the Creative Commons Attribution 4.0 License.
the Creative Commons Attribution 4.0 License.
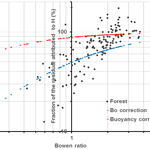
Footprint-weighted tile approach for a spruce forest and a nearby patchy clearing using the ACASA model
Kathrin Gatzsche
Wolfgang Babel
Eva Falge
Rex David Pyles
Kyaw Tha Paw U
Armin Raabe
Thomas Foken
The ACASA (Advanced Canopy–Atmosphere–Soil Algorithm) model, with a higher-order closure for tall vegetation, has already been successfully tested and validated for homogeneous spruce forests. The aim of this paper is to test the model using a footprint-weighted tile approach for a clearing with a heterogeneous structure of the underlying surface. The comparison with flux data shows a good agreement with a footprint-aggregated tile approach of the model. However, the results of a comparison with a tile approach on the basis of the mean land use classification of the clearing is not significantly different. It is assumed that the footprint model is not accurate enough to separate small-scale heterogeneities. All measured fluxes are corrected by forcing the energy balance closure of the test data either by maintaining the measured Bowen ratio or by the attribution of the residual depending on the fractions of sensible and latent heat flux to the buoyancy flux. The comparison with the model, in which the energy balance is closed, shows that the buoyancy correction for Bowen ratios >1.5 better fits the measured data. For lower Bowen ratios, the correction probably lies between the two methods, but the amount of available data was too small to make a conclusion. With an assumption of similarity between water and carbon dioxide fluxes, no correction of the net ecosystem exchange is necessary for Bowen ratios >1.5.
The comparison of modeled and measured energy and matter fluxes in a heterogeneous landscape is still a challenge. The fluxes measured with the eddy covariance technique are related to all surfaces on the upwind side of the measurements, and the influence of each surface on the measured data is given by the footprint function. The comparison of these flux measurements with one-dimensional models can be done over a homogeneous surface easily or over a heterogeneous surface if the model is parameterized for all surface characteristics and averaged according to the percentage of each surface in the footprint (tile approach; Mölders, 2012). The footprint varies with changing wind velocity and atmospheric stratification for each half-hourly-derived flux, and consequently the tile approach must also be modified every half hour. This approach was proposed by Foken and Leclerc (2004) and successfully improved by Göckede et al. (2005) and Biermann et al. (2014) for two separated surfaces. The tile approach is based on the application of the eddy covariance method with the correction of the storage term, which ignores any advection. The more appropriate approach would use the generalized eddy covariance method (Foken et al., 2012), which includes the advection of matter and fluxes. Unfortunately, matter advection could only be analyzed experimentally under very idealized conditions up to now. Experimental advection studies with an acceptable number of measurement points have failed (Aubinet et al., 2010, 2012). Furthermore, the resolution of available 3-D models (Sogachev et al., 2002; Sogachev and Lloyd, 2004) is too large (25–50 m) for the small-scale heterogeneities on the clearing (5–10 m). For our case, an experimental advection experiment cannot be realized and only an LES process study could be applied for a single 1 h case (Kanani-Sühring and Raasch, 2017). Therefore, the one-dimensional model with a tile approach according to the footprint of each eddy covariance measurement is the only realistic approach.
A further problem is the so-called unclosed energy balance. The energy balance with turbulence measurements (eddy covariance method; Aubinet et al., 2012) is not closed by an amount of up to 30 % according to the overview paper by Foken (2008), while the models close the energy balance by definition. The main reason for this is most likely the presence of fluxes caused by larger turbulence structures like secondary circulations (Foken, 2008). Measurement data must be corrected for energy balance closure before comparison with model outputs can be made. The missing flux will be distributed between the sensible and the latent heat flux according to the Bowen ratio (Twine et al., 2000) or the buoyancy flux (Charuchittipan et al., 2014). Carbon and other trace gas fluxes have not been corrected before the present study.
Simulating the turbulent transfer for heterogeneous landscapes utilizing a one-dimensional SVAT model (soil–vegetation–atmosphere transfer) represents a multifaceted challenge. For forest, coherent structures are a typical phenomenon of turbulent exchange (Gao et al., 1989; Bergström and Högström, 1989), and they can be measured with the eddy covariance technique. However, coherent structures modify the turbulent transfer of energy and matter in a manner that complicates capturing them with models. Higher-order closure models are able to overcome this problem (Deardorff, 1966). In this study the Advanced Canopy–Atmosphere–Soil Algorithm (ACASA; Pyles et al., 2000) has been utilized with a third-order closure scheme (Meyers and Paw U, 1986), and a more exact resolution of the turbulent structure for forest is provided. Thus, the ACASA model is much better suited to handling coherent structures and counter gradients than classical SVAT models with a first-order closure (Staudt et al., 2011). Falge et al. (2017) provide a summary of the results of all model studies made in the last nearly 20 years at the Waldstein–Weidenbrunnen site, without any discussion of specific problems.
This paper is based on the earlier study by Falge et al. (2017), in which the ACASA model was insufficiently applied for the clearing and compared with eddy covariance data. As a first guess, the tile approach for the clearing was derived from the relative percentages of the individual land cover classes of the whole clearing without any selection according to the footprint. Furthermore, the measured fluxes were corrected for the energy balance closure. The present paper extends and completes the first paper in the following directions: (i) For the clearing, a more precise concept of the comparison of flux measurements with 1-D modeling using a tile approach according to the half-hourly footprints. (ii) for the forest, a detailed discussion of the two different energy balance correction methods is provided. The inclusion of both surfaces in this study is necessary because flux studies often use MODIS (Moderate-Resolution Imaging Spectroradiometer) data with a grid size of 500 or 250 m. Since the windthrow in 2007, the satellite sees about 50 % forest and 50 % clearing in the relevant grid.
2.1 Waldstein–Weidenbrunnen site
The experimental data for the initialization of the model and the evaluation of its outputs were collected during the third intensive observation period (IOP3) of the EGER (ExchanGE processes in mountainous Regions) project (Foken et al., 2012). The IOP3 campaign took place from 13 June to 26 July 2011. The EGER project mainly aimed at capturing the relevant exchange processes within the soil–vegetation–atmosphere framework and their interactions at different scales, and the work in IOP3 thereby focused on the role of surface heterogeneities in atmospheric exchange and chemistry as well as biogeochemistry.
The experimental site (50∘8′ N, 11∘52′ E, about 775 m a.s.l.) is part of the Bayreuth Centre of Ecology and Environmental Research (BayCEER) and lies in the upper part of the Lehstenbach catchment (Fig. 1a). It is situated between two hilltops: to the southwest the Großer Waldstein (879 m a.s.l.) and the Bergkopf (857 m a.s.l.) to the northeast. The Lehstenbach catchment is part of the slopes of the Waldstein in the northwestern part of the Fichtelgebirge, a low mountain range in northeastern Bavaria, Germany and is mostly densely forested.
The measurements of IOP3 were carried out in a spruce forest next to the FLUXNET site Waldstein–Weidenbrunnen (DE-Bay) and in a nearby clearing with heterogeneous low vegetation located to the south of the FLUXNET site (Fig. 1b). The clearing was created by a windthrow on 18 January 2007 that was induced by the European windstorm Kyrill (Foken et al., 2012). The vegetation of the measurement site is heterogeneous and there is a slope of 3∘ from the pine forest to the clearing (from north to south). A more detailed description of the surrounding topography is provided by Foken et al. (2017a).
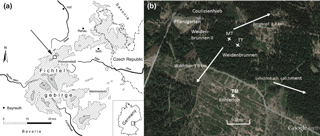
Figure 1(a) Location of the BayCEER research site at the Waldstein hillsides in the Fichtelgebirge (modified from Gerstberger et al., 2004), published with the kind permission of Springer, Berlin, Heidelberg, 2004. (b) Aerial picture of the patchy landscape showing meteorological towers and masts as well as the measuring points that are important for this study. MT: main tower, TT: turbulence tower, TM: turbulence mast; modified from Foken et al. (2017a) and published with the kind permission of Springer, Berlin, Heidelberg, 2017, and Google Earth, 2015.
The forest consists mainly of Norway spruce (Picea abies) with a stand height of about 27 m and a leaf area index (LAI) of 4.8 m2 m−2 (Foken et al., 2017a). The stand age is circa 60 years (estimate from 2013) and the forest structure is characterized by an open trunk space and a dense crown space. Most needles are located between 0.5 and 0.9 , where z is the measurement height and hc the stand height of 27 m. In the ground area, the understory comprises two-thirds crinkled hair grass (Deschampsia flexuosa) and moss (together LAI ≤0.5 m2 m−2), and one-third is characterized by blueberry (Vaccinium myrtillus) and young Norway spruce (Picea abies, together plant area index (PAI) of 3.5 m2 m−2). For more details, see Foken et al. (2012). The information regarding the overstory trees is summarized in Table 1. The vegetation of the clearing is a conglomeration of young spruce trees, blueberry (Vaccinium myrtillus), and different grasses (e.g., Calamagrostis, Agrostis, Poaceae). However, deadwood and bare soil are also elements of the clearing, and together they make up one-third of the ground cover of this area. The clearing also contains approximately 2 % young deciduous trees: alder (Alnus), maple (Acer), beech (Fagus), and sorbus (Sorbus), with a greater canopy height than the remaining species. Due to the heterogeneous vegetation at the clearing, no distinct mean canopy height can be estimated for the clearing. Table 1 provides an overview of the species present and their mean canopy height.
Table 1Vegetation at the forest and the Köhlerloh clearing with their main characteristics according to Foken et al. (2017a).
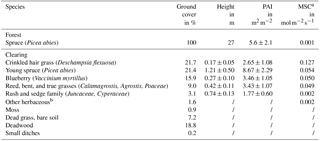
a MSC stands for minimal stomata conductance and is a model parameter utilized in ACASA.
b This includes Digitalis purpurea (common foxglove), Epilobium angustifolium (willow herb), and Urtica dioica (stinging nettle).
2.2 Experimental setup and data
During IOP3, high-frequency turbulence measurements were conducted at different measurement towers (Fig. 1b). For this study, the data from the towers in the forest (MT, TT) and the turbulence mast (TM) at the clearing have been especially utilized. The towers and masts were generally equipped with 3-D sonic anemometers to enable the collection of information about wind components (u, v, w) and the sonic temperature (TS). High-frequency gas analyzers for carbon dioxide () and water vapor (q) were installed in conjunction with sonic anemometers. This allowed the turbulent exchange for forest and clearing to be investigated with the devices as summarized in Table 2, and this has been utilized for comparison with simulations. Raw flux data (20 Hz) have been processed with the TK3 software (Mauder and Foken, 2015). The flux data have been additionally classified by their quality according to the classification scheme by Foken et al. (2004). For comparison with model results, only data with quality flags of 6 or better have been utilized. Furthermore, the flux measurements have been energy balance corrected with the methods described in Sect. 2.4.
The ACASA model needs half-hourly meteorological input values as well as mean values of soil temperature and soil moisture for initialization. These input values (see Table 2) are provided by standard measurements at the Pflanzgarten (Fig. 1b) and measurements at the main tower (MT) for the spruce forest as and at the turbulence mast (TM) for the clearing. Table 2 provides an overview of the meteorological input data for the two simulated sites and the corresponding instrumentation.
Three golden day periods (GDPs) were selected within IOP3 because of fair weather conditions and predominantly good performance of the measurement devices. The first GDP was from 26 to 29 June 2011 (corresponding to the 177th to 180th day of the year, DOY). On the last 3 days of this period, clear-sky conditions prevailed until 14:00 as did moderate westerly (26 and 27 June) or easterly (28 and 29 June) winds. The second GDP occurred from 4 to 8 July 2011 (DOY: 185 to 189), with the best weather conditions on 6 and 7 July and partly overcast sky on the rest of the days. The wind in this period was weak and blew from the west (4, 7, and 8 July) or east (5 and 6 July). Minor amounts of precipitation were measured on 4 and 8 July. For the last GDP from 14 to 17 July 2011 (DOY: 195 to 198) data are missing for the clearing at 5.5 m.
Table 2Meteorological instrumentation for the determination of the sensible and the latent heat flux as well as the net ecosystem exchange (NEE) for forest and clearing at different measurement towers in distinct heights, using the eddy covariance technique (with the frequency of 20 Hz applied as indicated), and additionally, the most important input parameter of the model (for this purpose the mean CO2 concentrations have been averaged from the turbulence data). Documentation of the complete data set is available in Foken (2017a).
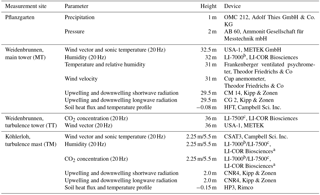
a LI-7000 at 2.25 m and LI-7500 at 5.5 m.
b Closed-path gas analyzer, c open-path gas analyzer.
2.3 The ACASA model
ACASA (Pyles et al., 2000) was utilized to simulate the turbulent transfer of heat, water vapor, and CO2 for spruce forest and clearing. ACASA is a multilayer SVAT model developed at the University of California, Davis. The main feature of ACASA is that the turbulent transfer within and above the canopy is calculated by a diabatic, third-order closure method, which is based on the theoretical work of Meyers and Paw U (1986, 1987). The multilayer structure of ACASA is represented by 20 evenly distributed atmospheric layers, which reach from trunk space to twice the canopy height, and by 15 soil layers. For the calculation of leaf, stem, and soil surface temperatures, the fourth-order polynomial scheme of Paw U and Gao (1988) is incorporated. Utilizing this polynomial allows for the calculation of the temperatures of these components without making substantial errors in the case of significant deviations from the ambient temperature. The model was considerably updated by the University of California, Davis (Pyles et al., 2000, 2003) and is still in use (Falk et al., 2014). For this study, the ACASA model was adapted from a version of April 2013.
Direct and diffuse radiation can be absorbed, transmitted, or reflected by the canopy, whereby these processes are dependent on the leaf and branch distribution of the plants. For this purpose, the aboveground biomass is distributed in 10 different leaf angle classes (Meyers, 1985). In order to enable a preferably exact allocation of the energy to sensible and latent heat fluxes, a leaf energy balance equation has to be solved. Plant physiological feedback to micro-environmental conditions is incorporated by the approaches of Leuning (1990) and Collatz et al. (1991) to the Ball–Berry stomatal conductance combined with photosynthetic rates calculated after an equation of Farquhar and Caemmerer (1982), whereby coupling follows the standards of Su and Paw U (1996). ACASA also includes canopy heat storage, which is mainly important for tall vegetation, and canopy interception of precipitation. For thermal and hydrological aspects the soil model is based on the one-dimensional diffusion equation (de Vries, 1952) as incorporated in MAPS Smirnova et al. (Mesoscale Analysis and Prediction System; 1997, 2000).
About 10 years ago the University of Bayreuth started to work with ACASA. The first issue was to use a sensitivity analysis to check whether the model could be applied for a Central European spruce forest (Staudt et al., 2010). We also found some model-specific problems, and the model was again updated by the University of California, Davis. Using the updated model, a first study for the Waldstein–Weidenbrunnen site was published (Staudt et al., 2011). This study showed that the ACASA model is more accurate (in comparison to a K-approach model) when coherent structures dominate at night, which has been investigated in many experiments and summarized by Thomas et al. (2017). The model was changed by Staudt et al. (2011) for the spruce-specific parameterizations in three parts. The first change relates to the soil respiration calculations and had already been examined by Staudt et al. (2010) for spruce. The second change was made in the calculation of photosynthesis according to Falge et al. (1996) because temperatures less than 10 ∘C are very common at the experimental site. The third adjustment relates to the day respiration for leaves because Tcherkez et al. (2008) quantified the day respiration as about one-half of the night respiration rate. Furthermore, Falge et al. (2017) determined several parameters of the plants of the clearing (see Table 16.1 of Falge et al., 2017).
2.4 Energy balance correction
The eddy covariance data on sensible heat, latent heat, and carbon dioxide flux have been energy balance corrected for comparison with the simulations.
For the correction of the energy fluxes, the residual (Res) arises from the following equation:
where QS denotes net radiation, H sensible heat flux, LE latent heat flux, G the ground flux heat, and ΔS the heat storage term of the aboveground biomass, which was determined according to the investigations of Haverd et al. (2007) and Lindroth et al. (2010). Here, the heat storage term was only estimated for the spruce forest because of its larger biomass compared to the vegetation in the clearing. The heat storage was calculated with the following relation:
with mf the aboveground biomass of the forest, cf the specific heat capacity of wood (cf=1702.8 ), and Ta the air temperature in the spruce canopy. In this study, two different methods for energy balance correction have been applied to the measurements of IOP3. The first correction method is introduced by Twine et al. (2000) and preserves the Bowen ratio. This method is usually utilized for the correction of heat fluxes under the assumption of measuring errors, but requires their scalar similarity. Henceforth, this method is abbreviated as EBC-Bo. The second correction method accounts for near-surface secondary circulations, which transport more sensible heat. Therefore, the alternative correction method (EBC-HB) proposed by Charuchittipan et al. (2014) utilizes the buoyancy flux ratio. Here, for the typical range of the Bowen ratio, more residual energy is ascribed to the sensible heat flux, as was done in several studies (Foken et al., 2011). The difference to the EBC-Bo correction is especially pronounced for low Bowen ratios. At night, the applicability of the corrections is limited due to the occurrence of minor or negative heat fluxes.
The discrepancy between measured and simulated NEE can be an effect of the unclosed energy balance on the CO2 fluxes. Therefore, we propose a new correction method on the basis of the good scalar similarity between the humidity and the carbon dioxide concentration mainly in the first part of the day (for scalar similarity see Ruppert et al., 2006), while the similarity between the temperature and other scalars often fails (Pearson et al., 1998). The measured NEE is corrected by referring to the energy-balance-corrected latent heat flux, which is introduced in Falge et al. (2017). For this purpose, the ratio k between the corrected and the uncorrected latent heat fluxes is calculated for both correction methods:
The measured NEE value was then multiplied by the respective correction factor kBo or kHB. This correction method is proposed for the NEE for the first time and the applicability of this approach is therefore evaluated. The correction is not very large. Therefore, until shown otherwise, the energy balance correction can be neglected for scalar fluxes.
2.5 Footprint model
Footprint models are currently a commonly used tool for the identification of the source area of flux measurements (Leclerc and Foken, 2014). For the footprint calculations of this study, a Lagrangian footprint model is applied (Rannik et al., 2000, 2003), whereby a spectral method for the nonlinear flux averaging of surface characteristics (friction, roughness length) according to Hasager and Jensen (1999) is employed (Göckede et al., 2006) because the linear averaging of the roughness length (input parameter of footprint models) is inaccurate. The sensitivity of the Lagrangian footprint model to the turbulence statistics was tested by Göckede et al. (2007) for the Waldstein–Weidenbrunnen site. A footprint climatology and footprint studies, including the effect of the footprint on the data quality, are available from Foken et al. (2017b). On average, more than 80 % of the target area is forest. Only for southerly winds and stable stratification does the Köhlerloh clearing have a significant influence on the fluxes measured at the main tower (MT).
In a study by Reithmaier et al. (2006) the land use for the Waldstein–Weidenbrunnen site was determined and mapped, and this was tested for footprint applications. Due to the distinct small-scale heterogeneities, the land cover map applied here has a high resolution (4.5×4.5 m2). This grid size is in agreement with the size of the typical heterogeneities and the recommendations for footprint analyses for low measuring heights (Leclerc and Foken, 2014). The calculated footprints for 30 min time periods have been used to generate tile approaches of the model.
3.1 Footprint climatology for the Köhlerloh clearing
The footprint climatology of the mainly forested Waldstein–Weidenbrunnen site is well described in many publications like Göckede et al. (2008) and summarized by Foken et al. (2017b). In the following, the footprint climatology of the clearing site Köhlerloh (turbulence mast, TM) is analyzed. Only for southerly winds and stable stratification does the Köhlerloh clearing have a significant influence on the fluxes measured at the main tower (MT). Therefore, only the footprint of the Köhlerloh site (turbulence mast, TM) is analyzed in the following.
As an overview, the footprint climatology for the period from 26 June to 17 July 2011 has been calculated and is a superposition of all individual footprints. Figure 2 shows the footprint climatology for the turbulence mast (TM) in two different heights at the clearing. The main difference in the footprint climatology between the different stratifications appears for stable conditions at both measuring heights. For unstable and neutral stratification, mainly the clearing contributes to the turbulent fluxes at the TM. For stable stratification and northerly wind directions, the spruce forest has an additional influence on the measured turbulent flux at the TM (see Fig. 2). For a 5.5 m measuring height, the footprint source areas are generally enhanced and/or extended. Regarding the stable stratification case, the northwesterly part of the spruce forest is only included in the outer source region of the turbulent flux at a 2.25 m measuring height and is more pronounced for a 5.5 m measuring height. Additionally, a slight influence from the northeasterly spruce areas connected to the forest edge is calculated for a 5.5 m measuring height.
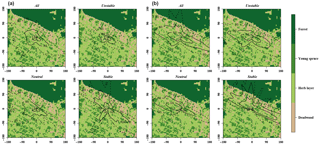
Figure 2Footprint climatology of the Köhlerloh clearing (26 June to 17 July 2011) at the turbulence mast (TM) for the three stability classes and the combination of all classes; (a) left four panels at a 2.25 m measuring height; (b) right four panels at a 5.5 m measuring height. Here, four different land use classes are considered, with the herb layer being composed of Deschampsia, Calamagrostis, Juncus, and Vaccinium. Distances are given in meters.
3.2 Comparison of modeled and measured fluxes
3.2.1 Daily cycles
Falge et al. (2017) have already compared the sensible and the latent heat flux as well as the NEE for the forest (MT) and the clearing (TM, 2.25 m). They found that the sensible heat flux corrected with the buoyancy flux (EBC-HB) agrees better with the modeled data than the flux corrected with the Bowen ratio (EBC-Bo) for the first golden day period. The result for the latent heat flux is similar and the NEE shows no significant differences. The NEE over the clearing was only about 50 % of the value over the forest.
In the following, we compare for the clearing the measured and the energy-balance-corrected fluxes with the tile approach of the model according to (i) the relevant footprint near the measurement point and (ii) the land cover distribution of the whole clearing. It was found that for the footprint of a 2.25 m height there are no significant differences between the modeling approaches due to the small size of the footprint (not shown). The results for TM with the footprint for a 5.5 m measuring height are shown in Fig. 3 for the first and in Fig. 4 for the second golden day period. The difference between the two model approaches is more significant in the first period, which had a Bowen ratio Bo≥1, while in the second period the Bowen ratio was often below 1. The differences in the land use characteristics were more dominant under drier conditions.
From the visual comparison of Figs. 3 and 4 it has been found that the buoyancy-corrected sensible and latent heat fluxes agree better with the model than the Bowen-ratio-corrected fluxes. The scatter is too large to allow any trend for the NEE to be seen. There is also no clear finding as to whether the footprint-weighted tile approach of the model (non-weighted approach not shown) gives better results. The integrated fluxes of the tile approaches for the whole clearing and the measured fluxes within the footprint of the turbulence mast for 5.5 m of height do not differ significantly, except for stable atmospheric stratification (Fig. 2). The land cover characteristics and soil water content probably have a significant influence. This can be seen by the high measured latent heat fluxes in the first golden day period (see Fig. 3). Regression analyses are discussed in the following section for both weighting methods.
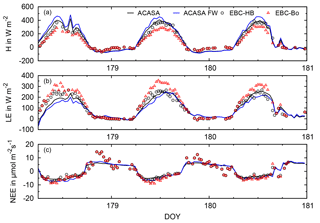
Figure 3Comparison of ACASA model simulations with measured turbulent fluxes at the TM (5.5 m measurement height) for the first GDP; (a) sensible heat (H), (b) latent heat (LE), and (c) net ecosystem exchange (NEE). The black solid line displays ACASA simulations weighted by the mean plant distribution of the whole clearing and the blue solid line indicates ACASA simulations weighted by different plant classes within the footprint of the flux measurements. Energy-balance-corrected flux measurements are shown by black circles for the correction utilizing the buoyancy flux ratio (EBC-HB) and red triangles for correction with the Bowen ratio (EBC-Bo).
3.2.2 Regression analyses
Neither the modeled nor the measured fluxes are free of errors and could be used as an independent parameter. Therefore, the errors are assumed to be similar and an orthogonal regression analysis for evenly distributed errors has been applied (Dunn, 2004). The regression has been done for the first two golden day periods for the forest and the clearing (with and without the footprint-weighted tile approach) for the sensible (H) and the latent (LE) heat flux as well as the net ecosystem exchange (NEE). Furthermore, the available energy (AE), as the difference between net radiation and the ground heat flux (Liebethal et al., 2005) as well as the heat storage in the biomass (the latter is only determined for forest), is shown. The results are given in Tables 3 and 4 and are partly illustrated in Fig. 5. Due to a non-equal distribution of the data points, the data have been binned for flux classes of the modeled data, whereby the number of bins has been chosen so that the measured flux data are equally distributed. Therefore, the observed trends in the data have not been artificially shifted due to the applied binning, and the excluded data (for cases with Bo<0 and too-small nighttime fluxes) have merely been located in one bin. Figure 5 is created without the confidence intervals for the regression curves because the fluxes only marginally scatter around the average regression curve and for the sake of improved clarity. Tables 3 and 4 additionally contain the results for the regressions through the point of origin because of the large intercepts observed in regression analysis. Nevertheless, the slope did not vary substantially between the two regression methods.
The analysis of the eddy covariance data shows that in all cases, except for the latent heat in the second golden day period, the fluxes are underestimated and an energy balance correction is necessary to obtain an agreement with the modeled data. All results are very similar for both golden day periods. For a discussion of the results included in Fig. 5 as well as Tables 3 and 4, the available energy is also shown and the therein considered fluxes are summarized. The net radiation is underestimated by the model by about 5–7 % for both sites (not shown). The ground heat flux and the storage in the biomass both amount to less than 10 % of the net radiation, with a large scatter between the measured and the modeled data. A significant difference from the 1:1 relationship has only been found for the ground heat flux of the clearing, which is higher by a factor of 2 for the experimental data. Due to the installation of the sensors the ground was probably less covered with vegetation and could thus store more energy. For the forest site, the underestimation of the available energy by the model was only about 4 %. For the clearing site, the modeled available energy has a constant offset of 30 W m−2 and was therefore higher than the experimental data.
The effect of the different energy balance corrections is not equal for both sites. For the forest site, both corrections of the sensible heat flux agree quite well with the model, with slightly better values for the buoyancy correction. For the latent heat flux of the forest site, the measurements corrected according to the Bowen ratio are in better agreement with the simulations. For Bo>1, the buoyancy correction, which assumes that only convection is the reason for the unclosed energy balance, overestimates the energy balance closure correction and the true correction might lie between the two correction methods. The turbulent fluxes might be slightly higher than the modeled fluxes because of the measured available energy being about 4 % higher, as can be seen after applying both corrections (see also Sect. 3.2.3).
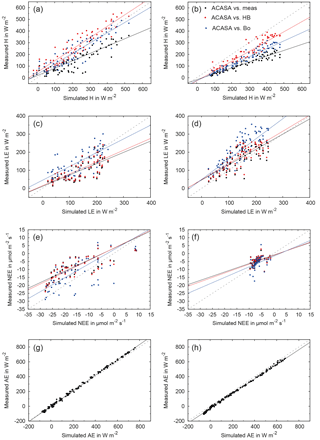
Figure 5Example of the regression analysis of the measured and the simulated (ACASA) data for GDP 1 for (a, b) the sensible heat flux (H); (c, d) the latent heat flux (LE); (e, f) the net ecosystem exchange (NEE); and (g, h) the available energy (AE) above the forest (a, c, e, g) and above the clearing at 5.5 m of height (footprint-weighted modeled fluxes; b, d, f, h) for uncorrected (black), Bowen-ratio-corrected (blue), and buoyancy-corrected (red) eddy covariance data. The regression lines are based on binned data for 50 W m−2 or 2∕4 classes of the modeled data and an orthogonal regression. The analysis has been achieved for the first and second golden day periods, with Tables 3 and 4 displaying the results of an orthogonal regression with and without assuming a zero intercept for the clearing and the forest, respectively.
Table 3Results of the comparison of the measured and the modeled flux data for different correction methods with an orthogonal regression for binned data points for the clearing with and without footprint weighting (for details, see Fig. 5; absolute values in W m−2 or for the NEE).
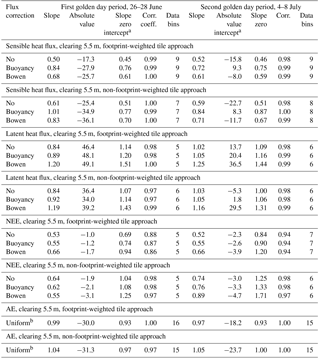
a Orthogonal regression considering a zero intercept.
b AE is the same for all three methods because the heat storage term of the canopy and the ground heat flux are uniformly estimated.
Table 4Results of the comparison of the measured and the modeled flux data for different correction methods with an orthogonal regression for binned data points for the forest (absolute values in W m−2 or for the NEE).
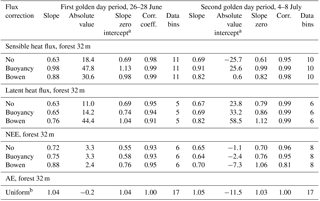
a Orthogonal regression considering a zero intercept.
b AE is the same for all three methods because the heat storage term of the canopy and the ground heat flux are uniformly estimated.
However, for the clearing with Bo<1, different results have been found: with the Bowen ratio correction, the sensible heat flux is significantly underestimated by about 30 % and the latent heat flux is overestimated by about 12 %. The buoyancy correction underestimates both fluxes by about 10 %. The measured latent heat flux is often larger than the modeled latent heat flux. This is probably a large effect of the plants in the footprint and the soil water content, which is not proportional to the land cover fraction used by the footprint model. Taking into account the 30 W m−2 higher available energy of the model, which is of the order of about 10–20 % of the turbulent fluxes (therefore, the modeled fluxes are higher by this value), the buoyancy correction is more appropriate for the clearing.
The correction of the NEE data seems to be necessary, but the underestimation by the model is still given. This could be an overestimation by the measured fluxes due to the increased mechanical turbulence and consequently also turbulent fluxes caused by the heterogeneous forest structure, as discussed by Foken (2017b). Furthermore, a related LES study by Kanani-Sühring and Raasch (2015) shows a maximal flux at a distance from the roughness step of about 10 times the canopy height that is nearly the location of the tower. Therefore, the Bowen ratio correction of the NEE for the forest seems to agree better, while over the clearing the fluxes are too small to allow useful conclusions to be made.
3.2.3 Analysis of the energy balance closure correction
In this section we used the modeled data, which close the energy balance by definition as an etalon, for the validation of the correction methods. Therefore, the modeled Bowen ratio was assumed to be true and the measured fluxes have been corrected in such a way that the energy balance was closed and the Bowen ratio agreed with the modeled Bowen ratio. Finally, the fraction of the residual attributed to the sensible heat flux has been determined and is shown in Fig. 6. Sometimes, when the measured latent heat flux was significantly larger than the modeled flux, the fraction was up to 150 %. This analysis was only made for the forest with a more homogeneous footprint. Figure 6 also shows, for comparison, the proposed corrections according to the Bowen ratio (Twine et al., 2000) and the buoyancy flux (Charuchittipan et al., 2014). Clearly, the assumption that convection is responsible for the energy balance problem and that a large part of the residual should be added to the sensible heat flux may only be true for Bo>1.5. For lower Bowen ratios, the real correction might lie between the two methods and for Bo<1 be more in accordance with the Bowen ratio correction. Unfortunately, the number of available data points for Bo<1.5 is very low, so the presented results can only be interpreted as a tendency. This means that secondary circulations probably responsible for the unclosed energy balance are not only generated by convective processes. Further research is necessary, including other well-parameterized models that close the energy balance very well, as are data sets for lower Bowen ratios. In all cases with high Bowen ratios, the attribution of residual energy to latent heat flux is low. Therefore, due to the assumption of similarity between the water and carbon dioxide fluxes, the NEE flux was only marginally corrected under these conditions. In conclusion, for spruce forests with typically high Bowen ratios, no correction of the NEE is necessary.
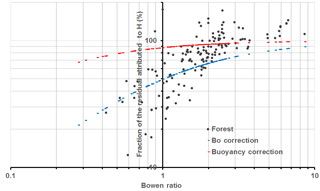
Figure 6Fraction of the residual attributed to the sensible heat flux for the forest site (GDP 1, 2, and 3), under the assumption that the model calculated the true Bowen ratio, and according to the correction methods with the Bowen ratio (Twine et al., 2000) and the buoyancy flux (Charuchittipan et al., 2014).
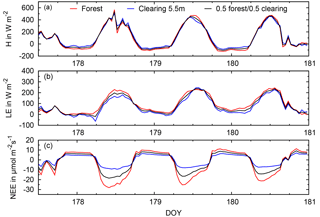
Figure 7Main daily cycle of the (a) sensible and (b) latent heat flux as well as (c) NEE for the first golden day period modeled with ACASA for the forest (red line) and the clearing at 5.5 m (tile approach for the different land use types of the clearing; blue line) and for a flux weighting of 50 % clearing and 50 % forest (black line).
3.2.4 Tile approach for a mixed forest site
The comparison of the modeled and the measured fluxes has shown that the ACASA model determines the fluxes for high and low vegetation with high accuracy and within the typical measurement uncertainty (Mauder et al., 2006, 2013) of eddy covariance measurements if the measured data are corrected for energy balance closure. This offers the possibility to model fluxes over larger heterogeneous areas, like a catchment, with a tile approach. Even when the fluxes above the different land use types are significantly different and are changing, e.g., with the Bowen ratio, the tile approach achieves appropriate results. To illustrate these differences, Fig. 7 shows mean daily cycles for the Waldstein–Weidenbrunnen forest site and the heterogeneous Köhlerloh clearing, whereby every surface contributes 50 % to the additionally shown weighted flux. The magnitudes of the sensible heat flux are generally higher at the forest than at the clearing. In contrast, during the day the latent heat flux is higher for the forest and during night the dewfall is higher for the clearing. The main differences for both surfaces occur for the NEE. The magnitude of the NEE for the forest is sometimes more than twice that from the clearing during the day. During the night, the differences between the NEE for the forest and the clearing are smaller. Falge et al. (2017) already showed a high variation in the NEE between the different species considered in the tile approach for the clearing. This variability is also present for the comparison of the NEE for the forest and the clearing. These differences are illustrated because the NEE data measured at the Waldstein–Weidenbrunnen site are used in many modeling studies within FLUXNET. For the specification of surface characteristics these studies often use remote sensing data, in which the forest and the clearing are contained in the relevant grid. Thus, the satellite-derived LAI and fractional PAR (photosynthetically active radiation) have to be interpreted as a mixture of forest and clearing. However, the FLUXNET station at Waldstein–Weidenbrunnen is characterized only as a forest measuring station, with Picea abies as the main vegetation type, because the fluxes have been obtained at the main tower (MT; Fig. 1b) or at the turbulence tower (TT) since 2007. Consequently, the NEE can hardly be reproduced through the consideration of the weighted vegetation parameterization.
The ACASA model, which was originally developed for tall vegetation, can also be used with high accuracy for low vegetation, as was demonstrated for the clearing, if the plant-specific parameters are appropriately implemented in the model. Therefore, it is applicable to consider ACASA in tile approaches for heterogeneous areas for tall and short vegetation or clearings.
The footprint-averaged tile approach did not produce significantly better results than the tile approach for the whole clearing, as was done by Falge et al. (2017). The footprint model is probably not accurate enough in the location of the effect levels of the footprint, considering the small-scale heterogeneities of the clearing in comparison to the size of the footprint area. This issue has already been shown in a comparison of different footprint models by Markkanen et al. (2009). For larger heterogeneities, this approach may be more appropriate. Furthermore, the clearing area contained randomly distributed very wet parts, which could not be classified in the land use characteristics of the tile approaches.
The small underestimation by the model of fluxes above the forest is probably a local overestimation of the fluxes at the turbulence tower (TT), which is adjacent to an area of the forest where higher fluxes are possible (Foken, 2017b). Large-eddy simulation studies have shown that at a distance of 10 times the canopy height from the forest edge, higher fluxes are possible (Kanani-Sühring and Raasch, 2015). Such a highly local effect is impossible to model in ACASA.
Assuming that the ACASA model is well parameterized and the available energy is accurately distributed to the sensible and the latent heat flux, a good agreement has been found with the energy-balance-corrected measurements. The correction with the buoyancy flux leads to better results, but this depends on the Bowen ratio; i.e., for Bo>1 it is better than for This result supports earlier findings (Ingwersen et al., 2011; Babel et al., 2014). The idea behind the buoyancy correction that buoyancy is the reason for secondary circulations is only partly true. Possible errors in the eddy covariance method cannot be inferred from the improved applicability of the Bowen ratio correction for low Bowen ratios (Bo<1). However, a recent investigation by Gao et al. (2017) showed a potential phase shift between the vertical wind component and the scalars, which might bias the determination of turbulent fluxes. This should be an objective of a further study with a data set containing more data for Bo<1. The correction of the NEE is probably useful, but the effect is not very significant for Bo>1; however, it might be more applicable for accumulated fluxes.
The paper is based on the master's degree thesis of KG, who was advised by AR and TF and supported by WB (application of the footprint modeling and turbulence data calculation), EF (plant parameters), and RDP and KTPU (application of ACASA). The additional analysis in this paper and the writing was done by KG, who was advised by TF.
The authors declare that they have no conflict of interest.
The first author acknowledges Ina Tegen for providing the opportunity to finalize this paper at the Leibniz Institute for Tropospheric Research. We thank the editor and the three anonymous reviewers for the helpful comments.
This research was funded within the DFG projects FO 226/16-1 and ME
2100/4-1 as well the DFG PAK 446 project, mainly through the subprojects ME
2100/5-1 and FO226/21-1, the German Federal Ministry of Education,
Science, Research and Technology (PT BEO 51-0339476 D), and the BaCaTeC
(Bayerisch-Kalifornische Hochschulzentrum) project Modellierung des
Energieaustausches zwischen der Atmosphäre und
Waldökosystemen. Partial support came from a grant from the US
National Science Foundation EF1137306 to the Massachusetts Institute
of Technology, sub-award 5710003122 to the University of California,
Davis. This publication was funded by the German Research Foundation
(DFG) and the University of Bayreuth within the funding program Open
Access Publishing.
Edited by: Andreas Ibrom
Reviewed by: three anonymous referees
Aubinet, M., Feigenwinter, C., Heinesch, B., Bernhofer, C., Canepa, E., Lindroth, A., Montagnani, L., Rebmann, C., Sedlak, P., and Gorsel, E. V.: Direct advection measurements do not help to solve the night-time CO2 closure problem: Evidence from three different forests, Agr. Forest Meteorol., 150, 655–664, https://doi.org/10.1016/j.agrformet.2010.01.016, special Issue on Advection: ADVEX and Other Direct Advection Measurement Campaigns, 2010. a
Aubinet, M., Vesala, T., and Papale, D. (Eds.): Eddy Covariance, A Practical Guide to Measurement and Data Analysis, Springer, Heidelberg, https://doi.org/10.1007/978-94-007-2351-1, 2012. a, b
Babel, W., Biermann, T., Coners, H., Falge, E., Seeber, E., Ingrisch, J., Schleuß, P.-M., Gerken, T., Leonbacher, J., Leipold, T., Willinghöfer, S., Schützenmeister, K., Shibistova, O., Becker, L., Hafner, S., Spielvogel, S., Li, X., Xu, X., Sun, Y., Zhang, L., Yang, Y., Ma, Y., Wesche, K., Graf, H.-F., Leuschner, C., Guggenberger, G., Kuzyakov, Y., Miehe, G., and Foken, T.: Pasture degradation modifies the water and carbon cycles of the Tibetan highlands, Biogeosciences, 11, 6633–6656, https://doi.org/10.5194/bg-11-6633-2014, 2014. a
Bergström, H. and Högström, U.: Turbulent exchange above a pine forest II. organized structures, Bound.-Lay. Meteorol., 49, 231–263, https://doi.org/10.1007/bf00120972, 1989. a
Biermann, T., Babel, W., Ma, W., Chen, X., Thiem, E., Ma, Y., and Foken, T.: Turbulent flux observations and modelling over a shallow lake and a wet grassland in the Nam Co basin, Tibetan Plateau, Theor. Appl. Climatol., 116, 301–316, https://doi.org/10.1007/s00704-013-0953-6, 2014. a
Charuchittipan, D., Babel, W., Mauder, M., Leps, J.-P., and Foken, T.: Extension of the Averaging Time in Eddy-Covariance Measurements and Its Effect on the Energy Balance Closure, Bound.-Lay. Meteorol., 152, 303–327, https://doi.org/10.1007/s10546-014-9922-6, 2014. a, b, c, d
Collatz, G., Ball, J., Grivet, C., and Berry, J.: Physiological and environmental regulation of stomatal conductance, photosynthesis and transpiration: a model that includes a laminar boundary layer, Agr. Forest Meteorol., 54, 107–136, https://doi.org/10.1016/0168-1923(91)90002-8, 1991. a
de Vries, D.: The thermal conductivity of granular material, Bulletin de l'Institut International du Froid, Annexure, 32, 115–131, 1952. a
Deardorff, J.: The Counter-Gradient Heat Flux in the Lower Atmosphere and in the Laboratory, J. Atmos. Sci., 23, 503–506, https://doi.org/10.1175/1520-0469(1966)023<0503:tcghfi>2.0.co;2, 1966. a
Dunn, G.: Statistical Evaluation of Measurement Errors, Arnold, London, 2004. a
Falge, E., Graber, W., Siegwolf, R., and Tenhunen, J.: A model of the gas exchange response of Picea abies to habitat conditions, Trees, 10, 277–287, https://doi.org/10.1007/pl00009649, 1996. a
Falge, E., Köck, K., Gatzsche, K., Voß, L., Schäfer, A., Berger, M., Dlugi, R., Pyles, R., Paw U, K., Raabe, A., and Foken, T.: Modeling of Energy and Matter Exchange, in: Energy and Matter Fluxes of a Spruce Forest Ecosystem, Ecological Studies, edited by: Foken, T., Springer, Cham, 379–414, https://doi.org/10.1007/978-3-319-49389-3_16, 2017. a, b, c, d, e, f, g, h
Falk, M., Pyles, R. D., Ustin, S. L., U, K. T. P., Xu, L., Whiting, M. L., Sanden, B. L., and Brown, P. H.: Evaluated Crop Evapotranspiration over a Region of Irrigated Orchards with the Improved ACASA-WRF Model, J. Hydrometeorol., 15, 744–758, https://doi.org/10.1175/JHM-D-12-0183.1, 2014. a
Farquhar, G. and Caemmerer, S.: Modelling of Photosynthetic Response to Environmental Conditions, in: Physiological Plant Ecology II, Water Relations and Carbon Assimilation, Encyclopedia of Plant Physiology, vol. 12B, chap. 16, edited by: Lange, O. L., Nobel, P. S., Osmond, C. B., and Ziegler, H., Springer, Berlin, 549–587, 1982. a
Foken, T.: The energy balance closure problem: an overview, Ecol. Appl., 18, 1351–1367, https://doi.org/10.1890/06-0922.1, 2008. a, b
Foken, T. (Ed.): Energy and Matter Fluxes of a Spruce Forest Ecosystem, Ecological Studies, Springer, Cham, 532 pp., https://doi.org/10.1007/978-3-319-49389-3, 2017a. a, b
Foken, T.: What can we learn for a better understanding of the turbulent exchange processes at FLUXNET sites, in: Energy and Matter Fluxes of a Spruce Forest Ecosystem, Ecological Studies edited by: Foken, T., Springer, Cham, 461–475, https://doi.org/10.1007/978-3-319-49389-3_19, 2017b. a, b
Foken, T. and Leclerc, M.: Methods and limitations in validation of footprint models, Agr. Forest Meteorol., 127, 223–234, https://doi.org/10.1016/j.agrformet.2004.07.015, 2004. a
Foken, T., Göckede, M., Mauder, M., Mahrt, L., Amiro, B., and Munger, J.: Post-field data quality control, in: Handbook of Micrometeorology: A Guide for Surface Flux Measurement and Analysis, edited by: Lee, X., Massmann, W., and Law, B., Kluwer, Dordrecht, 181–208, 2004. a
Foken, T., Aubinet, M., Finnigan, J., Leclerc, M., Mauder, M., and Paw U, K.: Results of a Panel Discussion about the Energy Balance Closure Correction for Trace Gases, B. Am. Meteorol. Soc., 92, ES13–ES18, https://doi.org/10.1175/2011bams3130.1, 2011. a
Foken, T., Meixner, F. X., Falge, E., Zetzsch, C., Serafimovich, A., Bargsten, A., Behrendt, T., Biermann, T., Breuninger, C., Dix, S., Gerken, T., Hunner, M., Lehmann-Pape, L., Hens, K., Jocher, G., Kesselmeier, J., Lüers, J., Mayer, J.-C., Moravek, A., Plake, D., Riederer, M., Rütz, F., Scheibe, M., Siebicke, L., Sörgel, M., Staudt, K., Trebs, I., Tsokankunku, A., Welling, M., Wolff, V., and Zhu, Z.: Coupling processes and exchange of energy and reactive and non-reactive trace gases at a forest site – results of the EGER experiment, Atmos. Chem. Phys., 12, 1923–1950, https://doi.org/10.5194/acp-12-1923-2012, 2012. a, b, c, d
Foken, T., Gerstberger, P., Köck, K., Siebicke, L., Serafimovich, A., and Lüers, J.: Description of the Waldstein Measuring Site, in: Energy and Matter Fluxes of a Spruce Forest Ecosystem, Ecological Studies, edited by: Foken, T., Springer, Cham, 19–38, https://doi.org/10.1007/978-3-319-49389-3_2, 2017a. a, b, c, d
Foken, T., Göckede, M., Lüers, J., Siebicke, L., Rebmann, C., Ruppert, J., and Thomas, C.: Development of Flux Data Quality Tools, in: Energy and Matter Fluxes of a Spruce Forest Ecosystem, Ecological Studies, edited by: Foken, T., Springer, Cham, 277–308, https://doi.org/10.1007/978-3-319-49389-3_12, 2017b. a, b
Gao, W., Shaw, R., and Paw U, K.: Observation of organized structures in turbulent flow within and above a forest canopy, Bound.-Lay. Meteorol., 47, 349–377, https://doi.org/10.1007/bf00122339, 1989. a
Gao, Z., Liu, H., Katul, G., and Foken, T.: Non-closure of the surface energy balance explained by phase difference between vertical velocity and scalars of large atmospheric eddies, Environ. Res. Lett., 12, 034025, http://stacks.iop.org/1748-9326/12/i=3/a=034025, 2017. a
Gerstberger, P., Foken, T., and Kalbitz, K.: The Lehstenbach and Steinkreuz Catchments in NE Bavaria, Germany, in: Ecological Studies, Biogeochemistry of forested catchments in a changing environment: a German case study, 172, edited by: Matzner, E., Springer Verlag, Berlin, Heidelberg, 15–44, 2004. a
Göckede, M., Markkanen, T., Mauder, M., Arnold, K., Leps, J.-P., and Foken, T.: Validation of footprint models using natural tracer measurements from a field experiment, Agr. Forest Meteorol., 135, 314–325, https://doi.org/10.1016/j.agrformet.2005.12.008, 2005. a
Göckede, M., Markkanen, T., Hasager, C. B., and Foken, T.: Update of a Footprint-Based Approach for the Characterisation of Complex Measurement Sites, Bound.-Lay. Meteorol., 118, 635–655, https://doi.org/10.1007/s10546-005-6435-3, 2006. a
Göckede, M., Thomas, C., Markkanen, T., Mauder, M., Ruppert, J., and Foken, T.: Sensitivity of Lagrangian Stochastic footprints to turbulence statistics, Tellus B, 59, 577–586, https://doi.org/10.1111/j.1600-0889.2007.00275.x, 2007. a
Göckede, M., Foken, T., Aubinet, M., Aurela, M., Banza, J., Bernhofer, C., Bonnefond, J. M., Brunet, Y., Carrara, A., Clement, R., Dellwik, E., Elbers, J., Eugster, W., Fuhrer, J., Granier, A., Grünwald, T., Heinesch, B., Janssens, I. A., Knohl, A., Koeble, R., Laurila, T., Longdoz, B., Manca, G., Marek, M., Markkanen, T., Mateus, J., Matteucci, G., Mauder, M., Migliavacca, M., Minerbi, S., Moncrieff, J., Montagnani, L., Moors, E., Ourcival, J.-M., Papale, D., Pereira, J., Pilegaard, K., Pita, G., Rambal, S., Rebmann, C., Rodrigues, A., Rotenberg, E., Sanz, M. J., Sedlak, P., Seufert, G., Siebicke, L., Soussana, J. F., Valentini, R., Vesala, T., Verbeeck, H., and Yakir, D.: Quality control of CarboEurope flux data – Part 1: Coupling footprint analyses with flux data quality assessment to evaluate sites in forest ecosystems, Biogeosciences, 5, 433–450, https://doi.org/10.5194/bg-5-433-2008, 2008. a
Hasager, C. B. and Jensen, N. O.: Surface-flux aggregation in heterogeneous terrain, Q. J. Roy. Meteor. Soc., 125, 2075–2102, https://doi.org/10.1002/qj.49712555808, 1999. a
Haverd, V., Cuntz, M., Leuning, R., and Keith, H.: Air and biomass heat storage fluxes in a forest canopy: Calculation within a soil vegetation atmosphere transfer model, Agr. Forest Meteorol., 147, 125–139, https://doi.org/10.1016/j.agrformet.2007.07.006, 2007. a
Ingwersen, J., Steffens, K., Högy, P., Warrach-Sagi, K., Zhunusbayeva, D., Poltoradnev, M., Gäbler, R., Wizemann, H.-D., Fangmeier, A., Wulfmeyer, V., and Streck, T.: Comparison of Noah simulations with eddy covariance and soil water measurements at a winter wheat stand, Agr. Forest Meteorol., 151, 345–355, https://doi.org/10.1016/j.agrformet.2010.11.010, 2011. a
Kanani-Sühring, F. and Raasch, S.: Spatial variability of scalar concentrations and fluxes downstream of a clearing-to-forest transition: A Large-Eddy Simulation study, Bound.-Lay. Meteorol., 155, 1–27, https://doi.org/10.1007/s10546-014-9986-3, 2015. a, b
Kanani-Sühring, F., and Raasch, S.: Enhanced Scalar Concentrations and Fluxes in the Lee of Forest Patches: A Large-Eddy Simulation Study, Bound.-Lay. Meteorol., 164, 1–17, https://doi.org/10.1007/s10546-017-0239-0, 2017. a
Leclerc, M. Y. and Foken, T.: Footprints in Micrometeorology and Ecology, Springer, Berlin, Heidelberg, https://doi.org/10.1007/978-3-642-54545-0, 2014. a, b
Leuning, R.: Modelling stomatal behaviour and photosynthesis of Eucalyptus gradis, Aust. J. Plant Physiol., 17, 159–175, https://doi.org/10.1071/PP9900159, 1990. a
Liebethal, C., Huwe, B., and Foken, T.: Sensitivity analysis for two ground heat flux calculation approaches, Agr. Forest Meteorol., 132, 253–262, https://doi.org/10.1016/j.agrformet.2005.08.001, 2005. a
Lindroth, A., Mölder, M., and Lagergren, F.: Heat storage in forest biomass improves energy balance closure, Biogeosciences, 7, 301–313, https://doi.org/10.5194/bg-7-301-2010, 2010. a
Markkanen, T., Steinfeld, G., Kljun, N., Raasch, S., and Foken, T.: Comparison of conventional Lagrangian stochastic footprint models against LES driven footprint estimates, Atmos. Chem. Phys., 9, 5575–5586, https://doi.org/10.5194/acp-9-5575-2009, 2009. a
Mauder, M. and Foken, T.: Eddy-Covariance Software TK3, https://doi.org/10.5281/zenodo.20349, 2015. a
Mauder, M., Liebetahl, C., Göckede, M., Leps, J., Beyrich, F., and Foken, T.: Processing and quality control of flux data during LITFASS-2003, Bound.-Lay. Meteorol., 121, 67–88, https://doi.org/10.1007/s10546-006-9094-0, 2006. a
Mauder, M., Cuntz, M., Drüe, C., Graf, A., Rebmann, C., Schmid, H. P., Schmidt, M., and Steinbrecher, R.: A strategy for quality and uncertainty assessment of long-term eddy-covariance measurements, Agr. Forest Meteorol., 169, 122–135, https://doi.org/10.1016/j.agrformet.2012.09.006, 2013. a
Meyers, T.: A simulation of the canopy microenvironment using higher order closure principles, Ph.D. thesis, Purdue University, West Lafayette, IN, USA, 153 pp., 1985. a
Meyers, T. and Paw U, K.: Testing of a higher-order closure model for airflow within and above plant canopies, Bound.-Lay. Meteorol., 37, 297–311, https://doi.org/10.1007/BF00122991, 1986. a, b
Meyers, T. and Paw U, K.: Modeling the plant canopy micrometeorology with higher-order closure techniques, Agr. Forest Meteorol., 41, 143–163, https://doi.org/10.1016/0168-1923(87)90075-X, 1987. a
Mölders, N.: Land-Use and Land-Cover Changes, Impact on climate and air quality, Springer, Dordrecht, Heidelberg, London, New York, 189 pp., https://doi.org/10.1007/978-94-007-1527-1, 2012. a
Paw U, K. and Gao, W.: Applications of solutions to non-linear energy budget equations, Agr. Forest Meteorol., 43, 121–145, https://doi.org/10.1016/0168-1923(88)90087-1, 1988. a
Pearson, R. J., Oncley, S. P., and Delany, A. C.: A scalar similarity study based on surface layer ozone measurements over cotton during the California Ozone Deposition Experiment, J. Geophys. Res.-Atmos., 103, 18919–18926, https://doi.org/10.1029/98JD01479, 1998. a
Pyles, R., Weare, B., and Paw U, K.: The UCD Advanced Canopy-Atmosphere-Soil Algorithm: Comparisons with observations from different climate and vegetation regimes, Q. J. Roy. Meteor. Soc., 126, 2951–2980, https://doi.org/10.1256/smsqj.56916, 2000. a, b, c
Pyles, R., Weare, B., Paw U, K., and Gustafson, W.: Coupling between the University of California, Davis, Advanced Canopy-Atmosphere-Soil Algorithm (ACASA) and MM5: Preliminary Results for July 1998 for Western North America, J. Appl. Meteorol., 42, 557–569, https://doi.org/10.1175/1520-0450(2003)042<0557:cbtuoc>2.0.co;2, 2003. a
Rannik, Ü., Aubinet, M., Kurbanmuradov, O., Sabelfeld, K. K., Markkanen, T., and Vesala, T.: Footprint Analysis For Measurements Over A Heterogeneous Forest, Bound.-Lay. Meteorol., 97, 137–166, https://doi.org/10.1023/A:1002702810929, 2000. a
Rannik, Ü., Markkanen, T., Raittila, J., Hari, P., and Vesala, T.: Turbulence Statistics Inside and Over Forest: Influence on Footprint Prediction, Bound.-Lay. Meteorol., 109, 163–189, https://doi.org/10.1023/A:1025404923169, 2003. a
Reithmaier, M. L., Göckede, M., Markkanen, T., Knohl, A., Churkina, G., Rebmann, C., Buchmann, N., and Foken, T.: Use of remotely sensed land use classification for a better evaluation of micrometeorological flux measurement sites, Theor. Appl. Climatol., 84, 219–233, https://doi.org/10.1007/s00704-005-0168-6, 2006. a
Ruppert, J., Thomas, C., and Foken, T.: Scalar Similarity for Relaxed Eddy Accumulation Methods, Bound.-Lay. Meteorol., 120, 39–63, https://doi.org/10.1007/s10546-005-9043-3, 2006. a
Smirnova, T., Brown, J., and Benjamin, S.: Performance of different soil model configurations in simulating ground surface temperature and surface fluxes, Mon. Weather Rev., 125, 1870–1884, https://doi.org/10.1175/1520-0493(1997)125<1870:podsmc>2.0.co;2, 1997. a
Smirnova, T., Brown, J., Benjamin, S., and Kim, D.: Parametrization of cold-season processes in the MAPS land-surface scheme, J. Geophys. Res.-Atmos., 105, 4077–4086, https://doi.org/10.1029/1999jd901047, 2000. a
Sogachev, A. and Lloyd, J.: Using a One-and-a-Half Order Closure Model of the Atmospheric Boundary Layer for Surface Flux Footprint Estimation, Bound.-Lay. Meteorol., 112, 467–502, https://doi.org/10.1023/B:BOUN.0000030664.52282.ee, 2004. a
Sogachev, A., Menzhulin, G., Heimann, M., and Lloyd, J.: A simple three-dimensional canopy–planetary boundary layer simulation model for scalar concentrations and fluxes, Tellus B, 54, 784–819, https://doi.org/10.1034/j.1600-0889.2002.201353.x, 2002. a
Staudt, K., Falge, E., Pyles, R. D., Paw U, K. T., and Foken, T.: Sensitivity and predictive uncertainty of the ACASA model at a spruce forest site, Biogeosciences, 7, 3685–3705, https://doi.org/10.5194/bg-7-3685-2010, 2010. a, b
Staudt, K., Serafimovich, A., Siebicke, L., Pyles, R., and Falge, E.: Vertical structure of evapotranspiration at a forest site (a case study), Agr. Forest Meteorol., 151, 709–729, https://doi.org/10.1016/j.agrformet.2010.10.009, 2011. a, b, c
Su, H. and Paw U, K.: Development of a coupled leaf and canopy model for the simulation of plant-atmosphere interactions, J. Appl. Meteorol., 35, 733–748, https://doi.org/10.1175/1520-0450(1996)035<0733:doacla>2.0.co;2, 1996. a
Tcherkez, G., Bligny, R., Gout, E., Mahé, A., Hodges, M., and Cornic, G.: Respiratory metabolism of illuminated leaves depends on CO2 and O2 conditions, P. Natl. Acad. Sci. USA, 105, 797–802, https://doi.org/10.1073/pnas.0708947105, 2008. a
Thomas, C. K., Serafimovich, A., Siebicke, L., Gerken, T., and Foken, T.: Coherent Structures and Flux Coupling, Springer, Cham, 113–135, https://doi.org/10.1007/978-3-319-49389-3_6, 2017. a
Twine, T., Kustas, W., Norman, J., Cook, D., Houser, P., Meyers, T., Prueger, J., Starks, P., and Wesely, M.: Correcting eddy-covariance flux underestimates over a grassland, Agr. Forest Meteorol., 103, 279–300, https://doi.org/10.1016/s0168-1923(00)00123-4, 2000. a, b, c, d