the Creative Commons Attribution 4.0 License.
the Creative Commons Attribution 4.0 License.
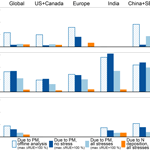
Resource and physiological constraints on global crop production enhancements from atmospheric particulate matter and nitrogen deposition
Colette L. Heald
David Kelly
Changing atmospheric composition, induced primarily by industrialization and climate change, can impact plant health and may have implications for global food security. Atmospheric particulate matter (PM) can enhance crop production through the redistribution of light from sunlight to shaded leaves. Nitrogen transported through the atmosphere can also increase crop production when deposited onto cropland by reducing nutrient limitations in these areas. We employ a crop model (pDSSAT), coupled to input from an atmospheric chemistry model (GEOS-Chem), to estimate the impact of PM and nitrogen deposition on crop production. In particular, the crop model considers the resource and physiological restrictions to enhancements in growth from these atmospheric inputs. We find that the global enhancement in crop production due to PM in 2010 under the most realistic scenario is 2.3, 11.0, and 3.4 % for maize, wheat, and rice, respectively. These crop enhancements are smaller than those previously found when resource restrictions were not accounted for. Using the same model setup, we assess the effect of nitrogen deposition on crops and find modest increases (∼ 2 % in global production for all three crops). This study highlights the need for better observations of the impacts of PM on crop growth and the cycling of nitrogen throughout the plant–soil system to reduce uncertainty in these interactions.
- Article
(2442 KB) - Companion paper
- BibTeX
- EndNote
Population growth is intensifying stress on global food production. Simultaneously, anthropogenic activities are changing many aspects of the earth system. This reinforces the need to better understand how crop production may be affected by changes to the water, air, light, and soil required for efficient growth. For example, Challinor et al. (2014) suggest a global decline in crop yield due to climate change of more than 10 % is likely by 2050. However, this is uncertain and the projected sign and magnitude varies by crop and region due to localized changes in factors such as temperature and precipitation combined with global carbon dioxide (CO2) enhancement (IPCC, 2014). Many studies have explored the impacts of climate and air quality on crop production, both globally and regionally, with various results depending on the tools, methods, and processes used by each (e.g., Burney and Ramanathan, 2014; Lobell and Burke, 2010; Shindell et al., 2011; Tai et al., 2014). Investigations of the impacts of air quality on crops, in particular, have focused mainly on the negative impact of ozone pollution (Avnery et al., 2011; Mills et al., 2011; Van Dingenen et al., 2009). In comparison, only limited work has been conducted to assess how atmospheric particulate matter (PM) impacts crop production (Greenwald et al., 2006; Schiferl and Heald, 2018), and this has been done without considering physiological limitations (e.g., rate and magnitude of carbon pool allocation) and other environmental stresses (e.g., water and nutrients).
Emitted from combustion and natural sources and formed through chemical oxidation in the atmosphere, PM is the leading cause of air quality issues globally and is responsible for over 4 million premature deaths per year (Cohen et al., 2017). PM also impacts crop production by modifying shortwave (SW) radiation reaching the surface. Through the scattering of light, PM decreases the total SW radiation at the earth's surface, which is made up of direct and diffuse light (SW = direct + diffuse). PM also increases the diffuse fraction (DF) of this SW radiation (DF = ). Increased DF more evenly distributes light throughout the canopy of a plant, redirecting light away from (at times over-saturated) leaves in direct sunlight and onto shaded leaves. In this way, plants can more efficiently make use of incoming solar radiation (Kanniah et al., 2012).
Previous studies of the impact of PM on plant productivity have largely focused on natural ecosystems, and forests in particular. Using network observations, Niyogi et al. (2004) showed that the CO2 sink, a measure of plant productivity, increases with PM, indicated by aerosol optical depth (AOD), over forests, but decreases for grasslands. More recent work related satellite AOD measurements to observations from CO2 flux towers to quantify the impact of diffuse light on plant productivity. In the Amazon forest, enhancements in net ecosystem exchange (NEE) of up to 29 % are observed when the DF reaches approximately 0.5 (Cirino et al., 2014). Strada et al. (2015) find an increase in midday gross primary productivity (GPP) of ∼ 13 % in US deciduous forests when DF is 0.4–0.6. Advanced canopy or leaf-scale process modeling has been used to further examine how PM impacts natural vegetation and the carbon cycle. The model framework of Strada and Unger (2016) shows little sensitivity in global total GPP (∼ 1–2 %) to PM pollution, with regional enhancements of ∼ 5–8 % in North America and Eurasia and ∼ 2 % in the Amazon, where forested canopies dominate. In China, Yue and Unger (2017) use AOD thresholds along with satellite observations and vegetation modeling to find the impact of PM pollution on net primary production (NPP) varies spatially from −3 to +6 %. When accounting for the direct impacts of PM on light, temperatures, and hydrology, Yue et al. (2017) find a net increase in NPP of 5 %.
The impact of PM on managed vegetation (crops) is less well studied than for natural vegetation. PM can increase growth and production of crops when the increase in efficiency outweighs the loss of SW radiation. This depends on the local light conditions (changes in SW vs. DF) and crop type (C3 vs. C4). C4 crops such as maize are less likely to be light saturated than C3 crops such as wheat. Niyogi et al. (2004) find that the CO2 sink increases over croplands with an increase in AOD. In contrast with their forest sites, Strada et al. (2015) find a decrease in midday GPP of ∼ 17 % associated with high observed AOD for a combination of US cropland and grassland sites. They attribute this difference to canopy architecture which minimizes leaf shading, and thus the impact of diffuse light, when the sun is overhead. Greenwald et al. (2006) use relationships between DF (determined by climatological AOD) and a crop's radiative use efficiency (RUE), a measure of how effective a plant converts light into carbon, from Sinclair et al. (1992) along with varying meteorology and a crop model to estimate the impact of PM on crop yield. Assuming no restrictions on growth due to stresses at several locations, they find a large variation in impacts based on the DF-to-ΔRUE relationship chosen. Under the maximum response relationship, maize increases by 0–10 %, wheat increases by 0–5 % and rice increases by 0–40 % under varying cloud conditions (Greenwald et al., 2006). Using this approach, but with a combined atmospheric chemistry and radiative transfer model to better simulate spatial and temporal variability of PM impacts on radiation, Schiferl and Heald (2018) estimate a global positive impact of PM of 12, 16, and 9 % on maize, wheat, and rice production, respectively, for the year 2010. While this study uses a simple representation of the PM impacts on crop productivity, the approach isolates the impact of PM on crop production, which is not easily estimated based on previous observational analyses or mechanistic models. Observed AOD impacts on radiation are convolved with the influence of clouds, and as it is difficult to isolate only PM impacts, we cannot easily translate the observed AOD-to-carbon flux relationships to the impacts of DF on RUE. Mechanistic model studies account for all land biomass; however, such models do not differentiate between individual crop characteristics (e.g., canopies, growing seasons). Furthermore, the observed and simulated changes in NEE and GPP in these studies do not correspond directly to crop production (the harvested biomass), but rather on carbon uptake or release.
Industrial agriculture, driven by the need to produce food for a growing human population, has modified the global nitrogen (N) cycle (e.g., Bouwman et al., 2013; Smil, 1999). By artificially fixing inert nitrogen gas into reactive forms, humans have increased the fluxes of nitrogen throughout the environment, including into the atmosphere, onto land, and into the water (Galloway and Cowling, 2002). Nitrogen species in the atmosphere, both reduced and oxidized, return to the surface through deposition processes after being transported away from source regions. Anthropogenic influences on this deposition change the nitrogen balance in land and water ecosystems. In natural systems, this can cause acidification and eutrophication, which negatively impacts the biosphere (Beem et al., 2010; Erisman et al., 2007). Nitrogen accumulation into ecosystems from deposition reduces biodiversity; secondary factors such as direct toxicity, soil acidification, and increased susceptibility to stress can be dominant locally (Bobbink et al., 2010). While remaining substantially higher than during preindustrial time, current rates of nitrogen deposition have recently declined over the US and Europe but are expected to increase in developing countries in the future (Lamarque et al., 2013). This will contribute to projected (for 2050) nitrogen surpluses in Africa and Latin America corresponding with increases in crop and livestock production (Bouwman et al., 2013).
By including the coupling of carbon and nitrogen in a land-surface model, Thornton et al. (2007) show that GPP is limited by the supply of nitrogen to the biosphere and simulate over 40 % less GPP than a case that does not include this limitation. This carbon–nitrogen coupling dampens the response of vegetation to CO2 concentration increases by over 70 %. The addition of atmospheric nitrogen deposition in the coupled system increases global GPP by ∼ 2 %. When integrated into a fully coupled earth system model, there is a decrease in carbon uptake from CO2 fertilization and an increase in carbon uptake from climate warming from the interactions between carbon and nitrogen. This increase in carbon uptake is due to enhanced nitrogen mineralization in the soil from a higher rate of decomposition (Thornton et al., 2009). Thomas et al. (2013) show that these simulated carbon–nitrogen responses for forests are smaller than those observed. Their model modifications result in greater retention of nitrogen deposition in biomass and a tighter coupling between nitrogen deposition and rising atmospheric CO2 concentration. The model better represents observations by increasing the aboveground carbon storage response to nitrogen deposition.
Nitrogen deposition can also impact crop production, by providing additional fertilization, increasing yields in areas which are nitrogen limited (Goulding et al., 1998). These areas include portions of Africa, South America, India, and eastern Europe (J. Liu et al., 2010; Mueller et al., 2012). Liu et al. (2013) show that in China, nitrogen deposition leads to increased nitrogen uptake in non-fertilized croplands, resulting in a small increase in yield (1 t ha−1) derived from a nitrogen uptake to yield ratio. Lassaletta et al. (2014) develop relationships between observed total nitrogen input and crop yield on a countrywide basis, but they do not disaggregate the impacts of deposition saying only that the input from deposition is small, but not negligible. While Ladha et al. (2016) estimate that 6 % of nitrogen contained in global maize, wheat, and rice comes from deposited nitrogen, to date, there has been no global study of the change of yield associated with nitrogen deposition, with most studies concentrating on the impacts of nitrogen deposition on interactions with atmospheric CO2 and carbon storage. Folberth et al. (2016) neglect nitrogen deposition in their study of soil and meteorological data uncertainties in crop models due to the lack of available deposition data in a form suitable for global crop models.
Finally, PM and nitrogen deposition are connected: the release of excess nitrogen from fertilizer application and livestock production in the form of ammonia (NH3) contributes to PM formation in the atmosphere under acidic conditions (Seinfeld and Pandis, 2006). Nitric acid (HNO3), an oxidized form of nitrogen oxides (NOx) emissions from mobile and industrial sources, contributes both to the nitrogen burden and these acidic conditions. Nitrogen can also be incorporated into PM as organic nitrates when biogenic volatile organic compounds (BVOCs) react with NOx (Mao et al., 2013). Recent global modeling studies incorporate more complex nitrogen transformations and cycling, such as the implementation of bi-directional ammonia fluxes into atmospheric chemistry models (Zhu et al., 2015) and climate-dependant agricultural nitrogen pathways into earth system models (Riddick et al., 2016).
Schiferl and Heald (2018) quantify the impact that air quality (ozone and PM) has on current and future global crop production. Their analysis, while consistent with the approach generally applied to estimate air quality impacts on crops in previous studies mentioned above, fails to account for the set of physical and biological restrictions placed on crop growth and production. In particular, they consider crop production enhancement due to the diffuse effect of PM to be unlimited. However, water and nitrogen stresses and physiological caps placed on crop production may dampen these responses. This study is a direct follow-up to Schiferl and Heald (2018), where we employ a crop model to simulate the enhancements in crop production associated with PM and nitrogen deposition simulated by an atmospheric chemistry and radiative transfer model and explore the potential impact of resource and physiological constraints on this production.
The GEOS-Chem model (http://www.geos-chem.org, last access: June 2015) simulates the global concentration of gases and particles in three dimensions. Simulated PM concentrations are read into the Rapid Radiative Transfer Model for GCMs (RRTMG) to estimate the impact of PM on radiation throughout the atmosphere (Heald et al., 2014). Together these models are referred to as GC-RT. The model version and setup used here is the same as for the standard 2010 emissions scenario described by Schiferl and Heald (2018). In brief: v10-01 of GC-RT is run at 2∘ × 2.5∘ horizontal resolution using GEOS-5 meteorology for the years 2009 and 2010 from the NASA Global Modeling and Assimilation Office (GMAO). In this study, PM refers to the sum of all simulated aerosol species: sulfate (), nitrate (), ammonium (), black carbon (BC), organic carbon (OC), sea salt, and dust. Inorganic aerosol thermodynamics are coupled to an ozone–VOC–NOx–oxidant chemical mechanism, where ISORROPIA II (Fountoukis and Nenes, 2007) handles the gas–particle phase partitioning of ammonium nitrate. GC-RT simulates wet and dry deposition of both aerosols and gases (Amos et al., 2012; Liu et al., 2001; Wang et al., 1998; Zhang et al., 2001). Major global anthropogenic gas emissions come from the Emission Database for Global Atmospheric Research (EDGAR) v4.2 (NOx, carbon monoxide – CO, sulfur dioxide – SO2), the Reanalysis of the TROpospheric chemical composition (RETRO) inventory (non-methane VOCs; Hu et al., 2015), and the Global Emission Inventory Activity (GEIA) inventory (NH3). These are overlaid by regional inventories where available (see Schiferl and Heald, 2018, for details). Additional NOx emissions are from lightning and soil, described by Murray et al. (2012) and Hudman et al. (2012), respectively. Directly emitted aerosol sources include anthropogenic BC and OC (Bond et al., 2007; Leibensperger et al., 2012), dust (Fairlie et al., 2007), and sea salt (Jaeglé et al., 2011). GC-RT uses a bulk aerosol scheme, where each aerosol species is described by a fixed log-normal size distribution, the physical and optical properties of which are described in Heald et al. (2014) and are accounted for in the radiative transfer scheme. PM sizes in GC-RT span several orders of magnitude, with mean diameters that range, for example, from BC, 0.04 µm, to sulfate, 0.14 µm, to dust, 8 µm.
In this study, we use the hourly output of surface SW radiation and the diffuse and direct portions of this SW radiation from GC-RT both with and without PM under all-sky (real time variation in cloudiness) conditions. These are used to calculate the DF of the SW radiation. While SW and DF respond differently to the differing properties of each PM type, here we consider the net effect of all PM. The impacts of PM described in this study account only for the direct radiation changes through light absorption and scattering, and do not consider secondary feedbacks of aerosol on clouds, meteorology, and hydrology. We also use daily output of nitrogen deposition flux from the atmosphere, including the wet and dry deposition simulated for all nitrogen species. Nitrogen mass deposited from five species, ammonia, ammonium, nitric acid, nitrate, and nitrogen dioxide (NO2), make up 98 % of the total simulated nitrogen deposition for 2010. Both the PM impacts on surface radiation and the nitrogen deposition flux from the atmosphere are derived from the same GC-RT simulation, providing consistency over the emissions, chemistry, and deposition schemes described above.
3.1 Model description
We use the Decision Support System for Agrotechnology Transfer (DSSAT) v4.6 crop system model (Hoogenboom et al., 2015), along with the parallel System for Integrating Impact Models and Sectors (pSIMS) v2.0 (Elliott et al., 2014), together called pDSSAT, to simulate the global production of maize, wheat, and rice. DSSAT provides a unified interface which combines various crop simulation models (Jones et al., 2003). Inherently a point model, DSSAT uses daily meteorological data (minimum temperature, maximum temperature, precipitation, solar radiation, wind speed, and relative humidity) along with soil and management information at a given location. The model then calculates a crop yield at harvest taking into account soil–plant–atmosphere dynamics throughout the growing season. Plant growth, in our case, is determined by the Crop Environment Resource Synthesis (CERES) model module for each crop. CERES modules, developed separately for maize, wheat, and rice, simulate the carbon and nitrogen pools, among other parameters, associated with the various plant parts (e.g., leaves, stems, roots, grain) throughout the growth stages of each crop type. Potential dry matter (carbon) production is determined as a function of the solar radiation, SW (see Eq. 1). The actual dry matter production at each time step is limited by the effects of non-optimal temperature, water stress, and/or nitrogen stress, if applicable. Water and nitrogen stresses are determined by comparing the requirements of each crop with the amount of each resource available to the plant. Dry matter produced is then distributed into the plant parts based on those associated with the growth stage at that time. The sensitivity of growth rates and physical limitations for each plant part during each growth stage is determined by the physiology of that crop and cultivar (Jones et al., 1986; Ritchie et al., 1998; Ritchie and Otter, 1985). The simulation of these individual plant parts, rather than only total carbon, is of critical importance for this study as we are concerned with the production of grain to address impacts on food security. A recent review of CERES performances for maize, wheat, and rice finds that the models reproduce observed grain yield well, with relative errors of ∼ 10, ∼ 20, and ∼ 10 %, respectively (Basso et al., 2016). They also find that secondary parameters such as soil temperature and nitrogen cycling were much less well represented.
pSIMS allows for the globally gridded simulation of crop yield by running DSSAT in parallel at various grid boxes using consistent data and setting input methods (Elliott et al., 2014). In our study, we set pDSSAT to run at 0.5∘ × 0.5∘ horizontal resolution. This is only limited by the availability of suitable global input data. pDSSAT uses daily meteorological information from AgMERRA (Ruane et al., 2015), a version of the NASA Modern-Era Retrospective Analysis for Research and Applications (MERRA) product developed for use in the Agricultural Model Intercomparison and Improvement Project (AgMIP; Rosenzweig et al., 2013). We note that this meteorological product is closely related to the GEOS-5 product which drives the GC-RT simulations. Soil inputs come from the Global Soil Dataset for Earth System Modeling (GSDE; Shangguan et al., 2014). Additional required information includes the range of planting dates (Portmann et al., 2010; Sacks et al., 2010), distribution of cultivars (based on local growing degree days – GDD), and fertilizer application amounts at each grid box. We use fertilizer information from the Spatial Production Allocation Model (SPAM; You et al., 2012). We highlight that direct fertilizer application is the only source of nitrogen supplied to crops in the pDSSAT model in addition to the baseline nitrogen content in each soil layer given by GSDE. Except for the soil inputs, which are modified in pSIMS v2.0, the pDSSAT input data listed above are consistent with those used by the Global Gridded Crop Model Intercomparison (GGCMI) portion of AgMIP (Rosenzweig et al., 2014).
3.2 Integration of GEOS-Chem with pDSSAT
Using the hourly SW, diffuse, and direct radiation output from GC-RT, we calculate the daily mean daytime (SW > 0) SW and DF for each GEOS-Chem grid box (2∘ × 2.5∘ horizontal resolution) for all of 2009 and 2010. We group the nitrogen deposition fluxes of individual species into two groups, reduced nitrogen (NHx) and oxidized nitrogen (NOy), and calculate the daily total flux for each group for the same time period. The daily SW and DF values, along with the daily NHx and NOy deposition flux values, are regridded to the pDSSAT grid and resolution using area-weighted regridding and integrated into the input meteorology.
For the PM simulations, the daily SW and DF are used in the pDSSAT crop-specific plant growth modules to modify the potential carbon production. Following each crop-specific CERES growth module, Eq. (1) is used for maize and wheat, and Eq. (2) is used for rice:
where Pcarb is the potential carbon production, SW is the daily mean shortwave radiation from GC-RT, and RUEs is crop-specific radiation use efficiency (Ritchie et al., 1998). For simulations with PM affecting SW and DF, SW modified by PM from GC-RT is used as input for the relationships in Eqs. (1) and (2) only and is not used in other functions dependent on solar radiation, such as evaporation (i.e., the GC-RT SW without PM remains applied to these processes). In this study, we apply only the maximum DF-to-ΔRUE relationship discussed in Schiferl and Heald (2018) to modify the RUEs based on the DF, where max ΔRUE = 100 % at DF = 0.8 (Greenwald et al., 2006). This represents the upper limit of potential PM impacts on crop production. We note that additional processes which impact plant productivity, such as evapotranspiration and water use efficiency, have also been shown in both observations and simulations to be affected by changes in DF (Lu et al., 2017; Wang et al., 2008). These second-order effects may dominate the crop response under certain conditions and therefore should be included in any assessment of the overall environmental impacts on crop growth. However, the goal of this work is to explore only the direct impact of radiation changes (due to PM) on crop productivity, enabling a comparison with Schiferl and Heald (2018).
For the nitrogen deposition simulations, NHx and NOy fluxes are applied daily as an additional source of fertilizer to the surface layer of the soil as and , respectively, due to their similar behaviors in soils (Ladha et al., 2016). We apply these deposition fluxes beginning 30 days prior to the planting date at each location. The timing of this initiation is uncertain, as the fate of deposited nitrogen is not well constrained, and the impacts of nitrogen deposition can be assessed over a single growing season to multi-year timescales (Goulding et al., 1998). Our selection of 30 days is therefore somewhat arbitrary. We discuss the impact of this assumption in Sect. 4.2.
3.3 Base simulation
We configure pDSSAT to run for 2009 and 2010 with water and nitrogen stress turned off. Our modification for potential carbon production using input from GC-RT is applied to SW only (with SW values from GC-RT without PM). Maize, wheat, and rice are simulated independently. We sample the results for each crop for the growing season ending in 2010. For example, crops planted in Northern Hemisphere spring and harvested in fall are grown entirely within 2010, while winter crops are planted in fall 2009 and harvested in spring 2010. These planting and harvest dates are determined within pDSSAT by the life cycle characteristics of each crop and vary based on the location-specific meteorological (e.g., GDD, timing of rainfall) and resource (e.g., fertilizer amount, irrigated vs. rainfed) inputs for that simulation. For a consistent comparison, we determine crop production by multiplying the pDSSAT crop yield by the crop area from the Global Agro-Ecological Zones (GAEZ) assessment for 2000 (FAO, 2016) scaled to 2010 as in Schiferl and Heald (2018), rather than by using the internal pDSSAT harvested area parameter. The results from this simulation, our base simulation, are shown in Fig. 1. Also as in Schiferl and Heald (2018), our figures focus on the industrialized areas of the Northern Hemisphere, which rely heavily on maize, wheat, and rice, though all numbers presented are global. Since our base simulation has no restrictions on water and nitrogen (both the nitrogen supply and irrigation are unlimited), the simulated crop production vastly surpasses that from GAEZ. For maize, this is 2062 Tg from pDSSAT compared to only 871 Tg from GAEZ. Simulated wheat production is 2591 Tg, and simulated rice production is 1250 Tg compared to GAEZ values of 667 and 705 Tg, respectively.
We rerun the crop model with water stress only, nitrogen stress only, and both stresses together to characterize the sensitivity of the base simulation to these resources (Fig. 1). Water stress occurs when the amount of soil water available is below the potential transpiration rate of the plant. For maize, the negative effect of water stress on production is most evident in the US Plains and northern China and produces a 29 % production reduction globally. The effect of water stress is globally larger on wheat (40 % reduction), and is largest in the southern US plains, northern China, and throughout western Asia. Rice production is impacted the least by water stress, with only a 14 % reduction in production when imposing water stress, mostly in northern India. Water stress is dependent on the precipitation prescribed from the meteorology of that growing season, so these results will vary from year to year.
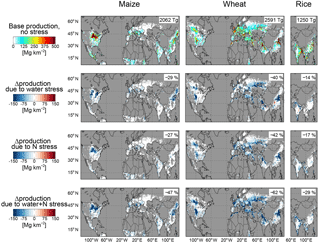
Figure 1Top row panels: crop production from base pDSSAT scenario (GC-RT SW only, no PM) with no stress applied for growing season ending in 2010. Difference in crop production due to water stress (second row panels), nitrogen (N) stress (third row panels), and both water and N stresses (bottom row panels). For each row, maize (left column panels), wheat (middle column panels), and rice (right column panels) are shown. Filtered for GAEZ base crop production greater than 0.01 Mg km−2. Global production (top row panels) or relative production change (second row–bottom row panels) shown in upper right of each map.
Nitrogen stress occurs when the plant tissue nitrogen concentration is less than the critical nitrogen concentration determined to provide optimal growth. In our base simulation, nitrogen stress follows different patterns compared to water stress for many regions and crops, although the global magnitudes in production reduction are similar. This response to carbon–nitrogen coupling is similar in sign and magnitude as that found for global GPP by Thornton et al. (2007). Maize production is affected by nitrogen stress primarily in the US plains and the American Midwest. Nitrogen stress for wheat is distributed into all regions, while the effect on rice production is again lowest globally, it is largest in Southeast (SE) Asia. We note that the apparent impact of nitrogen stress on maize in Midwestern US is magnified by the large crop area in this region. Nitrogen stress is more similar from year to year in the model as fertilizer application, which provides nitrogen to the soil, and inherent soil nitrogen content is identical for all simulation years. Small variations do exist as variable temperatures and radiation impact the onset of crop growth stages and use of nitrogen. Folberth et al. (2016) find that uncertainty in soil data can impact simulated crop yield variability more than meteorological variability, especially for no water stress (irrigated) and high nitrogen stress areas. In contrast, they find that irrigated areas with high nitrogen inputs show little difference between yield due to soil and meteorological input variability. Total production change due to both water and nitrogen stress does not combine linearly. This illustrates the interconnected system simulated by the crop model. Overall, these environmental and management constraints greatly reduce global crop production from its unstressed potential. They are important to consider when analyzing the impact of PM and nitrogen deposition on crop production.
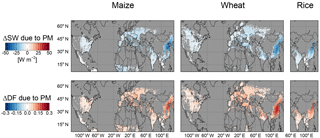
Figure 2Mean change in daytime (SW > 0) downward SW radiation (top row panels) and DF of the SW radiation (bottom row panels) at the surface due to PM from GC-RT. For pDSSAT growing season (determined by the base simulation) ending in 2010 for maize (left column panels), wheat (middle column panels), and rice (right column panels). Filtered for GAEZ base crop production greater than 0.01 Mg km−2.
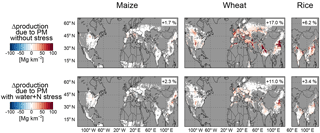
Figure 3Change in pDSSAT crop production due to PM with max ΔRUE = 100 % with no stress (top row panels) and water and nitrogen (N) stresses (bottom row panels) applied. For growing season ending in 2010 for maize (left column panels), wheat (middle column panels), and rice (right column panels). Filtered for GAEZ base crop production greater than 0.01 Mg km−2. Global relative production change shown in upper right of each map.
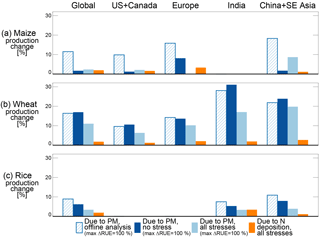
Figure 4Regional relative change in crop production due to PM with maxΔRUE = 100 %: offline analysis from Schiferl and Heald (2018) (blue bars with hatching), pDSSAT simulation with no stress (dark blue bars), and pDSSAT simulation with water and nitrogen stresses (light blue bars). Change due to nitrogen (N) deposition in orange. For growing season ending in 2010 for (a) maize, (b) wheat, and (c) rice. Regions with a base production lower than 5 % of the global total are not shown.
4.1 Impact of particulate matter on crop growth
To simulate the effect of PM on crop production, we run pDSSAT as above (for 2009 and 2010, sampling to the growing season ending 2010) with SW and DF input from GC-RT with and without PM. The differences in SW and DF due to PM over the pDSSAT growing season (determined by the base simulation) are shown in Fig. 2. PM has a negative effect on SW everywhere and positive effect on DF. The largest influence of PM is over China for all three crops. The influence is especially noticeable for wheat, where a growing season over the winter corresponds with higher PM concentrations. The difference between the simulations with and without PM is the change in production due to PM, and this is shown in Fig. 3. We perform this procedure first with no stress factors applied in order to compare to the results found in Schiferl and Heald (2018), referred to here as the “offline analysis”. The offline analysis uses a relativistic methodology which allows for unlimited growth enhancement (or loss) and is determined by the accumulated PM impacts throughout the growing season. In this pDSSAT simulation with no stress applied, global maize production increases by 1.7 %, wheat increases by 17.0 %, and rice increases by 6.2 %. Wheat production in the Indian and China + SE Asia regions is most affected by PM, and the regional proportional change is shown in Fig. 4. For wheat and rice, the proportional enhancement in crop production due to PM simulated with pDSSAT is very similar to that found in the offline analysis (Fig. 4). This is true globally and within each region. The pDSSAT scenario with no stress is closely related to the offline analysis, which was unrestricted in production enhancement, so this good comparison is expected.
Unlike for wheat and rice, the proportional increase in maize production due to PM simulated by the pDSSAT model is much lower than that from the offline analysis. This can be explained by a physiological restriction within the model which limits the maximum number of kernels per maize plant based on its genetic potential. Within pDSSAT, hybrid cultivars are limited to about 900 kernels per plant, while open-pollinated cultivars are limited to about 550 kernels per plant. When the maximum number of kernels per plant is reached, biomass is redistributed to other parts of the plant, such as the roots and stems. In the scenario above with no stress, PM only produces a 1.2 % increase in maize production over the US (Fig. 5), a region of substantial, high-intensity maize production. For most locations in this domain, pDSSAT simulates the maximum maize production dictated by the kernel number both with and without PM. When we artificially increase the limit by 500 kernels per plant, an arbitrary amount chosen for illustrative purposes, the maize production increases, as expected. Production without PM increases by 25 %, and production with PM increases by 34 %. This results in an 8.4 % increase in maize production due to PM over the US under no stress, which is similar to the approximately 10 % increase found in the offline analysis. This dependence on a kernel limit demonstrates the importance of including physiological limitations to growth as represented in a crop production model when addressing the air quality impacts on crops.
To investigate the more realistic effect of PM on crop production, we impose both water and nitrogen stress on our pDSSAT simulations. The results for this scenario (Figs. 3 and 4) indicate an 11 % increase in global wheat production due to PM and a 3.4 % increase in rice. These proportional enhancements are about one-third lower with stresses for wheat compared to without and about one-half for rice. While similar declines occur on a regional basis, these stresses have a larger impact on India for wheat, where nearly one-half of additional simulated wheat production is lost. For maize, including stress factors under the standard kernel restriction lowers the total production with and without PM, but allows for a larger proportional change due to PM in most areas (i.e., 1.7 % global production increase without stress, but 2.3 % with stresses) as more areas are producing below the production limit. When additional kernels are allowed with stresses turned on, production due to PM also increases, but to a lesser percentage compared to without stress (not shown).
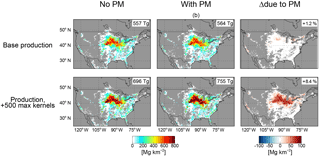
Figure 5For pDSSAT base production (top row panels) and production with increased maximum kernels per plant (bottom row panels) under no stress: maize production with no PM (left column panels), maize production with PM (with maxΔRUE = 100 %) (middle column panels), and maize production due to PM (right column panels). Filtered for GAEZ base crop production greater than 0.01 Mg km−2. Global production (left and middle column panels) or relative production change (right column panels) shown in upper right of each map.
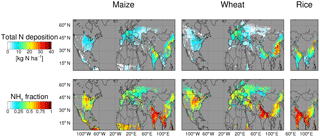
Figure 6Total nitrogen (N) deposition from GEOS-Chem (top row panels) and reduced nitrogen (NHx) fraction of this total (bottom row panels). For pDSSAT growing season (determined by the base simulation) ending in 2010 for maize (left column panels), wheat (middle column panels), and rice (right column panels). Filtered for GAEZ base crop production greater than 0.01 Mg km−2.
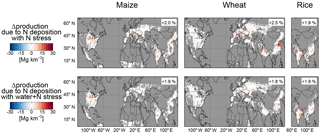
Figure 7Change in pDSSAT crop production due to nitrogen (N) deposition with N stress (top row panels) and water and N stresses (bottom row panels) applied. For growing season ending in 2010 for maize (left column panels), wheat (middle column panels), and rice (right column panels). Filtered for GAEZ base crop production greater than 0.01 Mg km−2. Global relative production change shown in upper right of each map.
4.2 Impact of nitrogen deposition on crop growth
To quantify the impact of nitrogen deposition on crop production, we run pDSSAT as above (for 2009 and 2010, sampling to the growing season ending 2010) with NHx and NOy atmospheric deposition fluxes from GEOS-Chem and compare the results to the base simulation, which contains no atmospheric nitrogen deposition (only direct fertilizer application). In this case, we do not consider PM impacts on radiation. The total nitrogen deposition flux for each crop over the base simulation growing season is shown in Fig. 6. There is high nitrogen deposition in India and China for all three crops, but especially wheat in China. The magnitude of nitrogen deposition from GEOS-Chem is generally lower than that applied as fertilizer in pDSSAT. For example, two fertilizer applications for maize span roughly 50–100 kg ha−1 each over the US, Europe, and China, whereas nitrogen deposition during the growing season rarely exceeds 20 kg ha−1 in China and India. However, nitrogen deposition is continuous, while fertilizer application is sporadic and limited temporally. We also plot the fraction of total nitrogen deposition made up of NHx in Fig. 6. This fraction is slightly higher in agricultural areas of the US, Europe, and China, where reduced species from agriculture mix with oxidized species from industry. In India, the NHx fraction is very high, as there is little industrial emission to offset the large agricultural emissions. While we do apply reduced and oxidized nitrogen deposition (NHx vs. NOy) separately in our simulations, this separation has little impact on our results as soil nitrification quickly converts all soil into in the pDSSAT model.
When accounting for both nitrogen and water stress, crop production increases globally by 1.9 % for maize, 1.8 % for wheat, and 1.9 % for rice due to atmospheric nitrogen deposition applied beginning 30 days before the planting date (Fig. 7). The largest impact of nitrogen deposition is for wheat in China, which receives large amounts of nitrogen through deposition and is highly sensitive to nitrogen stress (Fig. 1). Nitrogen deposited to the surface accumulates in the soil throughout the growing season, moving quickly to lower levels of the soil profile. When fertilizer applied toward the beginning of the growing season runs out, this additional nitrogen reservoir from deposition allows for a mitigation of nitrogen stress and furthers plant growth. The fate of nitrogen in soil is not well constrained, and the length of time nitrogen is retained in the soil and useful to the plant is uncertain. The regional impacts of nitrogen deposition on crop production for this scenario are shown in Fig. 4. In all cases, except for Indian rice, the nitrogen deposition effect is proportionally smaller than the enhancing effect of PM (disregarding the European maize simulation, which is restricted by kernel density).
When we apply nitrogen deposition to pDSSAT at the onset of the growing season, rather than 30 days prior to planting, we find that the impact of nitrogen deposition is dampened somewhat (production enhancement due to nitrogen deposition is then 1.6 % for maize, 1.5 % for wheat, and 1.5 % for rice). Conversely, applying nitrogen deposition in the crop model earlier enhances the increase in crop production. pDSSAT could be configured to run in series over numerous years, as done by H. L. Liu et al. (2010), to simulate the long-term impacts on nitrogen cycling, but the uncertainty regarding the timing and retention of nitrogen deposited onto soils would remain, especially if not evaluated against observations.
If water stress is removed, the proportional enhancement of nitrogen deposition on crop production is slightly higher, as shown in Fig. 7. The largest change is for wheat, which is more water stressed than maize and rice in the model globally.
To our knowledge, this is the first effort to integrate atmospheric air quality inputs into the dynamic simulation of a crop model. While ozone and PM air pollution have been incorporated into models which examine plant productivity, a crop model is needed to quantify the impacts on crop yield (not total biomass), the critical factor for understanding food security. This study takes into account crop-specific effects using the individual characteristics and distribution of each crop and the air pollution specific to the time frame when each crop is grown. In this way, we produce a better constrained assessment of the impacts of PM (radiation) and nitrogen deposition on crop production.
Using restrictions on water and nitrogen availability as well as physiological limitations from the crop model provides a more realistic estimate of the impact of PM on crop production than in our earlier work which considered no such restrictions (Schiferl and Heald, 2018). Maize production increases by only 2.3 % due to PM (11.5 % in Schiferl and Heald, 2018) using the max ΔRUE = 100 % relationship, while wheat increases by 11.0 % (16.4 %) and rice increases by 3.4 % (8.9 %). The positive effect of PM on crop production is lessened when considering realistic restrictions to crop growth, but remains significant throughout the globe, especially in northern China. While it is difficult to compare across studies with varying approaches and metrics, our results are consistent in sign with the change in the CO2 sink for crops due to PM found by Niyogi et al. (2004) and the global GPP change due to PM found by Strada and Unger (2016), noting that CO2 and GPP are not necessarily consistent with crop yield. We also find similar enhancements on a regional scale as Strada and Unger (2016), but for different regions, China and India in our case, as we do not consider the forested areas which dominate their results. For maize and wheat, the proportional increase in production is larger than the NPP increase found for all vegetation in China by Yue and Unger (2017). Our crop model is generally less responsive to PM than those enhancements found in forests in the locations studied by Cirino et al. (2014) and Strada et al. (2015), which is consistent with the smaller canopies of crops. However, we are inconsistent in sign with the negative response in GPP due to PM found by Strada et al. (2015), although their study convolves croplands with grasslands.
Given that PM is simulated using current emissions (2010), these enhancements are already folded into present-day crop production and may therefore be important to consider for air quality policy decisions which would reduce PM and thereby reduce production in areas with crops sensitive to PM. For example, the decline in PM associated with the recent decrease in US SO2 emissions has been shown to reduce US GPP by over 1 % since 1995 (Keppel-Aleks and Washenfelder, 2016). While this amount is small and aggregated for productivity over a large area, the impact of future PM change may be larger and more important to consider over a concentrated, highly polluted area. However, we note that our results assume the maximum sensitivity of crops to PM and therefore the impact of PM on food production may be more modest, especially when considering secondary effects of PM (e.g., hydrological, meteorological) which may offset such enhancements. More laboratory work is needed to understand how different crop varietals respond to changes in radiation throughout the growing season.
Our coupling of an atmospheric chemistry model with a crop model also provides an opportunity to explore the impact of atmospheric nitrogen deposition on crop production. We find that the impact of nitrogen deposition on crop production is significant, but more modest than the effect of PM. Our results are consistent with Thornton et al. (2007), who find an ∼ 2 % enhancement of global GPP due to nitrogen deposition. For crop yield, the impact of nitrogen deposition we find is also consistent in sign with Liu et al. (2013) over China. We underpredict the effect of nitrogen deposition on crops compared to the metric of sourced nitrogen content used by Ladha et al. (2016). This may be due to our relatively short assumed nitrogen deposition time frame. The fate of nitrogen in soil in managed ecosystems is a key uncertainty in estimating the response of crop production to changing atmospheric nitrogen deposition.
A future with enhanced fertilizer inputs to feed growing populations will, if applied in excess, increase nitrogen inputs through deposition as well, potentially enhancing crop production further. At the same time, lower future NOx emissions are likely due to regulatory efforts, which will reduce the nitrogen deposition flux. These reductions could also reduce PM in areas prone to ammonium nitrate formation. The future trajectory of nitrogen deposition and PM remain uncertain, and thus the net impact on global crop production is unclear. An increased understanding of the implications of nitrogen deposition on crop production may also lead to better optimization of fertilizer application in areas where this impact is substantial.
The crop model responses to DF and nitrogen deposition examined in this study are uncertain and may vary from year to year. More work is needed, particularly controlled laboratory studies, to understand and evaluate these responses. It is critical to develop realistic crop models with reliable sensitivity to environmental factors to understand the pressure on future food security. Crop models tuned to reproduce observed yields without accounting for PM impacts (both direct and secondary) and nitrogen deposition may be less reliable under future levels of air pollution.
The GC-RT and pDSSAT model data used in this study are archived at MIT and are available on request from the authors (schiferl@mit.edu). Emissions inventories implemented in GEOS-Chem v10-01 are available at https://github.com/GCST/hemco data_download (GEOS-Chem Support Team, 2015). The DSSAT and pSIMS models and input data are available through http://www.dssat.net and http://www.github.com/RDCEP/psims (Elliott et al., 2014; Hoogenboom et al., 2015), respectively. The FAO GAEZ crop database is available at http://gaez.fao.org (FAO, 2016).
The authors declare that they have no conflict of interest.
Funding for this research was provided by the Martin Family Fellowship for
Sustainability and the Abdul Latif Jameel World Water and Food Security Lab (J-WAFS)
at the Massachusetts Institute of Technology (MIT). The authors
thank the GEOS-Chem support staff and community for model documentation.
Edited by: Trevor Keenan
Reviewed by: two anonymous referees
Amos, H. M., Jacob, D. J., Holmes, C. D., Fisher, J. A., Wang, Q., Yantosca, R. M., Corbitt, E. S., Galarneau, E., Rutter, A. P., Gustin, M. S., Steffen, A., Schauer, J. J., Graydon, J. A., Louis, V. L. S., Talbot, R. W., Edgerton, E. S., Zhang, Y., and Sunderland, E. M.: Gas-particle partitioning of atmospheric Hg(II) and its effect on global mercury deposition, Atmos. Chem. Phys., 12, 591–603, https://doi.org/10.5194/acp-12-591-2012, 2012.
Avnery, S., Mauzerall, D. L., Liu, J., and Horowitz, L. W.: Global crop yield reductions due to surface ozone exposure: 1. Year 2000 crop production losses and economic damage, Atmos. Environ., 45, 2284–2296, https://doi.org/10.1016/j.atmosenv.2010.11.045, 2011.
Basso, B., Liu, L. and Ritchie, J. T.: A Comprehensive Review of the CERES-Wheat, – Maize and – Rice Models' Performances, in: Advances in Agronomy, vol. 136, edited by: Sparks, D. L., Academic Press, London, UK, 27–132, https://doi.org/10.1016/bs.agron.2015.11.004, 2016.
Beem, K. B., Raja, S., Schwandner, F. M., Taylor, C., Lee, T., Sullivan, A. P., Carrico, C. M., McMeeking, G. R., Day, D., Levin, E., Hand, J., Kreidenweis, S. M., Schichtel, B., Malm, W. C., and Collett Jr., J. L.: Deposition of reactive nitrogen during the Rocky Mountain Airborne Nitrogen and Sulfur (RoMANS) study, Environ. Pollut., 158, 862–872, https://doi.org/10.1016/j.envpol.2009.09.023, 2010.
Bobbink, R., Hicks, K., Galloway, J., Spranger, T., Alkemade, R., Ashmore, M., Bustamante, M., Cinderby, S., Davidson, E., Dentener, F., Emmett, B., Erisman, J. W., Fenn, M., Gilliam, F., Nordin, A., Pardo, L. and De Vries, W.: Global assessment of nitrogen deposition effects on terrestrial plant diversity: a synthesis, Ecol. Appl., 20, 30–59, https://doi.org/10.1890/08-1140.1, 2010.
Bond, T. C., Bhardwaj, E., Dong, R., Jogani, R., Jung, S., Roden, C., Streets, D. G., and Trautmann, N. M.: Historical emissions of black and organic carbon aerosol from energy-related combustion, 1850–2000, Global Biogeochem. Cy., 21, GB2018, https://doi.org/10.1029/2006GB002840, 2007.
Bouwman, L., Goldewijk, K. K., Hoek, K. W. V. D., Beusen, A. H. W., Vuuren, D. P. V., Willems, J., Rufino, M. C., and Stehfest, E.: Exploring global changes in nitrogen and phosphorus cycles in agriculture induced by livestock production over the 1900–2050 period, P. Natl. Acad. Sci. USA, 110, 20882–20887, https://doi.org/10.1073/pnas.1012878108, 2013.
Burney, J. and Ramanathan, V.: Recent climate and air pollution impacts on Indian agriculture, P. Natl. Acad. Sci. USA, 111, 16319–16324, https://doi.org/10.1073/pnas.1317275111, 2014.
Challinor, A. J., Watson, J., Lobell, D. B., Howden, S. M., Smith, D. R., and Chhetri, N.: A meta-analysis of crop yield under climate change and adaptation, Nat. Clim. Change, 4, 287–291, https://doi.org/10.1038/nclimate2153, 2014.
Cirino, G. G., Souza, R. A. F., Adams, D. K., and Artaxo, P.: The effect of atmospheric aerosol particles and clouds on net ecosystem exchange in the Amazon, Atmos. Chem. Phys., 14, 6523–6543, https://doi.org/10.5194/acp-14-6523-2014, 2014.
Cohen, A. J., Brauer, M., Burnett, R., Anderson, H. R., Frostad, J., Estep, K., Balakrishnan, K., Brunekreef, B., Dandona, L., Dandona, R., Feigin, V., Freedman, G., Hubbell, B., Jobling, A., Kan, H., Knibbs, L., Liu, Y., Martin, R., Morawska, L., Pope, C. A., Shin, H., Straif, K., Shaddick, G., Thomas, M., van Dingenen, R., van Donkelaar, A., Vos, T., Murray, C. J. L., and Forouzanfar, M. H.: Estimates and 25-year trends of the global burden of disease attributable to ambient air pollution: an analysis of data from the Global Burden of Diseases Study 2015, Lancet, 389, 1907–1918, https://doi.org/10.1016/S0140-6736(17)30505-6, 2017.
Elliott, J., Kelly, D., Chryssanthacopoulos, J., Glotter, M., Jhunjhnuwala, K., Best, N., Wilde, M., and Foster, I.: The parallel system for integrating impact models and sectors (pSIMS), Environ. Modell. Softw., 62, 509–516, https://doi.org/10.1016/j.envsoft.2014.04.008, 2014.
Erisman, J. W., Bleeker, A., Galloway, J., and Sutton, M. S.: Reduced nitrogen in ecology and the environment, Environ. Pollut., 150, 140–149, https://doi.org/10.1016/j.envpol.2007.06.033, 2007.
Fairlie, T. D., Jacob, D. J., and Park, R. J.: The impact of transpacific transport of mineral dust in the United States, Atmos. Environ., 41, 1251–1266, https://doi.org/10.1016/j.atmosenv.2006.09.048, 2007.
FAO: Global Agro-Ecological Zones (GAEZ), available at: http://gaez.fao.org, last access: April 2016.
Folberth, C., Skalský, R., Moltchanova, E., Balkovič, J., Azevedo, L. B., Obersteiner, M., and van der Velde, M.: Uncertainty in soil data can outweigh climate impact signals in global crop yield simulations, Nat. Commun., 7, 11872, https://doi.org/10.1038/ncomms11872, 2016.
Fountoukis, C. and Nenes, A.: ISORROPIA II: a computationally efficient thermodynamic equilibrium model for K+––––Na+–––Cl−–H2O aerosols, Atmos. Chem. Phys., 7, 4639–4659, https://doi.org/10.5194/acp-7-4639-2007, 2007.
Galloway, J. N. and Cowling, E. B.: Reactive nitrogen and the world: 200 years of change, Ambio, 31, 64–71, https://doi.org/10.1579/0044-7447-31.2.64, 2002.
GEOS-Chem Support Team: HEMCO emissions data, available at: https://github.com/GCST/hemco_data_download, last access: June 2015.
Goulding, K. W. T., Bailey, N. J., Bradbury, N. J., Hargreaves, P., Howe, M., Murphy, D. V., Poulton, P. R., and Willison, T. W.: Nitrogen deposition and its contribution to nitrogen cycling and associated soil processes, New Phytol., 139, 49–58, 1998.
Greenwald, R., Bergin, M. H., Xu, J., Cohan, D., Hoogenboom, G., and Chameides, W. L.: The influence of aerosols on crop production: A study using the CERES crop model, Agr. Syst., 89, 390–413, https://doi.org/10.1016/j.agsy.2005.10.004, 2006.
Heald, C. L., Ridley, D. A., Kroll, J. H., Barrett, S. R. H., Cady-Pereira, K. E., Alvarado, M. J., and Holmes, C. D.: Contrasting the direct radiative effect and direct radiative forcing of aerosols, Atmos. Chem. Phys., 14, 5513–5527, https://doi.org/10.5194/acp-14-5513-2014, 2014.
Hoogenboom, G., Jones, J. W., Wilkens, P. W., Porter, C. H., Boote, K. J., Hunt, L. A., Singh, U., Lizaso, J. I., White, J. W., Uryasev, O., Ogoshi, R., Koo, J., Shelia, V., and Tsuji, G. Y.: Decision Support System for Agrotechnology Transfer (DSSAT) Version 4.6, available at: http://www.dssat.net (last access: August 2016), 2015.
Hu, L., Millet, D. B., Baasandorj, M., Griffis, T. J., Travis, K. R., Tessum, C. W., Marshall, J. D., Reinhart, W. F., Mikoviny, T., Müller, M., Wisthaler, A., Graus, M., Warneke, C., and de Gouw, J.: Emissions of C6–C8 aromatic compounds in the United States: Constraints from tall tower and aircraft measurements, J. Geophys. Res.-Atmos., 120, 826–842, https://doi.org/10.1002/2014JD022627, 2015.
Hudman, R. C., Moore, N. E., Mebust, A. K., Martin, R. V., Russell, A. R., Valin, L. C., and Cohen, R. C.: Steps towards a mechanistic model of global soil nitric oxide emissions: implementation and space based-constraints, Atmos. Chem. Phys., 12, 7779–7795, https://doi.org/10.5194/acp-12-7779-2012, 2012.
IPCC: Climate Change 2014: Impacts, Adaptation, and Vulnerability, Working Group II Contribution to the Fifth Assessment Report of the Intergovernmental Panel on Climate Change, Cambridge University Press, Cambridge, UK and New York, NY, USA, 2014.
Jaeglé, L., Quinn, P. K., Bates, T. S., Alexander, B., and Lin, J.-T.: Global distribution of sea salt aerosols: new constraints from in situ and remote sensing observations, Atmos. Chem. Phys., 11, 3137–3157, https://doi.org/10.5194/acp-11-3137-2011, 2011.
Jones, C. A., Kiniry, J. R., and Dyke, P. T.: CERES-Maize: a simulation model of maize growth and development, Texas A & M University Press, College Station, TX, USA, 1986.
Jones, J. W., Hoogenboom, G., Porter, C. H., Boote, K. J., Batchelor, W. D., Hunt, L. A., Wilkens, P. W., Singh, U., Gijsman, A. J., and Ritchie, J. T.: The DSSAT cropping system model, Eur. J. Agron., 18, 235–265, https://doi.org/10.1016/S1161-0301(02)00107-7, 2003.
Kanniah, K. D., Beringer, J., North, P., and Hutley, L.: Control of atmospheric particles on diffuse radiation and terrestrial plant productivity A review, Prog. Phys. Geogr., 36, 209–237, https://doi.org/10.1177/0309133311434244, 2012.
Keppel-Aleks, G. and Washenfelder, R. A.: The effect of atmospheric sulfate reductions on diffuse radiation and photosynthesis in the United States during 1995–2013, Geophys. Res. Lett., 43, 9984–9993, https://doi.org/10.1002/2016GL070052, 2016.
Ladha, J. K., Tirol-Padre, A., Reddy, C. K., Cassman, K. G., Verma, S., Powlson, D. S., van Kessel, C., de Richter, D. B., Chakraborty, D., and Pathak, H.: Global nitrogen budgets in cereals: A 50-year assessment for maize, rice, and wheat production systems, Sci. Rep.-UK, 6, 19355, https://doi.org/10.1038/srep19355, 2016.
Lamarque, J.-F., Dentener, F., McConnell, J., Ro, C.-U., Shaw, M., Vet, R., Bergmann, D., Cameron-Smith, P., Dalsoren, S., Doherty, R., Faluvegi, G., Ghan, S. J., Josse, B., Lee, Y. H., MacKenzie, I. A., Plummer, D., Shindell, D. T., Skeie, R. B., Stevenson, D. S., Strode, S., Zeng, G., Curran, M., Dahl-Jensen, D., Das, S., Fritzsche, D., and Nolan, M.: Multi-model mean nitrogen and sulfur deposition from the Atmospheric Chemistry and Climate Model Intercomparison Project (ACCMIP): evaluation of historical and projected future changes, Atmos. Chem. Phys., 13, 7997–8018, https://doi.org/10.5194/acp-13-7997-2013, 2013.
Lassaletta, L., Billen, G., Grizzetti, B., Anglade, J., and Garnier, J.: 50 year trends in nitrogen use efficiency of world cropping systems: the relationship between yield and nitrogen input to cropland, Environ. Res. Lett., 9, 105011, https://doi.org/10.1088/1748-9326/9/10/105011, 2014.
Leibensperger, E. M., Mickley, L. J., Jacob, D. J., Chen, W.-T., Seinfeld, J. H., Nenes, A., Adams, P. J., Streets, D. G., Kumar, N., and Rind, D.: Climatic effects of 1950–2050 changes in US anthropogenic aerosols – Part 1: Aerosol trends and radiative forcing, Atmos. Chem. Phys., 12, 3333–3348, https://doi.org/10.5194/acp-12-3333-2012, 2012.
Liu, H., Jacob, D. J., Bey, I., and Yantosca, R. M.: Constraints from 210Pb and 7Be on wet deposition and transport in a global three-dimensional chemical tracer model driven by assimilated meteorological fields, J. Geophys. Res., 106, 12109–12128, https://doi.org/10.1029/2000JD900839, 2001.
Liu, H. L., Yang, J. Y., Drury, C. F., Reynolds, W. D., Tan, C. S., Bai, Y. L., He, P., Jin, J., and Hoogenboom, G.: Using the DSSAT-CERES-Maize model to simulate crop yield and nitrogen cycling in fields under long-term continuous maize production, Nutr. Cycl. Agroecosyst., 89, 313–328, https://doi.org/10.1007/s10705-010-9396-y, 2010.
Liu, J., You, L., Amini, M., Obersteiner, M., Herrero, M., Zehnder, A. J. B., and Yang, H.: A high-resolution assessment on global nitrogen flows in cropland, P. Natl. Acad. Sci. USA, 107, 8035–8040, https://doi.org/10.1073/pnas.0913658107, 2010.
Liu, X., Zhang, Y., Han, W., Tang, A., Shen, J., Cui, Z., Vitousek, P., Erisman, J. W., Goulding, K., Christie, P., Fangmeier, A., and Zhang, F.: Enhanced nitrogen deposition over China, Nature, 494, 459–462, https://doi.org/10.1038/nature11917, 2013.
Lobell, D. B. and Burke, M. B.: On the use of statistical models to predict crop yield responses to climate change, Agr. Forest Meteorol., 150, 1443–1452, https://doi.org/10.1016/j.agrformet.2010.07.008, 2010.
Lu, X., Chen, M., Liu, Y., Miralles, D. G., and Wang, F.: Enhanced water use efficiency in global terrestrial ecosystems under increasing aerosol loadings, Agr. Forest Meteorol., 237–238, 39–49, https://doi.org/10.1016/j.agrformet.2017.02.002, 2017.
Mao, J., Paulot, F., Jacob, D. J., Cohen, R. C., Crounse, J. D., Wennberg, P. O., Keller, C. A., Hudman, R. C., Barkley, M. P. and Horowitz, L. W.: Ozone and organic nitrates over the eastern United States: Sensitivity to isoprene chemistry, J. Geophys. Res.-Atmos., 118, 11256–11268, https://doi.org/10.1002/jgrd.50817, 2013.
Mills, G., Hayes, F., Simpson, D., Emberson, L., Norris, D., Harmens, H., and Büker, P.: Evidence of widespread effects of ozone on crops and (semi-)natural vegetation in Europe (1990–2006) in relation to AOT40- and flux-based risk maps, Global Change Biol., 17, 592–613, https://doi.org/10.1111/j.1365-2486.2010.02217.x, 2011.
Mueller, N. D., Gerber, J. S., Johnston, M., Ray, D. K., Ramankutty, N., and Foley, J. A.: Closing yield gaps through nutrient and water management, Nature, 490, 254–257, https://doi.org/10.1038/nature11420, 2012.
Murray, L. T., Jacob, D. J., Logan, J. A., Hudman, R. C., and Koshak, W. J.: Optimized regional and interannual variability of lightning in a global chemical transport model constrained by LIS/OTD satellite data, J. Geophys. Res.-Atmos., 117, 20307, https://doi.org/10.1029/2012JD017934, 2012.
Niyogi, D., Chang, H.-I., Saxena, V. K., Holt, T., Alapaty, K., Booker, F., Chen, F., Davis, K. J., Holben, B., Matsui, T., Meyers, T., Oechel, W. C., Pielke, R. A., Wells, R., Wilson, K., and Xue, Y.: Direct observations of the effects of aerosol loading on net ecosystem CO2 exchanges over different landscapes, Geophys. Res. Lett., 31, L20506, https://doi.org/10.1029/2004GL020915, 2004.
Portmann, F. T., Siebert, S., and Döll, P.: MIRCA2000 – Global monthly irrigated and rainfed crop areas around the year 2000: A new high-resolution data set for agricultural and hydrological modeling, Global Biogeochem. Cy., 24, GB1011, https://doi.org/10.1029/2008GB003435, 2010.
Riddick, S., Ward, D., Hess, P., Mahowald, N., Massad, R., and Holland, E.: Estimate of changes in agricultural terrestrial nitrogen pathways and ammonia emissions from 1850 to present in the Community Earth System Model, Biogeosciences, 13, 3397–3426, https://doi.org/10.5194/bg-13-3397-2016, 2016.
Ritchie, J. T. and Otter, S.: Description and performance of CERES-Wheat: a user-oriented wheat yield model, ARS – United States Department of Agriculture, Agricultural Research Service, Beltsville, MD, USA, 1985.
Ritchie, J. T., Singh, U., Godwin, D. C., and Bowen, W. T.: Cereal growth, development and yield, in: Understanding Options for Agricultural Production, edited by: Tsuji, G. Y., Hoogenboom, G., and Thornton, P. K., Kluwer Academic Publishers, Dordrecht, the Netherlands, 1998.
Rosenzweig, C., Jones, J. W., Hatfield, J. L., Ruane, A. C., Boote, K. J., Thorburn, P., Antle, J. M., Nelson, G. C., Porter, C., Janssen, S., Asseng, S., Basso, B., Ewert, F., Wallach, D., Baigorria, G., and Winter, J. M.: The Agricultural Model Intercomparison and Improvement Project (AgMIP): Protocols and pilot studies, Agr. Forest Meteorol., 170, 166–182, https://doi.org/10.1016/j.agrformet.2012.09.011, 2013.
Rosenzweig, C., Elliott, J., Deryng, D., Ruane, A. C., Müller, C., Arneth, A., Boote, K. J., Folberth, C., Glotter, M., Khabarov, N., Neumann, K., Piontek, F., Pugh, T. A. M., Schmid, E., Stehfest, E., Yang, H., and Jones, J. W.: Assessing agricultural risks of climate change in the 21st century in a global gridded crop model intercomparison, P. Natl. Acad. Sci. USA, 111, 3268–3273, https://doi.org/10.1073/pnas.1222463110, 2014.
Ruane, A. C., Goldberg, R., and Chryssanthacopoulos, J.: Climate forcing datasets for agricultural modeling: Merged products for gap-filling and historical climate series estimation, Agr. Forest Meteorol., 200, 233–248, https://doi.org/10.1016/j.agrformet.2014.09.016, 2015.
Sacks, W. J., Deryng, D., Foley, J. A., and Ramankutty, N.: Crop planting dates: an analysis of global patterns, Global Ecol. Biogeogr., 19, 607–620, https://doi.org/10.1111/j.1466-8238.2010.00551.x, 2010.
Schiferl, L. D. and Heald, C. L.: Particulate matter air pollution may offset ozone damage to global crop production, Atmos. Chem. Phys., 18, 5953–5966, https://doi.org/10.5194/acp-18-5953-2018, 2018.
Seinfeld, J. H. and Pandis, S. N.: Atmospheric Chemistry and Physics – From Air Pollution to Climate Change, 2nd Edn., John Wiley & Sons, Hoboken, NJ, USA, 2006.
Shangguan, W., Dai, Y., Duan, Q., Liu, B., and Yuan, H.: A global soil data set for earth system modeling, J. Adv. Model. Earth Syst., 6, 249–263, https://doi.org/10.1002/2013MS000293, 2014.
Shindell, D., Faluvegi, G., Walsh, M., Anenberg, S. C., Van Dingenen, R., Muller, N. Z., Austin, J., Koch, D., and Milly, G.: Climate, health, agricultural and economic impacts of tighter vehicle-emission standards, Nat. Clim. Change, 1, 59–66, https://doi.org/10.1038/nclimate1066, 2011.
Sinclair, T. R., Shiraiwa, T., and Hammer, G. L.: Variation in crop radiation – use efficiency with increased diffuse radiation, Crop Sci., 32, 1281, https://doi.org/10.2135/cropsci1992.0011183X003200050043x, 1992.
Smil, V.: Nitrogen in crop production: An account of global flows, Global Biogeochem. Cy., 13, 647–662, https://doi.org/10.1029/1999GB900015, 1999.
Strada, S. and Unger, N.: Potential sensitivity of photosynthesis and isoprene emission to direct radiative effects of atmospheric aerosol pollution, Atmos. Chem. Phys., 16, 4213–4234, https://doi.org/10.5194/acp-16-4213-2016, 2016.
Strada, S., Unger, N., and Yue, X.: Observed aerosol-induced radiative effect on plant productivity in the eastern United States, Atmos. Environ., 122, 463–476, https://doi.org/10.1016/j.atmosenv.2015.09.051, 2015.
Tai, A. P. K., Martin, M. V., and Heald, C. L.: Threat to future global food security from climate change and ozone air pollution, Nat. Clim. Change, 4, 817–821, https://doi.org/10.1038/nclimate2317, 2014.
Thomas, R. Q., Bonan, G. B., and Goodale, C. L.: Insights into mechanisms governing forest carbon response to nitrogen deposition: a model–data comparison using observed responses to nitrogen addition, Biogeosciences, 10, 3869–3887, https://doi.org/10.5194/bg-10-3869-2013, 2013.
Thornton, P. E., Lamarque, J.-F., Rosenbloom, N. A., and Mahowald, N. M.: Influence of carbon-nitrogen cycle coupling on land model response to CO2 fertilization and climate variability, Global Biogeochem. Cy., 21, GB4018, https://doi.org/10.1029/2006GB002868, 2007.
Thornton, P. E., Doney, S. C., Lindsay, K., Moore, J. K., Mahowald, N., Randerson, J. T., Fung, I., Lamarque, J.-F., Feddema, J. J., and Lee, Y.-H.: Carbon–nitrogen interactions regulate climate-carbon cycle feedbacks: results from an atmosphere–ocean general circulation model, Biogeosciences, 6, 2099–2120, https://doi.org/10.5194/bg-6-2099-2009, 2009.
Van Dingenen, R., Dentener, F. J., Raes, F., Krol, M. C., Emberson, L., and Cofala, J.: The global impact of ozone on agricultural crop yields under current and future air quality legislation, Atmos. Environ., 43, 604–618, https://doi.org/10.1016/j.atmosenv.2008.10.033, 2009.
Wang, K., Dickinson, R. E., and Liang, S.: Observational evidence on the effects of clouds and aerosols on net ecosystem exchange and evapotranspiration, Geophys. Res. Lett., 35, L10401, https://doi.org/10.1029/2008GL034167, 2008.
Wang, Y., Jacob, D. J., and Logan, J. A.: Global simulation of tropospheric O3-NOx-hydrocarbon chemistry: 3. Origin of tropospheric ozone and effects of nonmethane hydrocarbons, J. Geophys. Res., 103, 10757–10767, https://doi.org/10.1029/98JD00156, 1998.
You, L., Guo, Z., Koo, J., Ojo, W., Sebastian, K., Tenorio, M. T., Wood, S., and Wood-Sichra, U.: Spatial Production Allocation Model (SPAM) 2000 Version 3 Release 1, available at: http://MapSPAM.info (last access: 28 September 2013), 2012.
Yue, X. and Unger, N.: Aerosol optical depth thresholds as a tool to assess diffuse radiation fertilization of the land carbon uptake in China, Atmos. Chem. Phys., 17, 1329–1342, https://doi.org/10.5194/acp-17-1329-2017, 2017.
Yue, X., Unger, N., Harper, K., Xia, X., Liao, H., Zhu, T., Xiao, J., Feng, Z., and Li, J.: Ozone and haze pollution weakens net primary productivity in China, Atmos. Chem. Phys., 17, 6073–6089, https://doi.org/10.5194/acp-17-6073-2017, 2017.
Zhang, L., Gong, S., Padro, J., and Barrie, L.: A size-segregated particle dry deposition scheme for an atmospheric aerosol module, Atmos. Environ., 35, 549–560, https://doi.org/10.1016/S1352-2310(00)00326-5, 2001.
Zhu, L., Henze, D., Bash, J., Jeong, G.-R., Cady-Pereira, K., Shephard, M., Luo, M., Paulot, F., and Capps, S.: Global evaluation of ammonia bidirectional exchange and livestock diurnal variation schemes, Atmos. Chem. Phys., 15, 12823–12843, https://doi.org/10.5194/acp-15-12823-2015, 2015.