the Creative Commons Attribution 4.0 License.
the Creative Commons Attribution 4.0 License.
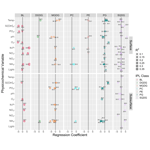
Lipid remodeling in phytoplankton exposed to multi-environmental drivers in a mesocosm experiment
Sebastian I. Cantarero
Edgart Flores
Harry Allbrook
Paulina Aguayo
Cristian A. Vargas
John E. Tamanaha
J. Bentley C. Scholz
Lennart T. Bach
Carolin R. Löscher
Ulf Riebesell
Balaji Rajagopalan
Nadia Dildar
Julio Sepúlveda
Lipid remodeling, the modification of cell membrane chemistry via structural rearrangements within the lipid pool of an organism, is a common physiological response amongst all domains of life to alleviate environmental stress and maintain cellular homeostasis. Whereas culture experiments and environmental studies of phytoplankton have demonstrated the plasticity of lipids in response to specific abiotic stressors, few analyses have explored the impacts of multi-environmental stressors at the community-level scale. Here, we study changes in the pool of intact polar lipids (IPLs) of a phytoplanktonic community exposed to multi-environmental stressors during a ∼ 2-month-long mesocosm experiment deployed in the eastern tropical South Pacific off the coast of Callao, Peru. We investigate lipid remodeling of IPLs in response to changing nutrient stoichiometries, temperature, pH, and light availability in surface and subsurface water masses with contrasting redox potentials, using multiple linear regressions, classification and regression trees, and random forest analyses. We observe proportional increases in certain glycolipids (namely mono- and diglycosyldiacylglycerol – MGDG and DGDG, respectively) associated with higher temperatures and oxic conditions, consistent with previous observations of their utility to compensate for thermal stress and their degradation under oxygen stress. N-bearing (i.e., betaine lipids and phosphatidylethanolamine – BLs and PE) and non-N-bearing (i.e., MGDG; phosphatidylglycerol, PG; and sulfoquinovosyldiacylglycerol, SQDG) IPLs are anti-correlated and have strong positive correlations with nitrogen-replete and nitrogen-depleted conditions, respectively, which suggests a substitution mechanism for N-bearing IPLs under nitrogen limitation. Reduced CO2(aq) availability and increased pH levels are associated with greater proportions of DGDG and SQDG IPLs, possibly in response to the lower concentration of CO2(aq) and the overall lower availability of inorganic carbon for fixation. A higher production of MGDG in surface waters corresponds well with its established photoprotective and antioxidant mechanisms in thylakoid membranes. The observed statistical relationships between IPL distributions, physicochemical parameters, and the composition of the phytoplankton community suggest evidence of lipid remodeling in response to environmental stressors. These physiological responses may allow phytoplankton to reallocate resources from structural or extrachloroplastic membrane lipids (i.e., phospholipids and betaine lipids) under high-growth conditions to thylakoid and/or plastid membrane lipids (i.e., glycolipids and certain phosphatidylglycerols) under growth-limiting conditions. Further investigation of the exact mechanisms controlling the observed trends in lipid distributions is necessary to better understand how membrane reorganization under multi-environmental stressors can affect the pools of cellular C, N, P, and S, as well as their fluxes to higher trophic levels in marine environments subjected to increasing environmental pressure. Our results suggest that future studies addressing the biogeochemical consequences of climate change in the eastern tropical South Pacific Ocean must take into consideration the impacts of lipid remodeling in phytoplankton.
- Article
(5733 KB) - Full-text XML
-
Supplement
(3392 KB) - BibTeX
- EndNote
The eastern tropical South Pacific (ETSP) is one of the most productive eastern boundary upwelling systems in the world (Chavez and Messié, 2009) and harbors one of the largest oxygen-deficient zones (ODZs) (Fuenzalida et al., 2009; Ulloa and Pantoja, 2009; Thamdrup et al., 2012). Global warming has led to the expansion of ODZs over recent decades, and they are expected to continue expanding due to the reduction in oxygen solubility with increasing temperature (Stramma et al., 2008, 2010; Gilly et al., 2013), as well as because of enhanced ocean stratification and reduced ventilation of the ocean's interior (Keeling et al., 2010). The future behavior of the ETSP upwelling system in a warmer world remains uncertain; increases in wind-induced upwelling intensity and duration (Gutiérrez et al., 2011; Bakun et al., 2010) may increase the supply of nutrients to the surface in coastal regions, whereas enhanced thermal stratification may reduce nutrient supply in the open ocean (Behrenfeld et al., 2006). Furthermore, upwelling regions are prone to highly variable pH (Capone and Hutchins, 2013), and the global ocean will experience a decreasing average pH as more CO2 accumulates in the atmosphere and is absorbed by the ocean (Jiang et al., 2019). Accordingly, major shifts in marine planktonic community composition, turnover rates (Henson et al., 2021), and adaptations (Irwin et al., 2015) are expected in future scenarios of ocean conditions, which are expected to lead to cascading effects on ocean biogeochemistry and marine ecosystems (Hutchins and Fu, 2017).
Primary productivity in the ETSP is predominantly regulated by the wind-induced upwelling of nutrients, light availability, and Fe limitation (Messié and Chavez, 2015). Thus, changes in the supply of inorganic N throughout the upwelling region of the ETSP are likely to induce significant shifts in the phytoplankton community composition. Longer upwelling seasons in nearshore environments could further stimulate productivity of fast-growing eukaryotic algae that currently dominate these systems (e.g., diatoms; Messié et al., 2009). However, shorter upwelling seasons or weaker upwelling currents could favor more survivalist or mixotrophic algae, in addition to N-fixing diazotrophs that thrive under widespread nitrogen limitation (Dutkiewicz et al., 2012). The rate of primary productivity in the surface ocean not only affects the supply of sinking organic matter and thus oxygen consumption via microbial respiration in the subsurface (Wyrtki, 1962), but also results in a shift of redox potentials that drives substantial losses of bioavailable N under reducing conditions at intermediate depths (Lam et al., 2009; Wright et al., 2012). Additionally, expected changes in ocean warming and stratification (Huertas et al., 2011; Morán et al., 2010; Yvon-Durocher, 2015), lowered dissolved oxygen concentration (Wu et al., 2012), and decreased pH (Dutkiewicz et al., 2015; Bach et al., 2017) will disrupt phytoplanktonic assemblages differently based on their individual tolerances and physiological plasticity. However, little is known of the physiological adaptations of phytoplankton on a community-level scale in response to multi-environmental stressors.
Phytoplankton have been shown to activate several lipid-based physiological mechanisms in response to environmental stimuli (Li-Beisson et al., 2019; Sayanova et al., 2017; Kong et al., 2018). In fact, intact polar lipids (IPLs) are a class of membrane lipids characterized by a polar head group typically attached to a glycerol backbone from which aliphatic chains are attached via ester and/or ether bonds (Sturt et al., 2004; Lipp et al., 2008; Schubotz et al., 2009; Van Mooy and Fredricks, 2010). Dominant planktonic lipid classes include phospholipids with a phosphate-bearing polar head group (e.g., phosphatidylcholine, PC; phosphatidylethanolamine, PE; and phosphatidylglycerol, PG), glycolipids featuring a sugar moiety in the polar head (e.g., monoglycosyldiacylglycerol, MGDG; diglycosyldiacylglycerol, DGDG; sulfoquinovosyldiacylglycerol, SQDG), and betaine lipids with a quaternary amine positively charged and attached to lipid chains (e.g., diacylglyceryl hydroxymethyl-trimethyl-β-alanine, DGTA; diacylglyceryl trimethylhomoserine, DGTS; and diacylglycerylcarboxy-N-hydroxymethyl-choline, DGCC) (Kato et al., 1996; Rütters et al., 2001; Zink et al., 2003; Suzumura, 2005; Van Mooy et al., 2006). The remodeling of IPLs, the main constituents of cell and organellar membranes, provides numerous physiological adjustments to attenuate environmental stressors impacting phytoplankton (Zienkiewicz et al., 2016). These include nutrient limitation (Van Mooy et al., 2009; Meador et al., 2017; Abida et al., 2015; Wang et al., 2016), homeoviscous regulation in response to changing temperature (Sato and Murata, 1980; Sinensky, 1974; Neidleman, 1987) and pH (Tatsuzawa and Takizawa, 1996; Poerschmann et al., 2004; Guckert and Cooksey, 1990; Jin et al., 2021), or photosynthetic function under varying light availability (Sato et al., 2003; Gašparović et al., 2013; Khotimchenko and Yakovleva, 2005). While IPL distributions in environmental studies are typically used as chemotaxonomic biomarkers that trace the presence and abundance of specific microbial groups (Sturt et al., 2004; Schubotz et al., 2009; Van Mooy and Fredricks, 2010), their distributions have been used in conjunction with additional microbial or geochemical measurements to assess how microbial metabolisms contribute to the chemical environment (Van Mooy et al., 2009; Wakeham et al., 2012; Schubotz et al., 2018; Cantarero et al., 2020). Yet few studies have explored how multiple environmental drivers impact IPL remodeling at the community level and in time series and the associated adaptability of phytoplankton to environmental change.
Lab-based culture experiments have been a major step forward in understanding how lipid remodeling may impact a biogeochemical system (as summarized above). However, a significant challenge remains in contextualizing these findings at the community scale. Conversely, observational studies from direct measurements of natural systems are often logistically limited in temporal scale and, consequently, do not fully capture the dynamics and heterogeneity of biogeochemical conditions. Mesocosms are experimental apparatuses at the interface between controlled culture experiments and environmental observations that allow for the examination of natural systems and entire ecosystems under semi-controlled conditions to explore the impacts of a changing climate and ocean system (Riebesell et al., 2013). Here, we study changes in the composition, diversity, and abundance of phytoplanktonic IPLs in response to changes in the biological, physical, and chemical composition of a marine ecosystem subjected to semi-controlled conditions in a 2-month-long mesocosm experiment off the coast of Peru. We investigate the potential for IPL remodeling amongst phytoplankton in response to multiple environmental stressors including nutrient availability, O2 concentration, pH, temperature, and light availability to highlight adaptation strategies available to phytoplankton in response to a changing ocean system.
2.1 Mesocosm deployment and sampling
On 22 February 2017, eight Kiel Off-Shore Mesocosms for Ocean Simulations (KOSMOS; Riebesell et al., 2013) were deployed just north of San Lorenzo Island, 6 km off the Peruvian coastline (12.0555° S, 77.2348° W; Fig. 1; Bach et al., 2020) in waters 30 m deep. Each individual mesocosm consisted of a cylindrical polyurethane bag (2 m diameter, 18.7 m length, 54.4 ± 1.3 m3 volume) suspended in an 8 m tall flotation frame. Water exchange was permitted via nets (mesh size 3 mm) for 3 d before the water mass inside each mesocosm was enclosed and isolated from the surrounding Pacific water by attaching sediment traps at the base (∼ 19 m length total). The enclosing of the mesocosms marked the start (day 0) of the 50 d experiment. As detailed in Bach et al. (2020), the experiment involved several manipulations, including the addition of ODZ water to simulate upwelling conditions, the addition of salt to maintain water stratification, and the introduction of organisms.
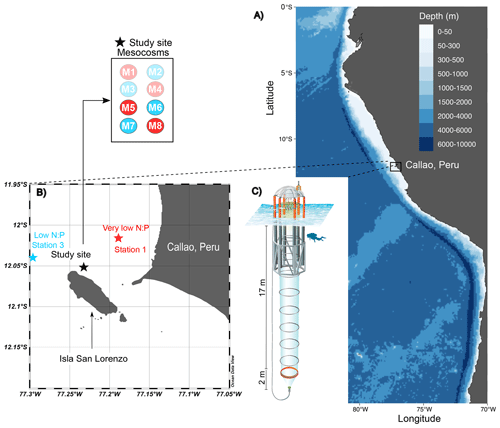
Figure 1The KOSMOS study site. (a) Overview map indicating the location of the study region in La Punta, Callao, Peru. (b) Detailed map of the study site with the mesocosm arrangement (M1–M8). Red (very low N:P) and blue (low N:P) colors indicate the different stations for ODZ water collection (1 and 3; star symbols) and replicates of the two different water treatments (numbers in circles in insert). (c) Diagram of a mesocosm unit with underwater bag dimensions. The figure is modified from Chen et al. (2022); software credit is Reiner Schlitzer, Ocean Data View, https://odv.awi.de (last access: 22 December 2023).
The collection of subsurface waters and their addition to the mesocosms are described in detail in Bach et al. (2020). Briefly, on experiment days 5 and 10, two batches of local subsurface ODZ water were collected from stations with varying nutrient stoichiometries (Table 1; Fig. 1) using deepwater collectors first reported by Taucher et al. (2017). The water mass collected from 30 m deep at station 1 (12.028323° S, 77.223603° W) was characterized by a very low N:P ratio (Table 1), whereas the water collected from 70 m deep at station 3 (12.044333° S, 77.377583° W) was characterized by a higher but still low N:P ratio (Table 1). On experiment day 8, 9 m3 of water was removed from each mesocosm at a depth between 11 and 12 m, and then on day 11, 10 m3 of ODZ water was injected into each mesocosm at depths between 14 and 17 m. On experiment day 12, the entire procedure was repeated but this time with 10 m3 removed between 8 and 9 m and 12 m3 added evenly between 1 and 9 m. To maintain stratification and preserve the low-O2 subsurface layer, a NaCl brine solution was injected evenly into the subsurface of all mesocosms on experiment day 13 (0.067 m3, 10 to 17 m depth) and experiment day 33 (0.046 m3, 12.5 to 17 m depth). On day 14, Peruvian scallop larvae (Argopecten purpuratus) were added (∼ 10 000 individuals m−3), and on day 31 fine flounder eggs (Paralichthys adspersus) were added (∼ 90 individuals m−3). However, few scallop larvae and no fish larvae were found in the mesocosms after the release, indicating that their influence on the plankton community was likely small (Bach et al., 2020).
Table 1Concentration of inorganic nutrients (µmol L−1), nutrient stoichiometry, and pHT of the two batches of ODZ water collected and added to the mesocosm experiment.

We sampled two integrated water depths of the mesocosms for suspended organic matter and biological and chemical characterization using 5 L integrating water samplers (IWSs; Hydro-Bios, Kiel) equipped with pressure sensors to collect water evenly within a desired depth range. The samples were collected across two integrated intervals of the water column representing surface and subsurface layers; sampling depths were slightly modified over the course of the experiment to accommodate changes in water stratification and the position of the chemocline (refer to Bach et al., 2020, for further details). The depths applied for surface and subsurface waters were 0–5 and 5–17 m on days 1 and 2, 0–10 and 10–17 m from day 3 to 28, and 0–12.5 and 12.5–17 m from day 29 to 50.
Samples of suspended organic matter for IPL analysis were collected from all eight mesocosms at both the surface and the subsurface sampling depths on non-consecutive days throughout the experiment. See details of changes in water depths above. Due to the labor- and time-intensive nature of IPL analysis, we focused on four mesocosms (two from each treatment) at both depths and from 9 different days spanning the 50 d experiment. We filtered 5 L of mesocosm water through pre-combusted, 142 mm Advantec glass fiber filters (GF75142MM) of 0.3 µm pore size. All samples were wrapped in combusted aluminum foil and shipped frozen to the Organic Geochemistry Laboratory at the University of Colorado Boulder for IPL extraction and analysis.
2.2 Water column physicochemistry
Depth profiles of salinity, temperature, O2 concentration, photosynthetically active radiation (PAR), and chlorophyll a (Chl a) fluorescence were measured through vertical casts using the CTD60M sensor system (Sea & Sun Technology). O2 concentrations were cross-verified with the Winkler O2 titration method performed via a micro-Winkler titration method described by Arístegui and Harrison (2002). Seawater pHT (pH on the total scale) was determined spectrophotometrically using m-cresol purple (mCP) indicator dye as described in Carter et al. (2013); see Chen et al. (2022) for details. See Bach et al. (2020) for additional detailed information on sampling methods for water column physicochemistry.
Samples for inorganic nutrients were filtered immediately upon arrival at the laboratories at the Instituto del Mar del Perú (IMARPE) using 0.45 µm Sterivex filters (Merck). The subsequent analysis was carried out using a continuous flow analyzer (QuAAtro AutoAnalyzer, SEAL Analytical) connected to a fluorescence detector (FP-2020, Jasco). The method for analyzing PO followed the procedure outlined by Murphy and Riley (1962), while Si(OH)4 was analyzed according to Mullin and Riley (1955). NO and NO were quantified through the formation of a pink azo dye as established by Morris and Riley (1963), and additional corrections to all colorimetric methods were achieved with the refractive index method developed by Coverly et al. (2012). Ammonium concentrations were determined fluorometrically following the method of Kérouel and Aminot (1997). Further methodological specifics and the respective limits of detection for each analysis can be found in Bach et al. (2020).
2.3 CHEMTAX analysis
Pigment samples were flash-frozen in liquid nitrogen directly after filtration and kept frozen on dry ice during transport to Germany for extraction as described by Paul et al. (2015). Concentrations of extracted pigments were measured by reverse-phase high-performance liquid chromatography (HPLC; Barlow et al., 1997) calibrated with commercially available standards. The relative contribution of distinct phytoplankton taxa was calculated with CHEMTAX, a program for calculating the taxonomic composition of phytoplankton populations (Mackey et al., 1996). Input pigment ratios specific to the Peruvian upwelling system, determined by DiTullio et al. (2005) and further described by Meyer et al. (2017), were incorporated in these calculations (see Bach et al., 2020).
2.4 Flow cytometry
Samples (650 µL) from each mesocosm were analyzed using an Accuri C6 (BD Biosciences) flow cytometer. The signal strength of the forward light scatter was used to distinguish phytoplankton groups, in addition to the light emission from red fluorescence of Chl a and the light emission from orange fluorescence of phycoerythrin. Size ranges were constrained via gravity filtration using sequential polycarbonate filters ranging from 0.2 to 8 µm and the strength of the forward light scatter signal. Additional details of this method can be found in Bach et al. (2017). In this study, we only report Synechococcus (0.8–3 µm) counts (cells mL−1) because Synechococcus is the only phytoplankton group that has been consistently selected to exhibit statistically significant trends with IPLs, likely due to the non-size-fractionated nature of IPL sampling.
2.5 Lipid extraction and analysis
Intact polar lipids were extracted from glass fiber filters via a modified version (Wörmer et al., 2015) of the original Bligh and Dyer extraction method (Bligh and Dyer, 1959). Samples were extracted by ultrasonication a total of five times, with three different extraction mixtures. Two extractions were performed using dichloromethane : methanol : phosphate buffer (aq) [, ], adjusted to a pH of ∼ 7.4, followed by another two extractions using dichloromethane : methanol : trichloroacetic acid buffer(aq) [, ], adjusted to a pH of ∼ 2.0. A final extraction was performed with dichloromethane : methanol [1:5, v:v]. After each addition, samples were vortexed for 30 s, sonicated for 10 min, and then centrifuged for 10 min at 3000 rpm while kept at 10 °C. The supernatant of each extraction mixture was then transferred to a separatory funnel where the organic fractions were washed and combined before solvent removal under a gentle N2 stream. Before analysis, the total lipid extractions (TLEs) were resuspended in dichloromethane:methanol (9:1 v:v) and filtered through a 0.45 µm polytetrafluoroethylene (PTFE) syringe filter.
Chromatographic separation and identification of IPLs were achieved using a Thermo Scientific UltiMate 3000 high-performance liquid chromatography (HPLC) interphase to a Q Exactive Focus Orbitrap quadrupole high-resolution mass spectrometer (HPLC-HRMS) via heated electrospray ionization (HESI) in positive mode, as described in detail in applications by Cantarero et al. (2020) and Flores et al. (2022). Briefly, a flow rate of 0.4 mL min−1 was applied to an Acquity BEH Amide column (150 mm, 2.1 mm, 1.7 µm) using a gradient program first described by Wörmer et al. (2013). All filtered TLEs were suspended in dichloromethane : methanol (9:1, ) prior to injection (10 µL) on the column. The following HESI conditions were applied: auxiliary gas temperature 425 °C, capillary temperature 265 °C, spray voltage 3.5 kV, sheath gas flow rate 35 arbitrary units (AU), auxiliary gas flow 13 AU, and S-lens RF level 55 AU. Samples were analyzed in full-scan mode to obtain an untargeted screening (or lipidomic profile) of each sample, in addition to targeted MS/MS mode for compound identification via diagnostic fragmentation patterns (e.g., Sturt et al., 2004; Schubotz et al., 2009; Wakeham et al., 2012). IPLs were identified by their exact masses, polar head groups, the total number of carbon atoms and unsaturations in the core structure, and their retention times. While other studies have analyzed IPLs under both positive and negative ionization modes to determine the composition of individual fatty acid chains in the core lipid structures, we took advantage of the high resolution of the Orbitrap mass spectrometer to focus on the diversity of head group combinations with total carbon atoms and unsaturation only (Cantarero et al., 2020).
Quantification of IPLs was achieved using a combination of an internal standard added (2 µg) to samples during extraction (C16 PAF, Avanti Polar Lipids), in addition to an external calibration curve consisting of 17 standards representing different IPL classes (see Cantarero et al., 2020, for full details of all internal, external, and deuterated standards). The intensity of each individual IPL identified in the HPLC-HESI-HRMS analysis was calibrated to a linear regression between peak areas and known concentrations of the same lipid class (or the most similar molecular structure) across a five-point dilution series (0.0001–10 ng µL−1). The detection limit, based on individual calibration curves, was determined to be 0.01 ng on the column except for DGTS (0.001 ng) and DGDG, MGDG, and SQDG classes (0.1 ng). Samples were analyzed across three separate analytical periods with weekly calibration curves to account for variation in the ionization efficiency of compounds over time. Overall, HPLC-ESI-HRMS is considered a semi-quantitative method due to changes in ionization efficiency of different IPL standards and environmental analytes. These changes are largely caused by differences in polar head group compared to the acyl chain length and degree of unsaturation (Yang and Han, 2011). Nonetheless, we investigate both relative (%) and absolute IPL abundances to compensate for the current analytical limitations in IPL quantification.
While we report IPL structural variations associated with different head groups and modifications in the core structure (i.e., unsaturation degree and carbon length of diacyl chains), we particularly focus on the former. Additionally, several IPLs thought to be absent in eukaryotic phytoplankton or far more abundant in bacteria and archaea (n=34 in total) have been removed to facilitate the analysis of trends that are predominantly phytoplanktonic in origin. These compounds include PME and PDME (phosphatidyl-N-methylethanolamine and phosphatidyl-N,N-dimethylethanolamine) and intact GDGT (glycerol dialkyl glycerol tetraether) classes. In addition, while we lack detailed structural information on core individual fatty acids, their combined carbon atoms can be used to deduce short (i.e., < 14 carbon atoms per chain) or odd-numbered fatty acid chains typically found in bacteria (Volkman et al., 1989, 1998; Russell and Nichols, 1999; Jónasdóttir, 2019). Thus, compounds conventionally regarded as bacterial (34 in total) were also removed to minimize their impact on the analysis of predominantly phytoplanktonic IPLs (165 in total). We recognize that while this selection approach reduces the influence of non-eukaryotic lipids, we still cannot rule out an undetermined contribution from heterotrophic bacteria to the IPL pool in this experiment. A summary of these IPL classes and their abbreviations is provided in Table 2.
2.6 Multiple linear regression
We performed multiple linear regression between 8 relevant environmental factors and 165 unique IPLs. With so many IPL–environmental-factor pairs to analyze, we used multiple linear regression (MLR) for quick and easily digestible outputs. In each MLR model, the relative abundance of individual IPL molecules was employed as a response variable, with environmental factors serving as predictor variables. Additionally, phytoplankton abundances were included to account for their linear effects on IPL distributions. The rationale behind this inclusion lies in the understanding that variations in phytoplankton abundance may exert a proportional and predictable impact on the abundance patterns of specific IPLs.
We prioritized linear relationships with IPL relative abundances (% abundances) to emphasize changes in the proportions of phytoplankton lipids, rather than their absolute concentration and contribution to biomass. This approach enables us to distinguish compositional changes in the lipid pool from variability in total biomass production. Additionally, MLRs were constrained to focus on the most abundant IPLs in this system, defined as those constituting more than 2.5 % to the total IPL pool. This restriction was implemented to reduce noise associated with low-abundance IPLs and enhance the robustness of analysis.
We chose to investigate linear relationships within individual depths (surface and subsurface) to focus on temporal changes within distinct environments, rather than comparing these two environments directly (see CART and random forest methods below). IPLs and environmental factors were permuted to tabulate the regression coefficient for each IPL–environmental-factor pair. Model coefficients were directly comparable due to centering and scaling of environmental and phytoplankton variables (see Eq. 1) to linearize the relationship and for better alignment with the model assumptions:
where Y is the dependent variable (or response); are the predictor variables (or independent variables); β0 is the intercept (constant term); β1, β2, …, βn are the coefficients representing the magnitude and direction of the relationship between the predictor variable and the dependent variable; and ε is the error term capturing the variability not explained by the model. Correlations in the MLR analysis were also controlled for the false discovery rate following the procedure of Benjamini and Hochberg (1995) to calculate adjusted p values and by applying an alpha cutoff of 0.1.
2.7 Classification and regression tree (CART) and random forest
Classification and regression trees (CARTs; Breiman et al., 1984) are predictive machine learning algorithms that partition and fit data along a predictor axis into homogenous subsets of the dependent variable. Regression trees are used for dependent variables with continuous values, while classification trees are used for categorical values. Here, we apply regression trees to diagnose the environmental and biological variables that affect IPL concentrations by polar head group class. We limited the size of the tree splits via a “pruning” process, where less significant variable splits are removed as determined by a deviance criterion resulting in the best-fit tree with the least mean-squared error. Additionally, predictor variables were selected in conjunction with random forest analyses, which rank the variable contribution to the model performance.
Random forests are derived from bootstrapping of observational data and the generation of many decision trees based on each bootstrap sample. We employed a total of 72 samples for the random forest analysis, which was run separately for each major IPL class (n=7) to identify the most important environmental predictors (n=12) for each individual class of lipid. The random forest method utilizes bootstrap aggregation to generate and average numerous permutations of an out-of-bag score in predictive performance. This approach offers an effective methodology for analyzing high-dimensional data with limited sample sizes (Biau and Scornet, 2016). This method has been widely adopted across various disciplines within the water sciences (Tyralis, 2019) and ecological and species distribution modeling (Luan et al., 2020), as well as in bioinformatics and high-throughput genomics (Chen and Ishwaran, 2012; Boulesteix et al., 2012). For a covariate vector, the predicted estimate of IPL class concentrations is an average of the many randomly configured decision trees with the same distribution. A randomly generated subset of decision trees is used to split each node, enabling reduced variance and correlation amongst individual trees and ideally improving the accuracy of model predictions (Breiman, 2001). The random forest algorithm also allows for the ranking of predictor variables based on the prediction performance and is reported here as the predictor's contribution in reducing the root mean square error (RMSE) in the model. For additional details on the random forest algorithm, please see Hastie et al. (2009). All CHEMTAX, flow cytometry, and physicochemical variables were included in the CART and random forest analyses as predictors. Regression tree (CART) splitting criteria are determined by evaluating the sum of squared deviations in all possible splits and selecting those that result in the greatest reduction in residual error. To prevent overfitting in the CART analysis, a pruning procedure is run to remove nodes that contribute little to the model accuracy based on a cost complexity measure. This procedure allows us to simplify the CART results and thus focus our interpretations on the most significant predictors of IPL head groups only. In the random forest model, following the averaged cross-validated accuracy estimates, we implemented a cutoff of a 5 % reduction in RMSE to eliminate variables that do not significantly reduce the error in the model prediction. This cutoff allows us to focus our interpretation of only variables that contribute significantly to the out-of-bag predictor performance (for further details, refer to Sect. S1 in the Supplement).
CART and random forest serve as ideal methods to explore environmental drivers of IPL distributions – not only because of their predictive performance but also because of their non-parametric nature, their diagnosis of variable importance, and their ability to handle non-linear interactions and small sample sizes (Tyralis et al., 2019). We do not employ these methods to predict the concentration of IPL classes but rather to identify the primary environmental and biological drivers of change in IPL class concentrations and potential interactions between environmental conditions and IPL remodeling amongst phytoplankton. Therefore, we focus our interpretations of both the CART and the random forest analyses on only the most significant predictors and their relative order of importance in the model predictions.
3.1 Oxygen and pHT
Oxygen concentration in surface waters ranged between ∼ 125 and 140 µmol L−1 before the ODZ water addition in all four mesocosms (Fig. 2a). The concentration dropped slightly (∼ 15 µmol L−1) following the water addition and steadily increased to a maximum between ∼ 185–220 µmol L−1 by day 28. Day 30 marked a significant drop in oxygen concentration by 30–60 µmol L−1 in all mesocosms with conditions stabilizing between 140 and 155 µmol L−1 by day 50. The temporal variability in pHT in surface waters showed a largely mirrored trend to that of oxygen content. Before ODZ water addition, pHT was 7.9–8.0, which dropped by ∼ 0.2 immediately following the water addition. After a few days of relatively stable pHT at ∼ 7.8 in all four mesocosms, the pHT increased significantly between days 18 and 28, reaching ∼ 8.1–8.2. From days 30–40, the pHT gradually decreased by approximately 0.2 in all mesocosms until increasing again (most notably in mesocosms 7 and 5) between days 42–50 to maxima ranging from 8.1–8.3.
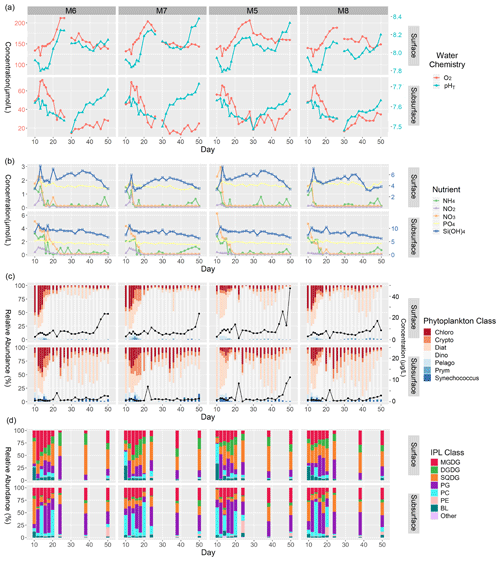
Figure 2Summary of major physicochemical, biological, and lipidomic measurements in surface and subsurface waters of mesocosms analyzed in this study from the time of deep-water injection to the end of the experiment. (a) Concentration of O2 (µmol L−1; left y axis), and pHT (total scale; right y axis). (b) Concentration of inorganic N (left y axis), P (left y axis), and Si(OH)4 (right y axis) expressed in µmol L−1. (c) Relative abundance (%) of phytoplankton classes (left y axis) as well as total Chl a concentrations expressed in µg L−1 (black line; right y axis). (d) Relative abundance (%) of major IPL classes based on head group contributions to the total IPL pool.
The subsurface waters were more oxygen-depleted compared to the surface and showed an earlier onset of increasing oxygen concentration, beginning immediately after the ODZ water addition. All four mesocosms reached maximum O2 levels (60–75 µmol L−1) by day 13 and began decreasing markedly by day 16. The lowest concentrations (∼ 15 µmol L−1 O2) were reached between days 30 and 34. Oxygen concentrations recovered slightly in the last 10–16 d of the experiment and were all within 30 (± 10) µmol L−1 of O2. The subsurface waters, again, showed similar temporal patterns in pHT to those in O2, except for mesocosm 7. The pHT was variable (∼ 7.60 ± 0.05) in the early portion of the experiment (days 10–16) but did not show a dramatic response to the ODZ water addition as was the case in the surface samples. The pHT did gradually decrease from days 18–30, with pHT minima in all four mesocosms reached on day 30 (∼ 7.45–7.50). Day 30 also marked the beginning of a significant increase in the pHT (∼ 0.15–0.20) across all mesocosms, with the pHT steadily increasing to ∼ 7.65–7.70 by day 50. Additional details on the carbonate chemistry of the mesocosms can be found in Chen et al. (2022).
3.2 Nutrient concentrations
In mesocosm surface waters, the nutrients NH, NO, NO, PO, and Si(OH)4 all showed consistent trends over time, with the highest concentrations occurring either just before (day 10) or immediately after (day 12) the ODZ water addition (Fig. 2b). Nitrogen species ranged between 1 and 3 µmol L−1 during this early part of the experiment, while Si(OH)4 ranged between 4 and 7 µmol L−1 and PO remained at ∼ 2 µmol L−1. The concentration of all nitrogen species dropped quickly to near-minimum values by day 15 and typically remained < 0.5 µmol L−1 for the remainder of the experiment. PO remained replete (> 1.5 µmol L−1 for the entirety of the experiment). Si(OH)4 dropped to ∼ 3–4 µmol L−1 by day 15 and gradually increased in all four mesocosms by 1–2 µmol L−1 until days 36–38, when concentrations gradually dropped to near-minimum values of 3–4 µmol L−1. There were periodic enrichments in NH, most notably between days 40–50, as discussed in Bach et al. (2020).
The mesocosm subsurface water nutrients showed similar temporal patterns to the surface but were generally more enriched in all nutrient species. All nitrogen species decreased in the few days following the ODZ water addition but at a more gradual pace than at the surface. Before and immediately after the water addition, NO was enriched between 4–6 µmol L−1 and NH between 2.5–4 µmol L−1. NO concentrations were ∼ 0.5–1 µmol L−1 in mesocosms 6 and 7 during this period but remained low (< 0.3 µmol L−1) in mesocosms 5 and 8. Broadly speaking, the nitrogen species all reached minimal values (< 0.1 µmol L−1) by days 18–20, except for in mesocosms 6 and 8, where NO persisted at significant concentrations (0.2–2.3 µmol L−1) until days 22 and 24, respectively. The remainder of the experiment was marked by typically depleted concentrations of all nitrogen species (< 0.1 µmol L−1), with occasional spikes in NH reaching up to 1 µmol L−1, particularly towards the end of the experiment. Subsurface PO concentrations were similar to those at the surface, remaining around ∼ 2.0 µmol L−1 for most of the experiment and gradually decreasing to concentrations of ∼ 1.5 µmol L−1 by the end of the experiment. Si(OH)4 similarly decreased from maximum concentrations of 4.0–4.5 µmol L−1 shortly after the ODZ water addition and gradually decreased by ∼ 1 µmol L−1 over the course of the experiment.
3.3 Total chlorophyll a
Chl a concentrations were highly variable throughout the experiment, particularly in subsurface waters (Fig. 2c). Concentrations were generally more elevated at the surface compared to the subsurface, with values ranging between ∼ 2.56–2.96 µg L−1 on day 10. In all mesocosms, the Chl a concentration increased to ∼ 7.94–14.01 µg L−1 after the ODZ water addition and through day 20. Day 22 showed a significant drop in Chl a concentrations to between 1.35 and 2.89 µg L−1 in mesocosms 7, 5, and 8. Chl a concentrations at the surface remained rather constant until days 36–40, where concentrations rapidly increased until maximum concentrations on days 48–50 (14.0–47.3 µg L−1). In subsurface waters, Chl a concentrations were notably lower than at the surface and ranged between 0.58–0.84 µg L−1 on day 10. After the ODZ water addition, Chl a concentrations increased slightly until day 14 but did not show consistent distributions amongst the four mesocosms afterwards; decreases were observed in mesocosms 6, 5, and 8, but an increase was observed in mesocosm 7. Chl a concentrations increased rapidly on day 22 in all four mesocosms, ranging between 4.03 and 8.44 µg L−1. While remaining highly variable, the concentrations generally increased after day 24, with a notably abrupt increase between days 40–44 to near-maximum values ranging between 2.58–11.3 µg L−1.
3.4 Phytoplankton community composition
The CHEMTAX-based phytoplankton community compositions demonstrated more variability in phytoplankton assemblages in the subsurface samples than at the surface (Fig. 2c). Before the ODZ water addition on day 10, surface waters in all four mesocosms showed similar phytoplankton distributions with high relative abundances of Bacillariophyceae, referred to from here on as diatoms (20 %–45 %); Chlorophyceae (15 %–50 %); and Dinophyceae, referred to as dinoflagellates (25 %–45 %). These distributions remained rather similar immediately after the water addition (day 12), but dinoflagellate contributions increased in the following days and ranged from ∼ 20 % of the total Chl a pool to up to 75 % by day 20. Notably, Cryptophyceae made minor contributions to the Chl a pool aside from during days 15–18 in mesocosm 6, when they contributed up to 25 %. Dinoflagellates largely dominated the Chl a pool for the remainder of the experiment with moderate blooms of diatoms between days 34–44 in mesocosms 7 and 8.
Subsurface waters exhibited greater variability in the phytoplankton assemblages and a greater contribution of Chlorophyceae, Cryptophyceae, Synechococcus, and diatoms to the Chl a pool than at the surface. Pre-addition waters (day 10) were dominated by diatoms making up > 75 % of the Chl a pool. In the first few days after the ODZ water addition (days 12–15), the relative abundance of Chlorophyceae increased to 25 %–45 % in mesocosms 6, 5, and 8, whereas Cryptophyceae contributed between 5 %–15 % of the total Chl a. During this period, diatoms continued to dominate mesocosm 7 but decreased gradually from 65 %–15 % of the phytoplankton community. Notably, in mesocosm 6, Cryptophyceae contributed a moderate amount to the Chl a pool beginning on day 15 (25 %) and gradually increased to 40 % by day 20. Mesocosms 7, 5, and 8 increased in Cryptophyceae as well, but amounts were limited to ∼ 10 %–25 %. Similarly to surface waters, dinoflagellates dominated the Chl a pool by day 20 and contributed 50 %–80 % of the Chl a pool; however, there was considerably greater variability in dinoflagellate abundance after day 20 in subsurface waters. Chlorophyceae remained a significant contributor for the rest of the experiment, typically ranging between 10 % and 25 % of the phytoplankton relative abundance. In mesocosms 7, 5, and 8, diatoms became the dominant contributor, totaling ∼ 50 % of the phytoplankton between days 32 and 44. By the final days of the experiment (days 48–50), dinoflagellates again made up most of the phytoplankton community (60 %–75 %). Pelagophyceae, Prymnesiophyceae, and Cyanophyceae (referred to here as Synechococcus) remained minor contributors throughout the entire experiment but showed maximum contributions of < 10 % on days 10–16. Mesocosm 6 showed a minor contribution (< 10 %) of Synechococcus from days 42–50 and, in mesocosm 7, from days 34–42.
3.5 IPL class distributions
The IPL distributions throughout the study can be summarized by the relative abundances of different classes determined by their polar head groups. IPL distributions were broadly consistent between mesocosms and treatments during the experiment but showed significant differences between surface and subsurface waters (Fig. 2d). Glycolipids such as SQDG, DGDG, and MGDG typically made up ∼ 50 %–75 % of the total IPL pool in the surface, whereas phospholipids such as PC and PG were dominant in the subsurface (∼ 50 %–75 %). The changes in IPL distribution from the surface samples over the course of the experiment were most apparent from days 10 to 12, 20 to 24, and 24 to 38. Day 10 of the experiment marks the sampling period immediately preceding the ODZ water addition and was the only day with a significant fraction of BLs (betaine lipids) in the total lipid pool (ranging from 25 %–30 % in the surface samples). Mesocosms 6 and 8 showed a similar distribution between days 10 and 12 of the experiment with large contributions of SQDGs (∼ 50 %), BLs (∼ 25 %–30 %), and MGDGs (∼ 20 %), while mesocosms 7 and 5 showed considerably more PCs (∼ 25 %–40 %) at the expense of SQDGs (< 10 %). In the 2 weeks following the deep-water addition (days 12 to 24), we saw increasing (albeit variable) relative abundances of PGs (from 5 %–50 %) and DGDGs (from 5 %–25 %) in surface waters. MGDGs made up a considerably larger fraction during this time in mesocosm 6 (up to 50 %); were more moderate in mesocosms 7, 5, and 8 (typically ∼ 25 %); and yet decreased in all mesocosms to < 5 % by day 24. The final sample days (38 and 50) showed a resurgence of glycolipid contributions, namely in SQDGs (25 %–55 %) and MGDGs (5 %–25 %), with moderate contributions of PGs (5 %–20 %) and DGDGs (5 %–20 %).
In the subsurface samples before the ODZ water addition, mesocosms 6, 7, and 8 showed moderate contributions of MGDGs (15 %–25 %), which were lower in mesocosm 5 (< 5 %). There were higher contributions of SQDGs in mesocosms 6 and 8 (50 % and 35 %, respectively) than in 7 and 5 (20 %–25 %). Instead, mesocosms 7 and 5 showed a greater contribution of PCs (45 %–55 %). All four mesocosms demonstrated some component of PGs ranging from 5 %–20 % of the total IPL pool. The 2 weeks following the ODZ water addition showed highly variable fluctuations between PCs and PGs as the dominant IPL classes, with the contributions of glycolipids (25 %–40 %) and betaine lipids (< 5 %) remaining consistent. Notably, day 24 marked a consistently low contribution of PCs, which persisted until the end of the experiment. The final sample days (38 and 50) showed similar contributions among MGDGs, SQDGs, and PGs that together dominated the total lipid pool.
3.6 Multiple linear regressions
We found statistically significant (p<0.05) linear relationships between the relative abundance of individual IPLs and environmental factors (Fig. 3). In surface waters, pHT showed several significant responses towards DGDGs, MGDGs, PGs, PEs, and SQDGs. Classes DGDG, MGDG, PE, and PG showed negative linear relationships with pHT. SQDGs (specifically SQDG-32:0), however, showed a strong positive linear response to pHT. In the subsurface, pHT led to significant linear effects only on SQDGs with a strong negative linear correlation.
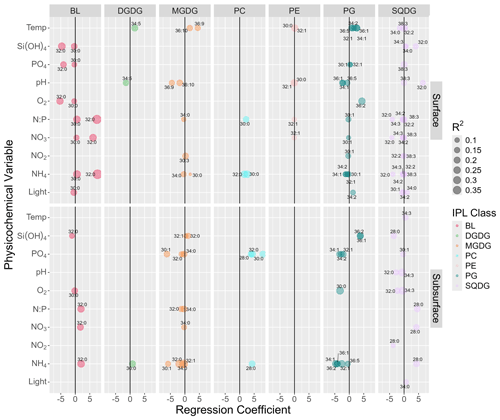
Figure 3Summary of multiple linear regression analysis between the abundances of major IPL molecules (> 2.5 % of total IPL pool) and physicochemical parameters showing only statistically significant (p<0.05) linear responses after controlling for the false discovery rate using a 0.1 alpha cutoff on adjusted p values. The size of circles indicates the magnitude of the linear-regression-adjusted R2. The upper and lower panels represent surface and subsurface waters, respectively. Numbers next to circles indicate the total number of carbon atoms and double bonds in core fatty acid chains.
The temperature of surface waters showed predominantly positive regression coefficients with several PG, MGDG, and DGDG molecules, with inconsistent correlations found in SQDGs. Whereas some PEs showed significant linear relationships with temperature, their regression coefficients were near 0. Similarly, in the subsurface, only one IPL structure (SQDG-34:3) showed a significant positive linear response but with a regression coefficient near 0.
Oxygen concentrations showed few linear correlations at the surface and were limited to two BLs (negative) and one PG (positive). In the subsurface, the strongest linear correlations were found between oxygen and PGs and SQDGs (negative).
Nutrient concentrations showed many significant linear responses, with regression coefficients of higher magnitude (up to ± 8) and adjusted R2 values of up to 0.5. The concentration of various forms of inorganic nitrogen had largely positive linear responses to BLs and PCs both at the surface and in the subsurface, with strongly negative responses in PGs and subsurface MGDGs. Among the SQDGs, many individual molecular species, with different fatty acid chains, responded linearly to all forms of inorganic N; the signs of these relationships were negative at the surface and generally positive in the subsurface (aside from NO). DGDGs showed only one positive linear response in the subsurface. PEs showed little response to inorganic nitrogen concentrations (regression coefficients near 0). PO concentrations showed only a few significant responses from BLs (negative) and PGs (slightly positive) at the surface. However, in the subsurface, PO showed strong linear responses (negative) to several MGDG and PG molecules and strong positive relationships with several PCs.
Light showed few linear responses amongst IPL relative abundances. At the surface, the strongest relationships were amongst PG (positive) and SQDG (mixed signs) and to a slight degree BLs (negative). In the subsurface, only one SQDG with a significant linear relationship was noted, and this was with a weak regression coefficient, indicating a range of light saturation with little to no linear effect on IPL distributions.
3.7 CART and random forest
All the predictive tree-based models showed improved performance with the inclusion of environmental variables (Figs. 4–7). The CART decision trees iteratively identified the key biological and physicochemical variables producing the best-performing model in the prediction of IPL concentrations. The random forest analysis complements these best-fit decision trees by calculating the percent reduction in the root mean square error (RMSE) associated with each variable. In conjunction, these two analyses highlight the most impactful variables in predicting the concentrations of a given IPL class. Overall, model performance amongst each IPL class can be compared by the strength of the correlation coefficients between observed and predicted concentrations and by the magnitude of the RMSE (Figs. 4–7).
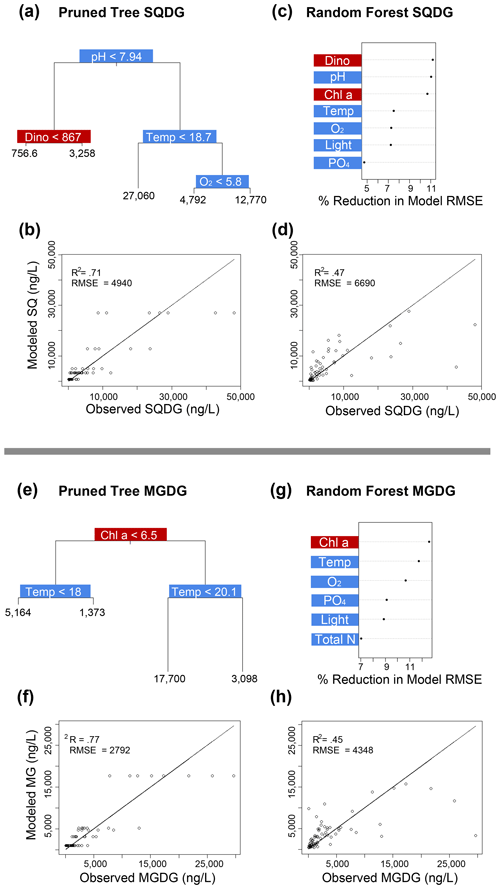
Figure 4Classification and regression tree (CART) and random forest analyses of selected IPL classes (top: SQDG; bottom: MGDG). Panels (a) and (e) indicate a summary of primary predictors in the best-fit CART (i.e., pruned tree), whereas panels (b) and (f) show model performance via adjusted R2 and RMSE. Panels (c) and (g) display random forest variable importance in the prediction of IPL classes as defined by percent reduction in RMSE, whereas panels (d) and (h) show model performance via adjusted R2 and RMSE. Environmental variables are depicted in blue, whereas biological variables are shown in red for both analyses.
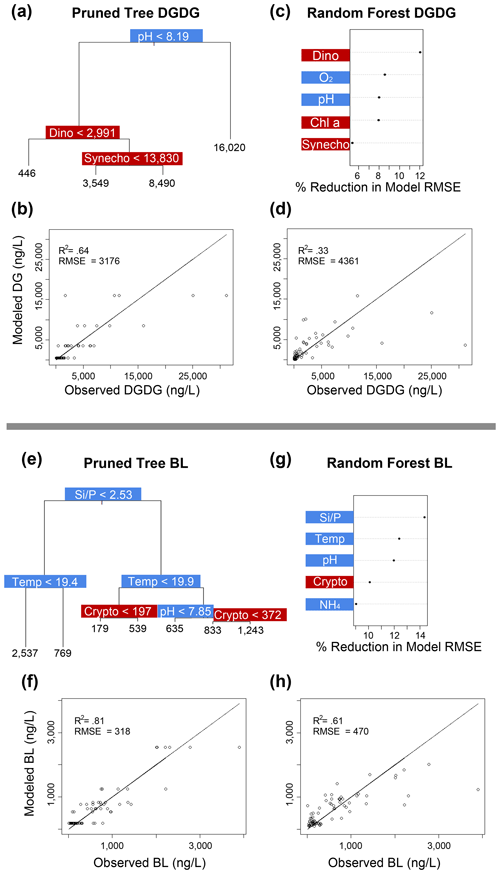
Figure 5Classification and regression tree (CART) and random forest analyses of selected IPL classes (top: DGDG; bottom: BL). Panels (a) and (e) indicate a summary of primary predictors in the best-fit CART (i.e., pruned tree), whereas panels (b) and (f) show model performance via adjusted R2 and RMSE. Panels (c) and (g) display random forest variable importance in the prediction of IPL classes as defined by percent reduction in RMSE, whereas panels (d) and (h) show model performance via adjusted R2 and RMSE. Environmental variables are depicted in blue, whereas biological variables are shown in red for both analyses.
Amongst several IPL classes (i.e., SQDGs, DGDGs, and BLs), pHT was consistently a significant contributing variable to IPL concentrations, as demonstrated by both the CART and the random forest analyses (Figs. 4a–d and 5). Notably, pHT was identified as the most important variable amongst the best-fit decision trees for both SQDGs and DGDGs, as well as the random forest model for SQDGs. Oxygen concentration was also frequently identified as a major contributing variable to model performance with MGDGs, DGDGs, PEs, and PCs (Figs. 4e–h, 5a–d, 6e–h, and 7a–d, respectively) and as moderately important with SQDGs (Fig. 4a–d). Temperature was selected as the most important variable in PE predictions (Fig. 6e–h) and a major contributing variable in SQDG, MGDG, BL, PG, and PC predictions (Figs. 4–7). Various forms of biologically available nitrogen were also important in the prediction of MGDG (Fig. 4e–h), BLs (NH; Fig. 5e–h), PE (NH; Fig. 6e–h), and PG (NH and NO; Fig. 6a–d). PO concentrations showed significant contribution to model performance amongst BLs (Fig. 5e–h, denoted as Si:P ratios), SQDGs, MGDGs, PGs, and PEs (Figs. 4a–d and e–h and 6a–d and e–h, respectively). Finally, light availability demonstrated secondary but significant importance in the prediction of SQDGs, MGDGs, PGs, PEs, and PCs (Figs. 4a–d and e–h, 6a–d and e–h, and 7, respectively).
Variables indicative of biological abundance were also identified as highly impactful for model performance, with Chl a concentration showing a significant contribution to all lipid classes except BLs (Figs. 4–7). Individual phytoplankton abundances were also shown to be important predictive variables, such as Cryptophyceae abundances for BLs; dinoflagellate abundances for DGDGs and SQDGs; Synechococcus abundances for DGDGs, PCs, PEs, and PGs; and diatoms for PCs.
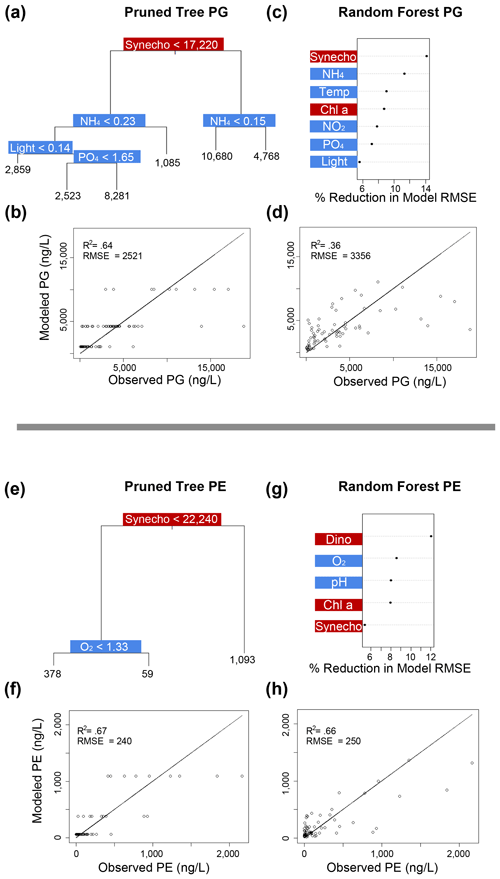
Figure 6Classification and regression tree (CART) and random forest analyses of selected IPL classes (top: PG; bottom: PE). Panels (a) and (e) indicate a summary of primary predictors in the best-fit CART (i.e., pruned tree), whereas panels (b) and (f) show model performance via adjusted R2 and RMSE. Panels (c) and (g) display random forest variable importance in the prediction of IPL classes as defined by percent reduction in RMSE, whereas panels (d) and (h) show model performance via adjusted R2 and RMSE. Environmental variables are depicted in blue, whereas biological variables are shown in red for both analyses.
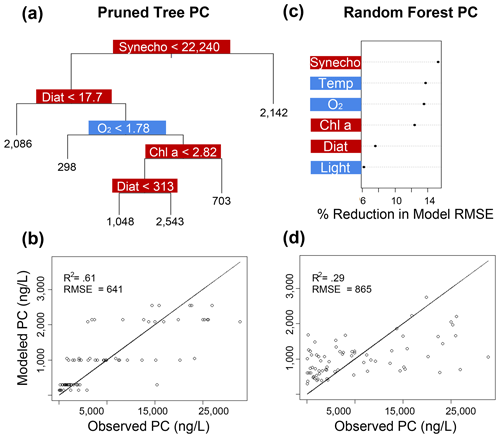
Figure 7Classification and regression tree (CART) and random forest analyses of selected IPL classes (PC). Panel (a) indicates a summary of primary predictors in the best-fit CART (i.e., pruned tree), whereas panel (b) shows model performance via adjusted R2 and RMSE. Panel (c) displays random forest variable importance in the prediction of IPL classes as defined by percent reduction in RMSE, whereas panel (d) shows model performance via adjusted R2 and RMSE. Environmental variables are depicted in blue, whereas biological variables are shown in red for both analyses.
3.8 Water treatments
The experiment consisted of applying two different treatments to the mesocosms, aimed at exploring the varying impacts of upwelling of ODZ waters with contrasting geochemical properties. The first treatment saw the introduction of water from a coastal area (station 1) with ODZ waters with a very low N:P ratio (0.1; see Table 1 and Fig. 2b) into the mesocosm. The second treatment performed the same process but with ODZ water from an offshore area (station 3) with a low N:P ratio (1.7; Table 1 and Fig. 2b). Despite the different chemical signatures of the added water masses, the resulting nutrient stoichiometries within the mesocosms were similar in between both treatments, likely due to both dilution effects and the time passed between water collection and addition (see Bach et al., 2020). We see largely similar responses in the IPL distributions, as well as in other biogeochemical variables between these two treatments; therefore, we largely focus our discussion on the temporal variation and differences between surface and subsurface environments in these analyses.
4.1 Biological abundances as drivers of IPL distributions
We expect phytoplankton abundances to exert first-order control on the production and distribution of IPLs in this experiment. The majority of the detected molecules have been demonstrated to be chemotaxonomic biomarkers of planktonic biomass (Sturt et al., 2004; Schubotz et al., 2009; Wakeham et al., 2012; Van Mooy and Fredricks, 2010; Cantarero et al., 2020). Previous work on the Humboldt Current System shows that the ratio of total IPLs to particulate organic carbon (POC) is high at the chlorophyll maximum and that the composition of IPLs found in these surface waters is consistent with predominantly phytoplanktonic biomass (Cantarero et al., 2020). In this mesocosm experiment, the depths of the chlorophyll maximum and oxycline are compressed into a 20 m water column which likely drives a greater contribution of phytoplanktonic IPLs in ODZ waters than would be expected in the natural environment. While we suggest that most of the biomass (and IPL content) measured at these high-chlorophyll depths is likely derived from phytoplankton, we cannot completely isolate or quantify the contribution of bacterial biomass to the total IPL pool.
Most IPL classes demonstrate phytoplankton abundances as a primary or major predictor in the CART and random forest analyses, such as dinoflagellates in the prediction of SQDGs (Fig. 4a, c), DGDGs (Figs. 5a, c), and PE (Fig. 6g); Synechococcus in the prediction of PGs (Fig. 6a, c), PEs (Fig. 6e, g), and PCs (Fig. 7a, c); and Chl a in the prediction of every IPL class barring BLs (a mostly minor IPL class in this experiment). Chl a appears to be most important in highly abundant IPL classes as an indicator of overall photosynthetic productivity, and dinoflagellates dominate the overall phytoplankton biomass for almost the entirety of the experiment after ODZ water addition. Synechococcus demonstrates covariance with the total phytoplankton biomass, yet it remains a relatively minor phytoplankton class. We suggest that the prevalence of biological sources as IPL predictors in the decision tree analyses generally indicates the variability in total phytoplankton biomass throughout the experiment.
Among phytoplankton, the glycolipids MGDG, DGDG, and SQDG are predominantly found in thylakoid membranes, whereas the phospholipids PC and PE, as well as BLs, are structural components in the cell membrane lipid bilayer (note PG is found in both; Guschina and Harwood, 2013). It is important to recognize that the relative proportions of IPLs vary in different phytoplankton classes (Harwood and Jones, 1989; Wada and Murata, 2009) and that many of these lipids, in particular phospholipids, can also be derived from heterotrophic bacterial biomass (Popendorf et al., 2011). Thus, the overall community composition is expected to be a major driver of IPL distributions in this system. However, given the prevalence of algal biomass and the steps taken to minimize bacterial contributions to the IPL pool (see Sect. 2.5), we focus our analysis on that of phytoplanktonic dynamics. We recognize that both phytoplankton abundances and the total planktonic community composition play a major role in the distribution of IPLs. Thus, while the data presented here may refine the phylogenetic association between biological sources and IPLs in marine systems, our main aim is to explore the role of multiple environmental forcings as an additional control on IPL distributions. The following sections focus on the evidence for direct environmental influence on IPL remodeling amongst the phytoplankton community and the potential implications of these physiological responses to broader aspects of ocean biogeochemistry.
4.2 Environmental variables as drivers of IPL remodeling
Since community composition can change concurrently and/or in response to environmental conditions, we employed two distinct strategies to isolate the role of lipid remodeling as a physiological response to environmental forcing only; firstly, the MLRs which subtract the variability explained by phytoplankton abundance (e.g., CHEMTAX results) in pairwise correlations between lipid abundances and environmental variables, and secondly, the decision tree analyses (CART and random forest) which rank variables by their impacts on the model performance.
Across virtually every major IPL class common to eukaryotic phytoplankton, we see evidence of environmental conditions exerting significant control on polar head group distributions in the MLRs (Fig. 3). Similarly, nutrient concentrations, pHT, temperature, O2 concentration, and light availability are consistently identified as statistically important variables in the prediction of IPL head groups in both the CART and the random forest analyses (Figs. 4–7). In addition, high-level comparisons in the relative abundances of IPLs and phytoplankton groups (Fig. 3c, d) suggest that certain environmental conditions may be associated with major shifts in IPL distributions.
An important distinction between the MLRs and the decision trees is that the MLRs are calculated within individual depth environments (surface and subsurface) to explore statistically significant linear relationships between abundant IPL molecules and changing environmental conditions. On the other hand, the decision trees explore the predictive power of physicochemical differences between the surface and subsurface environments for IPL distributions. The results of these two analyses are meant to be complementary in that they focus on the differences in environmental conditions between water depths as well as the temporal development of conditions within a given depth over the course of the experiment.
4.2.1 Nutrient availability
Nutrient limitation amongst phytoplankton leads to transitions in cellular activity, from the biosynthesis of growth and reproduction cellular components such as cell membranes to energy-storing molecules (Guschina and Harwood, 2013; Zienkiewicz et al., 2016). Nitrogen, an essential nutrient in photosynthesis and the biosynthesis of proteins/enzymes and nucleic acids, is typically acquired by marine phytoplankton through inorganic nitrogen species such as NO and NH. Some phytoplankton can also utilize organic nitrogen sources (Bronk et al., 2007), whereas diazotrophic cyanobacteria can fix dinitrogen gas into bioavailable nitrogen. The coastal region of the ETSP is typically considered to be seasonally co-limited by light, N, and Fe (Messié and Chavez, 2015). However, along the Peruvian shelf, Fe concentrations are elevated compared to offshore waters (Hutchins et al., 2002; Browning et al., 2018), and Fe is not considered a limiting source in this mesocosm experiment (Bach et al., 2020). In our mesocosm systems, the inorganic N:P ratio ranged between 0.13 and 4.67, with higher inorganic N in subsurface waters compared to the surface and with a N:P minimum reached by day 20. Bach et al. (2020) noted that a week after the ODZ water addition, increases in the ratios of particulate organic carbon to biogenic silica coincided with low inorganic N and high Si(OH)4 concentrations, suggesting a N-limited system. Thus, we consider our system to be overall nitrogen limited with varying degrees of severity throughout the course of the experiment. This N limitation is also reflected in the transition from predominantly diatoms, Chlorophyceae, and Cryptophyceae to a dominance of mixotrophic dinoflagellates approximately 4–6 d after the initial ODZ water treatment (Bach et al., 2020). Such shifts are consistent with the ecological advantage that dinoflagellates exhibit under N-limiting conditions as they can extract nitrogen from the dissolved organic nitrogen (DON) pool (Kudela et al., 2010) as well as from heterotrophy (Smalley et al., 2003).
In our study, all four mesocosms experienced limitations in inorganic nitrogen (as low as 0.24 µmol L−1) and consistently high concentrations of PO (ranging from 1.3 to 2.3 µmol L−1) throughout the entire experiment. Random forest analysis shows that the inorganic N concentration is an important predictor in the abundance of BL, PG, and MGDG (Figs. 5g, 6a, and 4e, respectively). The MLRs also indicate that many individual molecules from nearly every IPL head group have significant linear correlations with inorganic N concentrations (Fig. 3). Notably, the distributions of several abundant PCs and BLs with N in the head group structure are consistently positively correlated with inorganic N species, whereas other non-N-bearing IPLs are generally negatively correlated with inorganic N concentration (PG, SQDG, and MGDG), meaning that they are proportionally more abundant under more severe N limitation.
Under P limitation, phytoplankton are known to substitute non-phosphorus-containing glycolipids for phospholipids and reallocate the liberated P for other cellular demands (Van Mooy et al., 2009). We hypothesize that non-N-containing glycolipids and phospholipids may similarly be substituted for IPLs such as PCs and BLs as a mechanism for alleviating cellular N demand in low-inorganic-N conditions. Both PC and BL are found in extrachloroplast membranes (Kumari et al., 2013), whereas IPLs found in thylakoid membranes such as MGDG, PG, and SQDG are essential to the photosynthetic machinery. Indeed, the average ratio of total IPLs Chl a is up to 3 times higher at depth than in surface waters (Fig. S5), possibly pointing towards a reduced proportion of membrane lipids among phytoplankton subject to environmental stressors such as nutrient limitation (likely in addition to oxygen availability, temperature, and light levels). We note that at least a fraction of this trend could also be explained by the contribution of IPLs from heterotrophic bacteria.
More generally, nutrient limitation can cause phytoplankton to accumulate highly concentrated stores of energy in the form of triacylglycerols (TAGs) through the activation of multiple biosynthetic pathways (Zienkiewicz et al., 2016). These include synthesis via acyl units donated from phospholipids via the PDAT (phospholipid : diacylglycerol acyltransferase) enzyme (Dahlqvist et al., 2000) or other chloroplast membranes, as demonstrated in the homologous enzyme Cr-PDAT (Yoon et al., 2012), as well as several DGATs (diacylglycerol : acyl-CoA acyltransferases; Li et al., 2012; Li-Beisson et al., 2019). These enzymes represent significant pathways for TAG accumulation (Popko et al., 2016; Gu et al., 2021) are sensitive to N availability (Yoon et al., 2012; Li et al., 2012), and their encoding genes have so far been identified in green algae, diatoms, and heterokonts (Zienkiewicz et al., 2016). Because our dataset does not include TAG production, further work on this aspect could reveal whether the proportional changes in dominant phytoplanktonic IPLs correlated to N availability are also associated with TAG synthesis and, if so, determine whether recycling of membrane lipids is a significant contributor to these observed community-level distributions.
4.2.2 Inorganic carbon availability and pHT
Coastal upwelling zones are characterized by low-pHT subsurface waters associated with ODZs, where high fluxes of organic substrates sustain enhanced microbial respiration and the accumulation of CO2 (Capone and Hutchins, 2013). Thus, we explore evidence for membrane lipid remodeling amongst phytoplankton as a physiological response to varying pHT. We see evidence of pHT acting as a potential control on the composition of IPL head groups, particularly amongst SQDGs and DGDGs, as noted by the high importance rankings in both the CART and the random forest analyses (Figs. 4a and c and 5a and c). This likely represents the relatively high abundance of these glycolipids in the surface samples where the pHT is 0.2–0.6 higher than at depth. The MLRs show several negative correlations between MGDG, PG, and SQDG molecules containing unsaturated or polyunsaturated fatty acids with pHT (Fig. 3). The observed increased proportion of unsaturated IPLs at lower pHT is most apparent in surface waters where the variability in pHT is greatest (± 0.2). It has been suggested that lower pHT can induce greater proportions of saturated fatty acids as a mechanism to reduce membrane fluidity and prevent high proton concentrations in the cytoplasm (Tatsuzawa et al., 1996). However, this response remains limited to more extreme pHT ranges (e.g., ∼ 1–10), suggesting that other environmental factors (e.g., nutrient availability) are overprinting the potential impacts of pH on fatty acid saturation.
Rather than a direct consequence of modest changes in pHT on algal membrane fluidity, the observed changes in fatty acid profiles may be in part a response to the available forms of inorganic carbon for photosynthesis. Following the ODZ water addition, DIC (dissolved inorganic carbon) and pCO2 rapidly declined within a few days (Chen et al., 2022) due to high productivity. In natural waters, pCO2 maxima occur in ODZ waters where respiration rates are high (Vargas et al., 2021). Lower pCO2 at the surface may be a limiting factor for photosynthesis and growth; higher pHT in marine settings indicates a reliance on active transport of HCO for carbon fixation as opposed to a passive diffusion of CO2 (Azov et al., 1982; Moazami-Goudarzi et al., 2012). Enrichment of pCO2 has also been observed to induce an increased proportion of unsaturated fatty acids in microalgae (Morales et al., 2021), which may explain the negative correlation between the proportion of certain unsaturated glycolipids (as well as PGs) and pHT (high pCO2; see Fig. 3).
As mentioned above, phytoplankton can employ membrane lipids as substrates for TAG accumulation in phytoplankton under environmental stress. While nutrient limitation is often considered the primary regulator of TAG production, culture experiments also point towards the importance of pH and inorganic carbon availability (Guckert and Cooksey, 1990; Gardner et al., 2011), with different responses between a model diatom (Phaeodactylum tricornutum) and chlorophytes (CHLOR1 and Scenedesmus sp. WC-1), which is potentially related to individual carbon-concentrating mechanisms (Gardner et al., 2012). For instance, under nitrogen/phosphorus limitation, TAG production can be promoted if the supply of inorganic carbon is abundant (Peng et al., 2014). TAGs can also be synthesized and accumulated when inorganic carbon is limited by invoking the recycling of IPLs such as glycolipids and phospholipids (Peng et al., 2014). Thus, the higher proportions of SQDGs and DGDGs in the high-pH–low-CO2(aq) surface waters of our experiment could be in part related to their recycling under CO2 limitation.
Overall, our results are consistent with other experimental data in that pHT impacts the distribution of IPL head groups amongst certain phytoplankton groups. For instance, the relatively high glycolipid abundances in surface samples with higher pHT (most notably SQDG and DGDG; see Fig. S1) are likely not related to direct effects on membrane fluidity but rather to the availability of inorganic carbon and its effect on the recycling of phospholipids (as well as MGDGs), potentially for TAG synthesis. While our results are consistent with the IPL substrates for TAG synthesis observed so far (Dahlqvist et al., 2000; Yoon et al., 2012), additional analyses of TAG concentration and their its acid compositions amongst the phytoplanktonic community would aid in tracing the extent of membrane lipid degradation as a source of acyl units, as well as tracing the location of these biosynthetic pathways in the cell. Such work would aid in determining the extent to which TAG synthesis and IPL recycling relegate phytoplankton classes to certain depths of the water column based on the combined effects of nutrient availability and pHT (amongst other variables, e.g., light, temperature, O2).
4.2.3 Oxygen
Despite the harvesting of light energy during photosynthesis, photosynthetic organisms also rely on respiration for growth and free radical scavenging under both light and dark conditions (Raven and Beardall, 2003). Specifically, the availability of O2 (particularly during the dark phases or in low-light environments) influences the activation of metabolic responses, such as fermentative metabolism and acetate utilization (Yang et al., 2015). Differences in dark respiration rates relative to light-saturated photosynthesis among algae may confer advantages under varying oxygen availability (Geider and Osborne, 1989). Thus, the ability of some organisms to perform lipid remodeling in response to oxygen stress may partially shape the composition of the phytoplankton community.
In our analysis, the random forest models indicate a significant impact of O2 concentration in the prediction of nearly all IPL class abundances (Figs. 4–7). This pattern appears to be largely driven by differences in IPL distributions between surface (oxygenated) and subsurface (hypoxic; < ∼ 1.4 mL L−1 as defined by Naqvi et al., 2010) waters. Glycolipids (MGDG, DGDG, and SQDG) make up on average 28 % more of the IPL pool in the oxygenated surface waters (∼ 125–220 µmol L−1 O2) compared to oxygen-deficient subsurface waters (∼ 15–75 µmol L−1 O2). The MLRs, however, show no significant relationships between individual glycolipid relative abundances and O2 concentration in surface samples (Fig. 3); this is possibly due to surface waters remaining well oxygenated throughout the experiment. While several IPL moieties found in BLs, SQDGs, and PGs demonstrate mostly negative linear relationships (Fig. 3), we suggest that the most prominent and consistent relationships are driven by a major shift from oxic to hypoxic conditions (i.e., surface vs. subsurface) as opposed to a sensitivity to variable O2 concentrations.
Anaerobiosis amongst green algae has been demonstrated to impact lipid production, with significant reductions (by nearly 50 %) in polar lipid content and concomitant increases in fatty acids (Singh and Kumar, 1992). Gombos and Murata (1991) found that the cyanobacterium Prochlorothrix hollandica experienced a significant reduction in the relative abundance of MGDGs that coincided with moderate increases in SQDGs, DGDGs, and PGs under low-oxygen conditions. Furthermore, culture experiments of anaerobically grown Chlamydomonas reinhardtii resulted in both decreased membrane lipid yields (most notably amongst MGDGs and DGDGs; by > 50 %) and an accumulation of TAGs (Hemschemeier et al., 2013). It has been noted that oxygen stress appears to induce the degradation of fatty acids (∼ 30 % reduction under dark/anaerobic conditions), mostly amongst unsaturated fatty acids commonly found in MGDG and DGDG membrane lipids (16:4 and 18:3) used for TAG assembly (Liu, 2014; Hemschemeier et al., 2013). Glycolipids (i.e., MGDG and DGDG) appear to serve as important substrates for TAG production under low-oxygen conditions; however, beta oxidation of fatty acids requires oxygen to contribute to the degradation of acyl groups, potentially explaining why membrane lipid degradation is attenuated under more severe hypoxia (Liu, 2014). This physiological response to low-oxygen conditions in subsurface waters may explain the relatively high abundance of glycolipids in well-oxygenated surface waters.
Dinoflagellates have been shown to exhibit particularly high rates of dark respiration to light-saturated photosynthesis as compared to diatoms, Chlorophyceae, or most notably cyanobacteria (Geider and Osborne, 1989), possibly pointing towards a greater sensitivity to O2 concentrations. In addition to other environmental conditions, the greater relative abundance of Chlorophyceae, diatoms, and Cryptophyceae in the oxygen-deficient subsurface waters may reflect reduced respiration rates amongst these algae. Differences in the proportion of the glycolipids MGDG and DGDG amongst different algae, as well as their relative ability to recycle them under oxygen stress, may play a prominent role in their individual tolerances to oxygen limitation.
Higher proportions of glycolipids in surface waters may also be due to enhanced rates of microbial degradation under oxic conditions, which may be 2–4 times faster than under anoxic conditions, as tested in microcosm experiments (Ding and Sun, 2005). Relatively labile glycolipids can accumulate in the dissolved organic carbon pool (Gašparović et al., 2013). This observation aligns with the slower breakdown of SQDGs compared to phospholipids observed in IPL degradation experiments under aerobic conditions (Brandsma, 2011). This accumulation process, however, is unlikely in regions of the water column with large numbers of active living cells and highly active bacterial degradation. In fact, the distributions of IPLs across the ODZ of the ETSP indicate minor contributions of exported IPLs to greater depths, suggesting high surface recycling (Cantarero et al., 2020). However, specific experimental observations encompassing oxygen gradients ranging from well-oxygenated to fully anoxic conditions are necessary to derive more robust conclusions.
4.2.4 Temperature
Phytoplankton have been shown to respond variably to high growth temperatures depending on their individual tolerances (Huertas et al., 2011). Photosynthesis is considered the most heat-sensitive cellular function in photoautotrophs (Berry and Björkman, 1980). In this section we discuss the potential lipidomic responses to heat stress within the IPL distributions of the phytoplanktonic community. Temperature fluctuations affect membrane fluidity, a phenomenon commonly controlled by fatty acid desaturases (Sakamoto and Murata, 2002) that catalyze the production of unsaturated/saturated fatty acids to increase/decrease membrane fluidity (Lyon and Mock, 2014). We did not see evidence for temperature effects on the degree of unsaturation in our data (see Fig. S2). This is likely due to the overall narrow temperature range observed during the experiment (17.3–21.6 °C), which mirrors the natural variability observed in Callao (average monthly ranges ∼ 16.6–19.6 °C from 2017 to 2019; Masuda et al., 2023).
Despite the restricted temperature range and its lack of impact on the unsaturation degree of core lipids, the random forest analyses identified temperature as a significant variable in predicting all IPL classes based on their polar head groups aside from DGDG and PE (Figs. 4–7). This suggests that the response to temperature may vary among different IPL classes. The MLRs indicate consistently positive relationships between several glycolipids and temperature, suggesting a potential physiological compensation via membrane compositions for higher temperatures. Gašparović et al. (2013) noted an accumulation of glycolipids at temperatures > 19 °C in the northern Adriatic Sea, particularly from cyanobacterial synthesis of MGDGs. The sensitivity of photoautotrophs to thermal stress was also explored by Yang et al. (2006), who showed that DGDGs and MGDGs both increase the thermal stability of photosystem II, while phospholipids significantly decrease it. Experiments with a wild-type and mutant Chlamydomonas reinhardtii have shown that SQDGs are an essential component of thylakoid membranes to maintain stability under heat stress (Sato et al., 2003), although at considerably more extreme temperatures (41 °C). Heat stress has also been linked to the production of TAGs (Elsayed et al., 2017; Fakhry and El Maghraby, 2015), which can draw acyl units from degraded membrane lipids (Holm et al., 2022).
Interestingly, the relative abundance of DGDGs in our experiment shows the most prominent (R2 of 0.35–0.44), as well as statistically significant (p<0.05), linear relationship with temperature (see Fig. S3a). While temperature was not identified as an important variable for the prediction of DGDGs in either decision tree analysis, this may be due to other covariates, such as pH and O2, masking the effect of temperature. Our results indicate that phytoplankton may either produce DGDGs in greater abundance to alleviate thermal instability in photosystem II or preferentially degrade other thylakoid membranes (i.e., PGs, SQDGs, or MGDGs) in response to heat stress, leaving the remaining IPL pool relatively enriched in DGDGs. While several individual MGDG and SQDG molecules did demonstrate linear responses to temperature (Fig. 3), other stressors such as N availability, pH, and light levels may confound the effects of temperature on the overall abundance of these lipid classes.
4.2.5 Light availability
Bach et al. (2020) considered the overall productivity in these mesocosm experiments to be co-limited by N and light availability, which have been identified as key limiting factors in eastern boundary upwelling systems (Messié and Chavez, 2015). In our experiment, initial high biomass productivity led to self-shading effects that significantly reduced the PAR (Bach et al., 2020). While the maximum photon flux densities measured at noon over the course of the experiment were ∼ 500–600 µmol m−2 s−1, only ∼ 5 %–22 % and < 4 % were measured in surface (∼ 2 m) and subsurface (17 m) waters, respectively, from all four mesocosms. While both depth-integrated samples in our experiment are likely from light-limited planktonic communities, most PAR values (> 15 % i.e., 75–132 µmol m−2 s−1) in surface waters still demonstrate sufficiently high levels to be able to affect lipid production and accumulation (Gonçalves et al., 2013), especially under the combined effects of N deprivation (Yeesang and Cheirsilp, 2011; Jiang et al., 2011).
Light levels are identified by the random forest analyses to be a significant variable in the prediction of MGDGs, SQDGs, PGs, and PCs (Figs. 4, 6, and 7). While few studies have explored the direct effects of irradiance levels on IPL head group distributions, experimental cultures of Tichocarpus crinitus found increased IPL production of SQDGs, PGs, and PCs amongst shade-grown algae (Khotimchenko and Yakovleva, 2005). In Tichocarpus crinitus cultures, MGDGs, one of the most abundant thylakoid lipids, show decreased abundances under low-light conditions (Khotimchenko and Yakovleva, 2005). Our results show that both MGDGs and SQDGs are present in greater relative and absolute abundances in the surface waters where light is less limited or potentially inhibitive at times, suggesting an impact on thylakoid lipid compositions in terms of maintaining efficient photosynthetic rates under changing light conditions. MGDGs have been shown to provide important photoprotective and antioxidant mechanisms in diatoms (Wilhelm et al., 2014), potentially explaining their higher abundance in the surface waters and the significance of light in the random forest models. While the exact function of SQDGs in thylakoid membranes remains unclear, they have been observed to act as an antagonist to the aggregating action of MGDGs (Goss et al., 2009), leading to a disaggregating effect on light-harvesting complexes (Wilhelm et al., 2014). The latter potentially reflects planktonic responses to variable PAR (5 %–22 %) in the surface via adjustment of the proportions of SQDGs and MGDGs in the thylakoids (Gabruk et al., 2017; Nakajima et al., 2018).
The PC lipid class, primarily found in extrachloroplast membranes (Mimouni et al., 2018), consistently exhibits greater relative abundance in the subsurface compared to surface waters. We suggest that light levels at depth may play a role (albeit a secondary one; see Fig. S3b) in non-chloroplast IPL production when algal cells are configured for rapid growth and reproduction. Conversely, under higher light as well as under the combined effects of DIC and N limitation at the surface, algal cells are more prone to survival responses such as TAG production at the expense of IPLs. PG can be found in extrachloroplast membranes but is also an essential component of photosystems I and II, with important roles in electron transport processes (Sakurai et al., 2003; Wada and Murata, 2009; Kobayashi et al., 2017). The overall abundance of PGs contributing to the total IPL pool may be influenced by varying production of certain non-chloroplast membranes under lower-light conditions and other thylakoid membranes under high-light conditions, thus possibly explaining the highly variable overall contribution of PGs to the IPL pool. Additional analyses of specific fatty acid structures from intact PGs may illuminate sources within the cell, i.e., thylakoid PGs and extrachloroplastic PGs.
Light intensity has also been shown to have contradictory impacts on fatty acid composition, with some analyses observing increased unsaturation under high light intensity (Liu et al., 2012; Pal et al., 2011). Others have observed that excess light energy induces the synthesis of saturated fatty acids (SFAs) or monounsaturated fatty acids (MUFAs) in several algal species to prevent photochemical damage or the synthesis of polyunsaturated fatty acids (PUFAs) for the maintenance of photosynthetic membranes under low-light conditions (Khoeyi et al., 2012; Fábregas et al., 2004; Sukenik et al., 1993; Orcutt and Patterson, 1974). However, we see limited evidence in this experiment of a direct correlation between light intensities and fatty acid composition in the multiple linear regressions (Fig. 3). This may be related to differences in optimal irradiance levels between algal groups overlapping to confound any clear patterns.
Given that the normalized PAR in surface waters remains < 25 % and that light appears as a variable of significant but secondary importance in the random forest models, our results suggest that under these experimental conditions, low or variable light availability may amplify the effects of other environmental stressors (i.e., nitrogen availability, oxygen concentration, temperature, and inorganic carbon availability). The effects of self-shading from high biomass production early in the experiment, when greater nutrient availability was high, likely contributed to the markedly different IPL distributions observed between surface and subsurface waters. As a result, the fluctuations in PAR may play a role in the observed variations in the relative proportions of prevalent thylakoid membrane IPLs, including MGDGs, SQDGs, and PGs.
4.3 Implications of IPL remodeling in phytoplankton membranes
We expect the ongoing changing conditions in the ETSP, such as rising temperatures, ocean acidification, expanding ODZs, and shifts in upwelling-driven nutrient supply, to instigate multiple physiological responses amongst phytoplankton communities. IPL remodeling and the reallocation of resources among algae are likely to have cascading effects on the composition of phytoplanktonic communities, the nutritional value of algal biomass to higher trophic levels, and the cycling of organic carbon and nitrogen in the upper ocean. Here, we explore the potential consequences of these physiological responses to environmental conditions and outline future areas of investigation to better constrain the impacts of IPL remodeling on marine biogeochemistry.
4.3.1 Relative adaptability of phytoplankton classes to environmental change
IPL classes are partitioned either to the thylakoid membranes (i.e., MGDG, DGDG, SQDG, and PG) in variable distributions to maintain photosynthetic function or to extraplastidic membranes under growth and reproductive phases (BL, PC, PE, and PG). As environmental conditions change, the ability of phytoplankton to adjust these IPL compositions may be an important driver controlling the phytoplankton community structure. Within a few days of the ODZ water addition, the mesocosms became oligotrophic, which coincided with a major shift in phytoplankton groups, from predominantly diatoms, Chlorophyceae, and Cryptophyceae to a dominance of “survivalist” mixotrophic dinoflagellates. An obvious advantage of mixotrophic dinoflagellates to the largely N-limited conditions of this experiment comprises their ability to extract N from the DON pool and their having a lower dependence on light compared to the other previously mentioned phytoplankton groups.
To contextualize the impacts of IPL remodeling on the phytoplanktonic community, we performed an additional random forest analysis to identify the most important IPLs (both individual moieties and polar head group totals) in the prediction of individual phytoplankton classes. We applied this analysis to identify what IPL remodeling processes may be more readily available to each major phytoplankton class in this experiment (Fig. 8). While all the phytoplankton classes are likely to produce each IPL class to varying extents, the differences in their relative distributions may provide insight into differences in phytoplankton adaptability under changing environmental conditions. Dinoflagellates are heavily associated with high abundances of the glycolipids SQDG and DGDG, with far less apparent dependence on phospholipids and MGDGs (Fig. 8). The dominance of dinoflagellate biomass is attributed to the severe scarcity of inorganic nitrogen and relatively low light intensity due to self-shading effects (Bach et al., 2020). Dinoflagellates may also take advantage of the recycling of N-bearing PCs and BLs to alleviate nitrogen limitation or to produce energy-storing TAGs. The high proportion of DGDGs and SQDGs and recycling of MGDGs may also prove advantageous under high-pH–low-pCO2 conditions in surface waters, alleviating pH stressors or the energy investment in active HCO transport for photosynthesis. These IPL remodeling strategies may have played a role in the dominance of dinoflagellates under the post-upwelling experimental conditions.
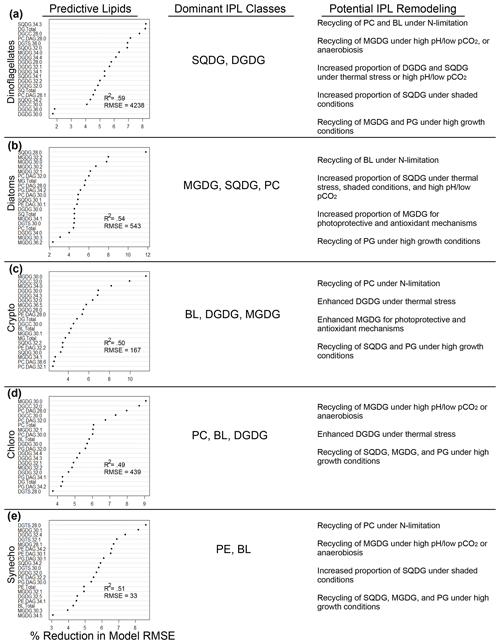
Figure 8Random-forest-based identification of the 20 most important individual IPLs in the prediction of phytoplankton groups. Dominant IPL classes and the potential remodeling advantages for each group are summarized. The nomenclature of IPL molecules follows that of the main text, starting with the type of head group, followed by the number of carbon atoms in fatty acid chains and the total number of double bonds in the fatty acid chains. The root mean square error (RMSE) of random forest models is defined as ng L−1 of Chl a, and the coefficient of determination (R2) is provided for each model fit.
In the early phases of the experiment and when inorganic N is readily available, diatoms (and to a lesser extent Cryptophyceae and Chlorophyceae) dominate the water column biomass. Each of these phytoplankton classes (as well as Synechococcus) indicates a greater dependence of the N-bearing PCs and/or BLs, potentially signaling a reduced capacity to scavenge these extrachloroplastic IPLs within the cell for N or as substrates for TAG production. Notably, these phytoplankton consistently make up a greater proportion of the total Chl a pool in subsurface waters, suggesting an ability to accommodate the lower O2 concentrations and pH in exchange for greater N availability (see summary in Fig. 8). Some of this adaptability may be related to the recycling of MGDGs for additional acyl units under hypoxia (most likely amongst Chlorophyceae and Synechococcus) and/or the generally higher relative production of structural membrane lipids under low-light growth conditions (see discussion about the impact of light in Sect. 4.2.5).
In the eastern tropical South Pacific (ETSP), upwelling events exhibit a distinctive trend of increasing frequency and intensity, setting the area apart from other eastern boundary systems (Abrahams et al., 2021; Oyarzún and Brierley, 2019). This phenomenon brings about important changes in environmental conditions, including a decrease in sea surface temperature (∼ 0.37° C, average from 1982 to 2019; Abrahams et al., 2021) alongside oxygen reduction and reduced pH in the coastal zones of the region (Pitcher et al., 2021). Simultaneously, there is an observed boost in primary productivity (Gutiérrez et al., 2011; Tretkoff, 2011). The impact of strong upwelling, followed by rapid thermal stratification when upwelling subsides, could signify abrupt changes that affect the community structure of phytoplankton (Gutiérrez et al., 2011). Such environmental changes favor species better adapted to stress conditions, such as mixotrophic dinoflagellates and silicoflagellates, at the expense of diatom populations (Hallegraeff, 2010), which could translate into a decrease in highly nutritious saturated fatty acids for higher trophic levels (Hauss et al., 2012). Furthermore, a potential reduction in diatom biomass and diversity could have consequences for the ocean biological carbon pump (Tréguer et al., 2018).
Under the ongoing and projected scenarios of climate change, our mesocosm experiment demonstrates how shifts in the phytoplankton community translate into changes in the lipid composition of their cell membranes. This adds weight to the school of thought that lipid remodeling amongst the phytoplankton community could have repercussions at higher trophic levels, as recently discussed by Holm et al. (2022), and on ocean biogeochemistry, as we discuss below.
4.3.2 Cycling of carbon, nitrogen, and sulfur
Combined, neutral (TAGs) and polar lipids (IPLs) make up on the order of 17.6 % to 34.7 % of the cell by dry weight under N-replete and N-deprived conditions, respectively (Morales et al., 2021), with other environmental stressors able to compound the accumulation of neutral lipids (Zienkiewicz et al., 2016). This represents a considerable portion of algal cellular carbon and total water column carbon stocks that supply the transfer of fatty acids to higher trophic levels (Twining et al., 2020). Trophic transfer of fatty acids to grazing zooplankton may be affected by the relative contribution of polar and neutral lipids, as they can have significantly different turnover rates (Burian et al., 2020). This suggests that the relative concentrations of TAGs/IPLs in phytoplanktonic communities may impact the rates of fatty acid remineralization or uptake into higher trophic levels. Additionally, while our analysis did not focus on individual fatty acid structures, other experiments investigating the effects of increasing pCO2 and nitrogen deficiency have demonstrated a reduction in the relative production of essential fatty acids resulting in negative reproductive effects on primary consumers (Meyers et al., 2019). In this context, our IPL results reveal modifications (among polar head groups, carbon numbers, and unsaturations) in response to divergent environmental conditions. However, further investigation of the relative concentrations and turnover rates between TAGs and IPLs, in addition to the production of essential fatty acids, is a critical next step in assessing the impacts of IPL remodeling on trophic transfer processes.
While IPLs are not a major source of cellular N compared to proteins, the highly labile nature of these molecules after cell death may be a potential source of rapidly recycled N via bacterial hydrolysis. On average, N-bearing IPLs such as PCs or BLs consist of ∼ 2 % N by molecular weight (estimated based on a typical 700 g mol−1 betaine lipid). Based on their abundance, they could contribute as much as 10.7 nmol L−1 of inorganic N in the highly N-depleted surface waters of this experiment. Inorganic N species are exceedingly low and often below the limit of detection (< ∼ 0.1 µmol L−1; see Methods for details), particularly in the surface samples for most of the experiment after the ODZ water addition. Thus, it is possible that the rapid degradation and recycling of N from IPLs could prove to constitute an additional supply of N under extremely limiting conditions. Given the diel variability in TAG production via eukaryotic phytoplankton and the potential impacts on energy and carbon fluxes (Becker et al., 2018), further analysis is needed to determine at what temporal scale IPLs may be recycled for TAG synthesis. While Becker et al. (2018) did not find evidence of diacylglycerol transferase (DGAT) activity related to diel cycles, additional investigations of other transferases or lipases relevant to the degradation of IPLs (e.g., PDAT or galactoglycerolipid lipase (PGD)) are critical.
IPLs may also be an important intermediate in the surface ocean sulfur cycle. Sulfur metabolites produced by phytoplankton (Durham et al., 2019) play central roles in microbial food webs by functioning in metabolic processes, contributing to membrane structure, supporting osmotic and redox balance, and acting as allelochemicals and signaling molecules (Moran and Durham, 2019). SQDGs are highly abundant IPLs, particularly in surface waters, and can constitute up to 60 nmol S L−1, potentially contributing to a significant proportion of the dissolved organic S in this region (ranging from ∼ 100–225 nmol L−1; Lennartz et al., 2019). The ability to catabolize SQDGs is widespread in heterotrophic bacteria and provides a highly ubiquitous source of sulfur (and carbon) substrates in the synthesis of other essential sulfur metabolites (Speciale et al., 2016). As such, SQDGs are considered major contributors to the global biosulfur cycle (Goddard-Borger and Williams, 2017), and their considerable production in phytoplankton membranes may be augmented by changes in temperature, light availability (i.e., shading effects), and pH (see discussion in Sect. 4.2.2).
Lipids are also sources of dissolved organic carbon (DOC) following hydrolysis by bacterial extracellular enzymes (Myklestad, 2000). Lipids have been observed as the most highly aged component of DOC and are considerably longer-lived than lipids found in suspended POC (Loh et al., 2004). However, much of the DOC pool remains uncharacterized (Nebbioso and Piccolo, 2013; Hansell and Carlson, 2014) and little is known regarding the specific processes forming refractory DOC fractions (Hansell, 2013). Yet, preliminary estimates of the total production of relatively refractory DOC (> 100-year lifetime) suggest its potential significance to global biogeochemistry (Legendre et al., 2015). Furthermore, it is still unclear how DOC cycling may be affected by changing ocean conditions (Wagner et al., 2020). Understanding the degradation pathways involved with abundant lipid pools and how they may contribute to the DOC pool in the surface ocean is of crucial importance to constraining the fluxes of refractory organic carbon pools.
We investigated the potential for phytoplankton to evoke lipid remodeling in response to multiple environmental stressors during a 2-month mesocosm experiment in the eastern tropical South Pacific Ocean off the coast of Peru. Our study is motivated by the need to understand how the expected changes in oceanographic conditions due to climate forcing in this area will impact the phytoplanktonic community driving primary productivity in the surface ocean, the chemistry of their cell membranes, and thus the chemical composition of particulate organic matter. A key aspect of our efforts was to statistically separate the relative roles of phytoplankton community composition and lipid remodeling in response to multiple environmental stressors in the composition of the environmental algal lipidome over time. Using a combination of multiple linear regressions, classification and regression trees, and random forest analyses, we report evidence of multiple environmental variables potentially acting as controls on IPL head groups, many of which are known to have specific functions in the chloroplast. The main takeaways of our study include the following:
-
IPLs are effective predictors of changes in the prevailing sources of phytoplankton biomass throughout the experiment.
-
The proportion of glycolipids (MGDG and SQDG) increases at higher temperatures, possibly to maintain thermal stability of the photosynthetic apparatus in response to thermal stress.
-
The relationship between light levels and the distribution of MGDGs and PGs possibly invokes photoprotective and antioxidant mechanisms provided by MGDGs, as well as affecting the role of PGs in electron transport processes in photosystems I and II.
-
Differences in the abundance of glycolipids between surface and subsurface waters appear to covary with oxygen availability. We expect this difference to be driven by the degradation of MGDGs and DGDGs under anaerobiosis or oxygen stress.
-
The reduction in N-bearing IPLs (i.e., BLs and PE) and the higher abundance of non-N-bearing IPLs (i.e., PG, SQDG, and MGDG) under more N-limited conditions suggest a possible IPL substitution mechanism to compensate for N limitation.
-
Whereas our study does not include the analysis of TAGs, our results are consistent with broader work suggesting that IPLs may be used as a substrate for the generation of acyl chains in TAG production in response to environmental stressors such as N limitation, variable pH or inorganic carbon availability, hypoxia, and varying light levels. However, such a mechanism in this mesocosm experiment remains to be tested in future work.
-
The cellular adaptations listed above likely contribute to the observed shifts in cellular content from structural or extrachloroplastic membrane lipids (i.e., PCs, PEs, BLs, and certain PGs) under high-growth conditions to thylakoid/plastid membrane lipids (i.e., MGDGs, DGDGs, SQDGs, and certain PGs) while exposed to environmental stressors.
We hypothesize that these remodeling processes may involve major shifts in the elemental stoichiometry of the cell and thus alter the fluxes of C, N, and S to higher trophic levels, signaling potential impacts on the broader cycling of these biorelevant elements under different scenarios of future environmental change. Therefore, we suggest that future studies addressing the biogeochemical consequences of climate change in the eastern tropical South Pacific Ocean must include the role of lipid remodeling in phytoplankton.
The environmental study data are available at https://doi.org/10.1594/PANGAEA.923395 (Bach et al., 2020b). Biomarker metadata that generate and support the findings of this study and R code are available at https://github.com/Guachan/IPLs-KOSMOS/tree/0.1.0 (last access: 20 December 2023) (https://doi.org/10.5281/zenodo.10408453, Guachan, 2023; Cantarero, 2023).
The supplement related to this article is available online at: https://doi.org/10.5194/bg-21-3927-2024-supplement.
JS and SIC designed the study. JS funded the study. JS and CAV funded sample collection during the mesocosm experiment. UR and LTB designed, funded, organized, and carried out the mesocosm experiment. PA, JS, LTB, and UR carried out sample collection. SIC led laboratory and analytical work with the assistance of ND and under the supervision of JS. SEI carried out data analysis. SIC, JET, BCS, and BR performed statistical analyzes. SIC wrote the manuscript with major editorial contributions from EF, HA, and JS and with comments from all co-authors.
At least one of the (co-)authors is a member of the editorial board of Biogeosciences. The peer-review process was guided by an independent editor, and the authors also have no other competing interests to declare.
Publisher’s note: Copernicus Publications remains neutral with regard to jurisdictional claims made in the text, published maps, institutional affiliations, or any other geographical representation in this paper. While Copernicus Publications makes every effort to include appropriate place names, the final responsibility lies with the authors.
This article is part of the special issue “Ecological and biogeochemical functioning of the coastal upwelling system off Peru: an in situ mesocosm study”. It is not associated with a conference.
We extend our gratitude to all participants in the KOSMOS Peru 2017 study for their invaluable assistance in mesocosm sampling and maintenance. Our special thanks go to the dedicated staff from GEOMAR Kiel and IMARPE for their support throughout the planning, preparation, and execution of this study. We are particularly grateful to Andrea Ludwig, Michelle Graco, Dimitri Gutierrez, Allanah Paul, Susanne Feiersinger, Kai Schulz, Jean-Pierre Bednar, Peter Fritsche, Paul Stange, Anna Schukat, and Michael Krudewig. We also express our appreciation to the captains and crews of BAP Morales, IMARPE VI, and BIC Humboldt for their support during the deployment and recovery of the mesocosms. We extend special thanks to the Marina de Guerra del Perú, specifically the submarine section of the navy in Callao, the Dirección General de Capitanías y Guardacostas, and the Club Náutico Del Centro Naval. KOSMOS Peru 2017 took place within the framework of the cooperation agreement between IMARPE and GEOMAR through the German Federal Ministry of Education and Research (BMBF) project ASLAEL 12-016 and the national project Integrated Study of the Upwelling System off Peru developed by the Directorate of Oceanography and Climate Change of IMARPE, PPR 137 CONCYTEC. We acknowledge all members of the Organic Geochemistry Laboratory in addition to Kaitlin Rempfert, Sebastian Kopf, Tom Marchitto, and Nicole Lovenduski at the University of Colorado Boulder and Matthew Long at NCAR for fruitful discussions. Publication of this article was funded by the University of Colorado Boulder Libraries Open Access Fund.
This study was funded by the US National Science Foundation CAREER Award 2047057 “MIcrobial Lipidomics in Changing Oceans” (MILCO) to Julio Sepúlveda; Sebastian Cantarero and Julio Sepúlveda received additional support from the Department of Geological Sciences and the Center for the Study of Origins at the University of Colorado Boulder. The Millennium Institute of Oceanography (IMO) ICN12_019 through the Agencia Nacional de Investigación y Desarrollo (ANID) – Millennium Science Initiative program – provided funding for sample collection. KOSMOS Peru 2017 was funded by the Collaborative Research Centre SFB 754 Climate-Biogeochemistry Interactions in the Tropical Ocean, under the German Research Foundation (DFG) and with the funding granted to Ulf Riebesell. Additional funding was provided by the EU project AQUACOSM through the European Union's Horizon 2020 research and innovation program under grant agreement no. 731065 and through the Leibniz Award 2012, granted to Ulf Riebesell.
This paper was edited by Hans-Peter Grossart and reviewed by three anonymous referees.
Abida, H., Dolch, L. J., Meï, C., Villanova, V., Conte, M., Block, M.A., Finazzi, G., Bastien, O., Tirichine, L., Bowler, C., Rébeillé, F., Petroutsos, D., Jouhet, J., and Maréchal, E.: Membrane glycerolipid remodeling triggered by nitrogen and phosphorus starvation in Phaeodactylum tricornutum, Plant Physiol., 167, 118–136, https://doi.org/10.1104/pp.114.252395, 2015.
Abrahams, A., Schlegel, R. W., and Smit, A. J.: Variation and change of upwelling dynamics detected in the world's eastern boundary upwelling systems, Front. Mar. Sci., 8, 626411, https://doi.org/10.3389/fmars.2021.626411, 2021.
Arístegui, J. and Harrison, W. G.: Decoupling of primary production and community respiration in the ocean: Implications for regional carbon studies, Aquat. Microb. Ecol., 29, 199–209, https://doi.org/10.3354/ame029199, 2002.
Azov, Y.: Effect of pH on inorganic carbon uptake in algal cultures, Appl. Environ. Microbiol., 43, 1300–1306, https://doi.org/10.1128/aem.43.6.1300-1306.1982, 1982.
Bach, L. T., Alvarez-Fernandez, S., Hornick, T., Stuhr, A., and Riebesell, U.: Simulated ocean acidification reveals winners and losers in coastal phytoplankton, PLoS One, 12, 1–22, https://doi.org/10.1371/journal.pone.0188198, 2017.
Bach, L. T., Paul, A. J., Boxhammer, T., von der Esch, E., Graco, M., Schulz, K. G., Achterberg, E., Aguayo, P., Arístegui, J., Ayón, P., Baños, I., Bernales, A., Boegeholz, A. S., Chavez, F., Chavez, G., Chen, S. M., Doering, K., Filella, A., Fischer, M., Grasse, P., Haunost, M., Hennke, J., Hernández-Hernández, N., Hopwood, M., Igarza, M., Kalter, V., Kittu, L., Kohnert, P., Ledesma, J., Lieberum, C., Lischka, S., Löscher, C., Ludwig, A., Mendoza, U., Meyer, J., Meyer, J., Minutolo, F., Cortes, J. O., Piiparinen, J., Sforna, C., Spilling, K., Sanchez, S., Spisla, C., Sswat, M., Moreira, M. Z., and Riebesell, U.: Factors controlling plankton community production, export flux, and particulate matter stoichiometry in the coastal upwelling system off Peru, Biogeosciences, 17, 4831–4852, https://doi.org/10.5194/bg-17-4831-2020, 2020a.
Bach, L. T., Paul, A. J., Boxhammer, T., von der Esch, E., Graco, M., Schulz, K. G., Achterberg, E., Aguayo, P., Arístegui, J., Ayón, P., Baños, I., Bernales, A., Boegeholz, A. S., Chavez, F., Chavez, G., Chen, S. M., Doering, K., Filella, A., Fischer, M., Grasse, P., Haunost, M., Hennke, J., Hernández-Hernández, N., Hopwood, M., Igarza, M., Kalter, V., Kittu, L., Kohnert, P., Ledesma, J., Lieberum, C., Lischka, S., Löscher, C., Ludwig, A., Mendoza, U., Meyer, J., Meyer, J., Minutolo, F., Cortes, J. O., Piiparinen, J., Sforna, C., Spilling, K., Sanchez, S., Spisla, C., Sswat, M., Moreira, M. Z., and Riebesell, U.: KOSMOS 2017 Peru mesocosm study: overview data, PANGAEA [data set], https://doi.org/10.1594/PANGAEA.923395, 2020b.
Bakun, A., Field, D. B., Redondo-Rodriguez, A., and Weeks, S. J.: Greenhouse gas, upwelling-favorable winds, and the future of coastal ocean upwelling ecosystems, Glob. Change Biol., 16, 1213–1228, https://doi.org/10.1111/j.1365-2486.2009.02094.x, 2010.
Barlow, R. G., Cummings, D. G., and Gibb, S. W.: Improved resolution of mono- and divinyl chlorophylls a and b and zeaxanthin and lutein in phytoplankton extracts using reverse phase C-8 HPLC, Mar. Ecol. Prog. Ser., 161, 303–307, 1997.
Becker, K. W., Collins, J. R., Durham, B. P., Groussman, R. D., White, A. E., Fredricks, H. F., Ossolinski, J. E., Repeta, D. J., Carini, P., Armbrust, E. V., and Van Mooy, B. A.: Daily changes in phytoplankton lipidomes reveal mechanisms of energy storage in the open ocean, Nat. Commun., 9, 5179, https://doi.org/10.1038/s41467-018-07346-z, 2018.
Behrenfeld, M. J., O'Malley, R. T., Siegel, D. A., McClain, C. R., Sarmiento, J. L., Feldman, G. C., Milligan, A. J., Falkowski, P. G., Letelier, R. M., and Boss, E. S.: Climate-driven trends in contemporary ocean productivity, Nature, 444, 752–755, https://doi.org/10.1038/nature05317, 2006.
Benjamini, Y. and Yosef Hochberg, Y.: Controlling the False Discovery Rate: A Practical and Powerful Approach to Multiple Testing, J. Roy. Stat. Soc. Ser. B, 57, 289–300, https://doi.org/10.1111/j.2517-6161.1995.tb02031.x, 1995.
Berry, J. and Bjorkman, O.: Photosynthetic Response and Adaptation to Temperature in Higher Plants, Annu. Rev. Plant Physiol., 31, 491–543, https://doi.org/10.1146/annurev.pp.31.060180.002423, 1980.
Brandsma, J.: The origin and fate of intact polar lipids in the marine environment, PhD thesis, Dept. of Marine Organic Biogeochemistry, Royal Netherlands Institute for Sea Research (NIOZ), 224 pp., ISBN: 978-90-6266-286-9, 2011.
Bligh, E. G. and Dyer, W. J.: A rapid method of total lipid extraction and purification, Can. J. Biochem. Pphysiol., 37, 911–917, https://doi.org/10.1139/o59-099, 1959.
Biau, G. and Scornet, E.: A random forest guided tour, Test, 25, 197–227, https://doi.org/10.1007/s11749-016-0481-7, 2016.
Boulesteix, A. L., Janitza, S., Kruppa, J., and König, I. R.: Overview of random forest methodology and practical guidance with emphasis on computational biology and bioinformatics, WIRES Data Min. Knowl., 2, 493–507, https://doi.org/10.1002/widm.1072, 2012.
Breiman, L., Friedman, J. H., Olshen, R. A., and Stone, C. J.: Classification And Regression Trees, 1st Edn., Chapman and Hall/CRC, https;//doi.org/10.1201/9781315139470, 1984.
Breiman, L.: Random Forests, Mach. Learn., 45, 5–32, https://doi.org/10.1023/A:1010933404324, 2001.
Browning, T. J., Rapp, I., Schlosser, C., Gledhill, M., Achterberg, E. P., Bracher, A., and Le Moigne, F. A. C.: Influence of Iron, Cobalt, and Vitamin B12 Supply on Phytoplankton Growth in the Tropical East Pacific During the 2015 El Niño, Geophys. Res. Lett., 45, 6150–6159, https://doi.org/10.1029/2018GL077972, 2018.
Bronk, D. A., See, J. H., Bradley, P., and Killberg, L.: DON as a source of bioavailable nitrogen for phytoplankton, Biogeosciences, 4, 283–296, https://doi.org/10.5194/bg-4-283-2007, 2007.
Burian, A., Nielsen, J. M., Hansen, T., Bermudez, R., and Winder, M.: The potential of fatty acid isotopes to trace trophic transfer in aquatic food-webs, Philos. T. R. Soc. B, 375, 1804, https://doi.org/10.1098/rstb.2019.0652, 2020.
Cantarero, S., Henríquez-Castillo, C., Dildar, N., Vargas, C., von Dassow, P., Cornejo-D'Ottone, M., and Sepúlvada, J.: Size-fractionated contribution of microbial biomass to suspended organic matter in the Eastern Tropical South Pacific oxygen minimum zone, Front. Mar. Sci., 7, 540643, https://doi.org/10.3389/fmars.2020.540643, 2020.
Capone, D. G. and Hutchins, D. A.: Microbial biogeochemistry of coastal upwelling regimes in a changing ocean, Nat. Geosci., 6, 711–717, https://doi.org/10.1038/ngeo1916, 2013.
Carter, B. R., Radich, J. A., Doyle, H. L., and Dickson, A. G.: An automated system for spectrophotometric seawater pH measurements, Limnol. Oceanogr. Method., 11, 16–27, https://doi.org/10.4319/lom.2013.11.16, 2013.
Chavez, F. P. and Messié, M.: A comparison of Eastern Boundary Upwelling Ecosystems, Prog. Oceanogr., 83, 80–96, https://doi.org/10.1016/j.pocean.2009.07.032, 2009.
Chen, S., Riebesell, U., Schulz, K. G., Esch, E. Von Der, Achterberg, E. P. and Bach, L. T.: Temporal dynamics of surface ocean carbonate chemistry in response to natural and simulated upwelling events during the 2017 coastal El Niño near Callao, Peru, Biogeosciences, 19, 295–312, https://doi.org/10.5194/bg-19-295-2022, 2022.
Chen, X. and Ishwaran, H.: Random forests for genomic data analysis, Genomics, 99, 323–329, https://doi.org/10.1016/j.ygeno.2012.04.003, 2012.
Coverly, S., Kérouel, R., and Aminot, A.: A re-examination of matrix effects in the segmented-flow analysis of nutrients in sea and estuarine water, Anal. Chim. Acta, 712, 94–100, https://doi.org/10.1016/j.aca.2011.11.008, 2012.
Dahlqvist, A., Ståhl, U., Lenman, M., Banas, A., Lee, M., Sandager, L., Ronne, H., and Stymne, S.: Phospholipid:diacylglycerol acyltransferase: An enzyme that catalyzes the acyl-CoA-independent formation of triacylglycerol in yeast and plants, P. Natl. Acad. Sci. USA, 97, 6487–6492, https://doi.org/10.1073/pnas.120067297, 2000.
Ding, H. and Sun, M. Y.: Biochemical degradation of algal fatty acids in oxic and anoxic sediment-seawater interface systems: Effects of structural association and relative roles of aerobic and anaerobic bacteria, Mar. Chem., 93, 1–19, https://doi.org/10.1016/j.marchem.2004.04.004, 2005.
DiTullio, G. R., Geesey, M. E., Mancher, J. M., Alm, M. B., Riseman, S. F., and Bruland, K. W.: Influence of iron on algal community composition and physiological status in the Peru upwelling system, Limnol. Oceanogr., 50, 1887–1907, https://doi.org/10.4319/lo.2005.50.6.1887, 2005.
Durham, B. P., Boysen, A. K., Carlson, L. T., Groussman, R. D., Heal, K. R., Cain, K. R., Morales, R. L., Coesel, S. N., Morris, R. M., Ingalls, A. E., and Armbrust, E. V.: Sulfonate-based networks between eukaryotic phytoplankton and heterotrophic bacteria in the surface ocean, Nat. Microbiol., 4, 1706–1715, https://doi.org/10.1038/s41564-019-0507-5, 2019.
Dutkiewicz, S., Ward, B. A., Monteiro, F., and Follows, M. J.: Interconnection of nitrogen fixers and iron in the Pacific Ocean: Theory and numerical simulations, Global Biogeochem. Cy., 26, 1–16, https://doi.org/10.1029/2011GB004039, 2012.
Dutkiewicz, S., Morris, J. J., Follows, M. J., Scott, J., Levitan, O., Dyhrman, S. T., and Berman-Frank, I.: Impact of ocean acidification on the structure of future phytoplankton communities, Nat. Clim. Change, 5, 1002–1006, https://doi.org/10.1038/nclimate2722, 2015.
Elsayed, K. N. M., Kolesnikova, T. A., Noke, A., and Klöck, G.: Imaging the accumulated intracellular microalgal lipids as a response to temperature stress, 3 Biotech., 7, 41, https://doi.org/10.1007/s13205-017-0677-x, 2017.
Fábregas, J., Maseda, A., Domínguez, A., and Otero, A.: The cell composition of Nannochloropsis sp. changes under different irradiances in semicontinuous culture, World J. Microbiol. Biotechnol., 20, 31–35, https://doi.org/10.1023/B:WIBI.0000013288.67536.ed, 2004.
Fakhry, E. M. and El Maghraby, D. M.: Lipid accumulation in response to nitrogen limitation and variation of temperature in nannochloropsis salina, Bot. Stud., 56, 6, https://doi.org/10.1186/s40529-015-0085-7, 2015.
Flores, E., Cantarero, S. I., Ruiz-Fernández, P., Dildar, N., Zabel, M., Ulloa, O., and Sepúlveda, J.: Bacterial and eukaryotic intact polar lipids point to in situ production as a key source of labile organic matter in hadal surface sediment of the Atacama Trench, Biogeosciences, 19, 1395–1420, https://doi.org/10.5194/bg-19-1395-2022, 2022.
Fuenzalida, R., Schneider, W., Garcés-Vargas, J., Bravo, L., and Lange, C.: Vertical and horizontal extension of the oxygen minimum zone in the eastern South Pacific Ocean, Deep-Sea Res. Pt. II, 56, 992–1003, https://doi.org/10.1016/j.dsr2.2008.11.001, 2009.
Gabruk, M., Mysliwa-Kurdziel, B., and Kruk, J.: MGDG, PG and SQDG regulate the activity of light-dependent protochlorophyllide oxidoreductase, Biochem. J., 474, 1307–1320, https://doi.org/10.1042/BCJ20170047, 2017.
Gardner, R., Peters, P., Peyton, B., and Cooksey, K. E.: Medium pH and nitrate concentration effects on accumulation of triacylglycerol in two members of the chlorophyta, J. Appl. Phycol., 23, 1005–1016, https://doi.org/10.1007/s10811-010-9633-4, 2011.
Gardner, R. D., Cooksey, K. E., Mus, F., Macur, R., Moll, K., Eustance, E., Carlson, R. P., Gerlach, R., Fields, M. W., and Peyton, B. M.: Use of sodium bicarbonate to stimulate triacylglycerol accumulation in the chlorophyte Scenedesmus sp. and the diatom Phaeodactylum tricornutum, J. Appl. Phycol., 24, 1311–1320, https://doi.org/10.1007/s10811-011-9782-0, 2012.
Gašparović, B., Godrijan, J., Frka, S., Tomažić, I., Penezić, A., Marić, D., Djakovac, T., Ivančić, I., Paliaga, P., Lyons, D., Precali, R., and Tepić, N.: Adaptation of marine plankton to environmental stress by glycolipid accumulation, Mar. Environ. Res., 92, 120–132, https://doi.org/10.1016/j.marenvres.2013.09.009, 2013.
Geider, J. R. and Osborne, B. A.: Respiration and microalgal growth: a review of the quantitative relationship between dark respiration and growth, New Phytol., 112, 327–341, https://doi.org/10.1111/j.1469-8137.1989.tb00321.x, 1989.
Gilly, W. F., Beman, J. M., Litvin, S. Y., and Robison, B. H.: Oceanographic and Biological Effects of Shoaling of the Oxygen Minimum Zone, Ann. Rev. Mar. Sci., 5, 393–420, https://doi.org/10.1146/annurev-marine-120710-100849, 2013.
Goddard-Borger, E. D. and Williams, S. J.: Sulfoquinovose in the biosphere: occurrence, metabolism and functions, Biochem. J., 1, 474, 827–849, https://doi.org/10.1042/BCJ20160508, 2017.
Gombos, Z. and Murata, N.: Lipids and fatty acids of prochlorothrix hollandica, Plant Cell Physiol., 32, 73–77, https://doi.org/10.1093/oxfordjournals.pcp.a078054, 1991.
Gonçalves, A. L., Pires, J. C., and Simões, M.: Lipid production of Chlorella vulgaris and Pseudokirchneriella subcapitata, Int. J. Energ. Environ. Eng., 4, 14, https://doi.org/10.1186/2251-6832-4-14, 2013.
Goss, R., Nerlich, J., Lepetit, B., Schaller, S., Vieler, A., and Wilhelm, C.: The lipid dependence of diadinoxanthin de-epoxidation presents new evidence for a macrodomain organization of the diatom thylakoid membrane, J. Plant Physiol., 166, 1839–1854, https://doi.org/10.1016/j.jplph.2009.05.017, 2009.
Gu, X., Cao, L., Wu, X., Li, Y., Hu, Q., and Han, D.: A lipid bodies-associated galactosyl hydrolase is involved in triacylglycerol biosynthesis and galactolipid turnover in the unicellular green alga chlamydomonas reinhardtii, Plants, 10, 675, https://doi.org/10.3390/plants10040675, 2021.
Guachan: Guachan/IPLs-KOSMOS: Initial Release, Zenodo [data set], https://doi.org/10.5281/zenodo.10408453, 2023.
Guckert, J. B. and Cooksey, K. E.: Triglyceride accumulation and fatty acid profile changes in Chlorella (Chlorophyta) during high Ph-induced cell cycle Inhibition, J. Phycol., 26, 72–79, https://doi.org/10.1111/j.0022-3646.1990.00072.x, 1990.
Guschina, I. A. and Harwood, J. L.: Algal lipids and their metabolism, in: Algae for Biofuels and Energy, edited by: Borowitzka, M. and Moheimani, N., Springer, Dordrecht, 17–36, https://doi.org/10.1007/978-94-007-5479-2_2, 2013.
Gutiérrez, D., Bouloubassi, I., Sifeddine, A., Purca, S., Goubanova, K., Graco, M., Field, D., Méjanelle, L., Velazco, F., Lorre, A., Salvatteci, R., Quispe, D., Vargas, G., Dewitte, B., and Ortlieb, L.: Coastal cooling and increased productivity in the main upwelling zone off Peru since the mid-twentieth century, Geophys. Res. Lett., 38, 1–6, https://doi.org/10.1029/2010GL046324, 2011.
Hallegraeff, G. M.: Ocean climate change, phytoplankton community responses, and harmful algal blooms: a formidable predictive challenge,J. Phycol., 46, 220–235, https://doi.org/10.1111/j.1529-8817.2010.00815.x, 2010.
Hansell, D. A.: Recalcitrant dissolved organic carbon fractions, Ann. Rev. Mar. Sci., 5, 421–445, https://doi.org/10.1146/annurev-marine-120710-100757, 2013.
Hansell, D. A. and Carlson, C. A.: Biogeochemistry of marine dissolved organic matter, Academic Press, https://doi.org/10.1016/C2012-0-02714-7, 2014.
Harwood, J. L. and Jones, A. L.: Lipid Metabolism in Algae, Adv. Bot. Res., 16, 1–53, 1989.
Hastie, T., Tibshirani, R., and Friedman, J.: Springer Series in Statistics, Elem. Stat. Learn., 27, 83–85, https://doi.org/10.1007/b94608, 2009.
Hauss, H., Franz, J., and Sommer, U.: Changes in N:P stoichiometry influence taxonomic composition and nutritional quality of phytoplankton in the Peruvian upwelling, J. Sea Res., 73, 74–85, https://doi.org/10.1016/j.seares.2012.06.010, 2012.
Hemschemeier, A., Casero, D., Liu, B., Benning, C., Pellegrini, M., Happe, T., and Merchant, S. S.: COPPER RESPONSE REGULATOR1-dependent and -independent responses of the Chlamydomonas reinhardtii transcriptome to dark anoxia, Plant Cell, 25, 3186–3211, https://doi.org/10.1105/tpc.113.115741, 2013.
Henson, S. A., Cael, B. B., Allen, S. R., and Dutkiewicz, S.: Future phytoplankton diversity in a changing climate, Nat. Commun., 12, 1–8, https://doi.org/10.1038/s41467-021-25699-w, 2021.
Holm, H. C., Fredricks, H. F., Bent, S. M., Lowenstein, D. P., Ossolinski, J. E., Becker, K. W., Johnson, W. M., Schrage, K., and Van Mooy, B. A. S.: Global Ocean lipidomes show a universal relationship between temperature and lipid unsaturation, Science, 376, 1487–1491, https://doi.org/10.1126/science.abn7455, 2022.
Huertas E., I., Rouco, M., López-Rodas, V., and Costas, E.: Warming will affect phytoplankton differently: Evidence through a mechanistic approach, Proc. R. Soc. B, 278, 3534–3543, https://doi.org/10.1098/rspb.2011.0160, 2011.
Hutchins, D. A., Hare, C. E., Weaver, R. S., Zhang, Y., Firme, G. F., DiTullio, G. R., Alm, M. B., Riseman, S. F., Maucher, J. M., Geesey, M. E., Trick, C. G., Smith, G. J., Rue, E. L., Conn, J., and Bruland, K. W.: Phytoplankton iron limitation in the Humboldt Current and Peru Upwelling, Limnol. Oceanogr., 47, 997–1011, https://doi.org/10.4319/lo.2002.47.4.0997, 2002.
Hutchins, D. A. and Fu, F.: Microorganisms and ocean global change, Nat. Microbiol., 2, 17058, https://doi.org/10.1038/nmicrobiol.2017.58, 2017.
Irwin, A. J., Finkel, Z. V., Müller-Karger, F. E., and Ghinaglia, L. T.: Phytoplankton adapt to changing ocean environments, P. Natl. Acad. Sci. USA, 112, 5762–5766, https://doi.org/10.1073/pnas.1414752112, 2015.
Jiang, L., Luo, S., Fan, X., Yang, Z., and Guo, R.: Biomass and lipid production of marine microalgae using municipal wastewater and high concentration of CO2, Appl. Energy, 88, 3336–3341, https://doi.org/10.1016/j.apenergy.2011.03.043, 2011.
Jiang, L. Q., Carter, B. R., Feely, R. A., Lauvset, S. K., and Olsen, A.: Surface ocean pH and buffer capacity: past, present and future, Sci. Rep., 9, 1–11, https://doi.org/10.1038/s41598-019-55039-4, 2019.
Jin, P., Liang, Z., Lu, H., Pan, J., Li, P., Huang, Q., Guo Y,, Zhong, J., Li, F., Wan, J., Overmans, S., and Xia, J.: Lipid remodeling reveals the adaptations of a marine diatom to ocean acidification, Front. Microbiol., 12, 748445, https://doi.org/10.3389/fmicb.2021.748445, 2021.
Jónasdóttir, S. H.: Fatty acid profiles and production in marine phytoplankton, Mar. Drugs, 17, 151, https://doi.org/10.3390/md17030151, 2019.
Kato, C., Masui, N., and Horikoshi, K.: Properties of obligately barophilic bacteria isolated from a sample of deep-sea sediment from the Izu-Bonin trench, J. Mar. Biotechnol., 4, 96–99, 1996.
Keeling, R. F., Körtzinger, A., and Gruber, N.: Ocean deoxygenation in a warming world, Ann. Rev. Mar. Sci., 2, 199–229, https://doi.org/10.1146/annurev.marine.010908.163855, 2010.
Kérouel, R. and Aminot, A.: Fluorometric determination of ammonia in sea and estuarine waters by direct segmented flow analysis, Mar. Chem., 57, 265–275, https://doi.org/10.1016/S0304-4203(97)00040-6, 1997.
Khoeyi, Z. A., Seyfabadi, J., and Ramezanpour, Z.: Effect of light intensity and photoperiod on biomass and fatty acid composition of the microalgae, Chlorella vulgaris, Aquac. Int., 20, 41–49, https://doi.org/10.1007/s10499-011-9440-1, 2012.
Khotimchenko, S. V. and Yakovleva, I. M.: Lipid composition of the red alga Tichocarpus crinitus exposed to different levels of photon irradiance, Phytochemistry, 66, 73–79, https://doi.org/10.1016/j.phytochem.2004.10.024, 2005.
Kobayashi, K., Endo, K., and Wada, H.: Specific distribution of phosphatidylglycerol to photosystem complexes in the thylakoid membrane, Front. Plant Sci., 8, 1–7, https://doi.org/10.3389/fpls.2017.01991, 2017.
Kong, F., Romero, I. T., Warakanont, J., and Li-Beisson, Y.: Lipid catabolism in microalgae, New Phytol., 218, 1340–1348, https://doi.org/10.1111/nph.15047, 2018.
Kudela, R. M., Seeyave, S., and Cochlan, W. P.: The role of nutrients in regulation and promotion of harmful algal blooms in upwelling systems, Prog. Oceanogr., 85, 122–135, https://doi.org/10.1016/j.pocean.2010.02.008, 2010.
Kumari, P., Bijo, A. J., Mantri, V. A., Reddy, C. R. K., and Jha, B.: Fatty acid profiling of tropical marine macroalgae: An analysis from chemotaxonomic and nutritional perspectives, Phytochemistry, 86, 44–56, https://doi.org/10.1016/j.phytochem.2012.10.015, 2013.
Lam, P., Kuypers, M. M. M., Lavik, G., Jensen, M. M., Vossenberg, J. Van De, Schmid, M., Woebken, D., Amann, R., Jetten, M. S. M., and Kuypers, M. M. M.: Revising the nitrogen cycle in the Peruvian oxygen minimum zone Phyllis, P. Natl. Acad. Sci. USA, 106, 2192–2205, https://doi.org/10.1038/nature0415, 2009.
Legendre, L., Rivkin, R. B., Weinbauer, M. G., Guidi, L., and Uitz, J.: The microbial carbon pump concept: Potential biogeochemical significance in the globally changing ocean, Prog. Oceanogr., 134, 432–450, https://doi.org/10.1016/j.pocean.2015.01.008, 2015.
Lennartz, S. T., von Hobe, M., Booge, D., Bittig, H. C., Fischer, T., Gonçalves-Araujo, R., Ksionzek, K. B., Koch, B. P., Bracher, A., Röttgers, R., Quack, B., and Marandino, C. A.: The influence of dissolved organic matter on the marine production of carbonyl sulfide (OCS) and carbon disulfide (CS2) in the Peruvian upwelling, Ocean Sci., 15, 1071–1090, https://doi.org/10.5194/os-15-1071-2019, 2019.
Li, X., Benning, C., and Kuo, M. H.: Rapid triacylglycerol turnover in Chlamydomonas reinhardtii requires a lipase with broad substrate specificity, Eukaryot. Cell, 11, 1451–1462, https://doi.org/10.1128/EC.00268-12, 2012.
Li-Beisson, Y., Thelen, J. J., Fedosejevs, E., and Harwood, J. L.: The lipid biochemistry of eukaryotic algae, Prog. Lipid Res., 74, 31–68, https://doi.org/10.1016/j.plipres2019.01.003, 2019.
Lipp, J. S., Morono, Y., Inagaki, F., and Hinrichs, K.-U.: Significant contribution of Archaea to extant biomass in marine subsurface sediments, Nature, 454, 991–994, https://doi.org/10.1038/nature07174, 2008.
Liu, J., Yuan, C., Hu, G., and Li, F.: Effects of light intensity on the growth and lipid accumulation of microalga Scenedesmus sp. 11-1 under nitrogen limitation, Appl. Biochem. Biotechnol., 166, 2127–2137, https://doi.org/10.1007/s12010-012-9639-2, 2012.
Liu, B.: Biochemical Characterization of Triacylglycerol Metabolism in Microalgae, PhD thesis, Biochemistry and Molecular Biology, Michigan State University, USA, 165 pp., ISBN: 97813037151741303715171, 2014.
Loh, A. N., Bauer, J. E., and Druffel, E. R. M.: Variable ageing and storage of dissolved organic components in the open ocean, Nature, 430, 877–881, https://doi.org/10.1038/nature02780, 2004.
Luan, J., Zhang, C., Xu, B., Xue, Y., and Ren, Y.: The predictive performances of random forest models with limited sample size and different species traits, Fish. Res., 227, 105534, https://doi.org/10.1016/j.fishres.2020.105534, 2020.
Lyon, B. R. and Mock, T.: Polar microalgae: New approaches towards understanding adaptations to an extreme and changing environment, Biology, 3, 56–80, https://doi.org/10.3390/biology3010056, 2014.
Mackey, M. D., Mackey, D. J., Higgins, H. W., and Wright, S. W.: CHEMTAX – A program for estimating class abundances from chemical markers: Application to HPLC measurements of phytoplankton, Mar. Ecol. Prog. Ser., 144, 265–283, https://doi.org/10.3354/meps144265, 1996.
Masuda, S., Kobayashi, M., Icochea Salas, L. A., and Rosales Quintana, G. M.: Possible link between temperatures in the seashore and open ocean waters of Peru identified by using new seashore water data, Progr. Earth Pt. Sci., 10, 38, https://doi.org/10.1186/s40645-023-00571-1, 2023.
Meador, T. B., Goldenstein, N. I., Gogou, A., Herut, B., Psarra, S., Tsagaraki, T. M., and Hinrichs, K. U.: Planktonic lipidome responses to Aeolian dust input in low-biomass oligotrophic marine mesocosms, Front. Mar. Sci., 4, 1–20, https://doi.org/10.3389/fmars.2017.00113, 2017.
Messié, M., Ledesma, J., Kolber, D. D., Michisaki, R. P., Foley, D. G., and Chavez, F. P.: Potential new production estimates in four eastern boundary upwelling ecosystems, Prog. Oceanogr., 83, 151–158, https://doi.org/10.1016/j.pocean.2009.07.018, 2009.
Messié, M. and Chavez, F. P.: Seasonal regulation of primary production in eastern boundary upwelling systems, Prog. Oceanogr., 134, 1–18, https://doi.org/10.1016/j.pocean.2014.10.011, 2015.
Meyer, J., Löscher, C. R., Lavik, G., and Riebesell, U.: Mechanisms of P* reduction in the eastern tropical South Pacific, Front. Mar. Sci., 4, 1–12, https://doi.org/10.3389/fmars.2017.00001, 2017.
Meyers, M. T., Cochlan, W. P., Carpenter, E. J., and Kimmerer, W. J.: Effect of ocean acidification on the nutritional quality of marine phytoplankton for copepod reproduction, PLoS One, 14, 1–22, https://doi.org/10.1371/journal.pone.0217047, 2019.
Mimouni, V., Couzinet-Mossion, A., Ulmann, L., and Wielgosz-Collin, G.: Lipids from microalgae, in: Microalgae in health and disease prevention, edited by: Levine, I. A. and Fleurence, J., 109–131, Academic Press, https://doi.org/10.1016/B978-0-12-811405-6.00005-0, 2018.
Moazami-Goudarzi, M. and Colman, B.: Changes in carbon uptake mechanisms in two green marine algae by reduced seawater pH, J. Exp. Mar. Bio. Ecol., 413, 94–99, https://doi.org/10.1016/j.jembe.2011.11.017, 2012.
Morales, M., Aflalo, C., and Bernard, O.: Microalgal lipids: A review of lipids potential and quantification for 95 phytoplankton species, Biomass Bioenerg., 150, 106108, https://doi.org/10.1016/j.biombioe.2021.106108, 2021.
Morán, X. A. G., López-Urrutia, Á., Calvo-Díaz, A., and LI, W. K. W.: Increasing importance of small phytoplankton in a warmer ocean, Glob. Change Biol., 16, 1137–1144, https://doi.org/10.1111/j.1365-2486.2009.01960.x, 2010.
Moran, M. A. and Durham, B. P.: Sulfur metabolites in the pelagic ocean, Nat. Rev. Microbiol., 17, 665–678, https://doi.org/10.1038/s41579-019-0250-1, 2019.
Morris, A. W. and Riley, J. P.: The determination of nitrate in sea water, Anal. Chim. Acta, 29, 272–279, https://doi.org/10.1016/S0003-2670(00)88614-6, 1963.
Mullin, J. B. and Riley, J. P.: The colorimetric determination of silicate with special reference to sea and natural waters, Anal. Chim. Acta, 12, 162–176, https://doi.org/10.1016/S0003-2670(00)87825-3, 1955.
Murphy, J. and Riley, J. P.: A modified single solution method for the determination of phosphate in natural waters, Anal. Chim. Acta, 27, 31–36, https://doi.org/10.1016/S0003-2670(00)88444-5, 1962.
Myklestad, S. M.: Dissolved Organic Carbon from Phytoplankton, in: Marine Chemistry, edited by: Wangersky, P. J., The Handbook of Environmental Chemistry, Springer, Berlin, Heidelberg, 111–148, https://doi.org/10.1007/10683826_5, 2000.
Nakajima, Y., Umena, Y., Nagao, R., Endo, K., Kobayashi, K., Akita, F., Suga, M., Wada, H., Noguchi, T., and Shen, J. R., Thylakoid membrane lipid sulfoquinovosyl-diacylglycerol (SQDG) is required for full functioning photosystem II in Thermosynechococccus elongatus, J. Biol. Chem., 293, 14786–14797, https://doi.org/10.1074/jbc.RA118.004304d, 2018.
Naqvi, S. W. A., Bange, H. W., Farías, L., Monteiro, P. M. S., Scranton, M. I., and Zhang, J.: Marine hypoxia/anoxia as a source of CH4 and N2O, Biogeosciences, 7, 2159–2190, https://doi.org/10.5194/bg-7-2159-2010, 2010.
Nebbioso, A. and Piccolo, A.: Molecular characterization of dissolved organic matter (DOM): A critical review, Anal. Bioanal. Chem., 405, 109–124, https://doi.org/10.1007/s00216-012-6363-2, 2013.
Neidleman, S. L.: Effects of temperature on lipid unsaturation, Biotechnol. Genet. Eng. Rev., 5, 245–268, https://doi.org/10.1080/02648725.1987.10647839, 1987.
Orcutt, D. M. and Patterson, G. W.: Effect of light intensity upon lipid composition of Nitzschia closterium (Cylindrotheca fusiformis), Lipids, 9, 1000–1003, https://doi.org/10.1007/BF02533825, 1974.
Oyarzún, D. and Brierley, C. M.: The future of coastal upwelling in the Humboldt current from model projections, Clim. Dynam., 52, 599–615, https://doi.org/10.1007/s00382-018-4158-7, 2019.
Pal, D., Khozin-Goldberg, I., Cohen, Z., and Boussiba, S.: The effect of light, salinity, and nitrogen availability on lipid production by Nannochloropsis sp., Appl. Microbiol. Biotechnol., 90, 1429–1441, https://doi.org/10.1007/s00253-011-3170-1, 2011.
Paul, A. J., Bach, L. T., Schulz, K. G., Boxhammer, T., Czerny, J., Achterberg, E. P., Hellemann, D., Trense, Y., Nausch, M., Sswat, M., and Riebesell, U.: Effect of elevated CO2 on organic matter pools and fluxes in a summer Baltic Sea plankton community, Biogeosciences, 12, 6181–6203, https://doi.org/10.5194/bg-12-6181-2015, 2015.
Peng, X., Liu, S., Zhang, W., Zhao, Y., Chen, L., Wang, H., and Liu, T.: Triacylglycerol accumulation of Phaeodactylum tricornutum with different supply of inorganic carbon, J. Appl. Phycol., 26, 131–139, https://doi.org/10.1007/s10811-013-0075-7, 2014.
Pitcher, G. C., Aguirre-Velarde, A., Breitburg, D., Cardich, J., Carstensen, J., Conley, D. J., Dewitte, B., Engel, A., EspinozaMorriberón, D., Flores, G., Garçon, V., Graco, M., Grégoire, M., Gutiérrez, D., Hernandez-Ayon, J. M., Huang, H.-H. M., Isensee, K., Jacinto, M. E., Levin, L., Lorenzo, A., Machu, E., Merma, L., Montes, I., Swa, N., Paulmier, A., Roman, M., Rose, K., Hood, R., Rabalais, N. N., Salvanes, A. G. V., Salvatteci, R., Sánchez, S., Sifeddine, A., Tall, A. W., Plas, A. K. v. d., Yasuhara, M., Zhang, J., and Zhu, Z. Y.: System controls of coastal and open ocean oxygen depletion, Prog. Oceanogr., 197, 102613, https://doi.org/10.1016/j.pocean.2021.102613, 2021.
Poerschmann, J., Spijkerman, E., and Langer, U.: Fatty acid patterns in Chlamydomonas sp. as a marker for nutritional regimes and temperature under extremely acidic conditions, Microb. Ecol., 48, 78–89, https://doi.org/10.1007/s00248-003-0144-6, 2004.
Popendorf, K. J., Lomas, M. W., and Van Mooy, B. A.: Microbial sources of intact polar diacylglycerolipids in the Western North Atlantic Ocean, Organ. Geochem., 42, 803–811, https://doi.org/10.1016/j.orggeochem.2011.05.003, 2011.
Popko, J., Herrfurth, C., Feussner, K., Ischebeck, T., Iven, T., Haslam, R., Hamilton, M., Sayanova, O., Napier, J., Khozin-Goldberg, I., and Feussner, I.: Metabolome analysis reveals betaine lipids as major source for triglyceride formation, and the accumulation of sedoheptulose during nitrogen-starvation of Phaeodactylum tricornutum, PLoS One, 11, 1–23, https://doi.org/10.1371/journal.pone.0164673, 2016.
Raven, J. A. and Beardall, J.: Carbohydrate metabolism and respiration in algae, in:Photosynthesis in algae, edited by: Larkum, A. W. D., Douglas, S. S., and Raven, J. A., 14, 205–224, Springer, Dordrecht, https://doi.org/10.1007/978-94-007-1038-2_10, 2003.
Riebesell, U., Czerny, J., Von Bröckel, K., Boxhammer, T., Büdenbender, J., Deckelnick, M., Fischer, M., Hoffmann, D., Krug, S. A., Lentz, U., Ludwig, A., Muche, R., and Schulz, K. G.: Technical Note: A mobile sea-going mesocosm system – New opportunities for ocean change research, Biogeosciences, 10, 1835–1847, https://doi.org/10.5194/bg-10-1835-2013, 2013.
Russell, N. J. and Nichols, D. S.: Polyunsaturated fatty acids in marine bacteria – a dogma rewritten, Microbiology, 145, 767–779, https://doi.org/10.1099/13500872-145-4-767, 1999.
Rütters, H., Sass, H., Cypionka, H., and Rullkötter, J.: Monoalkylether phospholipids in the sulfate-reducing bacteria Desulfosarcina variabilis and Desulforhabdus amnigenus, Arch. Microbiol., 176, 435–442, https://doi.org/10.1007/s002030100343, 2001.
Sakamoto, T. and Murata, N.: Regulation of the desaturation of fatty acids and its role in tolerance to cold and salt stress, Curr. Opin. Microbiol., 5, 206–210, https://doi.org/10.1016/S1369-5274(02)00306-5, 2002.
Sakurai, I., Hagio, M., Gombos, Z., Tyystjärvi, T., Paakkarinen, V., Aro, E. M., and Wada, H.: Requirement of Phosphatidylglycerol for Maintenance of Photosynthetic Machinery, Plant Physiol., 133, 1376–1384, https://doi.org/10.1104/pp.103.026955, 2003.
Sato, N. and Murata, N.: Temperature shift-induced responses in lipids in the blue-green alga, Anabaena variabilis, Biochim. Biophys. Acta, 619, 353–366, https://doi.org/10.1016/0005-2760(80)90083-1, 1980.
Sato, N., Aoki, M., Maru, Y., Sonoike, K., Minoda, A., and Tsuzuki, M.: Involvement of sulfoquinovosyl diacylglycerol in the structural integrity and heat-tolerance of photosystem II, Planta, 217, 245–251, https://doi.org/10.1007/s00425-003-0992-9, 2003.
Sayanova, O., Mimouni, V., Ulmann, L., Morant-Manceau, A., Pasquet, V., Schoefs, B., and Napier, J. A.: Modulation of lipid biosynthesis by stress in diatoms, Philos. Trans. R. Soc. B, 372, 20160407, https://doi.org/10.1098/rstb.2016.0407, 2017.
Schubotz, F., Wakeham, S. G., Lipp, J. S., Fredricks, H. F., and Hinrichs, K. U.: Detection of microbial biomass by intact polar membrane lipid analysis in the water column and surface sediments of the Black Sea, Environ. Microbiol., 11, 2720–2734, https://doi.org/10.1111/j.1462-2920.2009.01999.x, 2009.
Schubotz, F., Xie, S., Lipp, J. S., Hinrichs, K., and Wakeham, S. G.: Intact polar lipids in the water column of the eastern tropical North Pacific: abundace and structural variety of non-phosphorus lipids, Biogeosciences, 15, 6481–6501, https://doi.org/10.5194/bg-15-6481-2018, 2018.
Sinensky, M.: Homeoviscous adaptation: a homeostatic process that regulates the viscosity of membrane lipids in Escherichia coli, P. Natl. Acad. Sci. USA, 71, 522–525, https://doi.org/10.1073/pnas.71.2.522, 1974.
Singh, Y. and Kumar, H. D.: Lipid and hydrocarbon production by Botryococcus spp. under nitrogen limitation and anaerobiosis, World J. Microbiol. Biotechnol., 8, 121–124, https://doi.org/10.1007/BF01195829, 1992.
Smalley, G. W., Coats, D. W., and Stoecker, D. K.: Feeding in the mixotrophic dinoflagellate Ceratium furca is influenced by intracellular nutrient concentrations, Mar. Ecol. Prog. Ser., 262, 137–151, https://doi.org/10.3354/meps262137, 2003.
Speciale, G., Jin, Y., Davies, G. J., Williams, S. J., and Goddard-Borger, E. D.: YihQ is a sulfoquinovosidase that cleaves sulfoquinovosyl diacylglyceride sulfolipids, Nat. Chem. Biol., 12, 215–217, https://doi.org/10.1038/nchembio.2023, 2016.
Stramma, L., Johnson, G. C., Sprintall, J., and Mohrholz, V.: Expanding oxygen-minimum zones in the tropical oceans, Science , 320, 655–658, https://doi.org/10.1126/science.1153847, 2008.
Stramma, L., Schmidtko, S., Levin, L. A., and Johnson, G. C.: Ocean oxygen minima expansions and their biological impacts, Deep-Sea Res. Pt. I, 57, 587–595, https://doi.org/10.1016/j.dsr.2010.01.005, 2010.
Sturt, H. F., Summons, R. E., Smith, K., Elvert, M., and Hinrichs, K. U.: Intact polar membrane lipids in prokaryotes and sediments deciphered by high-performance liquid chromatography/electrospray ionization multistage mass spectrometry – New biomarkers for biogeochemistry and microbial ecology, Rapid Commun. Mass Sp., 18, 617–628, https://doi.org/10.1002/rcm.1378, 2004.
Sukenik, A., Zmora, O., and Carmeli, Y.: Biochemical quality of marine unicellular algae with special emphasis on lipid composition, II. Nannochloropsis sp., Aquaculture, 117, 313–326, https://doi.org/10.1016/0044-8486(93)90328-V, 1993.
Suzumura, M.: Phospholipids in marine environments: a review, Talanta, 66, 422–434, https://doi.org/10.1016/j.talanta.2004.12.008, 2005.
Tatsuzawa, H. and Takizawa, E.: Fatty Acid and Lipid Composition of the Acidophilic green alga Chlamydomonas Sp., J. Phycol., 32, 598–601, https://doi.org/10.1111/j.0022-3646.1996.00598.x, 1996.
Taucher, J., Bach, L. T., Boxhammer, T., Nauendorf, A., Achterberg, E. P., Algueró-Muñiz, M., Arístegui, J., Czerny, J., Esposito, M., Guan, W., Haunost, M., Horn, H. G., Ludwig, A., Meyer, J., Spisla, C., Sswat, M., Stange, P., Riebesell, U., Aberle-Malzahn, N., Archer, S., Boersma, M., Broda, N., Büdenbender, J., Clemmesen, C., Deckelnick, M., Dittmar, T., Dolores-Gelado, M., Dörner, I., Fernández-Urruzola, I., Fiedler, M., Fischer, M., Fritsche, P., Gomez, M., Grossart, H. P., Hattich, G., Hernández-Brito, J., Hernández-Hernández, N., Hernández-León, S., Hornick, T., Kolzenburg, R., Krebs, L., Kreuzburg, M., Lange, J. A. F., Lischka, S., Linsenbarth, S., Löscher, C., Martínez, I., Montoto, T., Nachtigall, K., Osma-Prado, N., Packard, T., Pansch, C., Posman, K., Ramírez-Bordón, B., Romero-Kutzner, V., Rummel, C., Salta, M., Martínez-Sánchez, I., Schröder, H., Sett, S., Singh, A., Suffrian, K., Tames-Espinosa, M., Voss, M., Walter, E., Wannicke, N., Xu, J., and Zark, M.: Influence of ocean acidification and deep water upwelling on oligotrophic plankton communities in the subtropical North Atlantic: Insights from an in situ mesocosm study, Front. Mar. Sci., 4, 85, https://doi.org/10.3389/fmars.2017.00085, 2017.
Thamdrup, B., Dalsgaard, T., and Revsbech, N. P.: Widespread functional anoxia in the oxygen minimum zone of the Eastern South Pacific, Deep-Sea Res. Pt. I, 65, 36–45, https://doi.org/10.1016/j.dsr.2012.03.001, 2012.
Tréguer, P., Bowler, C., Moriceau, B., Dutkiewicz, S., Gehlen, M., Aumont, O., Bittner, L., Dugdale, R., Finkel, Z., Iudicone, D., Jahn, O., Guidi, L., Lasbleiz, M., Leblanc, K., Levy, M., and Pondaven, P.: Influence of diatom diversity on the ocean biological carbon pump, Nat. Geosci, 11, 27–37, https://doi.org/10.1038/s41561-017-0028-x, 2018.
Tretkoff, E.: Research Spotlight: Coastal cooling and marine productivity increasing off Peru, Eos Transact. Am. Geophys. Union, 92, 184–184, https://doi.org/10.1029/2011eo210009, 2011.
Twining, C. W., Taipale, S. J., Ruess, L., Bec, A., Martin-Creuzburg, D., and Kainz, M. J.: Stable isotopes of fatty acids: Current and future perspectives for advancing trophic ecology, Philos. T. R. Soc. B, 375, 1804, https://doi.org/10.1098/rstb.2019.0641, 2020.
Tyralis, H., Papacharalampous, G., and Langousis, A.: A brief review of random forests for water scientists and practitioners and their recent history in water resources, Water, 11, 910, https://doi.org/10.3390/w11050910, 2019.
Ulloa, O. and Pantoja, S.: The oxygen minimum zone of the eastern South Pacific, Deep-Sea Res. Pt. II, 56, 987–991, https://doi.org/10.1016/j.dsr2.2008.12.004, 2009.
Van Mooy, B. A. S., Rocap, G., Fredricks, H. F., Evans, C. T., and Devol, A. H.: Sulfolipids dramatically decrease phosphorus demand by picocyanobacteria in oligotrophic marine environments, P. Natl. Acad. Sci. USA, 103, 8607–8612, https://doi.org/10.1073/pnas.0600540103, 2006.
Van Mooy, B. A. S., Fredricks, H. F., Pedler, B. E., Dyhrman, S. T., Karl, D. M., Koblížek, M., Lomas, M. W., Mincer, T. J., Moore, L. R., Moutin, T., Rappé, M. S., and Webb, E. A.: Phytoplankton in the ocean use non-phosphorus lipids in response to phosphorus scarcity, Nature, 458, 69–72, https://doi.org/10.1038/nature07659, 2009.
Van Mooy, B. A. S. and Fredricks, H. F.: Bacterial and eukaryotic intact polar lipids in the eastern subtropical South Pacific: Water-column distribution, planktonic sources, and fatty acid composition, Geochim. Cosmochim. Ac., 74, 6499–6516, https://doi.org/10.1016/j.gca.2010.08.026, 2010.
Vargas, C. A., Cantarero, S. I., Sepúlveda, J. A., Galán, A., De Pol Holz, R., Walker, B., Schneider, W., Farías, L., Cornejo D'Ottone, M., Walker, J., Xu, X., and Salisbury, J.: A source of isotopically light organic carbon in a low-pH anoxic marine zone, Nat. Commun., 12, 1604, https://doi.org/10.1038/s41467-021-21871-4, 2021.
Volkman, J. K., Jeffrey, S. W., Nichols, P. D., Rogers, G. I., and Garland, C. D.: Fatty acid and lipid composition of 10 species of microalgae used in mariculture, J. Exp. Mar. Bio. Ecol., 128, 219–240, https://doi.org/10.1016/0022-0981(89)90029-4, 1989.
Volkman, J. K., Barrett, S. M., Blackburn, S. I., Mansour, M. P., Sikes, E. L., and Gelin, F.: Microalgal biomarkers: A review of recent research developments, Org. Geochem., 29, 1163–1179, https://doi.org/10.1016/S0146-6380(98)00062-X, 1998.
Wada, H. and Murata, N.: Lipids in thylakoid membranes and photosynthetic cells, in: Lipids in Photosynthesis, Springer, Dordrecht, 1–9, https://doi.org/10.1007/978-90-481-2862-1_1, 2009.
Wagner, S., Schubotz, F., Kaiser, K., Hallmann, C., Waska, H., Rossel, P. E., Hansman, R., Elvert, M., Middelburg, J. J., Engel, A., Blattmann, T. M., Catalá, T. S., Lennartz, S. T., Gomez-Saez, G. V., Pantoja-Gutiérrez, S., Bao, R., and Galy, V.: Soothsaying DOM: A Current Perspective on the Future of Oceanic Dissolved Organic Carbon, Front. Mar. Sci., 7, 1–17, https://doi.org/10.3389/fmars.2020.00341, 2020.
Wakeham, S. G., Turich, C., Schubotz, F., Podlaska, A., Li, X. N., Varela, R., Astor, Y., Sáenz, J. P., Rush, D., Sinninghe Damsté, J. S., Summons, R. E., Scranton, M. I., Taylor, G. T., and Hinrichs, K. U.: Biomarkers, chemistry and microbiology show chemoautotrophy in a multilayer chemocline in the Cariaco Basin, Deep-Sea Res. Pt. I, 63, 133–156, https://doi.org/10.1016/j.dsr.2012.01.005, 2012.
Wang, X., Shen, Z., and Miao, X.: Nitrogen and hydrophosphate affects glycolipids composition in microalgae, Sci. Rep., 6 1–9, https://doi.org/10.1038/srep30145, 2016.
Wilhelm, C., Jungandreas, A., Jakob, T., and Goss, R.: Light acclimation in diatoms: From phenomenology to mechanisms, Mar. Genom., 16, 5–15, https://doi.org/10.1016/j.margen.2013.12.003, 2014.
Wörmer, L., Lipp, J. S., Schröder, J. M., and Hinrichs, K. U.: Application of two new LC–ESI–MS methods for improved detection of intact polar lipids (IPLs) in environmental samples, Org. Geochem., 59, 10–21, https://doi.org/10.1016/j.orggeochem.2013.03.004, 2013.
Wörmer, L., Lipp, J. S., and Hinrichs, K. U.: Comprehensive analysis of microbial lipids in environmental samples through HPLC-MS protocols, in: Hydrocarbon and lipid microbiology protocols, Springer, Berlin, Heidelber, 289–317, https://doi.org/10.1007/8623_2015_183, 2015.
Wright, J. J., Konwar, K. M., and Hallam, S. J.: Microbial ecology of expanding oxygen minimum zones, Nat. Rev. Microbiol., 10, 381–394, https://doi.org/10.1038/nrmicro2778, 2012.
Wu, R. S. S., Wo, K. T., and Chiu, J. M. Y.: Effects of hypoxia on growth of the diatom Skeletonema costatum, J. Exp. Mar. Biol. Ecol., 420/421, 65–68, https://doi.org/10.1016/j.jembe.2012.04.003, 2012.
Wyrtki, K.: The oxygen minima in relation to ocean circulation, Deep-Sea Res. Oceanogr. Abstr., 9, 11–23, https://doi.org/10.1016/0011-7471(62)90243-7, 1962.
Yang, C., Boggasch, S., Haase, W., and Paulsen, H.: Thermal stability of trimeric light-harvesting chlorophyll a/b complex (LHCIIb) in liposomes of thylakoid lipids, BBA-Bioenergetics, 1757, 1642–1648, https://doi.org/10.1016/j.bbabio.2006.08.010, 2006.
Yang, K. and Han, X.: Accurate quantification of lipid species by electrospray ionization mass spectrometry – Meets a key challenge in lipidomics, Metabolites, 1, 21–40, https://doi.org/10.3390/metabo1010021, 2011.
Yang, W., Catalanotti, C., Wittkopp, T. M., Posewitz, M. C., and Grossman, A. R.: Algae after dark: Mechanisms to cope with anoxic/hypoxic conditions, Plant J., 82, 481–503, https://doi.org/10.1111/tpj.12823, 2015.
Yeesang, C. and Cheirsilp, B.: Effect of nitrogen, salt, and iron content in the growth medium and light intensity on lipid production by microalgae isolated from freshwater sources in Thailand, Bioresour. Technol., 102, 3034–3040, https://doi.org/10.1016/j.biortech.2010.10.013, 2011.
Yoon, K., Han, D., Li, Y., Sommerfeld, M., and Hu, Q.: Phospholipid:Diacylglycerol acyltransferase is a multifunctional enzyme involved in membrane lipid turnover and degradation while synthesizing triacylglycerol in the unicellular green microalga chlamydomonas reinhardtii, Plant Cell, 24, 3708–3724, https://doi.org/10.1105/tpc.112.100701, 2012.
Yvon-Durocher, G., Allen, A. P., Cellamare, M., Dossena, M., Gaston, K. J., Leitao, M., Montoya, J. M., Reuman, D. C., Woodward, G., and Trimmer, M.: Five Years of Experimental Warming Increases the Biodiversity and Productivity of Phytoplankton, PLoS Biol., 13, 1–22, https://doi.org/10.1371/journal.pbio.1002324, 2015.
Zienkiewicz, K., Du, Z. Y., Ma, W., Vollheyde, K., and Benning, C.: Stress-induced neutral lipid biosynthesis in microalgae – Molecular, cellular and physiological insights, BBA-Mol. Cell Biol. L., 1861, 1269–1281, https://doi.org/10.1016/j.bbalip.2016.02.008, 2016.
Zink, K.-G., Wilkes, H, Disko, U., Elvert, M., and Horsfield, B.: Intact phospholipids – microbial “life markers” in marine deep subsurface sediments, Org. Geochem., 34, 755–769, https://doi.org/10.1016/S0146-6380(03)00041-X, 2003.