the Creative Commons Attribution 4.0 License.
the Creative Commons Attribution 4.0 License.
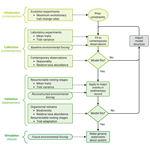
Ideas and perspectives: How sediment archives can improve model projections of marine ecosystem change
Isabell Hochfeld
Ben A. Ward
Anke Kremp
Juliane Romahn
Alexandra Schmidt
Miklós Bálint
Lutz Becks
Jérôme Kaiser
Helge W. Arz
Sarah Bolius
Laura S. Epp
Markus Pfenninger
Christopher A. Klausmeier
Elena Litchman
Global warming is a major threat to marine biodiversity and ecosystem functioning, with consequences that are yet largely unknown. To frame these consequences, we need to understand how marine ecosystems respond to warming and related environmental changes. Ecosystem models have proven to be a valuable tool in this respect, but their projections vary considerably. A major limitation in current ecosystem models may be that they largely ignore evolutionary processes, which nonetheless can be relevant on the simulated timescales. In addition, ecosystem models are usually fit to contemporary data and used predictively afterwards, without further validation that they are equally applicable to past (and, by inference, future) scenarios. A promising approach to validate evolutionary ecosystem models is the use of biological archives such as natural sediments, which record long-term ecosystem changes. Since the ecosystem changes present in sediment records are affected by evolution, evolution needs to be represented in ecosystem models not only to realistically simulate the future, but also the sediment record itself. The sediment record, in turn, can provide the required constraints on long-term evolutionary changes, along with information on past environmental conditions, biodiversity, and relative abundances of taxa. Here, we present a framework to make use of such information to validate evolutionary ecosystem models and improve model projections of future ecosystem changes. Using the example of phytoplankton, key players in marine systems, we review the existing literature and discuss (I) which data can be derived from ancient sedimentary archives, (II) how we can integrate these data into evolutionary ecosystem models to improve their projections of climate-driven ecosystem changes, and (III) future perspectives and aspects that remain challenging.
- Article
(1860 KB) - Full-text XML
- BibTeX
- EndNote
Driven by the reality of global warming as a major threat to marine biodiversity and ecosystem functioning, ecosystem models are increasingly used to estimate future changes in marine ecosystems. However, projected changes differ notably between models (Laufkötter et al., 2015, 2016; Tittensor et al., 2021), which may be due to the fact that evolutionary processes are generally neglected, even though they can be of great importance on the simulated timescales (Irwin et al., 2015; Jin and Agustí, 2018). The reliability of current model projections therefore remains questionable. Here, we propose to use data from sediment archives to validate evolutionary ecosystem models before using them predictively and discuss how this approach can improve model projections.
Compared with the period 1850–1900, global surface temperature has already increased by 1.25 °C and, under the most extreme emissions scenario, is expected to increase by up to a further 3.5 °C by the end of the century (scenario SSP5-8.5; Intergovernmental Panel on Climate Change (IPCC), 2023). The current state of warming has already caused changes in marine communities (Peer and Miller, 2014; Poloczanska et al., 2013; Wasmund et al., 2019), which perform ecosystem functions that are vital to human societies, including food production (Hollowed et al., 2013) and carbon sequestration (Hain et al., 2014). The response of these ecosystem services under ongoing global warming remains subject to great uncertainty, and there is a real but unknown risk of positive feedbacks, irreversible tipping points, and ecosystem collapse (Lenton et al., 2008). For example, IPCC models systematically underestimate the decline in Arctic summer sea ice, which suggests that a tipping point may already have or may soon be passed (Lenton et al., 2008; Stroeve et al., 2007). Passing this tipping point can cause strong nonlinear and long-term changes in the system, such as amplified warming and sea ice loss through a positive albedo feedback, and, in turn, major ecosystem change (Holland et al., 2006; Lenton et al., 2008).
Dynamic ecosystem models currently represent the best tool to understand complex feedbacks between evolving ecosystems and their environment, but it is a considerable challenge to develop models that would apply equally well to past, present, and future scenarios. Despite their great potential, current models project diverging changes in ecosystem functions like carbon cycling and net primary production (Laufkötter et al., 2015, 2016; Tittensor et al., 2021). For example, a recent coupled model intercomparison showed a variability of up to ±10 % in projected global net primary production, depending on the ecosystem model and earth system forcing used (Tittensor et al., 2021). This disagreement between models illustrates how critical it is to assess the validity of model projections.
To improve model projections, we need (I) to verify that the most relevant processes are considered and (II) to validate projections with long-term data. Regarding (I), current ecosystem models largely ignore a crucial process that can influence ecosystem responses to environmental changes on perennial timescales – evolutionary adaptation (Hattich et al., 2024; Irwin et al., 2015; O'Donnell et al., 2018). Some ecosystem models already consider adaptation, but only a small number of models have been compared to empirical data, both from experiments (Denman, 2017) and from sediment archives (Gibbs et al., 2020; Hinners et al., 2019). So far, however, testing against data has not been used to improve projections made by these models. With respect to (II), both experiments and marine monitoring studies cannot account for environmental changes on longer than decadal timescales, while experiments can hardly capture the complexity of real ecosystems. Natural archives such as sediments, however, allow for the reconstruction of long-term ecosystem responses to past environmental changes (Capo et al., 2021; Ellegaard et al., 2020). Sediments preserve abiotic and biotic environmental proxies (Hillaire-Marcel and de Vernal, 2007), other organismal remains such as DNA (Alsos et al., 2022; Monchamp et al., 2016; Zimmermann et al., 2023), and dormant resting stages. Dormant resting stages, which are formed by many phytoplankton and zooplankton taxa, are typically characterized by resistant, thick walls and the capacity to endure long phases of physiological dormancy (Ellegaard and Ribeiro, 2018). Similar to seeds, these resting stages can be resurrected and used for experiments (Bennington et al., 1991; Hinners et al., 2017; Isanta-Navarro et al., 2021). Since sediments can be dated, we can use the preserved information to derive long-term time series on past environmental conditions, biodiversity, relative taxa abundance, and adaptive changes in (functional) traits. Thus, sediment archives are well suited to constrain the long-term evolutionary changes needed to validate evolutionary ecosystem models. Evolution, in turn, needs to be represented in ecosystem models to simulate its impact on the sediment record.
Here, we discuss how we can use data from sediment archives to improve evolutionary ecosystem models and their projections of marine ecosystem change. Our approach focuses on phytoplankton, key players in marine ecosystems and respective models. Phytoplankton account for about half of global photosynthesis (Field et al., 1998), are the basis of the marine food web (Fenchel, 1988), represent an important component of biogeochemical cycles (Hutchins and Fu, 2017), and can even influence ocean physics (Hense, 2007; Sathyendranath et al., 1991). In addition, the large population sizes and short generation times of phytoplankton allow them to adapt quickly to changing environmental conditions (Aranguren-Gassis et al., 2019; Hattich et al., 2024; O'Donnell et al., 2018). All these factors, together with their long-lived dormant resting stages (Delebecq et al., 2020; Sanyal et al., 2022), make phytoplankton ideal model organisms for the approach we present here. Based on the existing literature, we discuss which data we can obtain from sediment archives, how we can use these data to improve evolutionary ecosystem models and their projections, and remaining challenges and future perspectives.
Sediment archives provide information on past ecosystem status, including both environmental and biological data (Fig. 1). Such data can be used to constrain evolutionary ecosystem models.
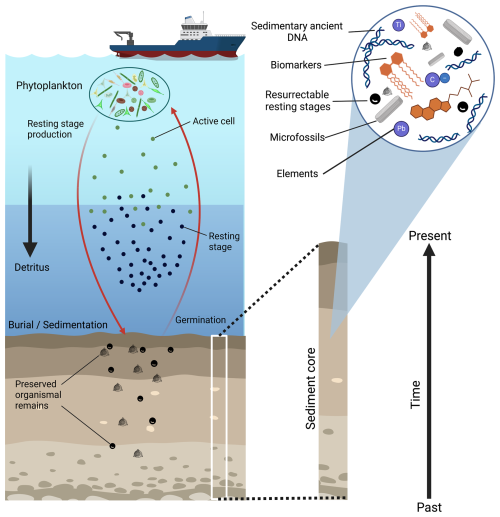
Figure 1Overview of different types of data (environmental and biological) that can be obtained from sediment archives. Left: schematic showing the deposition of organismal remains in the sediment. Red arrows indicate the production of dormant resting stages (thick-walled cells that can endure long phases of physiological dormancy), their deposition in the sediment, and the germination of resting stages from the sediment. The black arrow represents sinking of dead organic matter (detritus) to the seafloor. Preserved organismal remains, a mixture of resting stages and detritus, are shown in the sediment. Right: close-up of the sediment core showing different types of data that can be obtained. The figure was created with BioRender.com.
2.1 Dating sediment archives
Before working with sediment archives, the sediments must be dated accurately to obtain a well-constrained relationship between age and sediment depth, a so-called age model. Common sediment dating methods include radiocarbon dating, lead isotope dating, and event stratigraphy. Radiocarbon (14C) dating is based on the 14C half-life. By determining the amount of radioactive 14C relative to the 12C stable isotope, it is possible to estimate age ca. 50 000 years back in time (Hajdas et al., 2021). After 1950, radiocarbon dating is not applicable anymore due to the radiocarbon added artificially to the atmosphere by nuclear bomb tests. Therefore, sediments deposited after 1950 are dated using other methods such as lead isotope (210Pb) dating and event stratigraphy. While the 210Pb dating approach is based on the half-life of atmospheric 210Pb (Appleby, 2001), event stratigraphy is based on the detection of specific events such as nuclear bomb tests, volcanic eruptions, and other distinct anthropogenic impacts that are registered in, for example, chemical parameters of the sediments (Hancock et al., 2011; Lowe and Alloway, 2015). By combining all the dating methods mentioned above, it is possible to obtain robust age models of sediment cores over the last ca. 50 000 years. Other stratigraphic methods (e.g., oxygen isotope stratigraphy, biostratigraphy, or paleomagnetic stratigraphy) are applied to date older sediments deposited in aquatic environments (Bradley, 1999).
2.2 Environmental data
Abiotic and biotic proxies, or indicators, preserved in sediment archives allow for the reconstruction of physicochemical characteristics of past marine and limnic environments. For example, surface salinity can be estimated using lipids (alkenones) produced by micro-phytoplankton species of the order Isochrysidales (Kaiser et al., 2017; Medlin et al., 2008; Rosell-Melé, 1998). Some trace metals and their isotopes are indicators for past suboxic to euxinic conditions in the water column and/or the sediments (Brumsack, 2006; Dellwig et al., 2019). Relative assemblages of microfossils (e.g., resting stages of dinoflagellates, silica frustules of diatoms, calcareous shells of foraminifera) and their shell geochemistry provide important information not only on salinity, but also on pH, trophic state, and temperature, and are therefore powerful proxies (Cléroux et al., 2008; Hillaire-Marcel and de Vernal, 2007; Lear et al., 2002). Organic indexes based on biomarkers, e.g., alkenones (U; Prahl et al., 1988) or other membrane lipids derived from archaea (TEX86; Schouten et al., 2013) can be used to reconstruct surface and subsurface temperatures. These and many other physical methods, biological proxies, and geochemical tracers find their diverse applications in paleoceanography (Hillaire-Marcel and de Vernal, 2007).
Proxy-based reconstructions must be considered carefully as they may be biased due to preservation/degradation and influenced by local-to-regional environments. Using a multiproxy approach and calibration depending on the environment is important for reliable reconstructions. Reconstructed environmental conditions of the past can then be used as forcing for ecosystem models.
2.3 Biological data
Apart from information on environmental conditions, sediment archives provide a wide variety of biological information, such as biodiversity, relative taxa abundance, and trait adaptation. This biological information is valuable for validating evolutionary ecosystem models.
2.3.1 Microfossils
Traditionally, the focus of research on sediment archives has been on fossilized plankton remains. Fossil phytoplankton communities only represent species that consist of stable mineral structures (e.g., of silica or carbonate) or contain specific fossilizable molecules such as sporopollenin. Among dinoflagellates, only a fraction of the community produces resting cysts (Limoges et al., 2020; Van Nieuwenhove et al., 2020), which are preserved over time and can be used for quantitative paleoecological reconstructions and biostratigraphy. Diatoms, on the other hand, are well represented in the fossil record due to their resistant silica frustules with their diverse species-specific structures (Weckström, 2006). Some filamentous cyanobacteria also produce resistant resting stages, akinetes, constituting long-term records in brackish-marine and lake sediments (Wood et al., 2021). In lakes, chrysophyte cysts can provide long-term records that reveal group-specific phytoplankton dynamics over long timescales (Korkonen et al., 2017). While microfossil data provide continuous (semi-)quantitative records of the relative biomasses of the represented taxa and larger taxonomic groups (e.g., cyanobacteria, diatoms, and dinoflagellates) over geological timescales, their biodiversity information is limited. Only a fraction of taxa within the phytoplankton community is usually represented in the fossil record; therefore, respective data are likely biased (Bálint et al., 2018). Nevertheless, for those taxa that are suitable and sufficiently represented, highly informative demographic data can be generated from microfossil and resting stage records (Cermeño et al., 2013; Kremp et al., 2018; Matul et al., 2018). Furthermore, data on the temporal distribution of larger taxonomic groups as obtained from microfossil records can provide general information on trait composition and function of phytoplankton communities through taxonomic identity (Blank and Sánchez-Baracaldo, 2010).
2.3.2 Sedimentary ancient DNA
To capture biodiversity dynamics of phytoplankton through time, recent advances in sedimentary ancient DNA approaches can increase taxonomic coverage and resolution. DNA can be preserved for thousands or even millions of years in natural sedimentary archives, such as limnic sediments (Capo et al., 2021; Clarke et al., 2019), marine sediments (Armbrecht et al., 2022; Coolen et al., 2009, 2013), paleosols (Frindte et al., 2020; Semenov et al., 2020), and permafrost (Kjær et al., 2022; Willerslev et al., 2003). Compared to microfossils, a distinctive characteristic of ancient DNA data lies in their capability for broad taxonomic coverage. Because every organism contains DNA and the differences between species are defined by their DNA, in theory DNA could be used to identify any organism that became part of the sediment deposits (Bálint et al., 2018). Establishing relative abundances of organisms from their DNA record is challenging though. While fossilized remains of certain phytoplankton taxa can inform us about cell counts, DNA records can be informative about copy numbers of particular genes (Mejbel et al., 2021). Since gene copy numbers can vary by orders of magnitude across species, inferences about abundance can be challenging with methods that target many taxa at once (Vasselon et al., 2018). If the focus is on a narrow set of taxa, gene copy number information provided by quantitative analyses (quantitative polymerase chain reaction (qPCR) or droplet digital polymerase chain reaction (ddPCR)) might be more readily translated into abundance information, especially if the range of gene copy numbers per cell can be estimated for the focal species (Godhe et al., 2008). This approach potentially allows one to obtain demographic information on a targeted taxon in specific sediment horizons.
2.3.3 Resurrectable dormant resting stages
Living sediment archives are formed by temporal deposits of dormant resting stages, which can be obtained from phytoplankton that produce long-lived resting cells/seeds (Härnström et al., 2011; Hinners et al., 2017). Such archives are also represented in organisms such as marine microbes (Lomstein et al., 2012; Wörmer et al., 2019), terrestrial plants (McGraw et al., 1991; Sallon et al., 2008), and zooplankton (Kerfoot and Weider, 2004; Pauwels et al., 2010).
Laminated sediments, which form under anoxic conditions due to the absence of mixing by benthic fauna, contain distinct age cohorts of dormant or quiescent phytoplankton (Ellegaard et al., 2017). Such resting stages can germinate when exposed to oxygen, and cells start growing when suitable temperature, light, and nutrient conditions are provided. A number of studies have demonstrated the “resurrection” potential of different phytoplankton taxa after extended periods of resting, ranging from decades to millennia (Bolius et al., 2025; Härnström et al., 2011; Medwed et al., 2024; Sanyal et al., 2022).
Phytoplankton strains that have been re-established from germinated resting stages of different temporal sediment layers can be characterized phenotypically and genotypically (Härnström et al., 2011; Hinners et al., 2017; Medwed et al., 2024). Comparison of trait values among temporal cohorts provides information on trait changes, their rates of change, and the mechanisms behind those changes (Hattich et al., 2024). The general adaptive potential of phytoplankton has already been documented in resurrection experiments focusing on different phytoplankton functional traits, such as temperature-dependent growth and nutrient uptake (Hattich et al., 2024), resting stage formation (Hinners et al., 2017), and toxicity (Wood et al., 2021). The structure of ecosystem models is often based on such phytoplankton functional traits, which describe the dependence of phytoplankton organisms on external factors (e.g., reaction norms), their life cycle (e.g., life cycle transitions), or interactions with other organisms (e.g., cell size, toxicity). To realistically assess the extent of adaptations to environmental changes and to use this information for future ecosystem models, it is therefore important to collect comprehensive information on changes in phytoplankton functional traits using resurrection experiments.
Phenotypic trait data from resurrected cultures can also be linked to their underlying genetic components. A common method for this is represented by genome-wide association studies (GWASs) (Hirschhorn and Daly, 2005; Uffelmann et al., 2021; Visscher et al., 2017). GWASs connect variations in the DNA sequence, known as single-nucleotide polymorphisms (SNPs), to a specific trait. To do so, it is necessary to validate the candidate loci identified in GWASs by experiments that target the phenotypic functionality of these loci (Pfenninger, 2024). GWAS approaches can help to determine if certain functional groups of genes (e.g., those involved in oxidation or CO2 fixation) were selected for or lost over time. In addition, GWAS approaches can help to determine whether the traits of interest are polygenic and can thus be adequately modeled as continuous quantitative traits. The success of this method depends on several factors, including the quality of the phenotypic data and the accuracy of the genetic data. Moreover, when co-analyzing compositional DNA data and quantitative phenotypic data, careful transformation, standardization, and the use of specialized statistical methods are essential to avoid misleading conclusions (Gloor et al., 2017).
Ecosystem models provide a powerful tool to study the functioning of marine ecosystems and their responses to environmental change. For example, ecosystem models can be used to understand global patterns of phytoplankton diversity (Dutkiewicz et al., 2020; Ward et al., 2012). In addition, they can help to identify potential feedback loops (e.g., between cyanobacteria and their environment (Hense, 2007) and trade-offs (e.g., between phytoplankton diversity and productivity, Smith et al., 2016). Finally, ecosystem models can simulate how phytoplankton (and zooplankton) respond to different biotic and abiotic factors, including viruses (Krishna et al., 2024; Weitz et al., 2015), eutrophication (Gustafsson et al., 2012), ocean acidification (Dutkiewicz et al., 2015), and temperature changes (Elliott et al., 2005; Lee et al., 2018).
3.1 The neglected role of evolutionary adaptation in ecosystem models
Over the past few years, ecosystem models have been increasingly used to estimate the impact of global warming on marine ecosystems and their functioning. Although the results of such studies are relevant for stakeholders (Intergovernmental Panel on Climate Change (IPCC), 2023), current models vary widely in their formulations and predictions, with some models even disagreeing on the direction of change (Laufkötter et al., 2015, 2016; Tittensor et al., 2021). We argue that a major uncertainty in current models is that they do not account for the high adaptive potential of phytoplankton.
Experiments and observations have demonstrated that phytoplankton adaptation can be important on multi-year timescales (Aranguren-Gassis et al., 2019; Hattich et al., 2024; O'Donnell et al., 2018) and may hence alter predicted ecosystem changes notably (Ward et al., 2019). Indeed, a recent modeling study revealed that adaptation can significantly reduce simulated warming-related changes in phytoplankton phenology and relative taxa abundance (Hochfeld and Hinners, 2024a). Changes in phenology and relative taxa abundance, in turn, may have a direct impact on ecosystem functioning (Edwards and Richardson, 2004; Hochfeld and Hinners, 2024b; Litchman et al., 2015). To conclude, it is becoming increasingly clear that adaptation cannot be neglected in global warming simulations, calling current models and their predictive ability into question.
Evolutionary adaptation can be integrated into ecosystem models by allowing for one or more phytoplankton traits to change on intergenerational timescales. In the case of changing temperature, for example, phytoplankton thermal adaptation can be represented with an evolvable optimum temperature for growth (Beckmann et al., 2019; Kremer and Klausmeier, 2017). Different approaches exist to integrate adaptation into ecosystem models, with the most suitable approach depending on the research question; see Sect. 3.2 for details. Overviews can also be found in Beckmann et al. (2019) and Klausmeier et al. (2020b). However, integrating adaptation into ecosystem models brings new challenges, such as identifying the relevant traits and the associated limits and trade-offs (O'Donnell et al., 2018; Ward et al., 2019). One approach to obtain the necessary evolutionary information is represented by evolution experiments, in which populations are kept under controlled environmental conditions for long periods of time (weeks, months, or even years) in order to measure their evolutionary adaptation to these environmental conditions (Hinners et al., 2024; O'Donnell et al., 2018; Schaum et al., 2017). Since such experiments can neither replicate the complexity of real ecosystems nor long-term environmental change, we argue that sediment archives as “natural evolution experiments” represent a valuable complementary source of information, which we explain in Sect. 3.3.
3.2 Evolution in ecosystem models
Different approaches exist to integrate evolutionary processes into ecosystem models. The approaches differ in their biological complexity and their computational efficiency, which makes them suitable for different applications (see Table 1). Thus, the mutational algorithm should be chosen depending on the research question.
Individual-based models (IBMs) provide the most complex and most realistic representation of evolutionary adaptation. IBMs simulate individual cells with their individual phenotypic trait values (Beckmann et al., 2019; Clark et al., 2011; Collins, 2016); a prominent approach was developed by Beckmann et al. (2019), who assume that individual cells take up nutrients, grow, divide, and die. Evolution is implemented through random mutations, which occur every several hundred cell divisions (Lenski and Travisano, 1994). The new trait value of the mutated daughter cell is sampled randomly from a normal distribution, which is centered at the parental trait value with a prescribed standard deviation. Thus, small mutations are much more likely than large mutations, while beneficial and deteriorating mutations are equally likely. With natural selection acting over time, the cells with the highest fitness that need the lowest time to divide again become dominant. Maladapted cells, on the contrary, die off eventually if their doubling rate is lower than stochastic losses like mortality and grazing, leading to progressive adaptation of the entire population.
Simulating natural populations with millions of individuals requires a lot of computational power. To reduce computational power demands, identical cells are often grouped into one model variable (Hellweger and Bucci, 2009), a so-called super-individual or agent. Due to the larger number of cells combined into one agent, mutations are assumed to occur more frequently but with a smaller step size (i.e., standard deviation) (Beckmann et al., 2019). Even though agent-based models require less computational power than IBMs, they are usually only integrated into zero-dimensional model environments.
A computationally much more efficient approach is provided by continuous trait-diffusion models (Chen and Smith, 2018; Le Gland et al., 2021; Smith et al., 2016), which do not resolve individual phenotypes as discrete entities. Instead, they compute the mean and variance of a trait for the entire phytoplankton compartment (i.e., phytoplankton population). Trait variance represents the diversity of ecotypes, with mutations adding new variance that can be selected. Selection, in this context, means that the mean trait increases when higher trait values are associated with higher net growth rates. Due to their low computational power requirements, continuous trait-diffusion models can be integrated into 1D or 3D model environments. Their simplistic representation of evolution, however, comes at a price: While the shape of the trait distribution must be prescribed, trade-offs between traits are difficult to implement, and evolutionary branching cannot be represented.
Multi-compartment trait-diffusion models can be described as the discretized version of continuous trait-diffusion models. The phytoplankton compartment is divided into multiple sub-compartments that differ slightly in one or more trait values (Hinners et al., 2019; Kremer and Klausmeier, 2013; Sauterey et al., 2017). Mutations are implemented as small, non-random fluxes of biomass between the sub-compartments. This approach is of intermediate biological complexity, which is also reflected in computational power demands. Multi-compartment trait-diffusion models require more power than continuous trait-diffusion models but less than IBMs or agent-based models and can hence be integrated into 1D model environments.
3.3 Building an evolutionary ecosystem model including data from sediment archives
It is a considerable challenge to develop ecosystem models that can be applied equally well to past, present, and future scenarios. Most state-of-the-art ecosystem models are developed in a two-step process that comprises the definition of prior estimates of parameter values (initialization) and the iterative fit to contemporary observations through parameter adjustment (calibration). We argue that this approach relies too heavily on how an ecosystem is structured in the present, so that models may no longer be applicable when ecosystem structure has changed in the future. To avoid these problems, models should represent fundamental processes that apply more generally instead of being tailored to a specific ecosystem. The general applicability of a model can be tested with an additional step during model development, validation, which makes use of data from sediment archives. While validation is already common for atmosphere and ocean models (Hollingsworth, 1994; Tonani et al., 2015), it has been largely ignored by the ecosystem modeling community. A recent study presented a validation approach for non-evolutionary terrestrial ecosystem models, which is mainly based on plant remains (Alsos et al., 2024). Our approach focuses on phytoplankton, key players in marine ecosystems and respective models. Due to the high evolutionary potential of phytoplankton, we additionally consider evolutionary processes.
The development approach for evolutionary ecosystem models that we propose here comprises three different steps: initialization, calibration, and validation (Fig. 2). Both initialization and calibration are performed using contemporary data, while validation requires data from sediment archives. Only when all three steps have been completed should a model be used to simulate future ecosystem changes.
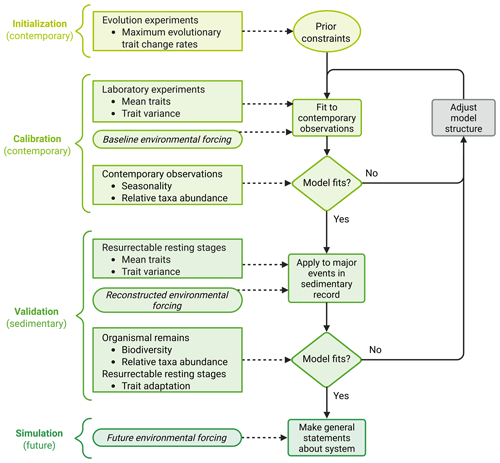
Figure 2Conceptual framework for the development of an evolutionary ecosystem model that can be applied equally well to past, present, and future scenarios. Shown are the three different steps of model development (initialization, calibration, validation), the following application of the model (simulation), and the data required for each step. The figure was created with BioRender.com.
Initialization requires prior estimates of parameter values that need to be valid regardless of the simulated environmental scenario. Such parameters include constraints on adaptation, such as maximum evolutionary trait change rates, which are, however, difficult to assess. For example, evolutionary trait change rates can be assessed by comparing ancestral trait values with those of populations that have evolved in a new environment for a specific time after accounting for plastic responses (Collins and Bell, 2004; Hutchins et al., 2015; Listmann et al., 2016). In addition, it is possible to measure changes in fitness proxies, most commonly population growth rate or lineage competitive ability (Elena and Lenski, 2003). However, interpretation is not straightforward since the relationship between fitness and its proxies may change over time (Collins et al., 2020). Finally, genetic mutation rates can be estimated via genome sequencing (e.g., Krasovec et al., 2019), but genetic mutation does not necessarily translate into trait changes. While functional traits may depend on multiple genes (epistasis), one gene may affect multiple traits (pleiotropy) (Lässig et al., 2017; Østman et al., 2012; Tyler et al., 2009). To conclude, evolutionary trait change rates can only be assessed roughly and require further adjustment in the next steps of model development.
The goal of model calibration is to fine tune the model parameters and the model structure until the model reproduces contemporary observations. To do so, initial values for mean traits and trait variance are required (Fig. 2). These parameters can be measured in the laboratory for recently sampled organisms (Lehtimäki et al., 1997; Vincent and Silvester, 1979). The model is then forced with a baseline environmental forcing, usually a steady seasonal forcing that represents present-day conditions. Using this forcing, the model is run until it reaches a steady state, where phenology and taxa abundances repeat each season. Simulated phenology and taxa abundances are then compared to contemporary observations from seagoing research and remote sensing. If the model does not reproduce the observations, the model parameters and structure are adjusted iteratively until the model output and the observations match. When adjusting parameter values, care must be taken to keep all values within a realistic range, especially when such a range can be constrained by measurements, e.g., for traits like half-saturation constants and maximum growth rates (Eppley et al., 1969; Hinners et al., 2017). When adjusting the model structure, on the other hand, it should be reconsidered whether the most relevant processes (e.g., life cycle transitions, mortality, ecological interactions such as grazing) are included and described realistically.
As the final step, model validation aims to test if the model is equally applicable to past, present, and (by implication) future scenarios by comparing the model output to independent validation data. We argue that data from sediment archives are ideally suited for validation, with a contemporarily calibrated model being successfully validated if it can represent major shifts in community structure and/or function that are present in the sediment record. As a first step, validation needs initial values for the mean and variance of the relevant traits. These parameters can be measured in the laboratory for resurrected organisms sampled from the sediment layer that corresponds to the beginning of the validation period. In addition, environmental conditions during the validation period must be reconstructed to create a forcing for the model. Extreme climatic periods such as the Pliocene (5.3–2.6 million years ago), when the global mean surface temperature was ∼2 °C higher than today, with more than +10 °C at high latitudes (Ballantyne et al., 2010; Haywood et al., 2000; Salzmann et al., 2008), or the last interglacial period (128 000–116 000 years ago; Muhs, 2002; Stirling et al., 1998), when Arctic temperatures were up to 5 °C higher than today (North Greenland Ice Core Project members, 2004), would be ideal to test the model's validity in extreme and changing climates. The simulated biodiversity and relative taxa abundances can then be compared to organismal remains from different sediment layers throughout the validation period. Similarly, simulated trait changes can be compared to results from resurrection experiments, which are performed with organisms from different sediment layers of the validation period. If the contemporarily calibrated model cannot reproduce major events in the sediment record, this implies that the model's structure and parameterization are not general to both contemporary and past systems and should therefore not be used to make predictions.
For example, Gibbs et al. (2020) used an evolutionary ecosystem model that was parameterized in accordance with contemporary laboratory measurements to reproduce an observed shift in the trophic status of coccolithophores after the end-Cretaceous mass extinction. However, while the model produced an evolutionary response that was qualitatively consistent with the sediment record, the simulated evolutionary response progressed at a rate that was orders of magnitude too fast. This indicates that the model would require further adjustments to allow for the reproduction of both contemporary and sedimentary data before the model could be used predictively.
While such a model could be recalibrated to fit the past data, we do not recommend this approach, because the ad hoc adjustment of the model parameters does not fix the underlying problem. In addition, calibration is not possible when making predictions. Therefore, instead of recalibrating the model to past data, we advocate for refining the model structure to better represent processes that do apply generally, across past, present, and future systems. After recalibration to contemporary data, the refined model could be tested again against past data. Repeating this process iteratively until both contemporary and past data can be reproduced with the same model assures that the model can provide meaningful statements about an ecosystem's possible response to future climate changes.
To estimate remaining model uncertainties, the uncertainties in parameter values and environmental forcing can be used for sensitivity experiments. The extent to which the model structure influences the results can be assessed by comparing the model results before and after the structural change.
3.4 Exemplary implementation
Below, we describe an exemplary implementation of our approach using the following research question: how did and will the phytoplankton community in the Baltic Sea change during the past and future 100 years, and what role does thermal adaptation play in these changes?
For our exemplary implementation, we assume that sediment cores have already been collected and analyzed. Thus, it is known which temperature-dependent traits have changed over the past 100 years when our approach is implemented. In this example, we assume that an adaptive response in the optimum temperature is evident in the sediment record, as reported in, for example, Hattich et al. (2024).
-
Ecosystem model setup and initialization. As a first step, the evolutionary ecosystem model structure is built. The model structure mathematically describes the dynamics and interactions between the components of the Baltic Sea ecosystem that are considered relevant for answering the research question. In this case, the model structure includes equations for nutrients, the most relevant phytoplankton functional groups in the Baltic Sea (dinoflagellates, diatoms, and cyanobacteria), and dead organic matter (detritus). For each phytoplankton functional group, a growth equation is formulated, including a limitation function for nutrients. In addition, functions for life cycle transitions (e.g., following Hochfeld and Hinners, 2024a) and mortality are implemented. The respective formulations and parameters (i.e., half- saturation constants, basic transition rates in life cycle transition functions, basic mortality rates in mortality functions) are adopted from the literature (e.g., Eppley et al., 1969; Hense and Beckmann, 2006; Hinners et al., 2019). A one-dimensional water column model is used for the simulations (e.g., GOTM; Li et al., 2021; Umlauf et al., 2005), and evolution is enabled using a multi-compartment model (e.g., Hinners et al., 2019). The evolutionary algorithm is initialized with experimentally derived evolutionary trait change rates for the optimum temperature, which can be obtained from the literature (e.g., Jin and Agustí, 2018; Listmann et al., 2016).
-
Model calibration. For calibration, the model needs to be complemented by temperature limitation functions for the simulated taxa. These temperature limitation functions are established from the thermal reaction norms of resurrected resting stages from the uppermost sediment layer, including the mean and the variance of the optimum temperature. In addition, a baseline temperature forcing is created to represent recent annual temperature fluctuations in the Baltic Sea, e.g., by using temperature data from the Copernicus database (https://marine.copernicus.eu/access-data, last access: 25 April 2025). The model is then run with the baseline forcing until a steady state is reached, where the simulated dynamics repeat each season. Finally, the model output is compared to existing, recent data on the relative abundances and seasonal dynamics of the simulated phytoplankton taxa (e.g., Hjerne et al., 2019). If the model does not reproduce the data, both the model structure (i.e., the functions for nutrient limitation, life cycle transitions, and mortality) and the corresponding parameters initialized in step 1 are adjusted until the model output fits the data.
-
Model validation. For validation, the model is initialized with mean optimum temperatures and variances from 100 years ago. For this purpose, data from resurrection experiments with resting stages from the corresponding historic sediment layer are used (e.g., Hattich et al., 2024). A hindcast temperature forcing for the last 100 years is reconstructed from the sedimentary record using biotic proxies such as microfossils and biomarkers (e.g., Kabel et al., 2012; Wittenborn et al., 2022). After applying the hindcast forcing to the model, model performance is evaluated based on a biological sediment assessment of changes in relative taxa abundance (using genetic analyses and the assessment of resting stages across the sediment, e.g., Kremp et al., 2018; Schmidt et al., 2024) and changes in the optimum temperatures of these taxa (using resurrection experiments, e.g., Hattich et al., 2024). If the model is not able to reproduce changes in relative taxa abundances and past adaptation of the optimum temperature, its structure needs to be refined. For example, the simulated evolutionary response may be less pronounced than the one derived from the sedimentary record. This issue could be fixed by increasing the evolutionary trait change rates until the model reproduces the observed evolutionary changes, which may result in unrealistically high evolutionary trait change rates. Instead of simply adjusting parameters, it may therefore be necessary to consider other hitherto disregarded processes that may exert an additional selection pressure on the focal taxa, for example, a temperature-dependent cyst mortality (Hinners et al., 2019). If the model reproduces past adaptive changes and relative taxa abundances with the new cyst mortality function, model performance is tested again for the baseline environmental forcing. If the revised model still reproduces the calibration data, it is equally applicable to the past 100 years and the present and can therefore be used for the intended simulations of future climate change.
Our approach has the potential to increase the informative value of model projections of marine ecosystem change. However, there are still some challenges associated with it.
A major challenge is posed by the low temporal resolution of sediment records, which can range from multi-centennial to annual depending on the sedimentation rate (Abrantes et al., 2005; Maslin et al., 2003). Thus, phenological information is missing even in high-resolution records, meaning that simulated phenology cannot be validated using data from sediment archives. Instead, simulated phenology can be validated using monitoring data, which may go back several decades (Wasmund et al., 2019).
Assuming that evolutionary change in phytoplankton can occur on a decadal timescale (Irwin et al., 2015), sediments that accumulate at similar or even higher rates, which is usually the case in lakes and marginal seas, should allow for the detection of evolutionary change (based on resurrection experiments or genetic analyses). At sites with lower sedimentation rates, rates of evolutionary change may be underestimated. It is therefore recommended to work in environments with high sedimentation rates (e.g., ≥1 mm yr−1) to investigate evolutionary changes. In addition, the age model uncertainty, which increases with the age of the sediments, can lead to inaccuracies in the determined rates of evolutionary change. Depending on the depositional environment, the preservation rates (decomposition rates) of organic matter vary greatly (Canfield, 1994; Hedges and Keil, 1995; Wakeham and Canuel, 2006). Preservation is lowest in oxic environments with low sedimentation rates and highest in anoxic environments with high sedimentation rates (Canfield, 1994). Differential preservation may also bias estimates of relative abundance, although structurally similar organic compounds are expected to be similarly preserved (Wakeham and Canuel, 2006). To determine possible effects of preservation on the estimates of (relative) abundance and evolutionary changes, it is important to estimate the content of total organic carbon in the sediment. For the investigation of evolutionary changes, it is therefore recommended to work in environments with high sedimentation rates, low age model uncertainty, and hypoxic to anoxic conditions. Among others, the Baltic Sea, the Black Sea, the Cariaco Basin, and many lakes represent such suitable environments.
Dormant resting stages that have been preserved in the sediment record and could be revived for experiments may not be representative of the entire population at the time of deposition, and therefore they may not be representative of its traits. However, assuming that the fittest individuals of a population were most abundant in the past and hence are most abundant in the sediment, we should be able to measure representative mean trait values for the population. Nevertheless, we cannot rule out that storage in the sediment may have distorted the measurable characteristics of a population. To obtain reliable estimates of trait variance, experimental studies on phytoplankton traits should therefore aim to characterize as many strains as possible, e.g., using high-throughput methods (Argyle et al., 2021). Proxies for the reconstruction of environmental conditions and DNA can also suffer from preservation/degradation biases and are therefore not independent from each other (Dommain et al., 2020; Mitchell et al., 2005; Wakeham and Canuel, 2006; Zonneveld et al., 2010).
Evolutionary models require knowledge of how rapidly and how far the aforementioned traits can change from generation to generation, as well as of the trade-offs between traits (Levins, 1962; Litchman et al., 2007) and the ultimate constraints on adaptation (Klausmeier et al., 2020a). Such information is available from evolution experiments (Hinners et al., 2024), but it is still unclear how applicable such information will be when moving from a highly simplified evolution experiment to a more complex community context. A major challenge is to link trait changes to changes in fitness. While the relationship between a fitness proxy and actual fitness may change over time (Collins et al., 2020), fitness is largely determined by species interactions (Schabhüttl et al., 2013). Based on the assumption that the fittest phytoplankton taxa are also the most abundant in the sediment, sediment archives make it possible to estimate the relative fitness of different taxa. However, the validity of this assumption might be challenged by ecological and evolutionary complexities, as well as possible preservation differences between taxa. Preservation differences might be evaluated by synthesizing information from different proxies for abundance (e.g., biomarkers and sedimentary ancient DNA). Knowledge on species' biology and ecology should be informative about the confounding effects of ecological and evolutionary complexities, such as demographic trade-offs, differential responses to environmental fluctuations (Melbinger and Vergassola, 2015), and temporal trade-offs (Betini et al., 2017). Consequently, the validity of the assumption that fitness equals abundance should be tested on a case-by-case basis.
Despite limitations and knowledge gaps, sediment archives represent a valuable source of information that has the potential to advance ecosystem model development and hence model projections of marine ecosystem change. As pointed out above, a crucial step in ecosystem model development is to make sure that models are equally applicable to past, present, and future scenarios before using them predictively. This requires validation data that are independent of the data used for calibration. Moreover, validation data need to cover the complexity of marine ecosystems and long-term environmental changes over hundreds to thousands of years. While data from laboratory, mesocosm, or marine monitoring studies only partly fulfill these criteria, sediment archives fulfill all of them. Furthermore, the approach presented here is not limited to phytoplankton but can be applied to other organisms that are well represented in the sediment record, such as marine microbes (Wörmer et al., 2019), zooplankton (Isanta-Navarro et al., 2021; Wersebe and Weider, 2023), viruses (Coolen, 2011), and terrestrial plants (Alsos et al., 2024). Depending on the group of organisms chosen, their applicability to evolution experiments, and their potential to survive in the sediment or to be identified by microfossils or DNA, our approach may require adjustment.
Marine communities perform functions that are essential for the environment and for humanity. However, it is largely unknown how these functions will change under global warming, and the possibility of positive feedbacks, irreversible tipping points, and ecosystem collapse must be considered. It is therefore crucial to develop tools that provide reliable estimates of future changes in marine ecosystems.
Ecosystem models represent a promising tool for predicting marine ecosystem change, but their current projections are largely inconsistent. Here, we present a promising approach that can increase the informative value of ecosystem model projections. We argue that a major uncertainty in current ecosystem models is that they largely ignore evolutionary processes, which can be highly relevant on perennial timescales. In addition, current ecosystem models are typically calibrated to contemporary data and then used for projections without validating that they are equally applicable to past (and, by implication, future) scenarios. We suggest not only to calibrate evolutionary ecosystem models against contemporary observations, but also to validate the calibrated models against major evolutionary ecosystem changes that are present in the sediment record. Contrary to data from conventional experiments and marine monitoring, sediment records make it possible to map both the complexity of real ecosystems and long-term environmental changes. Only if a contemporarily calibrated evolutionary ecosystem model can reproduce observations from the sediment record can we have some confidence in its projections of future ecosystem change.
Some challenges remain, especially regarding the low temporal resolution of sediment archives and potential biases due to preservation differences. Nevertheless, data from sediment archives provide a unique opportunity to learn from the past and hence have the potential to take ecosystem models and their projections of future ecosystem change a crucial step forward. The approach presented here is not limited to phytoplankton but can be applied to other organisms and ecosystems.
No data sets were used in this article.
IH: conceptualization; project administration; visualization; writing (original draft); writing (review and editing). BAW: conceptualization; writing (original draft); writing (review and editing). AK: conceptualization; writing (original draft); writing (review and editing). JR: conceptualization; visualization; writing (original draft); writing (review and editing). AS: conceptualization; visualization; writing (original draft); writing (review and editing). MB: conceptualization; writing (original draft); writing (review and editing). LB: conceptualization; writing (original draft); writing (review and editing). JK: conceptualization; writing (original draft); writing (review and editing). HWA: conceptualization; writing (review and editing). SB: conceptualization; writing (review and editing). LSE: conceptualization; writing (review and editing). MP: conceptualization; writing (review and editing). CAK: conceptualization; writing (review and editing). EL: conceptualization; writing (review and editing). JH: conceptualization; project administration; writing (original draft); writing (review and editing).
The contact author has declared that none of the authors has any competing interests.
Publisher’s note: Copernicus Publications remains neutral with regard to jurisdictional claims made in the text, published maps, institutional affiliations, or any other geographical representation in this paper. While Copernicus Publications makes every effort to include appropriate place names, the final responsibility lies with the authors.
We would like to thank Sebastian Naeher, Damien Eveillard, and one anonymous reviewer for their constructive feedback on our manuscript.
This research has been supported by the Leibniz-Institut für Ostseeforschung Warnemünde (grant no. K314/2020), a Royal Society University Research Fellowship, the US National Science Foundation (grant nos. 17-54250 and 21-24800), and the Hessisches Ministerium für Wissenschaft und Kunst (grant no. LOEWE/1/10/519/03/03.001(0014)/52).
This paper was edited by Sebastian Naeher and reviewed by Damien Eveillard and one anonymous referee.
Abrantes, F., Lebreiro, S., Rodrigues, T., Gil, I., Bartels-Jónsdóttir, H., Oliveira, P., Kissel, C., and Grimalt, J. O.: Shallow-marine sediment cores record climate variability and earthquake activity off Lisbon (Portugal) for the last 2000 years, Quaternary Sci. Rev., 24, 2477–2494, https://doi.org/10.1016/j.quascirev.2004.04.009, 2005.
Alsos, I. G., Rijal, D. P., Ehrich, D., Karger, D. N., Yoccoz N. G., Heintzman P. D., Brown, A. G., Lammers, Y., Pellissier, L., Alm, T., Bråthen, K. A., Coissac, E., Merkel, M. K. F., Alberti, A., Denoeud, F., Bakke, J., and PhyloNorway Consortium: Postglacial species arrival and diversity buildup of northern ecosystems took millennia, Sci. Adv., 8, eabo7434, https://doi.org/10.1126/sciadv.abo7434, 2022.
Alsos, I. G., Boussange, V., Rijal, D. P., Beaulieu, M., Brown, A. G., Herzschuh, U., Svenning, J.-C., and Pellissier, L.: Using ancient sedimentary DNA to forecast ecosystem trajectories under climate change, Philos. T. R. Soc. B, 379, 20230017, https://doi.org/10.1098/rstb.2023.0017, 2024.
Appleby, P. G.: Chronostratigraphic Techniques in Recent Sediments, in: Tracking Environmental Change Using Lake Sediments, Volume 1: Basin Analysis, Coring, and Chronological Techniques, edited by: Last, W. M. and Smol, J. P., Springer, Dordrecht, 171–203, https://doi.org/10.1007/0-306-47669-X_9, 2001.
Aranguren-Gassis, M., Kremer, C. T., Klausmeier, C. A., and Litchman, E.: Nitrogen limitation inhibits marine diatom adaptation to high temperatures, Ecol. Lett., 22, 1860–1869, https://doi.org/10.1111/ele.13378, 2019.
Argyle, P. A., Hinners, J., Walworth, N. G., Collins, S., Levine, N. M., and Doblin, M. A.: A High-Throughput Assay for Quantifying Phenotypic Traits of Microalgae, Front. Microbiol., 2910, 706235, https://doi.org/10.3389/fmicb.2021.706235, 2021.
Armbrecht, L., Weber, M. E., Raymo, M. E., Peck, V. L., Williams, T., Warnock, J., Kato, Y., Hernández-Almeida, I., Hoem, F., Reilly, B., Hemming, S., Bailey, I., Martos, Y. M. , Gutjahr, M., Percuoco, V., Allen, C., Brachfeld, S., Cardillo, F. G., Du, Z., Fauth, G., Fogwill, C., Garcia, M., Glüder, A., Guitard, M., Hwang, J.-H., Iizuka, M., Kenlee, B., O’Connell, S., Pérez, L. F., Ronge, T. A., Seki, O., Tauxe, L., Tripathi, S., and Zheng, X.: Ancient marine sediment DNA reveals diatom transition in Antarctica, Nat. Commun., 13, 5787, https://doi.org/10.1038/s41467-022-33494-4, 2022.
Bálint, M., Pfenninger, M., Grossart, H.-P., Taberlet, P., Vellend, M., Leibold, M. A., Englund, G., and Bowler, D.: Environmental DNA time series in ecology, Trends Ecol. Evol., 33, 945–957, https://doi.org/10.1016/j.tree.2018.09.003, 2018.
Ballantyne, A. P., Greenwood, D., Sinninghe Damsté, J., Csank, A., Eberle, J., and Rybczynski, N.: Significantly warmer Arctic surface temperatures during the Pliocene indicated by multiple independent proxies, Geology, 38, 603–606, https://doi.org/10.1130/G30815.1, 2010.
Beckmann, A., Schaum, C.-E., and Hense, I.: Phytoplankton adaptation in ecosystem models, J. Theor. Biol., 468, 60–71, https://doi.org/10.1016/j.jtbi.2019.01.041, 2019.
Bennington, C. C., McGraw, J. B., and Vavrek, M. C.: Ecological Genetic Variation in Seed Banks. II. Phenotypic and Genetic Differences Between Young and Old Subpopulations of Luzula Parviflora, J. Ecol., 79, 627–643, https://doi.org/10.2307/2260658, 1991.
Betini, G. S., McAdam, A. G., Griswold, C. K., and Norris, D. R.: A fitness trade-off between seasons causes multigenerational cycles in phenotype and population size, Elife, 6, e1877, https://doi.org/10.7554/eLife.18770, 2017.
Blank, C. and Sánchez-Baracaldo, P.: Timing of morphological and ecological innovations in the cyanobacteria–a key to understanding the rise in atmospheric oxygen, Geobiology, 8, 1–23, https://doi.org/10.1111/j.1472-4669.2009.00220.x, 2010.
Bolius, S., Schmidt, A., Kaiser, J., Arz, H. W., Dellwig, O., Karsten, U., Epp, L. S., and Kremp, A.: Resurrection of a diatom after 7000 years from anoxic Baltic Sea sediment, ISME J., 19, wrae252, https://doi.org/10.1093/ismejo/wrae252, 2025.
Bradley, R. S.: Paleoclimatology: Reconstructing Climates of the Quaternary, Academic Press, 2nd Edition, 613 pp., ISBN-13 978-0-12-124010-3, 1999.
Brumsack, H.-J.: The trace metal content of recent organic carbon-rich sediments: implications for Cretaceous black shale formation, Palaeogeogr. Palaeocl., 232, 344–361, https://doi.org/10.1016/j.palaeo.2005.05.011, 2006.
Canfield, D. E.: Factors influencing organic carbon preservation in marine sediments, Chem. Geol., 114, 315–329, https://doi.org/10.1016/0009-2541(94)90061-2, 1994.
Capo, E., Giguet-Covex, C., Rouillard, A., Nota, K., Heintzman, P. D., Vuillemin, A., Ariztegui, D., Arnaud, F., Belle, S., Bertilsson, S., Bigler, C., Bindler, R., Brown, A. G., Clarke, C. L., Crump, S. E., Debroas, D., Englund, G., Ficetola, G. F., Garner, R. E., Gauthier, J., Gregory-Eaves, I., Heinecke, L., Herzschuh, U., Ibrahim, A., Kisand, V., Kjær, K. H., Lammers, Y., Littlefair, J., Messager, E., Monchamp, M.-E., Olajos, F., Orsi, W., Pedersen, M. W., Rijal, D. P., Rydberg, J., Spanbauer, T., Stoof-Leichsenring, K. R., Taberlet, P., Talas, L., Thomas, C., Walsh, D. A., Wang, Y., Willerslev, E., van Woerkom, A., Zimmermann, H. H., Coolen, M. J. L., Epp, L. S., Domaizon, I., Alsos, I. G., and Parducci, L.: Lake sedimentary DNA research on past terrestrial and aquatic biodiversity: Overview and recommendations, Quaternary, 4, 6, https://doi.org/10.3390/quat4010006, 2021.
Cermeño, P., Marañón, E., and Romero, O. E.: Response of marine diatom communities to Late Quaternary abrupt climate changes, J. Plankton Res., 35, 12–21, https://doi.org/10.1093/plankt/fbs073, 2013.
Chen, B. and Smith, S. L.: CITRATE 1.0: Phytoplankton continuous trait-distribution model with one-dimensional physical transport applied to the North Pacific, Geosci. Model Dev., 11, 467–495, https://doi.org/10.5194/gmd-11-467-2018, 2018.
Clark, J. R., Daines, S. J., Lenton, T. M., Watson, A. J., and Williams, H. T.: Individual-based modelling of adaptation in marine microbial populations using genetically defined physiological parameters, Ecol. Modell., 222, 3823–3837, https://doi.org/10.1016/j.ecolmodel.2011.10.001, 2011.
Clarke, C. L., Edwards, M. E., Brown, A. G., Gielly, L., Lammers, Y., Heintzman, P. D., Ancin-Murguzur, F. J., Bråthen, K.-A., Goslar, T., and Alsos, I. G.: Holocene floristic diversity and richness in northeast Norway revealed by sedimentary ancient DNA (seda DNA) and pollen, Boreas, 48, 299–316, https://doi.org/10.1111/bor.12357, 2019.
Cléroux, C., Cortijo, E., Anand, P., Labeyrie, L., Bassinot, F., Caillon, N., and Duplessy, J.-C.: Mg Ca and Sr Ca ratios in planktonic foraminifera: Proxies for upper water column temperature reconstruction, Paleoceanography, 23, PA3214, https://doi.org/10.1029/2007PA001505, 2008.
Collins, S.: Growth rate evolution in improved environments under Prodigal Son dynamics, Evol. Appl., 9, 1179–1188, https://doi.org/10.1111/eva.12403, 2016.
Collins, S. and Bell, G.: Phenotypic consequences of 1,000 generations of selection at elevated CO2 in a green alga, Nature, 431, 566–569, https://doi.org/10.1038/nature02945, 2004.
Collins, S., Boyd, P. W., and Doblin, M. A.: Evolution, microbes, and changing ocean conditions, Annu. Rev. Mar. Sci., 12, 181–208, https://doi.org/10.1146/annurev-marine-010318-095311, 2020.
Coolen, M. J.: 7000 years of Emiliania huxleyi viruses in the Black Sea, Science, 333, 451–452, https://doi.org/10.1126/science.1200072, 2011.
Coolen, M. J., Saenz, J. P., Giosan, L., Trowbridge, N. Y., Dimitrov, P., Dimitrov, D., and Eglinton, T. I.: DNA and lipid molecular stratigraphic records of haptophyte succession in the Black Sea during the Holocene, Earth Planet. Sci. Lett., 284, 610–621, https://doi.org/10.1016/j.epsl.2009.05.029, 2009.
Coolen, M. J., Orsi, W. D., Balkema, C., Quince, C., Harris, K., Sylva, S. P., Filipova-Marinova, M., and Giosan, L.: Evolution of the plankton paleome in the Black Sea from the Deglacial to Anthropocene, P. Natl. Acad. Sci. USA, 110, 8609–8614, https://doi.org/10.1073/pnas.1219283110, 2013.
Delebecq, G., Schmidt, S., Ehrhold, A., Latimier, M., and Siano, R.: Revival of ancient marine dinoflagellates using molecular biostimulation, J. Phycol., 56, 1077–1089, https://doi.org/10.1111/jpy.13010, 2020.
Dellwig, O., Wegwerth, A., Schnetger, B., Schulz, H., and Arz, H. W.: Dissimilar behaviors of the geochemical twins W and Mo in hypoxic-euxinic marine basins, Earth-Sci. Rev., 193, 1–23, https://doi.org/10.1016/j.earscirev.2019.03.017, 2019.
Denman, K. L.: A model simulation of the adaptive evolution through mutation of the coccolithophore Emiliania huxleyi based on a published laboratory study, Front. Mar. Sci., 3, 286, https://doi.org/10.3389/fmars.2016.00286, 2017.
Dommain, R., Andama, M., McDonough, M. M., Prado, N. A., Goldhammer, T., Potts, R., Maldonado, J. E., Nkurunungi, J. B., and Campana, M. G.: The challenges of reconstructing tropical biodiversity with sedimentary ancient DNA: A 2200-year-long metagenomic record from Bwindi impenetrable forest, Uganda, Frontiers in Ecology and Evolution, 8, 218, https://doi.org/10.3389/fevo.2020.00218, 2020.
Dutkiewicz, S., Morris, J. J., Follows, M. J., Scott, J., Levitan, O., Dyhrman, S. T., and Berman-Frank, I.: Impact of ocean acidification on the structure of future phytoplankton communities, Nature Climate Change, 5, 1002–1006, https://doi.org/10.1038/nclimate2722, 2015.
Dutkiewicz, S., Cermeno, P., Jahn, O., Follows, M. J., Hickman, A. E., Taniguchi, D. A. A., and Ward, B. A.: Dimensions of marine phytoplankton diversity, Biogeosciences, 17, 609–634, https://doi.org/10.5194/bg-17-609-2020, 2020.
Edwards, M. and Richardson, A. J.: Impact of climate change on marine pelagic phenology and trophic mismatch, Nature, 430, 881–884, https://doi.org/10.1038/nature02808, 2004.
Elena, S. F. and Lenski, R. E.: Evolution experiments with microorganisms: the dynamics and genetic bases of adaptation, Nat. Rev. Genet., 4, 457–469, https://doi.org/10.1038/nrg1088, 2003.
Ellegaard, M. and Ribeiro, S.: The long-term persistence of phytoplankton resting stages in aquatic `seed banks', Biol. Rev., 93, 166–183, https://doi.org/10.1111/brv.12338, 2018.
Ellegaard, M., Dale, B., Mertens, K. N., Pospelova, V., and Ribeiro, S.: Dinoflagellate Cysts as Proxies for Holocene Environmental Change in Estuaries: Diversity, Abundance and Morphology, in: Applications of Paleoenvironmental Techniques in Estuarine Studies, Developments in Paleoenvironmental Research, Volume 20, edited by: Weckström, K., Saunders, K., Gell, P., and Skilbeck, C., Springer, Dordrecht, 295–312, https://doi.org/10.1007/978-94-024-0990-1_12, 2017.
Ellegaard, M., Clokie, M. R., Czypionka, T., Frisch, D., Godhe, A., Kremp, A., Letarov, A., McGenity, T. J., Ribeiro, S., and John Anderson, N.: Dead or alive: sediment DNA archives as tools for tracking aquatic evolution and adaptation, Communications Biology, 3, 169, https://doi.org/10.1038/s42003-020-0899-z, 2020.
Elliott, J. A., Thackeray, S. J., Huntingford, C., and Jones, R. G.: Combining a regional climate model with a phytoplankton community model to predict future changes in phytoplankton in lakes, Freshwater Biol., 50, 1404–1411, https://doi.org/10.1111/j.1365-2427.2005.01409.x, 2005.
Eppley, R. W., Rogers, J. N., and McCarthy, J. J.: Half-saturation constants for uptake of nitrate and ammonium by marine phytoplankton, Limnol. Oceanogr., 14, 912–920, https://doi.org/10.4319/lo.1969.14.6.0912, 1969.
Fenchel, T.: Marine plankton food chains, Annu. Rev. Ecol. Syst., 19, 19–38, https://doi.org/10.1146/annurev.es.19.110188.000315, 1988.
Field, C. B., Behrenfeld, M. J., Randerson, J. T., and Falkowski, P.: Primary production of the biosphere: integrating terrestrial and oceanic components, Science, 281, 237–240, https://doi.org/10.1126/science.281.5374.237, 1998.
Frindte, K., Lehndorff, E., Vlaminck, S., Werner, K., Kehl, M., Khormali, F., and Knief, C.: Evidence for signatures of ancient microbial life in paleosols, Sci. Rep., 10, 16830, https://doi.org/10.1038/s41598-020-73938-9, 2020.
Gibbs, S. J., Bown, P. R., Ward, B. A., Alvarez, S. A., Kim, H., Archontikis, O. A., Sauterey, B., Poulton, A. J., Wilson, J., and Ridgwell, A.: Algal plankton turn to hunting to survive and recover from end-Cretaceous impact darkness, Sci. Adv., 6, eabc9123, https://doi.org/10.1126/sciadv.abc9123, 2020.
Gloor, G. B., Macklaim, J. M., Pawlowsky-Glahn, V., and Egozcue, J. J.: Microbiome datasets are compositional: and this is not optional, Front. Microbiol., 8, 2224, https://doi.org/10.3389/fmicb.2017.02224, 2017.
Godhe, A., Asplund, M. E., Härnström, K., Saravanan, V., Tyagi, A., and Karunasagar, I.: Quantification of diatom and dinoflagellate biomasses in coastal marine seawater samples by real-time PCR, Appl. Environ. Microb., 74, 7174–7182, https://doi.org/10.1128/AEM.01298-08, 2008.
Gustafsson, B. G., Schenk, F., Blenckner, T., Eilola, K., Meier, H. M., Müller-Karulis, B., Neumann, T., Ruoho-Airola, T., Savchuk, O. P., and Zorita, E.: Reconstructing the development of Baltic Sea eutrophication 1850–2006, Ambio, 41, 534–548, https://doi.org/10.1007/s13280-012-0318-x, 2012.
Hain, M. P., Sigman, D., and Haug, G.: The biological Pump in the Past, in: Treatise on Geochemistry (Second Edition), edited by: Holland, H. D. and Turekian, K. K., Elsevier, Oxford, 8, 485–517, https://doi.org/10.1016/B978-0-08-095975-7.00618-5, 2014.
Hajdas, I., Ascough, P., Garnett, M. H., Fallon, S. J., Pearson, C. L., Quarta, G., Spalding, K. L., Yamaguchi, H., and Yoneda, M.: Radiocarbon dating, Nature Reviews Methods Primers, 1, 62, https://doi.org/10.1038/s43586-021-00058-7, 2021.
Hancock, G., Leslie, C., Everett, S., Tims, S., Brunskill, G., and Haese, R.: Plutonium as a chronomarker in Australian and New Zealand sediments: a comparison with 137Cs, J. Environ. Radioactiv., 102, 919–929, https://doi.org/10.1016/j.jenvrad.2009.09.008, 2011.
Härnström, K., Ellegaard, M., Andersen, T. J., and Godhe, A.: Hundred years of genetic structure in a sediment revived diatom population, P. Natl. Acad. Sci. USA, 108, 4252–4257, https://doi.org/10.1073/pnas.1013528108, 2011.
Hattich, G., Jokinen, S., Sildever, S., Gareis, M., Heikkinen, J., Junghardt, N., Segovia, M., Machado, M., and Sjöqvist, C.: Temperature optima of a natural diatom population increases as global warming proceeds, Nat. Clim. Change, 14, 518–525, https://doi.org/10.1038/s41558-024-01981-9, 2024.
Haywood, A. M., Valdes, P. J., and Sellwood, B. W.: Global scale palaeoclimate reconstruction of the middle Pliocene climate using the UKMO GCM: initial results, Global Planet. Change, 25, 239–256, https://doi.org/10.1016/S0921-8181(00)00028-X, 2000.
Hedges, J. I. and Keil, R. G.: Sedimentary organic matter preservation: an assessment and speculative synthesis, Mar. Chem., 49, 81–115, https://doi.org/10.1016/0304-4203(95)00008-F, 1995.
Hellweger, F. L. and Bucci, V.: A bunch of tiny individuals – Individual-based modeling for microbes, Ecol. Modell., 220, 8–22, https://doi.org/10.1016/j.ecolmodel.2008.09.004, 2009.
Hense, I.: Regulative feedback mechanisms in cyanobacteria-driven systems: a model study, Mar. Ecol. Prog. Ser., 339, 41–47, https://doi.org/10.3354/meps339041, 2007.
Hense, I. and Beckmann, A.: Towards a model of cyanobacteria life cycle – effects of growing and resting stages on bloom formation of N2-fixing species, Ecol. Modell., 195, 205–218, https://doi.org/10.1016/j.ecolmodel.2005.11.018, 2006.
Hillaire-Marcel, C. and de Vernal, A.: Proxies in late cenozoic paleoceanography, Elsevier, 1st Edition, 864 pp., ISBN 9780080525044, 2007.
Hinners, J., Kremp, A., and Hense, I.: Evolution in temperature-dependent phytoplankton traits revealed from a sediment archive: do reaction norms tell the whole story?, Proc. R. Soc. B: Biol. Sci., 284, 20171888, https://doi.org/10.1098/rspb.2017.1888, 2017.
Hinners, J., Hense, I., and Kremp, A.: Modelling phytoplankton adaptation to global warming based on resurrection experiments, Ecol. Modell., 400, 27–33, https://doi.org/10.1016/j.ecolmodel.2019.03.006, 2019.
Hinners, J., Argyle, P. A., Walworth, N. G., Doblin, M. A., Levine, N. M., and Collins, S.: Multi-trait diversification in marine diatoms in constant and warmed environments, P. Roy. Soc. B, 291, 20232564, https://doi.org/10.1098/rspb.2023.2564, 2024.
Hirschhorn, J. N. and Daly, M. J.: Genome-wide association studies for common diseases and complex traits, Nat. Rev. Genet., 6, 95–108, https://doi.org/10.1038/nrg1521, 2005.
Hjerne, O., Hajdu, S., Larsson, U., Downing, A. S., and Winder, M.: Climate Driven Changes in Timing, Composition and Magnitude of the Baltic Sea Phytoplankton Spring Bloom, Front. Mar. Sci., 6, 482, https://doi.org/10.3389/fmars.2019.00482, 2019.
Hochfeld, I. and Hinners, J.: Evolutionary adaptation to steady or changing environments affects competitive outcomes in marine phytoplankton, Limnol. Oceanogr., 69, 1172–1186, https://doi.org/10.1002/lno.12559, 2024a.
Hochfeld, I. and Hinners, J.: Phytoplankton adaptation to steady or changing environments affects marine ecosystem functioning, Biogeosciences, 21, 5591–5611, https://doi.org/10.5194/bg-21-5591-2024, 2024b.
Holland, M. M., Bitz, C. M., and Tremblay, B.: Future abrupt reductions in the summer Arctic sea ice, Geophys. Res. Lett., 33, L23503, https://doi.org/10.1029/2006GL028024, 2006.
Hollingsworth, A.: Validation and diagnosis of atmospheric models, Dynam. Atmos. Oceans, 20, 227–246, https://doi.org/10.1016/0377-0265(94)90019-1, 1994.
Hollowed, A. B., Barange, M., Beamish, R. J., Brander, K., Cochrane, K., Drinkwater, K., Foreman, M. G., Hare, J. A., Holt, J., Ito, S., Kim, S., King, J. R., Loeng, H., MacKenzie, B. R., Mueter, F. J., Okey, T. A., Peck, M. A., Radchenko, V. I., Rice, J. C., Schirripa, M. J., Yatsu, A., and Yamanaka, Y.: Projected impacts of climate change on marine fish and fisheries, ICES J. Mar. Sci., 70, 1023–1037, https://doi.org/10.1093/icesjms/fst081, 2013.
Hutchins, D. A. and Fu, F.: Microorganisms and ocean global change, Nat. Microbiol., 2, 17058, https://doi.org/10.1038/nmicrobiol.2017.58, 2017.
Hutchins, D. A., Walworth, N. G., Webb, E. A., Saito, M. A., Moran, D., McIlvin, M. R., Gale, J., and Fu, F.-X.: Irreversibly increased nitrogen fixation in Trichodesmium experimentally adapted to elevated carbon dioxide, Nat. Commun., 6, 8155, https://doi.org/10.1038/ncomms9155, 2015.
Intergovernmental Panel on Climate Change (IPCC): Summary for Policymakers, in: Climate Change 2023: Synthesis Report. Contribution of Working Groups I, II and III to the Sixth Assessment Report of the Intergovernmental Panel on Climate Change, edited by: Core Writing Team, Lee, H. and Romero, J., IPCC, Geneva, Switzerland, 1–34, https://doi.org/10.59327/IPCC/AR6-9789291691647.001, 2023.
Irwin, A. J., Finkel, Z. V., Müller-Karger, F. E., and Ghinaglia, L. T.: Phytoplankton adapt to changing ocean environments, P. Natl. Acad. Sci. USA, 112, 5762–5766, https://doi.org/10.1073/pnas.1414752112, 2015.
Isanta-Navarro, J., Hairston Jr., N. G., Beninde, J., Meyer, A., Straile, D., Möst, M., and Martin-Creuzburg, D.: Reversed evolution of grazer resistance to cyanobacteria, Nat. Commun., 12, 1945, https://doi.org/10.1038/s41467-021-22226-9, 2021.
Jin, P. and Agustí, S.: Fast adaptation of tropical diatoms to increased warming with trade-offs, Sci. Rep., 8, 17771, https://doi.org/10.1038/s41598-018-36091-y, 2018.
Kabel, K., Moros, M., Porsche, C., Neumann, T., Adolphi, F., Andersen, T. J., Siegel, H., Gerth, M., Leipe, T., Jansen, E., and Damsté, J. S. S.: Impact of climate change on the Baltic Sea ecosystem over the past 1,000 years, Nat. Clim. Change, 2, 871–874, https://doi.org/10.1038/nclimate1595, 2012.
Kaiser, J., van der Meer, M. T., and Arz, H. W.: Long-chain alkenones in Baltic Sea surface sediments: new insights, Org. Geochem., 112, 93–104, https://doi.org/10.1016/j.orggeochem.2017.07.002, 2017.
Kerfoot, W. C. and Weider, L. J.: Experimental paleoecology (resurrection ecology): chasing Van Valen's Red Queen hypothesis, Limnol. Oceanogr., 49, 1300–1316, https://doi.org/10.4319/lo.2004.49.4_part_2.1300, 2004.
Kjær, K. H., Winther Pedersen, M., De Sanctis, B., De Cahsan, B., Korneliussen, T. S., Michelsen, C. S., Sand, K. K., Jelavić, S., Ruter, A. H., Schmidt, A. M., Kjeldsen, K. K., Tesakov, A. S., Snowball, I., Gosse, J. C., Alsos, I. G., Wang, Y., Dockter, C., Rasmussen, M., Jørgensen, M. E., Skadhauge, B., Prohaska, A., Kristensen, J. Å., Bjerager, M., Allentoft, M. E., Coissac, E., PhyloNorway Consortium, Rouillard, A., Simakova, A., Fernandez-Guerra, A., Bowler, C., Macias-Fauria, M., Vinner, L., Welch, J. J., Hidy, A. J., Sikora, M., Collins, M. J., Durbin, R., Larsen, N. K., and Willerslev, E.: A 2-million-year-old ecosystem in Greenland uncovered by environmental DNA, Nature, 612, 283–291, https://doi.org/10.1038/s41586-022-05453-y, 2022.
Klausmeier, C. A., Osmond, M. M., Kremer, C. T., and Litchman, E.: Ecological limits to evolutionary rescue, Philos. T. R. Soc. B, 375, 20190453, https://doi.org/10.1098/rstb.2019.0453, 2020a.
Klausmeier, C. A., Kremer, C. T., and Koffel, T.: Trait-based ecological and eco-evolutionary theory, in: Theoretical Ecology: concepts and applications, edited by: McCann, K. S. and Gellner, G., Oxford University Press, 161–194, https://doi.org/10.1093/oso/9780198824282.003.0011, 2020.
Korkonen, S. T., Ojala, A., Kosonen, E. M., and Weckström, J. B.: Seasonality of chrysophyte cyst and diatom assemblages in varved Lake Nautajärvi–implications for palaeolimnological studies, J. Limnol., 76, 366–379, https://doi.org/10.4081/jlimnol.2017.1473, 2017.
Krasovec, M., Sanchez-Brosseau, S., and Piganeau, G.: First estimation of the spontaneous mutation rate in diatoms, Genome Biol. Evol., 11, 1829–1837, https://doi.org/10.1093/gbe/evz130, 2019.
Kremer, C. T. and Klausmeier, C. A.: Coexistence in a variable environment: eco-evolutionary perspectives, J. Theor. Biol., 339, 14–25, https://doi.org/10.1016/j.jtbi.2013.05.005, 2013.
Kremer, C. T. and Klausmeier, C. A.: Species packing in eco-evolutionary models of seasonally fluctuating environments, Ecol. Lett., 20, 1158–1168, https://doi.org/10.1111/ele.12813, 2017.
Kremp, A., Hinners, J., Klais, R., Leppänen, A.-P., and Kallio, A.: Patterns of vertical cyst distribution and survival in 100-year-old sediment archives of three spring dinoflagellate species from the Northern Baltic Sea, European J. Phycol., 53, 135–145, https://doi.org/10.1080/09670262.2017.1386330, 2018.
Krishna, S., Peterson, V., Listmann, L., and Hinners, J.: Interactive effects of viral lysis and warming in a coastal ocean identified from an idealized ecosystem model, Ecol. Modell., 487, 110550, https://doi.org/10.1016/j.ecolmodel.2023.110550, 2024.
Lässig, M., Mustonen, V., and Walczak, A. M.: Predicting evolution, Nature Ecology & Evolution, 1, 0077, https://doi.org/10.1038/s41559-017-0077, 2017.
Laufkötter, C., Vogt, M., Gruber, N., Aita-Noguchi, M., Aumont, O., Bopp, L., Buitenhuis, E., Doney, S. C., Dunne, J., Hashioka, T., Hauck, J., Hirata, T., John, J., Le Quéré, C., Lima, I. D., Nakano, H., Seferian, R., Totterdell, I., Vichi, M., and Völker, C.: Drivers and uncertainties of future global marine primary production in marine ecosystem models, Biogeosciences, 12, 6955–6984, https://doi.org/10.5194/bg-12-6955-2015, 2015.
Laufkötter, C., Vogt, M., Gruber, N., Aumont, O., Bopp, L., Doney, S. C., Dunne, J. P., Hauck, J., John, J. G., Lima, I. D., Seferian, R., and Völker, C.: Projected decreases in future marine export production: the role of the carbon flux through the upper ocean ecosystem, Biogeosciences, 13, 4023–4047, https://doi.org/10.5194/bg-13-4023-2016, 2016.
Le Gland, G., Vallina, S. M., Smith, S. L., and Cermeño, P.: SPEAD 1.0 – Simulating Plankton Evolution with Adaptive Dynamics in a two-trait continuous fitness landscape applied to the Sargasso Sea, Geosci. Model Dev., 14, 1949–1985, https://doi.org/10.5194/gmd-14-1949-2021, 2021.
Lear, C. H., Rosenthal, Y., and Slowey, N.: Benthic foraminiferal Mg/Ca-paleothermometry: A revised core-top calibration, Geochim. Cosmochim. Acta, 66, 3375–3387, https://doi.org/10.1016/S0016-7037(02)00941-9, 2002.
Lee, S., Hofmeister, R., and Hense, I.: The role of life cycle processes on phytoplankton spring bloom composition: a modelling study applied to the Gulf of Finland, J. Marine Syst., 178, 75–85, https://doi.org/10.1016/j.jmarsys.2017.10.010, 2018.
Lehtimäki, J., Moisander, P., Sivonen, K., and Kononen, K.: Growth, nitrogen fixation, and nodularin production by two Baltic Sea cyanobacteria, Appl. Environ. Microb., 63, 1647–1656, https://doi.org/10.1128/aem.63.5.1647-1656.1997, 1997.
Lenski, R. E. and Travisano, M.: Dynamics of adaptation and diversification: a 10,000-generation experiment with bacterial populations., P. Natl. Acad. Sci. USA, 91, 6808–6814, https://doi.org/10.1073/pnas.91.15.6808, 1994.
Lenton, T. M., Held, H., Kriegler, E., Hall, J. W., Lucht, W., Rahmstorf, S., and Schellnhuber, H. J.: Tipping elements in the Earth's climate system, P. Natl. Acad. Sci. USA, 105, 1786–1793, https://doi.org/10.1073/pnas.0705414105, 2008.
Levins, R.: Theory of fitness in a heterogeneous environment. I. The fitness set and adaptive function, Am. Nat., 96, 361–373, https://doi.org/10.1086/282245, 1962.
Li, Q., Bruggeman, J., Burchard, H., Klingbeil, K., Umlauf, L., and Bolding, K.: Integrating CVMix into GOTM (v6.0): a consistent framework for testing, comparing, and applying ocean mixing schemes, Geosci. Model Dev., 14, 4261–4282, https://doi.org/10.5194/gmd-14-4261-2021, 2021.
Limoges, A., Van Nieuwenhove, N., Head, M. J., Mertens, K. N., Pospelova, V., and Rochon, A.: A review of rare and less well known extant marine organic-walled dinoflagellate cyst taxa of the orders Gonyaulacales and Suessiales from the Northern Hemisphere, Mar. Micropaleontol., 159, 101801, https://doi.org/10.1016/j.marmicro.2019.101801, 2020.
Listmann, L., LeRoch, M., Schlüter, L., Thomas, M. K., and Reusch, T. B.: Swift thermal reaction norm evolution in a key marine phytoplankton species, Evol. Appl., 9, 1156–1164, https://doi.org/10.1111/eva.12362, 2016.
Litchman, E., Klausmeier, C. A., Schofield, O. M., and Falkowski, P. G.: The role of functional traits and trade-offs in structuring phytoplankton communities: scaling from cellular to ecosystem level, Ecol. Lett., 10, 1170–1181, https://doi.org/10.1111/j.1461-0248.2007.01117.x, 2007.
Litchman, E., de Tezanos Pinto, P., Edwards, K. F., Klausmeier, C. A., Kremer, C. T., and Thomas, M. K.: Global biogeochemical impacts of phytoplankton: a trait-based perspective, J. Ecol., 103, 1384–1396, https://doi.org/10.1111/1365-2745.12438, 2015.
Lomstein, B. A., Langerhuus, A. T., D'Hondt, S., Jørgensen, B. B., and Spivack, A. J.: Endospore abundance, microbial growth and necromass turnover in deep sub-seafloor sediment, Nature, 484, 101–104, https://doi.org/10.1038/nature10905, 2012.
Lowe, D. J. and Alloway, B. V.: Tephrochronology, in: Encyclopaedia of Scientific Dating Methods, edited by: Rink, W. J. and Thompson, J. W., Springer, Dordrecht, 783–799, https://doi.org/10.1007/978-94-007-6304-3_19, 2015.
Maslin, M. A., Pike, J., Stickley, C., and Ettwein, V.: Evidence of Holocene climate variability from marine sediments, in: Global Change in the Holocene, edited by: Mackay, A., Battarbee, R., Birks, J., and Oldfield, F., London, Arnold, 185–209, ISBN 978-0-340-81214-3, 2003.
Matul, A., Spielhagen, R. F., Kazarina, G., Kruglikova, S., Dmitrenko, O., and Mohan, R.: Warm-water events in the eastern Fram Strait during the last 2000 years as revealed by different microfossil groups, Polar. Res., 37, 1540243, https://doi.org/10.1080/17518369.2018.1540243, 2018.
McGraw, J., Vavrek, M., and Bennington, C.: Ecological genetic variation in seed banks I. Establishment of a time transect, The J. Ecol., 79, 617–625, https://doi.org/10.2307/2260657, 1991.
Medlin, L., Sáez, A. G., and Young, J. R.: A molecular clock for coccolithophores and implications for selectivity of phytoplankton extinctions across the K/T boundary, Mar. Micropaleontol., 67, 69–86, https://doi.org/10.1016/j.marmicro.2007.08.007, 2008.
Medwed, C., Karsten, U., Romahn, J., Kaiser, J., Dellwig, O., Arz, H., and Kremp, A.: Archives of cyanobacterial traits: insights from resurrected Nodularia spumigena from Baltic Sea sediments reveal a shift in temperature optima, ISME Communications, 4, ycae140, https://doi.org/10.1093/ismeco/ycae140, 2024.
Mejbel, H. S., Dodsworth, W., Baud, A., Gregory-Eaves, I., and Pick, F. R.: Comparing quantitative methods for analyzing sediment DNA records of cyanobacteria in experimental and reference lakes, Front. Microbiol., 12, 669910, https://doi.org/10.3389/fmicb.2021.669910, 2021.
Melbinger, A. and Vergassola, M.: The Impact of Environmental Fluctuations on Evolutionary Fitness Functions, Sci. Rep., 5, 15211, https://doi.org/10.1038/srep15211, 2015.
Mitchell, D., Willerslev, E., and Hansen, A.: Damage and repair of ancient DNA, Mutat. Res.-Fund. Mol. M, 571, 265–276, https://doi.org/10.1016/j.mrfmmm.2004.06.060, 2005.
Monchamp, M.-E., Walser, J.-C., Pomati, F., and Spaak, P.: Sedimentary DNA reveals cyanobacterial community diversity over 200 years in two perialpine lakes, Appl. Environ. Microb., 82, 6472–6482, https://doi.org/10.1128/AEM.02174-16, 2016.
Muhs, D. R.: Evidence for the timing and duration of the last interglacial period from high-precision uranium-series ages of corals on tectonically stable coastlines, Quaternary Res., 58, 36–40, https://doi.org/10.1006/qres.2002.2339, 2002.
North Greenland Ice Core Project members: High-resolution record of Northern Hemisphere climate extending into the last interglacial period, Nature, 431, 147–151, https://doi.org/10.1038/nature02805, 2004.
O'Donnell, D. R., Hamman, C. R., Johnson, E. C., Kremer, C. T., Klausmeier, C. A., and Litchman, E.: Rapid thermal adaptation in a marine diatom reveals constraints and trade-offs, Glob. Change Biol., 24, 4554–4565, https://doi.org/10.1111/gcb.14360, 2018.
Østman, B., Hintze, A., and Adami, C.: Impact of epistasis and pleiotropy on evolutionary adaptation, P. Roy. Soc. B-Biol. Sci., 279, 247–256, https://doi.org/10.1098/rspb.2011.0870, 2012.
Pauwels, K., De Meester, L., Put, S., Decaestecker, E., Decaestecker, E., and Stoks, R.: Rapid evolution of phenoloxidase expression, a component of innate immune function, in a natural population of Daphnia magna, Limnol. Oceanogr., 55, 1408–1413, https://doi.org/10.4319/lo.2010.55.3.1408, 2010.
Peer, A. and Miller, T.: Climate change, migration phenology, and fisheries management interact with unanticipated consequences, N. Am. J. Fish. Manage., 34, 94–110, https://doi.org/10.1080/02755947.2013.847877, 2014.
Pfenninger, M.: On the potential for GWAS with phenotypic population means and allele-frequency data (popGWAS), bioRxiv [preprint], https://doi.org/10.1101/2024.06.12.598621, 14 June 2024.
Poloczanska, E. S., Brown, C. J., Sydeman, W. J., Kiessling, W., Schoeman, D. S., Moore, P. J., Brander, K., Bruno, J. F., Buckley, L. B., Burrows, M. T., Duarte, C. M., Halpern, B. S., Holding, J., Kappel, C. V., O’Connor, M. I., Pandolfi, J. M., Parmesan, C., Schwing, F., Thompson, S. A., and Richardson, A. J.: Global imprint of climate change on marine life, Nat. Clim. Change, 3, 919–925, https://doi.org/10.1038/nclimate1958, 2013.
Prahl, F. G., Muehlhausen, L. A., and Zahnle, D. L.: Further evaluation of long-chain alkenones as indicators of paleoceanographic conditions, Geochim. Cosmochim. Acta, 52, 2303–2310, https://doi.org/10.1016/0016-7037(88)90132-9, 1988.
Rosell-Melé, A.: Interhemispheric appraisal of the value of alkenone indices as temperature and salinity proxies in high-latitude locations, Paleoceanography, 13, 694–703, https://doi.org/10.1029/98PA02355, 1998.
Sallon, S., Solowey, E., Cohen, Y., Korchinsky, R., Egli, M., Woodhatch, I., Simchoni, O., and Kislev, M.: Germination, genetics, and growth of an ancient date seed, Science, 320, 1464–1464, https://doi.org/10.1126/science.1153600, 2008.
Salzmann, U., Haywood, A., Lunt, D., Valdes, P., and Hill, D.: A new global biome reconstruction and data-model comparison for the Middle Pliocene, Global Ecol. Biogeogr., 17, 432–447, https://doi.org/10.1111/j.1466-8238.2008.00381.x, 2008.
Sanyal, A., Larsson, J., van Wirdum, F., Andrén, T., Moros, M., Lönn, M., and Andrén, E.: Not dead yet: Diatom resting spores can survive in nature for several millennia, Am. J. Bot., 109, 67–82, https://doi.org/10.1002/ajb2.1780, 2022.
Sathyendranath, S., Gouveia, A. D., Shetye, S. R., Ravindran, P., and Platt, T.: Biological control of surface temperature in the Arabian Sea, Nature, 349, 54–56, https://doi.org/10.1038/349054a0, 1991.
Sauterey, B., Ward, B., Rault, J., Bowler, C., and Claessen, D.: The implications of eco-evolutionary processes for the emergence of marine plankton community biogeography, Am. Nat., 190, 116–130, https://doi.org/10.1086/692067, 2017.
Schabhüttl, S., Hingsamer, P., Weigelhofer, G., Hein, T., Weigert, A., and Striebel, M.: Temperature and species richness effects in phytoplankton communities, Oecologia, 171, 527–536, https://doi.org/10.1007/s00442-012-2419-4, 2013.
Schaum, C.-E., Barton, S., Bestion, E., Buckling, A., Garcia-Carreras, B., Lopez, P., Lowe, C., Pawar, S., Smirnoff, N., Trimmer, M., and Yvon-Durocher, G.: Adaptation of phytoplankton to a decade of experimental warming linked to increased photosynthesis, Nat. Ecol. Evol., 1, 0094, https://doi.org/10.1038/s41559-017-0094, 2017.
Schmidt, A., Romahn, J., Andrén, E., Kremp, A., Kaiser, J., Arz, H. W., Dellwig, O., Bálint, M., and Epp, L. S.: Decoding the Baltic Sea's past and present: a simple molecular index for ecosystem assessment, Ecol. Indic., 166, 112494, https://doi.org/10.1016/j.ecolind.2024.112494, 2024.
Schouten, S., Hopmans, E. C., and Damsté, J. S. S.: The organic geochemistry of glycerol dialkyl glycerol tetraether lipids: A review, Org. Geochem., 54, 19–61, https://doi.org/10.1016/j.orggeochem.2012.09.006, 2013.
Semenov, M., Chernov, T., Zhelezova, A., Nikitin, D., Tkhakakhova, A., Ivanova, E., Xenofontova, N., Sycheva, S., Kolganova, T., and Kutovaya, O.: Microbial communities of interglacial and interstadial paleosols of the Late Pleistocene, Eurasian Soil Sci., 53, 772–779, https://doi.org/10.1134/S1064229320060101, 2020.
Smith, S. L., Vallina, S. M., and Merico, A.: Phytoplankton size-diversity mediates an emergent trade-off in ecosystem functioning for rare versus frequent disturbances, Sci. Rep., 6, 34170, https://doi.org/10.1038/srep34170, 2016.
Stirling, C., Esat, T., Lambeck, K., and McCulloch, M.: Timing and duration of the Last Interglacial: evidence for a restricted interval of widespread coral reef growth, Earth Planet. Sci. Lett., 160, 745–762, https://doi.org/10.1016/S0012-821X(98)00125-3, 1998.
Stroeve, J., Holland, M. M., Meier, W., Scambos, T., and Serreze, M.: Arctic sea ice decline: Faster than forecast, Geophys. Res. Lett., 34, L09501, https://doi.org/10.1029/2007GL029703, 2007.
Tittensor, D. P., Novaglio, C., Harrison, C. S., Heneghan, R. F., Barrier, N., Bianchi, D., Bopp, L., Bryndum-Buchholz, A., Britten, G. L., Büchner, M., Büchner, M., Cheung, W. W. L., Christensen, V., Coll, M., Dunne, J. P., Eddy, T. D., Everett, J. D., Fernandes-Salvador, J. A., Fulton, E. A., Galbraith, E. D., Gascuel, D., Guiet, J., John, J. G., Link, J. S., Lotze, H. K., Maury, O., Ortega-Cisneros, K., Palacios-Abrantes, J., Petrik, C. M., du Pontavice, H., Rault, J., Richardson, A. J., Shannon, L., Shin, Y.-J., Steenbeek, J., Stock, C. A., and Blanchard, J. L.: Next-generation ensemble projections reveal higher climate risks for marine ecosystems, Nat. Clim. Change, 11, 973–981, https://doi.org/10.1038/s41558-021-01173-9, 2021.
Tonani, M., Balmaseda, M., Bertino, L., Blockley, E., Brassington, G., Davidson, F., Drillet, Y., Hogan, P., Kuragano, T., Lee, T., Mehra, A., Paranathara, F., Tanajura, C. A. S., and Wang, H.: Status and future of global and regional ocean prediction systems, J. Oper. Oceanogr., 8, s201–s220, https://doi.org/10.1080/1755876X.2015.1049892, 2015.
Tyler, A. L., Asselbergs, F. W., Williams, S. M., and Moore, J. H.: Shadows of complexity: what biological networks reveal about epistasis and pleiotropy, Bioessays, 31, 220–227, https://doi.org/10.1002/bies.200800022, 2009.
Uffelmann, E., Huang, Q. Q., Munung, N. S., De Vries, J., Okada, Y., Martin, A. R., Martin, H. C., Lappalainen, T., and Posthuma, D.: Genome-wide association studies, Nature Reviews Methods Primers, 1, 59, https://doi.org/10.1038/s43586-021-00056-9, 2021.
Umlauf, L., Burchard, H., and Bolding, K.: GOTM-scientific documentation: version 3.2, Institut für Ostseeforschung, https://www.io-warnemuende.de/files/forschung/meereswissenschaftliche-berichte/mebe63_2005-gotm.pdf (last access: 28 April 2025), 2005.
Van Nieuwenhove, N., Head, M. J., Limoges, A., Pospelova, V., Mertens, K. N., Matthiessen, J., De Schepper, S., de Vernal, A., Eynaud, F., Londeix, L., Marretj, F., Penaud, A., Radih, T., and Rochon, A.: An overview and brief description of common marine organic-walled dinoflagellate cyst taxa occurring in surface sediments of the Northern Hemisphere, Mar. Micropaleontol., 159, 101814, https://doi.org/10.1016/j.marmicro.2019.101814, 2020.
Vasselon, V., Bouchez, A., Rimet, F., Jacquet, S., Trobajo, R., Corniquel, M., Tapolczai, K., and Domaizon, I.: Avoiding quantification bias in metabarcoding: Application of a cell biovolume correction factor in diatom molecular biomonitoring, Methods Ecol. Evol., 9, 1060–1069, https://doi.org/10.1111/2041-210X.12960, 2018.
Vincent, W. and Silvester, W.: Growth of blue-green algae in the Manukau (New Zealand) oxidation ponds – I. Growth potential of oxidation pond water and comparative optima for blue-green and green algal growth, Water Res., 13, 711–716, https://doi.org/10.1016/0043-1354(79)90234-3, 1979.
Visscher, P. M., Wray, N. R., Zhang, Q., Sklar, P., McCarthy, M. I., Brown, M. A., and Yang, J.: 10 years of GWAS discovery: biology, function, and translation, Am. J. Hum. Genet., 101, 5–22, https://doi.org/10.1016/j.ajhg.2017.06.005, 2017.
Wakeham, S. G. and Canuel, E. A.: Degradation and Preservation of Organic Matter in Marine Sediments, in: Marine Organic Matter: Biomarkers, Isotopes and DNA, The Handbook of Environmental Chemistry, Volume 2N, edited by: Volkman, J. K., Springer, Berlin, Heidelberg, 295–321, https://doi.org/10.1007/698_2_009, 2006.
Ward, B. A., Dutkiewicz, S., Jahn, O., and Follows, M. J.: A size-structured food-web model for the global ocean, Limnol. Oceanogr., 57, 1877–1891, https://doi.org/10.4319/lo.2012.57.6.1877, 2012.
Ward, B. A., Collins, S., Dutkiewicz, S., Gibbs, S., Bown, P., Ridgwell, A., Sauterey, B., Wilson, J., and Oschlies, A.: Considering the role of adaptive evolution in models of the ocean and climate system, J. Adv. Model. Earth Syst., 11, 3343–3361, https://doi.org/10.1029/2018MS001452, 2019.
Wasmund, N., Nausch, G., Gerth, M., Busch, S., Burmeister, C., Hansen, R., and Sadkowiak, B.: Extension of the growing season of phytoplankton in the western Baltic Sea in response to climate change, Mar. Ecol. Prog. Ser., 622, 1–16, https://doi.org/10.3354/meps12994, 2019.
Weckström, K.: Assessing recent eutrophication in coastal waters of the Gulf of Finland (Baltic Sea) using subfossil diatoms, J. Paleolimnol., 35, 571–592, https://doi.org/10.1007/s10933-005-5264-1, 2006.
Weitz, J. S., Stock, C. A., Wilhelm, S. W., Bourouiba, L., Coleman, M. L., Buchan, A., Follows, M. J., Fuhrman, J. A., Jover, L. F., Lennon, J. T., Middelboe, M., Sonderegger, D. L., Suttle, C. A., Taylor, B. P., Frede Thingstad, T., Wilson, W. H., and Eric Wommack, K.: A multitrophic model to quantify the effects of marine viruses on microbial food webs and ecosystem processes, ISME J., 9, 1352–1364, https://doi.org/10.1038/ismej.2014.220, 2015.
Wersebe, M. J. and Weider, L. J.: Resurrection genomics provides molecular and phenotypic evidence of rapid adaptation to salinization in a keystone aquatic species, P. Natl. Acad. Sci. USA, 120, e2217276120, https://doi.org/10.1073/pnas.2217276120, 2023.
Willerslev, E., Hansen, A. J., Binladen, J., Brand, T. B., Gilbert, M. T. P., Shapiro, B., Bunce, M., Wiuf, C., Gilichinsky, D. A., and Cooper, A.: Diverse plant and animal genetic records from Holocene and Pleistocene sediments, Science, 300, 791–795, https://doi.org/10.1126/science.1084114, 2003.
Wittenborn, A. K., Radtke, H., Dutheil, C., Arz, H. W., and Kaiser, J.: A downcore calibration of the TEX temperature proxy for the Baltic Sea, Cont. Shelf Res., 251, 104875, https://doi.org/10.1016/j.csr.2022.104875, 2022.
Wood, S. M., Kremp, A., Savela, H., Akter, S., Vartti, V.-P., Saarni, S., and Suikkanen, S.: Cyanobacterial akinete distribution, viability, and cyanotoxin records in sediment archives from the Northern Baltic Sea, Front. Microbiol., 12, 681881, https://doi.org/10.3389/fmicb.2021.681881, 2021.
Wörmer, L., Hoshino, T., Bowles, M. W., Viehweger, B., Adhikari, R. R., Xiao, N., Uramoto, G., Könneke, M., Lazar, C. S., Morono, Y., Inagaki, F., and Hinrichs, K.-U.: Microbial dormancy in the marine subsurface: global endospore abundance and response to burial, Sci. Adv., 5, eaav1024, https://doi.org/10.1126/sciadv.aav1024, 2019.
Zimmermann, H. H., Stoof-Leichsenring, K. R., Dinkel, V., Harms, L., Schulte, L., Hütt, M.-T., Nürnberg, D., Tiedemann, R., and Herzschuh, U.: Marine ecosystem shifts with deglacial sea-ice loss inferred from ancient DNA shotgun sequencing, Nat. Commun., 14, 1650, https://doi.org/10.1038/s41467-023-36845-x, 2023.
Zonneveld, K. A. F., Versteegh, G. J. M., Kasten, S., Eglinton, T. I., Emeis, K.-C., Huguet, C., Koch, B. P., de Lange, G. J., de Leeuw, J. W., Middelburg, J. J., Mollenhauer, G., Prahl, F. G., Rethemeyer, J., and Wakeham, S. G.: Selective preservation of organic matter in marine environments; processes and impact on the sedimentary record, Biogeosciences, 7, 483–511, https://doi.org/10.5194/bg-7-483-2010, 2010.
- Abstract
- Introduction
- Sediment archives – understanding phytoplankton responses to environmental change
- Integration of data from sediment archives into evolutionary ecosystem models
- Challenges and potential of using data from sediment archives for evolutionary ecosystem modeling
- Conclusions
- Data availability
- Author contributions
- Competing interests
- Disclaimer
- Acknowledgements
- Financial support
- Review statement
- References
- Abstract
- Introduction
- Sediment archives – understanding phytoplankton responses to environmental change
- Integration of data from sediment archives into evolutionary ecosystem models
- Challenges and potential of using data from sediment archives for evolutionary ecosystem modeling
- Conclusions
- Data availability
- Author contributions
- Competing interests
- Disclaimer
- Acknowledgements
- Financial support
- Review statement
- References