the Creative Commons Attribution 4.0 License.
the Creative Commons Attribution 4.0 License.
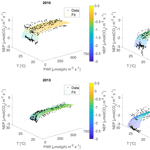
High-frequency productivity estimates for a lake from free-water CO2 concentration measurements
Maria Provenzale
Anne Ojala
Jouni Heiskanen
Kukka-Maaria Erkkilä
Ivan Mammarella
Pertti Hari
Timo Vesala
Lakes are important actors in biogeochemical cycles and a powerful natural source of CO2. However, they are not yet fully integrated in carbon global budgets, and the carbon cycle in the water is still poorly understood. In freshwater ecosystems, productivity studies have usually been carried out with traditional methods (bottle incubations, 14C technique), which are imprecise and have a poor temporal resolution. Consequently, our ability to quantify and predict the net ecosystem productivity (NEP) is limited: the estimates are prone to errors and the NEP cannot be parameterised from environmental variables. Here we expand the testing of a free-water method based on the direct measurement of the CO2 concentration in the water. The approach was first proposed in 2008, but was tested on a very short data set (3 days) under specific conditions (autumn turnover); despite showing promising results, this method has been neglected by the scientific community. We tested the method under different conditions (summer stratification, typical summer conditions for boreal dark-water lakes) and on a much longer data set (40 days), and quantitatively validated it comparing our data and productivity models. We were able to evaluate the NEP with a high temporal resolution (minutes) and found a very good agreement (R2≥0.71) with the models. We also estimated the parameters of the productivity–irradiance (PI) curves that allow the calculation of the NEP from irradiance and water temperature. Overall, our work shows that the approach is suitable for productivity studies under a wider range of conditions, and is an important step towards developing this method so that it becomes more widely used.
- Article
(931 KB) - Full-text XML
-
Supplement
(4700 KB) - BibTeX
- EndNote
Lakes are very important actors in the local and global carbon cycles (Battin et al., 2009; Tranvik et al., 2009). They both fix carbon, through photosynthesis of the in-lake primary producers, and release it, through respiration of all the aquatic organisms (primary producers, consumers and microbes), through photochemical reactions and by transmitting the received carbon from the catchment (lateral transport) back to the atmosphere in gaseous form (primarily as CO2). Many lakes – especially the oligotrophic ones typical of high latitudes – are net heterotrophic systems where the rate of community respiration exceeds that of primary production (Cole et al., 1994; Sobek et al., 2003); this contributes to make lakes one of the most important natural sources of greenhouse gases (Raymond et al., 2013). However, they are not yet fully integrated into the local and global carbon budgets, and the lacustrine carbon cycle is still poorly known (Cole et al., 2007).
In freshwater ecology, productivity studies have usually relied on the light and dark bottle method (Gaarder and Gran, 1927) and the 14C labelling technique (Steemann Nielsen, 1951; Peterson, 1980; Bender et al., 1987; Søndergaard, 2002). The first provides estimates both of the gross and the net primary productivity, whereas the latter gives an estimate that is between the gross and the net productivity, depending on the incubation time. These traditional methods require time- and effort-demanding measurements and have a poor temporal resolution. Periods of high productivity are easily missed (Karl et al., 2003) and, because of the low temporal resolution, the non-linear relationship between photosynthetically active solar radiation (PAR) and photosynthesis cannot be properly investigated. As a consequence, carbon balances may be imprecise and for instance the net ecosystem productivity (NEP) cannot be parameterised robustly as a function of ambient variables. Moreover, communities enclosed in bottles experience light and nutrient conditions far from the natural ones, since the movement of water or of the organisms themselves is limited (Mallin and Paerl, 1992; Reynolds, 2006), and the results can be unrealistic. Thus, advances in the methodology are necessary to better estimate freshwater ecosystem productivity and to expand our understanding of the carbon cycle in the water column.
In the last 15 years, free-water methods, not requiring sampling and incubation, have become more common. These methods, however, are usually based on the measurement of the O2 concentration in the water, which is then used as a proxy for CO2 (Hoellein et al., 2013; Solomon et al., 2013); this introduces uncertainties (Staehr et al., 2010). The respiratory quotient that has to be applied when transforming rates from O2 to CO2 has, in fact, large variations (Berggren et al., 2012).
To study the in-water photosynthesis and respiration, Hari et al. (2008) proposed a free-water method based on the direct measurement of the CO2 concentration in the water with non-dispersive infra-red (NDIR) CO2 probes, associated with a concomitant assessment of the CO2 flux between the lake and the atmosphere. The probes are designed to measure the CO2 concentration in the air, but by building a gas collection system the concentration in the water is obtained. Similar probes have also been used in Johnson et al. (2010), albeit not for productivity studies. The temporal resolution is 5 seconds, more than a hundredfold improvement over the traditional approaches. A requirement of the method is the concomitant assessment of the CO2 flux from the lake to the atmosphere. Information on the in-lake vertical CO2 flux is also needed (and, ideally, on the lateral transport as well). If such data are missing the method can be applied under specific conditions (e.g. stable stratification); it still allows for the parameterisation of the NEP from PAR and water temperature, from which the NEP can then be calculated under different conditions.
In Hari et al. (2008), the method was tested on a small boreal lake in Finland over 3 days only, during the autumn turnover. A cross comparison was carried out between different measurement methods, but the NEP was not mathematically parameterised and the method was not quantitatively verified. Despite the very short data set and the specific conditions, the results were promising: the relationship between PAR and NEP was clearly visible, the measured respiration rate was 16 times higher than with the bottle method and the measured productivity was 5 times higher than with the 14C technique. The numbers are in line with previous studies: Pace and Prairie (2005) reported similar discrepancies between an oxygen-based free-water approach and the bottle method in small lakes in Michigan, and a tendency of the 14C method to underestimate the productivity is well known (Howarth and Michaels, 2000). However, the method has been overlooked and has not been used for productivity calculations since 2008, possibly because of the limited testing.
Here we tested the method of Hari et al. (2008) on a different boreal lake, under different conditions and on a much longer data set, quantitatively verifying it. We continuously collected data for four summers, and then we focused on the periods when the lake was stably stratified, i.e. summer conditions typical of boreal dark-water lakes, in order to rule out the lateral CO2 flux and the CO2 flux from the deeper layers of the lake. Overall, we analysed 40 days of data. We calculated the NEP using the equations that are typically used in forest ecology, where high-frequency measurements are more common, in an effort of harmonising the procedures between different fields. Once we had the NEP with a high temporal resolution, we verified the relationship between the NEP and irradiance, using a saturating Michaelis–Menten model. We found an excellent agreement between the data and the model. From that, we could also estimate the parameters of the productivity–irradiance (PI) curves, specific to the in-lake communities. These parameters are very important because they allow for the calculation of the NEP from PAR and water temperature.
Whilst our efforts were mainly focused on method testing and development, we also checked whether the parameters of the PI curves we estimated changed significantly between the years. Our goal was to gather information on how sensitive the parameters are to variations in the communities living in the lake or in the environmental conditions. We investigated whether their behaviour could be related to their main drivers, water temperature and irradiance.
2.1 Study site
The study site is the boreal lake Kuivajärvi, in southern Finland (61∘50.743′ N, 24∘17.134′ E). Lake Kuivajärvi is a typical dark-water boreal lake. It is small and oblong and it is surrounded by managed coniferous forests. Its surface area is 0.62 km2 and its length is 2.6 km; its mean depth and maximum depth are 6.3 and 13.2 m, respectively. The lake is humic (surface median dissolved organic carbon concentration = 11.8 mg L−1 in 2011) and mesotrophic (surface median annual total nitrogen concentration = 370 µg L−1 and annual total phosphorus concentration = 14 µg L−1 in 2011), with a chlorophyll a (Chl a) concentration in the surface layer usually between 3 and 5 µg L−1 (median 4.8 µg L−1 in 2011), with summer values that can reach 30 µg L−1 (Miettinen et al., 2015). The lake is dark coloured: the Secchi depth ranges from 1.2 to 1.5 m (Heiskanen et al., 2015). The lake is dimictic and it is frozen for 5 months every year on average; the spring turnover occurs immediately after the ice-out in late April or early May, and after the turnover a thermocline starts developing. The thermocline deepens until the autumn turnover, and finally the lake freezes over in late November or early December (Heiskanen et al., 2015; Mammarella et al., 2015). Most of the inflow is through a permanent stream at the northern end, while the role of groundwater is small during summer. Temporary inflows appear at snowmelt, through several small ephemeral streams. The outflow is located at the southern end. The residence time was 522 days in 2011 and 655 days in 2013. A map with the location and bathymetry of the lake is available in the Supplement (Figs. S1 and S2).
2.2 Measurements
All the instruments were mounted on a raft, which was moored in the middle of the lake (see Fig. S2, for the exact position of the raft on the lake). To measure the CO2 concentration in the water, a closed system consisting of a NDIR probe (CARBOCAP® GMP343, Vaisala Oyj, Vantaa, Finland) for the CO2 concentration in the air, gas-impermeable tubes (stainless steel and Teflon) and a submerged gas-permeable tube (silicone rubber, Rotilabo 9572.1, Carl Roth GmbH and Co. KG, Karlsruhe, Germany) was built; the air was circulated continuously in the system by a diaphragm pump (KNF Neuberger Micro gas pump, KNF Neuberger AB, Stockholm, Sweden). Analog voltage outputs were used, logged with a Nokeval RMD680 serial transmitter to a ASCII file on a Windows-based computer. Since silicone rubber has an excellent permeability to CO2 (Carignan, 1998; Hari et al., 2008), the concentration of CO2 in the air circulating in the system equilibrated with that in the water around the submerged tube. Hence, the CO2 concentration in the water could be obtained from that in the air using the dependence of CO2 solubility on temperature and pressure. The CO2 concentration in the water (dissolved CO2), in µmol m−3, was calculated as
where is the CO2 gas phase mole fraction in the tube measured by the probe (in µmol mol−1), P is the total air pressure inside the system and KH is Henry's law constant (temperature dependent). For more details on the set-up see Hari et al. (2008), Heiskanen et al. (2014) and the Supplement (Fig. S3). The CO2 concentration in the water was measured at a depth of 0.2 m (determined by the depth of the submerged silicone tube). The system was operating continuously from May to September 2010–2014, but the data from year 2012 are not used here due to technical problems. The silicone tube was cleaned once a week to avoid biofouling and changed once a month. The CO2 sensors were calibrated using span and zero gases. A thermistor chain of 16 Pt100 resistance thermometers (depths: 0.2, 0.5, 1.0, 1.5, 2.0, 2.5, 3.0, 3.5, 4.0, 4.5, 5.0, 6.0, 7.0, 8.0, 10.0 and 12.0 m) was deployed and a PAR sensor (LI-192, LI-COR Inc., Nebraska, USA) for photosynthetic photon flux density (PPFD) was submerged in the water at the same depth as the CO2 measurement (0.2 m). An eddy covariance (EC) system (with ultrasonic anemometer USA-1, Metek GmbH, Germany and closed-path infra-red gas analyzer LI-7000, LI-COR Inc., Nebraska, USA; replaced in 2011 by enclosed-path infra-red gas analyzer LI-7200, LI-COR Inc., Nebraska, USA) was used to detect the CO2 flux between the lake and the atmosphere. The fluxes were calculated and quality screened according to the standard procedures, see Vesala et al. (2006), Mammarella et al. (2009, 2015) and the Supplement (Sect. S2). All the instruments were powered by mains electricity.
2.3 Calculation of the net ecosystem productivity
The net ecosystem productivity (NEP, µmol(CO2) m−2 s−1), also called net ecosystem uptake, can be defined as
where GPP (gross primary productivity) is the amount of carbon fixed by the primary producers through photosynthesis and Rh (ecosystem respiration) is the amount of carbon lost through respiration, both autotrophic and heterotrophic. Provided that there are no inorganic sinks or sources of CO2, the NEP is the opposite of the net ecosystem exchange (NEE), whose expression can be derived from the conservation of mass. Hence, considering the mass balance of CO2 in the mixed layer of the lake, where most of the photosynthesis takes place, and assuming that lateral transport of CO2 is of no importance, the NEP can also be expressed as
In Eq. (3), is the CO2 concentration in the water calculated from Eq. (1), Fa is the CO2 flux between the lake and the atmosphere (positive if from the lake to the atmosphere), Fu is the CO2 flux between the deeper and the mixed layer of the lake (positive if upwards), t is time and z is depth. The integration is computed between the mixing depth hmix and the surface. The mixing depth is defined as the depth at which the water temperature starts decreasing faster than one degree per metre (Staehr et al., 2010); in our case the average value for the entire study period was hmix=1.5 m. Given the dark water colour and the resulting low light conditions in the lake, there was no benthic primary production in the profundal zone. For years 2010 and 2011, another CO2 probe was located at a depth of 0.5 m, and its readings were consistent with those from the probe at 0.2 m, hence showing homogeneous CO2 concentrations in the mixed layer. While was measured by the probe and Fa by the EC system, we had no precise way of measuring Fu. This is the main reason behind our choice to limit the analysis to the summer days when the lake was stably stratified and it was safe to assume no gas was exchanged through the thermocline: Fu=0. The periods of stable stratifications were chosen on the basis of temperature plots and of the Schmidt stability of the lake, calculated with the LakeAnalyzer program, according to Read et al. (2011). For all the chosen days, the stability (Sc) is > 100 J m−2. However, not all days with Sc>100 J m−2 were used: days with strong winds or stable atmospheric stratification were discarded because of their impact on fluxes (for more detailed information, see the end of this section). For the time series of isotherms for the whole summers (from 1 June to 31 August), and the time series of isotherms, Schmidt stability, CO2 concentration and PAR at 0.2 m and air temperature for the periods of stable stratification chosen for analysis each year see the Supplement (Figs. S4–S14). Overall, we analysed 40 days in 10 periods occurring between mid-June and the end of July of each year.
It is worth pointing out that Eq. (3) resembles the equation used in terrestrial ecology to estimate the NEP. In fact, considering for example forest EC calculations (Foken et al., 2012), neglecting lateral transport, the NEP is
In Eq. (4), (ρd = dry air density, = CO2 mixing ratio) replaces as the CO2 concentration in the air instead of in the water, and z is the height (with hm = measuring height); is Fa, the CO2 flux from the forest to the atmosphere, calculated as the covariance between the fluctuations of the vertical wind velocity and the gas mixing ratio. High-frequency measurements for productivity are common in forest ecology. They are, however, less common in aquatic ecology, where traditional approaches are still widespread despite their limitations (low temporal resolution, unnatural conditions). Having different methodologies and different time resolutions creates a gap between the two fields, and makes comparing the estimates more difficult. Given that the terrestrial and aquatic ecosystems are a continuum through which carbon is cycled, using shared procedures is a step in the direction of connecting and integrating these ecosystems, in order to have more precise carbon budgets and a deeper knowledge of the carbon cycle.
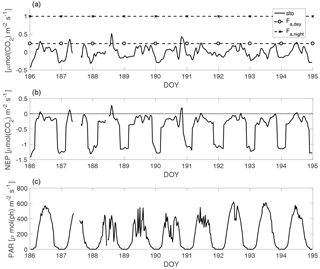
Figure 1A sample period of stable stratification in July 2010, representative of the studied periods (DOY is day of the year). In panel (a), the solid line (sto) is the first term of Eq. (3), the CO2 concentration change in time over the mixed layer, which is usually referred to as storage flux in forest ecology calculations; the dashed horizontal lines are the daytime and nighttime average CO2 fluxes from the lake to the atmosphere (Fa). In panel (b), the solid line is the NEP and the dotted line is the zero rate. In panel (c), the solid line is the PAR (photon flux density measured in the PAR wavelength range) average value in the mixed layer. The resolution is 30 min, except for the Fa average values.
Resuming our calculation of the NEP in aquatic ecosystems through Eq. (3), to increase the precision of the concentration data, half-hourly averages of from the raw 5-second data were used. A 30 min resolution is enough to capture the variations caused by the biological activity and at the same time filter out the ones caused by the physical mixing of the water (Staehr et al., 2010). However, the EC data set, which also has a resolution of 30 min, had many gaps, due to inherent problems of the EC technique (wind not blowing along the lake, stability or not fully developed turbulence resulting in quality criteria not met) and technical problems (instrument failures). Approximately 70 % of the data points for the summers were rejected or missing, with occurrences of consecutive days having no acceptable data points at all. Hence, for our data set, a point by point calculation of Fa in Eq. (3) was not possible. Even though in general it would not be needed, we had to use a daytime and a nighttime average value for Fa; we maintained the half-hourly calculation of the NEP to preserve the temporal resolution. The daytime and nighttime average Fa values were calculated separately for each year, combining all the studied periods of water stable stratification of the same summer. Before doing so, we checked that the environmental conditions (temperature and relative humidity cycles, incoming radiation, wind speed and direction, atmospheric stability) were similar for all the analysed days in the summer. In particular, since wind and atmospheric stability have the greatest influence on the fluxes (given that the lake water is thermally stratified), as verified in Heiskanen et al. (2014), we discarded any day with winds > 5 m s−1 or with stable atmospheric stratification. For the remaining days, the wind was always weak, with averages < 2.5 m s−1; at such low speeds, the influence of the wind on the flux is negligible (Cole and Caraco, 1998). Under these circumstances (i.e. warm and sunny summer days without strong wind events), the CO2 flux is expected to have similar daily cycles across the studied days, as is shown by the available EC data and by the EC data from years with more complete data sets. Day and night were defined on the basis of PAR. When using PAR, we are referring to the average PAR value in the mixed layer, obtained from the 0.2 m value through the lake light extinction coefficient (1.5). The threshold between day and night was set to 20 µmol(ph) m−2 s−1 and it was chosen by calculating the average value of PAR at which the CO2 concentration in the water started decreasing in the morning after accumulating during the night, or increasing again in the evening. Using this procedure, “day” represents the fraction of the time series when photosynthesis dominates over respiration, and not the times when photosynthesis takes place in absolute terms. We also estimated the uncertainties in the daytime and nighttime average values of Fa. We decided not to use the standard deviation, since individual 30 min EC data are characterised by significant scatter. Instead, we recalculated the daytime and nighttime averages randomly choosing only half of the data in the sample, and repeated the process 100 times. Then we checked how far apart the minimum and maximum average values we obtained were, and used that as uncertainty.
At this point, we were able to calculate the half-hourly values of NEP for each period.
2.4 Relationship between NEP and PAR
In humic lakes, photosynthesis is strongly driven by PAR, and the relationship can be described for instance by the Michaelis–Menten equation (Caperon, 1967; Kiefer and Mitchell, 1983). Assuming that the daytime respiration rate equals the nighttime respiration rate and that they depend exponentially on temperature (Carignan et al., 2000), the NEP can be expressed as
In Eq. (5), T is the water temperature (in ∘C) and Q10 is a non-dimensional temperature coefficient whose generally accepted value for freshwater communities (and the value we used) is 2; in the literature, values between 1.88 and 2.19 are reported: Reynolds (1984), Raven and Geider (1988) and Davison (1991). The parameters pmax, b and r0 represent the maximum potential photosynthetic rate, the half-saturation constant (i.e. the value of PAR at which the photosynthetic rate is half of the maximum rate) and the basal respiration rate, respectively. These parameters are important, since they allow the calculation of NEP from water temperature and PAR; their values can be obtained by fitting the model to the data.
After calculating the NEP, we plotted the NEP versus irradiance curves. We then fitted the model (Eq. 5) to the NEP data with the least-squares fitting method, in order to check the agreement between the data and the model and in order to estimate pmax, b and r0.
Each year was handled separately, since the conditions (PAR and water T) varied. We then verified whether the changes in the parameter values between the years were statistically significant. To do so, we calculated the parameters difference and its confidence interval (calculated as the uncertainty in the difference, from the confidence intervals of the parameters themselves), and checked whether it overlapped 0. If it did not, then the values were statistically significantly different.
3.1 General results
The NEP had the same trend as the incoming radiation, as expected; it had bigger negative values during the night, when only respiration took place, and smaller negative values during the day, when photosynthesis contributed with an uptake of CO2. However, the net productivity values are almost always negative, meaning that the ecosystem, overall, is heterotrophic and a source of CO2. In fact, the daytime and nighttime average values of the CO2 flux were also always positive, albeit having lower values during the day than during the night. This is not surprising: many lakes, especially at high latitudes, are supersaturated with respect to CO2 (Cole et al., 1994; Sobek et al., 2003); as a result, the CO2 flux is from the lake to the atmosphere also during the day, when the aquatic primary producers are photosynthesising and absorbing CO2.
Figure 1 shows the CO2 concentration change in time over the mixed layer (the first term in Eq. 3), which is usually referred to as storage flux in forest ecology calculations, the NEP, the average daytime and nighttime values of the CO2 flux ( and ) and PAR for a sample period of stable stratification in July 2010, representative of the analysed periods. The 9-day period in Fig. 1 is the longest of the entire data set. Generally, stable stratification lasted from 2 to 5 days; its short duration is due to the oblong shape of the lake, that makes it sensitive to wind action: as soon as the wind increases the mixing is enhanced (although complete mixing takes place only in spring and autumn).
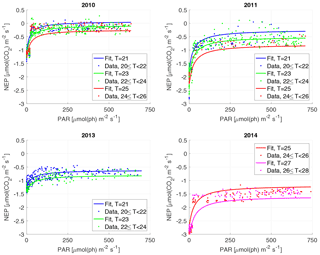
Figure 3The NEP versus PAR plots for each year. Each dot represents a 30 min interval, colour-classified according to water temperature classes, and the curves are calculated for the different temperatures. Note that the curves are not individual fits, but are the result of the year's 3-D fit, evaluated for the different temperatures. Water T is in ∘C.
Table 1Fit statistics, parameters of the NEP vs. PAR and water T model with 95 % confidence intervals (from Eq. 5), and average, minimum and maximum values of water T and PAR in the mixed layer for the studied periods of each year. RMSE, pmax and r0 in µmol(CO2) m−2 s−1, b and PAR in µmol(ph) m−2 s−1 and T in ∘C.

For the NEP versus PAR curves (Figs. 2–3), as mentioned above, we decided to draw a different plot for each year, instead of combining all the data points from all the years, since the conditions varied from year to year. Figure 2 displays the model curve calculated using the average water T of the studied periods of each year. From the plots, we can see that for low values of PAR, the NEP was strongly negative; then, as PAR increased, the NEP quickly increased as well; however, as already noted, the NEP always remained negative, indicating net heterotrophy. None of the years exhibited signs of photoinhibition: the NEP did not seem to decrease even at high (>500 µmol(ph) m−2 s−1) values of PAR. Differences can be seen between the years, with 2014 showcasing the smallest values of NEP. Year 2014 was particularly hot, so the strongly negative NEP can be due to increased respiration rates, given the strong dependency of Rh on temperature; year 2010 though displays the highest values of NEP despite having an intermediate average water temperature. Figure 3 concentrates on the dependence of the NEP on T. The model is calculated for different values of water T, ranging from the minimum to the maximum water temperatures recorded during the studied periods of each year. The NEP decreases with increasing temperature, due to higher respiration rates. Note that in both Figs. 3 and 4 and especially for years 2010 and 2014 there is a large separation between NEP across the chosen PAR threshold between night and day. This is caused by having to resort to daytime and nighttime average values for Fa. Finally, Fig. 4 features 3-D plots of the data and the curves, to visualise simultaneously the dependence of the NEP on PAR and water T. The curves have the expected trends, and this suggests that the measurement method and the equation used are proper tools for estimating the NEP at a high temporal resolution. The results of the fittings of the NEP versus PAR and T are reported in Table 1. Considering the assumptions we had to adopt, there is a very good agreement between the model and the data: the R2 values range from 0.71 to 0.84. This clearly indicates that the method used here allows the NEP to be parameterised as a function of irradiance and water temperature.
3.2 Inter-annual variability
We then focused on the inter-annual variability in the values of the model parameters (reported in Table 1). The differences in the parameter values between the years are mainly statistically significant. Only the value of b does not change significantly between any of the years: this means that the algal communities adapted to the light conditions in a similar way every year. The values of the other parameters change: pmax is comparable only between 2011 and 2014, and r0 is never comparable. The difference in pmax and r0 can be due to different total algal biomass in the lake. In general, we can say that variations in the environmental conditions might have led to changes in the communities living in the lake, or the communities might have responded differently to the environmental conditions; pmax and r0 seem to be more sensitive to variations than b.
The maximum photosynthetic rate pmax ranged between 1.55 (2014) and 0.63 (2013) µmol(CO2) m−2 s−1, and it was higher in 2011 and 2014 than in 2010 and 2013. The half-saturation constant b ranged between 22 (2010) and 33 (2013) µmol(ph) m−2 s−1, being higher in 2011, 2013 and 2014 than in 2010. The values of b are relatively small. It indicates that the phytoplankton communities were well adapted to the low light conditions (boreal area and dark-water lake) and were able to start photosynthesising even when the incoming radiation was low. The basal respiration r0 ranged between 0.228 (2010) and 0.482 (2014) µmol(CO2) m−2 s−1, being higher in 2011 and 2014 than in 2010 and 2013, as was the case with pmax. The parameters, however, do not appear to be strictly correlated to each other, and a clear and uniform pattern in their behaviour cannot be identified.
Finally, we investigated whether the changes in the model parameters can be explained in terms of changes, during the analysed periods, of the ambient variables that act as NEP drivers: water temperature and irradiance. The model parameters and the average, minimum and maximum values of water T and PAR for each year are reported in Table 1 (only the 40 analysed days are considered in these statistics). In 2010 and 2011 the surface water temperatures had similar average values of 22.9 and 22.7 ∘C, respectively. Year 2013 was slightly colder, with an average value of 21.5 ∘C, while year 2014 was warmer, with an average value of 25.6 ∘C. The minimum temperatures of the study periods were similar for 2010 and 2013 (≈20 ∘C), slightly higher for 2011 (20.7 ∘C) and notably higher for 2014 (23.2 ∘C). The maximum temperatures ranged between 23.5 (2013) and 28.3 (2014) ∘C. Overall, 2013 can be considered as a cold year, 2014 as a hot year, and 2010 and 2011 as intermediate years. The temperature variation pattern between the years cannot be easily linked to the variations in b. Concerning pmax, even though the largest value of pmax is associated with the warmest year (2014), and the smallest value of pmax with the coldest year (2013), years 2010 and 2011 had different values of pmax despite having similar temperatures. Besides, pmax and b are expected to depend more strongly on PAR than on T. Conversely, r0 can be expected to be larger when temperatures are higher. This happened in 2011 and 2014, but not in 2010, which still had relatively high temperatures. Possible explanations are changes in the Q10 value or the influence of other environmental variables. We did not investigate further possible changes in the Q10 value, because we did not have an independent way to estimate it and because its range is narrow according to the literature (Reynolds, 1984; Raven and Geider, 1988; Davison, 1991). Concerning PAR, in the analysed periods the average values in the mixed layer ranged from 162 (2013) to 227 (2014) µmol(ph) m−2 s−1, being higher in 2010 and 2011 than in 2013, and notably higher in 2014 than in all the other years. Remarkably also in 2014, despite the high values of PAR, the communities did not show signs of photoinhibition (a PARmax value of 741 for the mixed layer corresponds to a surface value of ≈1900 µmol(ph) m−2 s−1, given the light extinction coefficient of the lake of 1.5). Higher average PAR values could be responsible for larger pmax values, as observed in 2011 and 2014, and partially in 2010. However, the average PAR values are very similar in 2010 and 2011, while pmax values are not. Still, the very low value of pmax in 2013 could be explained by the low PARave value. The variations in b between the years, though, cannot be linked to the changes in PAR: 2013 and 2014, despite having very different PAR values, had similar b values. The trend in r0 also cannot be associated with the trend in PAR between the years. From what is said so far, the changes in PAR and water temperature alone cannot fully account for the changes in the model parameters. The long-term variations in the parameters probably have other drivers too, such as the composition of the algal communities; as already stated, a more extensive analysis would require such information and is beyond the scope of this paper.
3.3 Model choice
In aquatic sciences, other models for describing the dependence of photosynthesis on irradiance are more commonly used than the Michaelis–Menten equation. The Michaelis–Menten equation was chosen in an effort of harmonising productivity studies between aquatic and forest sciences, in order to study the carbon cycle consistently in the forest–lake continuum. However, we checked whether other models provided a better fit to the data. We used the equations by Smith (1936) and by Jassby and Platt (1976). Even though they agreed well with the data, they did not perform significantly better than the Michaelis–Menten equation: the R2 and RMSE values of the fits were very similar. Hence, we decided to proceed with our first choice. The Smith (1936) and Jassby and Platt (1976) model equations and fit statistics are reported in the Supplement (Sect. S3 and Table S1).
3.4 Out-of-sample validation
The analysis we performed was based on an in-sample comparison, since our goal was to check whether our method to calculate the NEP was in agreement with the PI models typically used (Michaelis–Menten, Smith (1936) and Jassby and Platt (1976) equations). However, for the Michaelis–Menten model, we also ran an out-of-sample validation for each year, in order to further verify the correspondence between the calculated NEP and the model. For each year, we randomly selected half of the data points and used them for the fit to calculate the model parameters. Then, for the other half of the sample, we estimated the NEP using the equation and the parameters we had obtained, and compared it to the originally calculated NEP. We both evaluated the correlation coefficient r between the two NEPs (the one calculated from the data and the one calculated from the model trained on half of the data points, then discarded), and the RMSE of the validations. The results are reported in Table 2, and show that the two NEP values compared well. The correlation coefficient r varies between 0.84 and 0.92 and the RMSE varies between 0.15 and 0.31 µmol(CO2) m−2 s−1.
4.1 Assumptions and uncertainties
Firstly, it is important to notice that we are working under the assumption that the NEE, which is what can be measured, is equal in magnitude to the NEP. This concept is widely accepted in the scientific community (Aubinet et al., 2012), for forests as well as for other environments such as lakes. The assumption is indeed strictly valid only when there are no sources and sinks of CO2 that do not involve conversion to or from organic C (Lovett et al., 2006). Such sources and sinks, however, are usually negligible, except for oceans.
The lateral transport of CO2 had to be ruled out for the sake of the calculations. A similar challenge is encountered in forest ecology studies as well, where the lateral transport in the air (advection) is also usually neglected. We are of course fully aware of the lake being a 3-D dynamic system. Besides, since this study focuses on the summer periods when the lake was stably stratified and there were no high winds or rains, the lateral transport is not expected to play a significant role here. This assumption is supported by Dinsmore et al. (2013), who showed that for lake Kuivajärvi most of the CO2 discharge happens at snowmelt or during heavy rains in the autumn. It is also supported by the mixed layer CO2 concentration time series, which show no sign of a long-term trend on top of the diurnal cycles (see Figs. S5–S14 in the Supplement).
Regarding oligotrophic lakes, it has been suggested that diurnal patterns in the epilimnion stratification and water convective motions (causing nighttime upwelling of CO2) are important drivers of the diurnal variation in the surface water CO2 concentration (Åberg et al., 2010). Lake Kuivajärvi though is mesotrophic (Chl a concentration is 5–30 µg L−1 during summer) and the primary production can be assumed to be the main driver of the CO2 concentration, as observed also in some other lakes with high Chl a (Hanson et al., 2003; Huotari et al., 2009). Also, we implemented strict selection criteria for the analysed periods to minimise the effect of upwelling CO2: the thermistor data indicate that the winds, despite being weak, were strong enough to keep the top 1.5 m of the water column well mixed both day and night, without disrupting the thermocline. Thus, no sign of hypolimnetic upwelling was detected. Under these conditions, diurnal stratification patterns and convective motions had a minor impact on the mixed layer of our lake. It is also important to note that the photochemical production of CO2 is generally negligible in humic lakes (Jonsson et al., 2001); its maximum contribution to the flux for a lake with similar characteristics as the one in our study lake was < 4 % over the whole growing season, and was detectable only in the top 10 cm of the water column (Vähätalo et al., 2000; Ojala et al., 2011).
Table 2Fit statistics (R2 and RMSE) calculated for half of the sample, correlation coefficient r and validation RMSE using the other half of the sample. RMSE in µmol(CO2) m−2 s−1.
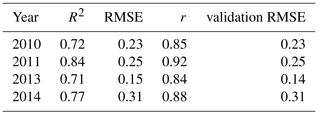
Our analysis was hindered by issues in the EC data set: due to inherent EC limitations and technical problems, the data set had many gaps and average daytime and nighttime Fa values had to be used. The relative uncertainty in them was, on average, 50 %. This uncertainty propagates to NEP through Eq. (3), and therefore to the parameter values as well. However, it does not undermine the good agreement between the model and the data, given that the average Fa values were calculated putting together all the periods of the same year. Therefore, each NEP data point has the same uncertainty and the same weight in the fit. The calculations could be improved with a better EC data set. Different methods could also be adopted to estimate the flux between the lake and the atmosphere. Chamber measurements could be used, but the time resolution could be an issue. They would need to be performed regularly. They could, however, be used to integrate the EC data set for example. Surface renewal models could also be used (Heiskanen et al., 2014). For further information on the comparison between different flux measurement methods, see Erkkilä et al. (2018).
4.2 Limitations and further development
In this study, we could not clearly link the environmental variables to the changes in the Michaelis–Menten model parameters, and more information on the algal communities living in the lake would have been required in order to expand the analysis. However, it is important to stress that the simplicity of this method lies in the fact that to estimate the parameters, which can then be used to calculate the productivity, information on the algal communities is not needed. It is needed only when widening the scope of the productivity studies: when, for example, the parameters themselves and their relationship with the environmental conditions or the specific phytoplankton communities are investigated. Knowledge on the algal communities would also help when extending the productivity calculation to the whole year. In our case, for example, the NEP rates and hence the parameters are representative of the late summer. In lake Kuivajärvi, where diatoms are abundant, it can be expected for the productivity to have a peak in the spring and another smaller peak in the autumn, at the turnover. More measurements at those times would be needed in order to understand whether the parameterisation is still valid under those conditions.
At the current stage, the method we present here is still very system specific, and assumptions about lateral and vertical CO2 exchange and photo-oxidation had to be made (negligible lateral exchange and photo-oxidation, no in-lake vertical exchange). However, the method can in principle be applied to any lake and under any condition, with an expansion of the instrumental set-up. Measurements or estimates of Fu, the CO2 flux from the deeper layer to the surface layer of the lake, would be needed in order to not limit the analysis to isothermal (as in Hari et al., 2008) or stable stratification (as here) conditions. This could be achieved for example by adding water column turbulence measurements to the CO2 concentration and temperature measurements. Chemical measurements would be needed to apply the method in clear-water lakes, where photo-oxidation could play an important role. Finally, information about CO2 discharge would be needed for lakes where or periods when lateral transport is not negligible.
The high-frequency direct CO2 concentration measurement method suggested in Hari et al. (2008) and tested only on 3 days of data under autumn turnover conditions was tested more extensively and under different conditions here, on a data set of 40 days of stable stratification typical of summer for dark-water lakes. The method proved to be suitable for lake productivity studies under isothermal (Hari et al., 2008) or stable stratification conditions: its high temporal resolution allowed us to calculate the net ecosystem productivity (NEP) at a temporal scale of minutes. A quantitative comparison between the NEP calculated with this method and the modelled NEP was also carried out for the first time, and it showed a very good agreement between the two, further validating the method. From that, we were able to accurately parameterise the net productivity as a function of the ambient variables, estimating the productivity parameters typical of the communities in the lake.
Overall, we believe that the method proposed in Hari et al. (2008) and further tested and developed here represents an improvement over the traditional approaches (bottle method and 14C technique), given its time resolution and the fact that it is a free-water approach. We also think that it is promising compared to the other more common free-water approach, the O2 method, since it is direct and the respiratory quotient is not needed. However, at the present stage it can be applied under a limited set of conditions (isothermal or stable stratification). Still, our study is an important step towards testing and developing the approach so that it becomes more general, also given the scarcity or even lack of high-frequency direct CO2 measurements for productivity studies (we are aware of only one other study where free-water CO2 measurements were used for metabolism studies; see Hanson et al., 2003). We are looking for further contributions by the research community and we think the method should be widely adopted, first in order to gather more information about its usability under different conditions and then also to have a broader network of productivity studies on lakes. This is all the more true given that the CO2 probes are also easy to set up and relatively inexpensive. The method requires at least a concomitant estimation of the CO2 flux from the lake to the atmosphere. In our case the EC technique was used, which is expensive and can be laborious in the data processing phase. However, chamber measurements or surface renewal models could be equally good options.
Additionally, the method also relies on equations that are typically adopted in terrestrial ecology studies for the calculation of the NEP, where high-frequency measurements are more commonplace than in aquatic research. Extensively applying the method would reduce the gap in the CO2 exchange measurements between aquatic and terrestrial ecology, which is beneficial in the framework of integrating research in different ecosystems, for which purpose a common language between different disciplines is needed. It would also help us achieve a better understanding of the biological processes behind the CO2 exchange. This, in turn, would expand our knowledge on the carbon cycle in the water, which is still limited, and would lead to a better integration of aquatic ecosystems in the local and global carbon budgets.
The data sets and the codes used in this paper can be obtained from the authors upon request.
The supplement related to this article is available online at: https://doi.org/10.5194/bg-15-2021-2018-supplement.
The authors declare that they have no conflict of interest.
We thank Jacob Zwart and another anonymous reviewer for their constructive
comments and suggestions, which helped improve the manuscript. This study was
funded by the University of Helsinki and the Finnish Cultural Foundation –
Häme fund (Hämeen rahasto – Suomen Kulttuurirahasto). Support also came
from the Academy of Finland, through the Academy Professor projects (1284701
and 1282842), the CarLAC project (281196), ICOS-Finland (1281255), the
Finnish Centre of Excellence in Atmospheric Science and from the EU project
GHG-LAKE (612642).
Edited by: David
Gillikin
Reviewed by: Jacob Zwart and one anonymous referee
Åberg, J., Jansson, M., and Jonsson, A.: Importance of water temperature and thermal stratification dynamics for temporal variation of surface water CO2 in a boreal lake, J. Geophys. Res., 115, G02024, https://doi.org/10.1029/2009JG001085, 2010. a
Aubinet, M., Vesala, T., and Papale, D.: Eddy Covariance A Practical Guide to Measurement and Data Analysis, Springer, Dordrecht, the Netherlands, 2012. a
Battin, T. J., Luyssaert, S., Kaplan, L. A., Aufdenkampe, A. K., Richter, A., and Tranvik, L. J.: The boundless carbon cycle, Nat. Geosci., 2, 598–600, 2009. a
Bender, M., Grande, K., Johnson, K., Marra, J., Williams, P. J., Sieburth, J., Pilson, M., Langdon, C., Hitchcock, G., Orchardo, J., Hunt, C., Donaghay, P., and Heinemann, K.: A comparison of four methods for determining planktonic community production, Limnol. Oceanogr., 32, 1085–1098, 1987. a
Berggren, M., Lapierre, J. F., and del Giorgio, P. A.: Magnitude and regulation of bacterioplankton respiratory quotient across freshwater environmental gradients, ISME J., 6, 984–993, 2012. a
Caperon, J.: Population growth in micro-organisms limited by food supply, Ecology, 48, 715–722, 1967. a
Carignan, R.: Automated determination of carbon dioxide oxygen and nitrogen partial pressures in surface waters, Limnol. Oceanaogr., 43, 969–975, 1998. a
Carignan, R., Planas, D., and Vis, C.: Planktonic production and respiration in oligotrophic Shield lakes, Limnol. Oceanogr., 45, 189–199, 2000. a
Cole, J. J. and Caraco, N. F.: Atmospheric exchange of carbon dioxide in a low-wind oligotrophic lake measured by the addition of SF6, Limnol. Oceanogr., 43, 647–656, 1998. a
Cole, J. J., Caraco, N. F., Kling, G. W., and Kratz, T. K.: Carbon dioxide supersaturation in the surface waters of lakes, Science-AAAS-Weekly Paper Edition, 265, 1568–1569, 1994. a, b
Cole, J. J., Prairie, Y. T., Caraco, N. F., McDowell, W. H., Tranvik, L. J., Striegl, R. G., Duarte, C. M., Kortelainen, P., Downing, J. A., Middelburg, J. J., and Melack, J.: Plumbing the global carbon cycle: Integrating inland waters into the terrestrial carbon budget, Ecosystems, 10, 171–184, 2007. a
Davison, I. R.: Environmental effects on algal photosynthesis: temperature, J. Phycol., 27, 2–8, 1991. a, b
Dinsmore, K. J., Wallin, M. B., Johnson, M. S., Billett, M. F., Bishop, K., Pumpanen, J., and Ojala, A.: Contrasting CO2 concentration discharge dynamics in headwater streams: A multi-catchment comparison, J. Geophys. Res.-Biogeo., 118, 445–461, 2013. a
Erkkilä, K.-M., Ojala, A., Bastviken, D., Biermann, T., Heiskanen, J. J., Lindroth, A., Peltola, O., Rantakari, M., Vesala, T., and Mammarella, I.: Methane and carbon dioxide fluxes over a lake: comparison between eddy covariance, floating chambers and boundary layer method, Biogeosciences, 15, 429–445, https://doi.org/10.5194/bg-15-429-2018, 2018. a
Foken, T., Aubinet, M., and Leuning, R.: The eddy covariance method, in: Eddy Covariance: A Practical Guide to Measurement and Data Analysis, edited by: Aubinet, M., Vesala, T., and Papale, D., 1–19, Springer, Dordrecht, the Netherlands, 2012. a
Gaarder, T. and Gran, H. H.: Investigations of the production of plankton in the Oslo Fjord, Rapp Proc Verb Conseil permanent international pour l'exploration de la mer, 42, 1–48, 1927. a
Hanson, P., Bade, D., Carpenter, S., and Kratz, T.: Lake metabolism: Relationships with dissolved organic carbon and phosphorus, Limnol. Oceanogr., 48, 1112–1119, 2003. a, b
Hari, P., Pumpanen, J., Huotari, J., Kolari, P., Grace, J., Vesala, T., and Ojala, A.: High frequency measurements of productivity of planktonic algae using rugged nondispersive infrared carbon dioxide probes, Limnol. Oceanogr.-Meth., 6, 347–354, 2008. a, b, c, d, e, f, g, h, i
Heiskanen, J., Mammarella, I., Haapanala, S., Pumpanen, J., Vesala, T., MacIntyre, S., and Ojala, A.: Effects of cooling and internal wave motions on gas transfer coefficients in a boreal lake, Tellus B, 66, 22827, https://doi.org/10.3402/tellusb.v66.22827, 2014. a, b, c
Heiskanen, J., Mammarella, I., Ojala, A., Stepanenko, V., Erkkilä, K. M., Miettinen, H., Sandström, H., Eugster, W., Leppäranta, M., Järvinen, H., Vesala, T., and Nordbo, A.: Effects of water clarity on lake stratification and lake-atmosphere heat exchange, J. Geophys. Res.-Atmos., 120, 7412–7428, 2015. a, b
Hoellein, T. J., Bruesewitz, D. A., and Richardson, D. C.: Revisiting Odum (1956): a synthesis of aquatic ecosystem metabolism, Limnol. Oceanogr., 58, 2089–2100, 2013. a
Howarth, R. W. and Michaels, A. F.: The measurement of primary production in aquatic ecosystems, 72–85, in: Methods in Ecosystems Science, edited by: Sala, O. E., Jackson, R. B., Mooney, H. A., and Howarth, R. W., Springer, New York, 2000. a
Huotari, J., Ojala, A., Peltomaa, E., Pumpanen, J., Hari, P., and Vesala, T.: Temporal variations in surface water CO2 concentrations in a boreal humic lake based on high-frequency measurements, Boreal Environ. Res., 14, 48–60, 2009. a
Jassby, A. D. and Platt, T.: Mathematical formulation of the relationship between photosynthesis and light for phytoplankton, Limnol. Oceanogr., 21, 540–547, 1976. a, b, c
Johnson, M. S., Billett, M. F., Dinsmore, K. J., Wallin, M., Dyson, K. E., and Jassal, R. S.: Direct and continuous measurement of dissolved carbon dioxide in freshwater aquatic systems – method and applications, Ecohydrology, 3, 68–78, 2010. a
Jonsson, A., Meili, M., Bergström, A.-K., and Jansson, M.: Whole-lake mineralization of allochthonous and autochthonous organic carbon in a large humic lake (Örträsket, N. Sweden), Limnol. Oceanogr., 46, 1691–1700, 2001. a
Karl, D. M., Laws, E. A., Morris, P., Williams, P. J., and Emerson, S.: Metabolic balance of the open sea, Nature, 426, 32, https://doi.org/10.1038/426032a, 2003. a
Kiefer, D. A. and Mitchell, B. G.: A simple steady state description of phytoplankton growth based on absorption cross section and quantum efficiency, Limnol. Oceanogr., 28, 770–776, 1983. a
Lovett, G. M., Cole, J. J., and Pace, M. L.: Is net ecosystem production equal to ecosystem carbon accumulation?, Ecosystems, 9.1, 152–155, 2006. a
Mallin, M. A. and Paerl, H. W.: Effects of variable irradiance on phytoplankton productivity in shallow estuaries, Limnol. Oceanogr., 37, 54–62, 1992. a
Mammarella, I., Launiainen, S., Grönholm, T., Keronen, P., Pumpanen, J., Rannik, Ü., and Vesala, T.: Relative humidity effect on the high-frequency attenuation of water vapor flux measured by a closed-path eddy covariance system, J. Atmos. Ocean. Tech., 26, 1856–1866, 2009. a
Mammarella, I., Nordbo, A., Rannik, Ü., Haapanala, S., Levula, J., Laakso, H., Ojala, A., Peltola, O., Heiskanen, J., Pumpanen, J., and Vesala, T.: Carbon dioxide and energy fluxes over a small boreal lake in Southern Finland, J. Geophys. Res.-Biogeo., 120, 1296–1314, 2015. a, b
Miettinen, H., Pumpanen, J., Heiskanen, J. J., Aaltonen, H., Mammarella, I., Ojala, A., Levula, J., and Rantakari, M.: Towards a more comprehensive understanding of lacustrine greenhouse gas dynamics – two-year measurements of concentrations and fluxes of CO2 CH4 and N2O in a typical boreal lake surrounded by managed forests, Boreal Environ. Res., 20, 75–89, 2015. a
Ojala, A., Lopez Bellido, J., Tulonen, T., Kankaala, P., and Huotari, J.: Carbon gas fluxes from a brown-water and a clear-water lake in the boreal zone during a summer with extreme rain events, Limnol. Oceanogr., 56, 61–76, 2011. a
Pace, M. L. and Prairie, Y. T.: Respiration in lakes, in: Respiration in Aquatic Ecosystems, edited by: del Giorgio, P. A. and Williams, P. J. leB., Oxford Univ. Press, New York, 103–121, 2005. a
Peterson, B. J.: Aquatic primary productivity and the 14C-CO2 method: a history of the productivity problem, Annu. Rev. Ecol. Syst., 11, 359–385, 1980. a
Raven, J. A. and Geider, R. J.: Temperature and algal growth, New Phytol., 11, 441–461, 1988. a, b
Raymond, P. A., Hartmann, J., Lauerwald, R., Sobek, S., McDonald, C., Hoover, M., Butman, D., Striegl, R., Mayorga, E., Humborg, C., Kortelainen, P., Dürr, H., Meybeck, M., Ciais, P., and Guth, P.: Global carbon dioxide emissions from inland waters, Nature, 503, 355–359, 2013. a
Read, J. S., Hamilton, D. P., Jones, I. D., Muraoka, K., Winslow, L. A., Kroiss, R., Wu, C. H., and Gaiser, E.: Derivation of lake mixing and stratification indices from high-resolution lake buoy data, Environ. Model. Softw., 26, 1325–1336, 2011. a
Reynolds, C. S.: The Ecology of Freshwater Phytoplankton, Cambridge Univ. Press, Cambridge, 1984. a, b
Reynolds, C. S.: The Ecology of Phytoplankton, Cambridge Univ. Press, Cambridge, 2006. a
Smith, E. L.: Photosynthesis in relation to light and carbon dioxide, P. Natl. Acad. Sci. USA, 22, 504–511, 1936. a, b, c
Sobek, S., Algsten, G., Bergström, A.-K., Jansson, M., and Tranvik, L. J.: The catchment and climate regulation of pCO2 in boreal lakes, Glob. Change Biol., 9, 630–641, 2003. a, b
Solomon, C. T., Bruesewitz, D. A., Richardson, D. C., Rose, K. C., Van de Bogert, M. C., Hanson, P. C., Kratz, T. K., Larget, B., Adrian, R., Babin, B. L., Chiu, C. Y., Hamilton, D. P., Gaiser, E. E., Hendricks, S., Istvanovics, V., Laas, A., O'Donnell, D. M., Pace, M. L., Ryder, E., Staehr, P. A., Torgersen, T., Vanni, M. J., Weathers, K. C., and Zhu, G.: Ecosystem respiration: Drivers of daily variability and background respiration in lakes around the globe, Limnol. Oceanogr., 58, 849–866, 2013. a
Søndergaard, M.: A biography of Einar Steeman Nielsen: the man and his science, in: Phytoplankton Productivity: Carbon Assimilation in Marine and Freshwater Ecosystems, edited by: Williams, P. J., Thomas, D. N., and Reynolds, C. S., Blackwell Science, Oxford, 1–15, 2002. a
Staehr, P. A., Bade, D., Van de Bogert, M. C., Koch, G. R., Williamson, C., Hanson, P., Cole, J. J., and Kratz, T.: Lake metabolism and the diel oxygen technique: state of the science, Limnol. Oceanogr.-Meth., 8, 628–644, 2010. a, b, c
Steemann Nielsen, E.: Measurement of the production of organic matter in the sea by means of carbon-14, Nature, 167, 684–685, 1951. a
Tranvik, L. J., Downing, J. A., Cotner, J. B., Loiselle, S. A., Striegl, R. G., Ballatore, T. J., Dillon, P., Finlay, K., Fortino, K., Knoll, L. B., Kortelainen, P., Kutser, T., Larsen, S., Laurion, I., Leech, D. M., McCallister, S. L., McKnight, D. M., Melack, J. M., Overholt, E., Porter, J. A., Prairie, Y., Renwick, W. H., Roland, F., Sherman, B. S., Schindler , D. W., Sobek, S., Tremblay, A., Vanni, M. J., Verschoor, A. M., von Wachenfeldt, E., and Weyhenmeyer, G. A.: Lakes and reservoirs as regulators of carbon cycling and climate, Limnol. Oceanogr., 54, 2298–2314, 2009. a
Vähätalo, A. V., Salkinoja-Salonen, M., Taalas, P., and Salonen, K.: Spectrum of the quantum yield for photochemical mineralization of dissolved organic carbon in a humic lake, Limnol. Oceanogr., 45, 664–676, 2000. a
Vesala, T., Huotari, J., Rannik, Ü., Suni, T., Smolander, S., Sogachev, A., Launiainen, S., and Ojala, A.: Eddy covariance measurements of carbon exchange and latent and sensible heat fluxes over a boreal lake for a full open-water period, J. Geophys. Res., 111, D11101, https://doi.org/10.1029/2005JD006365, 2006. a