the Creative Commons Attribution 4.0 License.
the Creative Commons Attribution 4.0 License.
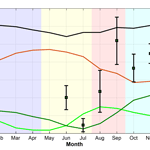
Leaf phenology as one important driver of seasonal changes in isoprene emissions in central Amazonia
Eliane G. Alves
Julio Tóta
Andrew Turnipseed
Alex B. Guenther
José Oscar W. Vega Bustillos
Raoni A. Santana
Glauber G. Cirino
Julia V. Tavares
Aline P. Lopes
Bruce W. Nelson
Rodrigo A. de Souza
Trissevgeni Stavrakou
David K. Adams
Scott Saleska
Antonio O. Manzi
Isoprene fluxes vary seasonally with changes in environmental factors (e.g., solar radiation and temperature) and biological factors (e.g., leaf phenology). However, our understanding of the seasonal patterns of isoprene fluxes and the associated mechanistic controls is still limited, especially in Amazonian evergreen forests. In this paper, we aim to connect intensive, field-based measurements of canopy isoprene flux over a central Amazonian evergreen forest site with meteorological observations and with tower-mounted camera leaf phenology to improve our understanding of patterns and causes of isoprene flux seasonality. Our results demonstrate that the highest isoprene emissions are observed during the dry and dry-to-wet transition seasons, whereas the lowest emissions were found during the wet-to-dry transition season. Our results also indicate that light and temperature cannot totally explain isoprene flux seasonality. Instead, the camera-derived leaf area index (LAI) of recently mature leaf age class (e.g., leaf ages of 3–5 months) exhibits the highest correlation with observed isoprene flux seasonality (R2=0.59, p<0.05). Attempting to better represent leaf phenology in the Model of Emissions of Gases and Aerosols from Nature (MEGAN 2.1), we improved the leaf age algorithm by utilizing results from the camera-derived leaf phenology that provided LAI categorized into three different leaf ages. The model results show that the observations of age-dependent isoprene emission capacity, in conjunction with camera-derived leaf age demography, significantly improved simulations in terms of seasonal variations in isoprene fluxes (R2=0.52, p<0.05). This study highlights the importance of accounting for differences in isoprene emission capacity across canopy leaf age classes and identifying forest adaptive mechanisms that underlie seasonal variation in isoprene emissions in Amazonia.
- Article
(2216 KB) - Full-text XML
-
Supplement
(154 KB) - BibTeX
- EndNote
Isoprene is considered the dominant contribution to biogenic volatile organic compound (BVOC) emissions from many landscapes and represents the largest input to total global BVOC emissions, with a magnitude of 400–600 Tg C yr−1 (see Table 1 of Arneth et al., 2008). This compound regulates large-scale biogeochemical cycles. For example, once in the atmosphere, isoprene has implications for chemical and physical processes due to its reactivity, influences on the atmospheric oxidative capacity, and its potential to form secondary organic aerosols (Claeys et al., 2004), which interact with solar radiation and act as effective cloud condensation nuclei. Moreover, isoprene emissions could play an important role in the carbon balance because it has the largest contribution to total BVOCs, which are regarded as highly significant for net ecosystem productivity, with their losses comparable to the magnitude of net biome productivity (Kesselmeier et al., 2002). Carbon dioxide is believed to be the fate of almost half of the carbon released in the form of BVOCs (Goldstein and Galbally, 2007).
Tropical forests are the largest source of isoprene for the atmosphere, contributing almost half of the estimated global annual isoprene emissions according to Model of Emissions of Gases and Aerosols from Nature (MEGAN) estimates (Guenther et al., 2006). Given that the Amazon basin is the largest territorial contribution to global tropical forests, this ecosystem is thought to be one of the most important sources of isoprene for the global atmosphere.
Recently, remotely sensed observations from multiple years have revealed seasonal changes in isoprene emissions over the Amazonian rainforest (Barkley et al., 2008, 2009, 2013; Bauwens et al., 2016). Apart from these remotely sensed data, only a few studies based on in situ data exist (Alves et al., 2016; Andreae et al., 2002; Kesselmeier et al., 2002; Kuhn et al., 2004a; Yáñez-Serrano et al., 2015). Some of these in situ studies indicate that environmental factors such as solar radiation and temperature are primary drivers of isoprene emissions (Andreae et al., 2002; Kesselmeier et al., 2002; Kuhn et al., 2004a; Yáñez-Serrano et al., 2015).
However, besides long-term seasonal variation in light and temperature, other biological factors might act on seasonal changes in isoprene emissions, as in the case of canopy phenology. Previous studies with temperate species have shown that isoprene emission capacity is affected by leaf age and ontogeny (Kuzma and Fall, 1993; Mayrhofer et al., 2005; Monson et al., 1994) because of the following: (1) isoprene synthase and other enzymes of the isoprene synthesis pathway (MEP pathway) depend on the leaf ontogeny – isoprene synthase activity is low or absent in very young leaves, increases gradually until full leaf maturation, and decreases with leaf senescence (Schnitzler et al., 1997); (2) for species with non-senescent leaves or with a life span of more than 1 year, foliage shading and time-dependent changes in the physiological activity of leaves could decrease isoprene emission capacity (Niinemets et al., 2004, 2010); and (3) leaf structure varies with leaf ontogenetic stage, indicating that seasonal isoprene emission capacity is also affected by seasonal structural changes in leaves (Niinemets et al., 2004, 2010).
Leaf phenology, with notable seasonal changes in the Amazonian rainforest, was just recently discovered (Huete et al., 2006; Lopes et al., 2016; Myneni et al., 2007; Saleska et al., 2016; Wagner et al., 2017), and there is still some debate about it (e.g., Morton et al., 2014; Samanta et al., 2010). For many years seasonal changes and leaf phenology were thought to be unimportant for tropical forests, which were assumed to be in an evergreen condition state. This led the scientific modeling community to assume that leaf phenology has little affect on forest and atmosphere gas exchanges in the tropics. However, after remote sensing studies showed seasonal biomass changes (Myneni et al., 2007) and seasonal changes in isoprene emissions (Barkley et al., 2009, 2013), models were improved in order to better represent seasonal biomass changes and leaf age in tropical forests.
MEGAN already uses variations in LAI to parameterize changes in leaf age to stimulate changes in the emission activity factor of isoprene emissions (Guenther et al., 2012). However, because leaf phenology in tropical forests is not as notable as in temperate forests, some insights on how changes in leaf age over the year may affect seasonal isoprene emissions are still missing, and there is a lack of representation of this process in models. Here, our goal is to demonstrate that leaf phenology affects seasonal changes in isoprene emissions and this is, in fact, new information for tropical forests.
In this study, we present observations of seasonal variation in isoprene flux, solar radiation, air temperature, and canopy phenology from a primary rainforest site in central Amazonia. The questions addressed are the following: (i) how much can seasonal isoprene fluxes be explained by variations in solar radiation, temperature, and leaf phenology? (ii) How can a consideration of leaf phenology observed in the field help to improve model estimates of seasonal isoprene emissions? To this end, we correlate ground-based isoprene flux measurements with environmental factors (light and temperature) and a biological factor (leaf phenology). We compare seasonal ground-based isoprene flux measurements to OMI satellite-derived isoprene flux. Lastly, we perform two simulations with MEGAN 2.1 to estimate isoprene fluxes: (1) with standard emission algorithms and (2) with a modification in the leaf age algorithm derived from observed leaf phenology.
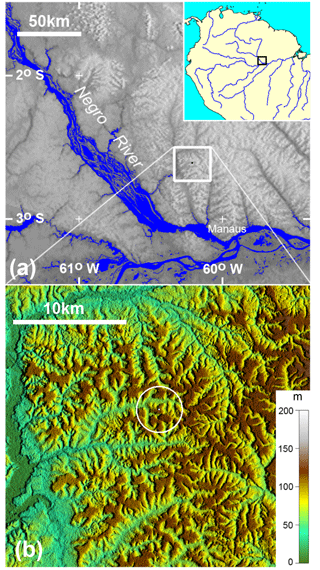
Figure 1Location of the experimental site in central Amazonia – K34 tower. Hill-shaded digital elevation data used as background topography are from the Shuttle Radar Topography Mission, with resolutions of ∼ 900 m (a) and ∼ 30 m (b). The white ring indicates a 2 km radius around the flux tower. The elevation scale for panel (b) is meters above sea level.
2.1 Site Description – Cuieiras Biological Reserve – K34 site
Isoprene fluxes were measured at the 53 m K34 tower (2∘36′32.6 S, 60∘12′33.4 W) on the Cuieiras Biological Reserve plateau, a primary rainforest reserve approximately 60 km northwest of Manaus in Amazonas state, Brazil (Fig. 1). The K34 tower has been widely utilized for the past 15 years for a range of meteorological studies, including energy and trace gas fluxes (de Araújo et al., 2010; Artaxo et al., 2013; Tóta et al., 2012) and also tropospheric variables such as precipitable water vapor (Adams et al., 2011, 2015). This reserve has an area of about 230 km2 and is managed by the National Institute for Amazonian Research (INPA). The site has a maximum altitude of 120 m and the topography is characterized by 31 % plateau, 26 % slope, and 43 % valley (Rennó et al., 2008). The vegetation in this area is considered mature, terra firme rainforest with a typical canopy height of 30 m with variation (20–45 m) throughout the reserve. More details about soils and vegetation at this site are provided in Alves et al. (2016). Annual precipitation is about 2500 mm and is dominated by deep atmospheric convection and associated stratiform precipitation, with December to May being the wet season and August to September the dry season when the monthly cumulative precipitation is less than 100 mm (Adams et al., 2013; Machado et al., 2004). Average air temperature ranges between 24 ∘C (in April) and 27 ∘C (in September; Alves et al., 2016).
2.2 Isoprene flux – relaxed eddy accumulation system (REA)
Isoprene flux measurements were conducted during intensive campaigns of 5 to 6 days between the 20th and 30th of each month during daytime (09:00–16:30 local time) from June 2013 to December 2013 at the K34 tower. The REA system utilized for the isoprene flux measurements was developed by the National Center for Atmospheric Research (NCAR; NCAR/BEACHON REA cassette sampler) and has two basic components: (1) the main REA box containing the adsorbent cartridges (stainless steel tubes filled with Tenax TA and Carbograph 5 TD adsorbents) for the up–down–neutral reservoirs, microcontroller, battery, selection valves, and mass flow controller (200 mL min−1; MKS Instruments Inc., model M100B01852CS1BV); and (2) a sonic anemometer (RM Young, model 81000VRE) for high-rate wind velocity measurements (10 Hz). This REA system was installed at a height of 48 m on the K34 tower (approximately 20 m above the mean canopy height).
The technique segregated the sample flow according to sonic-anemometer-derived vertical wind velocity over the flux averaging period (30 min). Isoprene fluxes (F) from the REA system over this period were estimated from
where b is an empirical proportionality coefficient (described below), σw is the standard deviation of w, and and are isoprene concentration averages in the up and down reservoirs, respectively (Bowling et al., 1998). The b coefficient was calculated from the sonic temperature and heat flux by rearranging the same equation, assuming scalar similarity (Monin–Obukhov similarity theory):
The REA sampler was operated with a “deadband” – a range of small w′ values centered on over which the air was sampled through the “neutral” line. The deadband used was ±0.6σw. The use of a deadband was advisable because this increased the differences in the measured concentrations () by sampling only larger eddies (with larger concentration fluctuations) into the up–down reservoirs, reducing the precision required for the analytical measurements. The b coefficient was also computed (from Eq. 2) using the same deadband. For this study, the b coefficient was calculated for every 30 min flux sampling period. The b coefficient averaged 0.40±0.06 and the flux measurements were filtered for b coefficients in the range of 0.3 to 0.6.
The air sampling was carried out with two tubing lines for up () and down () and one tubing line for neutral sampling air (±0.6σw – deadband), each consisting of approximately 1.5 m long tubes (polytetrafluoroethylene, PTFE) positioned such that they sampled air as close to the sonic anemometer as possible. Each inlet valve at the main REA box prevented air from entering the inactive tube (up in the case of down sampling (), down in the case of up sampling (), and both up and down in the case of deadband), which otherwise would compromise the concentration differences between the up and down reservoirs and consequently the flux calculation.
The microcontroller recorded the sonic anemometer data and triggered the segregation valves based on these data. The REA technique requires two initial data points prior to each flux averaging period to segregate the sample flow: (1) a mean vertical wind velocity, , and (2) σw. The determined the direction of the instantaneous vertical wind velocity () and σw was required to calculate the deadband threshold. Both the values of and σw were based on the values obtained from the last flux averaging period (30 min). The microcontroller stored all the necessary wind and temperature information to compute all the parameters required in Eqs. (1) and (2). More details on errors and uncertainties of the REA technique are found in Sect. S1 (Supplement).
2.3 Isoprene concentrations
The isoprene accumulated in the adsorbent cartridges was determined from laboratory analysis. The tube samples were analyzed with a thermal desorption system (TD; Markes International, UK) interfaced with a gas chromatograph–flame ionization detector (GC-FID; 19091J-413 series, Agilent Technologies, USA). After loading a tube in the ULTRA automatic sampler (model Ultra 1, Markes International, UK), which was connected to the thermal desorption system, the collected samples were dried by purging for 5 min with 50 sccm of ultrahigh-purity helium (all flow vented out of the split vent) before being transferred (300 ∘C for 10 min with 50 sccm of ultrapure nitrogen) to the thermal desorption cold trap held at −10 ∘C (Unity Series 1, Markes International, UK). During GC injection, the trap was heated to 300 ∘C for 3 min while back flushing with carrier gas (helium) at a flow rate of 6.0 sccm directed into the column (Agilent HP-5, 5 % phenyl methyl siloxane capillary, 30.0 m × 320 µm × 0.25 µm). The oven ramp temperature was programmed with an initial hold of 6 min at 27 ∘C, followed by an increase to 85 ∘C at 6 , followed by a hold at 200 ∘C for 6 min. The identification of isoprene from samples was confirmed by comparison of retention time with a solution of authentic isoprene liquid standard in methanol (10 µg mL−1 in methanol, Sigma-Aldrich, USA). The GC-FID was calibrated to isoprene by injecting 0.0, 23, 35, and 47 nL of the gas standard into separate tubes. The gas standard is 99.9 % of 500 ppb of isoprene in nitrogen (Apel & Riemer Environmental Inc., USA) and was injected into separate tubes at 11 mL min−1. The calibration curve (0.0, 23, 35, and 47 nL) was made thrice before the analysis of the sample tubes of each campaign, with a mean correlation coefficient equal to R2=0.98. In addition, two standard tubes (with 35 nL of isoprene) were run at every 20 sample tubes to check the system sensitivity. The limit of detection of isoprene was equal to 48.4 ppt. All tube samples were analyzed as described above with the exception of tube samples from June 2013 and July 2013. These were analyzed in a TD/GC-MS-FID system from the Atmospheric Chemistry Division, NCAR (see Sect. S1 of the Supplement for more details).
Isoprene concentration was determined using the sample volume that was passed through each tube. This volume was measured by the integration of the mass flow meter signal and stored within the REA data file. While sampling, the concentration found in the blank tubes connected to the cartridge cassette in the REA box, but without flow, was subtracted from the sample tube concentrations. The resulting concentration was used to calculate isoprene flux (Eq. 1) in .
2.4 Tower-camera-derived leaf phenology and demography
Upper canopy leaf phenology was monitored with a StarDot RGB imaging system (model NetCam XL 3MP) installed at 51 m of height on the K34 tower (Lopes et al., 2016; Nelson et al., 2014; Wu et al., 2016). The system used the native CMOS resolution of 1024×768 pixels and a varifocal lens (StarDot reference LEN-MV4510CS) adjusted to about 66∘ HFOV. The camera was set to automatic exposure and did not apply automatic color balance. The view was fixed with south azimuth toward a forested plateau area, monitoring the same crowns over time and excluding the sky so that autoexposure was based only on the forest. This system was locally controlled by a Compulab microcomputer (model Fit-PC2i), which stored the images in situ. Images were automatically logged every 2 min from 09:00 to 12:30 local time. Only images acquired near local noon and under overcast sky (having even diffuse illumination) were analyzed. Images were selected at 6-day intervals. The camera monitored the upper crown surfaces of 53 living trees over 24 months (1 December 2011 to 31 November 2013).
We used a camera-based tree inventory approach to monitor leaf phenology at this forest site (Lopes et al., 2016; Nelson et al., 2014; Wu et al., 2016). Specifically, we visually tracked the temporal trajectory of each tree crown and assigned them into one of three classes: “leaf flushing” (crowns that showed a large abrupt greening), “leaf abscising” (crowns that showed large abrupt greying, which is the color of bare upper canopy branches), or “no change”. We then aggregated our census to the monthly scale to derive the monthly average percentages of trees with new leaf flushing and with old leaf abscission. The percentage of tree crowns with green leaves (1 – the percentage of tree crowns with leaf abscission) is termed as “green crown fraction” (Wu et al., 2016). We obtained a camera-based canopy LAI by applying the same linear relationship between ground-measured LAI and camera-derived green crown fraction fitted at another central Amazon evergreen forest, the Tapajós K67 tower site (Wu et al., 2016). As the fraction of all crowns classified to the abscised state has been shown to be linearly and inversely proportional to total canopy LAI at seasonal timescales (Wu et al., 2016), it was used at K34 to provide a camera-based estimate of temporal variation in canopy LAI.
We also estimated the monthly canopy leaf demography by tracking the post-leaf-flush age of each crown's leaf cohort and sorting them into three leaf age classes throughout the year (young: ≤ 2 months; mature: 3–5 months; and old: ≥ 6 months; Nelson et al., 2014; Wu et al., 2016). By multiplying camera-derived total LAI by the camera-derived fraction of crowns in a given age class, LAIs were derived for the three leaf age classes: young leaf LAI, mature leaf LAI, and old leaf LAI.
2.5 Modeled isoprene flux estimates – MEGAN 2.1
Isoprene fluxes measured by REA (K34 site) were compared with those estimated by MEGAN 2.1. Isoprene emissions estimated by MEGAN 2.1 account for the main processes driving variations in emissions (Guenther et al., 2012). The isoprene flux activity factor for isoprene (γi) is proportional to the emission response to light (γP), temperature (γT), leaf age (γA), soil moisture (γSM), leaf area index (LAI), and CO2 inhibition () according to Eq. (3):
where CCE is the canopy environment coefficient. For this study, the canopy environment model of Guenther et al. (2006) was used with a CCE of 0.57. MEGAN 2.1 was run accounting for variations in light, temperature, and LAI. Based on changes in LAI, the model estimated foliage leaf age. Both CO2 inhibition and soil moisture activity factors were set equal to a constant of 1, assuming these parameters do not vary. In terms of soil moisture, no seasonal variation in the model was assumed because a previous study showed that during the dry season there is only a small reduction (∼ 10 %) in soil moisture compared to the wet season (Cuartas et al., 2012), and this reduction does not induce water stress to this forest region (Wagner et al., 2017). Moreover, based on the dataset of soil moisture from 2002 to 2006 (Cuartas et al., 2012), the soil moisture always exceeds the threshold for the isoprene drought response in MEGAN 2.1 (Guenther et al., 2012), which means that MEGAN would predict that there are no variations in isoprene emissions due to these observed changes in soil moisture. Details on model settings are found in Guenther et al. (2012).
Table 1Environmental and biological factors used to input MEGAN 2.1: number of days with data available for each variable for the year 2013.

a Number of days with images analyzed to derive CAMERA-LAI as described in
Sect. 2.4.
b Number of days that the satellite passed over the site domain.
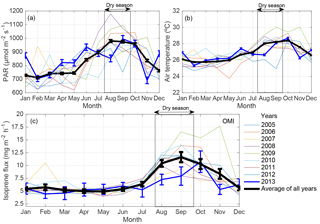
Figure 2(a) Monthly averages of photosynthetic active radiation (PAR) and (b) air temperature from 2005 to 2013 at the K34 tower site (measured every 30 min during 06:00–18:00 local time). (c) OMI satellite-derived isoprene flux at a resolution of 0.5 ∘C centered on the K34 tower site from 2005 to 2013. Monthly averages of isoprene flux were scaled to 10:00–14:00 local time. Error bars represent 1 standard error of the mean.
Photosynthetic photon flux density (PPFD) and air temperature inputs for all model simulations were obtained from measurements at the K34 tower. PPFD and air temperature measured at tower top every 30 min were hourly averaged. Data gaps during certain months occurred in 2013, but at least 15 days of hourly average PPFD and air temperature were obtained for model input. LAI inputs were acquired from the Moderate Resolution Imaging Spectroradiometer (MODIS) satellite observations for the same period of the isoprene flux measurements. The level-4 LAI product is composited every 8 days at 1 km resolution on a sinusoidal grid (MCD15A2H; Myneni, 2015). Additionally, by comparison with the standard MEGAN 2.1 model that uses MODIS-derived LAI variation, here we also used LAI fractionated into different leaf ages, which were obtained from tower camera observations (as described in the section above). The number of data inputs to the MEGAN simulations is summarized in Table 1.
2.6 Satellite-derived isoprene flux estimates
Top-down isoprene emission estimates over the 0.5∘ region around the tower were obtained by applying a grid-based source inversion scheme (Stavrakou et al., 2009, 2015) constrained by satellite formaldehyde (HCHO) columns and measured in the UV–Vis by the Ozone Monitoring Instrument (OMI) onboard the Aura satellite launched in 2004. HCHO is a high-yield intermediate product in the isoprene degradation process (Stavrakou et al., 2014). The source inversion was performed using the global chemistry transport model IMAGESv2 (Intermediate Model of Annual and Global Evolution of Species) at a resolution of and 40 vertical levels from the surface to the lower stratosphere (Stavrakou et al., 2014, 2015). The a priori isoprene emission inventory was taken from MEGAN–MOHYCAN (Stavrakou et al., 2014, http://emissions.aeronomie.be, last access: 15 January 2017, Bauwens et al., 2018). Given that the OMI overpass time is in the early afternoon (13:30 local time) and the mostly delayed production of formaldehyde from isoprene oxidation, the top-down emission estimates rely on the ability of MEGAN to simulate the diurnal isoprene emission cycle and on the parameterization of chemical and physical processes affecting isoprene and its degradation products in IMAGESv2. For this study, we use daily (24 h) mean satellite-derived isoprene emissions from January 2005 to December 2013. More details can be found in Stavrakou et al. (2009, 2015) and Bauwens et al. (2016).
The experimental site of this study showed seasonal variation in air temperature and in photosynthetic active radiation (PAR; Fig. 2a, b) that was comparable to the seasonality presented by the OMI satellite-derived isoprene fluxes for the K34 site domain (Fig. 2c). The interannual variation in the seasonality of these environmental factors, air temperature and PAR, was correlated with the one presented by the satellite-derived isoprene fluxes, with the highest correlation found between satellite-derived isoprene fluxes and air temperature. For isoprene fluxes and PAR, R2 ranged from 0.34 to 0.83 p<0.05; for isoprene fluxes and air temperature, R2 ranged from 0.61 to 0.91, with p<0.01, from 2005 to 2013. Maxima and minima of PAR, air temperature, and satellite-derived isoprene fluxes were observed during the dry and the dry-to-wet transition seasons and the wet and the wet-to-dry transition seasons, respectively.
Table 2Correlation coefficient, R2, of regressions for ground-based isoprene flux, satellite-derived isoprene flux, environmental factors, biological factors, and MEGAN 2.1 simulations.
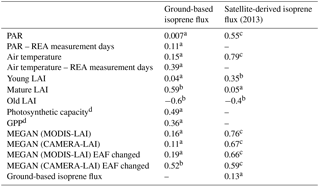
PAR: photosynthetic active radiation; GPP: gross primary productivity; EAF: emission activity factor; a not statistically significant (P>0.05); b statistically significant (P<0.05); c statistically significant (P<0.001); d data from Wu et al. (2016).
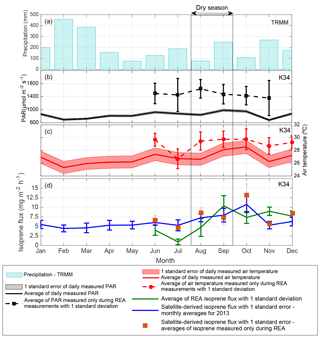
Figure 3(a) Monthly cumulative precipitation given by the Tropical Rainfall Measuring Mission (TRMM) for the K34 tower domain in 2013. (b) Monthly averages of PAR and (c) air temperature, both measured every 30 min during 06:00–18:00 local time at the K34 tower site in 2013. (d) Isoprene flux measured with the REA system at the K34 tower site in 2013 and OMI satellite-derived isoprene flux for the K34 tower region.
As opposed to the average (2005–2013) flux peaking in September, the 2013 results suggest a maximum in October and are found to be substantially lower during the 2013 dry season compared to the average of the dry season estimates (reduction of ∼ 31 %; Fig. 2c). The timing of the maximum is not supported by the ground-based observations, peaking in September, but the magnitudes of flux estimates in these two months are in good agreement. In the wet-to-dry transition period, the small reduction in satellite-based isoprene fluxes in July 2013, compared to the neighboring months, is corroborated by a similar behavior in the ground-based isoprene fluxes (Fig. 3d). However, the drop in the observations is much stronger than in the top-down estimates (factor of 3 vs. a 70 % difference).
In contrast to satellite-derived fluxes, ground-based isoprene fluxes measured with the REA system have not shown significant correlation with PAR and air temperature for the year 2013 (Table 2 and Fig. 3). Ground-based isoprene fluxes also showed maximum emissions during the dry season (September), but emissions remained high in the beginning of the wet season (December), which was not observed in the seasonal behavior of PAR and air temperature. When averages of air temperature and PAR measured only during the same days of REA isoprene flux measurements were compared to isoprene fluxes, the correlation coefficients increased, but were still not statistically significant (Table 2).
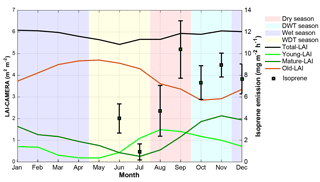
Figure 4CAMERA-LAI derived for the K34 tower site. CAMERA-LAI data are presented in three different leaf age classes: young LAI, mature LAI, and old LAI. Error bars represent 1 standard deviation from the mean. Background color shadings indicate each season and are explicit in the legend. DWT season and WDT season stand for the dry-to-wet transition season and the wet-to-dry transition season, respectively.
The forest leaf quantity, shown as leaf area index (LAI), varied little over the year when the total LAI was examined. However, when total LAI was fractionated into three different leaf age classes, namely young LAI (≤ 2 months), mature LAI (3–5 months), and old LAI (≥ 6 months), seasonal variation in each age class appears (Fig. 4). To understand how those LAI age fractions are related to the isoprene seasonality, ground-based fluxes of this compound were compared to the LAI age fractions estimated over the entire year (Fig. 4). The highest emissions were observed when the number of trees with mature leaves (mature LAI) was increasing and the number of trees with old leaves (old LAI) was decreasing. Considering seasonal changes in PAR, air temperature, and mature LAI, the latter presented the highest correlation coefficient, explaining 59 % of the seasonal isoprene emission variations (Table 2).
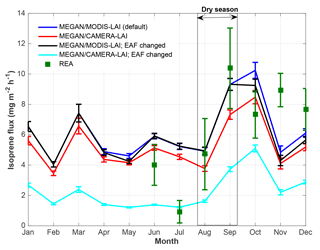
Figure 5Isoprene flux observed (REA) and estimated with MEGAN 2.1 default mode, leaf age algorithm driven by MODIS-LAI, and with MEGAN 2.1 leaf age algorithm driven by CAMERA-LAI. EAF stands for emission activity factor, which was changed for the different leaf age classes based on emissions of E. coriacea (Alves et al., 2014).
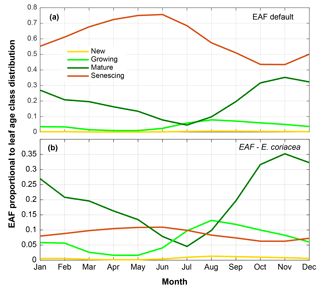
Figure 6(a) Emission activity factor (EAF) of isoprene for each leaf age class assigned in the default mode of MEGAN 2.1 proportional to leaf age class distribution derived from field observations (CAMERA-LAI). (b) Isoprene EAF for each leaf age class obtained from leaf-level measurements of the tree species E. coriacea, proportional to leaf age class distribution derived from field observations (CAMERA-LAI). Observations of the tree species E. coriacea (Alves et al., 2014) and CAMERA-LAI are both from the K34 site.
Isoprene flux simulations carried out with MEGAN 2.1 reveal similarities to the magnitudes observed during several months. But, MEGAN 2.1 did not fully capture the observed seasonal behavior (Fig. 5). Even though the leaf age algorithm of MEGAN 2.1 was parameterized with local leaf phenology observations, giving the highest correlation coefficient with observed fluxes (Table 2), isoprene flux simulations with local CAMERA-LAI inputs showed only a reduction in isoprene flux magnitudes. The seasonal behavior observed was the same as in the estimates from the default MEGAN 2.1 with MODIS-LAI inputs. Regressions between averages of observations and MEGAN 2.1 estimates, with CAMERA-LAI and MODIS-LAI inputs, were weak and not statistically significant (Table 2).
As a sensitivity test, observations of isoprene emission capacity at different leaf ages of a central Amazonian hyper-dominant tree species, Eschweilera coriacea (Alves et al., 2014), were used to parameterize the MEGAN 2.1 leaf age algorithm. Leaf-level measurements of isoprene emission capacity are scarce in Amazonia. To the best of the authors' knowledge, Alves et al. (2014) provide the only available data on leaf-level isoprene emission capacity at different leaf ages of a central Amazonian tree species, which were therefore used for the MEGAN sensitivity test.
Further simulations were performed with modifications in the leaf age emission activity factor (EAF). The EAF is dimensionless and defined as the emissions relative to the emissions of mature leaves, which by definition are set equal to 1. A new EAF was assigned for each age class based on observations of emissions of E. coriacea (Fig. 6). Leaf age fraction distribution was provided with LAI input from MODIS (MODIS-LAI) and from LAI-derived field observations (CAMERA-LAI; Fig. 4). The simulation with the leaf age algorithm parameterized for EAF changes and with MODIS-LAI was similar to the one without changes in the EAF (MEGAN 2.1 default). The simulation with leaf age algorithm parameterized with changes in the EAF and with CAMERA-LAI inputs showed reduced emissions, but a seasonal curve closer to that of isoprene flux observed at K34 (R2=0.52, p<0.05; Table 2).
This study addressed two main questions with respect to the seasonality of isoprene fluxes in central Amazonia and identified possible limitations in our current understanding related to these questions.
4.1 How much can seasonal isoprene fluxes be explained by variations in solar radiation, temperature, and leaf phenology?
Our finding that isoprene emissions are higher during the warmer season is consistent with previous findings that emissions from tropical tree species are light dependent and stimulated by high temperatures (Alves et al., 2014; Harley et al., 2004; Jardine et al., 2014; Kuhn et al., 2002, 2004a, b). Indeed, satellite-derived isoprene fluxes (2005–2013) were well correlated with PAR and even more with air temperature for all years. However, high ground-based isoprene emissions were observed until late in the dry-to-wet transition season, when mean PAR and air temperature were already decreasing.
The reasons why satellite-derived isoprene fluxes are weakly correlated with ground-based isoprene fluxes can be attributed to either the difference in the studied scales (e.g., local effects could have major influences on ground-based isoprene fluxes) and/or the uncertainties associated with the methodologies used to estimate or calculate fluxes. The high correlation between satellite-based fluxes and air temperature or PAR is not unexpected because higher temperatures and solar radiation fluxes favor isoprene emissions. Note, however, that the satellite-derived fluxes might also be subject to inherent uncertainties due to the existence of other HCHO sources, in particular biomass burning (during the dry season) and methane oxidation. Since these latter contributions are favored by high temperature and radiation levels, they could possibly contribute to the high correlation found between satellite-based isoprene and meteorological variables.
For the ground-based emissions, isoprene fluxes were determined by REA measurements that were carried out for 6 days per month. Therefore, the low correlation between ground-based isoprene fluxes and air temperature and PAR could partially result from limited qualified data.
Another factor correlated with ground-based isoprene fluxes is leaf phenology (in this study, LAI fractionated into age classes). The ground-based isoprene fluxes correlated better with variation in mature LAI than other factors (K34 site – R2=59 %, p<0.05), suggesting that the increasing isoprene emissions could partially follow the increasing of mature leaves (Fig. 4). Wu et al. (2016) suggested that leaf demography (canopy leaf age composition) and leaf ontogeny (age-dependent photosynthetic efficiency) are the main reasons for the seasonal variation of the ecosystem photosynthetic capacity in Amazonia. Photosynthesis supplies the carbon to the methyl erythritol phosphate pathway to produce isoprene (Delwiche and Sharkey, 1993; Harley et al., 1999; Lichtenthaler et al., 1997; Loreto and Sharkey, 1993; Rohmer, 2008; Schwender et al., 1997), and isoprene emissions are strongly dependent on leaf ontogenetic stage due to the developmental patterns of isoprene synthase activity that gradually increases with leaf maturation and decreases with leaf senescence (Alves et al., 2014; Kuzma and Fall, 1993; Mayrhofer et al., 2005; Monson et al., 1994; Niinemets et al., 2004, 2010; Schnitzler et al., 1997). Therefore, seasonal changes in the forest leaf age fractions may also influence the seasonality of isoprene emissions, suggesting higher emissions in the presence of more mature leaves and during high ecosystem photosynthetic capacity efficiency.
Understanding the correlations among light, temperature, leaf phenology (LAI fractionated into age classes), and isoprene is not straightforward. The weak correlation of seasonal changes between isoprene and light and temperature might be due to seasonal changes in the isoprene dependency on environmental factors and biological factors. Light and temperature peaked in the dry season; mature LAI, gross primary productivity (GPP), and photosynthetic capacity peaked in the wet season (Wu et al., 2016), and ground-based isoprene fluxes were high from the end of the dry to the dry-to-wet transition seasons. This might suggest that isoprene emissions are stimulated by light and high temperature during the beginning of the dry season and offset by the lower amount of mature leaves. During the wet season, isoprene emissions could be stimulated by the higher abundance of mature leaves and offset by the lower light availability and lower temperature. But, at the end of the dry and at dry-to-wet transition seasons, there is a combination of increased light and high temperature with a large amount of mature leaves, possibly favoring high isoprene emissions.
This is supported by findings of a temperate plant species showing that LAI dependency (changes in leaf age) was the most important factor affecting isoprene emission capacity, but when LAI decreased and senescence started at the end of the summer, the isoprene dependency on PAR and air temperature was as high as the period when PAR and air temperature reached their maximum (Brilli et al., 2016). This shows seasonal variation in the strength of dependency on each factor that affects emissions.
As discussed above, separating the effects of changing temperature and light from leaf phenology in canopy isoprene fluxes could allow for a more accurate quantification and a better understanding of seasonal isoprene flux. Here, we indicate that leaf phenology plays an important role in the seasonal variation of isoprene emissions, especially because different leaf ages present different isoprene emission capacity and the proportion of leaf age changes seasonally in Amazonia. However, when air temperature is the highest, isoprene emissions could be more stimulated by this factor, even though mature LAI is still not at its maximum. We suggest future research to verify whether tree species that present a regular seasonal leaf flushing are isoprene emitters and the strength of those emissions by leaf age.
4.2 How can a consideration of leaf phenology observed in the field help to improve model estimates of seasonal isoprene emissions?
Modeling of isoprene emissions from the Amazonian rainforest has been carried out for around 30 years. The first models were simplified and parameterized with observations from a few short field campaigns (see Table 1 of Alves et al., 2016). With the increase in available data, more driving forces of isoprene emissions were accounted for in the latest versions of models, as the case of MEGAN 2.1, which has been improved with a multilayer canopy model that accounts for light interception and leaf temperature within the canopy and includes changes in emissions due to leaf age that are typically driven by satellite retrievals of LAI development (Guenther et al., 2012).
The results presented here are from MEGAN 2.1 estimates with local observations of PAR, air temperature, and satellite-based leaf phenology. Initially, the default MEGAN 2.1 simulations did not fully capture the seasonal pattern of observed isoprene emissions, with nonsignificant correlation between model estimates and observations (R2=0.16, P>0.05; Table 2). This could be due to the near saturation of LAI seasonality in Amazonian evergreen forests and poor representation of leaf age effect on the isoprene emission capacity of tropical tree species in the default MEGAN 2.1. Furthermore, by using the camera-derived LAI phenology and the leaf age demographics to update the leaf age algorithm of the default MEGAN 2.1, we improved estimates of the proportion of leaves in different leaf age categories for the site, but there were a lack of observations for assigning the relative isoprene emission capacity for each age class.
It has been suggested that MEGAN uncertainties are mostly related to the short-term and long-term seasonality of the isoprene emission capacity (Niinemets et al., 2010). For instance, for an Asian tropical forest, isoprene emission capacity was reported to be 4 times lower than the default value of MEGAN (Langford et al., 2010), whereas aircraft flux measurements in the Amazon were 35 % higher than the MEGAN values (Gu et al., 2017) and satellite retrievals suggested significantly lower isoprene emissions (30–40 % in Amazonia and northern Africa) with respect to the MEGAN–MOHYCAN database (Bauwens et al., 2016). These all demonstrate that isoprene emission capacity is not well represented in the model for regions where there are few or no measurements.
For a sensitivity test, we parameterized the MEGAN 2.1 leaf age algorithm with observed isoprene emission capacity among different leaf ages of E. coriacea (Alves et al., 2014). The resulting simulation showed that by knowing the leaf age class distribution and the isoprene emission capacity for each age class, MEGAN 2.1 estimates can be improved and better agree with observations in terms of seasonal behavior. To date, there is very little information about isoprene emission capacity for different leaf ages of Amazonian plant species (Alves et al., 2014; Kuhn et al., 2004b). The scarcity of observational studies in the field, along with the huge biodiversity and heterogeneity of the Amazonian ecosystems, creates a challenge to optimize the isoprene emission capacity parameterization in MEGAN and other models. Therefore, while introducing local seasonal changes in canopy leaf age fractions in the model should improve estimates, seasonal variations in isoprene emission capacity also need to be characterized to better represent the effects of leaf phenology on tropical ecosystem isoprene emissions.
4.3 Possible limitations
This study correlates available data with different scales and approaches. Thus, there are limitations that need to be considered. One is the uncertainty related to the method used to measure ground-based isoprene fluxes. The uncertainties of the REA flux measurements ranged from 27.1 to 44.9 % (more details in Sect. S1 of the Supplement). However, this study shows the largest dataset of seasonal isoprene fluxes in Amazonia presented to date and the results presented here are similar to previous investigations when the same seasons are compared (see Table 1 of Alves et al., 2016).
Another limitation is the uncertainty of MEGAN estimates. It has been shown that models tend to agree with observations within ∼ 30 % for canopy-scale studies with site-specific parameters (Lamb et al., 1996). Here, part of the low correlation between observations and MEGAN 2.1 estimates is possibly due to short periods of measurements and data gaps. There were data gaps in PAR and temperature for a few months in 2013. This could influence the mean flux obtained from model estimates. Also, REA measurements were carried out in intensive campaigns of 6 days per month, which may not represent the flux for the entire month. Therefore, the limited data availability is still challenging our understanding of isoprene emission seasonality.
To understand the pattern of isoprene seasonal fluxes in Amazonia is a difficult task when considering the important role of Amazonian forests in accounting for global BVOC and very limited field-based observations in Amazonia. The seasonal variation of light and temperature is thought to primarily drive isoprene seasonal emissions. However, less notable factors in tropical forests might also influence ecosystem isoprene emission. Here, we suggest that leaf phenology, especially when accounting for the effect of leaf demography (canopy leaf age composition) and leaf ontogeny (age-dependent isoprene emission capacity), has an important effect on seasonal changes in the ecosystem isoprene emissions, which could play an even more important role in regulating ecosystem isoprene fluxes than light and temperature at a seasonal timescale in tropical forests. To the best of our knowledge, these results are the first to show the importance of leaf phenology on seasonal isoprene emissions in a tropical forest.
Although there are uncertainties related to measurements and modeling, the results presented here suggest that the unknown isoprene emission capacity for the different leaf age classes found in the forest may be the main reason why MEGAN 2.1 did not represent the observed seasonality of isoprene fluxes well. Additionally, some of these model uncertainties arise because of a lack of representation of canopy structure and light interception, including within-canopy variation in leaf functional traits, the leaf phenology within the canopy, the physical processes by which isoprene is transported within and above the forest canopy, chemical reactions that can take place within the canopy, and, the most difficult to assess, emission variation due to the huge biodiversity in Amazonia. Therefore, more detailed measurements of source and sink processes are encouraged to improve our understanding of the seasonality of isoprene emissions in Amazonia, which will improve surface emission models and subsequently lead to a better predictive vision of atmospheric chemistry, biogeochemical cycles, and climate.
Even though the data are still not available in any public repository, the data are available upon request from the first author.
The supplement related to this article is available online at: https://doi.org/10.5194/bg-15-4019-2018-supplement.
The authors declare that they have no conflict of interest.
The authors thank the National Institute for Amazonian Research (INPA) for
continuous support. We acknowledge the support by the Large Program of
Biosphere–Atmosphere Interactions (LBA) for the logistics and the
micrometeorological group for their collaboration concerning the
meteorological parameters. We acknowledge Kolby Jardine for providing the
gas standard to calibrate the analytical system and Paula Regina Corain
Lopes for the help with the fieldwork. Jin Wu is supported by the DOE–BER-funded
NGEE-Tropics project (contract no. DE- SC00112704) through Brookhaven National
Laboratory.
Edited by: David Bowling
Reviewed by: two anonymous referees
Adams, D. K., Fernandes, R. M. S., and Maia, J. M. F.: GNSS Precipitable Water Vapor from an Amazonian Rain Forest Flux Tower, J. Atmos. Ocean. Tech., 28, 1192–1198, https://doi.org/10.1175/JTECH-D-11-00082.1, 2011.
Adams, D. K., Gutman, S. I., Holub, K. L., and Pereira, D. S.: GNSS observations of deep convective time scales in the Amazon, Geophys. Res. Lett., 40, 2818–2823, 2013.
Adams, D. K., Fernandes, R. M. S., Holub, K. L., Gutman, S. I., Barbosa, H. M. J., Machado, L. A. T., Calheiros, A. J. P., Bennett, R. A., Robert Kursinski, E., Sapucci, L. F., Demets, C., Chagas, G. F. B., Arellano, A., Filizola, N., Rocha, A. A. A., Silva, R. A., Assunção, L. M. F., Cirino, G. G., Pauliquevis, T., Portela, B. T. T., Sá, A., De Sousa, J. M., and Tanaka, L. M. S.: The Amazon dense GNSS meteorological network: a new approach for examining water vapor and deep convection interactions in the Tropics, B. Am. Meteorol. Soc., 96, 2151–2165, https://doi.org/10.1175/BAMS-D-13-00171.1, 2015.
Alves, E. G., Harley, P., Gonçalves, J. F. C., Moura, C. E. S., and Jardine, K.: Effects of light and temperature on isoprene emission at different leaf developmental stages of eschweilera coriacea in central amazon, Acta Amaz., 44, 9–18, https://doi.org/10.1590/S0044-59672014000100002, 2014.
Alves, E. G., Jardine, K., Tota, J., Jardine, A., Yãnez-Serrano, A. M., Karl, T., Tavares, J., Nelson, B., Gu, D., Stavrakou, T., Martin, S., Artaxo, P., Manzi, A., and Guenther, A.: Seasonality of isoprenoid emissions from a primary rainforest in central Amazonia, Atmos. Chem. Phys., 16, 3903–3925, https://doi.org/10.5194/acp-16-3903-2016, 2016.
Andreae, M. O., Artaxo, P., Brandão, C., Carswell, F. E., Ciccioli, P., Da Costa, a. L., Gulf, A. D., Esteves, J. L., Gash, J. H. C., Grace, J., Kabat, P., Lelieveld, J., Malhi, Y., Manzi, A. O., Meixner, F. X., Nobre, a. D., Nobre, C., Ruivo, M. D. L. P., Silva-Dias, M. A., Stefani, P., Valentini, R., Von Jouanne, J., and Waterloo, M. J.: Biogeochemical cycling of carbon, water, energy, trace gases, and aerosols in Amazonia: The LBA-EUSTACH experiments, J. Geophys. Res.-Atmos., 107, D20, https://doi.org/10.1029/2001JD000524, 2002.
Arneth, A., Monson, R. K., Schurgers, G., Niinemets, Ü., and Palmer, P. I.: Why are estimates of global terrestrial isoprene emissions so similar (and why is this not so for monoterpenes)?, Atmos. Chem. Phys., 8, 4605–4620, https://doi.org/10.5194/acp-8-4605-2008, 2008.
Artaxo, P., Rizzo, L. V, Brito, J. F., Barbosa, H. M. J., Arana, A., Sena, E. T., Cirino, G. G., Bastos, W., Martin, S. T., and Andreae, M. O.: Atmospheric aerosols in Amazonia and land use change: from natural biogenic to biomass burning conditions, Faraday Discuss., 165, 203, https://doi.org/10.1039/c3fd00052d, 2013.
Barkley, M. P., Palmer, P. I., Kuhn, U., Kesselmeier, J., Chance, K., Kurosu, T. P., Martin, R. V., Helmig, D., and Guenther, A.: Net ecosystem fluxes of isoprene over tropical South America inferred from Global Ozone Monitoring Experiment (GOME) observations of HCHO columns, J. Geophys. Res., 113, D20304, https://doi.org/10.1029/2008JD009863, 2008.
Barkley, M. P., Palmer, P. I., De Smedt, I., Karl, T., Guenther, A., and Van Roozendael, M.: Regulated large-scale annual shutdown of Amazonian isoprene emissions?, Geophys. Res. Lett., 36, L04803, https://doi.org/10.1029/2008GL036843, 2009.
Barkley, M. P., De Smedt, I., Van Roozendael, M., Kurosu, T. P., Chance, K., Arneth, A., Hagberg, D., Guenther, A., Paulot, F., Marais, E., and Mao, J.: Top-down isoprene emissions over tropical South America inferred from SCIAMACHY and OMI formaldehyde columns, J. Geophys. Res.-Atmos., 118, 6849–6868, https://doi.org/10.1002/jgrd.50552, 2013.
Bauwens, M., Stavrakou, T., Müller, J.-F., De Smedt, I., Van Roozendael, M., van der Werf, G. R., Wiedinmyer, C., Kaiser, J. W., Sindelarova, K., and Guenther, A.: Nine years of global hydrocarbon emissions based on source inversion of OMI formaldehyde observations, Atmos. Chem. Phys., 16, 10133–10158, https://doi.org/10.5194/acp-16-10133-2016, 2016.
Bauwens, M., Stavrakou, T., Müller, J.-F., Van Schaeybroeck, B., De Cruz, L., De Troch, R., Giot, O., Hamdi, R., Termonia, P., Laffineur, Q., Amelynck, C., Schoon, N., Heinesch, B., Holst, T., Arneth, A., Ceulemans, R., Sanchez-Lorenzo, A., and Guenther, A.: Recent past (1979–2014) and future (2070–2099) isoprene fluxes over Europe simulated with the MEGAN–MOHYCAN model, Biogeosciences, 15, 3673–3690, https://doi.org/10.5194/bg-15-3673-2018, 2018.
Bowling, D. R., Turnipseed, A. A., Delany, A. C., Baldocchi, D. D., Greenberg, J. P., and Monson, R. K.: The use of relaxed eddy accumulation to measure biosphere-atmosphere exchange of isoprene and other biological trace gases, Oecologia, 116, 306–315, https://doi.org/10.1007/s004420050592, 1998.
Brilli, F., Gioli, B., Fares, S., Terenzio, Z., Zona, D., Gielen, B., Loreto, F., Janssens, I. A., and Ceulemans, R.: Rapid leaf development drives the seasonal pattern of volatile organic compound (VOC) fluxes in a “coppiced” bioenergy poplar plantation, Plant Cell Environ., 39, 539–555, https://doi.org/10.1111/pce.12638, 2016.
Claeys, M., Graham, B., Vas, G., Wang, W., Vermeylen, R., Pashynska, V., Cafmeyer, J., Maenhaut, W., Guyon, P., Andreae, M. O., and Artaxo, P.: Formation of secondary organic aerosols through photooxidation of isoprene, Science, 303, 1173–1176, https://doi.org/10.1126/science.1092805, 2004.
Cuartas, L. A., Tomasella, J., Nobre, A. D., Nobre, C. A., Hodnett, M. G., Waterloo, M. J., Oliveira, S. M. De, Randow, R. D. C. Von, Trancoso, R., and Ferreira, M.: Distributed hydrological modeling of a micro-scale rainforest watershed in Amazonia: Model evaluation and advances in calibration using the new HAND terrain model, J. Hydrol., 462–463, 15–27, https://doi.org/10.1016/j.jhydrol.2011.12.047, 2012.
de Araújo, A. C., Dolman, A. J., Waterloo, M. J., Gash, J. H. C., Kruijt, B., Zanchi, F. B., de Lange, J. M. E., Stoevelaar, R., Manzi, A. O., and Nobre, A. D.: The spatial variability of CO2 storage and the interpretation of eddy covariance fluxes in central Amazonia, Agr. Forest Meteorol., 150, 226–237, https://doi.org/10.1016/j.agrformet.2009.11.005, 2010.
Delwiche, C. F. and Sharkey, T. D.: Rapid appearance of 13C in biogenic isoprene when 13CO2 is fed to intact leaves, Plant Cell Environ., 16, 587–591, https://doi.org/10.1111/j.1365-3040.1993.tb00907.x, 1993.
Goldstein, A. H. and Galbally, I. E.: Known and Unexplored Organic Constituents in the Earth's Atmosphere, Environ. Sci. Technol., 41, 1514–1521, https://doi.org/10.1021/es072476p, 2007.
Gu, D., Guenther, A. B., Shilling, J. E., Yu, H., Huang, M., Zhao, C., Yang, Q., Martin, S. T., Artaxo, P., Kim, S., Seco, R., Stavrakou, T., Longo, K. M., Tóta, J., de Souza, R. A. F., Vega, O., Liu, Y., Shrivastava, M., Alves, E. G., Santos, F. C., Leng, G., and Hu, Z.: Airborne observations reveal elevational gradient in tropical forest isoprene emissions, Nat. Commun., 8, 15541, https://doi.org/10.1038/ncomms15541, 2017.
Guenther, A., Karl, T., Harley, P., Wiedinmyer, C., Palmer, P. I., and Geron, C.: Estimates of global terrestrial isoprene emissions using MEGAN (Model of Emissions of Gases and Aerosols from Nature), Atmos. Chem. Phys., 6, 3181–3210, https://doi.org/10.5194/acp-6-3181-2006, 2006.
Guenther, A. B., Jiang, X., Heald, C. L., Sakulyanontvittaya, T., Duhl, T., Emmons, L. K., and Wang, X.: The Model of Emissions of Gases and Aerosols from Nature version 2.1 (MEGAN2.1): an extended and updated framework for modeling biogenic emissions, Geosci. Model Dev., 5, 1471–1492, https://doi.org/10.5194/gmd-5-1471-2012, 2012.
Harley, P., Monson, R., and Lerdau, M.: Ecological and evolutionary aspects of isoprene emission from plants, Oecologia, 118, 109–123, 1999.
Harley, P., Vasconcellos, P., Vierling, L., Pinheiro, C. C. D. S., Greenberg, J., Guenther, A., Klinger, L., Almeida, S. S. De, Neill, D., Baker, T., Phillips, O., Malhi, Y., and De Almeida, S. S.: Variation in potential for isoprene emissions among Neotropical forest sites, Glob. Change Biol., 10, 630–650, https://doi.org/10.1111/j.1529-8817.2003.00760.x, 2004.
Huete, A. R., Didan, K., Shimabukuro, Y. E., Ratana, P., Saleska, S. R., Hutyra, L. R., Yang, W., Nemani, R. R., and Myneni, R.: Amazon rainforests green-up with sunlight in dry season, Geophys. Res. Lett., 33, 2–5, https://doi.org/10.1029/2005GL025583, 2006.
Jardine, K., Chambers, J., Alves, E. G., Teixeira, A., Garcia, S., Holm, J., Higuchi, N., Manzi, A., Abrell, L., Fuentes, J. D., Nielsen, L. K., Torn, M. S., and Vickers, C. E.: Dynamic balancing of isoprene carbon sources reflects photosynthetic and photorespiratory responses to temperature stress, Plant Physiol., 166, 2051–2064, https://doi.org/10.1104/pp.114.247494, 2014.
Kesselmeier, J., Ciccioli, P., Kuhn, U., Stefani, P., Biesenthal, T., Rottenberger, S., Wolf, A., Vitullo, M., Valentini, R., Nobre, A., Kabat, P., and Andreae, M. O.: Volatile organic compound emissions in relation to plant carbon fixation and the terrestrial carbon budget, Global Biogeochem. Cy., 16, 73-1–73-9, https://doi.org/10.1029/2001GB001813, 2002.
Kuhn, U., Rottenberger, S., Biesenthal, T., Wolf, A., Schebeske, G., Ciccioli, P., Brancaleoni, E., Frattoni, M., Tavares, T. M., and Kesselmeier, J.: Isoprene and monoterpene emissions of Amazonian tree species during the wet season: Direct and indirect investigations on controlling environmental functions, J. Geophys. Res., 107, 8071, https://doi.org/10.1029/2001jd000978, 2002.
Kuhn, U., Rottenberger, S., Biesenthal, T., Wolf, A., Schebeske, G., Ciccioli, P., Brancaleoni, E., Frattoni, M., Tavares, T. M., and Kesselmeier, J.: Seasonal differences in isoprene and light-dependent monoterpene emission by Amazonian tree species, Glob. Change Biol., 10, 663–682, https://doi.org/10.1111/j.1529-8817.2003.00771.x, 2004a.
Kuhn, U., Rottenberger, S., Biesenthal, T., Wolf, A., Schebeske, G., Ciccioli, P., and Kesselmeier, J.: Strong correlation between isoprene emission and gross photosynthetic capacity during leaf phenology of the tropical tree species Hymenaea courbaril with fundamental changes in volatile organic compounds emission composition during early leaf development, Plant Cell Environ., 27, 1469–1485, https://doi.org/10.1111/j.1365-3040.2004.01252.x, 2004b.
Kuzma, J. and Fall, R.: Leaf Isoprene Emission Rate Is Dependent on Leaf Development and the Level of Isoprene Synthase, Plant Physiol., 101, 435–440, 1993.
Lamb, B., Pierce, T., Baldocchi, D., Allwine, E., Dilts, S., Westberg, H., Geron, C., Guenther, A., Klinger, L., Harley, P., and Zimmerman, P.: Evaluation of forest canopy models for estimating isoprene emissions, J. Geophys. Res., 101, 22787–22797, https://doi.org/10.1029/96JD00056, 1996.
Langford, B., Misztal, P. K., Nemitz, E., Davison, B., Helfter, C., Pugh, T. A. M., MacKenzie, A. R., Lim, S. F., and Hewitt, C. N.: Fluxes and concentrations of volatile organic compounds from a South-East Asian tropical rainforest, Atmos. Chem. Phys., 10, 8391–8412, https://doi.org/10.5194/acp-10-8391-2010, 2010.
Lichtenthaler, H. K., Rohmer, M., and Schwender, J.: Two independent biochemical pathways for isopentenyl diphosphate and isoprenoid biosynthesis in higher plants, Physiol. Plant., 101, 643–652, https://doi.org/10.1111/j.1399-3054.1997.tb01049.x, 1997.
Lopes, A. P., Nelson, B. W., Wu, J., Graça, P. M. L. de A., Tavares, J. V., Prohaska, N., Martins, G. A., and Saleska, S. R.: Leaf flush drives dry season green-up of the Central Amazon, Remote Sens. Environ., 182, 90–98, https://doi.org/10.1016/j.rse.2016.05.009, 2016.
Loreto, F. and Sharkey, T. D.: On the relationship between isoprene emission and photosynthetic metabolites under different environmental conditions, Planta, 189, 420–424, https://doi.org/10.1007/BF00194440, 1993.
Machado, L. A. T., Laurent, H., Dessay, N., and Miranda, I.: Seasonal and diurnal variability of convection over the Amazonia: A comparison of different vegetation types and large scale forcing, Theor. Appl. Climatol., 78, 61–77, https://doi.org/10.1007/s00704-004-0044-9, 2004.
Mayrhofer, S., Teuber, M., Zimmer, I., Louis, S., Fischbach, R. J., and Schnitzler, J.-P.: Diurnal and seasonal variation of isoprene biosynthesis-related genes in grey poplar leaves, Plant Physiol., 139, 474–484, https://doi.org/10.1104/pp.105.066373, 2005.
Monson, R. K., Harley, P. C., Litvak, M. E., Wildermuth, M., Guenther, A. B., Zimmerman, P. R., and Fall, R.: Environmental and developmental controls over the seasonal pattern of isoprene emission from aspen leaves, Oecologia, 99, 260–270, https://doi.org/10.1007/BF00627738, 1994.
Morton, D. C., Nagol, J., Carabajal, C. C., Rosette, J., Palace, M., Cook, B. D., Vermote, E. F., Harding, D. J., and North, P. R. J.: Amazon forests maintain consistent canopy structure and greenness during the dry season, Nature, 506, 221–224, https://doi.org/10.1038/nature13006, 2014.
Myneni, R.: MCD15A2H MODIS/Terra+Aqua Leaf Area Index/FPAR 8-day L4 Global 500 m SIN Grid V006, NASA EOSDIS Land Processes DAAC, https://doi.org/10.5067/modis/mcd15a2h.006, 2015.
Myneni, R. B., Yang, W., Nemani, R. R., Huete, A. R., Dickinson, R. E., Knyazikhin, Y., Didan, K., Fu, R., Negrón Juárez, R. I., Saatchi, S. S., Hashimoto, H., Ichii, K., Shabanov, N. V, Tan, B., Ratana, P., Privette, J. L., Morisette, J. T., Vermote, E. F., Roy, D. P., Wolfe, R. E., Friedl, M. A., Running, S. W., Votava, P., El-Saleous, N., Devadiga, S., Su, Y., and Salomonson, V. V: Large seasonal swings in leaf area of Amazon rainforests, P. Natl. Acad. Sci. USA, 104, 4820–4823, https://doi.org/10.1073/pnas.0611338104, 2007.
Nelson, B., Tavares, J. V., Wu, J., Lopes, A. P., Marostica, S., Martins, G., Prohaska, N., Albert, L. P., de Araújo, A. C., Manzi, A. O., Saleska, S., and Huete, A.: Seasonality of central Amazon Forest Leaf Flush using tower mounted RGB Camera, AGU Fall Meeting, San Francisco, California, 15–19 December 2014, B11G-0107, 2014.
Niinemets, U., Loreto, F., and Reichstein, M.: Physiological and physicochemical controls on foliar volatile organic compound emissions, Trends Plant Sci., 9, 180–186, https://doi.org/10.1016/j.tplants.2004.02.006, 2004.
Niinemets, Ü., Arneth, A., Kuhn, U., Monson, R. K., Peñuelas, J., and Staudt, M.: The emission factor of volatile isoprenoids: stress, acclimation, and developmental responses, Biogeosciences, 7, 2203–2223, https://doi.org/10.5194/bg-7-2203-2010, 2010.
Rennó, C. D., Nobre, A. D., Cuartas, L. A., Soares, J. V., Hodnett, M. G., Tomasella, J., and Waterloo, M. J.: HAND, a new terrain descriptor using SRTM-DEM: Mapping terra-firme rainforest environments in Amazonia, Remote Sens. Environ., 112, 3469–3481, https://doi.org/10.1016/j.rse.2008.03.018, 2008.
Rohmer, M.: From molecular fossils of bacterial hopanoids to the formation of isoprene units: Discovery and elucidation of the methylerythritol phosphate pathway, Lipids, 43, 1095–1107, https://doi.org/10.1007/s11745-008-3261-7, 2008.
Saleska, S. R., Wu, J., Guan, K., Araujo, A. C., Huete, A., Nobre, A. D., and Restrepo-Coupe, N.: Dry-season greening of Amazon forests, Nature, 531, https://doi.org/10.1038/nature16457, 2016.
Samanta, A., Ganguly, S., Hashimoto, H., Devadiga, S., Vermote, E., Knyazikhin, Y., Nemani, R. R., and Myneni, R. B.: Amazon forests did not green-up during the 2005 drought, Geophys. Res. Lett., 37, 1–5, https://doi.org/10.1029/2009GL042154, 2010.
Schnitzler, J.-P., Lehning, A., and Steinbrecher, R.: Seasonal Pattern of Isoprene Synthase Activity in Quercus robur Leaves and its Significance or Modeling Isoprene Emission Rates, Bot. Acta, 110, 240–243, 1997.
Schwender, J., Zeidler, J., Gröner, R., Müller, C., Focke, M., Braun, S., Lichtenthaler, F. W., and Lichtenthaler, H. K.: Incorporation of 1-deoxy-D-xylulose into isoprene and phytol by higher plants and algae, FEBS Lett., 414, 129–134, https://doi.org/10.1016/S0014-5793(97)01002-8, 1997.
Stavrakou, T., Müller, J.-F., De Smedt, I., Van Roozendael, M., van der Werf, G. R., Giglio, L., and Guenther, A.: Global emissions of non-methane hydrocarbons deduced from SCIAMACHY formaldehyde columns through 2003–2006, Atmos. Chem. Phys., 9, 3663–3679, https://doi.org/10.5194/acp-9-3663-2009, 2009.
Stavrakou, T., Müller, J.-F., Bauwens, M., De Smedt, I., Van Roozendael, M., Guenther, A., Wild, M., and Xia, X.: Isoprene emissions over Asia 1979–2012: impact of climate and land-use changes, Atmos. Chem. Phys., 14, 4587–4605, https://doi.org/10.5194/acp-14-4587-2014, 2014.
Stavrakou, T., Müller, J.-F., Bauwens, M., De Smedt, I., Van Roozendael, M., De Mazière, M., Vigouroux, C., Hendrick, F., George, M., Clerbaux, C., Coheur, P.-F., and Guenther, A.: How consistent are top-down hydrocarbon emissions based on formaldehyde observations from GOME-2 and OMI?, Atmos. Chem. Phys., 15, 11861–11884, https://doi.org/10.5194/acp-15-11861-2015, 2015.
Tóta, J., Fitzjarrald, D. R., and da Silva Dias, M. A. F.: Amazon rainforest exchange of carbon and subcanopy air flow: Manaus LBA site – a complex terrain condition, Sci. World J., 2012, 165067, https://doi.org/10.1100/2012/165067, 2012.
Wagner, F. H., Hérault, B., Rossi, V., Hilker, T., Maeda, E. E., Sanchez, A., Lyapustin, A. I., Galvão, L. S., Wang, Y., and Aragão, L. E. O. C.: Climate drivers of the Amazon forest greening, PLoS One, 12, 1–15, https://doi.org/10.1371/journal.pone.0180932, 2017.
Wu, J., Albert, L. P., Lopes, A. P., Restrepo-Coupe, N., Hayek, M., Wiedemann, K. T., Guan, K., Stark, S. C., Christoffersen, B., Prohaska, N., Tavares, J. V., Marostica, S., Kobayashi, H., Ferreira, M. L., Campos, K. S., Silva, R. da, Brando, P. M., Dye, D. G., Huxman, T. E., Huete, A. R., Nelson, B. W., and Saleska, S. R.: Leaf development and demography explain photosynthetic seasonality in Amazon evergreen forests, Science, 351, 972–976, https://doi.org/10.1126/science.aad5068, 2016.
Yáñez-Serrano, A. M., Nölscher, A. C., Williams, J., Wolff, S., Alves, E., Martins, G. A., Bourtsoukidis, E., Brito, J., Jardine, K., Artaxo, P., and Kesselmeier, J.: Diel and seasonal changes of biogenic volatile organic compounds within and above an Amazonian rainforest, Atmos. Chem. Phys., 15, 3359–3378, https://doi.org/10.5194/acp-15-3359-2015, 2015.