5–32, https://doi.org/10.1023/A:1010933404324, 2001.
Breithaupt, J. L., Smoak, J. M., Smith III, T. J., Sanders, C. J., and Hoare, A.: Organic carbon burial rates in mangrove sediments: Strengthening the global budget, Global Biogeochem. Cy., 26, GB3011, https://doi.org/10.1029/2012GB004375, 2012.
Breithaupt, J. L., Smoak, J. M., Sanders, C. J., and Troxler, T. G.: Spatial Variability of Organic Carbon, CaCO3 and Nutrient Burial Rates Spanning a Mangrove Productivity Gradient in the Coastal Everglades, Ecosystems, 22, 844–858, https://doi.org/10.1007/s10021-018-0306-5, 2019.
Call, M., Maher, D. T., Santos, I. R., Ruiz-Halpern, S., Mangion, P., Sanders, C. J., Erler, D. V., Oakes, J. M., Rosentreter, J., Murray, R., and Eyre, B. D.: Spatial and temporal variability of carbon dioxide and methane fluxes over semi-diurnal and spring–neap–spring timescales in a mangrove creek, Geochim. Cosmochim. Ac., 150, 211–225, https://doi.org/10.1016/j.gca.2014.11.023, 2015.
Castillo, J., Apan, A., Maraseni, T., and Salmo, S.: Soil greenhouse gas fluxes in tropical mangrove forests and in land uses on deforested mangrove lands, CATENA, 159, 60–69, https://doi.org/10.1016/j.catena.2017.08.005, 2017.
Chen, G., Chen, B., Yu, D., Tam, N. F. Y., Ye, Y., and Chen, S.: Soil greenhouse gas emissions reduce the contribution of mangrove plants to the atmospheric cooling effect, Environ. Res. Lett., 11, 124019, https://doi.org/10.1088/1748-9326/11/12/124019, 2016.
Chen, G. C., Tam, N. F. Y., and Ye, Y.: Summer fluxes of atmospheric greenhouse gases N2O, CH4 and CO2 from mangrove soil in South China, Sci. Total Environ., 408, 2761–2767, https://doi.org/10.1016/j.scitotenv.2010.03.007, 2010.
Chuang, P. C., Young, M. B., Dale, A. W., Miller, L. G., Herrera-Silveira, J. A., and Paytan, A.: Methane fluxes from tropical coastal lagoons surrounded by mangroves, Yucatán, Mexico, J. Geophys. Res.-Biogeo., 122, 1156–1174, https://doi.org/10.1002/2017JG003761, 2017.
Cotovicz, L. C., Abril, G., Sanders, C. J., Tait, D. R., Maher, D. T., Sippo, J. Z., Holloway, C., Yau, Y. Y. Y., and Santos, I. R.: Methane oxidation minimizes emissions and offsets to carbon burial in mangroves, Nat. Clim. Chang., 14, 275–281, https://doi.org/10.1038/s41558-024-01927-1, 2024.
Curran, S., Kumar, A., Lutz, W., and Williams, M.: Interactions between Coastal and Marine Ecosystems and Human Population Systems: Perspectives on How Consumption Mediates This Interaction, Ambio, 31, 264–268, 2002.
Das, N. and Mandal, S.: Microbial populations regulate greenhouse gas emissions in Sundarban mangrove ecosystem, India, Acta Ecologica Sinica, 42, 641–652, https://doi.org/10.1016/j.chnaes.2021.07.011, 2022.
Das, S., Ganguly, D., Chakraborty, S., Mukherjee, A., and De, T.: Methane flux dynamics in relation to methanogenic and methanotrophic populations in the soil of Indian Sundarban mangroves, Mar. Ecol.-Evol. Persp., 39, e12493, https://doi.org/10.1111/maec.12493, 2018.
Duarte, C., Losada, I., Hendriks, I., Mazarrasa, I., and Marba, N.: The role of coastal plant communities for climate change mitigation and adaptation, Nat. Clim. Change, 3, 961–968, https://doi.org/10.1038/NCLIMATE1970, 2013.
Fang, C. and Moncrieff, J. B.: The variation of soil microbial respiration with depth in relation to soil carbon composition, Plant Soil, 268, 243–253, https://doi.org/10.1007/s11104-004-0278-4, 2005.
Forster, P., Ramaswamy, V., Artaxo, P., Berntsen, T., Betts, R., Fahey, D. W., Haywood, J., Lean, J., Lowe, D. C., Myhre, G., Nganga, J., Prinn, R., Raga, G., Schulz, M., van Dorland, R., Bodeker, G., Boucher, O., Collins, W. D., Conway, T. J., Dlugokencky, E., Elkins, J. W., Etheridge, D., Foukal, P., Fraser, P., Geller, M., Joos, F., Keeling, C. D., Kinne, S., Lassey, K., Lohmann, U., Manning, A. C., Montzka, S., Oram, D., O'Shaughnessy, K., Piper, S., Plattner, G.-K., Ponater, M., Ramankutty, N., Reid, G., Rind, D., Rosenlof, K., Sausen, R., Schwarzkopf, D., Solanki, S. K., Stenchikov, G., Stuber, N., Takemura, T., Textor, C., Wang, R., Weiss, R., and Whorf, T.: Changes in Atmospheric Constituents and in Radiative Forcing, in: Climate Change 2007: The Physical Science Basis. Contribution of Working Group I to the 4th Assessment Report of the Intergovernmental Panel on Climate Change, edited by: Solomon, S., Qin, D., Manning, M., Chen, Z., Marquis, M., Averyt, K. B., Tignor, M., and Miller, H. L., Cambridge University Press, Cambridge, United Kingdom and New York, USA, 129–234, ISBN 9780521880091, 2007.
Fox, E. W., Hill, R. A., Leibowitz, S. G., Olsen, A. R., Thornbrugh, D. J., and Weber, M. H.: Assessing the accuracy and stability of variable selection methods for random forest modeling in ecology, Environ. Monit. Assess., 189, 316, https://doi.org/10.1007/s10661-017-6025-0, 2017.
Frankignoulle, M., Pichon, M., and Gattuso, J.-P.: Aquatic Calcification as a Source of Carbon Dioxide, in: Carbon Sequestration in the Biosphere, Berlin, Heidelberg, 265–271, https://doi.org/10.1007/978-3-642-79943-3_18, 1995.
Gabr, S. S., Morsy, E. A., El Bastawesy, M. A., Habeebullah, T. M., and Shaaban, F. F.: Exploration of potential groundwater resources at Thuwal area, north of Jeddah, Saudi Arabia, using remote sensing data analysis and geophysical survey, Arab. J. Geosci., 10, 509, https://doi.org/10.1007/s12517-017-3295-3, 2017.
Garcias-Bonet, N., Delgado-Huertas, A., Carrillo-de-Albornoz, P., Anton, A., Almahasheer, H., Marbà, N., Hendriks, I. E., Krause-Jensen, D., and Duarte, C. M.: Carbon and Nitrogen Concentrations, Stocks, and Isotopic Compositions in Red Sea Seagrass and Mangrove Sediments, Front. Mar. Sci., 6, 267, https://doi.org/10.3389/fmars.2019.00267, 2019.
Genuer, R., Poggi, J.-M., and Tuleau-Malot, C.: Variable selection using random forests, Pattern Recogn. Lett., 31, 2225–2236, https://doi.org/10.1016/j.patrec.2010.03.014, 2010.
Gnanamoorthy, P., Chakraborty, S., Nagarajan, R., Ramasubramanian, R., Selvam, V., Burman, P. K. D., Sarathy, P. P., Zeeshan, M., Song, Q., and Zhang, Y.: Seasonal variation of methane fluxes in a mangrove ecosystem in south India: An eddy covariance-based approach, Estuar. Coast., 45, 551–566, https://doi.org/10.1007/s12237-021-00988-1, 2022.
Goevert, D. and Conrad, R.: Effect of substrate concentration on carbon isotope fractionation during acetoclastic methanogenesis by Methanosarcina barkeri and M. acetivorans and in rice field soil, Appl. Environ. Microb., 75, 2605–2612, https://doi.org/10.1128/AEM.02680-08, 2009.
Hardie, M. and Doyle, R.: Measuring Soil Salinity, in: Plant Salt Tolerance: Methods and Protocols, edited by: Shabala, S. and Cuin, T. A., Humana Press, Totowa, NJ, 415–425, https://doi.org/10.1007/978-1-61779-986-0_28, 2012.
Hien, H. T., Marchand, C., Aimé, J., and Cuc, N. T. K.: Seasonal variability of CO2 emissions from sediments in planted mangroves (Northern Viet Nam), Estuar. Coast. Shelf S., 213, 28–39, https://doi.org/10.1016/j.ecss.2018.08.006, 2018.
Howard, J., Hoyt, S., Isensee, K., Telszewski, M., and Pidgeon, E. (Eds.): Coastal blue carbon: methods for assessing carbon stocks and emissions factors in mangroves, tidal salt marshes, and seagrasses, Conservation International, Intergovernmental Oceanographic Commission of UNESCO, International Union for Conservation of Nature, Arlington, Virginia, USA, 184 pp., https://doi.org/10568/95127 (last access: 10 November 2023), 2014.
Jacotot, A., Marchand, C., and Allenbach, M.: Tidal variability of CO2 and CH4 emissions from the water column within a Rhizophora mangrove forest (New Caledonia), Sci. Total Environ., 631–632, 334–340, https://doi.org/10.1016/j.scitotenv.2018.03.006, 2018.
Jin, L., Lu, C.-Y., Ye, Y., and Ye, G.-F.: Soil Respiration in a Subtropical Mangrove Wetland in the Jiulong River Estuary, China, Pedosphere, 23, 678–685, https://doi.org/10.1016/S1002-0160(13)60060-0, 2013.
Kargas, G., Chatzigiakoumis, I., Kollias, A., Spiliotis, D., and Kerkides, P.: An Investigation of the Relationship between the Electrical Conductivity of the Soil Saturated Paste Extract ECe with the Respective Values of the Mass Soil/Water Ratios 1 : 1 and 1 : 5 (EC1:1 and EC1:5), Proceedings, 2, 661, https://doi.org/10.3390/proceedings2110661, 2018.
Khalil, A. S.: Mangroves of the red sea. The Red Sea: The formation, morphology, oceanography and environment of a young ocean basin, Springer Berlin, Heidelberg, Germany, 585–597, https://doi.org/10.1007/978-3-662-45201-1_33, 2015.
Kiers, H. A. and Smilde, A. K.: A comparison of various methods for multivariate regression with highly collinear variables, Stat. Method. Appl., 16, 193–228, https://doi.org/10.1007/s10260-006-0025-5, 2007.
Kitpakornsanti, K., Pengthamkeerati, P., Limsakul, A., Worachananant, P., and Diloksumpun, S.: Greenhouse gas emissions from soil and water surface in different mangrove establishments and management in Ranong Biosphere Reserve, Thailand, Regional Studies in Marine Science, 56, 102690, https://doi.org/10.1016/j.rsma.2022.102690, 2022.
Langodan, S., Cavaleri, L., Vishwanadhapalli, Y., Pomaro, A., Bertotti, L., and Hoteit, I.: The climatology of the Red Sea – part 1: the wind: THE WIND CLIMATOLOGY OF THE RED SEA, Int. J. Climatol, 37, 4509–4517, https://doi.org/10.1002/joc.5103, 2017.
Leopold, A., Marchand, C., Deborde, J., Chaduteau, C., and Allenbach, M.: Influence of mangrove zonation on CO2 fluxes at the sediment–air interface (New Caledonia), Geoderma, 202–203, 62–70, https://doi.org/10.1016/j.geoderma.2013.03.008, 2013.
Liu, J., Zhou, Y., Valach, A., Shortt, R., Kasak, K., Rey-Sanchez, C., Hemes, K. S., Baldocchi, D., and Lai, D. Y. F.: Methane emissions reduce the radiative cooling effect of a subtropical estuarine mangrove wetland by half, Glob. Change Biol., 26, 4998–5016, https://doi.org/10.1111/gcb.15247, 2020.
Liu, J., Valach, A., Baldocchi, D., and Lai, D. Y.: Biophysical controls of ecosystem-scale methane fluxes from a subtropical estuarine mangrove: Multiscale, nonlinearity, asynchrony and causality. Global Biogeochem. Cy., 36, e2021GB007179, https://doi.org/10.1029/2021GB007179, 2022.
Lovelock, C. E., Barbier, E., and Duarte, C. M.: Tackling the mangrove restoration challenge, PLOS Biol., 20, e3001836, https://doi.org/10.1371/journal.pbio.3001836, 2022.
Mackey, A.: Biomass of the mangrove Avicennia marina (Forsk.) Vierh. near Brisbane, south-eastern Queensland, Mar. Freshwater Res., 44, 721–725, https://doi.org/10.1071/MF9930721, 1993.
Marcott, S. A., Shakun, J. D., Clark, P. U., and Mix, A. C.: A Reconstruction of Regional and Global Temperature for the Past 11,300 Years, Science, 339, 1198–1201, https://doi.org/10.1126/science.1228026, 2013.
Moreno Muñoz, A. S., Guzmán Alvis, Á. I., and Benavides Martínez, I. F.: A random forest model to predict soil organic carbon storage in mangroves from Southern Colombian Pacific coast, Estuar. Coast. Shelf S., 299, 108674, https://doi.org/10.1016/j.ecss.2024.108674, 2024.
Myhre, G., Shindell, D., Bréon, F.-M., Collins, W., Fuglestvedt, J., Huang, J., Koch, D., Lamarque, J.-F., Lee, D., Mendoza, B., Nakajima, T., Robock, A., Stephens, G., Takemura, T., and Zhang, H.: Anthropogenic and Natural Radiative Forcing, in: Climate Change 2013: The Physical Science Basis. Contribution of Working Group I to the Fifth Assessment Report of the Intergovernmental Panel on Climate Change, edited by: Stocker, T. F., Qin, D., Plattner, G.-K., Tignor, M., Allen, S. K., Boschung, J., Nauels, A., Xia, Y., Bex, V., and Midgley, P. M., Cambridge University Press, Cambridge, United Kingdom and New York, NY, USA, 659–740, https://doi.org/10.1017/CBO9781107415324.018, 2013.
Nóbrega, G. N., Ferreira, T. O., Neto, M. S., Queiroz, H. M., Artur, A. G., Mendonça, E. D. S., Silva, E. D. O., and Otero, X. L.: Edaphic factors controlling summer (rainy season) greenhouse gas emissions (CO2 and CH4) from semiarid mangrove soils (NE-Brazil), Sci. Total Environ., 542, 685–693, https://doi.org/10.1016/j.scitotenv.2015.10.108, 2014.
Ouyang, X., Lee, S. Y., and Connolly, R. M.: Structural equation modelling reveals factors regulating surface sediment organic carbon content and CO2 efflux in a subtropical mangrove, Sci. Total Environ., 578, 513–522, https://doi.org/10.1016/j.scitotenv.2016.10.218, 2017.
Ouyang, X., Guo, F., and Lee, S. Y.: Multiple drivers for carbon stocks and fluxes in different types of mangroves, Sci. Total Environ., 906, 167511, https://doi.org/10.1016/j.scitotenv.2023.167511, 2024.
Padhy, S., Bhattacharyya, P., Dash, P., Reddy, C., Chakraborty, A., and Pathak, H.: Seasonal fluctuation in three mode of greenhouse gases emission in relation to soil labile carbon pools in degraded mangrove, Sundarban, India, Sci. Total Environ., 705, 135909, https://doi.org/10.1016/j.scitotenv.2019.135909, 2020.
Podgrajsek, E., Sahlée, E., Bastviken, D., Holst, J., Lindroth, A., Tranvik, L., and Rutgersson, A.: Comparison of floating chamber and eddy covariance measurements of lake greenhouse gas fluxes, Biogeosciences, 11, 4225–4233, https://doi.org/10.5194/bg-11-4225-2014, 2014.
Poffenbarger, H. J., Needelman, B. A., and Megonigal, J. P.: Salinity Influence on Methane Emissions from Tidal Marshes, Wetlands, 31, 831–842, https://doi.org/10.1007/s13157-011-0197-0, 2011.
Rosentreter, J. A. and Williamson, P.: Concerns and uncertainties relating to methane emissions synthesis for vegetated coastal ecosystems, Glob. Change Biol., 26, 5351–5352, https://doi.org/10.1111/gcb.15201, 2020.
Rosentreter, J. A., Maher, D. T., Erler, D. V., Murray, R., and Eyre, B. D.: Factors controlling seasonal CO2 and CH4 emissions in three tropical mangrove-dominated estuaries in Australia, Estuar. Coast. Shelf S., 215, 69–82, https://doi.org/10.1016/j.ecss.2018.10.003, 2018a.
Rosentreter, J. A., Maher, D. T., Erler, D. V., Murray, R., and Eyre, B. D.: Seasonal and temporal CO2 dynamics in three tropical mangrove creeks – A revision of global mangrove CO2 emissions, Geochim. Cosmochim. Ac., 222, 729–745, https://doi.org/10.1016/j.gca.2017.11.026, 2018b.
Saderne, V., Cusack, M., Almahasheer, H., Serrano, O., Masqué, P., Arias-Ortiz, A., Krishnakumar, P. K., Rabaoui, L., Qurban, M. A., and Duarte, C. M.: Accumulation of Carbonates Contributes to Coastal Vegetated Ecosystems Keeping Pace With Sea Level Rise in an Arid Region (Arabian Peninsula), J. Geophys. Res.-Biogeo., 123, 1498–1510, https://doi.org/10.1029/2017JG004288, 2018.
Saderne, V., Geraldi, N. R., Macreadie, P. I., Maher, D. T., Middelburg, J. J., Serrano, O., Almahasheer, H., Arias-Ortiz, A., Cusack, M., Eyre, B. D., Fourqurean, J. W., Kennedy, H., Krause-Jensen, D., Kuwae, T., Lavery, P. S., Lovelock, C. E., Marba, N., Masqué, P., Mateo, M. A., Mazarrasa, I., McGlathery, K. J., Oreska, M. P. J., Sanders, C. J., Santos, I. R., Smoak, J. M., Tanaya, T., Watanabe, K., and Duarte, C. M.: Role of carbonate burial in Blue Carbon budgets, Nat. Commun., 10, 1106, https://doi.org/10.1038/s41467-019-08842-6, 2019.
Saderne, V., Fusi, M., Thomson, T., Dunne, A., Mahmud, F., Roth, F., Carvalho, S., and Duarte, C. M.: Total alkalinity production in a mangrove ecosystem reveals an overlooked Blue Carbon component, Limnol. Oceanogr. Lett., 6, 61–67, https://doi.org/10.1002/lol2.10170, 2021.
Sanchez-Carrillo, S., Garatuza-Payan, J., Sanchez-Andres, R., Cervantes, F., Bartolome, M., Merino-Ibarra, M., and Thalasso, F.: Methane Production and Oxidation in Mangrove Soils Assessed by Stable Isotope Mass Balances, WATER, 13, 1867, https://doi.org/10.3390/w13131867, 2021.
Sanderman, J., Hengl, T., Fiske, G., Solvik, K., Adame, M. F., Benson, L., Bukoski, J. J., Carnell, P., Cifuentes-Jara, M., Donato, D., Duncan, C., Eid, E. M., zu Ermgassen, P., Lewis, C. J. E., Macreadie, P. I., Glass, L., Gress, S., Jardine, S. L., Jones, T. G., Nsombo, E. N., Rahman, M. M., Sanders, C. J., Spalding, M., and Landis, E.: A global map of mangrove forest soil carbon at 30 m spatial resolution, Environ. Res. Lett., 13, 055002, https://doi.org/10.1088/1748-9326/aabe1c, 2018.
Sea, M. A., Garcias-Bonet, N., Saderne, V., and Duarte, C. M.: Carbon dioxide and methane fluxes at the air–sea interface of Red Sea mangroves, Biogeosciences, 15, 5365–5375, https://doi.org/10.5194/bg-15-5365-2018, 2018.
Shukla, P. N., Pandey, K. D., and Mishra, V. K.: Environmental Determinants of Soil Methane Oxidation and Methanotrophs, Crit. Rev. Env. Sci. Tec., 43, 1945–2011, https://doi.org/10.1080/10643389.2012.672053, 2013.
Sippo, J. Z., Maher, D. T., Tait, D. R., Holloway, C., and Santos, I. R.: Are mangroves drivers or buffers of coastal acidification? Insights from alkalinity and dissolved inorganic carbon export estimates across a latitudinal transect, Global Biogeochem. Cy., 30, 753–766, https://doi.org/10.1002/2015GB005324, 2016.
Sjögersten, S., Aplin, P., Gauci, V., Peacock, M., Siegenthaler, A., and Turner, B. L.: Temperature response of ex-situ greenhouse gas emissions from tropical peatlands: Interactions between forest type and peat moisture conditions, Geoderma, 324, 47–55, https://doi.org/10.1016/j.geoderma.2018.02.029, 2018.
Smorkalov, I. A.: Soil Respiration Variability: Contributions of Space and Time Estimated Using the Random Forest Algorithm, Russ. J. Ecol., 53, 295–307, https://doi.org/10.1134/S1067413622040051, 2022.
Speiser, J. L., Miller, M. E., Tooze, J., and Ip, E.: A comparison of random forest variable selection methods for classification prediction modeling, Expert Syst. Appl., 134, 93–101, https://doi.org/10.1016/j.eswa.2019.05.028, 2019.
Sugiana, I. P., Faiqoh, E., Adame, M. F., Indrawan, G. S., Andiani, A. A. E., Dewi, I. G. A. I. P., and Dharmawan, I. W. E.: Soil greenhouse gas fluxes to the atmosphere during the wet season across mangrove zones in Benoa Bay, Indonesia, Asian J. Atmos. Environ., 17, 13, https://doi.org/10.1007/s44273-023-00014-9, 2023.
Sultan, S., Ahmad, F., and El-Hassan, A.: Seasonal variations of the sea level in the central part of the Red Sea, Estuar. Coast. Shelf S., 40, 1–8, https://doi.org/10.1016/0272-7714(95)90008-X, 1995.
Tavares, R. L. M., Oliveira, S. R. d. M., de Barros, F. M. M., Farhate, C. V. V., de Souza, Z. M., and Scala Junior, N. L.: Prediction of soil CO2 flux in sugarcane management systems using the Random Forest approach, Sci. agric. (Piracicaba, Braz.), 75, 281–287, https://doi.org/10.1590/1678-992X-2017-0095, 2018.
Teh, Y. A., Silver, W. L., and Conrad, M. E.: Oxygen effects on methane production and oxidation in humid tropical forest soils, Glob. Change Biol., 11, 1283–1297, https://doi.org/10.1111/j.1365-2486.2005.00983.x, 2005.
Tete, E., Viaud, V., and Walter, C.: Organic carbon and nitrogen mineralization in a poorly-drained mineral soil under transient waterlogged conditions: an incubation experiment, Eur. J. Soil Sci., 66, 427–437, https://doi.org/10.1111/ejss.12234, 2015.
Toczydlowski, A. J. Z., Slesak, R. A., Kolka, R. K., and Venterea, R. T.: Temperature and water-level effects on greenhouse gas fluxes from black ash (Fraxinus nigra) wet land soils in the Upper Great Lakes region, USA, Appl. Soil Ecol., 153, 103565, https://doi.org/10.1016/j.apsoil.2020.103565, 2020.
Tripati, A. K., Roberts, C. D., and Eagle, R. A.: Coupling of CO2 and Ice Sheet Stability Over Major Climate Transitions of the Last 20 Million Years, Science, 326, 1394–1397, https://doi.org/10.1126/science.1178296, 2009.
Van Dijk, A. I. J. M., Beck, H. E., Boergens, E., de Jeu, R. A. M., Dorigo, W. A., Frederikse, T., Güntner, A., Haas, J., Hou, J., Preimesberger, W., Rahman, J., Rozas Larraondo, P. R., and van der Schalie, R.: Global Water Monitor 2023, Summary Report, Global Water Monitor, http://www.globalwater.online (last access: 29 September 2024), 2023.
Wang, H., Liao, G., D'Souza, M., Yu, X., Yang, J., Yang, X., and Zheng, T.: Temporal and spatial variations of greenhouse gas fluxes from a tidal mangrove wetland in Southeast China, Environ. Sci. Pollut. Res., 23, 1873–1885, https://doi.org/10.1007/s11356-015-5440-4, 2016.
Yu, X., Yang, X., Wu, Y., Peng, Y., Yang, T., Xiao, F., Zhong, Q., Xu, K., Shu, L., He, Q., Tian, Y., Yan, Q., Wang, C., Wu, B., and He, Z.: Sonneratia apetala introduction alters methane cycling microbial communities and increases methane emissions in mangrove ecosystems, Soil Biol. Biochem., 144, 107775, https://doi.org/10.1016/j.soilbio.2020.107775, 2020.
Zeebe, R. E. and Wolf-Gladrow, D.: CO2 in Seawater: Equilibrium, Kinetics, Isotopes, Gulf Professional Publishing, 382 pp., ISBN 9780080929903, 2001.
Zhu, X., Sun, C., and Qin, Z.: Drought-Induced Salinity Enhancement Weakens Mangrove Greenhouse Gas Cycling, J. Geophys. Res.-Biogeo., 126, e2021JG006416, https://doi.org/10.1029/2021JG006416, 2021.