the Creative Commons Attribution 4.0 License.
the Creative Commons Attribution 4.0 License.
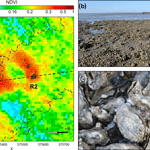
Satellite remote sensing reveals a positive impact of living oyster reefs on microalgal biofilm development
Caroline Echappé
Pierre Gernez
Vona Méléder
Bruno Jesus
Bruno Cognie
Priscilla Decottignies
Koen Sabbe
Laurent Barillé
Satellite remote sensing (RS) is routinely used for the large-scale monitoring of microphytobenthos (MPB) biomass in intertidal mudflats and has greatly improved our knowledge of MPB spatio-temporal variability and its potential drivers. Processes operating on smaller scales however, such as the impact of benthic macrofauna on MPB development, to date remain underinvestigated. In this study, we analysed the influence of wild Crassostrea gigas oyster reefs on MPB biofilm development using multispectral RS. A 30-year time series (1985–2015) combining high-resolution (30 m) Landsat and SPOT data was built in order to explore the relationship between C. gigas reefs and MPB spatial distribution and seasonal dynamics, using the normalized difference vegetation index (NDVI). Emphasis was placed on the analysis of a before–after control-impact (BACI) experiment designed to assess the effect of oyster killing on the surrounding MPB biofilms. Our RS data reveal that the presence of oyster reefs positively affects MPB biofilm development. Analysis of the historical time series first showed the presence of persistent, highly concentrated MPB patches around oyster reefs. This observation was supported by the BACI experiment which showed that killing the oysters (while leaving the physical reef structure, i.e. oyster shells, intact) negatively affected both MPB biofilm biomass and spatial stability around the reef. As such, our results are consistent with the hypothesis of nutrient input as an explanation for the MPB growth-promoting effect of oysters, whereby organic and inorganic matter released through oyster excretion and biodeposition stimulates MPB biomass accumulation. MPB also showed marked seasonal variations in biomass and patch shape, size and degree of aggregation around the oyster reefs. Seasonal variations in biomass, with higher NDVI during spring and autumn, were consistent with those observed on broader scales in other European mudflats. Our study provides the first multi-sensor RS satellite evidence of the promoting and structuring effect of oyster reefs on MPB biofilms.
- Article
(3276 KB) - Full-text XML
-
Supplement
(811 KB) - BibTeX
- EndNote
The Pacific oyster Crassostrea gigas (Thunberg) is one of the most cosmopolitan marine macroinvertebrates, mainly as a result of its introduction in many countries for aquaculture purposes (Ruesink et al., 2005). In Europe, it was massively imported in the 1970s and rapidly became the main cultivated species, following the decline of previously farmed oysters which had been struck by large-scale epizootic outbreaks (Grizel and Héral, 1991; Humphreys et al., 2014). During recent decades, C. gigas benefited from coastal eutrophication and rising sea temperature (Thomas et al., 2016), resulting in a poleward expansion of its distribution (Dutertre et al., 2010) and the formation of dense reefs along many coastal areas (Diederich, 2006; Brandt et al., 2008; Le Bris et al., 2016). In some ecosystems, wild C. gigas is now considered as a trophic competitor of its cultivated conspecifics (Cognie et al., 2006). Newly established oyster populations also impact biogeochemical fluxes and ecosystem processes, leading to both positive (e.g. nutrient recycling) and negative (e.g. biomass regulation) impacts on local primary producers (Prins et al., 1997; Troost, 2010).
In intertidal mudflats, the main primary producers are benthic microalgal assemblages commonly referred to as microphytobenthos (MPB) (MacIntyre et al., 1996). MPB develops within the upper millimetres of the sediment and migrates toward the sediment surface at low tide, forming transient photosynthetic biofilms (Kromkamp et al., 1998; Consalvey et al., 2004; Jesus et al., 2009; Perkins et al., 2010; Coelho et al., 2011). MPB can contribute up to 50 % of total primary production in estuarine and coastal ecosystems (Underwood and Kromkamp, 1999) and is an important food source for higher trophic levels (Miller et al., 1996). After resuspension in the water column (De Jonge and Van Beuselom, 1992), benthic microalgae become available for filter feeders such as oysters (Decottignies et al., 2007). However, MPB and oyster interactions are more complex than a simple predator–prey relationship. In Colden et al. (2016), experimental oyster reefs consisting of empty shells only were shown to modify the local hydrodynamic conditions. They also promote the trapping of fine particles, providing conditions more conducive to benthic microalgal development. Dame and Libes (1993) and Newell (2004) suggested that oysters stimulate microalgae growth through nutrient inputs derived from the mineralization of oyster excretion products (faeces and pseudo-faeces). It is as yet unclear, however, to what degree the growth-promoting effects are caused by the physical structure of the reef and/or the biological activity of live oysters.
At low tide, MPB biomass can be quantified with the normalized difference vegetation index (NDVI) (Méléder et al., 2003; van der Wal et al., 2010; Brito et al., 2013; Benyoucef et al., 2014), which uses chlorophyll a optical properties in the red and near-infrared spectral regions. MPB biomass is characterized by high heterogeneity occurring on various spatial scales (Chapman et al., 2010). Intra- and interannual MPB variability have previously been assessed on different scales using archived satellite images. For example, MPB seasonal cycles were described for several European mudflats using either medium-resolution (250 m) satellite data such as MODIS (van der Wal et al., 2010), or higher spatial resolution (10–20 m) data such as SPOT (Brito et al., 2013). Few remote sensing (RS) studies to date, however, have described MPB dynamics at both high spatial and temporal resolution. This can be explained by the reduced availability of high-spatial-resolution satellite time series together with the constraints related to the acquisition of RS data during low tide only. As a result, factors driving MPB spatio-temporal patterns on the mudflat scale are not yet fully understood. To our knowledge, MPB spatial structure and temporal variability have never been specifically addressed in relation to shellfish populations, although preliminary analysis of SPOT images suggested that oyster-farming proximity might positively affect MPB concentration (Méléder et al., 2003).
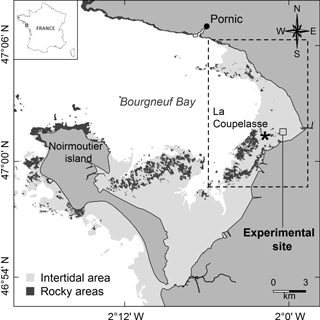
Figure 1Location of Bourgneuf Bay and the experimental site. The dashed box corresponds to a mudflat with some rocky areas mainly colonized by C. gigas wild oysters (Le Bris et al., 2016).
In the present study, a unique dataset of Landsat and SPOT images acquired at low tide were combined into a 30-year time series in order to study MPB spatio-temporal variations in relation to wild oyster reefs. This high-resolution satellite time series was used to (1) characterize the spatial distribution of MPB biomass around intertidal oyster reefs, and (2) investigate the impact of oysters on MPB biomass dynamics during an in situ ecological field experiment. The experiment consisted of the killing of wild C. gigas oysters from a reef surrounded by a clearly identified MPB patch. The preservation of the physical structure of the reef itself allowed us to specifically focus on the influence of live oysters on MPB biomass development by the means of a before–after control-impact analysis.
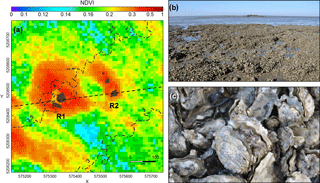
Figure 2(a) NDVI map of the experimental site (SPOT 5 image acquired on 8 September 2009). The sector is 600 × 600 m, limited by a channel on the left. The control reef (R1) and the impacted reef (R2), both formed of several emerging parts, are represented in brown. The grey dotted lines correspond to the bathymetric levels. The black dashed line represents the transect used in the analysis of the biomass variations between R1 and R2. (b) View of the reef where the oysters were burnt (R2); R1 is visible in the background. (c) Open shells of dead oysters after the burning (photographs acquired in September 2014).
2.1 Study site and experiment
Bourgneuf Bay is a macrotidal bay located south of the Loire estuary on the French Atlantic coast (47∘02′N, 2∘07′W) (Fig. 1), containing large intertidal mudflats (100 km2) colonized by microphytobenthic biofilms. The site is characterized by the extensive aquaculture of the Pacific oyster Crassostrea gigas (Thunberg, 1793). Oyster farms cover about 10 % of the intertidal area, while most of the rocky areas (about 17 % of the intertidal area) are colonized by wild oysters (Le Bris et al., 2016) or macroalgae (Combe et al., 2005).
MPB spatio-temporal distribution and spatial associations with oysters were analysed using RS of biofilms developing around wild oyster reefs. Perennial MPB biofilms dominated by epipelic diatoms (Barillé et al., 2007) were previously observed in the same area using satellite data (Méléder et al., 2003). In addition, in order to investigate the effect of oysters on MPB spatial distribution, an experiment was conducted following a before–after control-impact (BACI) design (Stewart-Oaten et al., 1986) on two C. gigas wild oyster reefs surrounded by MPB biofilms (Fig. 2). The reefs were selected to meet the following requirements: comparable size and oyster biomass, subject to similar environmental conditions and located at the same bathymetric level (between +2 and +3 m above chart datum), in an area distant from oyster farms. Based on GPS field measurements and photo-interpretation, the surface colonized by oysters was estimated to be 1044 m2 for the first reef and 894 m2 for the second reef. Before the experiment, their stocks of wild oysters were estimated at around 23 and 20 tons, respectively (Le Bris et al., 2016).
The BACI experiment was set up as follows. The first reef (hereafter designated by “R1”; left reef in Fig. 2a) was used as a control, while the oysters colonizing the second reef (hereafter designated by “R2”; right reef in Fig. 2a) were killed. This was achieved by bringing straw by boat and covering the reef with it, then setting fire to it during low tide, over two consecutive days on 16 and 17 July 2014. The burning of the reef allowed the oysters to be killed, while the physical structure of the reef itself and its shells remained intact. The impact of the oyster killing on the spatial distribution of MPB biomass around R1 and R2 before and after the experiment was assessed using satellite imagery. In addition to the analysis of spatial and seasonal patterns where the experiment took place (0.36 km2), hereafter referred to as “the experimental site” (Figs. 1, 2a), a larger area was also analysed to assess MPB seasonal variability on the scale of the mudflat (42.65 km2), hereafter referred to as “the mudflat area” (dashed area in Fig. 1).
2.2 Satellite data
A 30-year time series (1985–2015) of satellite data was built using a combination of Landsat and SPOT data (Table 1). Landsat 5 and 8 data were downloaded from the US Geological Survey (USGS) Earth Explorer data portal (http://earthexplorer.usgs.gov/). Most SPOT data were acquired on demand by the CNES (Centre National d'Etudes Spatiales) and Airbus Defence & Space, with the exception of the Take 5 data (Hagolle et al., 2015b) which were freely available from the Theia Land Data Centre web portal (https://www.theia-land.fr/en). The Theia data portal was also used to download some Landsat 5 data.
The originality of the dataset lies in its high spatial resolution (6 to 30 m), its long time duration (30 years) and the high number of selected images (47) due to the combination of several satellite missions (Table 1). All Landsat and SPOT sensors display only slight variations in the position and width of the red and the near-infrared (NIR) bands (Table 1), allowing for the calculation of comparable NDVI values (formula in Sect. 2.3) as a chlorophyll a proxy. NDVI interconsistency between Landsat and SPOT sensors was estimated using a synthetic hyperspectral library of benthic diatoms (Barillé et al., 2011). Library reflectance spectra covering a wide range of diatom biomass over different types of sediment were downscaled to each sensor spectral resolution and compared.
Careful data quality control was performed on the initial dataset. Data were first selected according to their acquisition parameters: images with a cloud cover higher than 10 % above the experimental site and the mudflat area were eliminated. Considering the location of the oyster reefs, images with a water height (based on lowest astronomical tide) superior to 2.5 m at the nearest reference harbour (Pornic harbour) at the time of the acquisition were excluded. In daylight, reflectance-based estimates of MPB biomass at the sediment surface can vary during tidal emersion due to vertical migration of the microalgae in the surface sediment layers (Serôdio et al., 1997). These migratory rhythms result in MPB accumulating at the sediment surface around mid- to low tide, and decreasing during ebb and flow. The latter will result in lower NDVI values. To avoid biases introduced by this phenomenon, the impact of image acquisition time during low tide on NDVI values was investigated. Images for which the NDVI was found to be impacted by MPB vertical migration or mudflat submersion (i.e. resulting in a low percentage of uncovered mudflat and/or abnormally low NDVI) were identified using the following criteria: water height, timing of low tide, MPB pixel count (total number of pixels identified as MPB within an image, i.e. excluding rocks, oyster reefs, water and macroalgae; see below), and outlier NDVI values. Images which did not follow a normal distribution based on each of these criteria were excluded. At the end of the quality-control process, a total of 47 images acquired from 1985 to 2015, in a time range between 09:51 and 11:36 UT, were selected to study MPB spatial and temporal patterns at the experimental site (see Table S1 in the Supplement). Due to the presence of clouds on the macroscale, a total of 44 images could be used for MPB analysis on the scale of the mudflat area.
2.3 Data processing
2.3.1 Satellite data processing
Landsat 5 (2009 to 2011) and SPOT Take 5 land surface reflectance products using the multisensory atmospheric correction and cloud screening (MACCS) method (Hagolle et al., 2015a) were directly available from the Theia web portal. Other Landsat and SPOT images were atmospherically corrected and converted into surface reflectance with the Fast Line-of-sight Atmospheric Analysis of Spectral Hypercubes (FLAASH, Matthew et al., 2000) method using ENVI 5.1. For coherence within the time series, the same FLAASH parameters (US atmospheric model, 40 km initial visibility, maritime aerosol model) were applied. The NDVI was then calculated from surface reflectance following Eq. (1):
where RNIR and RRed are respectively the reflectance in the near-infrared and red regions (Table 1). All data were projected in the WGS84 UTM30N coordinate reference system and downscaled to the lowest spatial resolution (30 m, Landsat resolution) by applying an inverse distance-weighted interpolation. All statistical analyses were carried out on these downscaled data.
Multispectral RS does not allow for the differentiation between micro- and macroalgae, leading to possible confusion between high-MPB and low-macroalgal biomasses (van der Wal et al., 2010, 2014). In this study, MPB biofilms were distinguished using two methods. First, a geometric mask was applied to the rocky areas in order to eliminate most macroalgae and epilithic microalgae (Le Bris et al., 2016). Secondly, a radiometric mask was applied to negative NDVI values to exclude water pixels and to NDVI > 0.4 to exclude macrophytes found on sediments. The latter threshold was chosen according to the maximum NDVI values observed on pixels corresponding to known MPB biofilms.
Spatial data analysis was carried out with R software (R Core Team, 2015) using gstat (Pebesma, 2004), maptools (Bivand and Lewin-Koh, 2015), raster (Hijmans, 2015), rgdal (Bivand et al., 2015), rgeos (Bivand and Rundel, 2015), and sp (Pebesma and Bivand, 2005; Bivand et al., 2013) packages.
2.3.2 Satellite data ground truthing
Spectroradiometric field measurements were performed in order to ground-truth the satellite-derived NDVI data (Forster and Jesus, 2006). Due to the difficulty of access, the experimental site was sampled only once and a nearby site (La Coupelasse, 1.5 km further) was sampled twice for matchup purposes (Table 2). An ASD FieldSpec 3FR spectroradiometer was used to measure the in situ radiance (mW cm−2 nm−1 sr−1) in the 350–2500 nm spectral range. Reflectance was calculated by dividing the surface radiance by the downwelling radiance measured with a 99 % reflectance standard panel (Spectralon® plate). Hyperspectral reflectance data were then downscaled to the resolution of the matching satellite data using the sensor spectral response function, and NDVI was calculated. A total number of 57 ground-truth stations were obtained from in situ transects conducted during the three sampling campaigns. For each matchup station, three replicates per 30×30 m satellite pixel were measured in situ, averaged and compared to the corresponding satellite NDVI pixel.
2.3.3 Spatial analysis of MPB around oyster reefs
On the scale of the experimental site, NDVI spatial distribution around the two oyster reefs was characterized using transect analysis (dashed line through R1 and R2 in Fig. 2) and spatial statistics. Well-defined MPB spatial structures were recurrently observed around the reefs, hereafter referred to as NDVI patches. These patches were delimited following the boundary detection method (Dale and Fortin, 2014), by defining a NDVI minimum threshold value allowing the connection of pixels of a common value in a closed contour line. This delimitation was performed using an algorithm applied independently on each image and reef. Patch spatial properties (area, shape, patchiness) were then extracted independently for each image using spatial metrics calculated with the R SDMtools package (VanDerWal et al., 2014) based on the patch statistics provided by the FRAGSTATS software (McGarigal et al., 2012). Each patch was described by measuring its area and, calculated following Eqs. (2) and (3), respectively, the fractal dimension index (FDI) and aggregation index (AI) (McGarigal et al., 2012):
with pij the perimeter (m) of patch ij, aij the area (m2) of patch ij, gij the number of like adjacencies (joins) between pixels of patch type i based on the single-count method, and the maximum number of like adjacencies (joins) between pixels of patch type i based on the single-count method. The FDI allows patch shape complexity to be characterized, with a value of 1 indicating very simple perimeters, and a value of 2 representing highly convoluted perimeters. The AI, expressed as a percentage, defines the percentage of patch spatial aggregation, with 0 % expressing a maximally disaggregated patch, and 100 % a patch maximally aggregated into a single, compact patch.
In order to extract average NDVI values associated with both patches (around R1 and R2) throughout the time series, a “distance buffer” was created for each reef. From the patch areas determined in each image as described above, average areas were computed for each patch. These average areas were then used to apply isotropic, fixed-distance buffers of the same average area around each reef across the whole time series. NDVI values included within the distance buffers were extracted and averaged for each reef throughout the time series. MPB biomass response to the oyster killing was analysed by comparing R1 and R2 NDVI average values before and after the experiment. This method allowed the background noise induced by natural spatial and temporal variability in MPB to be excluded (e.g. related to seasonal development), by focusing on the biomass variation recorded between the control (R1) and the impacted reef (R2).
A composite monthly signal over the 1985–2015 time series was determined by clustering and averaging NDVI monthly data across the mudflat area. No data were available for December and January due to image acquisition technical constraints during the winter period (e.g. sun elevation).
2.4 Statistical analysis
All data processing, statistical analyses and graphical results were performed using R software (R Core Team, 2015). NDVI normality was tested using the car package (Fox and Weisberg, 2011) and the Shapiro–Wilk normality test (p = 0.34, n = 44 for the mudflat area; p = 0.64, n = 47 for the experimental site). Correlation between in situ and satellite NDVI was tested using Pearson product–moment correlation and the slope of the linear regression model applied to the data was compared to the isometric relation x = y. The root mean squared error (RMSE) of predicted values (satellite data) vs. observed values (in situ data) was calculated using the “Metrics” package (Hamner, 2012) and also used to assess multi-sensor interconsistency. Mean NDVI differences were tested with Student's t test for two samples and Kruskal–Wallis Rank Sum Test for multiple, unbalanced samples.
3.1 Multi-sensor RS of intertidal mudflats
3.1.1 SPOT and Landsat interconsistency
No significant differences were found in NDVI values from the diatom library downscaled to the satellites' spectral resolution (Kruskal–Wallis rank sum test, p = 0.62, n = 93). The coefficient of determination of all sensors' regressions was very high (R2 > 0.99, p < 0.001, n = 93), with a slope not significantly different from 1 and a RMSE systematically lower than 0.03 (n = 93) (Table 3).
Sensor interconsistency was further verified by comparing SPOT and Landsat mean NDVI throughout the time series. No significant difference was found on the scale of the mudflat area (Student's t test, p = 0.72) nor on the scale of the experimental site (p = 0.74). Satellite data accuracy evaluated using in situ ground-truthing showed a significant correlation (R2 = 0.73, p < 0.001) between in situ and satellite NDVI (Fig. 3).
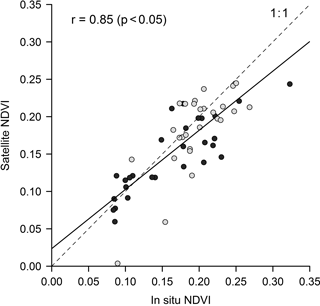
Figure 3Match-up between in situ and satellite NDVI (see Table 2). Black dots were sampled on the experimental site, while grey dots were sampled on the additional site of La Coupelasse. Pearson correlation coefficient was calculated. The slope of the linear regression model applied to the data (black linear regression line, RMSE = 0.04, n = 57) was not significantly different from 1 (a = 0.79, p = 0.67).
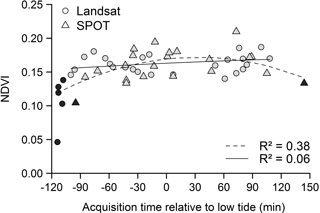
Figure 4Relationship between NDVI and timing of low tide across the mudflat area. Satellite (SPOT and Landsat) data mean NDVIs are represented according to their acquisition time relative to low tide (time = 0). Black symbols correspond to data characterized by a decrease of biomass due to the process of vertical migration and/or partial mudflat submersion (dotted polynomial regression line, p < 0.001). After removing them, the regression was no longer significant (grey symbols, full polynomial regression line, p = 0.30).
3.1.2 Influence of tidal stage on intertidal mudflat MPB RS
Satellite acquisition time in relation to the emersion period appeared to affect satellite NDVI measurements (Fig. 4). Unusually low NDVI values resulting either from MPB vertical migration and/or from partial mudflat submersion were detected on images acquired more than 100 min before and after low tide (black symbols in Fig. 4). These data were removed after which the remaining NDVI data (grey symbols in Fig. 4) were no longer correlated with any of the following tidal variables: acquisition time relative to low tide (Spearman correlation, r = 0.22, p = 0.15), water height (Pearson correlation, r = 0.20, p = 0.19), tide amplitude (Pearson correlation, r = −19, p = 0.21) and MPB coverage, as expressed by the number of pixels considered as MPB on the images (Pearson correlation, r = −11, p = 0.49). At the time of the acquisition, the average water height at the nearest reference harbour (Pornic, France) was 1.43 m (in a range from 0.34 to 2.29 m). For comparison, water height can be as high as 6 m during high-tide periods.
3.2 MPB spatio-temporal variability around oyster reefs
3.2.1 MPB spatial distribution on the mudflat scale
On average MPB covered 60.8 % ± 10.4 (mean ± SD) of the whole mudflat area. Over the 1985–2015 time series, MPB mean NDVI was 0.16 ± 0.02 for the mudflat area. Similar average values were observed for the experimental site. MPB spatial distribution generally showed regular patterns associated with bathymetry changes: NDVI maxima were consistently observed at about 2 m above the chart datum, whereas NDVI minima were located on the upper and lower shore. Visual interpretation of the RS images suggested that high NDVI values coincide with the proximity of oyster farms and wild oyster reefs (see Fig. S1 in the Supplement).
3.2.2 MPB spatial distribution around oyster reefs
Within the experimental site, NDVI spatial distribution was characterized by the existence of clearly defined patches around the oyster reefs (Fig. 2a). The identification of a patch around R1 and R2 was possible in 97 and 79 % of the images acquired before the BACI experiment, respectively. Patch mean NDVI was systematically higher-than-average NDVI value over the whole experimental site. No correlation was found between patch average NDVI and patch area for either R1 (R2 = 0.03, p = 0.22) or R2 (R2 = 0.02, p = 0.46). Distance buffers with diameters of 192 and 128 m were determined for R1 and R2 respectively (see Sect. 2.3.3).
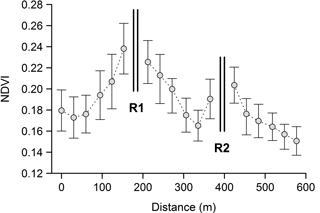
Figure 5NDVI extracted from a transect going through the two oyster reefs (see Fig. 2) based on satellite data acquired before the burning (1985–2014 time series, mean ± 95 % CI, n = 33). Black vertical bars correspond to the location of the reefs.
The average NDVI R1–R2 transect (dashed line in Fig. 2a) extracted from the data acquired before the BACI experiment highlights the influence of the oyster reefs on MPB spatial distribution (Fig. 5), with NDVI being consistently higher closer to the reefs, and decreasing with distance from each reef. A NDVI minimum was clearly observable between the two reefs, at about 150 m from R1 and 60 m from R2. Two transects crossing the reefs perpendicularly to the bathymetric lines (see Fig. S2) also showed high NDVI over a distance up to 150 m on either side of the reefs, with higher values recurrently observed for lower bathymetry, i.e. inferior to 2.5 m.
MPB FDI varied from 1 to 1.2, indicating that patches were characterized by simple and regular shapes. MPB AI ranged from 67 to 100 %, showing that the patches were generally very compact. Their shape was circular to elliptical most of the time, and in the latter case always expanded perpendicular to the bathymetric lines.
3.2.3 Seasonal variability
The NDVI showed significant seasonal variations on the scale of the mudflat area, the experimental site, around the control reef R1 and the impacted reef R2 (Kruskal–Wallis rank sum test, p < 0.05, p < 0.05, p < 0.01 and p < 0.05 respectively) (see Fig. S3). The MPB patch around R1 exhibited two pronounced MPB biomass peaks in April and October (Fig. 6a).
The spatial metrics of the MPB patches around R1 (Fig. 6b, c and d) and R2 (not shown) also showed clear seasonal variations, together with high interannual variability, as suggested by the pronounced error bars during the spring and autumnal periods. Patch areas around R1 and R2 were larger in spring than in summer, and the largest patches were observed in autumn, from September to November (Fig. 6b). Variations in patch area were associated with variations in patch structure, with high correlations between area and FDI (r = −0.6, p = 0.07), and between the area and the AI (r = 0.74, p < 0.05) (Fig. 6c, d). In general, larger patches corresponded to simpler (i.e. low FDI) and more aggregated shapes. Patches were especially compact around the reefs in September and October. From May to August, when MPB patch areas were smallest, their shapes became more complex (higher FDI) and less aggregated. Similar seasonal variations were generally observed for MPB patches in R1 and R2, but sometimes no patch could be delineated around R2 from March to June, corresponding to the spring–early summer period, despite high NDVI values. This was caused by the fact that the MPB biofilm around the reef was highly disaggregated (see also below).
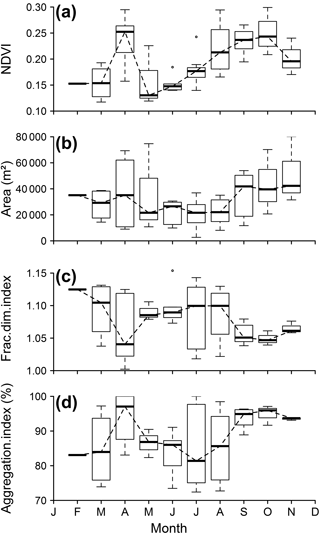
Figure 6NDVI and spatial metrics of the control reef NDVI patch during 1985–2015: (a) NDVI monthly variation in the control reef distance buffer, (b) patch area, (c) patch fractal dimension index and (d) patch aggregation index. Horizontal lines denote the median value, boxes represent first and third quartiles, and whiskers represent the last value within 1.5 times the interquartile distance.
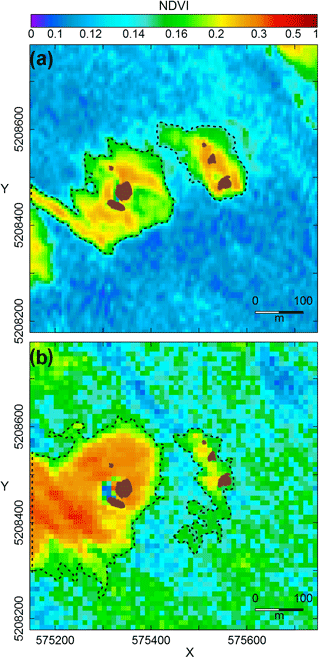
Figure 7MPB patches (dashed lines) around the control reef and the impacted reef (both represented in brown) showing the temporal evolution of their spatial structure before and after the BACI experiment: (a) 11 months before the BACI experiment (SPOT 6 image acquired on 20 August 2013), (b) 3 months after the experiment (SPOT 5 image acquired on 9 October 2014). Images are displayed at their original spatial resolution (6 and 10 m, respectively). Data were downscaled to 30 m for the analysis.
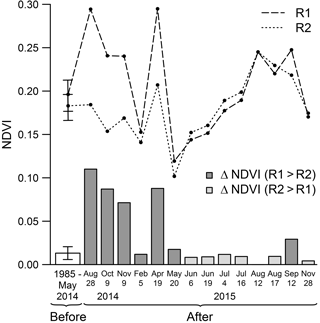
Figure 8R1 control reef (dashed line), R2 impacted reef (dotted line) mean NDVI, and R1 and R2 ΔNDVI (grey bars). Mean NDVI was calculated for each image of the time series based upon the distance buffer derived from the spatial metrics. R1 and R2 first points correspond to their respective mean NDVI before the BACI experiment (mean ± 95 % CI). The white bar corresponds to the mean difference observed between the patches before the BACI experiment (mean ± 95 % CI). The other values represent the means and differences observed between the patches on each image acquired after the BACI experiment.
3.3 Influence of oyster reefs on MPB: a BACI analysis
3.3.1 NDVI variations
During the autumn following the oyster killing, high mean NDVI values were observed around R1. In contrast, values around R2 tended to decrease, and in October 2014 the mean NDVI around R2 was below the pre-experiment monthly average value (Fig. 7). NDVI then gradually recovered to its usual level in the following months. Even higher NDVI values than the pre-experiment average were reached about 1 year later, from July to September 2015, despite the absence of a clearly identifiable patch structure around R2. This high NDVI was also observed around R1.
In order to distinguish the impact of the experiment from potential intersite variations, the difference of the averaged NDVI within the R1 and R2 buffers (hereafter denoted by ΔNDVI) was analysed before and after impact. Throughout the time series before the experiment, the average difference was 0.01 ± 0.02 (mean ± SD) (Fig. 8). The difference was significantly higher on the three images acquired within 3 months following the oyster killing (ΔNDVI was respectively 0.11, 0.09 and 0.07 in August, October and November 2014), as well as 9 months later in April 2015 (ΔNDVI of 0.09). The difference ratio is especially striking during the first months after the killing, considering that NDVI values around R1 were exceptionally high in 2014 compared to the seasonal average. One would therefore expect R2 NDVI values to also stand above average. The difference then progressively disappeared, and from May 2015 ΔNDVI was mostly within the range of values observed before the oyster killing.
3.3.2 Alteration of MPB spatial structure
The killing of the oysters colonizing R2 in July 2014 was followed by an alteration of MPB biomass and spatial distribution in the short and medium term. A clear change in spatial structure was observed for the first time 3 months after the biomanipulation on an image acquired during October 2014, with an increase in patch shape complexity and a decrease in aggregation percentage (Fig. 7). The impact was even more pronounced 1 year after the experiment as no patch could be identified around R2 on five consecutive images from July to September 2015. While MPB could still be observed in the vicinity of the reef, its structure was unusually disaggregated and did not allow for the detection of a clear patch. This had never been observed during this specific period of the year throughout the whole time series.
A high-spatial-resolution 30-year time series of Landsat and SPOT satellite data revealed the presence of persistent, highly concentrated MPB patches around two C. gigas oyster reefs in Bourgneuf Bay (France). The killing of oysters in one reef as part of a BACI experiment highlighted the positive impact of live oysters on MPB biomass development and dynamics. Taken together, our data thus suggest that the presence of live oyster reefs promotes MPB biomass development and affects MPB spatial distribution around the reef. This is consistent with the hypothesis that MPB development is stimulated by the release of dissolved organic and inorganic matter and biodeposits excreted by the oysters. As such, oysters and MPB would be connected in a local positive feedback loop with oysters “fertilizing” their main food source (Prins et al., 1997; Kasim and Mukai, 2006). Clear MPB seasonal dynamics consistent with the ecosystem location were also shown, and could be associated with variations in MPB patch shape. This first observation of a positive effect of live oyster reefs on MPB biofilms using RS time series can yield new insights into MPB dynamics and the impact of aquaculture on the environment.
4.1 Oyster reefs influence on MPB biofilm development
By focusing on an intertidal ecosystem dominated by long-established oyster communities, our 1985–2015 RS satellite time series revealed a close relationship between oysters and MPB biofilm development, with oyster reefs being associated with the presence of persistent MPB patches. The delimitation of such spatially explicit structures is generally not obvious when dealing with continuous variables (Jesus et al., 2005). In our study though, the systematically higher-than-average MPB biomass concentrations observed around the reefs allowed us to distinguish MPB patches from the background MPB biomass. In addition, the presence of reefs had a significant effect on the shape, area and degree of aggregation of the surrounding MPB patches in our BACI experiment. The negative impact of oyster killing on the surrounding MPB was reflected by its very limited autumnal increase in biomass compared to the typical autumnal development (see Sect. 4.2) around this reef and the control reef. Until mid-spring of the following year, MPB biomass was also lower than one would expect given the average pre-experiment values of the non-impacted biofilm. In situ reflectance measurements performed 10 months after the experiment confirmed this difference observed between the impacted and non-impacted biofilms (not shown). Oyster reefs, however, not only appeared to act as promoters of MPB biomass, but also as a factor structuring their spatial distribution. After the oysters were killed, MPB patches regularly showed a more complex and disaggregated structure instead of the regular patch shape usually observed. Variations in bathymetry, tidal dynamics, sediment type, irradiance and grazing had already been documented as factors structuring MPB spatio-temporal distribution (Brotas et al., 1995; Méléder et al., 2003; Sahan et al., 2007; Ribeiro et al., 2013). However, to date there are only a few studies which have explicitly investigated the influence of nearby macrobenthic communities on MPB dynamics (Dame, 1993; Méléder et al., 2003, 2007; Newell, 2004; Engel et al., 2017).
While nutrient availability has long been considered to be non-limiting in intertidal mudflats (Underwood and Kromkamp, 1999), the hypothesis of nutrient inputs coming from oysters is here advanced as possibly being the main factor explaining the higher biomass of surrounding MPB. Via the release of organic and inorganic matter through excretion and biodeposition (Dame, 1993; Cognie and Barillé, 1999; Newell, 2004; Buzin et al., 2015), oysters can have an impact by enriching the sediment around them, increasing nutrient availability and hence development of MPB (Dame, 1993; Garcia-Robledo et al., 2016). Oyster reefs are also known to have indirect effects such as modifying the structure of the surrounding sediment and the ambient hydrodynamic conditions, facilitating MPB establishment (Colden et al., 2016). In a recent paper on the impact of mussel beds on MPB biomass development, Engel et al. (2017) attributed the positive effect of such beds on MPB biomass to a combination of reduced hydrodynamic stress and increased nutrient availability (and also to potential changes in the associated invertebrate community). In contrast with the observations of Colden et al. (2016) and Engel et al. (2017), our BACI experiment, in which the oysters were killed while the physical structure of the reef itself was not modified, now allows the pure physical (hydrodynamic) effect to be distinguished from the biological (nutrient enrichment) effect of the oyster reefs, suggesting that the latter process is more important in our study area. In this respect, the resilience of MPB biomass development around the impacted reef, observed 1 year after the experiment, is probably due to the recolonization of the dead reef by young oysters, following the exceptionally high recruitment which occurred during autumn 2014 (Pouvreau et al., 2015). When measured in autumn 2015, 1 year after the experiment, the oysters newly colonizing the reef had already reached an average size of 32 ± 4 mm (n = 30), vs. an average size of 54.5 ± 17 mm (n = 30) for adult oysters on the control site.
While the positive impact of oyster reefs on MPB biomass is clear from both the long-term satellite and the BACI experimental data, a temporary negative effect of burning oyster biomass and straw on MPB biomass cannot be ruled out. Toxic compounds resulting from (incomplete) combustion of biomass could negatively affect MPB growth and as such reduce biomass. However, in our opinion the long-term negative effect on MPB biomass observed in the experiment is unlikely to be caused by such compounds because of the strong dilution effect caused by the daily tidal immersion and emersion at the study site. In contrast, the elimination of the above-mentioned oyster enrichment effect by oyster killing would have been a more lasting effect, as was observed in the experiment.
The use of a RS historical time series (29 years of data before the experiment and more than 1 year of data after it) allowed for a simultaneous collection of data for both the control and impact sites, and for the differentiation of seasonal and interannual variability from the variability due to the impact of the experiment. The paired monitoring of the control and impacted reefs confirmed a low intersite variability, while their proximity submitted them to similar environmental conditions (Stewart-Oaten et al., 1986). Unfortunately, the nature, scale and location (nearly 1 km from the shore) of the experiment made replication not possible. However, while we recognize the limitation of the unreplicated design, we believe that our experimental design is suitable to distinguish site-specific NDVI variations from the average mudflat trend (Hewitt et al., 2001), as (1) satellite data of the control site showed trends consistent with whole mudflat MPB temporal dynamics, and (2) they also revealed no response related to the experiment, underscoring their reliability as control values.
4.2 MPB temporal dynamics
MPB in Bourgneuf Bay exhibited pronounced large-scale seasonal dynamics together with a generally limited interannual variability, indicating a stable spatio-temporal structure over time (Ubertini et al., 2012). The combination of all images in a composite monthly signal (see Table S2) highlighted two peaks of MPB biomass occurring during spring and autumn. These variations were similar on both the reef and the whole mudflat scale, although peaks of biomass were more pronounced in the immediate proximity of the oyster reefs (see Fig. S3). Variations in MPB patch size, shape and degree of aggregation followed these seasonal trends, with MPB spatial structure tending to aggregate into larger and more regular patches around the oyster reefs during spring and autumn. The seasonal cycle in Bourgneuf Bay also corresponds to those observed in other European mudflats. In some northwestern European flats, MPB maxima have been detected slightly later in spring and in September (van der Wal et al., 2010). The summer depression has been observed in some more southerly mudflats such as Marennes-Oléron Bay (France) (Cariou-Le Gall and Blanchard, 1995), the Tagus estuary (Portugal) (Brito et al., 2013), Cadiz Bay (Spain) (Garcia-Robledo et al., 2016), and in the Wadden Sea (The Netherlands) (van der Wal et al., 2010; Stief et al., 2013), and has been related to increased macrobenthos grazing in summer (Peletier, 1996; Weerman et al., 2011). Low NDVI values in summer may also be due to thermo-inhibition when sediment temperature exceeds 25 ∘C (Blanchard et al., 1997).
4.3 RS as a tool to investigate multi-scale ecological processes
MPB colonizing mudflats around oyster reefs are dominated by epipelic life forms (Méléder et al., 2007) which often exhibit marked seasonal dynamics (Haubois et al., 2005). Pronounced variation in NDVI can also be observed on the scale of the tidal cycle (Méléder et al., 2003). This is confirmed by our data, which show that at about 1.5 to 2 h before and after low tide, NDVI is lower. This can mainly be attributed to upward and downward vertical migration of epipelic MPB after and before submersion, respectively. The water height 2 h after low tide corresponds to an average of more than 2.3 m on the scale of the mudflat area, meaning that the experimental site is almost covered by the tide. MPB migration is therefore very rapid (Herlory et al., 2004; Coelho et al., 2011), happening only shortly after the water leaves and before it comes back, as it was observed in Méléder et al. (2003). While RS provides large datasets of images, careful selection of images with respect to tidal stage is hence necessary to avoid observing low NDVI values related to vertical migration. Fortunately, the high speed of MPB migration allowed us to work with satellite data that could be obtained during most of the low-tide period. It should also be kept in mind that while MPB biomass at the surface of the sediment can change as a result of vertical migration (Brouwer and Stal, 2001; Chennu et al., 2013), it can also be affected by other exogenous factors such as temperature and irradiance (Saburova and Polikarpov, 2003; Jesus et al., 2009; Coelho et al., 2011). A better understanding of these temporal dynamics requires high spatial and temporal resolution RS data.
In this study, the combination of SPOT and Landsat data allowed monitoring of MPB dynamics across different spatial (101–104 m) and temporal (months to decades) scales. This underlines the interest of using multispectral, multi-sensor RS as a monitoring tool of MPB dynamics (Dube, 2012). A multi-sensor approach exposes different sources of variability coming from sensor technical features, differences in spatial and spectral resolutions, band position and width for the computation of indices, and choice of the atmospheric correction. However, the standardization of the data and careful quality control allows the building of robust and consistent satellite time series. Moreover, as NDVI is little influenced by the sediment background (Barillé et al., 2011), satellite RS constitutes a valuable tool to map MPB spatio-temporal dynamics over a variety of muddy and sandy ecosystems. Although it does not allow to identify the cause of the patterns observed, it provided a sufficiently explicit spatial tool able to describe MPB structure on the mesoscale. It also made it possible to quantify the size of the detectable reef footprint (Giles et al., 2009) through the NDVI in our study. Satellite RS hence facilitates the general detection of environmental and anthropogenic disturbances on large scales (Kerr and Ostrovsky, 2003) at a high spatial and temporal resolution (Ibrahim and Monbaliu, 2011).
Data ground-truthing, however, remains essential when using RS (Forster and Jesus, 2006) and should be performed according to the RS spatial resolution (Paterson et al., 1998), which was done here by adapting the sampling plan to match the size of the sensors' pixels. The issue of MPB microscale patchiness was limited by performing replicate measurements so as to be as representative as possible of the surface considered. Concerning MPB monitoring, Landsat and SPOT data did not allow the differentiation between micro- and macroalgae given the position of their spectral bands. The setting of empirical thresholds and field knowledge, however, allowed exclusion of non-MPB organisms. However, mixed signals due to the spatial association of objects showing different spectral signatures remains possible, and no field information is available for many mudflats worldwide, stressing the need for satellite data with higher spectral resolution than SPOT and Landsat. Moreover, while the downscaling of finer resolution data to the lowest one (i.e. Landsat 30 m spatial resolution) enabled the problems related to spatial heterogeneity between the sensors to be reduced, it may result in potential loss of information. The new generation of satellite data will enable a better analysis in both cases, with, for example, Sentinel 2 MultiSpectral Instrument providing 10 m resolution images, more spectral bands and high revisiting time (5 days with both Sentinel 2A and 2B combined).
All data are available upon request to the authors.
The supplement related to this article is available online at: https://doi.org/10.5194/bg-15-905-2018-supplement.
CE conducted the analysis, prepared the figures and wrote the paper. CE and PG conducted fieldwork. PG and LB helped conducting the analysis. PG, VM, BJ, BC, PD, KS and LB contributed to the writing of the paper.
The authors declare that they have no conflict of interest.
The authors wish to thank the CNES for the ISIS Program regarding the use of
SPOT satellite products. SPOT6-7 data were acquired by Airbus Defence and
Space in the frame of the MyGIC project. We also thank the pole THEIA and the
CESBIO for the provision of SPOT Take5 (CNES) and Landsat (USGS) data. The
ASD FieldSpec 3FR spectroradiometer was supplied by the Laboratoire de
Planétologie et Géodynamique (LPG UMR-CNRS 6112) of the University of
Nantes. We thank Laurent Godet for his help with spatial metrics. We
acknowledge the CNES and Région Pays de Loire for the funding of Caroline
Echappé's PhD. The oyster
experiment has been designed and implemented in the framework of the COSELMAR
project funded by the Région Pays de la Loire.
Edited by: Emmanuel Boss
Reviewed by: two anonymous referees
Barillé, L., Méléder, V., Combe, J.-P., Launeau, P., Rincé, Y., Carrère, V., and Morançais, M.: Comparative analysis of field and laboratory spectral reflectances of benthic diatoms with a modified Gaussian model approach, J. Exp. Mar. Biol. Ecol., 343, 197–209, https://doi.org/10.1016/j.jembe.2006.11.013, 2007.
Barillé, L., Mouget, J.-L., Méléder, V., Rosa, P., and Jesus, B.: Spectral response of benthic diatoms with different sediment backgrounds, Remote Sens. Environ., 115, 1034–1042, https://doi.org/10.1016/j.rse.2010.12.008, 2011.
Benyoucef, I., Blandin, E., Lerouxel, A., Jesus, B., Rosa, P., Meleder, V., Launeau, P., and Barille, L.: Microphytobenthos interannual variations in a north-European estuary (Loire estuary, France) detected by visible-infrared multispectral remote sensing, Estuar. Coast. Shelf S., 136, 43–52, 2014.
Bivand, R. and Lewin-Koh, N.: maptools: Tools for Reading and Handling Spatial Objects, R package version 0.8-37, available at: http://CRAN.R-project.org/package=maptools (last access: 31 January 2018), 2015.
Bivand, R. and Rundel, C.: rgeos: Interface to Geometry Engine – Open Source (GEOS), R package version 0.3-15, available at: http://CRAN.R-project.org/package=rgeos (last access: 31 January 2018), 2015.
Bivand, R., Keitt, T., and Rowlingson, B.: rgdal: Bindings for the Geospatial Data Abstraction Library, R package version 1.1-1, available at: http://CRAN.R-project.org/package=rgdal (last access: 31 January 2018), 2015.
Bivand, R. S., Pebesma, E., and Gómez-Rubio, V.: Applied Spatial Data Analysis with R, Springer New York, New York, NY, available at: http://link.springer.com/10.1007/978-1-4614-7618-4 (last access: 12 June 2015), 2013.
Blanchard, G. F., Guarini, J.-M., Gros, P., and Richard, P.: Seasonal Effect on the Relationship Between the Photosynthetic Capacity of Intertidal Microphytobenthos and Temperature, J. Phycol., 33, 723–728, https://doi.org/10.1111/j.0022-3646.1997.00723.x, 1997.
Brandt, G., Wehrmann, A., and Wirtz, K. W.: Rapid invasion of Crassostrea gigas into the German Wadden Sea dominated by larval supply, J. Sea Res., 59, 279–296, https://doi.org/10.1016/j.seares.2008.03.004, 2008.
Brito, A. C., Benyoucef, I., Jesus, B., Brotas, V., Gernez, P., Mendes, C. R., Launeau, P., Dias, M. P., and Barillé, L.: Seasonality of microphytobenthos revealed by remote-sensing in a South European estuary, Cont. Shelf Res., 66, 83–91, https://doi.org/10.1016/j.csr.2013.07.004, 2013.
Brotas, V., Cabrita, T., Portugal, A., Serôdio, J., and Catarino, F.: Spatio-temporal distribution of the microphytobenthic biomass in intertidal flats of Tagus Estuary (Portugal), Hydrobiologia, 300–301, 93–104, https://doi.org/10.1007/BF00024451, 1995.
Brouwer, J. F. C. and Stal, L. J.: Short-term dynamics in microphytobenthos distribution and associated extracellular carbohydrates in surface sediments of an intertidal mudflat, Mar. Ecol. Prog. Ser., 218, 33–44, 2001.
Buzin, F., Dupuy, B., Lefebvre, S., Barillé, L., and Haure, J.: Storage of Pacific oysters Crassostrea gigas in recirculating tank: Ammonia excretion and potential nitrification rates, Aquac. Eng., 64, 8–14, https://doi.org/10.1016/j.aquaeng.2014.11.007, 2015.
Cariou-Le Gall, V. and Blanchard, G. F.: Monthly HPLC measurements of pigment concentration from an intertidal muddy sediment of Marennes-Oléron Bay, France, Mar. Ecol. Prog. Ser., 121, 171–179, https://doi.org/10.3354/meps121171, 1995.
Chapman, M., Tolhurst, T., Murphy, R., and Underwood, A.: Complex and inconsistent patterns of variation in benthos, micro-algae and sediment over multiple spatial scales, Mar. Ecol. Prog. Ser., 398, 33–47, https://doi.org/10.3354/meps08328, 2010.
Chennu, A., Färber, P., Volkenborn, N., Al-Najjar, M. A. A., Janssen, F., de Beer, D., and Polerecky, L.: Hyperspectral imaging of the microscale distribution and dynamics of microphytobenthos in intertidal sediments: Hyperspectral imaging of MPB biofilms, Limnol. Oceanogr.-Meth., 11, 511–528, https://doi.org/10.4319/lom.2013.11.511, 2013.
Coelho, H., Vieira, S., and Serôdio, J.: Endogenous versus environmental control of vertical migration by intertidal benthic microalgae, Eur. J. Phycol., 46, 271–281, https://doi.org/10.1080/09670262.2011.598242, 2011.
Cognie, B. and Barillé, L.: Does bivalve mucus favour the growth of their main food source, microalgae?, Oceanol. Acta, 22, 441–450, https://doi.org/10.1016/S0399-1784(00)88727-7, 1999.
Cognie, B., Haure, J., and Barillé, L.: Spatial distribution in a temperate coastal ecosystem of the wild stock of the farmed oyster Crassostrea gigas (Thunberg), Aquaculture, 259, 249–259, https://doi.org/10.1016/j.aquaculture.2006.05.037, 2006.
Colden, A. M., Fall, K. A., Cartwright, G. M., and Friedrichs, C. T.: Sediment Suspension and Deposition Across Restored Oyster Reefs of Varying Orientation to Flow: Implications for Restoration, Estuaries Coasts, 39, 1435–1448, https://doi.org/10.1007/s12237-016-0096-y, 2016.
Combe, J.-P., Launeau, P., Carrère, V., Despan, D., Méléder, V., Barillé, L., and Sotin, C.: Mapping microphytobenthos biomass by non-linear inversion of visible-infrared hyperspectral images, Remote Sens. Environ., 98, 371–387, https://doi.org/10.1016/j.rse.2005.07.010, 2005.
Consalvey, M., Paterson, D. M., and Underwood, G. J.: The ups and downs of life in a benthic biofilm: migration of benthic diatoms, Diatom Res., 19, 181–202, 2004.
Dale, M. R. T. and Fortin, M.-J.: Spatial analysis: a guide for ecologists, 2nd Edn., Cambridge University Press, Cambridge, New York, 2014.
Dame, R. and Libes, S.: Oyster reefs and nutrient retention in tidal creeks, J. Exp. Mar. Biol. Ecol., 171, 251–258, 1993.
Dame, R. F. (Ed.): The role of bivalve filter feeder material fluxes in estuarine ecosystems, in: Bivalve Filter Feeders, Springer Berlin Heidelberg, Nato ASI Series (Series G: Ecological Sciences), 33, 245–269, 1993.
Decottignies, P., Beninger, P. G., Rincé, Y., Robins, R. J., and Riera, P.: Exploitation of natural food sources by two sympatric, invasive suspension-feeders: Crassostrea gigas and Crepidula fornicata, Mar. Ecol. Prog. Ser., 334, 179–192, https://doi.org/10.3354/meps334179, 2007.
De Jonge, V. N. and Van Beuselom, J. E. E.: Contribution of resuspended microphytobenthos to total phytoplankton in the Ems estuary and its possible role for grazers, Neth. J. Sea Res., 30, 91–105, https://doi.org/10.1016/0077-7579(92)90049-K, 1992.
Diederich, S.: High survival and growth rates of introduced Pacific oysters may cause restrictions on habitat use by native mussels in the Wadden Sea, J. Exp. Mar. Biol. Ecol., 328, 211–227, https://doi.org/10.1016/j.jembe.2005.07.012, 2006.
Dube, T.: Primary productivity of the intertidal mudflats of the Wadden Sea: a remote sensing method, thesis, University of Twente, Enschede, the Netherlands, 2012.
Dutertre, M., Beninger, P. G., Barillé, L., Papin, M., and Haure, J.: Rising water temperatures, reproduction and recruitment of an invasive oyster, Crassostrea gigas, on the French Atlantic coast, Mar. Environ. Res., 69, 1–9, https://doi.org/10.1016/j.marenvres.2009.07.002, 2010.
Engel, F. G., Alegria, J., Andriana, R., Donadi, S., Gusmao, J. B., van Leeuwe, M. A., Matthiessen, B., and Eriksson, B. K.: Mussel beds are biological power stations on intertidal flats, Estuar. Coast. Shelf S., 191, 21–27, https://doi.org/10.1016/j.ecss.2017.04.003, 2017.
Forster, R. M. and Jesus, B.: Field spectroscopy of estuarine intertidal habitats, Int. J. Remote Sens., 27, 3657–3669, https://doi.org/10.1080/01431160500500367, 2006.
Fox, J. and Weisberg, S.: An R companion to applied regression, 2nd Edn., SAGE Publications, Thousand Oaks, CA, USA, 2011.
Garcia-Robledo, E., Bohorquez, J., Corzo, A., Jimenez-Arias, J. L., and Papaspyrou, S.: Dynamics of inorganic nutrients in intertidal sediments: porewater, exchangeable, and intracellular pools, Front. Microbiol., 7, 761, https://doi.org/10.3389/fmicb.2016.00761, 2016.
Giles, H., Broekhuizen, N., Bryan, K. R., and Pilditch, C. A.: Modelling the dispersal of biodeposits from mussel farms: The importance of simulating biodeposit erosion and decay, Aquaculture, 291, 168–178, https://doi.org/10.1016/j.aquaculture.2009.03.010, 2009.
Grizel, H. and Héral, M.: Introduction into France of the Japanese oyster (Crassostrea gigas), ICES J. Mar. Sci., 47, 399–403, https://doi.org/10.1093/icesjms/47.3.399, 1991.
Hagolle, O., Huc, M., Pascual, D., and Dedieu, G.: A multi-temporal and multi-spectral method to estimate aerosol optical thickness over land, for the atmospheric correction of FormoSat-2, LandSat, VENUS and Sentinel-2 images, Remote Sens., 7, 2668–2691, https://doi.org/10.3390/rs70302668, 2015a.
Hagolle, O., Sylvander, S., Huc, M., Claverie, M., Clesse, D., Dechoz, C., Lonjou, V., and Poulain, V.: SPOT-4 (Take 5): Simulation of Sentinel-2 time series on 45 large sites, Remote Sens., 7, 12242–12264, https://doi.org/10.3390/rs70912242, 2015b.
Hamner, B.: Metrics: Evaluation metrics for machine learning, R package version 0.1.1, available at: http://CRAN.R-project.org/package=Metrics (last access: 31 January 2018), 2012.
Haubois, A.-G., Sylvestre, F., Guarini, J.-M., Richard, P., and Blanchard, G. F.: Spatio-temporal structure of the epipelic diatom assemblage from an intertidal mudflat in Marennes-Oléron Bay, France, Estuar. Coast. Shelf S., 64, 385–394, https://doi.org/10.1016/j.ecss.2005.03.004, 2005.
Herlory, O., Guarini, J.-M., Richard, P., and Blanchard, G.: Microstructure of microphytobenthic biofilm and its spatio-temporal dynamics in an intertidal mudflat (Aiguillon Bay, France), Mar. Ecol. Prog. Ser., 282, 33–44, 2004.
Hewitt, J. E., Thrush, S. E., and Cummings, V. J.: Assessing Environmental Impacts: Effects of Spatial and Temporal Variability at Likely Impact Scales, Ecol. Appl., 11, 1502–1516, https://doi.org/10.1890/1051-0761(2001)011[1502:AEIEOS]2.0.CO;2, 2001.
Hijmans, R. J.: raster: Geographic Data Analysis and Modeling. R package version 2.4-20, available at: http://CRAN.R-project.org/package=raster, (last access: 31 January 2018), 2015.
Humphreys, J., Herbert, R. J. H., Roberts, C., and Fletcher, S.: A reappraisal of the history and economics of the Pacific oyster in Britain, Aquaculture, 428–429, 117–124, https://doi.org/10.1016/j.aquaculture.2014.02.034, 2014.
Ibrahim, E. and Monbaliu, J.: Suitability of spaceborne multispectral data for inter-tidal sediment characterization: A case study, Estuar. Coast. Shelf S., 92, , 437–445, https://doi.org/10.1016/j.ecss.2011.01.017, 2011.
Jesus, B., Brotas, V., Marani, M., and Paterson, D. M.: Spatial dynamics of microphytobenthos determined by PAM fluorescence, Estuar. Coast. Shelf S., 65, 30–42, https://doi.org/10.1016/j.ecss.2005.05.005, 2005.
Jesus, B., Brotas, V., Ribeiro, L., Mendes, C. R., Cartaxana, P., and Paterson, D. M.: Adaptations of microphytobenthos assemblages to sediment type and tidal position, Cont. Shelf Res., 29, 1624–1634, https://doi.org/10.1016/j.csr.2009.05.006, 2009.
Kasim, M. and Mukai, H.: Contribution of benthic and epiphytic diatoms to clam and oyster production in the Akkeshi-ko estuary, J. Oceanogr., 3, 267–281, https://doi.org/10.1007/s10872-006-0051-9, 2006.
Kerr, J. T. and Ostrovsky, M.: From space to species: ecological applications for remote sensing, Trends Ecol. Evol., 18, 299–305, https://doi.org/10.1016/S0169-5347(03)00071-5, 2003.
Kromkamp, J., Barranguet, C., and Peene, J.: Determination of microphytobenthos PSII quantum efficiency and photosynthetic activity by means of variable chlorophyll fluorescence, Mar. Ecol. Prog. Ser., 162, 45–55, https://doi.org/10.3354/meps162045, 1998.
Le Bris, A., Rosa, P., Lerouxel, A., Cognie, B., Gernez, P., Launeau, P., Robin, M., and Barillé, L.: Hyperspectral remote sensing of wild oyster reefs, Estuar. Coast. Shelf S., 172, 1–12, https://doi.org/10.1016/j.ecss.2016.01.039, 2016.
MacIntyre, H. L., Geider, R. J., and Miller, D. C.: Microphytobenthos: The ecological role of the “secret garden” of unvegetated, shallow-water marine habitats. I. Distribution, abundance and primary production, Estuaries, 19, 186–201, https://doi.org/10.2307/1352224, 1996.
Matthew, M. W., Adler-Golden, S. M., Berk, A., Richtsmeier, S. C., Levine, R. Y., Bernstein, L. S., Acharya, P. K., Anderson, G. P., Felde, G. W., Hoke, M. L., Ratkowski, A. J., Burke, H. K., Kaiser, R. D. and Miller, D. P.: Status of atmospheric correction using a MODTRAN4-based algorithm, Proc. SPIE, 4049, 199–207, 2000.
McGarigal, K., Cushman, S. A., and Ene, E.: FRAGSTATS v4: Spatial Pattern Analysis Program for Categorical and Continuous Maps, Computer software program produced by the authors at the University of Massachusetts, Amherst, available at: http://www.umass.edu/landeco/research/fragstats/fragstats.html (last access: 31 January 2018), 2012.
Méléder, V., Launeau, P., Barille, L., and Rince, Y.: Microphytobenthos assemblage mapping by spatial visible-infrared remote sensing in a shellfish ecosystem, C. R. Biol., 326, 377–389, https://doi.org/10.1016/S1631-0691(03)00125-2, 2003.
Méléder, V., Rincé, Y., Barillé, L., Gaudin, P., and Rosa, P.: Spatio-temporal changes in microphytobenthos assemblages in a macrotidal flat (Bourgneuf Bay, France), J. Phycol., 43, 1177–1190, https://doi.org/10.1111/j.1529-8817.2007.00423.x, 2007.
Miller, D. C., Geider, R. J., and MacIntyre, H. L.: Microphytobenthos: The ecological role of the “`secret garden”' of unvegetated, shallow-water marine habitats. 2. Role in sediment stability and shallow-water food webs, Estuaries, 19, 202–212, https://doi.org/10.2307/1352225, 1996.
Newell, R. I. E.: Ecosystem influences of natural and cultivated populations of suspension-feeding bivalve molluscs: A review, J. Shellfish Res., 23, 51–61, 2004.
Paterson, D. M., Wiltshire, K. H., Miles, A., Blackburn, J., Davidson, I., Yates, M. G., McGrorty, S., and Eastwood, J. A.: Microbiological mediation of spectral reflectance from intertidal cohesive sediments, Limnol. Oceanogr., 43, 1207–1221, 1998.
Pebesma, E. J.: Multivariable geostatistics in S: the gstat package, Comput. Geosci., 30 683–691, 2004.
Pebesma, E. J. and Bivand, R. S.: Classes and methods for spatial data in R, R News, 5, 9–13, 2005.
Peletier, H.: Long-term changes in intertidal estuarine diatom assemblages related to reduced input of organic waste, Mar. Ecol. Prog. Ser., 137, 265–271, 1996.
Perkins, R., Lavaud, J., Serôdio, J., Mouget, J., Cartaxana, P., Rosa, P., Barille, L., Brotas, V., and Jesus, B.: Vertical cell movement is a primary response of intertidal benthic biofilms to increasing light dose, Mar. Ecol. Prog. Ser., 416, 93–103, https://doi.org/10.3354/meps08787, 2010.
Pouvreau, S., Petton, S., Queau, I., Haurie, A., Le Souchu, P., Alunno-Bruscia, M., Palvadeau, H., Auby, I., Maurer, D., D'Amico, F., Passoni, S., Barbier, C., Tournaire, M.-P., Rigouin, L., Rumebe, M., Fleury, E., Fouillaron, P., Bouget, J.-F., Pepin, J.-F., Robert, S., Grizon, J., Seugnet, J.-L., Chabirand, J.-M., Le Moine, O., Guesdon, S., Lagarde, F., Mortreux, S., Le Gall, P., Messiaen, G., Roque D'Orbcastel, E., Quemener, L., Repecaud, M., Mille, D., Geay, A., and Bouquet, A.-L.: Observer, analyser et gérer la variabilité de la reproduction et du recrutement de l'huître creuse en France?: Le Réseau Velyger, Rapport annuel 2014, 57 pp., available at: http://archimer.ifremer.fr/doc/00279/38990/ (last access: 5 December 2016), 2015.
Prins, T. C., Smaal, A. C., and Dame, R. F.: A review of the feedbacks between bivalve grazing and ecosystem processes, Aquat. Ecol., 31, 349–359, https://doi.org/10.1023/A:1009924624259, 1997.
R Core Team: R: A language and environment for statistical computing, RR Foundation for Statistical Computing, Vienna, Austria, 2015.
Ribeiro, L., Brotas, V., Rincé, Y., and Jesus, B.: Structure and diversity of intertidal benthic diatom assemblages in contrasting shores: a case study from the Tagus estuary1, J. Phycol., 49, 258–270, https://doi.org/10.1111/jpy.12031, 2013.
Ruesink, J. L., Lenihan, H. S., Trimble, A. C., Heiman, K. W., Micheli, F., Byers, J. E., and Kay, M. C.: Introduction of non-native oysters: ecosystem effects and restoration implications, Annu. Rev. Ecol. Evol. S., 36, 643–689, 2005.
Saburova, M. A. and Polikarpov, I. G.: Diatom activity within soft sediments: behavioural and physiological processes, Mar. Ecol. Prog. Ser., 251, 15–126, 2003.
Sahan, E., Sabbe, K., Creach, V., HernandezRaquet, G., Vyverman, W., Stal, L. J., and Muyzer, G.: Community structure and seasonal dynamics of diatom biofilms and associated grazers in intertidal mudflats, Aquat. Microb. Ecol., 47, 253–266, https://doi.org/10.3354/ame047253, 2007.
Serôdio, J., Marques da Silva, J., and Catarino, F.: Nondestructive tracing of migratory rhythms of intertidal benthic microalgae using in vivo chlorophyll a fluorescence, J. Phycol., 33, 542–553, 1997.
Stewart-Oaten, A., Murdoch, W. W., and Parker, K. R.: Environmental Impact Assessment: “Pseudoreplication” in Time?, Ecology, 67, 929–940, https://doi.org/10.2307/1939815, 1986.
Stief, P., Kamp, A., and de Beer, D.: Role of diatoms in the spatial-temporal distribution of intracellular nitrate in intertidal sediment, edited by: Thrush, S., PLoS ONE, 8, e73257, https://doi.org/10.1371/journal.pone.0073257, 2013.
Thomas, Y., Pouvreau, S., Alunno-Bruscia, M., Barillé, L., Gohin, F., Bryère, P., and Gernez, P.: Global change and climate-driven invasion of the Pacific oyster (Crassostrea gigas) along European coasts: a bioenergetics modelling approach, J. Biogeogr., 43, 568–579, https://doi.org/10.1111/jbi.12665, 2016.
Troost, K.: Causes and effects of a highly successful marine invasion: Case-study of the introduced Pacific oyster Crassostrea gigas in continental NW European estuaries, J. Sea Res., 64, 145–165, https://doi.org/10.1016/j.seares.2010.02.004, 2010.
Ubertini, M., Lefebvre, S., Gangnery, A., Grangeré, K., Gendre, R. L., and Orvain, F.: Spatial variability of benthic-pelagic coupling in an estuary ecosystem: consequences for microphytobenthos resuspension phenomenon, PLoS ONE, 7, e44155, https://doi.org/10.1371/journal.pone.0044155, 2012.
Underwood, G. J. C. and Kromkamp, J.: Primary production by phytoplankton and microphytobenthos in estuaries, in: Advances in Ecological Research, Academic Press Inc., Elsevier, 29, 93–153, https://doi.org/10.1016/S0065-2504(08)60192-0, 1999.
van der Wal, D., Wielemaker-van den Dool, A., and Herman, P. M. J.: Spatial synchrony in intertidal benthic algal biomass in temperate coastal and estuarine ecosystems, Ecosystems, 13, 338–351, https://doi.org/10.1007/s10021-010-9322-9, 2010.
van der Wal, D., van Dalen, J., Wielemaker-van den Dool, A., Dijkstra, J. T., and Ysebaert, T.: Biophysical control of intertidal benthic macroalgae revealed by high-frequency multispectral camera images, J. Sea Res., 90, 111–120, https://doi.org/10.1016/j.seares.2014.03.009, 2014.
VanDerWal, J., Falconi, L., Januchowski, S., Shoo, L., and Storlie, C.: SDMTools: Species Distribution Modelling Tools: Tools for processing data associated with species distribution modelling exercises, R package version 1.1-221, available at: http://CRAN.R-project.org/package=SDMTools (last access: 31 January 2018), 2014.
Weerman, E. J., Herman, P. M. J., and Van de Koppel, J.: Top-down control inhibits spatial self-organization of a patterned landscape, Ecology, 92, 487–495, https://doi.org/10.1890/10-0270.1, 2011.