the Creative Commons Attribution 4.0 License.
the Creative Commons Attribution 4.0 License.
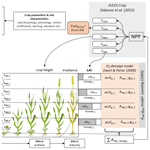
Development of the DO3SE-Crop model to assess ozone effects on crop phenology, biomass, and yield
Sam Bland
Nathan Booth
Zhaozhong Feng
Lisa Emberson
A substantial body of empirical evidence exists to suggest that elevated O3 levels are causing significant impacts on wheat yields at sites representative of highly productive arable regions around the world. Here we extend the DO3SE model (designed to estimate total and stomatal O3 deposition for risk assessment) to incorporate a coupled Anet–gsto model to estimate O3 uptake; an O3 damage module (that impacts instantaneous Anet and the timing and rate of senescence); and a crop phenology, carbon allocation, and growth model based on the JULES-crop model. The model structure allows scaling from the leaf to the canopy to allow for multiple leaf populations and canopy layers. The DO3SE-Crop model is calibrated and parameterised using O3 fumigation data from Xiaoji, China, for the year 2008 and for an O3-tolerant and sensitive cultivar. The calibrated model was tested on data for different years (2007 and 2009) and for two additional cultivars and was found to simulate key physiological variables, crop development, and yield with a good level of accuracy. The DO3SE-Crop model simulated the phenological stages of crop development under ambient and elevated O3 treatments for the test datasets with an R2 of 0.95 and an RMSE of 2.5 d. The DO3SE-Crop model was also able to simulate O3-induced yield losses of ∼11 %–19 % compared to observed yield losses of 12 %–34 %, with an R2 of 0.68 (n=20) and an RMSE of 76 g m−2. Additionally, our results indicate that the variance in yield reduction is primarily attributed to the premature decrease in carbon assimilation to the grains caused by accelerated leaf senescence, which is brought forward by 3–5 d under elevated O3 treatments.
- Article
(5520 KB) - Full-text XML
-
Supplement
(1566 KB) - BibTeX
- EndNote
Ground-level ozone (O3) is considered the most critical air pollutant causing global damage to agricultural crops. Elevated O3 concentrations are particularly problematic in Asia, where decades of rapid economic growth, industrialisation, and urbanisation have seen sharp rises in pollutant emissions associated with burning fossil fuels (Lin et al., 2017), causing substantial O3-induced crop yield losses across the region (Z. Feng et al., 2022). At the same time, climate change is considered a substantial threat to arable productivity through changes in average and extreme temperature and precipitation profiles across the region (IPCC, 2021). Reductions in precipitation are considered responsible for poor harvests in recent years (Liu et al., 2010), and rising temperatures that reduce the length of the crop growing season are thought to have caused losses in crop yield (Malhi et al., 2021). There is now substantial evidence showing that stresses from O3 pollution and climate variability interact, causing either additive, synergistic, or antagonistic responses in crop development, growth, and yield (Sillmann et al., 2021). The threat posed by these stresses is a particular cause for concern in Asia since the continent contributes approximately 43 % of the global wheat production, with China contributing the highest production levels at 17 % of the global wheat supply (Feng et al., 2021). O3 levels are rising substantially in important wheat-growing areas in China such as the North China Plain and the Yangtze River Delta (Li et al., 2020; Zhang et al., 2023). Concern over O3 impacts led to the implementation in 2013 of a range of policies to try to reduce O3 precursor emissions across China. These included a comprehensive management plan to control volatile organic compounds (VOCs) from key industries, an atmospheric pollution prevention and control law of the People's Republic of China, and a 2020 VOC management plan (Li et al., 2021). As a result, nitrogen oxide (NOx) emissions, an important O3 precursor, have decreased by 21 % from 2013 to 2017 (Li et al., 2021). By contrast, VOCs have only slightly decreased by 2 % over the same period. Since China has a VOC-limited O3 regime, the reductions in NOx lead to rather insignificant changes in O3 concentration (Li et al., 2021), though evidence suggests that reductions in O3 may be higher in rural areas than in urban areas (Lee et al., 2020). This implies future policies to tackle ground-level O3 pollution in China need to increase their focus on reducing VOCs along with NOx (Lee et al., 2020) and also emphasise the importance of being able to make assessments of O3 damage to key receptors such as staple crops.
At present, methods to assess the risk to crop productivity from changes in O3 and climate variables use a variety of different O3 risk assessment methods (Ronan et al., 2020) and crop models as discussed in depth in Emberson et al. (2018). In the past, O3 risk assessment methods relied heavily on dose–response relationships, empirically derived relationships that assess changes in a response variable (most commonly yield) against an O3 exposure metric (concentration or, more recently, flux-based indices) (Pleijel et al., 2022). By contrast, methods to assess the impact of climate variables (most commonly changes in temperature, precipitation, and CO2 concentration) tend to use crop models since these allow the integration of the combined effect of a number of different variables acting simultaneously to affect crop development, growth, and yield (Schauberger et al., 2019). A new generation of crop models that include O3 damage are now being developed and applied and have the potential to estimate the combined effect of O3 and climate variables on crop development, biomass, and yield. Such models can arguably be classified into two types of crop model: firstly, those that rely on O3 metrics (e.g. AOT40 or M7) to modify crop growth determined by radiation use efficiency (Guarin et al., 2019, 2024) or evapotranspiration (Droutsas et al., 2020); secondly, those that estimate stomatal O3 uptake to modify crop growth determined by photosynthesis and subsequent carbon assimilation (Tao et al., 2017; Schauberger et al., 2019; Nguyen et al., 2024). The DO3SE-Crop model falls into the latter category of photosynthesis-based crop models and was developed to bridge the gap between O3 risk assessment modelling methods and crop models.
The DO3SE model is an O3 deposition model that can be embedded within atmospheric chemistry transport models (e.g Simpson et al., 2012) and uses either a multiplicative or coupled Anet–gsto model to estimate stomatal O3 flux (Pande et al., 2024). The accumulated stomatal O3 flux has been successfully used as a damage metric (PODy – phytotoxic ozone dose over a threshold y; LRTAP, 2017) to predict O3-induced yield loss (Pande et al., 2024). The ability of the DO3SE model to simulate Anet, as well as the inclusion of a process-based O3 damage module for both instantaneous Anet and early and enhanced senescence (after Ewert and Porter, 2000), lends itself to the development of the DO3SE model as a process-based crop model. The inclusion of resistance algorithms that can assess the transport of O3 concentrations from a reference height above a canopy down to the canopy top means the model can be embedded within existing atmospheric chemistry transport schemes and hence applied to regional- or global-scale O3 risk assessment whilst also modelling O3 deposition. A comparison of the coupled stomatal Anet–gsto model with the multiplicative gsto model within the DO3SE framework was made in Pande et al. (2024), and it showed that the Anet–gsto model performed equally well, if not better, when used to develop O3 dose–response relationships for European wheat. This provides evidence of the suitability of the new photosynthesis-based gsto model in DO3SE.
In this study, we describe the development of a new DO3SE-Crop model which builds on the modified stomatal deposition component of the DO3SE model (Pande et al., 2024) so that both CO2 uptake for carbon assimilation and O3 uptake via the stomata can be modelled consistently. Further, we have incorporated the UK JULES-crop model (Osborne et al., 2015) to allocate assimilated carbon to plant components (roots, leaves, stems, and harvest organs) according to crop development stage. We also take account of the modifying effect of O3 on instantaneous Anet as well as accumulated Anet via O3 effects on the onset and rate of leaf senescence and timing of crop maturity through incorporation of algorithms developed by Ewert and Porter (2000). The UK JULES-crop model is used since this is the UK land surface exchange scheme in the UK Earth System Model (UKESM) (Osborne et al., 2015) which has recently been developed to include exchange and impact of trace gases (including O3) along with other biogeochemical cycling between the atmosphere and the land surface (Leung et al., 2020). This would in the future allow comparison of the UK JULES-crop model, which uses O3 mechanisms that modify instantaneous Anet to mimic changes in yield consistent with flux–response relationships (Sitch et al., 2007), with the alternative O3 damage mechanisms used within DO3SE-Crop.
Here, we calibrate and evaluate the DO3SE-Crop model using an experimental FACE dataset collected in Xiaoji, China. This allows us to investigate the ability of the model to simulate O3 damage for a comparable agro-ecological region where crop productivity is severely threatened by both O3 pollution and climate change. The key objectives of the paper are to assess the ability of DO3SE-Crop to simulate (i) key phenological stages, (ii) the relationship between leaf-level physiological variables and within-canopy O3 concentrations, (iii) C allocation to different parts of the crop, and (iv) O3-induced yield losses for tolerant and sensitive cultivars.
2.1 DO3SE-Crop model
Here we describe the development of the DO3SE-Crop model. In this study, version 4.39.16 of the DO3SE-Crop model was used (Bland, 2024) for wheat (Triticum aestivum), which is widely considered to be one of the most sensitive staple crops to O3 (Feng et al., 2018). The key components of DO3SE-Crop are illustrated in Fig. 1. The model integrates meteorological data, crop parameters, and site characteristics to simulate the impact of O3 on crop yield. Model inputs are irradiance, temperature, relative humidity, precipitation, air pressure, wind speed, and O3 concentration at a reference height (Cz) to calculate atmospheric resistances (Ra) and boundary layer resistances (Rb) for O3 deposition to the crop canopy. It further incorporates crop-specific parameters related to leaf physiology, phenology, and carbon coefficients, alongside site-specific data (latitude, longitude, and elevation) to simulate crop growth at stages from sowing to maturity, denoted by the development index (DVI). The canopy is divided into four vertical layers, each characterised by the sunlit leaf area index (LAIsun) and the shaded (LAIsh) leaf area index, which influence the photosynthetic capacity (Vcmax) and O3 uptake in each layer. The model accounts for in-canopy resistance (rinc) and external resistance (rext) in each layer, affecting the O3 flux (accfst) and its impact on net photosynthesis (Anet) and stomatal conductance (). The Anet–gsto relationship is modelled using the Leuning (1995) model. Damage from O3 is estimated after Ewert and Porter (2000) for different canopy layers, which are aggregated to give the overall O3 impact on canopy Anet, which is integrated according to the JULES-crop model (Osborne et al., 2015), which uses the daily accumulated canopy Anet to calculate the net primary productivity (NPP). The NPP is then distributed as carbon to various parts of the crop (roots (Croot), stems (Cstem), leaves (Cleaf), harvestable organs (Charv)). The Charv provides the yield and grain dry matter, Cleaf provides the LAI, and Cstem provides the crop height. The DO3SE-Crop model requires hourly input meteorological and O3 concentration data which are used to produce output on either an hourly (i.e. leaf physiology and short-term O3 damage variables) or daily (i.e. phenology, soil moisture, long-term O3 damage, C allocation, biomass, and yield variables) time step.
2.2 DO3SE-Crop phenology
The DO3SE-Crop model uses thermal time to define the rate of crop development in relation to the timing of three key developmental stages, TTemr (the period from sowing to emergence), TTveg (the period of emergence to start of grain filling), and TTrep (the period from the start of grain filling to maturity), based on the method of Osborne et al. (2015). Thermal time is calculated by accumulating an effective temperature (Teff) using base (Tb), optimum (To), and maximum (Tm) cardinal temperatures as shown in Eq. (1):
where Tair is the surface air temperature in °C, and Teff is at a maximum when Tair=To; this point denotes the highest developmental rate. Teff declines as the temperature falls or rises above To, with a linear decrease in crop development. Teff is zero, i.e. no development, when Tair falls below or rises above Tb and Tm, respectively; i.e. . During the sowing to emergence phase, development is dependent on Tb, whereas during the vegetative and reproductive phase, development depends on Tm or To.
Winter wheat requires vernalisation (a period of exposure to low temperature during germination to accelerate flowering). Vernalisation alters the length of TTveg and hence flowering initiation, with subsequent effects on later growth stages such as heading. Vernalisation occurs when the minimum (VTmin) and maximum (VTmax) daily temperature is less than 15 and 30 °C, respectively (Zheng et al., 2015). Accumulated vernalised days (Vdd) are calculated as the sum of vernalised and devernalised days from emergence to the start of anthesis (Zheng et al., 2015) as shown in Eq. (2):
where for VTmax <30 °C and VTmin<15 °C, for VTmax>30 °C, and Vdd<10 d.
The vernalisation factor (VF) decreases from 1 to 0 as (Vdd) increases. VF depends on a cultivar-specific vernalisation coefficient (PIV) as described by Eq. (3):
Photoperiod (PP) or day length also affects the occurrence and timing of the flowering stage and is calculated according to latitude using standard solar geometry to estimate day length (Jones, 1992). The photoperiod factor (PF) represents the sensitivity to PP, which decreases from 1 to 0 as the photoperiod shortens and is estimated according to a cultivar-specific photoperiod coefficient (PID) after Tao et al. (2012) as described in Eq. (4):
Crop development is related to the development index (DVI) after Osborne et al. (2015), which takes values of −1 upon sowing, 0 on emergence, 1 at anthesis, and 2 at crop maturity. The DO3SE-Crop model DVI equations have been modified from Osborne et al. (2015) to take account of the photoperiod and vernalisation for winter wheat (see Eq. 5); for spring wheat these factors are omitted:
DO3SE-Crop allows for any number of representative leaf populations (pop) and canopy layers (n) to be defined over the course of the crop growing season by dividing leaf populations as they emerge evenly across the canopy layers defined by LAI. In this study, we used a single leaf population and four canopy layers (i.e. pop =1; n=4) for simplicity. The crop sowing is assumed to be at DVI (start of TTemr) and emergence at DVI =0 (start of TTveg). The flag leaf is assumed to develop at DVI =1, at the commencement of TTrep, marking the initiation of anthesis (Astart flowering) and flag leaf emergence, which typically occurs 4–5 d prior to the onset of anthesis and is further divided into expanding and senescing leaf periods (i.e. tlep and tlse) with a default ratio of 0.67 to 0.33 for each of these periods. Maturity is assumed at DVI =2, at the end of TTrep. The model allows estimation of the PODy metric by accumulating stomatal O3 flux from the start of anthesis to maturity. The total canopy leaf life span (TTleaf) of the crop is distributed over the DVI between 0 and 2. The total lifespan (Tl) covers the full period from sowing to maturity, corresponding to DVI between −1 and 2. The relationship between these different variables is described in Fig. 2.
2.3 DO3SE-Crop leaf-level physiology
Key leaf-level physiological variables of the DO3SE-Crop model are Anet and gsto. Net photosynthesis is simulated using the biochemical photosynthesis-based model initially developed by Farquhar et al. (1980) and since modified by Sharkey et al. (2007). The coupled Anet–gsto model of Leuning (1995) is used to estimate gsto from Anet, which means that gsto is regulated by the demand of CO2 for Anet on consideration of environmental conditions and crop physiology. Ozone stress, causing both instantaneous effects on Anet and long-term effects on Anet via leaf senescence, is simulated based on algorithms developed by Ewert and Porter (2000).
2.3.1 Leaf net photosynthesis (Anet)
The Anet model assumes that photosynthesis is constrained depending on prevailing environmental conditions according to three main mechanisms: rubisco activity (Ac); ribulose-1,5-bisphosphate (RuBP) regeneration, which is constrained by the speed of electron transport (Aj); and the low rate of transfer of photosynthetic products (most frequently triose phosphate consumption) (Ap) (Sharkey et al., 2007) and by soil water stress (fPAW). The algorithm for Ac, which is based on Medlyn et al. (2002) and modified in DO3SE-Crop to include the O3 damage functions, is given in Eq. (6):
where Vcmax (µmol CO2 m−2 s−1) is the maximum carboxylation capacity at 25 °C, Ci (µmol mol−1) and Oi (mmol mol−1) are the intercellular CO2 and O2 partial pressures, Kc (µmol mol−1) and K0 (mmol mol−1) are the rubisco Michaelis–Menten constants for CO2 and O2, Γ∗ (µmol mol−1) is the CO2 compensation point in the absence of respiration, fO3,s(d) is the factor that accounts for the cumulative stomatal O3 flux effect on Vcmax over the course of a day, and fLS is the factor that accounts for the cumulative stomatal O3 flux effect over the course of a leaf life span on leaf senescence. Section 2.3.2 gives a full description of the methods used to estimate O3 damage. The fPAW factor is calculated by Eq. (7):
PAW is the amount of water in the soil (in % terms) which is available to the plant estimated according to the DO3SE models' single-soil-layer bucket model (Büker et al., 2012). At PA =100 % the soil is at field capacity; at PAW the soil is at wilting point. PAWt is the threshold PAW, above which it is assumed there is no constraint on Ac, defined as 50 % after LRTAP (2017). Only once PAW<PAWt will soil water begin to limit gsto and hence stomatal O3 flux.
The constraint on photosynthesis due to the rate of electron transport Aj is described in Eq. (8):
where J is the electron transport rate (µmol CO2 m−2 s−1), and the parameters a and b denote the electron requirements for the formation of NADPH and ATP, respectively (Sharkey et al., 2007)
Finally, the photosynthesis limitation due to the low rate of transfer of photosynthetic products Ap (µmol CO2 m−2 s−1) is given in Eq. (9):
The leaf net photosynthesis (Anet) in µmol CO2 m−2 s−1 is calculated by Eq. (10):
where leaf dark respiration (Rd) in µmol CO2 m−2 s−1 is calculated as Vcmax×Rdcoeff, where Rdcoeff is the leaf dark respiration coefficient initially set equal to 0.015 after Clark et al. (2011) – a value provided for C3 grasses.
2.3.2 Short- and long-term O3 damage to Ac
The short-term impact of O3 on Ac is calculated according to the fO3,s(d) factor (between 0 and 1), which allows for an instantaneous effect of O3 on photosynthesis when stomatal O3 flux (fst), in nmol O3 m−2 s−1 and calculated as described later in Sect. 1.2.3, overwhelms detoxification and repair mechanisms (Betzelberger et al., 2012; Y. Feng et al., 2022) and is estimated following Ewert and Porter (2000). Here, represents the relationship between fst and a potential decrease in Ac calculated for every hour of the day by Eq. (11):
where γ1 (dimensionless) and γ2 (nmol O3 m−2 s−1)−1 are both short-term O3 damage coefficients, with γ1 representing the O3 detoxification threshold below which no damage occurs to the photosynthetic system and γ2 determines the effect of fst on Ac once this detoxification threshold is exceeded; fO3,s(d) and (i.e. fO3,s(d) at the end of the previous day) are calculated by Eq. (12),
where (dimensionless) represents incomplete recovery from O3 overnight, which depends on leaf age according to Eq. (13),
The long-term impact of O3 on Vcmax represented by the fLs term represents the longer-term accumulation of stomatal O3 flux (accfst), causing degradation to the rubisco enzyme, which triggers early and enhanced senescence of mature leaves (Gelang et al., 2000; Osborne et al., 2019). The accfst term is accumulated from 200 °C days before anthesis until maturity to be consistent with LRTAP (2017), which defines this as the O3-sensitive period for wheat. The simulation of fLs (and fLA used in the short-term O3 effect) is related to thermal-time-defined periods over the course of a leaf population life span TTleaf as described in Fig. 2.
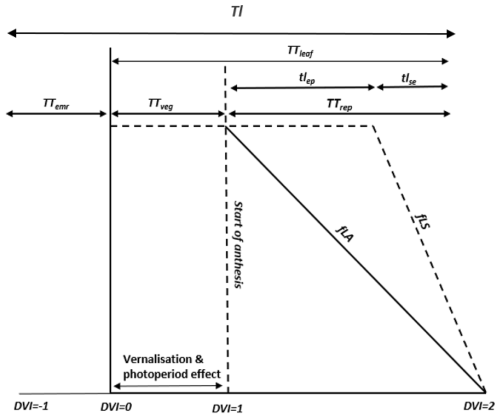
Figure 2The division of thermal-time-defined periods (TTemr, TTveg, TTrep, and TTleaf and the relationship with fLA and fLs) for the canopy, as represented in this study by a single leaf population.
The O3 effect on fLs is first simulated by estimating a weighted accumulated fst () modified from Ewert and Porter (2000) by Eq. (14):
where γ3 determines the occurrence of senescence once a critical cumulative stomatal O3 flux CLsO3 (in mmol m−2) has been exceeded. The rate of senescence is determined by γ4, which determines the onset of senescence, and γ5, which determines maturity as described in Eq. (15):
where tlep is the thermal time accumulated by a leaf (LTT) in °C days between a fully expanded leaf and the start of leaf senescence, is tlep with an O3 effect which may bring senescence earlier, tlse is the LTT in °C days between the onset of senescence and maturity, and is tlse with an O3 effect which may bring maturity earlier. fLs is estimated by Eq. (16):
2.3.3 Stomatal conductance (gsto)
The coupled photosynthesis–stomatal-conductance (Anet–gsto) model based on Leuning (1995) and modified for vapour pressure deficit (VPD) is used to estimate and stomatal conductance to CO2 in µmol CO2 m−2 s−1 as described in Eq. (17),
where fmin (µmol m−2 s−1) is the minimum daytime (Leuning, 1990). The parameter m (dimensionless) is the composite sensitivity of to assimilation rate and vapour pressure deficit (VPD), with the relationship between VPD and relative stomatal conductance (fVPD) estimated by Eq. (18),
where VPD0 is an empirical parameter, defined using boundary line analysis, describing the variation in relative stomatal conductance with VPD (Danielsson et al., 2003; Pleijel et al., 2007). cs (mmol mol−1) is the external CO2 concentration at the leaf surface and is calculated from the external CO2 concentration at the upper surface of the leaf boundary layer ca (mmol mol−1) so that after Masutomi (2023), where is the boundary layer conductance to CO2 (in mol m−2 s−1), and conversion factors for gases and heat across the boundary layer are given in Sect. S1a.
Finally, is converted to in mmol O3 m−2 s−1 by dividing by 1000 and using the conversion factor 0.96, which assumes that the ratio of the diffusivities of gases in air is equal to the inverse of the square root of the ratio of molecular weights (as described in Campbell and Norman, 1998); see also Sect. S1b).
2.3.4 Stomatal ozone flux (fst)
Stomatal [O3] flux (fst in nmol m−2 s−1) is calculated after the method described in the UNECE mapping manual (LRTAP, 2017) described in Eq. (19):
where Cl is the [O3] at the upper surface of the laminar layer of a leaf (nmol O3 m−3). Ozone concentration in ppb can be converted to nmol m−3 by multiplying O3 in ppb by P/(, where P is the atmospheric pressure (1.013×105 in Pascal), R is the universal gas constant (8.31447 J mol K−1), and Tair,k is surface air temperature in °K. To convert (mol O3 m−2 s−1) to (m s−1) we assume a standard temperature (20 °C) and P divided by 41 to give the conductance value in m s−1. The term represents the O3 deposition rate to the leaf through resistances rb (the quasi-laminar resistance (s m−1)) and rc (the leaf surface resistance (s m−1)), which allow for both stomatal and non-stomatal deposition to the leaf surface. rc is , where gext is (s m−1). is estimated by Eq. (20):
where the factor 1.3 accounts for the differences in diffusivity between heat and O3 (see Sect. S1a), L is the cross-wind leaf dimension (m), and ul is the wind speed (m s−1) at the top of the leaf laminar boundary layer. The leaf boundary layer resistance to CO2 is estimated using a value of 1.24 for the difference between heat and CO2 in place of the 1.3 value for O3 (Campbell and Norman, 1998).
2.4 DO3SE-Crop canopy
The DO3SE-Crop model uses a multi-layer approach to scale from leaf to the canopy. We assume that wind, irradiance, [O3] concentration, and leaf nitrogen content are the key environmental conditions which change with the cumulative canopy leaf area index (LAI) and influence leaf physiology and therefore canopy layer estimates of Anet, , and gext; other environmental variables (e.g. Tair and VPD) are assumed to remain constant over the canopy.
2.4.1 Canopy irradiance
Changes in irradiance through the canopy are described as sunlit and shaded canopy fractions and the associated quantity of direct and diffuse photosynthetically active radiation (PAR, W m−2); these are estimated according to increasing levels of cumulative LAI using the methods of (de Pury and Farquhar, 1997); full details are given in the Sect. S2. Application of this method requires the canopy to be divided into layers of equal LAI, including both green LAI (LAIG) and brown (LAIB) LAI.
PAR absorbed per unit leaf area is divided into PARdir and PARdiff, which also includes scattered (re-reflected by the canopy) beam calculated by
where PARdir is the absorbed beam plus scattered beam PAR (photosynthetically active radiation) per unit leaf area, PARdiff is the absorbed diffuse plus scattered diffuse PAR per unit leaf area, ρcb is the canopy reflection coefficient for beam PAR, ρcd is the canopy reflection coefficient for diffuse PAR, is the beam and scattered beam PAR extinction coefficient, is the diffuse and scattered diffuse PAR extinction coefficient, β is the solar elevation above the horizontal plane of the Earth's surface, Ib(0) is beam PAR per unit ground area at the top of the canopy, and Id(0) is diffuse PAR per unit ground area at the top of the canopy.
Estimates of the LAI fractions of sunlit (LAIsun) and shaded (LAIsh) parts of each canopy layer (i) are made by Eqs. (23) and (24):
where β is the solar elevation angle (see Sect. S3), and
The DO3SE-Crop model simulates LAI as part of the crop growth model, and LAI is assumed to be evenly distributed across all layers (see Sect. 1.4.2 and Eq. 43).
Therefore, PAR for the sunlit part of each layer (PARsun) can be described as
where PARsh is absorbed PAR by shaded leaves per unit leaf area; PARbsun is beam PAR absorbed by sunlit leaves per unit leaf area; and can be written as and , where αl is angle of irradiance beam on the leaf normal and σ is the leaf scattering coefficient for PAR.
Similarly, PAR for the shaded part of each layer (PARsun) can be described as
where can be written as , is , and PARbs (LAI) is absorbed scattered beam PAR per unit leaf area.
2.4.2 Canopy [O3] concentration
O3 concentration will vary as a function of O3 loss to the canopy (i.e. deposition via the stomates and external plant parts) and O3 replacement from ambient air concentrations above the canopy. Limited data have been collected showing how O3 concentrations vary with canopy depth in semi-natural communities (Jaggi et al., 2006). These data suggest that a minimum, bottom of canopy O3 concentration (Czb) is about 0.2 times that at the top of the canopy (Czh) and that the O3 concentration difference within the canopy is closely related to the LAI of the canopy layers.
Since each canopy layer can be assumed to be a parallel sink, the O3 flux to a layer depends on the conductance (inverse of resistance) of that layer and the O3 concentration at the top of the layer (Ci; with C0 being Czh (i.e. the O3 concentration at height Ch, the top of the canopy)); we follow and generalise the work of Waggoner (1971) by separating the canopy into nL leaf layers. We calculate the O3 concentration for each layer, Ci, from O3 intake, Ii, by
with rc,i the leaf surface resistance to O3 for layer i. Ii is calculated as the solution to a system of linear equations. The in-canopy aerodynamic resistance for layer i is described in terms of rc,i, Ii, and the resistances of the bulk air among the leaves (Ri). Assuming a uniform O3 concentration C0 above the canopy, we use generalised equations from Waggoner (1971) to calculate the difference in O3 concentration between the exterior air and the leaf interior. For the top layer, this difference is C0 minus 0, while for each lower layer, the difference decreases progressively depending on the resistances and fluxes within the canopy. This O3 concentration difference is calculated by
for the top canopy layer,
for each canopy layer i between the top layer and the bottom layer, and
for the bottom layer of the canopy between the lowest leaf layer and the ground. These can also be written into the matrix form
which can be numerically solved for Ix when and R1≠0.
Resistances for each layer are calculated as described in the Supplement (Sect. S5) using standard DO3SE deposition modelling methods (Emberson et al., 2000, 2001).
2.4.3 Canopy maximum carboxylation capacity (Vcmax)
We allow for an exponential decrease in leaf N with canopy depth, which will influence both the photosynthetic capacity (Vcmax) and dark respiration (Rdc). Photosynthetic capacity at each canopy layer i is calculated by Eq. (30):
where ne (mol CO2 m−2 s−1 kg C (kg N)−1) is a constant relating leaf nitrogen to rubisco carboxylation capacity, n0 (kg N[kg C]−1) is the leaf N concentration at the top of the canopy, and kN is a nitrogen profile coefficient initially set at 0.78 after (Clark et al., 2011). The model assumes non-limiting conditions for soil nitrogen, in accordance with the experimental data.
2.4.4 Canopy photosynthesis (Anetc)
Net canopy photosynthesis (Anetc) determines the amount of C assimilated by the entire canopy that can subsequently be allocated to different plant parts (i.e. less than the C respired for plant growth and maintenance; see Sect. 1.4.1); the amount of C assimilation will ultimately determine whole-plant biomass. The net photosynthesis for each canopy layer (Aneti) is calculated according to the LAI fraction of that layer that is sunlit (LAIsun,i) and shaded (LAIsh,i) within the layer (i), multiplied by the net photosynthesis of the sunlit (Anet) and shaded leaf (Anet), respectively, described by Eqs. (31) and (32):
with Anetc calculated by
Anetc is converted from µmol CO2 m−2 s−1 to kg C m−2 d−1 by multiplying by 3600 (converting from seconds to hours), multiplying by 1.2 (representing the kg of C per mol), and summing each hourly Anetc over the course of a day. This Anetc is used in the Eq. (37).
2.4.5 Canopy stomatal conductance ()
Similarly, canopy layer (i) stomatal conductance to O3 () is converted from by assuming a diffusivity ratio of 0.96 to convert from CO2 to O3 and is calculated by Eq. (33) with whole-canopy stomatal conductance calculated by Eq. (34):
This is converted from in Eq. (33) by dividing the conductance value in mmol m−1 s−1 by 41 000 (assuming standard temperature (20 °C) and air pressure (1.013×105 Pa)) to give conductance in m s−1.
2.5 Crop biomass, LAI, height, and yield variables
The following section describes how to estimate crop biomass, important canopy characteristics (LAI and crop height (h)), and yield variables from accumulated calculations of Anetc over the course of the growing season following (Osborne et al., 2015).
2.5.1 Crop biomass (NPP and GPP)
The simulation of crop growth requires an estimate of the net primary productivity (NPP) which is calculated at the end of each day and summed over the growing season. Carbon is assumed to be allocated to five key crop components: root, leaf, stem, harvest, and reserve pools (Osborne et al., 2015). This carbon allocation is ultimately used to simulate leaf area index (LAI), canopy height (h), biomass, harvest index, and yield at the end of each day throughout the growing season.
NPP (kg C m−2 d−1) is accumulated throughout the day using the JULES-crop approach to model crop growth (Osborne et al., 2015) described in Eq. (35),
where GPP is the gross primary productivity (kg C m−2 d−1) and Rp is plant respiration divided into maintenance (Rpm) and growth (Rpg) respiration (kg C m−2 d−1) (Clark et al., 2011), where and where Rpg is assumed to be a fixed fraction of the NPP as shown in Eq. (36),
where Rgcoeff is the growth respiration coefficient which was initially set to 0.25 based on the value for all PFTs (i.e. forests and grasses including crops) in Clark et al. (2011). GPP is calculated by Eq. (37):
where Anetc is net canopy photosynthesis (see Eq. 28) and fPAWRdc is the soil-moisture modified canopy dark respiration (kg C m−2 d−1), where , with Rdcoeff initially assumed to be 0.015 based on Clark et al. (2011); Vcmax,i is the maximum carboxylation efficiency for each canopy layer i, which decreases from the top to bottom of the canopy (see Eq. 30); and fPAW is calculated in Eq. (7).
Leaf maintenance respiration (Rpm) is assumed to be equivalent to the soil moisture modified canopy dark respiration, while root and stem respiration are assumed to be independent of soil moisture but to have the same dependencies on C content. We assume a fixed relationship between C and N contents of these organs so that Rpm can be estimated by Eq. (38):
The C accumulating as NPP each day is divided into five carbon pools, i.e. root (Croot), leaf (Cleaf), stem (Cstem), reserve (Cresv), and harvest (Charv) (kg C m−2 d−1), according to partition coefficients (see Eq. 39) allowing for accumulation of C in these pools over the course of the crop growth period:
where τ is the fraction of stem C that is partitioned into the reserve pool. prootpleaf, pstem, and pharv=1. The partition coefficients are related to the crop development stage (DVI) and hence effective thermal time (TTeff) since emergence. The partition coefficients are based on Osborne et al. (2015) and provided as a function of DVI using six parameters to continuously describe varying partition coefficients over the duration of the crop growing season. We use the same multinomial logistic as that described in Osborne et al. (2015) to define this function according to Eq. (40):
where DVI is the development index, and α and β are partition parameters. These parameters describe the shape of the thermal-time varying partition coefficient for leaves, roots, and stems.
Once C is no longer partitioned to stems, C from the stem reserve pool will mobilise to the harvest pool at a rate of 10 % per day following Osborne et al. (2015) and described by Eq. (41):
Total leaf C is divided between green leaf C (Cleaf, green) and brown leaf carbon (Cleaf, brown). Carbon from the Cleaf, green will mobilise to the harvest pool at a rate of 5% per day after Osborne et al. (2015) and to the Cleaf, brown at a rate of 24 % per day once fLS >1 as described in Eq. (42):
2.5.2 Leaf area index (LAI) and stem height (h)
At the end of each day, the C content of the stem and leaf is used to estimate LAI by Eqs. (43) and (44):
The values Υ and δ were determined by fitting the values to the paired values of DVI and specific leaf area (SLA). The value of fc is 0.5 (unitless) and denotes the carbon fraction of dry matter.
The amount of C in the stem is used to calculate the crop height h in metres by Eq. (45):
where k and λ were determined by fitting the value Cstem and h.
2.5.3 Yield variables
According to Osborne et al. (2015) yield can be calculated from the C allocated to the harvest pool (Charv) at the end of the growing season as described in Eq. (46):
where harvested C is converted to total biomass (using the conversion factor fc=0.5), i.e. by multiplying the harvested C by and then by 1/0.84 (Dw) to account for the grain moisture content (Mulvaney and Devkota, 2020). Charv includes both chaff and grain; however, O3 fumigation experimentalists tend to only include grain when calculating total crop yield at the end of the growing season, so we assume 15 % of the yield is chaff and include a grain-to-ear ratio, Eg, of 0.85. Dividing by 1000 converts yield from kg C m−2 to g C m−2, the unit most often used to describe experimental yield results.
Evaluation of the DO3SE-Crop model uses a variety of growth dry matter (DM) metrics. Some of the most important metrics and their calculations are “straw DM”, which is calculated as the sum of carbon allocated to Cstem, Cleaf, and Cresv; “ear DM”, which is calculated from Charv excluding the moisture content (Dw) conversion; “grain DM”, which is calculated from Charv excluding both the moisture content (Dw) conversion and removing the chaff fraction conversion Eg; “above-ground DM”, which is the straw DM plus the ear DM; “below-ground DM”, which is converted from Croot; and “harvest index”, which is the grain DM divided by the above-ground DM. In all cases the fc conversion factor is used to convert from, for example, g C m−2 to g DM m−2.
3.1 Xiaoji China experimental dataset
The DO3SE-Crop model was used to analyse the O3-FACE (Free Air Concentration Enrichment) experimental data collected in Xiaoji, Jiangdu, Jiangsu Province, China. The wheat crop was grown in fully open-air field conditions for three consecutive growing seasons from 2007 to 2009. The dataset includes four modern cultivars of winter wheat (Triticum aestivum L.) grown under ambient (AA) and elevated (E) O3, with the elevated treatment being, on average, 25 % above the ambient O3 concentrations from early March/April to the end of May each year. The four cultivars were Yannong 19 (strong-gluten wheat, hereafter Y19), Yangmai 16 (medium-gluten wheat, hereafter Y16), Yangmai 15 (weak-gluten wheat, hereafter Y15), and Yangfumai 2 (weak-gluten wheat, hereafter Y2) (Zhu et al., 2011).
Soil water availability was sufficient for optimum wheat crop growth, so we assumed there was no soil moisture stress (Feng et al., 2012). Any data gaps were filled following the AgMIP-O3 gap-filling protocol (see Sect. S4). For large O3 data gaps (i.e. greater than 2 weeks) occurring outside the O3 fumigation period, we used scaled WRF-Chem (version 4.2) data for Xiaoji (Conibear et al., 2018) to ensure consistency in model calibration and potential applications across China. The dataset provides grain yield components, including the number of ears per square metre, the number of grains per ear, and the grain dry matter (grain DM, in g m−2) (Feng et al., 2011, 2016). Additional physiological datasets (i.e. Anet, Vcmax, Jmax, and (converted to as described in Sect. S1b)) are also provided, but only for the year 2008 for all cultivars (Y2, Y19, Y15, and Y16) and for the flag leaf. The 2008 data also include measurements of chlorophyll (in mg m−2) which can be used to assess the level of senescence experienced by the leaf (Mariën et al., 2019). Since the year 2008 also showed significant differences in grain DM between AA and E O3 treatments (a mean relative yield difference of 6.73 for all cultivars; see Table S2b), this year was used to train the DO3SE-Crop model with other years (i.e. 2007 and 2009) used to test the model.
Further experimental details are provided in Feng et al. (2011, 2016). Table 1 describes the average, minimum, and maximum values for all measured variables required to run the DO3SE-Crop model collected at the Xiaoji site for each year. Additionally, the M7 (mean 7 h O3 concentration over the exposure period in ppb) is included for both AA and E O3 treatments. Measurements were taken at a height of 2 m above the ground surface.
3.2 DO3SE-Crop calibration and evaluation
Development and calibration of the DO3SE-Crop model with the Xiaoji experimental dataset followed three main steps: (i) sensitivity analysis to identify key model parameters to calibrate, (ii) calibration of these key parameters for a single year and both tolerant and sensitive cultivars, and (iii) evaluation of key DO3SE-Crop model outputs for different years and cultivars from those used in model calibration.
To perform the sensitivity analysis we used the SALib Python library (Iwanga et al., 2022; Herman and Usher, 2017). The analysis requires ranges to be specified for the parameters (identified by an initial manual calibration) that are included in the sensitivity analysis. For physiological parameters, ranges were determined by considering the range of these parameters in the literature. For carbon allocation parameters, the range was identified by considering the maximum and minimum values of these parameters that would result in appropriate dry matter partitioning within the plant. Once the ranges were identified, the sensitivity analysis was run using the extended Fourier amplitude sensitivity analysis, which has been commonly used by other crop modellers to improve their calibrations (Silvestro et al., 2017; Vazquez-Cruz et al., 2014). From the sensitivity analysis outputs (see Fig. S6), the parameters whose variation contributes the most to variations in selected modelling outputs (in this case photosynthetic rate and yield) were identified as the key model outputs for calibration. Using this method we identified the following DO3SE-Crop parameters as those most important to calibrate: (i) leaf photosynthesis parameters (Vcmax25, Jmax25, kN, m, and VPD0) and (ii) C allocation parameters (αroot, αleaf, αstem, Υ , τ) and related dark respiration coefficients (Rdcoeff and Rgcoeff) which were later included in the calibration after identifying issues with overestimated respiration, likely due to the use of parameter values designed for broad plant functional types, which may not be suitable for wheat. O3 damage module parameters related to senescence (γ3, γ4, γ5, and CLsO3) were not included in the sensitivity analysis, as γ3 and CLsO3 are already recognised as important for calibration, and γ4 and γ5 were introduced in this study to represent the start (SOS) and end (EOS) of senescence, making both essential for calibration. Phenology parameters were also excluded as earlier studies have shown these are relatively straightforward to calibrate using automated methods for a range of environmental conditions (Nguyen et al., 2024). We note that assessing the probability distribution of these ranges would also be useful but consider this outside the scope of the current paper due largely to data limitations.
The DO3SE-Crop model was then calibrated using the 2008 dataset for the Y2 and Y16 cultivars. The year 2008 was selected since this showed a substantial difference in yield of 208 and 148 g m−2 between the AA and EO3 treatments for the Y2 and Y16 cultivars, respectively. These cultivars were chosen since they were identified as the most sensitive (Y2) and tolerant (Y16) cultivars according to the experimental analysis conducted by Feng et al. (2016). See Fig. 5, which shows a diagram representing the calibration process. Calibration of the phenology module used only the Y2 cultivar, AA O3 treatment data describing the timing of emergence, anthesis, and maturity to calibrate key phenology parameters (Tb, T0, Tm, VTmin, VTmax, PIV and PID, TTemr, TTveg, TTrep, and Tl). The phenology calibration was automated by computationally applying a genetic algorithm (Wang, 1997), an optimisation technique with gradient decent to find the best parameters. This uses a combination of crossover strategy (selecting parameters randomly from parameter pairings) and mutation strategy (which takes a parameter range and uses incremental step changes) to identify the parameters which give the highest R2 and lowest root mean square error (RMSE) when compared with observations of the timing (day of year) of anthesis and maturity.
Calibration of the leaf physiology, canopy C allocation, and O3 damage DO3SE-Crop modules was performed manually. This required that an initial value and range be defined for each parameter, which were defined from a combination of observations from the Xiaoji experimental dataset as well as values taken from the literature (see Tables A1 and A2 of the Appendix A for details). The model was manually calibrated until certain conditions were satisfied, as explained below. Calibration of the leaf physiology parameters (Vcmax, Jmax, kN, m, and VPD0) was performed only for the Y2 cultivar, AA O3 treatment whilst keeping all other parameters fixed. This calibration aimed to achieve a maximum Anet value of 30 µmol CO2 m−2 s−1 and a value of 350 mmol O3 m−2 PLA s−1, consistent with the maximum values observed in the Xiaoji dataset (Zhu et al., 2011). We calibrated Vcmax and Jmax as measurements are only provided for Y2 and Y16 cultivars and only for certain points during the growth period, and we know that Vcmax and Jmax can vary seasonally.
Calibration of the C allocation parameters (αroot, αleaf, αstem, Υ, τ) and related dark respiration coefficients (Rdcoeff and Rgcoeff) was also performed keeping all other parameters fixed. This calibration aimed to achieve the following criteria: a stem dry matter to leaf dry matter ratio (RSL) of approximately 2:1 (Huang et al., 2022), relative growth of different plant parts (i.e. leaves, stem, roots, grain) consistent with profiles found in the literature (Osborne et al., 2015; Penning de Vries, 1989), a modelled grain DM within ±30 % of the observed, an above-ground DM value of between 1200–1600 g m−2, an LAI value between 4–7 m2 m−2, and an Rd value of between 30 % and 60 % of Anet (Amthor et al., 2019). We calibrated C allocation parameters as the JULES-crop model calibration has only been performed for broad, global-scale application for wheat (Osborne et al., 2015) and therefore requires further calibration for application under Chinese conditions. Further, the observed dataset does not provide any information with regards to the change in carbon allocation parameters due to ozone. The C allocation parameters were only calibrated for ambient ozone conditions, and we only investigate the effect of ozone on C assimilation (not C allocation).
Finally, calibration of the O3 parameters (γ3, γ4, and γ5) was performed using 2008 data for the Y2 and Y16 cultivars whilst again keeping the other parameters fixed. Calibration was targeted so that the difference in grain DM between ambient and elevated O3 treatments was as close as possible to ±10 % of the observed.
The manual calibration process consisted of three stages as explained above, as well as comparisons with established information on wheat growth from the literature. By reducing the number of parameters involved in the calibration, the chance of equifinality (multiple combinations of parameters yielding similar results) was minimised (Beven, 2006). The parameters identified by the sensitivity analysis were varied within realistic ranges to obtain a parameterisation that closely approximates wheat physiological processes. Multiple parameterisations were tested to avoid convergence on local minima in R2 and RMSE. While further fine-tuning of the parameter ranges could potentially improve yield prediction, it might also disrupt simulations of other key plant processes, such as carbon allocation or photosynthesis. The calibration approach balances the need for accurate output simulation with the physiological realism required for wheat growth under the conditions of this study. Though it is difficult to claim that the absolute optimal parameter set has been achieved, this limitation is common to any model calibration (Wallach, 2011). The current parameterisation represents a physiologically realistic simulation of wheat growth under the conditions of the present study using a robust calibration method.
Evaluation of the DO3SE-Crop model was conducted using Xiaoji data for 2007 and 2009 for all cultivars and 2008 data for Y19 and Y16 cultivars. This evaluation tested the ability of the calibrated DO3SE-Crop model to simulate grain DM using R2 and RMSE statistical tests.
We first examine the model's ability to simulate the key phenological development stages since this is key to simulating the variation in C allocation to different plant parts over the course of the growing season and hence how O3 exposure will influence growth and yield which is determined by the timing and length of the grain-filling period. We also explore how DO3SE-Crop simulates within-canopy [O3] profiles to understand which layers of the canopy are most important in determining O3 response. We then examine the ability of the model to simulate leaf-level physiology and C allocation to the different parts of the crop. Lastly, the impact of both instantaneous and long-term O3 damage on the crop's final grain DM is evaluated for different cultivars and years.
i. Crop phenology
The Xiaoji dataset provides sowing and harvest dates for all cultivars for each year but only provides the date of the timing of anthesis for the years 2008 and 2009 for all cultivars. We assume that DVI =1 is equivalent to the start of anthesis and that this occurs 4–5 d after flag leaf emergence as shown in Fig. 2. We determine the influence of O3 on the start and end of senescence (SOS and EOS) using the breakpoint method (described in Pande et al., 2024) to assess significant changes in the chlorophyll values that indicate senescence onset and rate of change for the quantification of tlep and tlse. This method is applied to chlorophyll data collected in 2008 under both AA and E O3 treatments for the Y2 cultivar. We then assume that these key phenology parameters (i.e. TTemr, TTveg, TTrep, tlep, and tlse) are consistent across cultivars and years. Our results in Fig. 3 suggests this is a reasonable assumption; however, we appreciate that assuming these phenology parameters will work for a wider variety of cultivar types (e.g. early or late sown and/or maturing) and years with rather different meteorological conditions needs to be done with caution.
Figure S1 shows the modelled vs. observed timing of anthesis and harvest for the training dataset. Figure 3 shows the same for the test dataset. For the test dataset there is a variation of 2 to 4 and 1 to 6 d for the modelled anthesis and maturity in relation to observed anthesis and maturity, respectively, with observed phenology tending to be a little later than modelled. The Tl ranges between 1325 and 1478 °C days for the 3 years, with crop sowing occurring between 315 and 324 d of year and harvests occurring between 135 and 151 d of year (of the following year). The number of days from the modelled crop sowing to harvest was between 181 and 191 for the 3 years, compared to 198 and 201 for the observations.
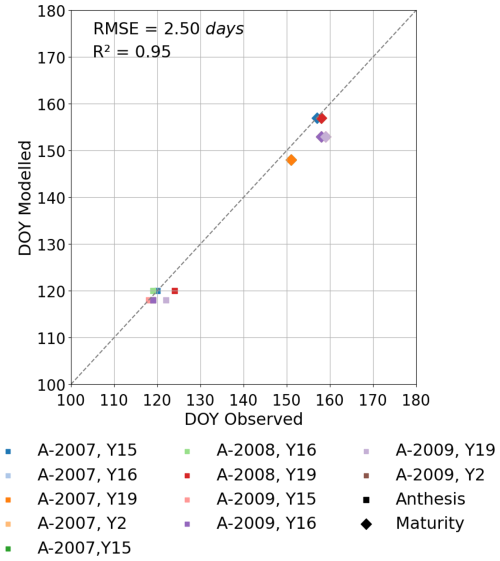
Figure 3Modelled vs. observed phenological stages provided as day of year (DOY) for the test dataset (i.e. excluding the year 2008 for the Y2 cultivar).
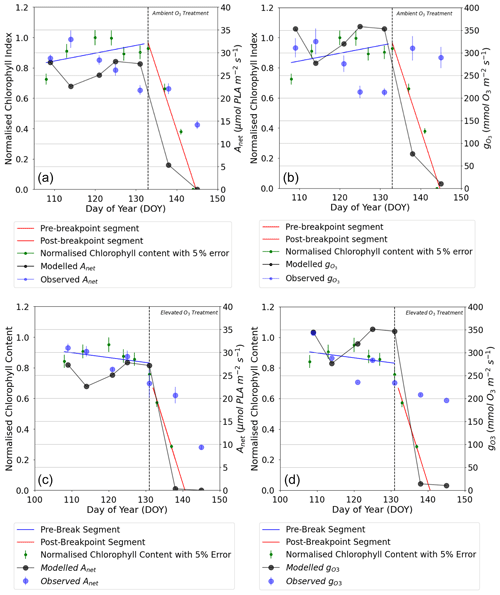
Figure 4Comparison of daily maxima seasonal profiles of DO3SE-Crop modelled canopy leaf vs. observed flag leaf data for (a) AA O3 treatment Anet, (b) AA O3 treatment , (c) E O3 treatment Anet, and (d) E O3 treatment for the period from the anthesis (i.e. TTrep) for the year 2008 and the Y16 cultivar. The left (solid blue line) and right (solid red line) represent the segment fits to the normalised chlorophyll content values for application of the breakpoint method to define the SOS (start of senescence) shown as the dashed black line. The green scatter solid dots, along with their standard measurement error, represent the normalised observed chlorophyll content values (see Fig. 7 for further details).
ii. Leaf physiology variables (Anet, )
The DO3SE-Crop model was able to simulate the seasonal Anet and with values ranging from 0 to 27 µmol CO2 m−2 s−1 and 10 to 351 mmol O3 m−2 s−1 for Anet and , respectively, over the course of the growing season (see Fig. 4). The simulated daily maximum values of modelled , at 351 mmol O3 m−2 s−1, were within the range of the observed value of 340 mmol O3 m−2 s−1. Similarly, the modelled daily maximum Anet is 27 µmol CO2 m−2 s−1 compared to observed value of 28 µmol CO2 m−2 s−1 for the period between anthesis and 10 d before maturity for the year 2008, for the Y16 cultivar (similar results were obtained for the Y2 cultivar; see Fig. S5). In Fig. 4a and b, the steep decline in modelled Anet and is not seen in the observed dataset. This discrepancy may occur since the simulated Anet and values represent sunlit parts of the upper canopy which comprise both green and senesced leaf material. In contrast, observed Anet and values are measured specifically on the flag leaf and most likely only for the green parts of the leaf, since the LI-6400 photosynthesis system mounted with a 6400–40 leaf chamber fluorometer (used to measure Anet and in the Xiaoji experiment, Feng et al., 2016) will not provide values for senesced leaf material. See also Fig. 4, which combines Anet and with observed normalised chlorophyll content and clearly shows the leaf is senescing as predicted by the model. However, the observed decline in chlorophyll values closely matches the decrease in modelled Anet and , with the model accurately capturing the timing of the earlier onset of senescence, which occurred 0–3 d earlier in the AA and EO3 treatments. It is useful to note that the calibrated Vcmax and Jmax values match the observed values within ±2 µmol CO2 m2 s−1.
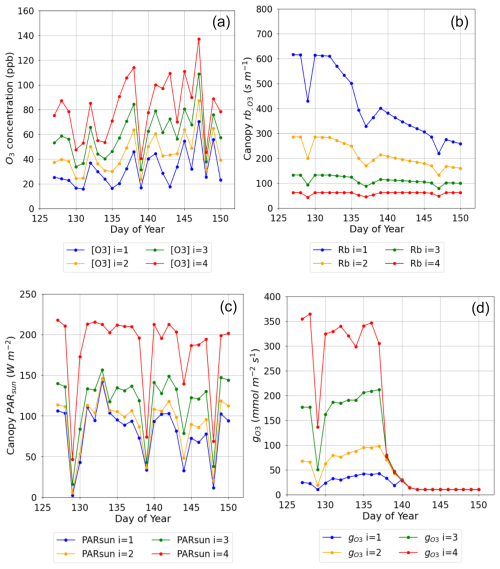
Figure 5Plot showing variation in key O3 deposition terms as daily maxima by canopy layer (NB i=4 is the top canopy layer, n=4) (a) O3 concentration at the top of each layer, (b) leaf boundary layer resistance by canopy layer (), (c) PAR for the sunlit LAI component of each layer (PARsun), and (d) leaf-level stomatal conductance to O3 () for the period from anthesis (i.e. TTrep) for the Y16 cultivar and for the E O3 treatment in 2008.
iii. Within-canopy variation in O3 and physiology
An important determinant of O3 deposition and damage is stomatal O3 deposition (our ), which is a function of within-canopy transfer of O3 and stomatal and non-stomatal deposition. The multi-layer aspect of the DO3SE-Crop model allows within-canopy stomatal and non-stomatal O3 deposition to be simulated. Figure 5 shows the variation in key variables that determine total and stomatal O3 canopy deposition across four canopy layers as a midday average over the course of the tlep period of the flag leaf, for the year 2008 and the Y16 cultivar.
Table 2Simulations of percent of grain DM loss that compare ambient (AA) and elevated (E O3) treatments with a pre-industrial O3 scenario divided between grain DM losses caused by the instantaneous effect O3 on photosynthesis and the long-term O3 effect on senescence. The effects of both damage O3 mechanisms acting together are also shown.
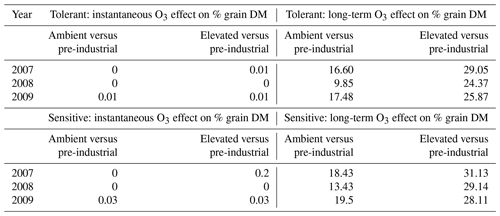
Figure 5a shows a decrease of within-canopy O3 concentration from highs of around 140 ppb to values within the range of 10 to 50 ppb between the top of the canopy and bottom canopy layer; the penetration of O3 into the canopy increases over time as the canopy senescence and O3 uptake is reduced. Similarly, PARsun is reduced from maximum values of around 200 W m−2 at the top of the canopy to values of around 100 W m−2 in the lower canopy layers even on sunny days (see Fig. 5c). The leaf (Fig. 5b) increases with canopy depth with resistances in the region of approximately 50 s m−1 at the top of the canopy to values of around 600 s m−1 at the bottom of the canopy; this will limit stomatal O3 uptake in the lower canopy layers; finally, these factors combine to influence canopy level (Fig. 5d), which is reduced from values of around 350 at the top of the canopy to 20 nmol O3 m−2 s−1 at the bottom of the canopy layer; these differences in leaf and are reduced with the onset of senescence. This analysis shows the importance of interplay between these different factors for an accurate whole-canopy estimate of O3 deposition.
iv. Crop development, biomass, and yield
The dry matter dynamics of the different parts of the crop are shown in Fig. 6. The modelled grain DM value of 851 g m−2 was reasonably close to the observed value of 888 g m−2. The stem to leaf dry matter ratio (RSL) is 2.1:1 and therefore in the range provided in the literature (Huang et al., 2022). The above-ground biomass values of 1510 g m−2 also match reasonably well against the 1200 to 1600 g m−2 range described in the literature (Huang et al., 2022; Liu et al., 2022). Further, the partition fraction profiles are consistent with those of Osborne et al. (2015) as shown in Fig. 6a, with the main differences being that the modelled stem and root partition profiles are somewhat higher and lower, respectively. The JULES model comparison is provided for illustrative purposes only (i.e. this model has not been calibrated with the Xiaoji data but rather is a parameterisation suggested for global application).
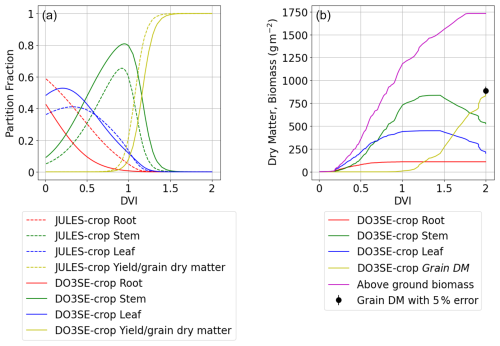
Figure 6Seasonal profiles (i.e. plotted against DVI) of carbon allocation variables for the Xiaoji calibrated DO3SE-Crop model (i.e. AA O3 treatment, year 2008, and Y16 cultivar) with panel (a) showing the partition fractions of the daily accumulated NPP partitioned to roots, stems, leaves, and grains for the Xiaoji calibrated DO3SE-Crop model (solid lines) vs. the JULES-crop model (dashed line) calibrated for global application after Osborne et al. (2015) and panel (b) showing the DM (in g m−2) of daily accumulated NPP partitioned to roots, stems, leaves, and grains, with the observed final grain DM for Y16 cultivar in 2008 also shown (solid black dot with 5 % error).
v. O3-induced yield loss difference between tolerant and sensitive cultivars: instantaneous and long-term senescence impact
The grain DM is assumed to be damaged by both the instantaneous impact of O3 (Farage et al., 1991) on photosynthesis and a longer-term O3 effect that can lead to enhanced senescence (Y. Feng et al., 2022). To explore which of these damage mechanisms is most important, we calculated the difference in the grain DM caused by carbon assimilation for the AA and E O3 treatments as compared to a simulated very low O3 treatment representing pre-industrial conditions (for which Cz O3 concentration did not exceed 15 ppb) for the tolerant (Y16) and sensitive (Y2) cultivar for each of the 3 years (see Table 2). We found a negligible effect of O3 (0 % to 0.2 %) on grain DM due to the instantaneous effect of O3 on photosynthesis, which could perhaps be partly due to the to the crops ability to recover photosynthetic capacity overnight, compared to a highly significant (9.85 % to 31.13 %) impact due to the long-term O3 effect on carbon assimilation via the enhancement of senescence on final grain DM. Table S3 shows the observed percent of grain DM loss compared to a modelled pre-industrial O3 scenario due to the combination of instantaneous and long-term ozone effect.
vi. Senescence
The breakpoint method (Mariën et al., 2019) was used to determine the onset (SOS) and end (EOS) of senescence and maturity, respectively, using the chlorophyll data which were available for the year 2008 and the Y16 and Y2 cultivars. Results in Figs. 7 and S4 show that the E O3 treatment for cultivars Y16 and Y2 brought forward the SOS by 3 and 5 d (see Fig. 7), respectively, and EOS by 6 and 9 d (see Fig. S4), respectively. Figure 7 also shows the fLS profile which denotes the DO3SE-Crop models' accumulated stomatal O3 flux effect on senescence; it is clear that fLS is able to simulate the change in normalised chlorophyll content reasonably well. The slope of the ambient fLS is already steep since the ambient treatment already has rather high O3 levels as is now made clear in Table 1 with a value of 47 ppb. According to the M7 wheat dose–response relationship this would result in a yield loss of ∼5 %.
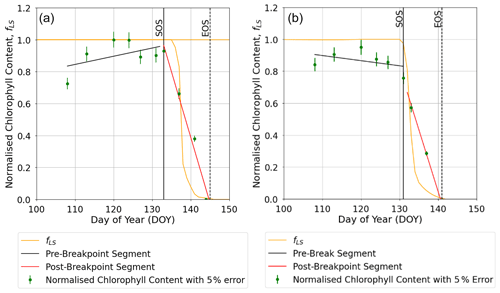
Figure 7Profiles of O3-induced leaf senescence for the Y16 cultivar for the (a) AA O3 treatment and (b) E O3 treatment. The timing of the SOS (solid black line) and EOS (dashed black line) was determined by applying the breakpoint method to the chlorophyll data and is shown in relation to the fLS simulations of senescence (solid yellow line). The observed normalised chlorophyll content data, shown as filled green symbols, include error bars representing the standard deviation of the measurements.
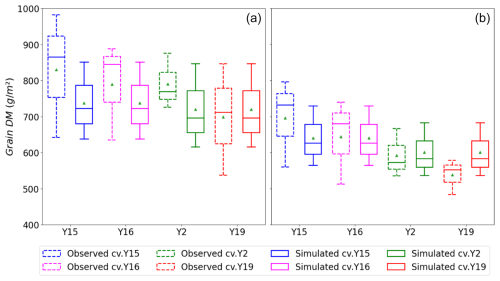
Figure 8Box plots (crosses: 0.01 and 0.99 percentiles; box: 0.25 quartile, median, and 0.75 quartile; triangle: mean) of simulated and observed wheat grain DM for the tolerant (Y15 and Y16) and sensitive (Y2 and Y19) cultivars under (a) AA and (b) E O3 treatment for the years 2007, 2008, and 2009; these data include the whole dataset.
vii. Grain DM simulations across years and between cultivars
Figure 8 shows a box plot of the modelled vs. observed grain DM for the sensitive (Y2, Y19) and tolerant (Y15, Y16) cultivars for each O3 treatment (AA and E) for the years 2007, 2008, and 2009 (i.e. all data). Given the variability in the experimental data, the model simulates the difference in grain DM between the AA and E O3 treatments reasonably well with a simulated reduction in grain DM of 29 to 131 g m−2 compared with observed values of 81 to 165 g m−2 for the tolerant cultivars and 49 to 196 g m−2 compared with observed values of 54 to 293 g m−2 for the sensitive cultivars, respectively. The most notable difference is that there is a larger range in the simulated grain DM losses of the modelled sensitive cultivars, though the simulated mean value for absolute grain DM suggests a more conservative influence of O3, with yields at 610 g m−2 vs. observed average yields of 590 g m−2.
Finally, Fig. 9 shows the relationship between modelled vs. observed grain DM (in g m−2) as a scatter plot; a linear regression through these data gives an R2 value of 0.68 and RMSE of 76 g m−2, showing the model is able to simulate with reasonable accuracy the differences in absolute yield for different cultivars and for different years. There are some instances of both underestimation and overestimation; however, the deviations from the 1:1 line are not excessively large. These models test results compare with an R2 of 0.92 (n=4) and an RMSE of 25.49 g m2 for the training dataset (Y2 and Y16 cultivar and year 2008; see Fig. S3); the stronger agreement between observed and modelled training dataset, as well as the reasonable agreement for the entire dataset, would suggest the model is not over-fitted. We find that we tend to underestimate the O3-induced relative yield loss (RYL) by between −2.76 and 15.34 % (observed minus modelled RYL) across all years and cultivars.
The DO3SE-Crop model was found capable of simulating O3 damage to grain yield for O3-FACE conditions at the experimental site in Xiaoji, China, with a good degree of accuracy. Simulated relative yield losses (RYLs) between AA and E O3 treatments for all years ranged from 11 % to 14 % and from 13 % to 19 % for tolerant and sensitive cultivars, respectively; these tend to be lower (particularly for the more extreme O3-induced yield losses of the sensitive cultivars) than the observed values of 13 % to 20 % and 10 % to 35 %. Overall, simulations of tolerant and sensitive cultivars underestimated RYLs by 4 % and 7 %, respectively, on average across years and cultivars (see data in Sect. S6). This would suggest that O3-induced yield losses can be more reliably modelled for tolerant cultivars, possibly because additional processes causing O3-induced yield losses in sensitive cultivars are not captured. Such processes might include the effect of O3 on the allocation of carbon to different plant parts (Feng et al., 2008) or O3 inducing additional respiratory costs via the upregulation of defence mechanisms (Biswas et al., 2008). The model was also able to simulate absolute grain DM reasonably well. Under AA O3 levels, grain DM simulated for all years and cultivars was between 616 and 851 g m−2 compared to observations of between 537 and 982 g m−2. There is a tendency to overestimate grain DM under ambient conditions and underestimate grain DM under elevated O3, which is reflected in the RYL values.
Overall, the DO3SE-Crop model simulation results compare favourably to results made by the MCWLA-Wheat model (Tao et al., 2017), which was also calibrated for the Xiaoji experimental conditions but without distinction between tolerant and sensitive varieties. MCWLA-Wheat simulations of absolute yield varied from ∼5700 to 9000 kg ha−1 (compared to ∼5700 to 9800 kg ha−1) for ambient and from ∼4800 to 8000 kg ha−1 (compared to ∼5200 to 8000 kg ha−1) for elevated O3 treatments. A mean relative yield loss of 14 % was simulated by the model.
It is useful to set these site-specific estimates of O3-induced yield losses in the context of yield losses estimated using more traditional, concentration-based O3 risk assessment methods. A seminal paper by Z. Feng et al. (2022) estimated mean relative yield losses across East Asia due to ambient O3 concentrations at 33 % (with a mean range of 28 % to 37 %) according to a mean monitored O3 concentrations of 30.9 ppm h expressed as AOT40 (6-month accumulated daytime O3 concentration above a threshold of 40 ppb). The mean difference in AOT40 (accumulated over only 75 d) between the AA and E O3 treatments at Xiaoji across all years was 7.8 ppm h, giving a mean relative yield loss of approximately 10 % to 20 % depending on year and cultivar. As such, our modelled results in terms of RYLs between AA and E O3 treatments are consistent with these broader results for East Asia.
Crop phenology plays a crucial role in determining the timing of the important O3 exposure period (i.e. from anthesis to maturity) and hence O3 damage. Evaluation of the DO3SE-Crop phenology model shows the model is able to accurately simulate crop phenology for the 3 years at Xiaoji (R2=0.95 and RMSE =2.5; see Fig. 3). Estimating the correct timing of anthesis is crucial since the period from anthesis to crop maturity is the O3-sensitive period. During this period, accumulated stomatal O3 flux (accfst) will contribute to early and enhanced senescence once the critical threshold (CLsO3) is exceeded. This period also coincides with carbon accumulation in the grain (Kohut et al., 1987; Feng et al., 2008), which may be limited by O3-induced early onset or enhanced senescence. The DO3SE-Crop model was developed to accommodate the full range of effects of O3 on senescence with revised functions, similar to those first developed by Ewert and Porter (2000), and was able to modify both the O3-induced onset of senescence and the O3 effect on maturity. This is important since experimental evidence has shown that O3 can bring forward the maturity date; for example, the flag leaf was found to have senesced 25 d earlier in a high-O3 treatment, compared to a charcoal-filtered treatment (Grandjean and Fuhrer, 1989; Gelang et al., 2000). O3 was also found to cause differences in the time to maturity of the flag leaf, with Shi et al. (2009) reporting that maturity was brought forward by 8 d under an elevated O3 treatment (50 % higher than ambient). Currently, other crop models with O3 damage functions (e.g. MCWLA-Wheat (Tao et al., 2017) and LINTULCC-2 (Y. Feng et al., 2022)) are only able to bring the O3-induced onset of senescence earlier.
The DO3SE-Crop model is also able to simulate differential O3 uptake in each canopy layer. Figure 5 shows that the majority of stomatal O3 uptake occurs in the sunlit layers of the upper canopy. Similar results were found in an experimental study on a productive grassland in Switzerland; Jaggi et al. (2006) found that different levels of O3 exposure to canopy components predominantly located in the upper and lower parts of the canopy support a multi-layer approach to modelling O3 uptake. Therefore, the focus on the upper canopy by flux-based O3 metrics (e.g. the phytotoxic ozone dose PODy; LRTAP, 2017) seems rational in the absence of multi-layer modelling. Crop models such as LINTULCC-2 (Y. Feng et al., 2022) also focus on estimating stomatal O3 uptake at the top of the canopy to estimate O3-induced yield losses. For wheat, such an approach is further supported by the fact that the upper canopy layers consist of the flag leaf, which plays a crucial role in photosynthesis and grain filling (Pleijel et al., 2007). The multi-layer functionality of the DO3SE-Crop model may, however, become more useful when considering crops that partition assimilated carbon to harvest organs earlier in their growing season such as potato (Okrah et al. 2023).
Our results show that the DO3SE-Crop model was able to estimate the seasonal course of leaf Anet and daily maxima observed at the Xiaoji site (see Fig. 4a) and when compared to other literature describing leaf physiological variables (Guan et al., 2015; Li et al., 2022). This suggests the coupled Anet–gsto model is working for Chinese conditions (having previously been applied to and evaluated for European O3 experimental conditions – see Pande et al., 2024). The leaf physiology parameters used in this study (i.e. for Asian conditions and cultivars) are higher than parameters for European studies. For Europe, Vcmax values of between 60 and 90 µmol CO2 m−2 s−1 were found in the literature (Y. Feng et al., 2022; Pande et al., 2024; Van Oijen and Ewert, 1999) compared to the observed mean maximum value of 137 µmol CO2 m−2 s−1 at Xiaoji which was used in this study. Similarly, European Jmax values ranged from 160 to 180 µmol CO2 m−2 s−1 (Feng et al., 2021; Pande et al., 2024; Van Oijen and Ewert, 1999) compared to the observed Xiaoji mean maximum value of 228 µmol CO2 m−2 s−1. Even though these leaf physiology parameters are higher, absolute yields for these Chinese cultivars are consistent with those found under European conditions. This most likely reflects the importance of other environmental conditions (e.g. high vapour pressure deficits) limiting leaf carbon assimilation. Moreover, the complex interactions between O3 exposure and the plants' physiological responses also play a crucial role. Ozone significantly affected antioxidative enzymes, thereby limiting overall photosynthetic efficiency and yield, particularly in O3-sensitive cultivars, despite their ability to maintain high carboxylation capacity.
Ensuring the seasonal variation in carbon allocation to the different components of the crop (i.e. roots, stem, leaves, and harvest organs) is essential for the simulation of crop growth and yield. There are limited data in the literature that provide these variables, so we compare our results to the carbon allocation profiles described for wheat provided in the original JULES-crop model description, recognising this is intended for wheat grown globally. The DO3SE-Crop model carbon allocation to the stem and roots is comparatively higher than what was simulated by JULES-crop (Osborne et al., 2015; see Fig. 6a). However, we can justify the carbon allocation coefficients used for Xiaoji since the DO3SE-Crop model was able to distribute carbon to different plant components to produce a well-proportioned plant over the course of the growing season; this was determined by the calibration to a number of key crop variables (i.e. ratios of plant respiration, LAI, stem to leaf dry matter, above-ground components, and grain dry matter). Importantly, when applied to the test dataset (i.e. excluding 2008 data for the Y2 and Y16 cultivar), the model was found to simulate the grain dry matter under ambient and elevated O3 treatments to within 7.9 %–8.7 % of the observed values (R2=0.68, 76 g m−2 see Fig. 9).
The DO3SE-Crop model, similar to other crop models with O3 damage functions (i.e. MCWLA-Wheat (Tao et al., 2017), LINTULCC-2 (Y. Feng et al., 2022), and WOFOST (Nguyen et al., 2024)) has the capacity to simulate both the instantaneous and long-term O3 impact on wheat grain yield. The instantaneous O3 effect on photosynthesis may cause leaf cell damage and decrease the supply of carbohydrate precursors, which can significantly decrease , Vcmax, and leaf chlorophyll content (Farage et al., 1991). Elevated O3 also leads to generation of reactive oxygen species (ROS) in plant cells, which can cause oxidative damage to various cellular components. Rubisco, the enzyme responsible for carbon fixation in the photosynthetic process, can be particularly susceptible to this damage, leading to a reduced carboxylation rate (Vcmax). Such an O3 effect on Vcmax reduces net photosynthesis and can also induce early senescence, shortening the grain-filling period (Triboi and Triboi-Blondel, 2002).
Results from the DO3SE-Crop model found a larger impact on yield due to the long-term O3 impact causing relative yield loss of between 10 % and 31 % compared to only between 0 % and 0.2 % resulting from the instantaneous O3 impact on photosynthesis. Previous studies have also found that the long-term O3 effect has a larger impact on yield compared to the instantaneous effect of O3 on photosynthesis (Emberson et al., 2018; Brewster et al., 2024). Senescence is an age-dependent process of degradation and degeneration that allows nutrients to be re-distributed to different plant organs (Lim et al., 2007). Under O3 stress, this process is often found to occur earlier and more rapidly in leaves as well as at the whole-plant or crop canopy scale (Brewster et al., 2024). The causes of this early and accelerated senescence are not completely understood but may be related to O3-induced enhanced expression of many genes involved in natural senescence (Miller et al., 1999). Elevated O3 was also found to inhibit sugar export from leaves (Yadav et al., 2020; Feng et al., 2024), which could trigger early onset of leaf senescence.
The DO3SE-Crop model accounts for the impact of O3 on the rubisco enzyme by incorporating modified (Ewert and Porter, 2000) functions for instantaneous and long-term O3 impact on Vcmax as an important parameter used to characterise the crop photosynthetic capacity (Ewert and Porter, 2000; Osborne et al., 2019). The DO3SE-Crop model assumes that the O3 will only accumulate on exceedance of a stomatal O3 flux threshold of 6 nmol O3 m−2 s−1. The long-term O3 impact mechanism of the DO3SE-Crop model simulated the effect of senescence on Vcmax reasonably well as evidenced by the reduction in leaf chlorophyll content. We used the breakpoint method (Yang et al., 2016; Mariën et al., 2019) to estimate the SOS and EOS using the day of the year and measured chlorophyll content (Figs. 7 and S4). It is crucial to accurately model the timing of SOS and EOS correctly as this determines the O3 effect on the duration of the grain-filling period and hence the difference in yield loss due to different O3 treatments. For example, we modelled a difference of 3 to 5 d in SOS and 6 to 9 d in EOS on average across years for the sensitive and tolerant cultivar, respectively.
China's wheat breeding programme has seen more than 1850 varieties used across China between the 1920s and 2014, leading to increased yields from less than 1 to more than 5 t ha−1 (Qin et al., 2015). Here, albeit with an extremely limited dataset, we parameterise the DO3SE-Crop model for tolerant and sensitive wheat crop cultivars, since many experimental studies have shown that the response of different cultivars to O3 stress differs (Biswas et al., 2008). Based on the available data, the model seemed able to capture the difference in grain dry matter between these different cultivar groups across different years reasonably well when compared to the observed dataset (R2=0.68; see Fig. 8). Such a cultivar sensitivity-based parameterisation can provide additional information on the certainty of regional yield loss estimates given the large number of wheat varieties grown across China. However, when applying the model to a broader region, it would be advisable to calibrate phenology for different agro-ecological zones as the temperature changes across China, impacting the duration of the key phenological stages such as anthesis and maturity (Luo et al., 2021). Additionally, carbon allocation parameters may need adjustment, as studies have shown changes in dry matter content across different agro-ecological zones (Hussain and Bangash, 2017).
We have shown that the newly developed DO3SE-Crop model can be calibrated for O3-tolerant and sensitive wheat varieties for O3-FACE site conditions at Xiaoji in China. The model can simulate crop phenology, leaf physiology, crop growth, and yield reasonably well across different years. The model is also able to simulate the effect of O3 stress on grain yield distinguishing the extent of O3 damage resulting from the same O3 treatment on cultivars with differing O3 sensitivities. The DO3SE-Crop model also has the advantage of simulating O3 transfer and deposition dynamics within the wheat crop canopy, which could in the future improve our understanding of whole-canopy O3 effects for crops with different carbon allocation profiles. The ability of the model to estimate relative yield losses across years also suggests the model is fit for purpose to assess the effects of O3 under a variety of climate variable and O3 concentration conditions.
An open version (version 4.39.16) of the DO3SE-Crop model, as used in the present study, can be found on both https://doi.org/10.5281/zenodo.11620482 and https://github.com/DO3SE/pyDO3SE-open/tree/v4.39.16, last access: 11 June 2024 (Bland, 2024).
The datasets referred to in this study are not publicly accessible. These data were obtained from third-party sources under specific usage agreements. Researchers interested in accessing these datasets should contact the respective data providers directly.
The supplement related to this article is available online at: https://doi.org/10.5194/bg-22-181-2025-supplement.
PP, LE, and ZF: conceptualisation. PP and ZF: data curation. PP: formal analysis. PP, LE, and ZF: methodol-ogy. PP, SB, NB, JC, and LE: software – DO3SE-Crop. LE and ZF: supervision. PP: visualisation and writing – original draft preparation. PP, SB, NB, JC, ZF, and LE: writing – review and editing.
The contact author has declared that none of the authors has any competing interests.
Publisher’s note: Copernicus Publications remains neutral with regard to jurisdictional claims made in the text, published maps, institutional affiliations, or any other geographical representation in this paper. While Copernicus Publications makes every effort to include appropriate place names, the final responsibility lies with the authors.
This article is part of the special issue “Tropospheric Ozone Assessment Report Phase II (TOAR-II) Community Special Issue (ACP/AMT/BG/GMD inter-journal SI)”. It is a result of the Tropospheric Ozone Assessment Report, Phase II (TOAR-II, 2020–2024).
We would like to thank the anonymous reviewers for their insightful comments and suggestions, which helped clarify and improve the quality of this paper.
This research has been financially supported by the Science and Technology Facilities Council (STFC) research grant (ST/V002481/1) for the “Pollution and Climate Smart Agriculture in China” (PaCSAC) project which supported initial development of the DO3SE-Crop model. Support from the Royal Society through the International Exchanges 2021 Cost Share (NSFC) grant (IEC\NSFC\211154) facilitated the UK–China collaboration to parameterise the DO3SE-Crop model within the project “Understanding the role of air pollution and climate on staple crop yields and nutrition in China”. We also received support from the STFC research grant (ST/Y005317/1) under the EO4AgroClimate programme for the project “Towards a digital twin of cropping systems based on ingestion of EO into process-based crop models” which helped refine the DO3SE-Crop model for a broader set of country applications.
This paper was edited by Paul Stoy and reviewed by three anonymous referees.
Amthor, J. S., Bar-Even, A., Hanson, A. D., Millar, A. H., Stitt, M., Sweetlove, L. J., and Tyerman, S. D.: Engineering strategies to boost crop productivity by cutting respiratory carbon loss, Plant Cell, 31, 297–314, https://doi.org/10.1105/tpc.18.00743, 2019.
Betzelberger, A. M., Gillespie, K. M., McGrath, J. M., Koester, R. P., Nelson, R. L., and Ainsworth, E. A.: Ozone exposure response for U.S. soybean cultivars: Linear reductions in photosynthetic potential, biomass, and yield, Plant Physiology, American Society of Plant Biologists, 160, 1827–1839, https://doi.org/10.1104/pp.112.205591, 2012.
Beven, K.: A manifesto for the equifinality thesis, J. Hydrol., 320, 18–36, https://doi.org/10.1016/j.jhydrol.2005.07.007, 2006.
Biswas, D. K., Xu, H., Li, Y. G., Sun, J. Z., Wang, X. Z., Han, X. G., and Jiang, G. M.: Assessing the genetic relatedness of higher ozone sensitivity of modern wheat to its wild and cultivated progenitors/relatives, J. Exp. Bot., 59, 951–963, https://doi.org/10.1093/jxb/ern022, 2008.
Bland, S.: SEI-DO3SE/pyDO3SE-open: V4.39.11 (v4.39.11), Zenodo [code], https://doi.org/10.5281/zenodo.11620482, 2024.
Brewster, C., Fenner, N., and Hayes, F.: Chronic ozone exposure affects nitrogen remobilization in wheat at key growth stages, Sci. Total Environ., 908, 168288, https://doi.org/10.1016/j.scitotenv.2023.168288, 2024.
Büker, P., Morrissey, T., Briolat, A., Falk, R., Simpson, D., Tuovinen, J.-P., Alonso, R., Barth, S., Baumgarten, M., Grulke, N., Karlsson, P. E., King, J., Lagergren, F., Matyssek, R., Nunn, A., Ogaya, R., Peñuelas, J., Rhea, L., Schaub, M., Uddling, J., Werner, W., and Emberson, L. D.: DO3SE modelling of soil moisture to determine ozone flux to forest trees, Atmos. Chem. Phys., 12, 5537–5562, https://doi.org/10.5194/acp-12-5537-2012, 2012.
Campbell, G. S. and Norman, J. M.: An introduction to Environmental Biophysics, Second Edition, Springer, New York, 286 pp., https://doi.org/10.1007/978-1-4612-1626-1, 1998.
Clark, D. B., Mercado, L. M., Sitch, S., Jones, C. D., Gedney, N., Best, M. J., Pryor, M., Rooney, G. G., Essery, R. L. H., Blyth, E., Boucher, O., Harding, R. J., Huntingford, C., and Cox, P. M.: The Joint UK Land Environment Simulator (JULES), model description – Part 2: Carbon fluxes and vegetation dynamics, Geosci. Model Dev., 4, 701–722, https://doi.org/10.5194/gmd-4-701-2011, 2011.
Conibear, L., Butt, E. W., Knote, C., Spracklen, D. V., and Arnold, S. R.: Current and Future Disease Burden From Ambient Ozone Exposure in India, GeoHealth, 2, 334–355, https://doi.org/10.1029/2018GH000168, 2018.
Danielsson, H., Karlsson, G. P., Karlsson, P. E., and Pleijel, H. H.: Ozone uptake modelling and flux-response relationships–an assessment of ozone-induced yield loss in spring wheat, Atmos. Environ., 37, 475–485, https://doi.org/10.1016/S1352-2310(02)00924-X, 2003.
de Pury, D. G. G. and Farquhar, G. D.: Simple Scaling of Photosynthesis from Leaves to Canopies Without the Errors of Big-Leaf Models, Funct. Plant Biol., 24, 537–557, https://doi.org/10.1111/j.1365-3040.1997.00094.x, 1997.
Droutsas, I., Challinor, A. J., Arnold, S. R., Mikkelsen, T. N., and Hansen, E. M. Ø.: A new model of ozone stress in wheat including grain yield loss and plant acclimation to the pollutant, Eur. J. Agron., 120, 126125, https://doi.org/10.1016/j.eja.2020.126125, 2020.
Emberson, L. D., Ashmore, M. R., Cambridge, H. M., Simpson, D., and Tuovinen, J.-P.: Modelling stomatal ozone flux across Europe, Environ. Pollut., 109, 403–413, https://doi.org/10.1016/S0269-7491(00)00043-9, 2000.
Emberson, L. D., Ashmore, M. R., Simpson, D., Tuovinen, J.-P., and Cambridge, H. M.: Modelling and mapping ozone deposition in Europe, Water Air Soil Poll., 577–582, 2001.
Emberson, L. D., Pleijel, H., Ainsworth, E. A., van den Berg, M., Ren, W., Osborne, S., Mills, G., Pandey, D., Dentener, F., Büker, P., Ewert, F., Koeble, R., and Van Dingenen, R: Ozone effects on crops and consideration in crop models, Eur. J. Agron., 100, 19–34, https://doi.org/10.1016/j.eja.2018.06.002, 2018.
Ewert, F. and Porter, J. R.: Ozone effects on wheat in relation to CO2: Modelling short-term and long-term responses of leaf photosynthesis and leaf duration, Glob. Change Biol., 6, 735–750, https://doi.org/10.1046/j.1365-2486.2000.00351.x, 2000.
Farage, P. K., Long, S. P., Lechner, E. G., and Baker, N. R.: The sequence of change within the photosynthetic apparatus of wheat following short-term exposure to ozone, Plant Physiol., 95, 529–535, https://doi.org/10.1104/pp.95.2.529, 1991.
Farquhar, G. D., von Caemmerer, S., and Berry, J. A.: A biochemical model of photosynthetic CO2 assimilation in leaves of C3 species, Planta, 149, 78–90, https://doi.org/10.1007/BF00386231, 1980.
Feng, Y., Nguyen, T. H., Alam, M. S., Emberson, L., Gaiser, T., Ewert, F., and Frei, M: Identifying and modelling key physiological traits that confer tolerance or sensitivity to ozone in winter wheat, Environmental Pollution, Elsevier Ltd, 304, 119251, https://doi.org/10.1016/j.envpol.2022.119251, 2022.
Feng, Y., Alam, M. S., Yan, F., and Frei, M.: Alteration of carbon and nitrogen allocation in winter wheat under elevated ozone, Plant Sci., 338, 111924, https://doi.org/10.1016/j.plantsci.2023.111924, 2024.
Feng, Z., Kobayashi, K., and Ainsworth, E. A.: Impact of elevated ozone concentration on growth, physiology, and yield of wheat (Triticum aestivum L.): a meta-analysis, Glob. Change Biol., 14, 2696–2708, https://doi.org/10.1111/j.1365-2486.2008.01673.x, 2008.
Feng, Z., Pang, J., Kobayashi, K., Zhu, J., and Otr, R. D.: Differential responses in two varieties of winter wheat to elevated ozone concentration under fully open-air field conditions, Glob. Change Biol., 17, 580–591, https://doi.org/10.1111/j.1365-2486.2010.02184.x, 2011.
Feng, Z., Tang, H., Uddling, J., Pleijel, H., Kobayashi, K., Zhu, J., Oue, H., and Guo, W.: A stomatal ozone flux-response relationship to assess ozone-induced yield loss of winter wheat in subtropical China, Environ. Pollut., 164, 16–23, https://doi.org/10.1016/j.envpol.2012.01.014, 2012.
Feng, Z., Wang, L., Pleijel, H., Zhu, J., and Kobayashi, K.: Differential effects of ozone on photosynthesis of winter wheat among cultivars depend on antioxidative enzymes rather than stomatal conductance, Sci. Total Environ., 572, 404–411, https://doi.org/10.1016/j.scitotenv.2016.08.083, 2016.
Feng, Z., Uddling, J., Tang, H., Zhu, J., and Kobayashi, K.: Comparison of crop yield sensitivity to ozone between open-top chamber and free-air experiments, Glob. Change Biol., 24, 2231–2238, https://doi.org/10.1111/gcb.14077, 2018.
Feng, Z., Agathokleous, E., Yue, X., Oksanen, E., Paoletti, E., Sase, H., Gandin, A., Koike, T., Calatayud, V., Yuan, X., Liu, X., De Marco, A., Jolivet, Y., Kontunen-Soppela, S., Hoshika, Y., Saji, H., Li, P., Li, Z., Watanabe, M., and Kobayashi, K.: Emerging challenges of ozone impacts on Asian plants: Actions are needed to protect ecosystem health, Ecosystem Health and Sustainability, 7, 1911602. https://doi.org/10.1080/20964129.2021.1911602, 2021.
Feng, Z., Xu, Y., Kobayashi, K., Dai, L., Zhang, T., Agathokleous, E., Calatayud, V., Paoletti, E., Mukherjee, A., Agrawal, M., Park, R. J., Oak, Y. J., and Yue, X.: Ozone pollution threatens the production of major staple crops in East Asia, Nature Food, 3, 47–56, https://doi.org/10.1038/s43016-021-00422-6, 2022.
Gelang, J., Pleijel, H., Sild, E., Danielsson, H., Younis, S., Selldén, G., and Wallin, G.: Rate and duration of grain filling in relation to flag leaf senescence and grain yield in spring wheat (Triticum aestivum) exposed to different concentrations of ozone, Physiol. Plantarum, 110, 366–375, https://doi.org/10.1111/j.1399-3054.2000.1100311.x, 2000.
Grandjean, A. and Fuhrer, J.: Growth and leaf senescence in spring wheat (Triticum aestivum) grown at different ozone concentrations in open-top field chambers, Environ. Pollut., 59, 299–314, 1989.
Guan, X., Song, L., Wang, T. C., Turner, N., and Li, F.: Effect of Drought on the Gas Exchange, Chlorophyll Fluorescence and Yield of Six Different-Era Spring Wheat Cultivars, J. Agron. Crop Sci., 201, 253–266, https://doi.org/10.1111/jac.12103, 2015.
Guarin, J. R., Kassie, B., Mashaheet, A. M., Burkey, K., and Asseng, S.: Modeling the effects of tropospheric ozone on wheat growth and yield, Eur. J. Agron., 105, 13–23, https://doi.org/10.1016/j.eja.2019.03.001, 2019.
Guarin, J. R., Jägermeyr, J., Ainsworth, E. A., Oliveira, F. A. A., Asseng, S., Boote, K., Elliott, J., Emberson, L., Foster, I., Hoogenboom, G., Kelly, D., Ruane, A. C., and Sharps, K.: Modeling the effects of tropospheric ozone on the growth and yield of global staple crops with DSSAT v4.8.0, Geosci. Model Dev., 17, 2547–2567, https://doi.org/10.5194/gmd-17-2547-2024, 2024.
Herman, J. and Usher, W.: SALib: An open-source Python library for sensitivity analysis, J. Open Source Softw., 2, 97, https://doi.org/10.21105/joss.00097, 2017.
Huang, H., Huang, J., Li, X., Feng, J., Zhuo, W., Wu, Y., Niu, Q., Su, W., and Yin, Y.: A dataset of winter wheat aboveground biomass in China during 2007–2015 based on data assimilation, Scientific Data, Springer US, 9, 1–11, https://doi.org/10.1038/s41597-022-01305-6, 2022.
Hussain, A. and Bangash, R.: Impact of Climate Change on Crops' Productivity across Selected Agro-ecological Zones in Pakistan, The Pakistan Development Review, 56, 163–187, https://www.jstor.org/stable/26875191, 2017.
IPCC: Climate Change 2021: The Physical Science Basis. Contribution of Working Group I to the Sixth Assessment Report of the Intergovernmental Panel on Climate Change, Cambridge University Press, https://doi.org/10.1017/9781009157896, 2021.
Iwanaga, T., Usher, W., and Herman, J.: Toward SALib 2.0: Advancing the accessibility and interpretability of global sensitivity analyses, Socio-Environ. Syst. Model., 4, 18155, https://doi.org/10.18174/sesmo.18155, 2022.
Jaggi, M., Ammann, C., Neftel, J., and Fuhrer, J.: Environmental control of profiles of ozone concentration in a grassland canopy, Atmos. Environ., 40, 5496–5507, https://doi.org/10.1016/j.atmosenv.2006.01.025, 2006.
Jones, H. G.: Plants and microclimate: A quantitative approach to environmental plant physiology, Cambridge University Press, ISBN 0521425247, 1992.
Kohut, R. J., Amundson, R. G., Laurence, J. A., Colavito, L., van Leuken, P., and King, P.: Effects of Ozone and Sulfur Dioxide on Yield of Winter Wheat, Phytopathology, 77, 71–74, https://doi.org/10.1094/Phyto-77-71, 1987.
Kutman, U. B., Yildiz, B., and Cakmak, I.: Effect of nitrogen on uptake, remobilization and partitioning of zinc and iron throughout the development of durum wheat, Plant Soil, 342, 149–164, https://doi.org/10.1007/s11104-010-0679-5, 2011.
Lee, J. D., Drysdale, W. S., Finch, D. P., Wilde, S. E., and Palmer, P. I.: UK surface NO2 levels dropped by 42
Leung, F., Williams, K., Sitch, S., Tai, A. P. K., Wiltshire, A., Gornall, J., Ainsworth, E. A., Arkebauer, T., and Scoby, D.: Calibrating soybean parameters in JULES 5.0 from the US-Ne2/3 FLUXNET sites and the SoyFACE-O3 experiment, Geosci. Model Dev., 13, 6201–6213, https://doi.org/10.5194/gmd-13-6201-2020, 2020.
Leuning, R.: Modeling stomatal behavior and photosynthesis of Eucalyptus grandis, Aust. J. Plant Physiol., 17, 159–175, 1990.
Leuning, R.: A critical appraisal of combined stomatal models for C3 plants, Plant, Cell Environ., 18, 339–355, 1995.
Li, A., Zhou, Q., and Xu, Q.: Prospects for ozone pollution control in China: An epidemiological perspective, Environ. Pollut., 285, 117670, https://doi.org/10.1016/j.envpol.2021.117670, 2021.
Li, D., Shindell, D., Ding, D., Lu, X., Zhang, L., and Zhang, Y.: Surface ozone impacts on major crop production in China from 2010 to 2017, Atmos. Chem. Phys., 22, 2625–2638, https://doi.org/10.5194/acp-22-2625-2022, 2022.
Li, K., Jacob, D. J., Shen, L., Lu, X., De Smedt, I., and Liao, H.: Increases in surface ozone pollution in China from 2013 to 2019: anthropogenic and meteorological influences, Atmos. Chem. Phys., 20, 11423–11433, https://doi.org/10.5194/acp-20-11423-2020, 2020.
Lim, P. O., Kim, H. J., and Nam, H. G.: Leaf senescence, Annu. Rev. Plant Biol., 58, 115–136, https://doi.org/10.1146/annurev.arplant.57.032905.105316, 2007.
Lin, M., Horowitz, L. W., Payton, R., Fiore, A. M., and Tonnesen, G.: US surface ozone trends and extremes from 1980 to 2014: quantifying the roles of rising Asian emissions, domestic controls, wildfires, and climate, Atmos. Chem. Phys., 17, 2943–2970, https://doi.org/10.5194/acp-17-2943-2017, 2017.
Liu, B., Asseng, S., Liu, L., Tang, L., Cao, W., and Zhu, Y.: Testing the responses of four wheat crop models to heat stress at anthesis and grain filling, Glob. Change Biol., 22, 1890–1903, https://doi.org/10.1111/gcb.13212, 2016.
Liu, S., Mo, X., Lin, Z., Xu, Y., Ji, J., Wen, G., and Richey, J.: Crop yield responses to climate change in the Huang-Huai-Hai Plain of China, Agr. Water Manage., 97, 1195–1209, https://doi.org/10.1016/j.agwat.2010.03.012, 2010.
Liu, Z., Doherty, R. M., Wild, O., O'Connor, F. M., and Turnock, S. T.: Tropospheric ozone changes and ozone sensitivity from the present day to the future under shared socio-economic pathways, Atmos. Chem. Phys., 22, 1209–1227, https://doi.org/10.5194/acp-22-1209-2022, 2022.
LRTAP: Mapping critical levels for vegetation, chapter III of manual on methodologies and criteria for modelling and mapping critical loads and levels and air pollution effects, risks and trends, UNECE Convention on Long-range Transboundary Air Pollution, 2017.
Lu, C. and Fan, L.: Winter wheat yield potentials and yield gaps in the North China Plain, Field Crops Res., 143, 98–105, https://doi.org/10.1016/j.fcr.2012.09.015, 2013.
Luo, Y., Zhang, Z., Li, Z., Chen, Y., Zhang, L., Cao, J., and Tao, F.: Identifying the spatiotemporal changes of annual harvesting areas for three staple crops in China by integrating multi-data sources, Environ. Res. Lett., 15, 074003, https://doi.org/10.1088/1748-9326/ab80f0, 2020.
Luo, Y., Zhang, Z., Zhang, L., and Cao, J.: Spatiotemporal patterns of winter wheat phenology and its climatic drivers based on an improved pDSSAT model, Sci. China Earth Sci., 64, 2144–2160, https://doi.org/10.1007/s11430-020-9821-0, 2021.
Malhi, G. S., Kaur, M., and Kaushik, P.: Impact of Climate Change on Agriculture and Its Mitigation Strategies: A Review, Sustainability, 13, 1318, https://doi.org/10.3390/su13031318, 2021.
Mariën, B., Balzarolo, M., Dox, I., Leys, S., Marchand J. L., Géron, C., Portillo-Estrada, M., AbdElgawad, H., Asard, H., and Campioli, M.: Detecting the onset of autumn leaf senescence in deciduous forest trees of the temperate zone, New Phytol., 224, 166–176, https://doi.org/10.1111/nph.15991, 2019.
Masutomi, Y.: The appropriate analytical solution for coupled leaf photosynthesis and stomatal conductance models for C3 plants, Ecol. Model., 481, 110306, https://doi.org/10.1016/j.ecolmodel.2023.110306, 2023.
Medlyn, B. E., Dreyer, E., Ellsworth, D., Forstreuter, M., Harley, P., Kirschbaum, M., Roux, X., Montpied, P., Strassemeyer, J., Walcroft, A., Wang, K., and Loustau, D.: Temperature response of parameters of a biochemically based model of photosynthesis. II. A review of experimental data, Plant Cell Environ., 25, 1167–1179, https://doi.org/10.1046/j.1365-3040.2002.00891.x, 2002.
Miller, J. D., Arteca, R. N., and Pell, E. J.: Senescence-Associated Gene Expression during Ozone-Induced Leaf Senescence in Arabidopsis, Plant Physiol., 120, 1015, https://doi.org/10.1104/pp.120.4.1015, 1999.
Mulvaney, M. J. and Devkota, P. J.: Adjusting Crop Yield to a Standard Moisture Content, EDIS, University of Florida George A Smathers Libraries, https://doi.org/10.32473/edis-ag442-2020, 2020.
Nagarajan, S., Rane, J., Maheswari, M., and Gambhir, P. N.: Effect of Post-Anthesis Water Stress on Accumulation of Dry Matter, Carbon and Nitrogen and Their Partitioning in Wheat Varieties Differing in Drought Tolerance, J. Agron. Crop Sci., 183, 129–136, https://doi.org/10.1046/j.1439-037x.1999.00326.x, 1999.
Nguyen, T. H., Cappelli, G. A., Emberson, L., Ignacio, G. F., Irimescu, A., Francesco, S., Fabrizio, G., Booth, N., Boldeanu, G., Bermejo, V., Bland, S., Frei, M., Ewert, F., and Gaiser, T.: Assessing the spatio-temporal tropospheric ozone and drought impacts on leaf growth and grain yield of wheat across Europe through crop modeling and remote sensing data, Eur. J. Agron., 153, 127052, https://doi.org/10.1016/j.eja.2023.127052, 2024.
Okrah, A., Li, S., Agathokleous, E., and Feng, Z.: Elevated ozone effects on potato leaf physiology, growth, and yield: a meta-analysis, Environ. Sci. Pollut. Res., 30, 120483–120495, https://doi.org/10.1007/s11356-023-30854-5, 2023.
Osborne, S., Pandey, D., Mills, G., Hayes, F., Harmens, H., Gillies, D., Bücker, P., and Emberson, L.: New Insights into Leaf Physiological Responses to Ozone for Use in Crop Modelling, Plants, 8, 84, https://doi.org/10.3390/plants8040084, 2019.
Osborne, T., Gornall, J., Hooker, J., Williams, K., Wiltshire, A., Betts, R., and Wheeler, T.: JULES-crop: a parametrisation of crops in the Joint UK Land Environment Simulator, Geosci. Model Dev., 8, 1139–1155, https://doi.org/10.5194/gmd-8-1139-2015, 2015.
Pande, P., Hayes, F., Bland, S., Booth, N., Pleijel, H., and Emberson, L. D.: Ozone Dose-Response Relationships for Wheat Can Be Derived Using Photosynthetic-Based Stomatal Conductance Models, Agr. Forest Meteorol., 356, 110150, https://doi.org/10.1016/j.agrformet.2024.110150, 2024.
Penning de Vries, F. W. T.: Simulation of Ecophysiological Processes of Growth in Several Annual Crops, Simulation Monographs, Vol. 29, Netherlands Centre for Agricultural Publishing and Documentation, Int. Rice Res. Inst., 271 pp., ISBN 9022010007, 9789022010006, 1989.
Pleijel, H., Danielsson, H., Emberson, L., Ashmore, M., and Mills, G.: Ozone Risk Assessment for Agricultural Crops in Europe: Further Development of Stomatal Flux and Flux–Response Relationships for European Wheat and Potato, Atmos. Environ., 41, 3022–3040, https://doi.org/10.1016/j.atmosenv.2006.12.002, 2007.
Pleijel, H., Danielsson, H., and Broberg, M. C.: Benefits of the Phytotoxic Ozone Dose (POD) index in dose-response functions for wheat yield loss, Atmos. Environ., 268, 118797, https://doi.org/10.1016/j.atmosenv.2021.118797, 2022.
Qin, X., Zhang, F., Liu, C., Yu, H., Cao, B., Tian, S., Liao, Y., and Siddique, K.: Wheat Yield Improvements in China: Past Trends and Future Directions, Field Crops Res., 177, 117–124, https://doi.org/10.1016/j.fcr.2015.03.013, 2015.
Ronan, A. C., Ducker, J. A., Schnell, J. L., and Holmes, C. D.: Have improvements in ozone air quality reduced ozone uptake into plants?, Elem. Sci. Anth., 8, 2, https://doi.org/10.1525/elementa.399, 2020.
Schauberger, B., Rolinski, S., Schaphoff, S., and Müller, C.: Global Historical Soybean and Wheat Yield Loss Estimates from Ozone Pollution Considering Water and Temperature as Modifying Effects, Agr. Forest Meteorol., 265, 1–15, https://doi.org/10.1016/j.agrformet.2018.11.004, 2019.
Sharkey, T. D., Bernacchi, C. J., Farquhar, G. D., and Singsaas, E. L.: Fitting Photosynthetic Carbon Dioxide Response Curves for C3 Leaves, Plant Cell Environ., 30, 1035–1040, https://doi.org/10.1111/j.1365-3040.2007.01710.x, 2007.
Shi, G., Yang, L., Wang, Y., Kobayashi, K., Zhu, J., Tang, H., Pan, S., Chen, T., Liu, G., and Wang, Y.: Impact of elevated ozone concentration on yield of four Chinese rice cultivars under fully open-air field conditions, Agr. Ecosyst. Environ., 131, 178–184, https://doi.org/10.1016/j.agee.2009.01.009, 2009.
Sillmann, J., Aunan, K., Emberson, L., Bücker, P., van Oort, B. V., O'Neill, C., Otero, N., Pandey, D., and Brisebois, A.: Combined Impacts of Climate and Air Pollution on Human Health and Agricultural Productivity, Environ. Res. Lett., 16, 074001, https://doi.org/10.1088/1748-9326/ac1df8, 2021.
Silvestro, P. C., Pignatti, S., Yang, H., Yang, G., Pascucci, S., Castaldi, F., and Casa, R.: Sensitivity analysis of the AquaCrop and SAFY crop models for the assessment of water-limited winter wheat yield in regional scale applications, PLoS ONE, 12, e0187485, https://doi.org/10.1371/journal.pone.0187485, 2017.
Simpson, D., Benedictow, A., Berge, H., Bergström, R., Emberson, L. D., Fagerli, H., Flechard, C. R., Hayman, G. D., Gauss, M., Jonson, J. E., Jenkin, M. E., Nyíri, A., Richter, C., Semeena, V. S., Tsyro, S., Tuovinen, J.-P., Valdebenito, Á., and Wind, P.: The EMEP MSC-W chemical transport model – technical description, Atmos. Chem. Phys., 12, 7825–7865, https://doi.org/10.5194/acp-12-7825-2012, 2012.
Sitch, S., Cox, P. M., Collins, W. J., and Huntingford, C.: Indirect Radiative Forcing of Climate Change Through Ozone Effects on the Land-Carbon Sink, Nature, 448, 791–795, https://doi.org/10.1038/nature06059, 2007.
Tao, F., Zhang, S., and Zhang, Z.: Spatiotemporal changes of wheat phenology in China under the effects of temperature, day length and cultivar thermal characteristics, Eur. J. Agron., 43, 201–212, https://doi.org/10.1016/j.eja.2012.06.004, 2012.
Tao, F., Feng, Z., Tang, H., Chen, Y., and Kobayashi, K.: Effects of Climate Change, CO2 and O3 on Wheat Productivity in Eastern China, Singly and in Combination, Atmos. Environ., 153, 182–193, https://doi.org/10.1016/j.atmosenv.2017.01.032, 2017.
Triboi, E. and Triboi-Blondel, A. M.: Productivity and Grain or Seed Composition: A New Approach to an Old Problem – Invited Paper, Eur. J. Agron., 16, 163–186, https://doi.org/10.1016/S1161-0301(01)00146-0, 2002.
Van Oijen, M. and Ewert, F.: The effects of climatic variation in Europe on the yield response of spring wheat cv. Minaret to elevated CO2 and O3: an analysis of open-top chamber experiments by means of two crop growth simulation models, Eur. J. Agron., 10, 249–264, https://doi.org/10.1016/s1161-0301(99)00014-3, 1999.
Vazquez-Cruz, M. A. and Guzman, R.: Global sensitivity analysis by means of EFAST and Sobol' methods and calibration of the reduced state-variable TOMGRO model using genetic algorithms, Comput. Electron. Agric., 100, 1–12, https://doi.org/10.1016/j.compag.2013.10.006, 2014.
Wallach, D.: Crop model calibration: A statistical perspective. Agron. J., 103, 1141–1153, https://doi.org/10.2134/agronj2010.0432, 2011.
Wang, Q. J.: Using Genetic Algorithms to Optimise Model Parameters, Environ. Model. Softw., 12, 27–34, https://doi.org/10.1016/S1364-8152(96)00030-8, 1997.
Yadav, D. S., Mishra, A. K., Rai, R., Chaudhary, N., Mukherjee, A., Agrawal, S. B., and Agrawal, M.: Responses of an Old and a Modern Indian Wheat Cultivar to Future O3 Levels: Physiological, Yield and Grain Quality Parameters, Environ. Pollut., 263, 113939, https://doi.org/10.1016/j.envpol.2020.113939, 2020.
Yang, L., Liu, S., Tsoka, S., and Papageorgiou, L. G.: Mathematical Programming for Piecewise Linear Regression Analysis, Expert Syst. Appl., 44, 156–167, https://doi.org/10.1016/j.eswa.2015.08.034, 2016.
Zhang, X., Xu, W., Zhang, G., Lin, W., Zhao, H., Ren, S., Zhou, G., Chen, J., and Xu, X.: First long-term surface ozone variations at an agricultural site in the North China Plain: Evolution under changing meteorology and emissions, Sci. Total Environ., 860, 160520, https://doi.org/10.1016/j.scitotenv.2022.160520, 2023.
Zhao, P., Zhou, Y., Li, F., Ling, X., Deng, N., Peng, S., and Man, J.: The Adaptability of APSIM-Wheat Model in the Middle and Lower Reaches of the Yangtze River Plain of China: A Case Study of Winter Wheat in Hubei Province, Agronomy, 10, 981, https://doi.org/10.3390/agronomy10070981, 2020.
Zheng, B., Chenu, K., Doherty, A., and Chapman, S.: The APSIM-Wheat Module (7.5 R3008), APSIM Initiative, 44 pp., https://www.apsim.info/documentation/model-documentation/crop-module-documentation/wheat/ (last access: 28 December 2024), 2015.
Zhu, X., Feng, Z., Sun, T., Liu, X., Tang, H., Zhu, J., Guo, W., and Kobayashi, K.: Effects of Elevated Ozone Concentration on Yield of Four Chinese Cultivars of Winter Wheat Under Fully Open-Air Field Conditions, Glob. Change Biol., 17, 2697–2706, https://doi.org/10.1111/j.1365-2486.2011.02400.x, 2011.