the Creative Commons Attribution 4.0 License.
the Creative Commons Attribution 4.0 License.
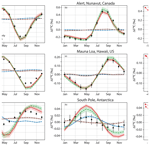
No increase is detected and modeled for the seasonal cycle amplitude of δ13C of atmospheric carbon dioxide
Sebastian Lienert
Sönke Zaehle
Measurements of the seasonal cycle of δ13C of atmospheric CO2 (δ13Ca) provide information on the global carbon cycle and the regulation of carbon and water fluxes by leaf stomatal openings on ecosystem and decadal scales. Land biosphere carbon exchange is the primary driver of δ13Ca seasonality in the Northern Hemisphere (NH). We use isotope-enabled simulations of the Bern3D-LPX (Land surface Processes and eXchanges) Earth system model of intermediate complexity and fossil fuel emission estimates with a model of atmospheric transport to simulate atmospheric δ13Ca at globally distributed monitoring sites. Unlike the observed growth of the seasonal amplitude of CO2 at northern sites, no significant temporal trend in the seasonal amplitude of δ13Ca was detected at most sites, consistent with the insignificant model trends. Comparing the preindustrial (1700) and modern (1982–2012) periods, the modeled small-amplitude changes at northern sites are linked to the near-equal increase in background atmospheric CO2 and the seasonal signal of the net atmosphere–land δ13C flux in the northern extratropical region, with no long-term temporal changes in the isotopic fractionation in these ecosystems dominated by C3 plants. The good data–model agreement in the seasonal amplitude of δ13Ca and in its decadal trend provides implicit support for the regulation of stomatal conductance by C3 plants towards intrinsic water use efficiency growing proportionally to atmospheric CO2 over recent decades. Disequilibrium fluxes contribute little to the seasonal amplitude of the net land isotope flux north of 40° N but contribute near equally to the isotopic flux associated with growing season net carbon uptake in tropical and Southern Hemisphere (SH) ecosystems, pointing to the importance of monitoring δ13Ca over these ecosystems. We propose applying seasonally resolved δ13Ca observations as an additional constraint for land biosphere models and underlying processes for improved projections of the anthropogenic carbon sink.
- Article
(4290 KB) - Full-text XML
-
Supplement
(692 KB) - BibTeX
- EndNote
The seasonal variations in the carbon exchange fluxes between the atmosphere and the surface cause a seasonal cycle in atmospheric CO2 (Ca) (Keeling et al., 1996; Graven et al., 2013; Masarie et al., 2014) and its stable isotopic signature (δ13Ca) (Keeling, 1960; Keeling et al., 1984, 1989, 2005; GLOBALVIEW-CO2C13, 2009), with δ13C defined as δ13C = , where Rsample and Rstd are the abundance ratios of the sample and the carbonate standard Pee Dee Belemnite (PDB; 0.0112372), respectively (Craig, 1957). Observations of the atmospheric seasonal cycles in background tropospheric air provide large-scale information on the carbon fluxes between the atmosphere, ocean, and land (Heimann et al., 1989, 1998) and constraints for models used to project Ca and global warming.
The additional information of δ13C data in comparison to carbon data stems from differences in fractionation for different carbon fluxes. Carbon isotopic fractionation describes the preferential transfer of light 12C compared to heavier 13C. The degree of fractionation is different for the different physical, chemical, and biological processes (Mook, 1986), causing differences in the isotopic composition of carbon reservoirs and fluxes. The seasonal δ13Ca variations result from the combination of carbon and isotopic fluxes from fossil fuel burning, land use, and the exchange of the atmosphere with the ocean and land biosphere. Comparing results of carbon-isotope-enabled models with observations of δ13Ca is useful to assess whether the mix of carbon and isotopic sink and source fluxes is represented consistently in comparison with the observations. δ13Ca observations offer, therefore, a benchmark for evaluating and improving Earth system models.
Fractionation is particularly large during the assimilation of CO2 from the atmosphere by plants following the C3 photosynthesis pathway, which are responsible for most of the global productivity (Still et al., 2003). Importantly, changes in isotopic fractionation by C3 plants are indicative of changes in stomatal conductance, regulating the leaf-internal CO2 mole fraction and thus photosynthesis (Farquhar, 1989; Saurer and Voelker, 2022; Cernusak and Ubierna, 2022). Photosynthesis, the associated water loss, and evaporative cooling are key characteristics of ecosystem function that are central to the cycles of carbon, nitrogen, water, and energy (Keenan et al., 2013; Knauer et al., 2017) and to the land sink of anthropogenic carbon. Acquisition of CO2 for photosynthesis is accompanied by the loss of water through the stomatal pores that govern, by their conductance, the diffusion of these two gases between the leaf interior and the atmosphere. A key question is how ecosystems adjust their overall conductance and, thereby, co-regulate carbon uptake and plant growth, regulating water loss and evaporative cooling under rising Ca, growing nitrogen inputs to ecosystems, and increasing water vapor deficits under global warming. Many studies relying on multi-decadal- to century-scale tree-ring δ13C records and free-air CO2 enrichment (FACE) experiments suggest that small changes in isotopic fractionation and intrinsic water use efficiency, the ratio of assimilation to conductance, grow roughly proportionally with Ca (Voelker et al., 2016; Saurer et al., 2014; Kauwe et al., 2013; Peñuelas et al., 2011; Keller et al., 2017; Frank et al., 2015). In contrast, Battipaglia et al. (2013) and Keenan et al. (2013) suggest a scenario where conductance and the flows of carbon and water are downregulated under increasing Ca. Keeling et al. (2017), analyzing decadal-scale change in seasonally detrended δ13Ca and the annual atmospheric budgets of carbon and 13C, find a decrease in isotopic fractionation of global mean net primary production; the change is attributed to changes in fractionation associated with mesophyll conductance and photorespiration of C3 plants, and intrinsic water use efficiency is inferred to grow proportionally with Ca. Conflicting results for 20th-century changes in fractionation and intrinsic water use efficiency are also found in global land biosphere models (Keller et al., 2017).
The observational records from globally distributed monitoring sites (Keeling et al., 1996; Graven et al., 2013; Masarie et al., 2014) demonstrate a significant growth trend in the seasonal cycle amplitude (SA) of Ca (Bacastow et al., 1985; Keeling et al., 1996; Graven et al., 2013; Barlow et al., 2015; Piao et al., 2018) driven by changes in the seasonality of net land carbon uptake (Graven et al., 2013; Forkel et al., 2016). The observed seasonal cycle and amplitude growth of Ca are widely used to evaluate carbon cycle models and system understanding by transporting fluxes from terrestrial, oceanic, and fossil fuel sources with a model of atmospheric transport to obtain local Ca anomalies (Heimann et al., 1998; Dargaville et al., 2002; Scholze et al., 2008; Peng et al., 2015; Lienert and Joos, 2018). Studies address the role of different climatic drivers and terrestrial carbon cycle processes such as drought, land use, warming, productivity, and soil respiration (Heimann et al., 1989, 1998; Graven et al., 2013; Forkel et al., 2016; Ito et al., 2016; Bastos et al., 2019; Wang et al., 2020) and surface-to-atmosphere C fluxes (e.g., Peylin et al., 2013). SAs(Ca) and their temporal trends at different monitoring sites are used for constraining an ensemble of land biosphere model simulations (Lienert and Joos, 2018).
Comparable studies analyzing the temporal trends in SA(δ13Ca) and the seasonal cycle of δ13Ca are scarce. While seasonally resolved atmospheric δ13Ca measurements are available (GLOBALVIEW-CO2C13, 2009; Keeling et al., 2001), these seasonally resolved records are yet to be fully utilized in the context of process-based carbon cycle models. Heimann et al. (1989) simulated the spatiotemporal distribution of δ13Ca and Ca with an atmospheric transport model using estimates of net primary production (NPP) and heterotrophic respiration based on satellite data and surface temperature and prescribed surface ocean CO2, demonstrating the dominant role of land biosphere fluxes for Northern Hemisphere (NH) seasonality and finding relevant signals from the ocean and land in the Southern Hemisphere (SH). Van der Velde et al. (2018) applied their CarbonTracker Data Assimilation System for CO2 and 13CO2 by varying the net exchange fluxes of CO2 and 13CO2 in ocean and terrestrial biosphere models and propagating the fluxes through an atmospheric transport model to solve for weekly adjustments to fluxes and isotopic terrestrial discrimination, minimizing differences between observed and estimated mole fractions. They identified a decrease in stomatal conductance on a continent-wide scale during a severe drought. Ballantyne et al. (2011) applied an analytical regression approach to analyze the differences in isotopic signatures between Northern Hemisphere site data and free-troposphere background data from Niwot Ridge to infer seasonal variations in the source signature of the net atmosphere–land biosphere flux and to evaluate models of stomatal conductance. Observations of δ13Ca seasonal cycles were used to investigate isotopic fractionation (Ballantyne et al., 2010) and trends in the phenology of northern terrestrial ecosystems (Gonsamo et al., 2017) but to our knowledge have not been used for analyzing trends in SA(δ13Ca) globally.
This study addresses the following main questions:
-
Is the seasonal cycle of δ13Ca observed at a network of globally distributed sites represented well in model simulations? How large are the contributions of ocean, land, and fossil fuel fluxes to δ13Ca seasonality?
-
What are the temporal trends in SA(δ13Ca) in the observational records, and are the modeled trends in SA(δ13Ca) consistent with the observed trends?
-
What are the different drivers of SA(δ13Ca) versus SA(Ca) and of their temporal trends? Is a model scenario with intrinsic water use efficiency growing proportional with Ca consistent with δ13Ca seasonality data?
We simulate atmospheric δ13Ca and Ca at 19 globally distributed sites using the matrix representation of an atmospheric transport model and net atmosphere-to-surface fluxes of CO2 and δ13(CO2) from an Earth system model of intermediate complexity (EMIC) alongside gridded fossil fuel emission estimates and changes in land use and the distribution of C3 and C4 crops. We compare model results to observations and analyze trends in SA(δ13Ca) using the records of the Scripps CO2 program (Keeling et al., 2001) and the Cooperative Global Atmospheric Data Integration Project (2013) product. We discuss the implications of our results for changes in the fractionation by C3 plants, their stomatal controls, and associated carbon and water fluxes. We develop a theoretical framework to explain the trends in SA(δ13Ca) and decompose net carbon and isotope land biosphere fluxes into underlying component fluxes and changes in carbon fluxes and fractionation. The framework could serve future studies, e.g., studies applying an ensemble of different models for multi-model evaluation, and provide more robust conclusions in comparison to using a single model chain.
2.1 Bern3D-LPX Earth system model of intermediate complexity
Spatially resolved surface-to-atmosphere CO2 and 13CO2 fluxes are simulated with the Bern3D-LPX (Land surface Processes and eXchanges) Earth system model of intermediate complexity. Here, the ocean–atmosphere model Bern3D (Jeltsch-Thömmes and Joos, 2020; Battaglia and Joos, 2018; Ritz et al., 2011) is coupled to the dynamic global vegetation model (DGVM) framework of the Land surface Processes and eXchanges (LPX) model, LPX-Bern v1.4 (Lienert and Joos, 2018). The Bern3D model features a 41 × 40 horizontal ocean grid (about 9° × 4.5°) with 32 depth layers coupled to a single-layer energy–moisture balance atmosphere (Ritz et al., 2011). In Bern3D, carbon and its isotopes are implemented as tracers with fractionation for air–sea and sea–air gas exchange, aquatic chemistry, and the production of organic material and CaCO3 as a function of surface ocean temperature, aqueous CO2, and the speciation of dissolved inorganic carbon, as described by Jeltsch-Thömmes and Joos (2023). LPX-Bern simulates the coupled cycling of carbon, nitrogen, and water (Xu-Ri and Prentice, 2008; Wania et al., 2009a, b; Stocker et al., 2014) and vegetation dynamics using plant functional types (Sitch et al., 2003). It is run here at a 3.75° × 2.5° resolution. Grid cells are subdivided into different land use classes (mineral soil, wetlands, crop, pasture, urban). Carbon isotopes were added (Scholze et al., 2003) using a photosynthetic fractionation scheme (Lloyd and Farquhar, 1994) and without further isotopic fractionation during the transfer through the vegetation, litter, soil, and product pools. The scheme neglects fractionation by boundary layer transport and ternary effects associated with the interaction of CO2, water, and air (Farquhar and Cernusak, 2012), and fractionation by dark-day respiration is set to zero, while fractionation by the following terms is explicitly considered: stomatal conductance (with a scaling factor of 4.4 ‰), dissolution and liquid transport (1.8 ‰), carboxylation (27.5 ‰), and photorespiration (8 ‰ and the CO2 compensation point that would occur in the absence of dark respiration, Γ*, increases with temperature) (Lloyd and Farquhar, 1994). The signature of respired carbon reflects the signature of carbon assimilated at previous times; the lag times between assimilation and respiration are dictated by the turnover timescales of the various pools, depending on temperature and soil moisture. Land carbon and isotope fluxes respond to altered climate, which influences, for example, photosynthesis through temperature and water limitation, fire frequency, and autotrophic and heterotrophic respiration rates; respond to increasing Ca, which stimulates photosynthesis and affects water use efficiency (“CO2 fertilization”); respond to land use (Strassmann et al., 2008), which causes, for example, transfer of tree carbon to the atmosphere, litter, and product pools after deforestation and shifts from natural vegetation to C3 and C4 crops and pasture; and respond to altered nitrogen deposition and the addition of nitrogen fertilizer on managed land alleviating nitrogen limitation.
Bern3D and LPX-Bern were spun up individually, followed by a 500-year coupled spinup to preindustrial equilibrium (1700 CE; 276.3 ppm, −6.27 ‰). A transient simulation, Estandard, from 1700 to 2020 is driven by annual fossil fuel carbon emissions (including the contribution from cement production) (Friedlingstein et al., 2020), net land use area changes (Hurtt et al., 2020), and non-CO2 radiative forcing. δ13C of the fossil fuel emissions follows Andres et al. (2017) for 1751–2014 and is set to the value for 1751 before. For 2014–2020, signatures of major source categories (coal, oil, gas, cement) are assumed to be constant and combined with the emission sources from Friedlingstein et al. (2020), following the approach of Andres et al. (2000). Here, we explicitly distinguish land use classes for C3 and C4 crops and prescribe their extent, and net land use area changes are based on the Land-Use Harmonization 2 dataset (Hurtt et al., 2020). Nitrogen deposition and nitrogen fertilization are taken from the Global N2O Model Intercomparison Project (Tian et al., 2018). Nitrogen (N) is a limiting nutrient in LPX, and plant growth is downregulated under N stress, which tends to reduce plant growth and plant growth responses to rising Ca compared to a model with absent N cycling. The monthly wind stress climatology from the NCEP/NCAR Reanalysis produced by the National Centers for Environmental Prediction (NCEP) and the National Center for Atmospheric Research (NCAR) (Kalnay et al., 1996) is prescribed to the ocean. Climatic Research Unit (CRU) Time Series (TS) version 4.05 high-resolution gridded data of month-by-month variation in climate (CRU TS v4.05) (Harris et al., 2020) are used for the land model. For 1700–1900 and the spinup, the climate of 1901–1931 is recycled. A baseline is provided by a control simulation, termed Econtrol, without anthropogenic CO2 emissions; absent radiative forcing from non-CO2 species (e.g., from CH4, N2O, ozone); and with land use, nitrogen deposition, and nitrogen fertilization at the 1700 level, as well as recycling the 1901–1931 land climate. Ca and δ13Ca evolve freely in all simulations presented and remain at their preindustrial values in Econtrol.
2.2 Atmospheric transport model TM3 and the seasonal cycles of Ca and δ13Ca
We employ the transport matrices of the global atmospheric tracer model TM3, a three-dimensional transport model (Heimann and Körner, 2003; Kaminski et al., 1998; Schürmann et al., 2016), to translate surface–atmosphere fluxes from Bern3D-LPX and fossil fuel emissions into Ca and δ13Ca anomalies at 19 measurement sites across the globe. Before transport, the fluxes are remapped to the TM3 grid with 72 × 48 grid cells (5° × 3.75°). Here, the matrices span 1982 to 2012 and are only available if there is also a CO2 measurement available at the corresponding site. Each matrix represents the sensitivity of the local atmospheric concentration for a given month to the local surface fluxes of the previous period, spanning up to 48 months. The transport model is initialized with equal Ca and δ13Ca at all sites.
For 13C, the signature-weighted net atmosphere-to-surface flux is
δ13fas,net is in units of mol ‰ m−2 yr−1. x indicates location and t time at the monthly and spatial (5° × 3.75°) resolution of TM3. The net carbon fluxes (fas,net; mol m−2 yr−1); their signatures (δ13Cas,net); and, therefore, δ13fas,net, are readily available for fossil fuel emissions, including cement production (Andres et al., 2009a, b). Bern3D-LPX simulates two-way exchange of CO2 and 13CO2 from and to the ocean and land surface. Net transfer rates are determined by the difference in these gross fluxes to yield the atmosphere-to-surface net fluxes, fas,net and δ13fas,net, of Bern3D-LPX.
The matrices are applied with fas,net to compute anomalies in Ca and with δ13fas,net to compute anomalies in 13CO2. We get δ13Ca from . This method of transporting signature-weighted net fluxes was chosen instead of separately transporting 13CO2 and 12CO2. Both approaches were tested and showed very similar results, except for numerical issues in months having very small local 12CO2 anomalies for the second approach.
Ocean, land, and fossil fuel fluxes from the standard simulation are transported separately to quantify the contributions of these individual components to the seasonal variations in Ca and δ13Ca. For Econtrol, fossil fuel fluxes are not transported, consistent with the model setup. A limitation is that transport matrices are only available for the period of 1982 to 2012, limiting the analysis period and direct model–data comparison to 3 decades only.
2.3 Site data
Background CO2 from 19 monitoring sites for which transport matrices are available is used for comparison with simulated Ca and δ13Ca and to determine observation-based trends in their SAs. The Cooperative Global Atmospheric Data Integration Project (2013) product is used for Ca. For δ13Ca, the records of the Scripps CO2 program (Keeling et al., 2001) for Alert, Mauna Loa, and the South Pole from monthly averaged flask data are used. These records span a longer period than the available transport matrices. For the remaining 16 sites, the shorter (1994 to 2009) records of GLOBALVIEW-CO2C13 (2009) are used. In the main paper, we focus on 3 out of the 19 available transport sites: Alert (82.5° N, Canada), Mauna Loa (19.5° N, Hawaii), and the South Pole (90° S, Antarctica). Results for the other sites are shown in the Supplement and Table 1. The Scripps and GLOBALVIEW-CO2C13 data are on slightly different scales (Lueker et al., 2020); this does not affect our analysis of seasonal anomalies. The δ13Ca records of GLOBALVIEW-CO2C13 (2009) span the order of a decade and are, therefore, not used for trend detection, although we evaluated trends from the simulations for the GLOBALVIEW sites (Table 2, excluding Key Biscayne). We require at least 10 monthly values for a year to be included in the linear regression.
Additional δ13Ca monthly flask data from the Scripps CO2 program (Keeling et al., 2001) are used for analyzing temporal trends in the SA(δ13Ca). We focus on eight sites with more than 20 years of data: Alert (ALT, 82° N), Nuvuk (formerly Point Barrow) (PTB, 71° N), La Jolla (LJO, 33° N), Mauna Loa Observatory (MLO, 20° N), Cape Kumukahi (KUM, 20° N), Christmas Island (CHR, 2° N), Samoa (SAM, 14° S), and the South Pole (SPO, 90° S). The data are provided as (i) monthly samples, (ii) a fit to these monthly samples, and (iii) the monthly samples but with missing values replaced by fitted values. We also used the original, non-gap-filled data and years with at least 9, 10, or 11 monthly values per year in the regression.
We develop a simplified conceptual framework to qualitatively explore the influence of carbon and isotope fluxes on the seasonal cycles of Ca and δ13Ca. For illustration, the atmosphere is considered to be well-mixed in this section; the atmospheric transport operator is linear, and the findings may qualitatively also apply to spatially resolved fluxes. The budgets for the atmospheric inventories of carbon and 13C are approximated (Tans et al., 1993) as
Na and Na⋅δ13Ca are the atmospheric inventories of carbon and (approximately) of 13C (in mol ‰). Fas,net and are the globally integrated net atmosphere-to-surface carbon and 13C flux; is the signature of the global net carbon flux. We set , where c is a unit conversion factor. Solving Eqs. (2) and (3) for the changes in Ca and δ13Ca yields
with being the global integral of
The superscript * indicates that the 13C fluxes (e.g., in units of mol ‰ yr−1 m−2 for ) are referenced to the atmospheric signature. Equation (6) corresponds to Eq. (1) for the net atmosphere-to-surface isotopic flux but is now referenced to the atmospheric signature instead of the signature of 0 ‰ of the Vienna Pee Dee Belemnite standard as in Eq. (1). In this way, a positive (negative) flux causes a negative (positive) change in δ13Ca.
Equations (4) and (5) are readily integrated over the growing season from the intra-annual maximum to the minimum (subscripts indicate max and min) in Ca and the corresponding beginning, tbeg, and end, tend, of the growing season to get the seasonal cycle amplitude (SA) for the two tracers and (cumulative) net fluxes (see Appendix A for calculation of SA for a flux):
Equations (5) and (8) provide important insights. First, changes in δ13Ca and its seasonal cycle are driven by ; seasonal changes in Ca, the denominator in Eq. (5), are small compared to Ca, and Ca is considered constant within a given year (the error associated with this approximation is less than 3 %). Second, the background CO2 mole fraction, Ca, modulates the magnitude of the δ13Ca seasonal cycle. SA(δ13Ca) would be larger under low preindustrial Ca than under modern Ca for equal seasonal variations in . Correspondingly, SA(δ13Ca) does not change over time as long as the relative changes in SA() and in Ca are equal. Equations (7) and (8) were derived for a globally well-mixed atmosphere and global fluxes but analogously also apply for the tracer seasonality at individual sites, with the integral on the right-hand side of Eqs. (7) and (8) representing the integral of (transport-weighted) fluxes over the region influencing tracer seasonality at the site. We recall that the above equations and conclusions were derived by assuming a well-mixed atmosphere, while in reality spatial flux patterns and transport and their changes influence seasonal cycles at individual atmospheric sites. Further, the start and end of the growing season are assumed to coincide with the switch in the sign of the isotopic flux; this is the case in our model for zonally integrated fluxes. These seasonal fluxes will be presented in Sect. 4.3. Equations (2) to (8) are for illustrating the influence of carbon and carbon isotope fluxes on the seasonal cycles of Ca and δ13Ca; they were not used for calculating numerical results.
The notation and sign convention introduced above are applied in this paper. In brief, fi,j defines a one-way flux from the source reservoir i to the receiving reservoir j and is positive. The isotopic signature of this flux is δ13Ci,j. The net flux from reservoir i to reservoir j is and is the difference between the corresponding one-way fluxes; e.g., . is positive if the net flux results in the transfer of mass from i to j.
Table 1The seasonal cycle amplitude of Ca and δ13Ca from the standard simulation (mod; Estandard) and observations (obs) for 19 monitoring sites and the period of 1982–2012. The increase over the industrial period is estimated from the difference between the standard simulation and the preindustrial control ().
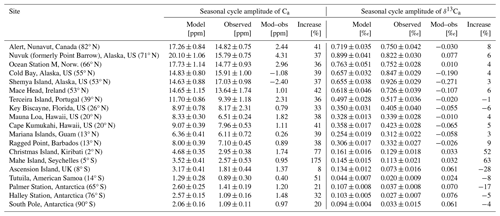
4.1 Seasonal cycles of atmosphere–surface fluxes, Ca, and δ13Ca
The Bern3D-LPX model simulates (Estandard) large seasonal variations in the net land biosphere–atmosphere exchange of CO2 and 13CO2, whereas seasonal variations in ocean–atmosphere fluxes are much smaller (Fig. 1). This seasonality is broadly consistent with estimates of regional air–land carbon flux seasonality from an atmospheric inversion (Gurney et al., 2004) and air–sea flux seasonality from surface ocean pCO2 observations (Landschützer et al., 2014; Takahashi et al., 2009; Fay et al., 2021), except in the Southern Ocean and in the northern subpolar gyres. The LPX land biosphere model shows the expected uptake of isotopically depleted carbon, resulting in positive fas,net and negative during the summer and vice versa in winter.
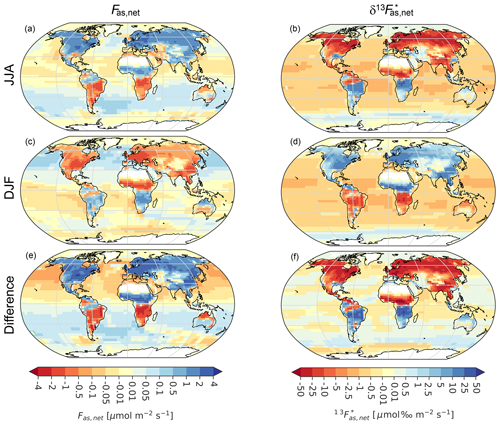
Figure 1Net seasonal atmosphere-to-surface fluxes. Fluxes are for (a, c, e) carbon and (b, d, f) the δ13C-weighted carbon flux, (see Sect. 3), from the standard simulation (Estandard) and are averaged over 1982–2012 for (a, b) June, July, and August (JJA); (c, d) December, January, and February (DJF); and (e, f) JJA minus DJF. Note the non-linear color bars with blue colors in panels (a) to (d) indicating a lowering in atmospheric CO2 and δ13C.
The Bern3D ocean model shows a negative in the low latitudes and midlatitudes, small modern fluxes in the northern subpolar gyres, and a positive flux in the Southern Ocean in both seasons (Fig. 1). These modern Bern3D fluxes are driven by the atmosphere–ocean isotopic disequilibrium, here defined as the isotopic signature of the atmosphere-to-surface carbon flux minus the signature of the surface-to-atmosphere flux (δdis,as; Eq. A2), with a negative δdis,as in the low latitudes and midlatitudes, a small modern disequilibrium in northern high latitudes, and a positive δdis,as south of 50° S, consistent with observations (Menviel et al., 2015; Quay et al., 2017; Becker et al., 2018).
The preindustrial δdis,as and are negative in the low latitudes and midlatitudes and positive in high-latitude ocean regions (not shown), mainly driven by the temperature dependency of isotopic fractionation during air–sea exchange and the cycling of marine biological matter (see Fig. 1 of Menviel et al., 2015, to compare Bern3D and LOVECLIM results for δdis,as). Fossil fuel emissions cause a negative flux perturbation worldwide, shifting the net isotopic fluxes to more negative values over the industrial period.
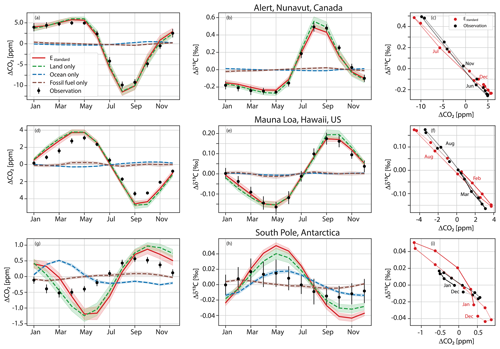
Figure 2The simulated (red) seasonal cycle of atmospheric Ca (left, a, d, g) and its signature δ13Ca (middle, b, e, h) compared to observations (black dots). In the rightmost panels (c, f, i) the seasonal anomalies (Δ) of Ca are plotted against those of δ13Ca, with lines connecting the monthly values (dots) fading from January to December. Results are for Alert, northern Canada (a, b, c); Mauna Loa, Hawaii (d, e, f); and the South Pole (g, h, i). Simulated values are from transporting net TM3 fluxes of the Bern3D-LPX Estandard simulation from all (red, Estandard), terrestrial (green, dashed), oceanic (blue, dashed), and fossil fuel sources (brown, dashed). The observational and model anomalies are computed from monthly values between 1982 and 2012 if both the measurements and transport matrices are available. Error bars and shading correspond to the standard deviation from the interannual variability in monthly values.
Figure 2 compares the mean seasonal cycles of Ca and δ13Ca from Estandard with measurements from 1982 (at Alert from 1985) to 2012 at three sites and with factorial simulations, where the fluxes of the land (dashed green line), ocean (dashed blue line), and fossil fuel emissions (dashed brown lines) were considered individually (see Table 1 and Figs. S1 and S2 in the Supplement for additional sites). For the Northern Hemisphere (NH) sites of Alert (top panels) and Mauna Loa (middle panels), the seasonal variations are dominated by the terrestrial biosphere fluxes, with minor contributions from ocean fluxes and fossil fuel emissions.
Both the timing and amplitude of the observed seasonal cycle of Ca and δ13Ca are captured reasonably well by Estandard (Fig. 2). The simulated SA(Ca) and its interannual variability (IAV) are overestimated compared to observations at Alert (17.3 ± 0.84 ppm versus 14.8 ± 0.75 ppm) and Mauna Loa (8.3 ± 0.30 ppm versus 6.5 ± 0.24 ppm). SA(δ13Ca) matches the observations (ALT – 0.72 ± 0.035 ‰ versus 0.75 ± 0.042 ‰; MLO – 0.34 ± 0.013 ‰ versus 0.33 ± 0.028 ‰). Good model–data agreement in the phasing of the seasonal cycle of Ca relative to δ13Ca is demonstrated for Alert in panel (c), where monthly anomalies in δ13Ca are plotted versus anomalies in Ca. Both the observations and the model show hysteresis throughout the year, with the loop rotating clockwise. At Mauna Loa, the rotation direction of the hysteresis loop is clockwise in the simulation and counterclockwise in the observations (panel f). Still, the observed hysteresis is small, with offsets of less than 0.03 ‰. The hysteresis arises as the ratio between the rate of change in δ13Ca versus the rate of change in Ca varies over the year (Keeling et al., 1989; Heimann et al., 1989). This non-linearity in the atmospheric tracer relationship originates from seasonally varying transport in combination with spatially and temporally varying relationships of atmosphere–surface δ13C to CO2 flux. For example, the isotopic signature of the growing season net atmosphere-to-land carbon flux δ13Cal,net is −13.4 ‰ for the northern high-latitude region (>40° N) but only −10.7 ‰ for the region 10–40° N, and the signal observed at any measurement site results from varying contributions from these and other latitudinal bounds given intra-annually varying winds and hence transport.
Results for the South Pole are different than for the NH sites (Fig. 2g, h, i). Neither the timing nor the amplitude of Ca (2.1 ± 0.16 ppm simulated versus 1.1 ± 0.11 ppm observed) and δ13Ca (0.094 ± 0.004 ‰ versus 0.033 ± 0.015 ‰) agrees with observations. SA(Ca) and SA(δ13Ca) at the South Pole are observed to be 14 and 23 times smaller than at Alert, respectively. The absolute data–model mismatches are therefore not as drastic as the relative mismatches. The disagreement between simulation and observational estimates is also apparent when considering the scatter plot in panel (i). The model shows a complex hysteresis relationship, whereas the observations display a clockwise loop.
The remote Antarctic sites (the South Pole, Palmer, and Halley) show an expected relatively larger dependence on the ocean, but the terrestrial contribution still dominates in the model (Figs. 2, S1, and S2). The Ca seasonal cycle resulting from atmosphere–ocean flux is shifted by up to 6 months compared to observations at the South Pole and the other two Antarctic sites (Palmer, Halley; blue lines versus black dots in Fig. S1), pointing to biases in the Bern3D ocean flux. Observation-based analyses indicate stronger ocean CO2 uptake in summer than in winter in the Southern Ocean (Jin et al., 2024; Long et al., 2021; Fay et al., 2021) in contrast to results from Bern3D (Fig. 1) and more complex ocean models (Hauck and Völker, 2015) and several Earth system models from CMIP5 (Majkut et al., 2014) and CMIP6 (Joos et al., 2023). The simulated amplitude and phasing of the δ13Ca seasonal cycle resulting from the ocean are broadly in line with observations at the Antarctic sites (Fig. S2). The air–sea isotopic disequilibrium is large in the Southern Ocean, and the two-way air–sea and sea–air exchange fluxes yield a substantial net isotopic flux, even under low net carbon flux. Temperature-dependent fractionation is higher in winter than in summer, and the air–sea gas exchange piston velocity and, in turn, the isotope fluxes are larger under high winds in winter than in summer in the modeled Southern Ocean, consistent with the observed seasonal phasing of δ13Ca at the Antarctic sites. Errors in modeled Southern Ocean fluxes are expected to have a minor impact on simulated SA(Ca) and SA(δ13Ca) at NH sites, where the influence of land fluxes dominates by far (Figs. 2, S1, S2).
Considering all extratropical Northern Hemisphere sites, model–data mismatches are less than 30 % for SA(Ca) and SA(δ13Ca) and their root-mean-square errors (RMSEs) are 2.6 ppm and 0.14 ‰, respectively. For the tropical and SH sites, large relative data–model deviations of up to 140 % for SA(Ca) and up to 290 % for SA(δ13Ca) are evident, although absolute deviations are less than 1.8 ppm, and 0.18 ‰ and the corresponding RMSEs are 1.2 ppm and 0.05 ‰ (Table 1).
Interannual variability in simulated SA(δ13Ca) compares reasonably well with observations at sites in the NH subtropics and extratropics (average of the 1σ standard deviation of 12 sites is 0.031 ‰ in Estandard versus 0.031 ‰ in observations) and in the tropics and SH (0.009 ‰ versus 0.013 ‰) (Table 1). Similar agreement between simulated and observation-derived IAV holds for SA(Ca) (NH extratropics –0.75 versus 0.96 ppm; tropics and SH – 0.29 versus 0.30). This suggests that the variability in the seasonal amplitude of the carbon and isotope fluxes is reasonably represented by LPX-Bern. The correct simulation of variability can be challenging and van der Velde et al. (2013) report too low an interannual variability in the annually integrated isotopic disequilibrium flux for their model.
4.2 Temporal trends in the seasonal cycle amplitude of δ13Ca and Ca
Detection of trends in SA(δ13Ca) may be hampered by interannual-to-decadal variability, short record lengths, and a small SA(δ13Ca) in comparison to measurement uncertainty and variability, as is typical at Southern Hemisphere sites. For example, dividing SA(δ13Ca) by 2 standard deviations of IAV yields a signal-to-noise ratio (Keller et al., 2014) below 2.7 at SH sites and as low as 1.1 at the South Pole and on American Samoa (Table 1). Thus, SA(δ13Ca) would need to roughly double over the observational period for a trend in SA(δ13Ca) to emerge from the noise of IAV at these two sites. The situation is more favorable for trend detection at NH extratropical sites (Table 1), where the signal-to-noise ratio ranges between 9 and 16, and changes of 6 % to 11 % in SA(δ13Ca) would emerge.
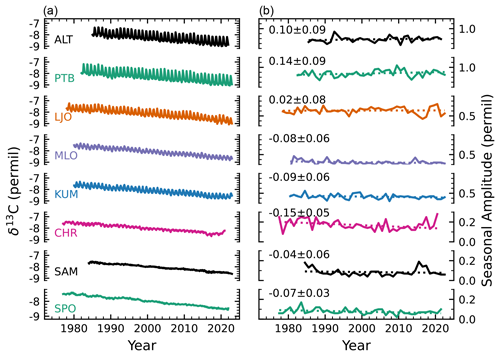
Figure 3Temporal evolution of δ13Ca (a) and its seasonal amplitude (b) from data of the Scripps network (Keeling et al., 2001). Gap-filled data provided by Scripps are used for the eight sites. The slope and its standard error from a linear regression through the seasonal amplitude data (dotted) are given in ‰ per century. Trends are not different from zero based on a two-sided t test and a significance level of 5 %, except at Christmas Island (CHR) and the South Pole (SPO). Sites are ordered according to latitude (Alert (ALT, 82° N), Nuvuk (formerly Point Barrow) (PTB, 71° N), La Jolla (LJO, 33° N), Mauna Loa Observatory (MLO, 20° N), Cape Kumukahi (KUM, 20° N), Christmas Island (CHR, 2° N), Samoa (SAM, 14° S), and the South Pole (SPO, 90° S)).
Table 2Temporal trends in the seasonal cycle amplitude of Ca and δ13Ca from the standard simulation (Estandard) and observations for 19 monitoring sites from 1982 to 2012. Observational data of Ca are from the GLOBALVIEW-CO2 product and are fitted for the period of 1982–2012, while the data for δ13Ca are from Scripps and are fitted as shown in Fig. 3. The seasonal cycle amplitude of a given year is only computed if at least 10 monthly values are available. The number of years included in the trend calculation for SA(Ca) and model-based SA(δ13Ca) is given in parentheses. The observed trend for Ca is affected by anomalous values at Key Biscayne, which is not included. Over the period of 1982–2012, significant trends (two-sided t test at 5 % significance) are only found for Alert, Nuvuk (formerly Point Barrow), Ocean Station M, and Mahe Island for observed Ca; for Mariana Islands, Mahe Island, Palmer, Halley, and the South Pole for simulated Ca; and for Ascension, Mahe Island, and the South Pole for simulated δ13Ca. The decadal-scale trends are given per century for better readability.
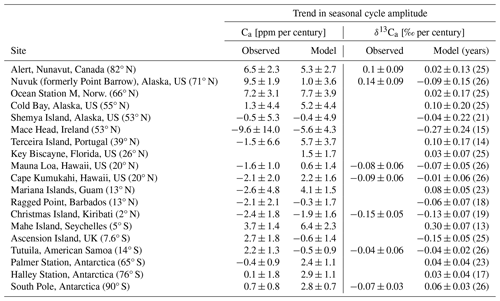
Temporal trends in SA(δ13Ca) from the Scripps gap-filled data are not statistically different from zero, except at the tropical site Christmas Island and at the South Pole (Fig. 3). Averaging the trends across all eight sites yields −0.0038 ± 0.026 ‰ per century (mean ± 1 SD of the mean), and averaging the trends for the extratropical sites ALT, PTB, and LJO yields +0.09 ± 0.06 ‰ per century, with both averaged trends not statistically different from zero. The trend for the NH extratropical sites translates into a change in SA(δ13Ca) of around 5 ± 3 % over the 40-year observational period. For the fitted data, trends are statistically different from zero only at two sites (La Jolla and Christmas Island). This is consistent with Gonsamo et al. (2017), who did not detect a temporal trend in SA(δ13Ca) and seasonal phasing by fitting Scripps daily flask data from the four sites of Alert, Nuvuk (formerly Point Barrow), La Jolla, and Mauna Loa. In summary, observed temporal trends in SA(δ13Ca) are small (≤ 0.15 ‰per century) and are not statistically different from zero (at p<0.05) at individual sites. A significant negative trend is found for the tropical site Christmas Island, and detection of trends is difficult at the Southern Hemisphere sites, where SA(δ13Ca) is small.
Simulated trends in SA(δ13Ca) are small (often less than 0.01 ‰ per decade) and statistically insignificant (5 % level) except at three SH sites (Ascension, Mahe, the South Pole) with a small seasonal cycle amplitude (Table 2). Observed relative trends in SA(Ca) are larger than in SA(δ13Ca) in the northern high latitudes and are statistically significant at Alert, Nuvuk (formerly Point Barrow), Ocean Station, and Mahe Island but insignificant at all other sites over the 1982–2012 analysis period. Simulated trends in SA(Ca) are insignificant, except at four SH sites and on the Mariana Islands.
We compare model (m) and observed (o) slopes (β) to probe model–observation agreement. Under the null hypothesis of no slope difference, the statistic (where sβ is the standard error in the β slope estimate) is Student's t distributed (Welch, 1947). Trends are different when the T values are larger than the 0.975 quantile of a t distribution with ν degrees of freedom (). Modeled and observed trends are different at one site, the South Pole, for SA(δ13Ca) and at one site (Nuvuk, formerly Point Barrow) for SA(Ca). As will become clear in the next section, the largest surface–atmosphere isotope fluxes and temporal changes in these fluxes are simulated in the region north of 40° N. We are therefore interested in quantifying how well the model represents temporal changes in SA(Ca) in this region and over a 40-year period, representative of the δ13Ca observational record. For the five NH high-latitude sites with more than 20 years of data, uncertainties in the temporal changes in SA(Ca) range between 5 % and 13 % at individual sites over a 40-year period. The average trend in SA(Ca) for these five NH sites (Alert, Nuvuk (formerly Point Barrow), Ocean Station M, Cold Bay, Shemya Island) is 4.8 ± 1.6 ppm per century (31 ± 10 % per century) from observations and 3.8 ± 1.8 ppm per century (22 ± 11 % per century) from the model. These estimates translate into a relative change in SA(Ca) of around 4 % to 5 % over a 40-year period. This suggests that our model chain accurately represents the observed temporal changes in SA(Ca) in the NH extratropical atmosphere.
Given the mostly insignificant trends at individual sites over the model analysis period of 1982–2012, the question of whether larger trends are detected when considering longer timescales arises. Century-scale trends, or their absence, can be readily estimated in the simulations by comparing SA(Ca) and SA(δ13Ca) for the modern period (1982–2012) (Estandard) and the preindustrial control (Econtrol) (Table 1; solid red versus dashed blue lines in Figs. S3 and S4). For Ca, a growth in SA is clearly visible (12.2 to 17.25 ppm at Alert, 6 to 8.3 ppm at Mauna Loa, 1.7 to 2.1 ppm at the South Pole). Across all 19 sites, SA(Ca) has grown by 44 % ± 35 % (mean ± standard deviation) from 1700 CE to 1982–2012. The growth in SA(Ca) ranges between 33 % and 42 % across the 12 extratropical NH sites (Table 1).
For δ13Ca, Econtrol and Estandard exhibit an almost identical SA averaged across all 19 sites (2 % ± 16 % lower in Econtrol than in Estandard). The change in SA(δ13Ca) from the preindustrial (Econtrol) to modern (Estandard) periods ranges between −6 % and 9 % across the 12 extratropical NH sites, whereas more diverse results (−28 % to +63 %) are simulated at the tropical and SH sites (Fig. S4, Table 1). The change in SA(δ13Ca), , does not emerge from the noise of variability (N = 2 standard deviations from IAV of Estandard), except at one tropical (Christmas Island) and three SH sites (Ascension Island, Mahe Island, Palmer Station); we require for the signal S to emerge (Keller et al., 2014). The fact that trends in SA(Ca) and the near-zero trends in SA(δ13Ca) are better identified by the difference between the modern and preindustrial periods than by regression over the modern period motivates us to focus on the comparison between Estandard versus Econtrol in the remaining result sections.
4.3 Zonal decomposition of seasonal land–biosphere fluxes
4.3.1 Changes in the seasonal amplitude of land–biosphere fluxes and δ13Ca over the historical period
Next, we address the near-absent temporal trends in SA(δ13Ca) at NH sites by analyzing the zonally averaged cumulative growing season flux of , i.e., SA() (Fig. 4). The northern midlatitude to high-latitude ecosystem fluxes exhibit the largest seasonal cycle, followed by tropical rain-green forests and savannahs in Estandard. This flux pattern contributes to the larger SA(δ13Ca) in the NH extratropics versus tropical and SH sites. A similar latitudinal flux pattern holds for Econtrol.
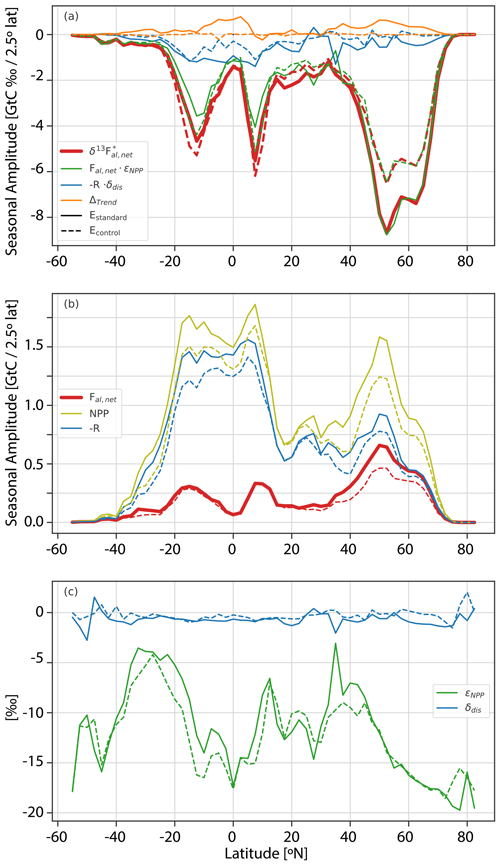
Figure 4The seasonal amplitude per 2.5° latitude band of the signature-weighted, detrended net atmosphere–land flux, , in the period of 1982–2012 is shown in (a) in red (see Eq. 8). This quantity is the sum of three constituent seasonal amplitudes (Eq. 9 and Appendix A): net land–atmosphere flux weighted with photosynthetic fractionation (, green) plus release fluxes weighted with the disequilibrium signature (, blue) plus the contribution to the seasonal amplitude by the underlying trend of (Δtrend, orange) (sign convention – green + blue + orange = red). In (b), the seasonal amplitudes of (non-detrended) net carbon fluxes are shown. The net atmosphere–land flux (fal,net, red) is split into net primary productivity (NPP, olive) and release flux (R, blue). In (c) the corresponding fractionation of photosynthesis εNPP and the disequilibrium signature δdis,la are shown. All values are for the period with smaller than zero (∼ growing season). The results from the standard simulation (Estandard, solid lines) are compared to the preindustrial control simulation (Econtrol, dashed lines).
Turning to the change over the historical period, SA() is 28 % larger for the region north of 15° N (30 % larger for > 40° N and 20 % larger for 15–40° N) for Estandard than for Econtrol. This growth is comparable to the observed increase in Ca of 32 % from the preindustrial period to the reference period of 1982–2012. In contrast, Econtrol sometimes exhibits larger SA() than Estandard in the tropical and SH ecosystems (Fig. 4). Following Eq. (8), the near-proportional growth in the SA() and in annual mean Ca in the NH extratropics is consistent with the absence of any major long-term change in SA(δ13Ca) at extratropical NH sites (Table 1 and Fig. S4). SA(δ13Ca) and its change at extratropical NH sites is dominated by the large SA() in the northern extratropics (Fig. 4), and transport from low-latitude regions is less important. On the other hand, the large extratropical SA() influences SA(δ13Ca) and its temporal changes at lower latitudes. Without this influence, we would, based on Eq. (8), expect a decrease in SA(δ13Ca) outside the extratropics, given that the relative increase in annual mean Ca is larger than the increase in SA() in these regions.
Factorial simulations with an individual forcing kept at preindustrial levels show small individual contributions by climate change, fossil fuel emissions, and land use to the industrial period growth in SA(δ13Ca) at northern extratropical sites (Figs. S3 and S4). This suggests that the statistically insignificant trend in SA(δ13Ca) at northern extratropical sites is not caused by offsetting impacts of climate change versus increasing Ca. Fossil fuel emissions cause an increase and land use change a reduction in SA(δ13Ca) at low-latitude and southern sites (Figs. S3 and S4). We attribute the dampening influence of land use change to the replacement of C4 plants by C3 crops, causing a general shift in the fractionation during photosynthesis to less negative values south of ∼ 45° N (Fig. 4c). This damping influence highlights the importance of considering spatiotemporal variations in C3 and C4 plant distributions when analyzing δ13Ca. In summary, the results suggest that the near-proportional growth in SA() and in Ca is mainly responsible for the statistically insignificant trend in SA(δ13Ca) at high-northern-latitude sites and contributes to the statistically insignificant trend in SA(δ13Ca) at other NH sites via atmospheric transport.
For CO2, the amplitude of the modeled zonally averaged net atmosphere-to-land CO2 flux, SA(fal,net), shows the largest values in the NH extratropics and a large increase over the historical period of 33 % in the region 15–90° N (15–40° N – 26 %; 40–90° N – 37 %) driven by a larger increase in NPP than release fluxes (R), whereas SA(fal,net) is smaller in the tropics and SH and shows hardly any changes from the preindustrial (Econtrol) to modern (Estandard) periods south of 20° N. These results are consistent with previous studies showing northern ecosystems progressively taking up more carbon during the growing season (Graven et al., 2013; Forkel et al., 2016; Piao et al., 2018; Bastos et al., 2019). For example, using carbon fluxes from two atmospheric inversions and 11 land models, Bastos et al. (2019) find a positive trend in SA(fal,net) north of 40° N and small or no growth in SA(fal,net) between 25 and 40° N.
4.3.2 The coupling between the seasonal amplitude of Ca and δ13Ca
SA(Ca) and SA(δ13Ca) are partly coupled by the underlying carbon fluxes. The question to which extent SA(δ13Ca) holds information independent from SA(Ca) arises. We decompose in a contribution linked to the net atmosphere-to-land carbon flux, , and an isotopic disequilibrium flux (see Appendix A and Sect. 3 for notation; fal,net is positive for a flux into the land biosphere):
NPP is the net primary productivity of all plants within a grid cell. R is the sum of all land biosphere release fluxes to the atmosphere, such as those from heterotrophic respiration, fire, mortality, and product pools, except autotrophic respiration. δ13CR is the signature of R, and δ13CNPP is the signature of NPP, with εNPP (or εal) representing the (flux-weighted) fractionation by NPP. The difference in signatures of R and NPP is the isotopic disequilibrium, δdis,la. Here, as in LPX-Bern, we have assumed that the uptake difference between gross primary production (GPP) and NPP is released on short timescales and without further carbon isotope fractionation.
Equation (9), together with Eqs. (7) and (8), provides insights into the driving factors for the seasonal amplitudes. Putting the ocean aside (Heimann et al., 1989), SA(Ca) is driven by the spatiotemporal pattern of NPP−R , whereas SA(δ13Ca) is additionally influenced by seasonal variations in εNPP, and the disequilibrium flux (). The latter is indicative of the transit time of carbon through the land biosphere.
The decomposition of zonally averaged SA() into the amplitude of constituent fluxes and their isotopic signatures is displayed in Fig. 4 and Table S1 in the Supplement. On the global average, SA() contributes to SA() with a fraction of 90 % for both Estandard and Econtrol. For the region north of 40° N, SA() contributes only 7 % to SA() in Estandard and is almost negligible in Econtrol (2 %). In Econtrol, SA() and δdis,la, albeit to a smaller extent than in Estandard, are not negligible due to the lagged response of the respiration signatures to natural changes in εNPP. A small contribution (Δtrend) to the isotopic flux seasonality in Estandard arises from the long-term increase in flux (Fig. 4; see Appendix A). The small contribution of the disequilibrium flux () relative to the net flux (; Eq. 9) arises as the seasonal amplitude of the carbon release flux R is similar in magnitude to that of the net land carbon uptake fal,net in the northern extratropics (blue versus red lines in Fig. 4b), while the disequilibrium δdis,la is an order of magnitude smaller than εNPP (Fig. 4c). Thus in LPX, SA(δ13Ca) is dominated by the growing season net carbon uptake flux in northern high latitudes, suggesting that SA(δ13Ca) holds little information on the isotopic disequilibrium at high-latitude sites. Rather, the additional information of SA(δ13Ca) compared to SA(Ca) is on the magnitude of εNPP at northern high-latitude sites. In contrast, the contribution by the disequilibrium flux SA() and the contribution by the net carbon flux SA() are nearly equal in the tropics (10° S–10° N) and the SH (Fig. 4, Table S1), suggesting that SA(δ13Ca) potentially holds additional information on R and carbon turnover in these regions in comparison to SA(Ca).
The zonal variation in (growing season) photosynthetic fractionation εNPP is mainly due to differences in vegetation composition, with C4 plants having considerably lower photosynthetic fractionation than C3 plants (Fig. 4c). Land use and the evolving distribution of C3 and C4 crops are prescribed in the model, and C4 grasses are more prevalent than C3 grasses in low-latitude dryland ecosystems. Accordingly, maxima in flux-weighted, zonal-mean εNPP are simulated at 35° N, 12° N, and broadly around 30° S. Minima are simulated for the C3-dominated high-latitude ecosystems and tropical rainforest zone. In Estandard, εNPP is generally less negative than in Econtrol and increased by 1.18 ‰ (9 % in relative units) on the global average (SA(NPP)-weighted), mainly due to the increase in the prevalence of C4 plants, while εNPP remains time-invariant in the C3-dominated ecosystems north of 45° N (Fig. 4c). To estimate the influence of the increase in C4 prevalence on global mean εNPP (but not on global GPP), we run a factorial simulation, , with the fractionation formulation for all C4 plants replaced by that for C3 plants. The difference between Estandard and , i.e., the change in fractionation attributable to C4 plants, amounts to about 1.5 ‰ on a global average (1982–2012 versus 1720–1750) (Fig. S5), pointing again to the importance of C3 and C4 plant distribution changes for δ13Ca.
Keeling et al. (2017) analyzed the atmospheric budgets of carbon and 13C using seasonally detrended data, using a three-box land model with time-invariant overturning timescales, using globally uniform isotopic fractionation, and neglecting changes in C3 and C4 distribution in their standard setup. They found that global mean εNPP decreased by 0.66 ± 0.34 ‰ from 1975 to 2005 and attributed this change to changes in fractionation associated with mesophyll conductance and photorespiration of C3 plants. It appears challenging to detect and attribute changes in the fractionation of global mean NPP with a box model, given uncertainties in NPP (Graven et al., 2024) and changes in C3 versus C4 plant distribution.
While the influence of the gross exchange flux and the isotopic disequilibrium on δ13Ca seasonality is modeled to be small at northern sites of today, it remains to be explored how global warming will change these parameters, e.g., due to changes in fire frequency and tree mortality, and affect δ13Ca and the information provided by continued δ13Ca observations. We may also expect different disequilibrium fluxes and, in turn, δ13Ca seasonality if the global carbon sink is driven by a stimulation of NPP, e.g., by CO2 fertilization (Walker et al., 2021) as in LPX-Bern, versus a change in tree longevity (Bugmann and Christof, 2011; Körner, 2017). It remains to be investigated, e.g., by applying perturbed-parameter ensembles and sensitivity simulations, whether such differences indeed significantly affect δ13Ca seasonality.
Monitoring Ca and δ13Ca over tropical and SH land regions could potentially provide valid information to disentangle NPP, respiration, and net carbon fluxes, given the substantial contribution of the disequilibrium flux to SA(). However, the seasonality of δ13Ca and Ca at the tropical background monitoring sites analyzed in this study is strongly influenced by long-range transport, adding uncertainty to the interpretation of seasonal signals at background sites. Ideally, seasonally resolved observations are taken in air masses influenced primarily by regional land biosphere fluxes – thereby minimizing uncertainties from long-range transport – and interpreted with the help of atmospheric transport and land biosphere models (Botía et al., 2022). For example, the data may be assimilated into atmospheric transport models applied in inverse mode to infer surface carbon and isotope fluxes or assimilated into isotope-enabled land biosphere models combined with atmospheric transport to optimize parameters governing modeled carbon and isotope fluxes (Peylin et al., 2016; van der Velde et al., 2018; Castro-Morales et al., 2019).
4.4 Implications for stomatal conductance and water use
Our result of a time-invariant εNPP in northern extratropical regions holds implications for carbon and water fluxes and evaporative cooling. The good agreement between observations and model results for SA(δ13Ca) and its temporal trend at northern sites provides implicit support for regulation of stomatal conductance by C3 plants towards a constant ratio of the CO2 mole fraction in the leaf intercellular space (ci) and ambient atmospheric air (ca) on the continental scale. Following Farquhar (1989) and Cernusak et al. (2013), εNPP is approximately proportional to :
with a (4.4) and b (27) being constants. Two contrasting scenarios are published for the regulation of leaf stomatal conductance for C3 plants. First, many site studies (Voelker et al., 2016; Saurer et al., 2014; Kauwe et al., 2013; Peñuelas et al., 2011; Frank et al., 2015; Keller et al., 2017) suggest a regulation of stomatal conductance towards a constant and, hence, suggest that ci grows proportionally to ca. An absent temporal trend in translates into an absent trend in εNPP and vice versa (Eq. 10). Focusing on regions north of > 40° N, where carbon fluxes are largest and C3 plants dominate, LPX-Bern simulates a small role of isotopic disequilibrium fluxes and a dominant influence of net atmosphere–surface fluxes on SA(δ13Ca) (Fig. 4, green versus blue lines). Importantly, LPX-Bern simulates small temporal changes in the (flux-weighted) fractionation of the zonally and seasonally integrated NPP at northern sites (Fig. 4c, green lines) and a stomatal regulation towards constant . In turn, the good model–data agreement in the temporal trends of SA(Ca) and SA(δ13Ca) implies consistency with the observational evidence for this scenario towards constant .
In contrast, Battipaglia et al. (2013) and Keenan et al. (2013) suggest a regulation of stomatal conductance towards a constant ci and a decreasing ratio of under rising Ca. Evaluating Eq. (10) for 1980–2022, the period with δ13Ca measurements, yields a decrease in εNPP of 15 % (−3.0 ‰ to −3.8 ‰) for an initial ratio in the range of 0.7 to 0.9 and constant ci. We argue that the good observation–model agreement in the simulated trends in SA(Ca) implies that the influence of the simulated net atmosphere–land carbon flux is realistic, and SA(δ13Ca) would decrease if εNPP decreases. A decrease in SA(δ13Ca) of 15 % would emerge from the noise of variability at individual northern sites. Taken together, we suggest that the scenario towards constant is consistent with the observations, whereas the scenario towards constant ci appears less likely. However, uncertainties remain, and our conclusions for the two scenarios of stomatal regulation await confirmation by other modeling studies.
The two scenarios imply large differences in water fluxes (Knauer et al., 2017). The intrinsic water use efficiency (iWUE), the ratio between assimilation of CO2 by photosynthesis (A) and conductance of CO2 (g), is, as εNPP, a function of ci and ca:
iWUE would have increased from 1980 to 2022 by 23 % for constant but by 77 % to 231 % for constant ci, assuming an initial of 0.7 to 0.9. In the latter scenario, stomatal conductance and correspondingly water loss per stomatal pore would have decreased strongly over the last decades.
Equation (10) is an approximation (Farquhar et al., 1982; Lloyd and Farquhar, 1994; Farquhar and Cernusak, 2012; Cernusak et al., 2013) considered to be sufficient for many applications by Cernusak et al. (2013) and applied in the publications cited in the previous two paragraphs. However, there are four contributions only implicitly considered by choosing parameter b in Eq. (10), and these may contribute small temporal trends to εNPP. In turn, inferred would also have a temporal trend for a constant εNPP. We estimate the trend contribution of these additional terms to be of small magnitude (<1 ‰) in comparison to the 3 ‰ to 3.8 ‰ difference estimated for our two scenarios (see Appendix B for details).
We explored the global-scale mechanisms driving the observed seasonal cycles of δ13C of atmospheric CO2 (δ13Ca) and of atmospheric CO2 at 19 monitoring sites using atmosphere–surface fluxes from the Bern3D-LPX Earth system model of intermediate complexity and fossil fuel emissions in combination with transport matrices from the TM3 atmospheric transport model. We find good data–model agreement at northern and tropical sites. No significant trends are detected or modeled in the seasonal cycle amplitude of δ13Ca at most monitoring sites, in contrast to the positive trends in the seasonal amplitude of CO2. We attribute the statistically insignificant trend in the seasonal amplitude of δ13Ca to a near-equal percentage increase in the growing season net carbon uptake and isotope flux and in the background atmospheric CO2 in the northern extratropical land regions. Over the industrial period and at low-latitude and SH sites, land use change has a dampening influence on δ13Ca seasonality through the replacement of C3 plants by C4 crops. Modeled isotopic disequilibrium fluxes have a small influence on the seasonal signal of δ13Ca at NH sites but play an important role in tropical and SH ecosystems, suggesting that monitoring the δ13Ca seasonality over tropical and SH land would provide valuable information on gross carbon exchange fluxes and the timescales of carbon turnover in the land biosphere. Our results, based on a single model chain, provide implicit support for a regulation of the stomatal conductance of C3 plants towards a constant ratio on biome scales and for intrinsic water use efficiency to grow proportionally to atmospheric CO2 over recent decades, with implications for carbon and water fluxes. More generally, the results suggest that observations of the δ13Ca seasonal cycle offer highly useful information on carbon and water cycle processes. We recommend seasonally resolved δ13Ca observations as a constraint for land biosphere models used to simulate the terrestrial sink of anthropogenic carbon and land use emissions, for example, using perturbed parameter ensembles in Bayesian approaches (Lienert and Joos, 2018; van der Velde et al., 2018). Future studies may employ an ensemble of isotope-enabled models and perturbed parameter ensembles to elucidate whether our findings are robust and to show which models or process assumptions are compatible or incompatible with δ13Ca data for improved projections of atmospheric CO2 and global warming.
We reformulate the net isotope flux in terms of net and gross carbon fluxes, isotopic fractionation, and isotopic disequilibrium (e.g., Mook, 1986; Joos and Bruno, 1998) to diagnose their influence on the seasonal cycles.
The fractionation for a gross flux, e.g., from the atmosphere to the surface, is
with δ13Cas the signature of the gross flux from a to s (fas) and δ13Ca the signature of the source. The isotopic disequilibrium (or difference) between atmosphere–surface gross fluxes is
The net carbon and isotope fluxes are the differences between the gross fluxes:
Rearranging yields
For the land biosphere (l), it follows from Eqs. (A3) and (A5) that
with
NPP is the net primary productivity of all plants within a grid cell. R is the sum of all release fluxes to the atmosphere, such as those from heterotrophic respiration, fire, mortality, and product pools, except autotrophic respiration. δ13CR is the signature of R, and δ13CNPP is the signature of NPP, with εNPP (or εal) representing the (flux-weighted) fractionation by NPP.
The seasonal amplitudes of and its components are calculated as follows. The time series of is detrended and normalized to zero. The trend is computed by a rolling 12-month mean of . Then, the resulting trend curve is subtracted from (disregarding the first and last 6 months of the original series) to get a detrended curve. Finally, the detrended curve is normalized by subtracting its period mean. Δtrend (e.g., in units of mol ‰ yr−1 m−2) is the difference between after and before this detrending and normalizing procedure. We define a seasonal mask to compute seasonal amplitudes of fluxes and their signatures. For each model year, we identify months in which detrended is negative or equal to zero (roughly corresponding to the growing season). The sum of fluxes of these months is then termed the seasonal amplitude in a given year. For , this procedure is consistent with considering the difference between maximum and minimum values of the detrended cumulative sum of . Accordingly, the seasonal amplitudes of the component fluxes contributing to are computed by summation over months where is less than or equal to zero within a given year. Component fluxes are [], [], [Δtrend] and, further, [NPP], [R], and [NPP−R] (Fig. 4). These component fluxes are not detrended to readily calculate the signatures δdis,la and εNPP by division of the seasonal amplitude isotopic flux with the corresponding seasonal amplitude carbon flux.
We note that the annual climatological mean values of the isotopic disequilibrium (δdis,la), the net carbon flux (fal,net), and the net isotopic flux () vanish by definition for the preindustrial equilibrium. However, this does not hold for their seasonal amplitudes. Further, detrending before the computation of its seasonal amplitude is consistent with the calculation of the Ca and δ13Ca seasonal amplitude from the detrended atmospheric time series.
The seasonal cycles of Ca or δ13Ca are computed from observations and the TM3 results using the following procedure for either Ca or δ13Ca. Months with missing values in either the observations or the TM3 simulation are masked in the TM3 and observational time series. Then the time series are detrended using a 12-month rolling mean, and the overall mean of the series is set to zero to get (for year, y, and month, m) seasonal anomalies ΔCa(y,m) and Δδ13Ca(y,m). Finally, the period means for each calendar month, and , are computed by averaging over all corresponding monthly values. Additionally, the standard deviation is computed for each calendar month to inform about the interannual variability in the seasonality. The period-mean SA is computed as the difference between the month with the highest () and lowest () value in and , respectively. For individual years, we computed SA by the difference from the extreme monthly values of each year.
In Sect. 4.4, we applied a simplified expression for fractionation of C3 plants during photosynthesis (εNPP) and used this expression to translate trends in εNPP to trends in and in iWUE. The potential contributions to trends in εNPP from neglected ternary effects, dark-day respiration, and transport through the mesophyll and photorespiration are discussed in this appendix.
The isotopic fraction for C3 photosynthesis is framed as a multi-step process considering the transport of CO2 and the underlying gradients in CO2 mole fractions from the ambient air (mole fraction – ca) to the leaf surface (cs) in the intercellular air spaces (ci) and the sites of carboxylation (cc), as well as the fractionation during carboxylation, dark-day respiration, Rd, and photorespiration (Cernusak, 2013). The transport of CO2 equals the consumption of CO2 by assimilation, A: , with g being the conductance of the stomatal pores and the boundary layer and gm the mesophyll conductance. The relationship can be rewritten as . If A increases in proportion to ca and g and gm is assumed to be constant, then it follows that and are also constant. In turn, the fractionation associated with the boundary layer and stomatal conductance (); a=4.4 ‰), mesophyll conductance (); am=1.8 ‰), and carboxylation () remain constant. The overall influence of mesophyll transport on εNPP can also be written as (Keeling et al., 2017).
Keeling et al. (2017) assumed that decreases over time, with A increasing by 45 % for a doubling of CO2, and that therefore fractionation by the mesophyll contribution would change by −0.006 ‰ ppm−1, i.e., a change in εNPP of 0.47 ‰ for the CO2 increase of 78 ppm from 1980 to 2022. On the other hand, Campbell et al. (2017) observationally constrained the growth in gross primary production over the 20th century to be 31 ± 5 %, larger than the increase in ca of 25 %. Accordingly, increases, and the mesophyll trend contribution is positive. With the central parameter values of Keeling et al. (2017) (A=9 µmol m−2 s−1, gm=0.2 mol m−2 s−1, CO2 = 355 ppm), the contribution is +0.002 ‰ ppm−1. Keeling et al. (2017) also estimated changes in fractionation associated with photorespiration (; f=12 ‰) to be −0.004 ‰ ppm−1 assuming a constant CO2 compensation point, Γ*. The real sensitivity must be smaller, as Γ* increases with temperature and because Keeling et al. (2017) applied an estimate for the CO2 compensation point in the presence of Rd (43 ppm) instead of the absence of Rd ( ppm). Further, fractionation during day respiration is (Cernusak et al., 2013), roughly about 0 to −0.3 ‰ for e in the range of 0 ‰ to 5 ‰; we apply RuBisCO carboxylation rates, Vc, of 11 µmol m−2 s−1 derived from the value of A=9 µmol m−2 s−1 by Keeling et al. (2017), (Rd=1 µmol m−2 s−1 and ). Finally, ternary effects of about −0.7 ‰ (0.024 × b) increase with water vapor deficit (Farquhar and Cernusak, 2012). Given the small amplitudes of these two contributions, their temporal trends have also been small over recent decades.
The data from the Scripps CO2 program are available here: https://scrippsco2.ucsd.edu/data/atmospheric_co2/ (last access: 17 April 2023, Keeling et al., 2001). The GLOBALVIEW data from the NOAA Global Monitoring Laboratory were downloaded here: https://gml.noaa.gov/ccgg/globalview/ (last access: 27 April 2022, Cooperative Global Atmospheric Data Integration Project, 2013). The model data displayed in the figures and plotting scripts are available here: https://zenodo.org/records/14051464 (last access: 7 November 2024, https://doi.org/10.5281/zenodo.14051464, Joos et al., 2024).
The supplement related to this article is available online at: https://doi.org/10.5194/bg-22-19-2025-supplement.
FJ and SL wrote the paper with inputs from SZ. SL performed all model runs, and FJ did the statistical analyses. SL and FJ produced the figures and tables.
The contact author has declared that none of the authors has any competing interests.
The work reflects only the authors' view; the European Commission and their executive agency are not responsible for any use that may be made of the information the work contains.
Publisher's note: Copernicus Publications remains neutral with regard to jurisdictional claims made in the text, published maps, institutional affiliations, or any other geographical representation in this paper. While Copernicus Publications makes every effort to include appropriate place names, the final responsibility lies with the authors.
We thank the researchers of the Cooperative Atmospheric Data Integration Project, NOAA Earth System Research Laboratories (ESRL), Boulder, Colorado, and of the Scripps CO2 program for making their CO2 and δ13C data freely available. We also thank Martin Heimann for suggesting that we plot seasonal anomalies of CO2 versus those of δ13C. A special thanks goes to Christoph Köstler for providing the TM3 transport matrices and to Aurich Jeltsch-Thömmes for help with Bern3D. We thank Gerbrand Koren and an anonymous reviewer for their careful reviews and Ji-Hyung Park for editing the paper.
This project has received funding from the European Union's Horizon 2020 research and innovation program under grant agreement no. 821003 (project 4C, Climate–Carbon Interactions in the Current Century) and from the Swiss National Science Foundation (project no. 200020_200511).
This paper was edited by Ji-Hyung Park and reviewed by Gerbrand Koren and one anonymous referee.
Andres, R., Marland, G., Boden, T., and Bischof, S.: Carbon dioxide emissions from fossil fuel consumption and cement manufacture, 1751–1991, and an estimate of their isotopic composition and latitudinal distribution, in: The Carbon Cycle, edited by: Wigley, T. M. L. and Schimmel, D., Cambridge University Press, New York, USA, 53–62, ISBN 0 521 58337 3, 2000. a
Andres, R., Boden, T., and Marland, G.: Monthly Fossil-Fuel CO2 Emissions: Mass of Emissions Gridded by One Degree Latitude by One Degree Longitude – 2016, ESS-DIVE [data set], https://doi.org/10.3334/CDIAC/FFE.MONTHLYMASS.2016, 2009a. a
Andres, R., Boden, T., and Marland, G.: Monthly Fossil-Fuel CO2 Emissions: Isomass of Emissions Gridded by One Degree Latitude by One Degree Longitude, ESS-DIVE [data set], https://data.ess-dive.lbl.gov/view/doi:10.3334/CDIAC/FFE.MONTHLYISOMASS.2016 (last access: 23 December 2024), 2009b. a
Andres, R., Boden, T., and Marland, G.: Annual Fossil-Fuel CO2 Emissions: Global Stable Carbon Isotopic Signature, ESS-DIVE [data set], https://doi.org/10.3334/CDIAC/FFE.DB1013.2017, 2017. a
Bacastow, R. B., Keeling, C. D., and Whorf, T. P.: Seasonal amplitude increase in atmospheric CO2 concentration at Mauna Loa, Hawaii, 1959–1982, J. Geophys. Res.-Atmos., 90, 10529–10540, https://doi.org/10.1029/JD090iD06p10529, 1985. a
Ballantyne, A. P., Miller, J. B., and Tans, P. P.: Apparent seasonal cycle in isotopic discrimination of carbon in the atmosphere and biosphere due to vapor pressure deficit, Global Biogeochem. Cy., 24, 1–16, https://doi.org/10.1029/2009GB003623, 2010. a
Ballantyne, A. P., Miller, J. B., Baker, I. T., Tans, P. P., and White, J. W. C.: Novel applications of carbon isotopes in atmospheric CO2: what can atmospheric measurements teach us about processes in the biosphere?, Biogeosciences, 8, 3093–3106, https://doi.org/10.5194/bg-8-3093-2011, 2011. a
Barlow, J. M., Palmer, P. I., Bruhwiler, L. M., and Tans, P.: Analysis of CO2 mole fraction data: first evidence of large-scale changes in CO2 uptake at high northern latitudes, Atmos. Chem. Phys., 15, 13739–13758, https://doi.org/10.5194/acp-15-13739-2015, 2015. a
Bastos, A., Ciais, P., Chevallier, F., Rödenbeck, C., Ballantyne, A. P., Maignan, F., Yin, Y., Fernández-Martínez, M., Friedlingstein, P., Peñuelas, J., Piao, S. L., Sitch, S., Smith, W. K., Wang, X., Zhu, Z., Haverd, V., Kato, E., Jain, A. K., Lienert, S., Lombardozzi, D., Nabel, J. E. M. S., Peylin, P., Poulter, B., and Zhu, D.: Contrasting effects of CO2 fertilization, land-use change and warming on seasonal amplitude of Northern Hemisphere CO2 exchange, Atmos. Chem. Phys., 19, 12361–12375, https://doi.org/10.5194/acp-19-12361-2019, 2019. a, b, c
Battaglia, G. and Joos, F.: Marine N2O Emissions From Nitrification and Denitrification Constrained by Modern Observations and Projected in Multimillennial Global Warming Simulations, Global Biogeochem. Cy., 32, 92–121, https://doi.org/10.1002/2017GB005671, 2018. a
Battipaglia, G., Saurer, M., Cherubini, P., Calfapietra, C., McCarthy, H. R., Norby, R. J., and Francesca Cotrufo, M.: Elevated CO2 increases tree-level intrinsic water use efficiency: insights from carbon and oxygen isotope analyses in tree rings across three forest FACE sites, New Phytol., 197, 544–554, https://doi.org/10.1111/nph.12044, 2013. a, b
Becker, M., Steinhoff, T., and Körtzinger, A.: A Detailed View on the Seasonality of Stable Carbon Isotopes Across the North Atlantic, Global Biogeochem. Cy., 32, 1406–1419, https://doi.org/10.1029/2018GB005905, 2018. a
Botía, S., Komiya, S., Marshall, J., Koch, T., Gałkowski, M., Lavric, J., Gomes-Alves, E., Walter, D., Fisch, G., Pinho, D. M., Nelson, B. W., Martins, G., Luijkx, I. T., Koren, G., Florentie, L., Carioca de Araújo, A., Sá, M., Andreae, M. O., Heimann, M., Peters, W., and Gerbig, C.: The CO2 record at the Amazon Tall Tower Observatory: A new opportunity to study processes on seasonal and inter-annual scales, Glob. Change Biol., 28, 588–611, https://doi.org/10.1111/gcb.15905, 2022. a
Bugmann, H. and Christof, B.: Will the CO2 fertilization effect in forests be offset by reduced tree longevity?, Oecologia, 165, 533–544, https://doi.org/10.1007/s00442-010-1837-4, 2011. a
Campbell, J. E., Berry, J. A., Seibt, U., Smith, S. J., Montzka, S. A., Launois, T., Belviso, S., Bopp, L., and Laine, M.: Large historical growth in global terrestrial gross primary production, Nature, 544, 84–87, 2017. a
Castro-Morales, K., Schürmann, G., Köstler, C., Rödenbeck, C., Heimann, M., and Zaehle, S.: Three decades of simulated global terrestrial carbon fluxes from a data assimilation system confronted with different periods of observations, Biogeosciences, 16, 3009–3032, https://doi.org/10.5194/bg-16-3009-2019, 2019. a
Cernusak, L. A. and Ubierna, N.: Carbon Isotope Effects in Relation to CO2 Assimilation by Tree Canopies, 291–310, Springer International Publishing, Cham, ISBN 978-3-030-92698-4, https://doi.org/10.1007/978-3-030-92698-4_9, 2022. a
Cernusak, L. A., Ubierna, N., Winter, K., Holtum, J. A. M., Marshall, J. D., and Farquhar, G. D.: Environmental and physiological determinants of carbon isotope discrimination in terrestrial plants, New Phytol., 200, 950–965, https://doi.org/10.1111/nph.12423, 2013. a, b, c, d
Cooperative Global Atmospheric Data Integration Project: Multi-laboratory compilation of synchronized and gap-filled atmospheric carbon dioxide records for the period 1979–2012 (obspack_co2_1_GLOBALVIEW-CO2_2013_v1.0.4_2013-12-23), NOAA Global Monitoring Laboratory [data set], https://doi.org/10.3334/OBSPACK/1002, 2013. a, b
Craig, H.: Isotopic standards for carbon and oxygen and correction factors for mass-spectrometric analysis of carbon dioxide, Geochim. Cosmochim. Ac., 12, 133–149, https://doi.org/10.1016/0016-7037(57)90024-8, 1957. a
Dargaville, R. J., Heimann, M., McGuire, A. D., Prentice, I. C., Kicklighter, D. W., Joos, F., Clein, J. S., Esser, G., Foley, J., Kaplan, J., Meier, R. A., Melillo, J. M., Moore, B., Ramankutty, N., Reichenau, T., Schloss, A., Sitch, S., Tian, H., Williams, L. J., and Wittenberg, U.: Evaluation of terrestrial carbon cycle models with atmospheric CO2 measurements: Results from transient simulations considering increasing CO2, climate, and land-use effects, Global Biogeochem. Cy., 16, 39-1–39-15, https://doi.org/10.1029/2001gb001426, 2002. a
Farquhar, G.: Carbon Isotope Discrimination And Photosynthesis, Annu. Rev. Plant Phys., 40, 503–537, https://doi.org/10.1146/annurev.arplant.40.1.503, 1989. a, b
Farquhar, G. and Cernusak, L.: Ternary effects on the gas exchange of isotopologues of carbon dioxide, Plant Cell Environ., 35, 1221–1231, https://doi.org/10.1111/j.1365-3040.2012.02484.x, 2012. a, b, c
Farquhar, G. D., O'Leary, M. H., and Berry, J. A.: On the relationship between carbon isotope discrimination and the intercellular carbon dioxide concentration in leaves, Aust. J. Plant Physiol., 9, 121–137, 1982. a
Fay, A. R., Gregor, L., Landschützer, P., McKinley, G. A., Gruber, N., Gehlen, M., Iida, Y., Laruelle, G. G., Rödenbeck, C., Roobaert, A., and Zeng, J.: SeaFlux: harmonization of air–sea CO2 fluxes from surface pCO2 data products using a standardized approach, Earth Syst. Sci. Data, 13, 4693–4710, https://doi.org/10.5194/essd-13-4693-2021, 2021. a, b
Forkel, M., Carvalhais, N., Rödenbeck, C., Keeling, R., Heimann, M., Thonicke, K., Zaehle, S., and Reichstein, M.: Enhanced seasonal CO2 exchange caused by amplified plant productivity in northern ecosystems, Science, 351, 696–699, https://doi.org/10.1126/science.aac4971, 2016. a, b, c
Frank, D. C., Poulter, B., Saurer, M., Esper, J., Huntingford, C., Helle, G., Treydte, K., Zimmermann, N. E., Schleser, G. H., Ahlstrom, A., Ciais, P., Friedlingstein, P., Levis, S., Lomas, M., Sitch, S., Viovy, N., Andreu-Hayles, L., Bednarz, Z., Berninger, F., Boettger, T., D/`Alessandro, C. M., Daux, V., Filot, M., Grabner, M., Gutierrez, E., Haupt, M., Hilasvuori, E., Jungner, H., Kalela-Brundin, M., Krapiec, M., Leuenberger, M., Loader, N. J., Marah, H., Masson-Delmotte, V., Pazdur, A., Pawelczyk, S., Pierre, M., Planells, O., Pukiene, R., Reynolds-Henne, C. E., Rinne, K. T., Saracino, A., Sonninen, E., Stievenard, M., Switsur, V. R., Szczepanek, M., Szychowska-Krapiec, E., Todaro, L., Waterhouse, J. S., and Weigl, M.: Water-use efficiency and transpiration across European forests during the Anthropocene, Nat. Clim. Change, 5, 579–583, 2015. a
Friedlingstein, P., O'Sullivan, M., Jones, M. W., Andrew, R. M., Hauck, J., Olsen, A., Peters, G. P., Peters, W., Pongratz, J., Sitch, S., Le Quéré, C., Canadell, J. G., Ciais, P., Jackson, R. B., Alin, S., Aragão, L. E. O. C., Arneth, A., Arora, V., Bates, N. R., Becker, M., Benoit-Cattin, A., Bittig, H. C., Bopp, L., Bultan, S., Chandra, N., Chevallier, F., Chini, L. P., Evans, W., Florentie, L., Forster, P. M., Gasser, T., Gehlen, M., Gilfillan, D., Gkritzalis, T., Gregor, L., Gruber, N., Harris, I., Hartung, K., Haverd, V., Houghton, R. A., Ilyina, T., Jain, A. K., Joetzjer, E., Kadono, K., Kato, E., Kitidis, V., Korsbakken, J. I., Landschützer, P., Lefèvre, N., Lenton, A., Lienert, S., Liu, Z., Lombardozzi, D., Marland, G., Metzl, N., Munro, D. R., Nabel, J. E. M. S., Nakaoka, S.-I., Niwa, Y., O'Brien, K., Ono, T., Palmer, P. I., Pierrot, D., Poulter, B., Resplandy, L., Robertson, E., Rödenbeck, C., Schwinger, J., Séférian, R., Skjelvan, I., Smith, A. J. P., Sutton, A. J., Tanhua, T., Tans, P. P., Tian, H., Tilbrook, B., van der Werf, G., Vuichard, N., Walker, A. P., Wanninkhof, R., Watson, A. J., Willis, D., Wiltshire, A. J., Yuan, W., Yue, X., and Zaehle, S.: Global Carbon Budget 2020, Earth Syst. Sci. Data, 12, 3269–3340, https://doi.org/10.5194/essd-12-3269-2020, 2020. a, b
GLOBALVIEW-CO2C13: Cooperative Atmospheric Data Integration Project – d13C of Carbon Dioxide, CD-ROM, NOAA ESRL, Boulder, Colorado, USA, 2009. a, b, c
Gonsamo, A., D'Odorico, P., Chen, J. M., Wu, C., and Buchmann, N.: Changes in vegetation phenology are not reflected in atmospheric CO2 and seasonality, Glob. Change Biol., 23, 4029–4044, https://doi.org/10.1111/gcb.13646, 2017. a, b
Graven, H. D., Keeling, R. F., Piper, S. C., Patra, P. K., Stephens, B. B., Wofsy, S. C., Welp, L. R., Sweeney, C., Tans, P. P., Kelley, J. J., Daube, B. C., Kort, E. A., Santoni, G. W., and Bent, J. D.: Enhanced Seasonal Exchange of CO2 by Northern Ecosystems Since 1960, Science, 341, 1085–1089, https://doi.org/10.1126/science.1239207, 2013. a, b, c, d, e, f
Graven, H. D., Warren, H., Gibbs, H. K., Khatiwala, S., Koven, C., Lester, J., Levin, I., Spawn-Lee, S. A., and Wieder, W.: Bomb radiocarbon evidence for strong global carbon uptake and turnover in terrestrial vegetation, Science, 384, 1335–1339, https://doi.org/10.1126/science.adl4443, 2024. a
Gurney, K. R., Law, R. M., Denning, A. S., Rayner, P. J., Pak, B. C., Baker, D., Bousquet, P., Bruhwiler, L., Chen, Y.-H., Ciais, P., Fung, I. Y., Heimann, M., John, J., Maki, T., Maksyutov, S., Peylin, P., Prather, M., and Taguchi, S.: Transcom 3 inversion intercomparison: Model mean results for the estimation of seasonal carbon sources and sinks, Global Biogeochem. Cy., 18, GB1010, https://doi.org/10.1029/2003GB002111, 2004. a
Harris, I., Osborn, T. J., Jones, P., and Lister, D.: Version 4 of the CRU TS monthly high-resolution gridded multivariate climate dataset, Sci. Data, 7, 1–18, https://doi.org/10.1038/s41597-020-0453-3, 2020. a
Hauck, J. and Völker, C.: Rising atmospheric CO2 leads to large impact of biology on Southern Ocean CO2 uptake via changes of the Revelle factor, Geophys. Res. Lett., 42, 1459–1464, https://doi.org/10.1002/2015GL063070, 2015. a
Heimann, M. and Körner, S.: The Global Atmospheric Tracer Model TM3, Technical Reports, Max-Planck-Institut für Biogeochemie, Max-Planck-Institut für Biogeochemie, Jena (Germany), Vol. 5, https://www.bgc-jena.mpg.de/archived/, (last access: 23 December 2024), 2003. a
Heimann, M., Keeling, C. D., and Tucker, C. J.: A three dimensional model of atmospheric CO2 transport based on observed winds: 3. Seasonal cycle and synoptic time scale variations, in: Aspects of Climate Variability in the Pacific and the Western Americas, 277–303, American Geophysical Union (AGU), ISBN 9781118664285, https://doi.org/10.1029/GM055p0277, 1989. a, b, c, d, e
Heimann, M., Esser, G., Haxeltine, A., Kaduk, J., Kicklighter, D. W., Knorr, W., Kohlmaier, G. H., McGuire, A. D., Melillo, J., Moore, B., Otto, R. D., Prentice, I. C., Sauf, W., Schloss, A., Sitch, S., Wittenberg, U., and Würth, G.: Evaluation of terrestrial carbon cycle models through simulations of the seasonal cycle of atmospheric CO2: First results of a model intercomparison study, Global Biogeochem. Cy., 12, 1–24, https://doi.org/10.1029/97GB01936, 1998. a, b, c
Hurtt, G. C., Chini, L., Sahajpal, R., Frolking, S., Bodirsky, B. L., Calvin, K., Doelman, J. C., Fisk, J., Fujimori, S., Klein Goldewijk, K., Hasegawa, T., Havlik, P., Heinimann, A., Humpenöder, F., Jungclaus, J., Kaplan, J. O., Kennedy, J., Krisztin, T., Lawrence, D., Lawrence, P., Ma, L., Mertz, O., Pongratz, J., Popp, A., Poulter, B., Riahi, K., Shevliakova, E., Stehfest, E., Thornton, P., Tubiello, F. N., van Vuuren, D. P., and Zhang, X.: Harmonization of global land use change and management for the period 850–2100 (LUH2) for CMIP6, Geosci. Model Dev., 13, 5425–5464, https://doi.org/10.5194/gmd-13-5425-2020, 2020. a, b
Ito, A., Inatomi, M., Huntzinger, D. N., Schwalm, C., Michalak, A. M., Cook, R., King, A. W., Mao, J., Wei, Y., Post, W. M., Wang, W., Arain, M. A., Huang, S., Hayes, D. J., Ricciuto, D. M., Shi, X., Huang, M., Lei, H., Tian, H., Lu, C., Yang, J., Tao, B., Jain, A., Poulter, B., Peng, S., Ciais, P., Fisher, J. B., Parazoo, N., Schaefer, K., Peng, C., Zeng, N., and Zhao, F.: Decadal trends in the seasonal-cycle amplitude of terrestrial CO2 exchange resulting from the ensemble of terrestrial biosphere models, Tellus B, 68, 28968, https://doi.org/10.3402/tellusb.v68.28968, 2016. a
Jeltsch-Thömmes, A. and Joos, F.: Modeling the evolution of pulse-like perturbations in atmospheric carbon and carbon isotopes: the role of weathering–sedimentation imbalances, Clim. Past, 16, 423–451, https://doi.org/10.5194/cp-16-423-2020, 2020. a
Jeltsch-Thömmes, A. and Joos, F.: Carbon Cycle Responses to Changes in Weathering and the Long-Term Fate of Stable Carbon Isotopes, Paleoceanogr. Paleocl., 38, e2022PA004577, https://doi.org/10.1029/2022PA004577, 2023. a
Jin, Y., Keeling, R. F., Stephens, B. B., Long, M. C., Patra, P. K., Rödenbeck, C., Morgan, E. J., Kort, E. A., and Sweeney, C.: Improved atmospheric constraints on Southern Ocean CO2 exchange, P. Natl. Acad. Sci., 121, e2309333121, https://doi.org/10.1073/pnas.2309333121, 2024. a
Joos, F. and Bruno, M.: Long-term variability of the terrestrial and oceanic carbon sinks and the budgets of the carbon isotopes 13C and 14C, Global Biogeochem. Cy., 12, 277–295, https://doi.org/10.1029/98GB00746, 1998. a
Joos, F., Hameau, A., Frölicher, T. L., and Stephenson, D. B.: Anthropogenic Attribution of the Increasing Seasonal Amplitude in Surface Ocean pCO2, Geophys. Res. Lett., 50, e2023GL102857, https://doi.org/10.1029/2023GL102857, 2023. a
Joos, F., Lienert, S., and Zaehle, S.: No increase is detected and modelled for the seasonal cycle amplitude of δ13C of atmospheric carbon dioxide: scripts and data to prepare figures, Zenodo [data set], https://doi.org/10.5281/zenodo.14051464, 2024. a
Kalnay, E., Kanamitsu, M., Kistler, R., Collins, W., Deaven, D., Gandin, L., Iredell, M., Saha, S., White, G., Woollen, J., Zhu, Y., Leetmaa, A., Reynolds, R., Chelliah, M., Ebisuzaki, W., Higgins, W., Janowiak, J., Mo, K. C., Ropelewski, C., Wang, J., Jenne, R., and Joseph, D.: The NCEP/NCAR 40-Year Reanalysis Project, B. Am. Meteorol. Soc., 77, 437–471, https://doi.org/10.1175/1520-0477(1996)077<0437:TNYRP>2.0.CO;2, 1996. a
Kaminski, T., Heimann, M., and Giering, R.: A Global Scale Inversion of the Transport of CO2 Based on a Matrix Representation of an Atmospheric Transport Model Derived by Its Adjoint, in: Air Pollution Modeling and Its Application XII, 247–255, Springer US, Boston, MA, https://doi.org/10.1007/978-1-4757-9128-0_26, 1998. a
Kauwe, M. G., Medlyn, B. E., Zaehle, S., Walker, A. P., Dietze, M. C., Hickler, T., Jain, A. K., Luo, Y., Parton, W. J., Prentice, I. C., Smith, B., Thornton, P. E., Wang, S., Wang, Y.-P., Wårlind, D., Weng, E., Crous, K. Y., Ellsworth, D. S., Hanson, P. J., Kim, H.-S., Warren, J. M., Oren, R., and Norby, R. J.: Forest water use and water use efficiency at elevated CO2: a model-data intercomparison at two contrasting temperate forest FACE sites, Glob. Change Biol., 19, 1759–1779, https://doi.org/10.1111/gcb.12164, 2013. a
Keeling, C. D.: The Concentration and Isotopic Abundances of Carbon Dioxide in the Atmosphere, Tellus, 12, 200–203, https://doi.org/10.1111/j.2153-3490.1960.tb01300.x, 1960. a
Keeling, C. D., Carter, A. F., and Mook, W. G.: Seasonal, latitudinal, and secular variations in the abundance and isotopic ratios of atmospheric CO2: 2. Results from oceanographic cruises in the tropical Pacific Ocean, J. Geophys. Res.-Atmos., 89, 4615–4628, https://doi.org/10.1029/JD089iD03p04615, 1984. a
Keeling, C. D., Bacastow, R. B., Carter, A. F., Piper, S. C., Whorf, T. P., Heimann, M., Mook, W. G., and Roeloffzen, H.: A three dimensional model of atmospheric CO2 transport based on observed winds: 1. Analysis of Observational Data, in: Aspects of Climate Variability in the Pacific and the Western Americas, 165–236, American Geophysical Union (AGU), ISBN 9781118664285, https://doi.org/10.1029/GM055p0165, 1989. a, b
Keeling, C. D., Chin, J. F. S., and Whorf, T. P.: Increased activity of northern vegetation inferred from atmospheric CO2 measurements, Nature, 382, 146–149, https://doi.org/10.1038/382146a0, 1996. a, b, c
Keeling, C. D., Piper, S. C., Bacastow, R. B., Wahlen, M., Whorf, T. P., Heimann, M., and Meijer, H. A.: Exchanges of atmospheric CO2 and 13CO2 with the terrestrial biosphere and oceans from 1978 to 2000. I. Global aspects, SIO Reference Series, No. 01-06, San Diego, p. 88, Scripps Institution of Oceanography [data sets], https://escholarship.org/uc/item/09v319r9 (last access: 23 December 2024), https://scrippsco2.ucsd.edu/data/atmospheric_co2 (last access: 17 April 2023), 2001. a, b, c, d, e
Keeling, C. D., Piper, S. C., Bacastow, R. B., Wahlen, M., Whorf, T. P., Heimann, M., and Meijer, H. A.: Atmospheric CO2 and 13CO2 Exchange with the Terrestrial Biosphere and Oceans from 1978 to 2000: Observations and Carbon Cycle Implications, in: A History of Atmospheric CO2 and Its Effects on Plants, Animals, and Ecosystems, edited by: Baldwin, I. T., Caldwell, M. M., Heldmaier, G., Jackson, R. B., Lange, O. L., Mooney, H. A., Schulze, E.-D., Sommer, U., Ehleringer, J. R., Denise Dearing, M., and Cerling, T. E., 83–113, Springer New York, New York, NY, ISBN 978-0-387-27048-7, https://doi.org/10.1007/0-387-27048-5_5, 2005. a
Keeling, R. F., Graven, H. D., Welp, L. R., Resplandy, L., Bi, J., Piper, S. C., Sun, Y., Bollenbacher, A., and Meijer, H. A. J.: Atmospheric evidence for a global secular increase in carbon isotopic discrimination of land photosynthesis, P. Natl. Acad. Sci., 114, 10361–10366, https://doi.org/10.1073/pnas.1619240114, 2017. a, b, c, d, e, f, g, h
Keenan, T. F., Hollinger, D. Y., Bohrer, G., Dragoni, D., Munger, J. W., Schmid, H. P., and Richardson, A. D.: Increase in forest water-use efficiency as atmospheric carbon dioxide concentrations rise, Nature, 499, 324–327, https://doi.org/10.1038/nature12291, 2013. a, b, c
Keller, K. M., Joos, F., and Raible, C. C.: Time of emergence of trends in ocean biogeochemistry, Biogeosciences, 11, 3647–3659, https://doi.org/10.5194/bg-11-3647-2014, 2014. a, b
Keller, K. M., Lienert, S., Bozbiyik, A., Stocker, T. F., Churakova (Sidorova), O. V., Frank, D. C., Klesse, S., Koven, C. D., Leuenberger, M., Riley, W. J., Saurer, M., Siegwolf, R., Weigt, R. B., and Joos, F.: 20th century changes in carbon isotopes and water-use efficiency: tree-ring-based evaluation of the CLM4.5 and LPX-Bern models, Biogeosciences, 14, 2641–2673, https://doi.org/10.5194/bg-14-2641-2017, 2017. a, b
Knauer, J., Zaehle, S., Reichstein, M., Medlyn, B. E., Forkel, M., Hagemann, S., and Werner, C.: The response of ecosystem water-use efficiency to rising atmospheric CO2 concentrations: sensitivity and large-scale biogeochemical implications, New Phytol., 213, 1654–1666, https://doi.org/10.1111/nph.14288, 2017. a, b
Körner, C.: A matter of tree longevity, Science, 355, 130–131, https://doi.org/10.1126/science.aal2449, 2017. a
Landschützer, P., Gruber, N., Bakker, D. C. E., and Schuster, U.: Recent variability of the global ocean carbon sink, Global Biogeochem. Cy., 28, 927–949, https://doi.org/10.1002/2014GB004853, 2014. a
Lienert, S. and Joos, F.: A Bayesian ensemble data assimilation to constrain model parameters and land-use carbon emissions, Biogeosciences, 15, 2909–2930, https://doi.org/10.5194/bg-15-2909-2018, 2018. a, b, c, d
Lloyd, J. and Farquhar, G.: 13C discrimination during CO2 assimilation by the terrestrial biosphere, Oecologia, 99, 201–215, https://doi.org/10.1007/BF00627732, 1994. a, b, c
Long, M. C., Stephens, B. B., McKain, K., Sweeney, C., Keeling, R. F., Kort, E. A., Morgan, E. J., Bent, J. D., Chandra, N., Chevallier, F., Commane, R., Daube, B. C., Krummel, P. B., Loh, Z., Luijkx, I. T., Munro, D., Patra, P., Peters, W., Ramonet, M., Rödenbeck, C., Stavert, A., Tans, P., and Wofsy, S. C.: Strong Southern Ocean carbon uptake evident in airborne observations, Science, 374, 1275–1280, https://doi.org/10.1126/science.abi4355, 2021. a
Lueker, T., Keeling, R., Bollenbacher, A., Walker, S., Morgan, E., and Brooks, M.: Calibration Methodology for the Scripps and stable Isotope program 1992–2018, UC San Diego, Scripps Institution of Oceanography, https://escholarship.org/uc/item/4n93p288 (last access: 23 December 2024), p. 39, 2020. a
Majkut, J. D., Carter, B. R., Frölicher, T. L., Dufour, C. O., Rodgers, K. B., and Sarmiento, J. L.: An observing system simulation for Southern Ocean carbon dioxide uptake, Philos. T. R. Soc. A, 372, 20130046, https://doi.org/10.1098/rsta.2013.0046, 2014. a
Masarie, K. A., Peters, W., Jacobson, A. R., and Tans, P. P.: ObsPack: a framework for the preparation, delivery, and attribution of atmospheric greenhouse gas measurements, Earth Syst. Sci. Data, 6, 375–384, https://doi.org/10.5194/essd-6-375-2014, 2014. a, b
Menviel, L., Mouchet, A., Meissner, K. J., Joos, F., and England, M. H.: Impact of oceanic circulation changes on atmospheric δ13CO2, Global Biogeochem. Cy., 29, 1944–1961, https://doi.org/10.1002/2015GB005207, 2015. a, b
Mook, W.: 13C in atmospheric CO2, Neth. J. Sea Res., 20, 211–223, https://doi.org/10.1016/0077-7579(86)90043-8, 1986. a, b
Peng, S., Ciais, P., Chevallier, F., Peylin, P., Cadule, P., Sitch, S., Piao, S., Ahlström, A., Huntingford, C., Levy, P., Li, X., Liu, Y., Lomas, M., Poulter, B., Viovy, N., Wang, T., Wang, X., Zaehle, S., Zeng, N., Zhao, F., and Zhao, H.: Benchmarking the seasonal cycle of CO2 fluxes simulated by terrestrial ecosystem models, Global Biogeochem. Cy., 29, 46–64, https://doi.org/10.1002/2014GB004931, 2015. a
Peylin, P., Law, R. M., Gurney, K. R., Chevallier, F., Jacobson, A. R., Maki, T., Niwa, Y., Patra, P. K., Peters, W., Rayner, P. J., Rödenbeck, C., van der Laan-Luijkx, I. T., and Zhang, X.: Global atmospheric carbon budget: results from an ensemble of atmospheric CO2 inversions, Biogeosciences, 10, 6699–6720, https://doi.org/10.5194/bg-10-6699-2013, 2013. a
Peylin, P., Bacour, C., MacBean, N., Leonard, S., Rayner, P., Kuppel, S., Koffi, E., Kane, A., Maignan, F., Chevallier, F., Ciais, P., and Prunet, P.: A new stepwise carbon cycle data assimilation system using multiple data streams to constrain the simulated land surface carbon cycle, Geosci. Model Dev., 9, 3321–3346, https://doi.org/10.5194/gmd-9-3321-2016, 2016. a
Peñuelas, J., Canadell, J. G., and Ogaya, R.: Increased water-use efficiency during the 20th century did not translate into enhanced tree growth, Global Ecol. Biogeogr., 20, 597–608, https://doi.org/10.1111/j.1466-8238.2010.00608.x, 2011. a
Piao, S., Liu, Z., Wang, Y., Ciais, P., Yao, Y., Peng, S., Chevallier, F., Friedlingstein, P., Janssens, I. A., Peñuelas, J., Sitch, S., and Wang, T.: On the causes of trends in the seasonal amplitude of atmospheric CO2, Glob. Change Biol., 24, 608–616, https://doi.org/10.1111/gcb.13909, 2018. a, b
Quay, P. D., Sonnerup, R., Munro, D. R., and Sweeney, C.: Anthropogenic CO2 accumulation and uptake rates in the Pacific Ocean based on changes in the of dissolved inorganic carbon, Global Biogeochem. Cy., 31, 59–80, https://doi.org/10.1002/2016GB005460, 2017. a
Ritz, S. P., Stocker, T. F., Joos, F., Ritz, S. P., Stocker, T. F., and Joos, F.: A Coupled Dynamical Ocean–Energy Balance Atmosphere Model for Paleoclimate Studies, J. Climate, 24, 349–375, https://doi.org/10.1175/2010JCLI3351.1, 2011. a, b
Saurer, M. and Voelker, S.: Intrinsic Water-Use Efficiency Derived from Stable Carbon Isotopes of Tree-Rings, 481–498, Springer International Publishing, Cham, ISBN 978-3-030-92698-4, https://doi.org/10.1007/978-3-030-92698-4_17, 2022. a
Saurer, M., Spahni, R., Frank, D. C., Joos, F., Leuenberger, M., Loader, N. J., McCarroll, D., Gagen, M., Poulter, B., Siegwolf, R. T., Andreu-Hayles, L., Boettger, T., Dorado Liñán, I., Fairchild, I. J., Friedrich, M., Gutierrez, E., Haupt, M., Hilasvuori, E., Heinrich, I., Helle, G., Grudd, H., Jalkanen, R., Levanič, T., Linderholm, H. W., Robertson, I., Sonninen, E., Treydte, K., Waterhouse, J. S., Woodley, E. J., Wynn, P. M., and Young, G. H.: Spatial variability and temporal trends in water-use efficiency of European forests, Glob. Change Biol., 20, 3700–3712, https://doi.org/10.1111/gcb.12717, 2014. a
Scholze, M., Kaplan, J. O., Knorr, W., and Heimann, M.: Climate and interannual variability of the atmosphere-biosphere 13CO2 flux, Geophys. Res. Lett., 30, 1–4, https://doi.org/10.1029/2002GL015631, 2003. a
Scholze, M., Ciais, P., and Heimann, M.: Modeling terrestrial 13C cycling: Climate, land use and fire, Global Biogeochem. Cy., 22, 1–13, https://doi.org/10.1029/2006GB002899, 2008. a
Schürmann, G. J., Kaminski, T., Köstler, C., Carvalhais, N., Voßbeck, M., Kattge, J., Giering, R., Rödenbeck, C., Heimann, M., and Zaehle, S.: Constraining a land-surface model with multiple observations by application of the MPI-Carbon Cycle Data Assimilation System V1.0, Geosci. Model Dev., 9, 2999–3026, https://doi.org/10.5194/gmd-9-2999-2016, 2016. a
Sitch, S., Smith, B., Prentice, I. C., Arneth, A., Bondeau, A., Cramer, W., Kaplan, J. O., Levis, S., Lucht, W., Sykes, M. T., Thonicke, K., and Venevsky, S.: Evaluation of ecosystem dynamics, plant geography and terrestrial carbon cycling in the LPJ dynamic global vegetation model, Glob. Change Biol., 9, 161–185, https://doi.org/10.1046/j.1365-2486.2003.00569.x, 2003. a
Still, C. J., Berry, J. A., Collatz, G. J., and DeFries, R. S.: Global distribution of C3 and C4 vegetation: Carbon cycle implications, Global Biogeochem. Cy., 17, 6-1–6-14, https://doi.org/10.1029/2001GB001807, 2003. a
Stocker, B. D., Spahni, R., and Joos, F.: DYPTOP: a cost-efficient TOPMODEL implementation to simulate sub-grid spatio-temporal dynamics of global wetlands and peatlands, Geosci. Model Dev., 7, 3089–3110, https://doi.org/10.5194/gmd-7-3089-2014, 2014. a
Strassmann, K. M., Joos, F., and Fischer, G.: Simulating effects of land use changes on carbon fluxes: past contributions to atmospheric CO2 increases and future commitments due to losses of terrestrial sink capacity, Tellus B, 60, 583–603, 2008. a
Takahashi, T., Sutherland, S. C., Wanninkhof, R., Sweeney, C., Feely, R. A., Chipman, D. W., Hales, B., Friederich, G., Chavez, F., Sabine, C., Watson, A., Bakker, D. C., Schuster, U., Metzl, N., Yoshikawa-Inoue, H., Ishii, M., Midorikawa, T., Nojiri, Y., Körtzinger, A., Steinhoff, T., Hoppema, M., Olafsson, J., Arnarson, T. S., Tilbrook, B., Johannessen, T., Olsen, A., Bellerby, R., Wong, C., Delille, B., Bates, N., and de Baar, H. J.: Climatological mean and decadal change in surface ocean pCO2, and net sea–air CO2 flux over the global oceans, Deep-Sea Res. Pt. II, 56, 554–577, https://doi.org/10.1016/j.dsr2.2008.12.009, 2009. a
Tans, P. P., Berry, J. A., and Keeling, R. F.: Oceanic observations: A new window on ocean CO2 uptake, Global Biogeochem. Cy., 7, 353–368, https://doi.org/10.1029/93GB00053, 1993. a
Tian, H., Yang, J., Lu, C., Xu, R., Canadell, J. G., Jackson, R. B., Arneth, A., Chang, J., Chen, G., Ciais, P., Gerber, S., Ito, A., Huang, Y., Joos, F., Lienert, S., Messina, P., Olin, S., Pan, S., Peng, C., Saikawa, E., Thompson, R. L., Vuichard, N., Winiwarter, W., Zaehle, S., Zhang, B., Zhang, K., and Zhu, Q.: The Global N2O Model Intercomparison Project, B. Am. Meteorol. Soc., 99, 1231–1251, https://doi.org/10.1175/BAMS-D-17-0212.1, 2018. a
van der Velde, I. R., Miller, J. B., Schaefer, K., Masarie, K. A., Denning, S., White, J. W. C., Tans, P. P., Krol, M. C., and Peters, W.: Biosphere model simulations of interannual variability in terrestrial exchange, Global Biogeochem. Cy., 27, 637–649, https://doi.org/10.1002/gbc.20048, 2013. a
van der Velde, I. R., Miller, J. B., van der Molen, M. K., Tans, P. P., Vaughn, B. H., White, J. W. C., Schaefer, K., and Peters, W.: The CarbonTracker Data Assimilation System for CO2 and δ13C (CTDAS-C13 v1.0): retrieving information on land–atmosphere exchange processes, Geosci. Model Dev., 11, 283–304, https://doi.org/10.5194/gmd-11-283-2018, 2018. a, b
Voelker, S. L., Brooks, J. R., Meinzer, F. C., Anderson, R., Bader, M. K.-F., Battipaglia, G., Becklin, K. M., Beerling, D., Bert, D., Betancourt, J. L., Dawson, T. E., Domec, J.-C., Guyette, R. P., Körner, C., Leavitt, S. W., Linder, S., Marshall, J. D., Mildner, M., Ogée, J., Panyushkina, I., Plumpton, H. J., Pregitzer, K. S., Saurer, M., Smith, A. R., Siegwolf, R. T. W., Stambaugh, M. C., Talhelm, A. F., Tardif, J. C., Van de Water, P. K., Ward, J. K., and Wingate, L.: A dynamic leaf gas-exchange strategy is conserved in woody plants under changing ambient CO2: evidence from carbon isotope discrimination in paleo and CO2 enrichment studies, Glob. Change Biol., 22, 889–902, https://doi.org/10.1111/gcb.13102, 2016. a
Walker, A. P., De Kauwe, M. G., Bastos, A., Belmecheri, S., Georgiou, K., Keeling, R. F., McMahon, S. M., Medlyn, B. E., Moore, D. J., Norby, R. J., Zaehle, S., Anderson-Teixeira, K. J., Battipaglia, G., Brienen, R. J., Cabugao, K. G., Cailleret, M., Campbell, E., Canadell, J. G., Ciais, P., Craig, M. E., Ellsworth, D. S., Farquhar, G. D., Fatichi, S., Fisher, J. B., Frank, D. C., Graven, H., Gu, L., Haverd, V., Heilman, K., Heimann, M., Hungate, B. A., Iversen, C. M., Joos, F., Jiang, M., Keenan, T. F., Knauer, J., Körner, C., Leshyk, V. O., Leuzinger, S., Liu, Y., MacBean, N., Malhi, Y., McVicar, T. R., Penuelas, J., Pongratz, J., Powell, A. S., Riutta, T., Sabot, M. E., Schleucher, J., Sitch, S., Smith, W. K., Sulman, B., Taylor, B., Terrer, C., Torn, M. S., Treseder, K. K., Trugman, A. T., Trumbore, S. E., van Mantgem, P. J., Voelker, S. L., Whelan, M. E., and Zuidema, P. A.: Integrating the evidence for a terrestrial carbon sink caused by increasing atmospheric CO2, New Phytol., 229, 2413–2445, https://doi.org/10.1111/nph.16866, 2021. a
Wang, S., Zhang, Y., Ju, W., Chen, J. M., Ciais, P., Cescatti, A., Sardans, J., Janssens, I. A., Wu, M., Berry, J. A., Campbell, E., Fernández-Martínez, M., Alkama, R., Sitch, S., Friedlingstein, P., Smith, W. K., Yuan, W., He, W., Lombardozzi, D., Kautz, M., Zhu, D., Lienert, S., Kato, E., Poulter, B., Sanders, T. G. M., Krüger, I., Wang, R., Zeng, N., Tian, H., Vuichard, N., Jain, A. K., Wiltshire, A., Haverd, V., Goll, D. S., and Peñuelas, J.: Recent global decline of CO2 fertilization effects on vegetation photosynthesis, Science, 370, 1295–1300, https://doi.org/10.1126/science.abb7772, 2020. a
Wania, R., Ross, I., and Prentice, I. C.: Integrating peatlands and permafrost into a dynamic global vegetation model: 1. Evaluation and sensitivity of physical land surface processes, Global Biogeochem. Cy., 23, 1–19, https://doi.org/10.1029/2008GB003412, 2009a. a
Wania, R., Ross, I., and Prentice, I. C.: Integrating peatlands and permafrost into a dynamic global vegetation model: 2. Evaluation and sensitivity of vegetation and carbon cycle processes, Global Biogeochem. Cy., 23, 1–15, https://doi.org/10.1029/2008GB003413, 2009b. a
Welch, B. L.: The Generalization of “Student's” Problem when Several Different Population Variances are Involved, Biometrika, 34, 28–35, https://doi.org/10.2307/2332510, 1947. a
Xu-Ri and Prentice, I. C.: Terrestrial nitrogen cycle simulation with a dynamic global vegetation model, Glob. Change Biol., 14, 1745–1764, https://doi.org/10.1111/j.1365-2486.2008.01625.x, 2008. a
- Abstract
- Introduction
- Methods
- The influence of carbon and isotope fluxes on the seasonal cycles of Ca and δ13Ca: a conceptual framework
- Results
- Conclusions
- Appendix A: Decomposition of and the calculation of seasonal amplitudes
- Appendix B: Uncertainties in the relationship between εNPP and
- Code and data availability
- Author contributions
- Competing interests
- Disclaimer
- Acknowledgements
- Financial support
- Review statement
- References
- Supplement
- Abstract
- Introduction
- Methods
- The influence of carbon and isotope fluxes on the seasonal cycles of Ca and δ13Ca: a conceptual framework
- Results
- Conclusions
- Appendix A: Decomposition of and the calculation of seasonal amplitudes
- Appendix B: Uncertainties in the relationship between εNPP and
- Code and data availability
- Author contributions
- Competing interests
- Disclaimer
- Acknowledgements
- Financial support
- Review statement
- References
- Supplement