the Creative Commons Attribution 4.0 License.
the Creative Commons Attribution 4.0 License.
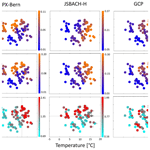
Air temperature and precipitation constraining the modelled wetland methane emissions in a boreal region in northern Europe
Aki Tsuruta
Jarmo Mäkelä
Jurek Müller
Maria Tenkanen
Eleanor Burke
Sarah Chadburn
Vilma Mannisenaho
Thomas Kleinen
Hanna Lee
Antti Leppänen
Tiina Markkanen
Stefano Materia
Paul A. Miller
Daniele Peano
Olli Peltola
Benjamin Poulter
Maarit Raivonen
Marielle Saunois
David Wårlind
Sönke Zaehle
Wetland methane responses to temperature and precipitation are studied in a boreal wetland-rich region in northern Europe using ecosystem process models. Six ecosystem models (JSBACH-HIMMELI, LPX-Bern, LPJ-GUESS, JULES, CLM4.5, and CLM5) are compared to multi-model means of ecosystem models and atmospheric inversions from the Global Carbon Project and upscaled eddy covariance flux results for their temperature and precipitation responses and seasonal cycles of the regional fluxes. Two models with contrasting response patterns, LPX-Bern and JSBACH-HIMMELI, are used as priors in atmospheric inversions with Carbon Tracker Europe–CH4 (CTE-CH4) in order to find out how the assimilation of atmospheric concentration data changes the flux estimates and how this alters the interpretation of the flux responses to temperature and precipitation. Inversion moves wetland emissions of both models towards co-limitation by temperature and precipitation. Between 2000 and 2018, periods of high temperature and/or high precipitation often resulted in increased emissions. However, the dry summer of 2018 did not result in increased emissions despite the high temperatures. The process models show strong temperature and strong precipitation responses for the region (51 %–91 % of the variance explained by both). The month with the highest emissions varies from May to September among the models. However, multi-model means, inversions, and upscaled eddy covariance flux observations agree on the month of maximum emissions and are co-limited by temperature and precipitation. The setup of different emission components (peatland emissions, mineral land fluxes) has an important role in building up the response patterns. Considering the significant differences among the models, it is essential to pay more attention to the regional representation of wet and dry mineral soils and periodic flooding which contribute to the seasonality and magnitude of methane fluxes. The realistic representation of temperature dependence of the peat soil fluxes is also important. Furthermore, it is important to use process-based descriptions for both mineral and peat soil fluxes to simulate the flux responses to climate drivers.
- Article
(4298 KB) - Full-text XML
-
Supplement
(1131 KB) - BibTeX
- EndNote
Wetlands are the largest natural source of methane, contributing about 30 %–40 % to the global methane emissions (Saunois et al., 2020; Poulter et al., 2017). Wetlands considered in this study include those on peatlands, mineral lands, and periodically inundated (i.e. flooded) lands. Temperature, soil moisture, water table depth, and primary production drive the carbon accumulation, respiration, and methane emissions from peatlands and are modelled by ecosystem process models using atmospheric climate data, such as temperature and precipitation, as input to the simulations. Methane production takes place in water-saturated peat soil layers with limited oxygen availability via anoxic decomposition of soil organic matter by methanogenic microbes. There are accurate peatland maps for the northern regions based on in situ data of peat layer thickness (e.g. Xu et al., 2018; Tanneberger et al., 2017), which enable estimations of the peatland methane emissions by process models if the soil water table level and soil carbon processes providing substrate for methane production are well represented. Land not covered by peatlands includes mineral land. In addition, mineral lands can act as a source of methane if the soil is very moist or inundated (Lohila et al., 2016; Wolf et al., 2012; see also Bansal et al., 2023), with a significant contribution from the organic layer on top of the soil. The soil moisture and land inundation can also be estimated by models together with peat accumulation, though it is still challenging (e.g. Loisel et al., 2021; Ito et al., 2020). Soil moisture is an important input variable for mineral soil emission modelling (e.g. Curry, 2007).
In an attempt to realistically take into account the dynamical changes in the total methane-emitting area, many process models use wetland extent from remote sensing. However, this feature is badly represented especially in the boreal zone because forests shadow the inundated areas and lakes are easily misinterpreted as inundated lands (e.g. Olefeldt et al., 2021; Mahoney et al., 2020; Battaglia et al., 2021; Cohen et al., 2016; Chapman et al., 2016; Papa et al., 2006). Lakes do have methane emissions that may contribute up to one-third of boreal biogenic emissions (Guo et al., 2020), but descriptions of lake methane processes are often missing from ecosystem models. Large lakes and rivers have been mapped with high precision, but small ponds, pools, seasonal inundation, and low-order streams that may have high methane emissions are challenging to detect accurately (Olefeldt et al., 2021). Permanent water bodies (e.g. lakes, rivers, and reservoirs) are usually removed to only cover inundated and non-inundated vegetated wetlands (Zhang et al., 2021). Inundation products are used either as static maps or with inter-annual/month-to-month variation. As a result, the model predictions of regional annual cycles of methane emission differ and the future estimates of the total global methane emissions are highly variable (Stocker et al., 2014; Saunois et al., 2020). Therefore, instead of studying the response to wetland extent, it is useful to take a more climate-oriented perspective on the drivers of the methane emission in order to make better predictions of the responses to future climate change (Koffi et al., 2020). Furthermore, it is important to emphasise the regional approaches, as the drivers of emissions vary widely in their spatial distribution, climate, and ecosystem type (Stavert et al., 2022). It is important to study the responses of the regional emissions to air temperature and precipitation, as they define the response of regional wetland emissions to climate change.
Precipitation is the primary environmental driver for soil water dynamics during the growing season, and it can immediately impact the surface soil moisture, while its effect on the water table becomes apparent after a few days or weeks (e.g. Rinne et al., 2020; Gao et al., 2016). Widespread periodical inundation, i.e. flooding, may appear in spring due to melting snow. In the future, the amount of precipitation is projected to increase in the boreal zone (Putnam and Broeker, 2017; Ruosteenoja et al., 2016). This would potentially lead to wetland expansion (Poulter et al., 2017), although increased evapotranspiration may counteract this (Helbig et al., 2020). Furthermore, rising temperature enhances methanogenesis in the wet soils (Koffi et al., 2020). While wetland extent is the most significant driver of methane emissions in process models (Poulter et al., 2017), soil temperature was shown to be the dominant driver for the inter-annual variability in methane emissions in North America and soil moisture in the Western Siberian lowlands in Russia (Thompson et al, 2017) according to atmospheric inversion modelling and analysis of the results using climate reanalysis data (Dee et al., 2011). Soil moisture was also connected to soil carbon content and methane emissions in Fennoscandia (Albuhaisi et al., 2023) and in Finnish landscape-level studies (Räsänen et al., 2021; Vainio et al., 2021).
Atmospheric inverse models rely on atmospheric methane concentrations, and they provide a top-down view of the methane emissions. Their results can be used to study responses of regional methane emissions to climate drivers. In process models, the responses are more subject to how the processes were built, how dependencies were constructed, and how the fluxes were upscaled. Therefore, it is worthwhile to compare the atmospheric inversion models to process models and study how their regional emission estimates and climate responses differ. Here we compare temperature and precipitation responses from ecosystem process models participating in the H2020-CRESCENDO project for model development. We compare their results to the ensemble of models from the Global Carbon Project (GCP) 2020 estimation of the global methane budget (Saunois et al., 2020). We use two of the models and the average of the GCP land ecosystem model ensemble (Saunois et al., 2020; Poulter et al., 2017) as priors of wetland emissions to inversions with Carbon Tracker Europe–CH4 (CTE-CH4; Tsuruta et al., 2017). We determine the sensitivity of the inversion to its prior and how this changes the interpretation of the flux responses to precipitation and temperature change in the boreal region in Fennoscandia. As a result, we obtain an assessment for process-based models using atmospheric inversion modelling, providing guidance on how to improve their climate responses. We get an estimate of how the temperature and precipitation responses vary between the process models and how the extreme climate conditions of the recent years are reflected in the methane emissions.
The ecosystem process models are introduced here together with the inversion system, observations, and other materials used in this study. Of the ecosystem models, the wetland descriptions of JSBACH-HIMMELI, LPJ-GUESS, JULES, CLM4.5, and CLM5 were further developed in the recent H2020-CRESCENDO project, and results of the standalone simulations made for the project are used here. CLM5 and JULES results from the coupled Earth system model simulations were also retrieved from the recent Coupled Model Intercomparison Project Phase 6 (CMIP6; Eyring et al., 2016) data archive and utilised in the work. We also include the LPX-Bern v.1.4 model, which participated in Global Carbon Project (GCP) 2020 estimation of the global methane budget (Saunois et al., 2020). Furthermore, the ensemble mean of 12 ecosystem models from GCP is used for comparisons with the individual models and with the GCP ensemble mean of atmospheric inversions. The ensemble mean of the GCP ecosystem models, along with two ecosystem models with contrasting responses (JSBACH-HIMMELI and LPX-Bern), were used as priors in atmospheric inversions with Carbon Tracker Europe–CH4. Models and simulation setups are briefly introduced below and in Table 1.
2.1 JSBACH-HIMMELI
The ecosystem process model JSBACH version 3.2 with HIMMELI methane module version 1.0 (hereafter called JSBACH-H) was applied in this work. JSBACH is the land component of the Max Planck Institute Earth System Model (MPI-ESM) version 1.2 (Mauritsen et al., 2019) and includes a multilayer hydrology model (Hagemann and Stacke, 2015) and a representation of soil carbon by the YASSO model (Goll et al., 2015). The HIMMELI model, coupled to JSBACH, describes the emission of methane from peatlands (Raivonen et al., 2017), including production, oxidation, diffusion, plant transport, and ebullition processes in a multilayer wetland scheme. For soil organic matter (SOM) decomposition, JSBACH employs the soil carbon model YASSO (Tuomi et al., 2009; Goll et al., 2015). The specific conditions of peatlands were taken into account in YASSO, following the approach in the peatland carbon model for LPJ (Kleinen et al., 2012) and the JSBACH peatland implementation in Kleinen et al. (2020). YASSO uses four C pools for leaf and woody litter, representing the carbon fractions soluble in acid (A), water (W), and ethanol (E), as well as a non-soluble (N) fraction. A fifth carbon pool is a humus pool containing SOM that has already undergone substantial decomposition. For the application to peatlands, the humus pool was modified to represent a catotelm carbon pool containing the carbon in the permanently anoxic part of the soil column. For anaerobic decomposition in the acrotelm, a fraction of the soil column determined was below the current water table. Decomposition rates were reduced in this part of the soil column by multiplying decomposition rate constants for all C pools with a modification factor ηanox=0.35, following Wania et al. (2010). For the peatland-specific decomposition in the acrotelm, the relative mass flow magnitudes from non-soluble to acid-soluble were reduced from in the original formulation to for the peatland case. Furthermore, the mass flow magnitude from the non-soluble to the catotelm C pool was set to pN2cato=0.17 for improved peat accumulation rate. The water table level is simulated using a TOPMODEL approach (Kleinen et al., 2020), and the substrate for methane production is received from JSBACH soil anoxic respiration. Other versions of JSBACH-H have lately been developed for studying drained peatland forest management options (Tyystjärvi et al., 2024; Li et al., 2024). Methane fluxes in mineral lands are driven by soil moisture from the JSBACH hydrology model. The wet mineral soil emissions depend on the soil heterotrophic respiration from JSBACH, and a soil moisture threshold is applied for the emissions using the approach by Spahni et al. (2011). Soil sink for methane is calculated using a model by Curry et al. (2007) for methane diffusion and oxidation in dry soils. JSBACH-H was run at 0.1° resolution over the domain (Fig. 1), with land cover from EU-CORINE interpreting bogs and inland freshwater marshes as methane-emitting peatlands (HIMMELI approach) and all other lands as mineral lands. For reference, global runs were also made with 1.875° resolution following the GCP protocol (see Sect. 1.1.8).
2.2 LPX-Bern
The Land surface Processes and eXchanges (LPX-Bern) model version 1.4 (Lienert and Joos, 2018; Stocker, 2013; Spahni et al., 2013; Spahni et al., 2011) is a dynamic global vegetation model. The vegetation composition for a given land-use class is determined dynamically, allowing the different plant functional types to compete for resources. The configuration with the Dynamical Peatland model based on TOPMODEL (DYPTOP) combines an inundation model with a model that determines peatland growth conditions to simulate the peatland spatial distribution and temporal changes. DYPTOP accounts for the feedback between inundation dynamics, regional hydrology, and peatland establishment, and it estimates the distribution of peatlands versus mineral lands. The LPX-Bern model simulates peatland-specific soil carbon dynamics informed by water table position and peatland-specific vegetation classes (sphagnum and graminoids relevant for the boreal zone) and by the interaction of the carbon and nitrogen cycles. Methane production, oxidation, and transport processes are calculated according to Wania et al. (2010). Model runs were originally made with 0.5° resolution, following the GCP protocol (see Sect. 1.1.8).
2.3 LPJ-GUESS
The Lund–Potsdam–Jena General Ecosystem Simulator version 4.0 (LPJ-GUESS; Lindeskog et al., 2013; Smith et al., 2014) with methane module WHyMe (Wania et al., 2010) is a process-based dynamic vegetation and biogeochemistry model and the terrestrial biosphere component in the European community earth system model, EC-Earth-Veg (Hazeleger and Bitanja, 2012; Döscher et al., 2022). LPJ-GUESS land use is described by the Land Use Harmonization version 2 (Hurtt et al., 2020). WHyMe simulates methane production, three pathways of methane transport (diffusion, plant-mediated transport, and ebullition), and methane oxidation. LPJ-GUESS-WHyMe standalone simulations for the CRESCENDO project were run using a prescribed peatland map at a 0.5° resolution.
2.4 CLM
The Community Land Model (CLM) is the land component of the Community Earth System Model (CESM). CLM uses the biogeochemical configuration of Biome-BGC (Koven et al., 2013). CLM version 4.5 (Oleson et al., 2013) is the land component of CESM version 1.2 and of the CMCC Coupled Model version 2 (CMCC-CM2; Cherchi et al., 2019) and CMCC Earth System Model version 2 (CMCC-ESM2, Lovato et al., 2022). CLM version 5.0 (Lawrence et al., 2019) is the land component of CESM version 2 (Danabasoglu et al., 2020) and of the Norwegian Earth System Model 2 (NORESM2; Seland et al., 2020). CLM4.5 and CLM5 differ, for example, in their description of nutrient dynamics, hydrology parameterisation, root profile, nitrogen cycling, and phenology (Lawrence et al., 2019); a new feature in CLM5 is the rain threshold for growth of deciduous vegetation (Peano et al., 2021). The methane emission scheme in CLM includes production, oxidation, ebullition, diffusion, and plant transport processes in several soil layers (Meng et al., 2012; Riley et al., 2011). Methane production in the soil layers is calculated as a fraction of aerobic respiration and takes into account, for example, soil pH. Aerobic respiration depends on soil temperature, carbon content, and soil moisture. The methane oxidation rate is co-limited by oxygen concentration and methane concentration. The total emissions of a grid cell are calculated for the land area that is considered water-saturated. The saturated and unsaturated grid cell area fractions are determined according to a topographic index approach. In addition, the model explicitly takes into account multiple processes during, for example, the melting period; thus the saturated fraction can vary largely over the growing season, affecting the methane emissions.
CLM4.5 simulations were originally made at 1.25×0.9375° resolution and CLM5 at a 0.5° resolution. Results of the CLM5/NorESM2-LM coupled simulation from the CMIP6 data archive (https://esgf-node.llnl.gov/projects/cmip6/, last access: 30 October 2023) are also used in this study.
2.5 JULES
JULES-ES version 1.0 (JULES) is the Earth system configuration of the Joint UK Land Environment Simulator and the land component of the UK community Earth System Model (UKESM1; Sellar et al., 2019). The wetland methane emission in JULES is calculated from soil temperature and substrate availability, and this is then multiplied by the grid box saturated fraction (calculated using a topographic index approach) to give the grid box methane emissions (Gedney et al., 2004). Recently, the scheme was updated to calculate methane production on multiple vertical soil layers (Comyn-Platt et al., 2018). It also includes an empirical decay factor for oxidation (see Chadburn et al., 2020). Wetland JULES standalone simulations were run at a 0.5°×0.5° resolution. Results of the JULES-ES/UKESM1-0-LLES-ES-1.0 coupled simulation from the CMIP6 data archive (https://esgf-node.llnl.gov/projects/cmip6/, last access: 30 October 2023) are also used in this study.
2.6 Global Carbon Project models
The Global Carbon Project (GCP) effort for assessing global methane emissions (Saunois et al., 2020) included contributions from ecosystem models and atmospheric inversion models. The land surface model simulations followed a protocol (Saunois et al., 2020) where the models were run with the prescribed remote-sensing-based dataset, for which the wetland area and dynamics vary from year to year (Wetland Area Dynamics for Methane Modeling (WAD2M); Zhang et al., 2021), and common climate drivers. This ensemble, in which we used data from 12 models (ELM, DLEM, TEM_MDM, TRIPLEX-GHG, JSBACH, JULES, LPJ-MPI, LPJ-WSL, LPJ-GUESS, LPX-Bern, ORCHIDEE, and VISIT), is hereafter referred to as GCP-diag. We also used data from model runs where the models used their own approaches to simulate the wetland distributions. This ensemble mean of 8 models (ELM, JSBACH, JULES, LPJ-MPI, LPJ-WSL, LPX-Bern, ORCHIDEE, and VISIT) is referred to below as GCP-prog. The GCP effort included atmospheric inversion model simulations to provide a top-down view of emissions informed by atmospheric concentration observations. Here we used a mean of five inversion frameworks (TM5-4DVAR, NIES-TM, NICAM-TM 4D-VAR, GELCA, and CTE-CH4l; setups described in Saunois et al., 2020) to compare the seasonal cycle of process model wetland emissions to seasonality from inversions. Wetland methane fluxes were extracted from the flux totals by the participating research groups, and the wetland proportion of the total flux thus depends on the individual approaches chosen and on the priors used. The wetland priors for the inversions were obtained from different sources. The WETCHIMP ensemble mean (Melton et al., 2013), or, for example, the VISIT ecosystem model, was used by the inversion models listed above. In the GCP protocol, the prior wetland emission information needed for the inversions was obtained from the climatological mean of models from a previous study by Poulter et al. (2017). The GCP-prior from the protocol was used in this work as a prior for Carbon Tracker Europe–CH4 inversions, along with other priors from LPX-Bern and JSBACH-H (see Sect. 1.1.9).
2.7 Ecosystem model simulations
The experimental setups to run the ecosystem models typically include spin-up by recycling the climate mean and variability from a decadal time period in the beginning of the 20th century, along with transient carbon dioxide, climate, and land-use runs over several decades until the present day. The CRESCENDO models (JULES, CLM4.5, CLM5, and LPJ-GUESS) were run with the climate from CRUNCEP version 7 (Viovy, 2018) from 1901–2014. For the GCP models and LPX-Bern, the simulations covered the period from 1901 through the end of 2018 (GCP 2017), forced by CRU-JRA reconstructed climate fields (Harris et al., 2014). JSBACH-H was run from 1999 to 2018 with the climate from CRU-HARMONIE and globally with CRU-JRA.
Ecosystem process model results were provided on a 0.5° grid (or 0.1° for JSBACH-H). The JSBACH-H, LPX-Bern, and GCP-prior results were remapped by bilinear interpolation onto a 1×1° grid for use in CTE-CH4 atmospheric inversions. Analyses of flux results, including ecosystem process model results, atmospheric inversion results, and upscaled eddy fluxes, took place on a 1×1° grid.
2.8 CTE-CH4
Carbon Tracker Europe–CH4 (CTE-CH4) is a data assimilation system that optimises total global CH4 fluxes (Tsuruta et al., 2017), developed from Carbon Tracker Europe for CO2 (Peters et al., 2005; van der Laan-Luijkx et al., 2017). The system is based on an ensemble Kalman filter with 500 ensemble members and a fixed-lag assimilation window of 5 weeks. Atmospheric methane observation data, mostly surface in situ observations from the OBSPACK v2.0 compilation (Cooperative Global Atmospheric Data Integration Project, 2020), are assimilated into the system. In northern Europe there were over 10 atmospheric stations that continuously or semi-continuously observed methane between the years 2005 and 2018 (see Fig. 1 and Supplement Table S1). The TM5 atmospheric chemistry transport model (Krol et al., 2005) is applied to simulate the atmospheric transport of methane. The runs were forced by the ERA-Interim meteorological reanalysis (Dee et al., 2011). The prior natural surface fluxes, which are optimised by CTE-CH4, come from ecosystem process models. Results from the two models introduced above (LPX-Bern and JSBACH-H) and the mean of the GCP models were used as priors in the inversions. The fluxes are optimised on a 1×1° resolution in Europe, but here we studied the sum of emissions from a region in northern Europe (Fennoscandia), as the posterior fluxes from the inversions were better constrained over that larger region than 1×1°; i.e. the flux uncertainty becomes very large in pixel resolution given the limited number of surface stations. For anthropogenic emissions (optimised separately) we used estimates from EDGAR v5.0 (Crippa et al., 2020), for fire we used GFED v4.1s (Giglio et al., 2013), for termites we used VISIT (Ito and Inatomi, 2012), and for oceanic sources we used estimates based on ECMWF data (Tsuruta et al., 2017). The atmospheric sink was calculated using prescribed data obtained from the TM5 chemistry model (e.g. OH fields). The setup of CTE-CH4 is described in more detail in Tenkanen et al. (2021).
2.9 Upscaled flux observations
The gridded wetland flux product was based on the upscaling of observed (eddy covariance) methane fluxes (Peltola et al., 2019). Fluxes from 25 northern (>45° N) sites were used in constructing random forest models, which consist of a large number of regression trees. Random forest is a machine-learning algorithm that can be used for classification or regression analyses (Breiman, 2001). The random forest model originally had 15 explanatory input variables, e.g. temperature, precipitation, satellite data of greenness index. The upscaled product was prepared for three wetland maps: LPX-Bern DYPTOP (Stocker et al., 2014), GLWD (Lehner and Döll, 2004), and PEATMAP (Xu et al., 2018). The comparisons were made against the grid-wise mean of the three emission maps available for the years 2013 and 2014.
2.10 Climate
Meteorological data for studying temperature and precipitation responses were obtained from CRU-JRA. CRU gridded datasets are found to be suitable for vegetation analyses and are well comparable to, for example, MERRA-2 and ERA5-Land reanalysis datasets, performing well even in remote areas with few observations (Zandler et al., 2020). The CRU-JRA dataset is constructed by re-gridding reanalysis data (JRA, produced by the Japanese Meteorological Agency; Kobayashi et al., 2015), aligned with the CRU TS 4.04 data (Harris, 2019, 2020). The CRU-JRA dataset includes 0.5°×0.5° gridded 2 m temperature and total precipitation, which are used in this work.
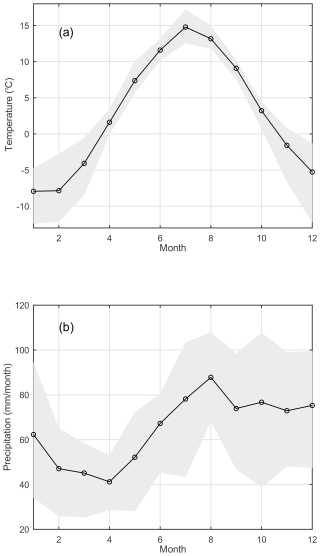
Figure 2Mean seasonal cycle of (a) temperature and (b) precipitation in Fennoscandia over the years 2000–2018 (CRU-JRA dataset, 2020). Shading refers to the highest and lowest monthly averages.
To test the effect of alternative temperature and precipitation data in addition to CRU-JRA, we studied the coupled model runs from the CMIP6 archive, where JULES was coupled with UK-ESM and CLM5 was coupled with NOR-ESM2 and thus subject to and interacting with the climate from the coupled model. The results did not change much in terms of placing the highest methane emissions in the temperature–precipitation space. Air temperature explained 76 % of the flux variation in JULES and 71 % in the coupled model run and 48 % of the flux variation in CLM5 and 37 % in the coupled model run. Precipitation explained less than 10 % of the flux variation in all model runs (Supplement Fig. S1). To further test the impact of two different bias-corrected datasets, we plotted CRU-HARMONIE and CRU-JRA against JSBACH-H results (Fig. S2), showing only small differences regarding the response of methane emissions to temperature (temperature explained 82 % of the flux variation in both cases) and precipitation (<5.5 %). It was therefore deemed appropriate to use the bias-corrected CRU-JRA dataset in our analysis.
The summer months from May to October were examined, as their mean temperatures were always above zero in Fennoscandia (Fig. 2). In May, the soil may still be in freezing or meltwater/inundation state in the northernmost parts of Fennoscandia, but, as some of the models above already produce high emissions in that month, we decided to include May in the calculation. The summer months, or length of the thermal growing season, varies considerably when moving from south to north in the study region. In the southernmost parts, it is on average >220 d long, while, in the northernmost parts, it is <100 d long (Aalto et al., 2022). Monthly average temperatures were calculated for May to October over the time period from 2000 to 2018. Average precipitation was calculated using precipitation data from the current month and 1 month before to include the delay effect in soil water content and thereby correlate better with methane emissions (see e.g. Poulter et al., 2017).
Flux correlations with precipitation and temperature were calculated using the MATLAB® statistical package. The proportion of CH4 emission variance explained by temperature (T) and precipitation (P) and both together (TP) was solved using the regress function in the statistical package, performing a least-squares fit of flux results on a linear model with temperature and precipitation as predictors (see also Chatterjee and Hadi, 1986).
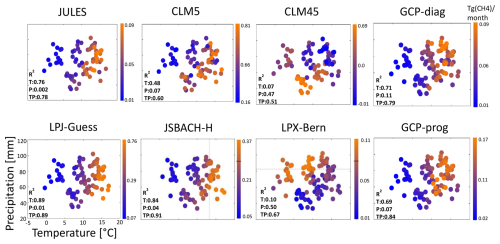
Figure 3Temperature and precipitation responses of wetland methane emissions from six ecosystem models and the mean of GCP diagnostic and prognostic models in Fennoscandia. Circles refer to monthly averages in May–October during the years 2000–2018. R2: proportion of CH4 emission variance explained by temperature (T) and precipitation (P) and both together (TP). The dashed line in the LPX-Bern figure shows the 75th percentile of precipitation values. The dashed line in the JSBACH-H figure shows the 75th percentile of temperature values. The black lines in the colour scales show the 75th percentile of flux values.
Natural wetland fluxes, including those from peatlands, wet and dry mineral lands, and inundated lands, are studied below for their growing season temperature and precipitation responses in Fennoscandia. Six process models and the mean CH4 emissions from the GCP models are included, along with results from CTE-CH4 inversions. The results are analysed in order to examine how the inversions propose to change the prior CH4 emissions and how the correlations with temperature and precipitation, and the seasonal cycle of emissions, change in the posterior.
3.1 Temperature and precipitation responses
The responses of the monthly CH4 emissions to temperature and precipitation varied among the models in Fennoscandia. According to JULES, LPJ-GUESS, JSBACH-H, and CLM5, the highest emissions coincided with high temperature (see Fig. 3), while, in CLM4.5, the highest emissions resided in the mid-temperature–low precipitation range. In LPX-Bern the highest emissions coincided with high precipitation (see Fig. 3). GCP-diag and GCP-prog had the highest emissions more evenly distributed in the high temperature–high precipitation regime, as could be expected from a mean of several models.
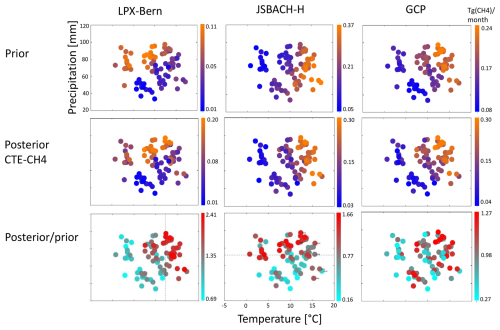
Figure 4Temperature and precipitation responses of prior, posterior, and posterior/prior methane emissions in Fennoscandia. Circles refer to monthly averages in May–October during the years 2000–2018. The line in the LPX-Bern posterior/prior figure shows the 64th percentile of temperature values and the highest flux increases from the prior (posterior/prior >2.0). The line in the JSBACH-H posterior/prior figure shows the 51st percentile of precipitation values and the highest flux increases from the prior (posterior/prior >1.0).
The regressions in Fig. 3 show the correlation of LPJ-GUESS, JSBACH-H, and JULES emissions with temperature, indicating that the variance explained was significant, as R2 values for temperature were between 0.76 and 0.89 and P-values were <0.01. Correlations with precipitation were generally weaker but still dominated over temperature in LPX-Bern () and CLM4.5 (). According to a linear statistical model (Chatterjee and Hadi, 1986) with both temperature and precipitation as predictors, air temperature and precipitation could together explain at maximum 91 % of the flux variation (JSBACH-H) but sometimes only 51 % (CLM4.5). P-values for the full model were always <0.01. Of the ecosystem models examined, temperature could explain most () of the JSBACH flux variance and precipitation could explain a large part () of the LPX-Bern flux variance. Because of these contrasting features, these two models were chosen as priors in an inversion modelling experiment. The GCP-prior (, ; see Fig. 4) was applied as a third prior for reference.
LPX-Bern, JSBACH-H, and GCP-prior were used as prior fluxes in the CTE-CH4 inversions for Fennoscandia. In total, inversions increased the emissions from LPX-Bern priors by 33 % and decreased from JSBACH-H priors by 21 %, thus bringing the flux estimates closer together. The inversion increased emissions from the GCP prior in the northern parts of Fennoscandia, where peatlands are mostly located, and reduced emissions in the southern parts, in total decreasing by 6 %. The inversions also increased emissions from the LPX-Bern prior, especially in northern Fennoscandia, while there were both decreases and increases from the JSBACH-H prior, with decreases being stronger on average (Fig. S3).
For LPX-Bern, the largest posterior increases, i.e. posterior/prior multipliers, were suggested for the high-temperature months (Fig. 4). The highest increases from the prior (posterior/prior >2.0, i.e. above the 92nd percentile of all values) occurred above a mean monthly temperature of 12.3 °C (64th percentile of all temperature values; see Fig. 4). The highest increase was proposed for July 2014, with the second-highest mean temperature of 16.7 °C. However, the July 2018 record-high heatwave with a mean temperature of 17.2 °C was not among the highest posterior increases. The precipitation was a record low, only 43 mm in July, which may explain the result if the prior did not fully capture the possible drought effect and the increase in the posterior was therefore modest. Some of the highest-precipitation months, such as August 2008 and 2016 and July–September 2007, with precipitation exceeding 100 mm, were already above average in the prior emissions but still experienced a large increase in the posterior. This could be because the inversion proposed an increase in the high-temperature regime from the prior, and temperatures during these months were above the 62nd percentile of all values.
For JSBACH-H, the largest increases were mostly proposed in the high-precipitation regime. The highest increases from the prior (above unity, i.e. above the 88th percentile of all values) occurred above 72 mm of precipitation (51st percentile; see Fig. 4). Anomalous high-precipitation periods, such as those in August 2008 and 2016 and July–September 2007, were significantly increased (> 89th percentile) in the posterior emissions, similarly to LPX-Bern. JSBACH-H predicted the largest prior emissions during the warmest months, with July 2018 being the highest, followed by July 2014, 2010, 2005, and 2006. In the posterior the fluxes were decreased, but the emissions still stayed above the 62nd percentile of all values except for 2018, which was close to average. A decrease in the soil water table may play a role, as July 2018 suffered from a lack of precipitation. The same was true for July 2014 and July 2006, but these months were not clearly distinguishable from the precipitation-abundant months July 2005 and July 2010 based on posterior emissions.
In simulations with the GCP-prior, the largest increases were appointed to months with the highest prior fluxes. The increases were rather scattered over the temperature–precipitation space. In addition, July 2018 did not show high posterior fluxes, aligning with the JSBACH-H results. The highest-precipitation months were also high in the posterior. The overall posterior emission pattern followed that of the prior. There was no bias towards high-temperature or high-precipitation regimes, which suggests a balanced prior. The temperature and precipitation correlations of the posterior fluxes were generally weaker than those of the prior for all models (Table S2). However, the correlations of the flux multiplier indicated a stronger temperature response in LPX-Bern and a stronger precipitation response in JSBACH-H than in the prior. For the GCP-prior, the flux multiplier correlations were weak.
3.2 Model components and seasonal cycle
In order to find the reasons behind the specific temperature and precipitation responses, we studied the mean seasonal cycles of the emissions and also the model components which had different seasonal cycles. The total wetland fluxes were summed up from peatland emissions, wet and dry mineral land fluxes, and emissions from inundated lands. These components cover most of the land area in the study region. However, if we regard wetland area as land area which is wet enough to emit methane, only a fraction of the land area is included in the wetland area. Wet mineral land area, inundated land area, and peatland area with a water table level close to the soil surface all contribute to the total wetland area and total wetland emissions (see Figs. S5–S8).
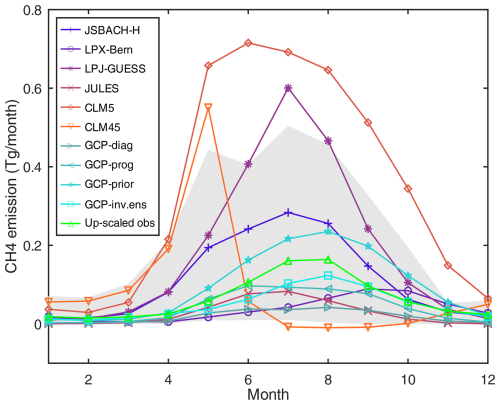
Figure 5Seasonal cycle of methane emissions in Fennoscandia according to ecosystem models; the mean of GCP diagnostic, prognostic, prior, and inversion ensemble models (Saunois et al., 2020); and upscaled eddy covariance flux observations (Peltola et al., 2019). Shading refers to the largest and smallest members of the GCP diagnostic model ensemble.
The peak of the total emissions varied from May (CLM4.5) to September (LPX-Bern); see Fig. 5. The GCP inversion results (mean of five inversion models) peaked during August, similarly to the prior. Each model presented differences in seasonal cycle and peak month, as they differed in wetland area and model process descriptions. On the contrary, the use of different climate-forcing data products or results from coupled climate simulations as drivers showed minor differences in methane emission responses (Figs. S1 and S2). We also studied the seasonal cycle of the upscaled eddy covariance flux observations (Fig. 5; see also Fig. S9). The upscaled eddy covariance flux observations (estimated from the results of Peltola et al. (2019) using the mean of the three flux maps) had a broader maximum in July–August; however, the temporal extent of the data was quite limited, only 2 years. Inspection of the model fluxes for the same time period, however, did not reveal significant differences.
JSBACH-H and LPX-Bern were studied more closely because of their contrasting temperature and precipitation dependencies (see Sect. 3.1) and differences in seasonal cycles. The LPX-Bern components for peatland fluxes and wet mineral land emissions were largest in magnitude and comparable to each other in Fennoscandia, but their seasonal cycles were somewhat different (Fig. 6). The soil moisture and consequently the wet mineral land area peaked in autumn (Fig. S8), contrary to JSBACH-H (Fig. S6); thus the wet mineral land emissions were at maximum in October in contrast to peatland emissions, which were at maximum in August. When all components were summed up, an annual cycle was created with maximum wetland emissions in September and October. JSBACH-H wetland emissions were strongly dominated by the peatland component, which had a maximum in July. Wet mineral land component had a broad maximum from August to October, and the dry mineral land sink had a maximum during the warmest summer months (July and August; see Fig. 2). All components added together suggested highest emissions in July.
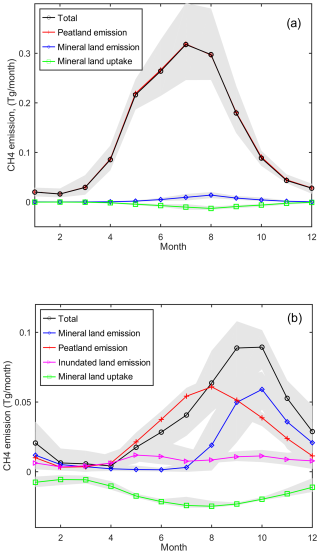
Figure 6Seasonal cycle of CH4 emission components in Fennoscandia for (a) JSBACH-H and (b) LPX-Bern. Shading refers to maximum and minimum monthly emissions over the years 2000–2018.
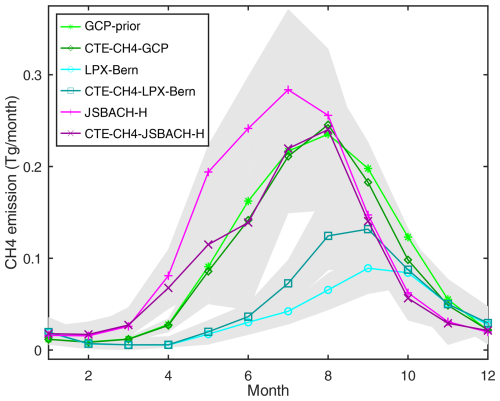
Figure 7Seasonal cycle of the wetland CH4 emissions in Fennoscandia according to the CTE-CH4 inversion model, with JSBACH-H, LPX-Bern, and GCP-prior as priors. Shading refers to maximum and minimum monthly emissions over the years 2000–2018.
The CTE-CH4 inversions moved the monthly flux maximum from July to August when JSBACH-H was used as prior in the inversion and from September–October to August–September when LPX-Bern was used as prior (Fig. 7). The flux maximum of the GCP-prior was in August and did not change in the posterior. Comparing the change maps for northern Fennoscandia, the inversion with the JSBACH-H and LPX-Bern priors positioned the fluxes to a higher level in August, while, in July, the fluxes were placed at a lower level with respect to the seasonal mean adjustment (Fig. S4). This indicates similar changes in the posterior regardless of the prior; i.e. the highest emissions were placed in August. The changes mostly took place in northern peatland areas with high methane emissions.
According to process models, air temperature and precipitation explain a large proportion of the variation in wetland methane emissions from Fennoscandia, which is not surprising given that they comprise major seasonal forcing of the models. Some models (JSBACH-H, LPJ-GUESS, and JULES) were clearly more constrained by temperature. The reason behind this behaviour is linked to strong temperature dependencies in the process descriptions (production, oxidation, transport). According to models and observations, precipitation has a dual role: it presumably increases the wetland area by wetting dry upland soils and raises the water table in the permanent wetlands. In models, weak precipitation constraint could arise from using a constant value or completely neglecting the wet mineral soil emissions or from maintaining static proportions of wet and dry mineral land area over the growing season. For JSBACH-H, the reason could possibly be in the temperature dependency of the peatland processes or wet mineral land area that is too small as opposed to dry land, and, in LPJ-GUESS, only peatland emissions were included, and they had consistently high water table levels. LPX-Bern emissions were strongly constrained by precipitation. In LPX-Bern, the wet mineral lands had a large contribution to the emissions, with the dynamic wet mineral land area being at its largest after prolonged precipitation and generally in autumn (September–October), when the modelled evapotranspiration had already decreased from high-growing-season levels. According to observations at a boreal site in Hyytiälä, Finland, however, mineral lands were wet and emitted more methane during the early season (May–July) than in August–October (Vainio et al., 2021). This suggests that the model should have drier soils in the autumn and that the modelled wet mineral soil emissions should have an earlier maximum. In total, the Hyytiälä site always acted as a sink of methane with confined emission patches. The representativity of the findings may be limited, since the observations covered only 2 years, but similar findings have been published before (e.g. Kaiser et al., 2018; Warner et al., 2019).
A modelling study by Poulter et al. (2017) concluded that, in boreal regions, CH4 emissions were best correlated with wetland area, followed by temperature and precipitation (as applied with a 1-month delay). However, according to Poulter et al. (2017), methane emissions were highly correlated with temperature in some models (e.g. JULES, similarly to our study) which had a high temperature sensitivity. In general, the increased high-latitude emissions were consistent with the increase in boreal air temperatures. Sensitivity of the boreal methane emissions to air temperature was confirmed by Koffi et al. (2020), who noted that the co-limitation of temperature and precipitation would emerge for the more southern climate zones, whereas, in our study, co-limitation was also found to be present in the boreal zone. According to Fig. 2 in Koffi et al. (2020), LPX-Bern was slightly less temperature-sensitive in the boreal zone than the other models, in agreement with the results in our study. Flux-observation-based global and northern latitude studies by Knox et al. (2019, 2021) and Peltola et al. (2019) also emphasised the importance of temperature in controlling the wetland emissions, though the water table level might become important at sites where the water table level is below the surface for a significant part of the year.
The summer months with the highest mean temperatures were not always anomalous in CTE-CH4 posterior fluxes. JSBACH-H predicted the largest prior emissions during the warmest months. In the posterior, and especially in July 2018, the fluxes were decreased, possibly because of the decrease in soil water table level, as June and July 2018 suffered from a lack of precipitation (see e.g. Peters et al., 2020). Rinne et al. (2020) also noted that methane emissions in four out of five Fennoscandian wetland sites were decreased in 2018 due to a decrease in water table levels. This suggests that many wetlands suffered from drought, which could have affected the regional results. The summer months with high precipitation often resulted in high posterior emissions. August was the month of the average seasonal precipitation maximum, while the average temperature maximum was in July. Large increases in the posterior were assigned to high-precipitation periods, e.g. in August 2008 and 2016 and in late summer 2007; however, the year 2011, with observed high methane emissions from upland soil in northern Fennoscandia (Lohila et al., 2016), did not stand out in posterior emissions. It is possible that the signal observed by Lohila et al. (2016) was not seen in the larger region of this study but only in northern Fennoscandia.
The CTE-CH4 inversions quite unanimously attempted to move the seasonal maximum of the emissions towards August. This is supported by the GCP inversion ensemble and the observation-based upscaled eddy covariance fluxes mapped over Fennoscandia. Warwick et al. (2016) also found that seasonal cycles of methane mixing ratios at northern high latitudes above 50° N were improved when the seasonal maximum in northern high-latitude wetland emissions predicted by process models was delayed by 1 month from July to August. In our work, many models had their seasonal maxima in July or August, notable exceptions being CLM4.5 and CLM5 (bias towards spring) and LPX-Bern (bias towards autumn). The dominance of mineral land over peatland emissions (such as in LPX-Bern) may delay the month of the maximum emissions and use a large wetland extent in late summer. Placing more peatlands in the southern parts of the region (such as in GCP-diag) or having a weak temperature response may possibly result in a longer seasonal emission maximum. A pronounced inundation period after snowmelt could induce large methane emissions in spring (such as in CLM4.5 and CLM 5). Phenology may also play a role; however, the modelled start of the growing season was usually delayed from satellite observations in northern latitudes (Peano et al., 2021), which means that the early methane emission maximum is not caused by an overly early start in model photosynthesis. According to flux measurements at boreal peatlands, the month of highest emissions was July or August depending on the year (e.g. Rinne et al., 2020). Lake emissions are often not present in process models but are seen by inversions. They might delay the emission maximum in dry years when wetland emissions diminish towards the end of the summer due to the decreasing soil water table level but lake emissions continue. The CTE-CH4 posterior fluxes, the GCP model ensembles, and the observation-based upscaled eddy covariance fluxes all indicate an emission maximum in August. This provides a coherent view of the seasonality in contrast to the large variation in ecosystem model results.
The ecosystem models showed variable responses of methane emissions to temperature and precipitation for the Fennoscandia region. However, multi-model means, inversions, and upscaled eddy covariance flux observations agreed on the month of maximum emissions and had rather balanced temperature and precipitation responses (i.e. both temperature and precipitation explain the variance in fluxes) which were not significantly changed from prior to posterior in inversions. When two models with contrasting response patterns were used as priors to inversion, the inversion attempted to move emissions of both in posterior towards co-limitation of temperature and precipitation, i.e. to be sensitive to changes in both environmental variables.
The setup of different emission components (peatland emissions and mineral land fluxes) is important in building up the response patterns, as they contribute to the total flux seen by inversions. Peatland emissions determine the month of maximum emissions in the models that are more sensitive to temperature, while wet mineral soil emissions determine the timing of the maximum in the case of strong precipitation sensitivity. This applies to average response patterns when data from several years are used to determine the responses, noting that the models are sensitive to precipitation in the cases of severe seasonal droughts leading to water table drawdown in peatlands, which in turn leads to reductions in methane emissions. Depending on the model, wet mineral soil and inundated land emissions can modify the seasonality of methane emissions together with peatland emissions. Peatland emissions are considered to be the main component of methane emissions, and wet mineral lands are often not considered to be as important. However, despite the smaller emission per unit area, the wet regions emitting methane can be very large; thus they can change the seasonality of the regional emissions. Furthermore, the wet mineral lands are implemented in different ways in the models and thus may produce different outcomes for the total flux. Therefore, it is essential to pay more attention to the role of the individual emission components, their magnitude, their annual cycle, and their spatial extent in different regions and, in general, to consider how the fluxes should be scaled up from site to region (see also Bansal et al., 2023; Knox et al., 2021; Treat et al., 2018; Tuovinen et al., 2019). To perform well in the Fennoscandia region, it is expected that a model will need to consider peatland and mineral land source and sink components of methane emissions and use up-to-date land cover description. If the peatland extent is simulated by the model, it needs to be validated against land cover data from bottom-up inventories. Similarly, for soil wetness or inundation, the model should be validated against satellite data wherever possible. The models should use process-based descriptions for both mineral and peat soil fluxes to simulate the flux responses to climate drivers. Furthermore, it is important to study the overall responses of the total emissions to air temperature and precipitation, as they define the response of wetland emissions to climate change.
The data processed for this study will be available on the FMI Research Data Repository METIS (https://doi.org/10.57707/FMI-B2SHARE.23392F65B1354D49ABD5146AA5589F9B, Aalto, 2025).
The supplement related to this article is available online at: https://doi.org/10.5194/bg-22-323-2025-supplement.
TA designed the study, processed and analysed the data, and wrote the paper; AT, JaM, MT, and VM processed and analysed data; AT, JuM, EB, SC, HL, AL, TM, SM, PAM, DP, OP, BP, MR, MS, DW, and SZ performed model simulations and/or made simulation data available; and AT, JaM, JuM, MT, VM, YG, TK, HL, AL, TM, SM, PM, DP, OP, BP, MR, MS, DW, and SZ reviewed and commented on the paper.
At least one of the (co-)authors is a member of the editorial board of Biogeosciences. The peer-review process was guided by an independent editor, and the authors also have no other competing interests to declare.
Publisher’s note: Copernicus Publications remains neutral with regard to jurisdictional claims made in the text, published maps, institutional affiliations, or any other geographical representation in this paper. While Copernicus Publications makes every effort to include appropriate place names, the final responsibility lies with the authors.
The FMI authors would like to thank national and EU projects for financial support (see details below). Thomas Kleinen acknowledges support from the German Federal Ministry of Education and Research (BMBF) through the project Palmod, grant no. 01LP1921A. CH4 in situ observations collected over the US Southern Great Plains were supported by the Office of Biological and Environmental Research of the US Department of Energy under grant no. DE-AC02-05CH11231 as part of the Atmospheric Radiation Measurement (ARM) programme, the ARM Aerial Facility (AAF), and the Terrestrial Ecosystem Science (TES) programme. We acknowledge the data from the Global Methane Budget 2000–2017 (2020). The authors would like to thank the atmospheric data providers (see Table S1) and especially ICOS Finland, Sweden, and Norway for providing the data on atmospheric methane concentrations. We thank the PIs Juha Hatakka, Tuomas Laurila, and Hermanni Aaltonen (PAL, UTO, KMP, SOD); Ari Leskinen and Kari Lehtinen (PUI); Janne Levula and Ivan Mammarella (SMR); Per Marklund, Mikaell Ottosson-Löfvenius, and Eric Larmanou (SVB); Michal Heliasz and Tobias Biermanns (HTM); Irene Lehner and Meelis Mölder (NOR); Cathrine Lund Myhre, Stephen M. Platt, Ove Hermansen, and Chris Lunder (BIR); and Elena Kozlova and Andrew Manning (KJN) for providing the methane data.
This research has been supported by the Academy of Finland Centre of Excellence (grant no. 272041); Flagships ACCC (grant no. 337552) and FAME (grant no. 359196); FIRI – ICOS Finland (grant no. 345531); UPFORMET (grant no. 307331); CHARM (grant no. 364975), GHGSUPER (grant no. 351311); the European Space Agency ESRIN (grant nos. 4000125046/18/I-NB MethEO, 4000137895/22/IAG MethaneCAMP, AO/1-10901/21/I-DT AMPAC-Net, and ESA AO/1-11844/23/I-NS ESA SMART-CH4); JTF (VISIO); CSC (FICOCOSS); the EUH2020 and Horizon project grants CRESCENDO (grant no. 641816), VERIFY (grant no. 776810), ALFAWETLANDS (grant no. 101056844), WETHORIZONS (grant no. 101056848), EYE-CLIMA (grant no. 101081395), and IM4CA (grant no. 101183460); and the EU LIFE+ project (LIFE21-CCMLV-LIFE PeatCarbon, grant no. 101074396).
This paper was edited by Daniel S. Goll and reviewed by two anonymous referees.
Aalto, J., Pirinen, P., Kauppi, P. E., Rantanen, M., Lussana, C., Lyytikäinen-Saarenmaa, P., and Gregow, H.: High-resolution analysis of observed thermal growing season variability over northern Europe, Clim. Dynam., 58, 1477–1493, https://doi.org/10.1007/s00382-021-05970-y, 2022.
Aalto, T.: Model data for “Air temperature and precipitation constraining the modelled wetland methane emissions in a boreal region in Northern Europe”, Finnish Meteorological Institute [data set], https://doi.org/10.57707/FMI-B2SHARE.23392F65B1354D49ABD5146AA5589F9B, 2025.
Albuhaisi, Y. A. Y., van der Velde, Y., and Houweling, S.: The Importance of Spatial Resolution in the Modeling of Methane Emissions from Natural Wetlands, Remote Sens., 15, 2840, https://doi.org/10.3390/rs15112840, 2023.
Bansal, S., Post van der Burg, M., Fern, R. R., Jones, J. W., Lo, R., McKenna, O. P., Tangen, B. A., Zhang, Z., and Gleason, R. A.: Large increases in methane emissions expected from North America's largest wetland complex, Sci. Adv., 9, eade1112, https://doi.org/10.1126/sciadv.ade1112, 2023.
Battaglia, M. J., Banks, S., Behnamian, A., Bourgeau-Chavez, L., Brisco, B., Corcoran, J., Chen, Z., Huberty, B., Klassen, J., Knight, J., Morin, P., Murnaghan, K., Pelletier, K., and White, L.: Multi-Source EO for Dynamic Wetland Mapping and Monitoring in the Great Lakes Basin, Remote Sens., 13, 599, https://doi.org/10.3390/rs13040599, 2021.
Breiman, L.: Random Forests, Mach. Learn., 45, 5–32, https://doi.org/10.1023/A:1010933404324, 2001.
Chadburn, S. E., Aalto, T., Aurela, M., Baldocchi, D., Biasi, C., Boike, J., Burke, E. J., Comyn-Platt, E., Dolman, A.J., Duran-Rojas, C., Fan, Y., Friborg, T., Gao, Y., Gedney, N., Göckede, M., Hayman, G. D., Holl, D., Hugelius, G., Kutzbach, L., Lee, H., Lohila, A., Parmentier, F.-J. W., Sachs, T., Shurpali, N. J., and Westermann, S.: Modeled Microbial Dynamics Explain the Apparent Temperature Sensitivity of Wetland Methane Emissions, Global Biogeochem. Cy., 34, e2020GB006678, https://doi.org/10.1029/2020GB006678, 2020.
Chapman, B., Hess, L., and Lucas, R.: Remote Sensing of Water in Wetlands: Inundation Patterns and Extent, in: The Wetland Book: I: Structure and Function, Management and Methods, edited by: Finlayson, C. M., Everard, M., Irvine, K., McInnes, R. J., Middleton, B. A., van Dam, A. A., and Davidson, N. C., Springer Netherlands, Dordrecht, 1–9, https://doi.org/10.1007/978-94-007-6172-8_317-1, 2016.
Chatterjee, S. and Hadi, A. S.: Influential Observations, High Leverage Points, and Outliers in Linear Regression, Stat. Sci., 1, 379–416, 1986.
Riley, W. J., Subin, Z. M., Lawrence, D. M., Swenson, S. C., Torn, M. S., Meng, L., Mahowald, N. M., and Hess, P.: Barriers to predicting changes in global terrestrial methane fluxes: analyses using CLM4Me, a methane biogeochemistry model integrated in CESM, Biogeosciences, 8, 1925–1953, https://doi.org/10.5194/bg-8-1925-2011, 2011.
Cohen, J., Riihimäki, H., Pulliainen, J., Lemmetyinen, J., and Heilimo, J.: Implications of boreal forest stand characteristics for X-band SAR flood mapping accuracy, Remote Sens. Environ., 186, 47–63, https://doi.org/10.1016/j.rse.2016.08.016, 2016.
Cooperative Global Atmospheric Data Integration Project: Multi-laboratory compilation of atmospheric methane data for the period 1957–2018, obspack_ch4_1_GLOBALVIEWplus_v2.0_2020-04-24, NOAA Earth System Research Laboratory, Global Monitoring Division, https://doi.org/10.25925/20200424, 2020.
Comyn-Platt, E., Hayman, G., Huntingford, C., Chadburn, S. E., Burke, E. J., Harper, A. B., Collins, W. J., Webber, C. P., Powell, T., Cox, P. M., Gedney, N., and Sitch, S.: Carbon budgets for 1.5 and 2 ° C targets lowered by natural wetland and permafrost feedbacks, Nat. Geosci., 11, 568–573, https://doi.org/10.1038/s41561-018-0174-9, 2018.
CRU-JRA dataset: University of East Anglia Climatic Research Unit and Harris, I. C.: CRU JRA v2.0: A forcings dataset of gridded land surface blend of Climatic Research Unit (CRU) and Japanese reanalysis (JRA) data, Jan. 1901 – Dec. 2018, Centre for Environmental Data Analysis, https://catalogue.ceda.ac.uk/uuid/7f785c0e80aa4df2b39d068ce7351bbb (last access: 30 October 2023), 2020.
Curry, C. L.: Modeling the soil consumption of atmospheric methane at the global scale, Global Biogeochem. Cy. 21, https://doi.org/10.1029/2006GB002818, 2007.
Dee, D. P., Uppala, S. M., Simmons, A. J., Berrisford, P., Poli, P., Kobayashi, S., Andrae, U., Balmaseda, M. A., Balsamo, G., Bauer, P., Bechtold, P., Beljaars, A. C. M., van de Berg, L., Bidlot, J., Bormann, N., Delsol, C., Dragani, R., Fuentes, M., Geer, A. J., Haimberger, L., Healy, S. B., Hersbach, H., Hólm, E. V., Isaksen, L., Kållberg, P., Köhler, M., Matricardi, M., McNally, A. P., Monge-Sanz, B.M., Morcrette, J.-J., Park, B.-K., Peubey, C., de Rosnay, P., Tavolato, C., Thépaut, J.-N., and Vitart, F.: The ERA-Interim reanalysis: configuration and performance of the data assimilation system, Q. J. Roy. Meteor. Soc., 137, 553–597, https://doi.org/10.1002/qj.828, 2011.
Crippa, M., Solazzo, E., Huang, G., Guizzardi, D., Koffi, E., Muntean, M., Schieberle, C., Friedrich, R., and Janssens-Maenhout, G: High resolution temporal profiles in the Emissions Database for Global Atmospheric Research, Sci. Data, 7, 121, https://doi.org/10.1038/s41597-020-0462-2, 2020.
Danabasoglu, G., Lamarque, J.-F., Bacmeister, J., Bailey, D. A., DuVivier, A. K. Edwards, J., Emmons, L. K., Fasullo, J., Garcia, R., Gettelman, A., Hannay, C., Holland, M. M., Large, W. G., Lauritzen, P. H., Lawrence, D. M., Lenaerts, J. T. M., Lindsay, K., Lipscomb, W. H., Mills, M. J., Neale, R., Oleson, K. W., Otto-Bliesner, B., Phillips, A. S., Sacks, W., Tilmes, S., van Kampenhout, L., Vertenstein, M., Bertini, A., Dennis, J., Deser, C., Fischer, C., Fox-Kemper, B., Kay, J. E., Kinnison, D., Kushner, P. J., Larson, V. E., Long, M. C., Mickelson, S., Moore, J. K., Nienhouse, E., Polvani, L., Rasch, P. J., and Strand, W. G.: The Community Earth System Model Version 2 (CESM2), J. Adv. Model. Earth Sy., 12, e2019MS001916, https://doi.org/10.1029/2019MS001916, 2020.
Döscher, R., Acosta, M., Alessandri, A., Anthoni, P., Arsouze, T., Bergman, T., Bernardello, R., Boussetta, S., Caron, L.-P., Carver, G., Castrillo, M., Catalano, F., Cvijanovic, I., Davini, P., Dekker, E., Doblas-Reyes, F. J., Docquier, D., Echevarria, P., Fladrich, U., Fuentes-Franco, R., Gröger, M., v. Hardenberg, J., Hieronymus, J., Karami, M. P., Keskinen, J.-P., Koenigk, T., Makkonen, R., Massonnet, F., Ménégoz, M., Miller, P. A., Moreno-Chamarro, E., Nieradzik, L., van Noije, T., Nolan, P., O'Donnell, D., Ollinaho, P., van den Oord, G., Ortega, P., Prims, O. T., Ramos, A., Reerink, T., Rousset, C., Ruprich-Robert, Y., Le Sager, P., Schmith, T., Schrödner, R., Serva, F., Sicardi, V., Sloth Madsen, M., Smith, B., Tian, T., Tourigny, E., Uotila, P., Vancoppenolle, M., Wang, S., Wårlind, D., Willén, U., Wyser, K., Yang, S., Yepes-Arbós, X., and Zhang, Q.: The EC-Earth3 Earth system model for the Coupled Model Intercomparison Project 6, Geosci. Model Dev., 15, 2973–3020, https://doi.org/10.5194/gmd-15-2973-2022, 2022.
Eyring, V., Bony, S., Meehl, G. A., Senior, C. A., Stevens, B., Stouffer, R. J., and Taylor, K. E.: Overview of the Coupled Model Intercomparison Project Phase 6 (CMIP6) experimental design and organization, Geosci. Model Dev., 9, 1937–1958, https://doi.org/10.5194/gmd-9-1937-2016, 2016.
Gao, Y., Markkanen, T., Thum, T., Aurela, M., Lohila, A., Mammarella, I., Kämäräinen, M., Hagemann, S., and Aalto, T.: Assessing various drought indicators in representing summer drought in boreal forests in Finland, Hydrol. Earth Syst. Sci., 20, 175–191, https://doi.org/10.5194/hess-20-175-2016, 2016.
Gedney, N., Cox, P. M., and Huntingford, C.: Climate feedback from wetland methane emissions, Geophys. Res. Let., 31, 20, https://doi.org/10.1029/2004GL020919, 2004.
Giglio, L., Randerson, J. T., and van derWerf, G. R.: Analysis of daily, monthly, and annual burned area using the fourth-generation global fire emissions database (GFED4), J. Geophys. Res.-Biogeo., 118, 317–328, https://doi.org/10.1002/jgrg.20042, 2013.
Goll, D. S., Brovkin, V., Liski, J., Raddatz, T., Thum, T., and Todd-Brown, K. E. O.: Strong Dependence of CO2 Emissions from Anthropogenic Land Cover Change on Initial Land Cover and Soil Carbon Parametrization, Global Biogeochem. Cy., 29, 1511–1523, https://doi.org/10.1002/2014GB004988, 2015.
Guo, M., Zhuang, Q., Tan, Z., Shurpali, N., Juutinen, S., Kortelainen, P., and Martikainen, P. J.: Rising methane emissions from boreal lakes due to increasing ice-free days, Environ. Res. Lett. 15, 064008, https://doi.org/10.1088/1748-9326/ab8254, 2020.
Hagemann, S. and Stacke, T.: Impact of the soil hydrology scheme on simulated soil moisture memory, Clim. Dynam., 44, 1731–1750, https://doi.org/10.1007/s00382-014-2221-6, 2015.
Harris, I., Jones, P. D., Osborn, T. J., and Lister, D. H.: Updated high-resolution grids of monthly climatic observations – the CRU TS3.10 Dataset, Int. J. Climatol., 34, 623–642, 2014.
Harris, I., Osborn, T. J., Jones, P., and Lister, D.: Version 4 of the CRU TS monthly high-resolution gridded multivariate climate dataset, Sci. Data, 7, 109, https://doi.org/10.1038/s41597-020-0453-3, 2020.
Hazeleger, W. and Bintanja, R.: Studies with the EC-Earth seamless earth system prediction model, Clim. Dynam., 39, 2609–2610, https://doi.org/10.1007/s00382-012-1577-8, 2012.
Helbig, M., Waddington, J. M., Alekseychik, P., Amiro, B. D., Aurela, M., Barr, A. G., Black, T. A., Blanken, P. D., Carey, S. K., Chen, J., Chi, J., Desai, A. R., Dunn, A., Euskirchen, E. S., Flanagan, L. B., Forbrich, I., Friborg, T., Grelle, A., Harder, S., Heliasz, M., Humphreys, E. R., Ikawa, H., Isabelle, P.-E., Iwata, H., Jassal, R., Korkiakoski, M., Kurbatova, J., Kutzbach, L., Lindroth, A., Löfvenius, M. O., Lohila, A., Mammarella, I., Marsh, P., Maximov, T., Melton, J. R., Moore, P. A., Nadeau, D. F., Nicholls, E. M., Nilsson, M. B., Ohta, T., Peichl, M., Petrone, R. M., Petrov, R., Prokushkin, A., Quinton, W. L., Reed, D. E., Roulet, N. T., Runkle, B. R. K., Sonnentag, O., Strachan, I. B., Taillardat, P., Tuittila, E.-S., Tuovinen, J.-P., Turner, J., Ueyama, M., Varlagin, A., Wilmking, M., Wofsy, S. C., and Zyrianov, V.: Increasing contribution of peatlands to boreal evapotranspiration in a warming climate, Nat. Clim. Change, 10, 555–560, https://doi.org/10.1038/s41558-020-0763-7, 2020.
Hurtt, G. C., Chini, L., Sahajpal, R., Frolking, S., Bodirsky, B. L., Calvin, K., Doelman, J. C., Fisk, J., Fujimori, S., Klein Goldewijk, K., Hasegawa, T., Havlik, P., Heinimann, A., Humpenöder, F., Jungclaus, J., Kaplan, J. O., Kennedy, J., Krisztin, T., Lawrence, D., Lawrence, P., Ma, L., Mertz, O., Pongratz, J., Popp, A., Poulter, B., Riahi, K., Shevliakova, E., Stehfest, E., Thornton, P., Tubiello, F. N., van Vuuren, D. P., and Zhang, X.: Harmonization of global land use change and management for the period 850–2100 (LUH2) for CMIP6, Geosci. Model Dev., 13, 5425–5464, https://doi.org/10.5194/gmd-13-5425-2020, 2020.
Ito, A. and Inatomi, M.: Use of a process-based model for assessing the methane budgets of global terrestrial ecosystems and evaluation of uncertainty, Biogeosciences, 9, 759–773, https://doi.org/10.5194/bg-9-759-2012, 2012.
Ito, A., Hajima, T., Lawrence, D.M., Brovkin, V., Delire, C., Guenet, B., Jones, C. D., Malyshev, S., Materia, S., McDermid, S. P., Peano, D., Pongratz, J., Robertson, E., Shevliakova, E., Vuichard, N., Wårlind, D., Wiltshire, A., and Ziehn, T.: Soil carbon sequestration simulated in CMIP6-LUMIP models: implications for climatic mitigation, Environ. Res. Lett., 15, 124061, https://doi.org/10.1088/1748-9326/abc912, 2020.
Kaiser, K. E., McGlynn, B. L., and Dore, J. E.: Landscape analysis of soil methane flux across complex terrain, Biogeosciences, 15, 3143–3167, https://doi.org/10.5194/bg-15-3143-2018, 2018.
Kleinen, T., Brovkin, V., and Schuldt, R. J.: A dynamic model of wetland extent and peat accumulation: results for the Holocene, Biogeosciences, 9, 235–248, https://doi.org/10.5194/bg-9-235-2012, 2012.
Kleinen, T., Mikolajewicz, U., and Brovkin, V.: Terrestrial methane emissions from the Last Glacial Maximum to the preindustrial period, Clim. Past, 16, 575–595, https://doi.org/10.5194/cp-16-575-2020, 2020.
Knox, S. H., Jackson, R. B., Poulter, B., McNicol, G., Fluet-Chouinard, E., Zhang, Z., Hugelius, G., Bousquet, P., Canadell, J. G., Saunois, M., Papale, D., Chu, H., Keenan, T. F., Baldocchi, D., Torn, M. S., Mammarella, I., Trotta, C., Aurela, M., Bohrer, G., Campbell, D. I., Cescatti, A., Chamberlain, S., Chen, J., Chen, W., Dengel, S., Desai, A. R., Euskirchen, E., Friborg, T., Gasbarra, D., Goded, I., Goeckede, M., Heimann, M., Helbig, M., Hirano, T., Hollinger, D. Y., Iwata, H., Kang, M., Klatt, J., Krauss, K. W., Kutzbach, L., Lohila, A., Mitra, B., Morin, T. H., Nilsson, M. B., Niu, S., Noormets, A., Oechel, W. C., Peichl, M., Peltola, O., Reba, M. L., Richardson, A. D., Runkle, B. R. K., Ryu, Y., Sachs, T., Schäfer, K. V. R., Schmid, H. P., Shurpali, N., Sonnentag, O., Tang, A. C. I., Ueyama, M., Vargas, R., Vesala, T., Ward, E. J., Windham-Myers, L., Wohlfahrt, G., and Zona, D.: FLUXNET-CH4 Synthesis Activity: Objectives, Observations, and Future Directions, B. Am. Meteor. Soc., 100, 2607–2632, https://doi.org/10.1175/BAMS-D-18-0268.1, 2019.
Knox, S. H., Bansal, S., McNicol, G., Schafer, K., Sturtevant, C., Ueyama, M., Valach, A. C., Baldocchi, D., Delwiche, K., Desai, A. R., Euskirchen, E., Liu, J., Lohila, A., Malhotra, A., Melling, L., Riley, W., Runkle, B. R. K., Turner, J., Vargas, R., Zhu, Q., Aalto, T., Fluet-Chouinard, E., Goeckede, M., Melton, J. R., Sonnentag, O., Vesala, T., Ward, E., Zhang, Z., Feron, S., Ouyang, Z., Alekseychik, P., Aurela, M., Bohrer, G., Campbell, D. I., Chen, J., Chu, H., Dalmagro, H. J., Goodrich, J. P., Gottschalk, P., Hirano, T., Iwata, H., Jurasinski, G., Kang, M., Koebsch, F., Mammarella, I., Nilsson, M. B., Ono, K., Peichl, M., Peltola, O., Ryu, Y., Sachs, T., Sakabe, A., Sparks, J. P., Tuittila, E.-S., Vourlitis, G. L., Wong, G. X., Windham-Myers, L., Poulter, B., and Jackson, R. B.: Identifying dominant environmental predictors of freshwater wetland methane fluxes across diurnal to seasonal time scales, Global Change Biol., 27, 3581–3604, https://doi.org/10.1111/gcb.15661, 2021.
Kobayashi, S., Ota, Y., Harada, Y., Ebita, A., Moriya, M., Onoda, H., Onogi, K., Kamahori, H., Kobayashi, C., Endo, H., Miyaoka, K., and Takahashi, K.: The JRA-55 Reanalysis: General Specifications and Basic Characteristics, J.mMeteor. Soc. Jpn. II, 93, 5–48, https://doi.org/10.2151/jmsj.2015-001, 2015.
Koffi, E. N., Bergamaschi, P., Alkama, R., and Cescatti, A.: An observation-constrained assessment of the climate sensitivity and future trajectories of wetland methane emissions, Sci. Adv. 6, eaay4444, https://doi.org/10.1126/sciadv.aay4444, 2020.
Koven, C. D., Riley, W. J., Subin, Z. M., Tang, J. Y., Torn, M. S., Collins, W. D., Bonan, G. B., Lawrence, D. M., and Swenson, S. C.: The effect of vertically resolved soil biogeochemistry and alternate soil C and N models on C dynamics of CLM4, Biogeosciences, 10, 7109–7131, https://doi.org/10.5194/bg-10-7109-2013, 2013.
Krol, M., Houweling, S., Bregman, B., van den Broek, M., Segers, A., van Velthoven, P., Peters, W., Dentener, F., and Bergamaschi, P.: The two-way nested global chemistry-transport zoom model TM5: algorithm and applications, Atmos. Chem. Phys., 5, 417–432, https://doi.org/10.5194/acp-5-417-2005, 2005.
Lawrence, D. M., Fisher, R. A., Koven, C. D., Oleson, K. W., Swenson, S. C., Bonan, G., Collier, N., Ghimire, B., Kampenhout, L. van, Kennedy, D., Kluzek, E., Lawrence, P. J., Li, F., Li, H., Lombardozzi, D., Riley, W. J., Sacks, W. J., Shi, M., Vertenstein, M., Wieder, W. R., Xu, C., Ali, A. A., Badger, A. M., Bisht, G., Broeke, M. van den, Brunke, M. A., Burns, S. P., Buzan, J., Clark, M., Craig, A., Dahlin, K., Drewniak, B., Fisher, J. B., Flanner, M., Fox, A. M., Gentine, P., Hoffman, F., Keppel-Aleks, G., Knox, R., Kumar, S., Lenaerts, J., Leung, L. R., Lipscomb, W. H., Lu, Y., Pandey, A., Pelletier, J. D., Perket, J., Randerson, J. T., Ricciuto, D. M., Sanderson, B. M., Slater, A., Subin, Z. M., Tang, J., Thomas, R. Q., Martin, M. V., and Zeng, X.: The Community Land Model Version 5: Description of New Features, Benchmarking, and Impact of Forcing Uncertainty, J. Adv. Model. Earth Sy., 11, 4245–4287, https://doi.org/10.1029/2018MS001583, 2019.
Lehner, B. and Döll, P.: Development and validation of a global database of lakes, reservoirs and wetlands, J. Hydrol., 296, 1–22, https://doi.org/10.1016/j.jhydrol.2004.03.028, 2004.
Li, X., Markkanen, T., Korkiakoski, M., Lohila, A., Leppänen, A., Aalto, T., Peltoniemi, M., Mäkipää, R., Kleinen, T., and Raivonen, M.: Modelling alternative harvest effects on soil CO2 and CH4 fluxes from peatland forests, Sci. Total Environ., 951, 175257, https://doi.org/10.1016/j.scitotenv.2024.175257, 2024.
Lienert, S. and Joos, F.: A Bayesian ensemble data assimilation to constrain model parameters and land-use carbon emissions, Biogeosciences, 15, 2909–2930, https://doi.org/10.5194/bg-15-2909-2018, 2018.
Lindeskog, M., Arneth, A., Bondeau, A., Waha, K., Seaquist, J., Olin, S., and Smith, B.: Implications of accounting for land use in simulations of ecosystem carbon cycling in Africa, Earth Syst. Dynam., 4, 385–407, https://doi.org/10.5194/esd-4-385-2013, 2013.
Lohila, A., Aalto, T., Aurela, M., Hatakka, J., Tuovinen, J.-P., Kilkki, J., Penttilä, T., Vuorenmaa, J., Hänninen, P., Sutinen, R., Viisanen, Y., and Laurila, T.: Large contribution of boreal upland forest soils to a catchment-scale CH4 balance in a wet year, Geophys. Res. Lett., 43, 2946–2953, https://doi.org/10.1002/2016GL067718, 2016.
Loisel, J., Gallego-Sala, A. V., Amesbury, M. J., Magnan, G., Anshari, G., Beilman, D. W., Benavides, J. C., Blewett, J., Camill, P., Charman, D. J., Chawchai, S., Hedgpeth, A., Kleinen, T., Korhola, A., Large, D., Mansilla, C. A., Müller, J., van Bellen, S., West, J. B., Yu, Z., Bubier, J. L., Garneau, M., Moore, T., Sannel, A. B. K., Page, S., Väliranta, M., Bechtold, M., Brovkin, V., Cole, L. E. S., Chanton, J. P., Christensen, T. R., Davies, M. A., De Vleeschouwer, F., Finkelstein, S. A., Frolking, S., Gałka, M., Gandois, L., Girkin, N., Harris, L. I., Heinemeyer, A., Hoyt, A. M., Jones, M. C., Joos, F., Juutinen, S., Kaiser, K., Lacourse, T., Lamentowicz, M., Larmola, T., Leifeld, J., Lohila, A., Milner, A. M., Minkkinen, K., Moss, P., Naafs, B. D. A., Nichols, J., O'Donnell, J., Payne, R., Philben, M., Piilo, S., Quillet, A., Ratnayake, A. S., Roland, T. P., Sjögersten, S., Sonnentag, O., Swindles, G. T., Swinnen, W., Talbot, J., Treat, C., Valach, A. C., and Wu, J.: Expert assessment of future vulnerability of the global peatland carbon sink, Nat. Clim. Change, 11, 70–77, https://doi.org/10.1038/s41558-020-00944-0, 2021.
Lovato, T., Peano, D., Butenschön, M., Materia, S., Iovino, D., Scoccimarro, E., Fogli, P. G., Cherchi, A., Bellucci, A., Gualdi, S., Masina, S., and Navarra, A.: CMIP6 Simulations With the CMCC Earth System Model (CMCC-ESM2), J. Adv. Model. Earth Sy., 14, e2021MS002814, https://doi.org/10.1029/2021MS002814, 2022.
Mahoney, C., Merchant, M., Boychuk, L., Hopkinson, C., and Brisco, B.: Automated SAR Image Thresholds for Water Mask Production in Alberta's Boreal Region, Remote Sens., 12, 2223, https://doi.org/10.3390/rs12142223, 2020.
Mauritsen, T., Bader, J., Becker, T., Behrens, J., Bittner, M., Brokopf, R., Brovkin, V., Claussen, M., Crueger, T., Esch, M., Fast, I., Fiedler, S., Fläschner, D., Gayler, V., Giorgetta, M., Goll, D. S., Haak, H., Hagemann, S., Hedemann, C., Hohenegger, C., Ilyina, T., Jahns, T., Jimenéz-de-la-Cuesta, D., Jungclaus, J., Kleinen, T., Kloster, S., Kracher, D., Kinne, S., Kleberg, D., Lasslop, G., Kornblueh, L., Marotzke, J., Matei, D., Meraner, K., Mikolajewicz, U., Modali, K., Möbis, B., Müller, W. A., Nabel, J. E. M. S., Nam, C. C. W., Notz, D., Nyawira, S.-S., Paulsen, H., Peters, K., Pincus, R., Pohlmann, H., Pongratz, J., Popp, M., Raddatz, T. J., Rast, S., Redler, R., Reick, C. H., Rohrschneider, T., Schemann, V., Schmidt, H., Schnur, R., Schulzweida, U., Six, K. D., Stein, L., Stemmler, I., Stevens, B., Storch, J.-S. von, Tian, F., Voigt, A., Vrese, P., Wieners, K.-H., Wilkenskjeld, S., Winkler, A., and Roeckner, E.: Developments in the MPI-M Earth System Model version 1.2 (MPI-ESM1.2) and Its Response to Increasing CO2, J. Adv. Model. Earth Sy., 11, 998–1038, https://doi.org/10.1029/2018MS001400, 2019.
Melton, J. R., Wania, R., Hodson, E. L., Poulter, B., Ringeval, B., Spahni, R., Bohn, T., Avis, C. A., Beerling, D. J., Chen, G., Eliseev, A. V., Denisov, S. N., Hopcroft, P. O., Lettenmaier, D. P., Riley, W. J., Singarayer, J. S., Subin, Z. M., Tian, H., Zürcher, S., Brovkin, V., van Bodegom, P. M., Kleinen, T., Yu, Z. C., and Kaplan, J. O.: Present state of global wetland extent and wetland methane modelling: conclusions from a model inter-comparison project (WETCHIMP), Biogeosciences, 10, 753–788, https://doi.org/10.5194/bg-10-753-2013, 2013.
Meng, L., Hess, P. G. M., Mahowald, N. M., Yavitt, J. B., Riley, W. J., Subin, Z. M., Lawrence, D. M., Swenson, S. C., Jauhiainen, J., and Fuka, D. R.: Sensitivity of wetland methane emissions to model assumptions: application and model testing against site observations, Biogeosciences, 9, 2793–2819, https://doi.org/10.5194/bg-9-2793-2012, 2012.
Olefeldt, D., Hovemyr, M., Kuhn, M. A., Bastviken, D., Bohn, T. J., Connolly, J., Crill, P., Euskirchen, E. S., Finkelstein, S. A., Genet, H., Grosse, G., Harris, L. I., Heffernan, L., Helbig, M., Hugelius, G., Hutchins, R., Juutinen, S., Lara, M. J., Malhotra, A., Manies, K., McGuire, A. D., Natali, S. M., O'Donnell, J. A., Parmentier, F.-J. W., Räsänen, A., Schädel, C., Sonnentag, O., Strack, M., Tank, S. E., Treat, C., Varner, R. K., Virtanen, T., Warren, R. K., and Watts, J. D.: The Boreal–Arctic Wetland and Lake Dataset (BAWLD), Earth Syst. Sci. Data, 13, 5127–5149, https://doi.org/10.5194/essd-13-5127-2021, 2021.
Oleson, K. W., Lawrence, D. M., Bonan, G. B., Drewniak, B., Huang, M., Levis, S., Li, F., Riley, W. J., Swenson, S. C., Thornton, P. E., Bozbiyik, A., Fisher, R., Heald, C. L., Kluzek, E., Lamarque, F., Lawrence, P. J., Leung, L. R., Muszala, S., Ricciuto, D. M., Sacks, W., Sun, Y., Tang, J., and Yang, Z.-L.: Technical Description of version 4.5 of the Community Land Model (CLM), NCAR/TN-503+STR, NCAR Technical Note, ISSN 2153-2400, 2013.
Papa, F., Prigent, C., Rossow, W. B., Legresy, B., and Remy, F.: Inundated wetland dynamics over boreal regions from remote sensing: the use of Topex-Poseidon dual-frequency radar altimeter observations, Int. J. Remote Sens. 27, 4847–4866, https://doi.org/10.1080/01431160600675887, 2006.
Peano, D., Hemming, D., Materia, S., Delire, C., Fan, Y., Joetzjer, E., Lee, H., Nabel, J. E. M. S., Park, T., Peylin, P., Wårlind, D., Wiltshire, A., and Zaehle, S.: Plant phenology evaluation of CRESCENDO land surface models – Part 1: Start and end of the growing season, Biogeosciences, 18, 2405–2428, https://doi.org/10.5194/bg-18-2405-2021, 2021.
Peltola, O., Vesala, T., Gao, Y., Räty, O., Alekseychik, P., Aurela, M., Chojnicki, B., Desai, A. R., Dolman, A. J., Euskirchen, E. S., Friborg, T., Göckede, M., Helbig, M., Humphreys, E., Jackson, R. B., Jocher, G., Joos, F., Klatt, J., Knox, S. H., Kowalska, N., Kutzbach, L., Lienert, S., Lohila, A., Mammarella, I., Nadeau, D. F., Nilsson, M. B., Oechel, W. C., Peichl, M., Pypker, T., Quinton, W., Rinne, J., Sachs, T., Samson, M., Schmid, H. P., Sonnentag, O., Wille, C., Zona, D., and Aalto, T.: Monthly gridded data product of northern wetland methane emissions based on upscaling eddy covariance observations, Earth Syst. Sci. Data, 11, 1263–1289, https://doi.org/10.5194/essd-11-1263-2019, 2019.
Peters, W., Miller, J. B., Whitaker, J., Denning, A. S., Hirsch, A., Krol, M. C., Zupanski, D., Bruhwiler, L., and Tans, P. P.: An ensemble data assimilation system to estimate CO2 surface fluxes from atmospheric trace gas observations, J. Geophys. Res.-Atmos., 110, D24, https://doi.org/10.1029/2005JD006157, 2005.
Peters, W., Bastos, A., Ciais, P., and Vermeulen, A.: A historical, geographical and ecological perspective on the 2018 European summer drought, Philos. T. Roy. Soc. B, 375, 20190505, https://doi.org/10.1098/rstb.2019.0505, 2020.
Poulter, B., Bousquet, P., Canadell, J. G., Ciais, P., Peregon, A., Saunois, M., Arora, V. K., Beerling, D. J., Brovkin, V., Jones, C. D., Joos, F., Gedney, N., Ito, A., Kleinen, T., Koven, C. D., McDonald, K., Melton, J. R., Peng, C., Peng, S., Prigent, C., Schroeder, R., Riley, W. J., Saito, M., Spahni, R., Tian, H., Taylor, L., Viovy, N., Wilton, D., Wiltshire, A., Xu, X., Zhang, B., Zhang, Z., and Zhu, Q.: Global wetland contribution to 2000–2012 atmospheric methane growth rate dynamics, Environ. Res. Lett., 12, 094013, https://doi.org/10.1088/1748-9326/aa8391, 2017.
Putnam, A. E. and Broecker, W. S.: Human-induced changes in the distribution of rainfall, Sci. Adv., 3, e1600871, https://doi.org/10.1126/sciadv.1600871, 2017.
Raivonen, M., Smolander, S., Backman, L., Susiluoto, J., Aalto, T., Markkanen, T., Mäkelä, J., Rinne, J., Peltola, O., Aurela, M., Lohila, A., Tomasic, M., Li, X., Larmola, T., Juutinen, S., Tuittila, E.-S., Heimann, M., Sevanto, S., Kleinen, T., Brovkin, V., and Vesala, T.: HIMMELI v1.0: HelsinkI Model of MEthane buiLd-up and emIssion for peatlands, Geosci. Model Dev., 10, 4665–4691, https://doi.org/10.5194/gmd-10-4665-2017, 2017.
Ruosteenoja, K., Jylhä, K., and Kämäräinen, M.: Climate Projections for Finland Under the RCP Forcing Scenarios, Geophysica, 51, 17–50, 2016.
Rinne, J., Tuovinen, J.-P., Klemedtsson, L., Aurela, M., Holst, J., Lohila, A., Weslien, P., Vestin, P., Łakomiec, P., Peichl, M., Tuittila, E.-S., Heiskanen, L., Laurila, T., Li, X., Alekseychik, P., Mammarella, I., Ström, L., Crill, P., and Nilsson, M. B.: Effect of the 2018 European Drought on Methane and Carbon Dioxide Exchange of Northern Mire Ecosystems, Philos. T. R. Soc. B, 375, 20190517, https://doi.org/10.1098/rstb.2019.0517, 2020.
Räsänen, A., Manninen, T., Korkiakoski, M., Lohila, A., and Virtanen, T.: Predicting catchment-scale methane fluxes with multi-source remote sensing, Landscape Ecol., 36, 1177–1195, https://doi.org/10.1007/s10980-021-01194-x, 2021.
Saunois, M., Stavert, A. R., Poulter, B., Bousquet, P., Canadell, J. G., Jackson, R. B., Raymond, P. A., Dlugokencky, E. J., Houweling, S., Patra, P. K., Ciais, P., Arora, V. K., Bastviken, D., Bergamaschi, P., Blake, D. R., Brailsford, G., Bruhwiler, L., Carlson, K. M., Carrol, M., Castaldi, S., Chandra, N., Crevoisier, C., Crill, P. M., Covey, K., Curry, C. L., Etiope, G., Frankenberg, C., Gedney, N., Hegglin, M. I., Höglund-Isaksson, L., Hugelius, G., Ishizawa, M., Ito, A., Janssens-Maenhout, G., Jensen, K. M., Joos, F., Kleinen, T., Krummel, P. B., Langenfelds, R. L., Laruelle, G. G., Liu, L., Machida, T., Maksyutov, S., McDonald, K. C., McNorton, J., Miller, P. A., Melton, J. R., Morino, I., Müller, J., Murguia-Flores, F., Naik, V., Niwa, Y., Noce, S., O'Doherty, S., Parker, R. J., Peng, C., Peng, S., Peters, G. P., Prigent, C., Prinn, R., Ramonet, M., Regnier, P., Riley, W. J., Rosentreter, J. A., Segers, A., Simpson, I. J., Shi, H., Smith, S. J., Steele, L. P., Thornton, B. F., Tian, H., Tohjima, Y., Tubiello, F. N., Tsuruta, A., Viovy, N., Voulgarakis, A., Weber, T. S., van Weele, M., van der Werf, G. R., Weiss, R. F., Worthy, D., Wunch, D., Yin, Y., Yoshida, Y., Zhang, W., Zhang, Z., Zhao, Y., Zheng, B., Zhu, Q., Zhu, Q., and Zhuang, Q.: The Global Methane Budget 2000–2017, Earth Syst. Sci. Data, 12, 1561–1623, https://doi.org/10.5194/essd-12-1561-2020, 2020.
Seland, Ø., Bentsen, M., Olivié, D., Toniazzo, T., Gjermundsen, A., Graff, L. S., Debernard, J. B., Gupta, A. K., He, Y.-C., Kirkevåg, A., Schwinger, J., Tjiputra, J., Aas, K. S., Bethke, I., Fan, Y., Griesfeller, J., Grini, A., Guo, C., Ilicak, M., Karset, I. H. H., Landgren, O., Liakka, J., Moseid, K. O., Nummelin, A., Spensberger, C., Tang, H., Zhang, Z., Heinze, C., Iversen, T., and Schulz, M.: Overview of the Norwegian Earth System Model (NorESM2) and key climate response of CMIP6 DECK, historical, and scenario simulations, Geosci. Model Dev., 13, 6165–6200, https://doi.org/10.5194/gmd-13-6165-2020, 2020.
Sellar, A. A., Jones, C. G., Mulcahy, J. P., Tang, Y., Yool, A., Wiltshire, A., O'Connor, F. M., Stringer, M., Hill, R., Palmieri, J., Woodward, S., Mora, L. de, Kuhlbrodt, T., Rumbold, S. T., Kelley, D. I., Ellis, R., Johnson, C. E., Walton, J., Abraham, N. L., Andrews, M. B., Andrews, T., Archibald, A. T., Berthou, S., Burke, E., Blockley, E., Carslaw, K., Dalvi, M., Edwards, J., Folberth, G. A., Gedney, N., Griffiths, P. T., Harper, A. B., Hendry, M. A., Hewitt, A. J., Johnson, B., Jones, A., Jones, C. D., Keeble, J., Liddicoat, S., Morgenstern, O., Parker, R. J., Predoi, V., Robertson, E., Siahaan, A., Smith, R. S., Swaminathan, R., Woodhouse, M. T., Zeng, G., and Zerroukat, M.: UKESM1: Description and Evaluation of the U.K. Earth System Model, J. Adv. Model. Earth Sy., 11, 4513–4558, https://doi.org/10.1029/2019MS001739, 2019.
Smith, B., Wårlind, D., Arneth, A., Hickler, T., Leadley, P., Siltberg, J., and Zaehle, S.: Implications of incorporating N cycling and N limitations on primary production in an individual-based dynamic vegetation model, Biogeosciences, 11, 2027–2054, https://doi.org/10.5194/bg-11-2027-2014, 2014.
Spahni, R., Wania, R., Neef, L., van Weele, M., Pison, I., Bousquet, P., Frankenberg, C., Foster, P. N., Joos, F., Prentice, I. C., and van Velthoven, P.: Constraining global methane emissions and uptake by ecosystems, Biogeosciences, 8, 1643–1665, https://doi.org/10.5194/bg-8-1643-2011, 2011.
Spahni, R., Joos, F., Stocker, B. D., Steinacher, M., and Yu, Z. C.: Transient simulations of the carbon and nitrogen dynamics in northern peatlands: from the Last Glacial Maximum to the 21st century, Clim. Past, 9, 1287–1308, https://doi.org/10.5194/cp-9-1287-2013, 2013.
Stavert, A. R., Saunois, M., Canadell, J. G., Poulter, B., Jackson, R. B., Regnier, P., Lauerwald, R., Raymond, P. A., Allen, G. H., Patra, P. K., Bergamaschi, P., Bousquet, P., Chandra, N., Ciais, P., Gustafson, A., Ishizawa, M., Ito, A., Kleinen, T., Maksyutov, S., McNorton, J., Melton, J. R., Müller, J., Niwa, Y., Peng, S., Riley, W. J., Segers, A., Tian, H., Tsuruta, A., Yin, Y., Zhang, Z., Zheng, B., and Zhuang, Q.: Regional trends and drivers of the global methane budget, Global Change Biol., 28, 182–200, https://doi.org/10.1111/gcb.15901, 2022.
Stocker, B. D., Spahni, R., and Joos, F.: DYPTOP: a cost-efficient TOPMODEL implementation to simulate sub-grid spatio-temporal dynamics of global wetlands and peatlands, Geosci. Model Dev., 7, 3089–3110, https://doi.org/10.5194/gmd-7-3089-2014, 2014.
Tanneberger, F., Tegetmeyer, C., Busse, S., Barthelmes, A., and 55 others: The peatland map of Europe, Mires Peat, 19, 1–17, https://doi.org/10.19189/MaP.2016.OMB.264, 2017.
Tenkanen, M., Tsuruta, A., Rautiainen, K., Kangasaho, V., Ellul, R., and Aalto, T.: Utilizing Earth Observations of Soil Freeze/Thaw Data and Atmospheric Concentrations to Estimate Cold Season Methane Emissions in the Northern High Latitudes, Remote Sens., 13, 5059, https://doi.org/10.3390/rs13245059, 2021.
Thompson, R. L., Sasakawa, M., Machida, T., Aalto, T., Worthy, D., Lavric, J. V., Lund Myhre, C., and Stohl, A.: Methane fluxes in the high northern latitudes for 2005–2013 estimated using a Bayesian atmospheric inversion, Atmos. Chem. Phys., 17, 3553–3572, https://doi.org/10.5194/acp-17-3553-2017, 2017.
Treat, C. C., Bloom, A. A., and Marushchak, M. E.: Nongrowing season methane emissions–a significant component of annual emissions across northern ecosystems, Glob. Change Biol., 24, 3331–3343, https://doi.org/10.1111/gcb.14137, 2018.
Tsuruta, A., Aalto, T., Backman, L., Hakkarainen, J., van der Laan-Luijkx, I. T., Krol, M. C., Spahni, R., Houweling, S., Laine, M., Dlugokencky, E., Gomez-Pelaez, A. J., van der Schoot, M., Langenfelds, R., Ellul, R., Arduini, J., Apadula, F., Gerbig, C., Feist, D. G., Kivi, R., Yoshida, Y., and Peters, W.: Global methane emission estimates for 2000–2012 from CarbonTracker Europe-CH4 v1.0, Geosci. Model Dev., 10, 1261–1289, https://doi.org/10.5194/gmd-10-1261-2017, 2017.
Tuomi, M., Thum, T., Järvinen, H., Fronzek, S., Berg, B., Harmon, M., Trofymow, J., Sevanto, S., and Liski, J.: Leaf litter decomposition – Estimates of global variability based on Yasso07 model, Ecol. Model., 220, 3362–3371, https://doi.org/10.1016/j.ecolmodel.2009.05.016, 2009.
Tuovinen, J.-P., Aurela, M., Hatakka, J., Räsänen, A., Virtanen, T., Mikola, J., Ivakhov, V., Kondratyev, V., and Laurila, T.: Interpreting eddy covariance data from heterogeneous Siberian tundra: land-cover-specific methane fluxes and spatial representativeness, Biogeosciences, 16, 255–274, https://doi.org/10.5194/bg-16-255-2019, 2019.
Tyystjärvi, V., Markkanen, T., Backman, L., Raivonen, M., Leppänen, A., Li, X., Ojanen, P., Minkkinen, K., Hautala, R., Peltoniemi, M., Anttila, J., Laiho, R., Lohila, A., Mäkipää, R., and Aalto, T.: Future methane fluxes of peatlands are controlled by management practices and fluctuations in hydrological conditions due to climatic variability, Biogeosciences, 21, 5745–5771, https://doi.org/10.5194/bg-21-5745-2024, 2024.
Vainio, E., Peltola, O., Kasurinen, V., Kieloaho, A.-J., Tuittila, E.-S., and Pihlatie, M.: Topography-based statistical modelling reveals high spatial variability and seasonal emission patches in forest floor methane flux, Biogeosciences, 18, 2003–2025, https://doi.org/10.5194/bg-18-2003-2021, 2021.
van der Laan-Luijkx, I. T., van der Velde, I. R., van der Veen, E., Tsuruta, A., Stanislawska, K., Babenhauserheide, A., Zhang, H. F., Liu, Y., He, W., Chen, H., Masarie, K. A., Krol, M. C., and Peters, W.: The CarbonTracker Data Assimilation Shell (CTDAS) v1.0: implementation and global carbon balance 2001–2015, Geosci. Model Dev., 10, 2785–2800, https://doi.org/10.5194/gmd-10-2785-2017, 2017.
Viovy, N.: CRUNCEP Version 7 – Atmospheric Forcing Data for the Community Land Model, Research Data Archive at the National Center for Atmospheric Research, Computational and Information Systems Laboratory, https://doi.org/10.5065/PZ8F-F017, 2018.
Wania, R., Ross, I., and Prentice, I. C.: Implementation and evaluation of a new methane model within a dynamic global vegetation model: LPJ-WHyMe v1.3.1, Geosci. Model Dev., 3, 565–584, https://doi.org/10.5194/gmd-3-565-2010, 2010.
Warner, D. L., Guevara, M., Inamdar, S., and Vargas, R.: Upscaling soil-atmosphere CO2 and CH4 fluxes across a topographically complex forested landscape, Agr. Forest Meteorol., 264, 80–91, https://doi.org/10.1016/j.agrformet.2018.09.020, 2019.
Warwick, N. J., Cain, M. L., Fisher, R., France, J. L., Lowry, D., Michel, S. E., Nisbet, E. G., Vaughn, B. H., White, J. W. C., and Pyle, J. A.: Using δ13C-CH4 and δD-CH4 to constrain Arctic methane emissions, Atmos. Chem. Phys., 16, 14891–14908, https://doi.org/10.5194/acp-16-14891-2016, 2016.
Wolf, K., Flessa, H., and Veldkamp, E.: Atmospheric methane uptake by tropical montane forest soils and the contribution of organic layers, Biogeochemistry, 111, 469–483, https://doi.org/10.1007/s10533-011-9681-0, 2012.
Xu, J., Morris, P. J., Liu, J., and Holden, J.: PEATMAP: Refining estimates of global peatland distribution based on a meta-analysis, Catena, 160, 134–140, https://doi.org/10.1016/j.catena.2017.09.010, 2018.
Zandler, H., Senftl, T., and Vanselow, K. A.: Reanalysis datasets outperform other gridded climate products in vegetation change analysis in peripheral conservation areas of Central Asia, Sci. Rep., 10, 22446, https://doi.org/10.1038/s41598-020-79480-y, 2020.
Zhang, Z., Fluet-Chouinard, E., Jensen, K., McDonald, K., Hugelius, G., Gumbricht, T., Carroll, M., Prigent, C., Bartsch, A., and Poulter, B.: Development of the global dataset of Wetland Area and Dynamics for Methane Modeling (WAD2M) , Earth Syst. Sci. Data, 13, 2001–2023, https://doi.org/10.5194/essd-13-2001-2021, 2021.