the Creative Commons Attribution 4.0 License.
the Creative Commons Attribution 4.0 License.
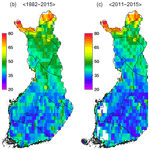
Monitoring changes in forestry and seasonal snow using surface albedo during 1982–2016 as an indicator
Terhikki Manninen
Tuula Aalto
Tiina Markkanen
Mikko Peltoniemi
Kristin Böttcher
Sari Metsämäki
Kati Anttila
Pentti Pirinen
Antti Leppänen
Ali Nadir Arslan
The surface albedo time series, CLARA-A2 SAL, was used to study trends in the snowmelt start and end dates, the melting season length and the albedo value preceding the melt onset in Finland during 1982–2016. In addition, the melt onset from the JSBACH land surface model was compared with the timing of green-up estimated from Moderate Resolution Imaging Spectroradiometer (MODIS) data. Moreover, the melt onset was compared with the timing of the greening up based on MODIS data. Similarly, the end of snowmelt timing predicted by JSBACH was compared with the melt-off dates based on the Finnish Meteorological Institute (FMI) operational in situ measurements and the Fractional Snow Cover (FSC) time-series product provided by the EU FP7 CryoLand project. It was found that the snowmelt date estimated using the 20 % threshold of the albedo range during the melting period corresponded well to the melt estimate of the permanent snow layer. The longest period, during which the ground is continuously half or more covered by snow, defines the permanent snow layer (Solantie et al., 1996). The greening up followed within 5–13 days the date when the albedo reached the 1 % threshold of the albedo dynamic range during the melting period. The time difference between greening up and complete snowmelt was smaller in mountainous areas than in coastal areas. In two northern vegetation map areas (Northern Karelia–Kainuu and Southwestern Lapland), a clear trend towards earlier snowmelt onset (5–6 days per decade) and increasing melting season length (6–7 days per decade) was observed. In the forested part of northern Finland, a clear decreasing trend in albedo (2 %–3 % per decade in absolute albedo percentage) before the start of the melt onset was observed. The decreasing albedo trend was found to be due to the increased stem volume.
- Article
(3135 KB) - Full-text XML
- BibTeX
- EndNote
Surface albedo is the fraction of incoming solar radiation reflected hemispherically by the surface. It is one of the essential climate variables (ECVs). It serves as an indicator of climate change, and changes in the albedo also affect the climate (Karlsson et al., 2016). Regarding the net climate effect, both land carbon budget and properties of the land surface (e.g. albedo, surface roughness) are globally significant (Davin and de Noblet-Ducoudré, 2010). The boreal forest zone is sensitive to changes in local and global climate and provides an important component to the Northern Hemisphere carbon budget (Parry et al., 2007). The forest cover has a very significant influence on the Northern Hemisphere albedo (Bonan et al., 1992; Randerson et al., 2006). Change in albedo is an important mechanism by which forests modify climate in boreal regions, but the net effect is uncertain due to simultaneous change in carbon sequestration (Betts, 2000). If the length of the snow-covered season decreases and snow melts earlier in spring due to climate change, the albedo of the forest areas decreases earlier in spring, which enhances climate change. On the other hand, if the northern forest edge moves further north due to climate change, the winter- and springtime albedo will show a notable decrease, which will enhance climate change. In addition, changes in species, e.g. from deciduous (such as mountain forests typical of Arctic and subarctic areas) to coniferous, would decrease the albedo of the snow-covered season noticeably. Previously, it has been shown that the climatic and vegetational zones are equivalent in the boreal forests (Solantie, 2005). Hence, a significant change in climate would result in a change in vegetational zones.
Changes in land management may already have a significant effect on surface temperature, equal to that of land cover change (Luyssaert et al., 2014). Changes in forest management practices can markedly affect the boreal forest albedo through changes in leaf area index (LAI), biomass or canopy density, especially in winter and spring when the forest floor is covered by snow. In Finland, the forests are to a large extent managed, and the changes in forest structure and stocking are monitored by the National Forest Inventory (Tomppo et al., 2011).
In the future, the average temperature in Finland will rise more (Ruosteenoja et al., 2016a) and faster than the global average (Ruosteenoja et al., 2016b; Parry et al., 2007). Also the precipitation is estimated to increase, but in winter more of it will be rain than snow (Jylhä et al., 2012). On average, the changes will be larger in winter than in summer (Ruosteenoja, 2013; Ruosteenoja et al., 2016b). Warming will be fastest in northern Finland (Ruosteenoja, 2013) and very low temperatures seem to appear more rarely. However, sub-freezing temperatures will persist in the northern parts of the country. It is anticipated that the snow cover period will become shorter especially in southern Finland, while in northern Finland the snow depth may actually increase due to increased snowfall. Generally, the observations indicate a larger decreasing trend in snow extent during spring than the global climate models (Derksen and Brown, 2012; Thackeray et al., 2016). The variation between diverse model results is large and can be partly attributed to missing or oversimplified process descriptions.
Arctic warming has caused the Arctic sea ice extent to decline rapidly (Vihma, 2014). There is evidence that such a decline, together with changed heat and moisture budgets and increased snow cover in Eurasia, is connected to the change towards atmospheric circulation patterns resembling the negative phase of the North Atlantic Oscillation and Arctic Oscillation, favouring cold winters in Europe and northeastern Eurasia. According to observations, cold, snow-rich winters have become more common again in large parts of Europe since 2005 (e.g. Cohen et al., 2010, 2012; Petoukhov and Semenov, 2010; Osborn, 2011). Global climate models have challenges in representing the recent changes in the Arctic. The models may underestimate the observed negative Arctic Oscillation due to sea ice and snow cover changes (Handorf, 2015). The strengthening and westward shift of the Siberian high-pressure system is too weak in the models as compared to reanalysis data (Dee et al., 2011). The models have problems in the gravity wave response and upward vertical propagation (Vihma, 2014). They have not reproduced the observed winter cooling over large parts of Eurasia, and the sea ice and land surface components (or ecosystem models) of the climate models have difficulties in correct representation of the inter-annual variability of the Arctic sea ice cover and terrestrial snow cover. Therefore, observations of snow and its annual cycle are needed to discover the recent trends. Furthermore, when climate model output is used to drive a land ecosystem model to predict the evolution of snow cover, it is necessary to adjust climatic variables with observational data e.g. by means of bias-correction methodologies.
The EU Life+ MONIMET (http://monimet.fmi.fi, last access: 14 January 2019) project aimed at increasing the turnover of climate and other data sources and creating indicators of seasonal fluctuations that can be used to assess the vulnerability of boreal forest and peatland ecosystems to climate change. The approach was based on a combination of climate data, models, field observations, phenological camera network, eddy-covariance measurements and remote sensing, which together can provide a comprehensive view on the changes in the phenology of vegetation, snow cover, and ecosystem carbon and heat cycle (Böttcher et al., 2014, 2016; Linkosalmi et al., 2016; Arslan et al., 2017; Peltoniemi et al., 2018a; Tanis et al., 2018). Satellite data sets processed in the MONIMET project were specifically used to derive large-scale indicators for the growing season and the snowmelt onset and melt-off date.
In this work we study whether there were changes of significant scale in snowmelt period and winter albedo during recent decades, 1982–2016, and compare the trends to changes in forest biomass. The surface albedo data record, CLARA-A2 SAL (Anttila et al., 2016a, b; Karlsson et al., 2017), was used to study trends in the snowmelt start and end dates, the melting season length and the albedo value preceding the melt onset. We also use the snow-melt-off date derived from the EU FP7 CryoLand project Pan-European Fractional Snow Cover (FSC) time series available for years 2001–2016 (Metsämäki et al., 2018). The snowmelt end dates operationally produced at the Finnish Meteorological Institute (FMI) from weather station observations are used as reference data. In addition, we use the land ecosystem model JSBACH (Raddatz et al., 2007; Reick et al., 2013) to derive snowmelt onset and end dates for the time range 1981–2011. The hourly climatic forcing for the land ecosystem model was produced with a regional climate model REMO (Jacob and Podzun, 1997; Jacob et al., 2001) that was constrained with ERA-Interim weather (Dee et al., 2011) and further bias corrected (Räisänen and Räty, 2013; Räty et al., 2014) with FMI gridded temperature and precipitation data (Aalto et al., 2013) to minimize the climate model inherent biases (Böttcher et al., 2016). The changes in the albedo values preceding the melting season are compared with trends in the stem volume in order to evaluate whether the changes in the forest explain the changes in albedo. The stem volume values per forest centre area were obtained from the National Forest Inventory data sets. An albedo model (Manninen and Stenberg, 2009) is used to link stem volumes, LAI and albedo.
2.1 Data sets
2.1.1 Vegetation zones
The digital vegetation zone map of Finland was provided by the Finnish Environment Institute (SYKE). The area was reduced to 13 main regions (Fig. 1, Table 1) by removing small islands from the map. Since the surface albedo product used in this study has a spatial resolution of only 0.25∘, the removed smaller islands would cause mixed pixel problems. Even now the narrow coastal areas (Åland, Ostrobothnian coast) are prone to extra scatter due to mixed pixels. Hence, they were removed from the final analysis. The vegetation zones stretch from hemiboreal to northern boreal.
2.1.2 Forest data
The National Forest Inventory of Finland surveys forests based on a uniform and dense sampling grid and provides unbiased estimates of forest volume and other forest variables. The results of the inventories have been estimated for 15 forest centres (Fig. 1) in inventory reports (Korhonen et al., 2000a, b, 2001, 2013, 2017; Salminen, 1993; Salminen and Salminen, 1998; Tomppo et al., 1998, 2001, 2003, 2004, 2005). In this study, we compiled time series of forest volume and area estimates of forests based on published inventory reports.
The sampling system of the National Forest Inventory has changed from regional to continuous sampling in the past decades. In the regional sampling, the country was divided up into 15 forest centres. One or more regions (forest centres) were inventoried each year, while all regions were inventoried during the full inventory period (5–7 years). After finishing one inventory period, a new inventory period started and regions were inventoried again. More recently, in and since inventory no. 10 the whole area of Finland has been inventoried within 1 year, but with lesser density. The full sampling density was reached within the inventory period, and forest resource estimates were again published. We assigned the forest volume estimates of newer inventories (10 and 11) to the mean years of the inventory periods. The northernmost forest centre, Lapland, further provides the estimates separately for the northern and southern parts in the most recent forest inventory.
The types and fraction of the forest area in each forest centre are shown in Table 2, as well as the average solar-zenith-angle value at melt onset time. The narrow coastal areas of Åland and the Southern and Ostrobothnian coasts were removed from the analysis due to mixed pixel problems of the satellite-based albedo product.
2.1.3 Snow cover melt-off data
The snow cover melt-off date used operationally at the Finnish Meteorological Institute is defined to be the first day after the longest period of complete snow cover in a winter when open areas have been continuously at least half covered by snow from the day of the beginning of permanent snow cover (Solantie et al., 1996; Kersalo and Pirinen, 2009). Complete disappearance of snow cover in open areas is reached typically 10 days later (Solantie et al., 1996). The snow-melt-off dates were calculated using the in situ snow depth measurements and the results were interpolated in a 10 km × 10 km grid.
2.1.4 Surface albedo data
The data record used in this study (CLARA-A2 SAL) covers the years 1982–2015 and is based on homogenized AVHRR data. It has been developed in the Satellite Application Facility project on Climate Monitoring, CM SAF, which is financially supported by the European Organization for the Exploitation of Meteorological Satellites (EUMETSAT). The data record is described in more detail in Anttila et al. (2016a, b, 2018) and Karlsson et al. (2016, 2017). The retrieved albedo is defined to the wavelength range 0.25–2.5 µm and the observations are averaged to a 0.25∘ grid, which is also the resolution of the final product. The albedo values are given in the range 0 %–100 %. The annual mean surface albedo values preceding the onset of snowmelt were determined for the 13 regions matching the vegetation zones of Finland and for the 16 forest centre areas of Finland. Also the mean starting and end dates of the snowmelt and the length of the melting season were calculated for those regions (Sect. 2.2.1).
2.1.5 Fractional snow cover data
At-pixel fractional snow cover (% of ground area covered by snow) is extracted from the pre-Copernicus CryoLand snow mapping service (Nagler et al., 2015), which provides Pan-European FSC maps starting from 2001 at 500 m (0.05∘) spatial resolution. The method applied in the FSC retrieval is SCAmod (Metsämäki et al., 2005, 2012), complemented by some additional NDSI (normalized difference snow index) rules for detecting the snow-free areas (Metsämäki et al., 2018). SCAmod detects FSC not only for non-forested areas, but is also able to capture the under-canopy snow. This type of snow product featuring snow on ground, not just viewable snow, shows high FSC if the forest floor (and under-subcanopy low vegetation) is snow covered, even though from the satellite sensor's point of view the forest looks darker in terms of albedo. The gained accuracy (using in situ FSC observations at Finnish snow transects as reference) is 15 %–20 %.
2.1.6 MODIS data
Daily composites of Moderate Resolution Imaging Spectroradiometer (MODIS) data were used for the determination of the start of growth of deciduous canopy (Sect. 2.2.4) during the period 2001–2016. The Terra MODIS level-1B data were retrieved from the National Aeronautics and Space Administration (NASA)'s Level 1 product and Atmosphere Archive and Distribution System (LAADS) and, from 2010 onwards, from the receiving station of the Finnish Meteorological Institute at Sodankylä. The data were processed to top-of-atmosphere (TOA) reflectances and projected into a geographic latitude and longitude grid. The normalized difference water index (NDWI) was calculated from near-infrared (841–876 nm) and mid-infrared (1628–1652 nm) TOA reflectances (Gao, 1996). Further details of the preprocessing are given in Böttcher et al. (2016).
2.2 Melting season start and end determination
2.2.1 Start and end date of snowmelt season from surface albedo data
Previously, the end of the melting season of seasonal snow has been successfully estimated using the standard deviation of the weekly means of albedo data (Rinne et al., 2009). Now, the CLARA-A2 SAL pentad mean albedo values were studied by fitting sigmoids matching the melting season, using nonlinear regression similar to the method by Böttcher et al. (2014). For each pixel and year, the pentads from the end of January until the end of August were used. The date of snowmelt onset was taken to be the date at which the sigmoid reached 99 % of its variation range. Similarly, the end of the snowmelt season was defined to be the day in which the sigmoid reached 1 % of its variation range. The length of the melting season was then the difference between these two dates. The albedo value corresponding to the onset of melting was used as the representative albedo value preceding the melting season (Anttila et al., 2018).
At first, a rough sigmoid fitting was carried out iteratively, with the maximum number of iterations being five. The reason for iteration is that the nonlinear fit outcome depends on the choice of initial parameter values of the nonlinear function to be fitted. After that, the albedo values lower than the mid-sigmoid values were removed from the period preceding the melting season as temporary melt events. Similarly, possible albedo values higher than mid-sigmoid values were removed from the period succeeding the melting season as temporary snowfalls or cloud masking errors of the albedo product. Then the sigmoid was fitted anew, using again a maximum of five iterations. In some areas, right after the end of the snowmelt, new growth of vegetation started to increase the albedo level so soon that it affected the sigmoid fitting. Therefore, the minimum albedo value starting from the mid-sigmoid was sought. A second order polynomial was fitted to the part of the albedo curve starting from that point. This regression polynomial was used to remove the growing season increase in the albedo but leave the pointwise variation of that part of the original curve (i.e. the blue curve of original data is replaced with the black curve in further nonlinear fitting in Fig. 2). The updated albedo curve was then used as the basis of a new sigmoid fit with the maximum number of iterations being five. If the value of the coefficient of determination R2 was smaller for the second sigmoid fit than for the first fit and R2 was smaller than 0.99 for the first fit, the latter sigmoid fit was carried out anew with the maximum number of iterations being now increased to 15. Finally, the sigmoid of the two rounds that had on average the smallest fit residuals was chosen.
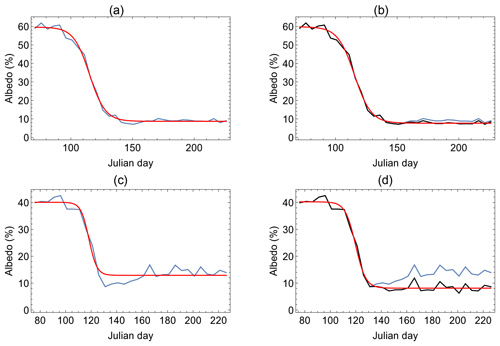
Figure 2Examples of sigmoid fitting. The original data points are shown in blue; the fit in red; and the data points, from which the vegetation greening effect has been removed, in black. (a) Initial fit of the easy case, (b) final fit of the easy case, (c) initial fit of a case with strong influence of vegetation greening on albedo and (d) final fit of the case with strong influence of vegetation greening on albedo.
In some cases, the snowmelt onset could not be determined, because it had started already before the first cloud-free albedo pentad was available for the pixel and year in question. For the regional mean values of the melt onset and end dates and albedo at the melt onset date, only the pixels for which the melt onset date was available were included in the analysis.
2.2.2 Start and end date of snowmelt season from ecosystem models
The JSBACH land surface model (Raddatz et al., 2007; Reick et al., 2013) resolves land surface physical and biogeochemical processes involved in surface energy balance as well as water and carbon balances within soil and vegetation. In addition to its principal use to serve as a land surface boundary for the Max Planck Institute for Meteorology Earth System Model (MPI–ESM) (Stevens et al., 2013), JSBACH can be driven with prescribed weather data. In this work, JSBACH was driven using the bias-corrected hourly REMO data for the years 1980–2011 with a spin-up period of 30 years before the forward run to equilibrate soil water and soil temperature. The spatial domain of JSBACH is also Fennoscandia at a resolution of 0.167∘, but in the present study only Finnish territory is investigated.
The JSBACH set-up used requires seven mutually consistent meteorological drivers: 2 m air temperature (Tair) and specific humidity, 10 m wind velocity, short-wave and long-wave radiation, potential short-wave radiation and precipitation (Pr) prescribed in hourly time resolution. For providing these drivers we used the regional climate model REMO (Jacob and Podzun, 2007; Jacob et al., 2001) with an implementation of surface properties by Gao et al. (2015). The modelling domain for REMO was Fennoscandia, with a grid resolution of 0.167∘, corresponding approximately to 18 km. Lateral boundary data were taken from ERA-Interim, a global atmospheric reanalysis produced by the European Centre for Medium-Range Weather Forecasts (ECMWF) (Dee et al., 2011). The forward run covered the period from 1979 to 2011 and it was preceded by a 10-year spin-up to equilibrate soil moisture and temperature.
Model-specific biases are inherent for regional climate models (Christensen et al., 2008; Teutschbein and Siebert, 2012) and it is known that REMO exhibits too-cold winter temperatures in an Eastern European area that covers most of Finland (Pietikäinen et al., 2012; Gao et al., 2015). The modelled summer and autumn temperatures tend to exceed observations. Moreover, REMO overestimates precipitation in northern Europe throughout the year. To account for the biases, we adjusted air temperature and precipitation against gridded homogenized weather data for 1980–2011 provided by the Finnish Meteorological Institute (Aalto et al., 2013) using a quantile–quantile type bias-correction algorithm for daily mean temperature (Räisänen and Räty, 2013), while daily cumulative precipitation was adjusted using parametric quantile mapping (Räty et al., 2014). Finally, the daily corrections were applied to the hourly modelled air temperature and precipitation values.
In JSBACH surface grid cells are divided into fractions of four most prevalent plant functional types (PFT) that are characterized with properties such as maximum leaf area, phenology type, growth rate, shedding rate and photosynthesis parameters. In addition to PFT fractions, each grid cell is characterized with a maximum fraction of the land area that can support plant growth.
Snowfall fraction of the total precipitation is given as follows:
Pr_sn is further distributed to surface and canopy reservoirs with the constant fraction of 0.25 to be intercepted by the canopy. In addition to the snowfall fraction the fate of the canopy reservoir is constrained by sublimation and melting that is temperature regulated and due to wind-blow (Roesch et al., 2001). Furthermore, the accumulation of snow is limited by LAI. At the ground the snow budget composes of the excess snowfall fraction after canopy interception, sublimation and melting.
The snow depth was used as an indicator of the melting season start and end. During early spring the changes in model albedo are very strongly dominated by changes in snow cover, since the changes in the leaf area of the coniferous forest are minor and the bud burst of broadleaved forest occurs later in spring. The modelled melting season timing and duration were studied by fitting a sigmoid to each grid cell of the yearly springtime snow depth data. The fit was done in two phases: the first sigmoid was fitted between days of year 30 and 200 to include days of yearly maximum and minimum snow cover. As modelled snow cover did not typically show a plateau before the start of the melt period, but tended to increase monotonously until the start of the melting, values lower than the first fit were rejected from the part of the time series prior to the point where the sigmoid reaches its half of the range value. If fewer than 15 data points were left after the rejection, the grid cell in that year was rejected. Otherwise the sigmoid was fitted to the remaining data series. After the second fit a sanity check was made to reject the fittings implying either a too-small or too-large difference between the sigmoid parameter values representing the snow cover levels before and after the melting. Because the snowmelt starts rather gradually and ends abruptly in the modelled snow depth data, asymmetric criteria of 98 % and 8 % of the sigmoid variation range were used for the start and end dates of the melting period, respectively. Finally, for each vegetation zone yearly regional means of start and end dates were calculated.
2.2.3 Snow-melt-off date from fractional snow cover data
The detection of the snow-melt-off date from the FSC map time series provides for each pixel the first day with snow-free terrain, but ignores short (a few days) intervening snow-free periods within a long snow season (Metsämäki et al., 2018). The melt-off date from the FSC time series is identified as the beginning of a snow-free period (FSC = 0 %) after a period of snow observations (FSC > 0 %). A pixel is assigned the status “melted” after a period of at least six subsequent snow-free days is found (however with possible intervening cloudy observations) if the number of the snow-free days represents 80 % of all cloud-free observations after the first day of the snow-free period of interest. The first day of this period is accepted as a candidate for the melt-off date. After the candidate day, a new snow period is allowed if at least three subsequent snow-covered days are observed. Then a new search for the melt-off date is launched the same way as before.
2.2.4 Start of the growing season of deciduous canopy from MODIS data
The start of the growing season of deciduous vegetation in Finland, usually referred to as greening up, was determined from NDWI using the method described in Böttcher et al. (2016). The NDWI was utilized because the detection of the greening up in boreal areas from the normalized difference vegetation index (NDVI) can be affected by melting of snow, whereas the NDWI allows a differentiation between snowmelt and greening up (Delbart et al., 2006). The start of the growing season showed good correspondence with the date of birch bud break (RMSE of 1 week) at Finnish phenological sites when the corresponding MODIS pixels are dominated by deciduous forest. The data set for the period 2001–2016 has a spatial resolution of .
2.2.5 Albedo model calculations
The effect of snow cover on surface albedo of coniferous forests can be estimated using an albedo model based on the photon recollision probability (Manninen and Stenberg, 2009). The forest parameters required are LAI, the clumping factor and the single-scattering albedo of needles. In addition, the sun-zenith-angle value is needed. The average value of the sun zenith angle of the forest centres at the start day of snowmelt was used in the calculations (Table 2). The snow cover of the forest floor is described using its albedo. The forest inventory does not contain LAI information and a strong relationship between the stem volume and LAI cannot be expected at a high spatial resolution. Yet, in larger areas one could assume that the LAI of a forest is statistically related to the stem volume per area V according to LAI . When comparing the albedo simulations to the satellite measurements in a larger area, one has to take into account also the fraction of the forested area. The albedo of the forest centre areas was simulated for the years matching the National Forest Inventory during 1963–2011 varying the coefficient k in the range 0.1–1.5. The optimal value of k was defined to be the value corresponding to smallest difference between the simulated and satellite-based albedo values. A relatively strong relationship between the optimal value of k and the fraction of the forest area in the boreal forest zone was found (Fig. 3). The area left outside the regression (Northern Lapland) is dominated by deciduous species (mountain birch); hence its LAI value in winter is not related to the stem volume similarly as for the coniferous species.
3.1 Seasonal snowmelt timing
3.1.1 Snowmelt onset
The onset of melting was determined using the sigmoids determined both from the satellite-based albedo data, CLARA-A2 SAL pentads, and the daily JSBACH model calculations of the snow depth. The comparison of these melting season start dates is shown in Fig. 4. The overall agreement is good, and some of the scatter can be attributed to using a different variable for the data (albedo) and the model (snow depth). For the model calculations, the snow depth was used as the indicator of the snowmelt onset rather than the albedo, because the snow depth is more related to the snow accumulation throughout the seasonal cold period, whereas the albedo is sensitive to the prevailing weather conditions just before the melt onset. The snow depth describes the whole snowpack, whereas the albedo describes only the topmost layer of it. The surface albedo product showed a negative trend (about half a day per year) in two areas: Northern Karelia–Kainuu and Southwestern Lapland (Table 3, Fig. 5). For the other areas, the coefficient of determination for a linear trend of 5-year average values was smaller than 0.5. The annual variation of the melt onset date is so large (the standard deviation being on average 12 days for the time range 1982–2015) that it easily masks a long-term trend. This was even more evident in the land ecosystem model calculation results, for which a low frequency variation dominated the time window so that no region showed a marked trend even in the 5-year moving average curve. In particular, variable melt onset timing is in the coastal regions (Southwestern Finland and Southern Ostrobothnia) and in the Lake district. For those regions, the standard deviation values of the melt onset date are 14.3, 14.7 and 14.6, respectively. For the Northern Fjeld Lapland, the vicinity of the Barents Sea also causes a higher standard deviation (13.4) of the melt onset date. A large part of Finland is coastal, and consequently the sea ice extent of the Baltic Sea has a strong effect on the weather and climate, not only changes in air temperature or precipitation preceding the melt onset, which are the dominating drivers in some regions of the Northern Hemisphere (Anttila et al., 2018). When using 10-year moving averages for the trend analysis based on the albedo data, a negative trend (R2 > 0.5) of the melt onset date was detected in Northern Karelia–Kainuu, Southwestern Lapland, Ostrobothnia, Kuusamo district and Lake district. Although the time series of 34 years is not really long enough for using 10-year averages, the results, however, confirm the intuitive impression that the snowmelt starts earlier than it used to do in the past, as the two distinct areal trends showed.
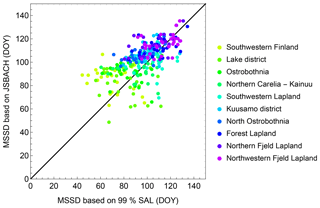
Figure 4The melt onset day of the snow cover in Finland during 1982–2011 based on the CLARA-A2 SAL product and JSBACH model snow depth.
Table 3The observed trends of the melt onset and melting season length during 1982–2015 according to the CLARA-A2 SAL data of the regions based on the vegetation zones (Table 1). Values for the slope and the coefficient of determination are shown both for annual data and for moving averages of 5 years. The cases for which the moving average of 5 years shows a significant coefficient of determination are indicated with bold fonts.
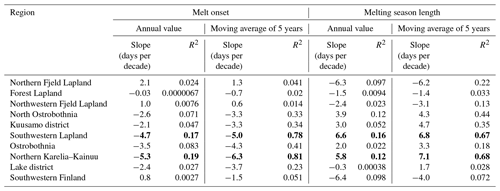
3.1.2 End of snowmelt
The end of snowmelt was determined using the albedo data, the snow depth value of the land ecosystem model and the snow-melt-off date derived from the satellite-based fractional snow cover estimate for each area of the vegetation zone map. The results were compared with the FMI snowmelt maps provided operationally by the climate service of FMI (Solantie et al., 1996; Fig. 6). The overall agreement is good for the melt-off date derived from MODIS-based FSC and the FMI operational melt-off date derived using in situ data taking into account that the satellite-based melt-off date refers to a completely snow-free case, whereas the in situ-based melt-off date refers to the end of the permanent snow cover when half of open areas still have snow (Fig. 6). The 1 % threshold for the albedo produced a clearly later end of the melt date than the in situ-based snow-melt-off date and model results. This is understandable, because the FMI operational melt-off date does not correspond to a completely snow-free case and the albedo value is very sensitive to even small amounts of snow. The day in which the albedo-based sigmoid reached 20 % of its variation range matched the official end date of snowmelt well and the 15 % value matched the FSC-based melt-off date well. The difference between dates corresponding to the 20 % and 1 % threshold values of the albedo-based sigmoids is of the order of 13 days, which is in line with the 10-day difference, during which half of snow-free open areas become completely snow-free (Solantie et al., 1996). Results from JSBACH coincide well with the operationally observed end of permanent snow cover. Probably the snow-off bias inherited from the climate model surface parametrizations explain why the zero value of snow depth of JSBACH matches the time when half of open areas are snow-free. It has been shown that, for example in ECHAM5, the snowmelt occurs at too-low temperatures (Räisänen et al., 2014). Consequently, too much of the surface net radiation is consumed in melting snow and too little in heating the air.
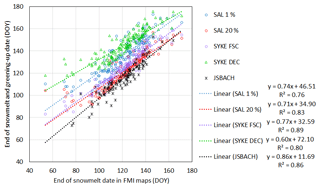
Figure 6Comparison of diverse estimates of the melt-off day of snow cover. The FMI snow maps of end of permanent snow cover are based on in situ snow depth measurements. The satellite-based melt-off date estimates are derived using the surface albedo product CLARA-A2 SAL (Anttila et al., 2016a; Karlsson et al., 2017) and the FSC snow product calculated from MODIS images (Metsämäki et al., 2018). The JSBACH model estimate of end of snowmelt is also shown. For comparison the greening-up date of deciduous vegetation (SYKE DEC; Böttcher et al., 2016) is also presented.
No systematic trend was observed for the end of snowmelt date for moving 5-year averages of the albedo or the land ecosystem model data. It seems that the onset of snowmelt depends more clearly on the climate and the end of snowmelt is more sensitive to the prevailing weather conditions at that time.
The areal advance of spring is demonstrated by the FMI operational melt-off map, the surface albedo 20 % threshold map, the FSC melt-off map and the greening-up map in years 2006 and 2012 (Fig. 7). The general agreement is good, and the overall zones of equal timing are obvious.
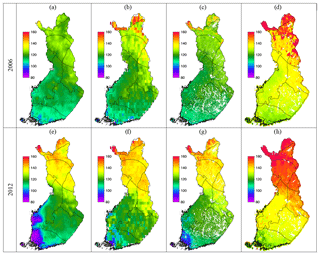
Figure 7The progress of spring in 2006 and 2012 starting from (a, e) the melt-off day of the permanent snow cover in Finland based on in situ snow depth measurements (Solantie et al., 1996; Kersalo and Pirinen, 2009), (b, f) the surface albedo reaching the 20 % threshold value of its dynamic range during the melting season (Anttila et al., 2016a; Karlsson et al., 2017), (c, g) the complete disappearance of the snow cover based on the FSC product (Metsämäki et al., 2018) and (d, h) the greening-up day based on MODIS NDWI (Böttcher et al., 2016).
3.1.3 Length of the melting season of snow
The length of the melting season was determined as the difference between the dates corresponding to the end of the snowmelt and the onset of the snowmelt. The 99 % and 1 % threshold values were used for the albedo sigmoids. A clear trend of increasing melting period length was observed for moving 5-year averages of the areas Northern Karelia–Kainuu and Southwestern Lapland, where the snowmelt starts earlier than before (Table 3, Fig. 8). For the length of the melting season, the standard deviation during 1982–2015 was on average, naturally, even larger than for the melt onset date, with the value being as high as 16 days. The largest values occurred again in Southwestern Finland (20.3), Southern Ostrobothnia (17.5), Lake district (17.1) and Northern Fjeld Lapland (20.0). When using 10-year moving averages in the trend analysis, the result was that the melting season length increased in Northern Karelia–Kainuu, Kuusamo district, Southwestern Lapland and North Ostrobothnia and decreased in Northern Fjeld Lapland.
3.2 Start of the growing season
The start of the growing season of deciduous vegetation based on MODIS NDWI values turned out to almost coincide with the timing of the 1 % threshold value of the surface albedo, being only slightly later (Fig. 6). This is probably due to the large fraction of deciduous species being in open areas and on the forest floor, where the growing starts only after the soil has become completely snow-free and where even small amounts of remaining snow have a large effect on the albedo. The difference between the date when the SAL value reached 1 % of its dynamic range during the melting season and the date of the greening up varied in the vegetation zones on average between 5 and 13 days during the 16 years of comparison. The largest difference took place on the coast and the shortest in mountain areas, where greening of mountain birch can occur even before the snowmelt (Shutova et al., 2006; Peltoniemi et al., 2018b). In addition, trees in the northern boreal region have a lower temperature requirement for bud burst than in the south, and the day length is already longer in the north at the time of bud burst. Therefore, the intensity of the response to temperature increases from south to north and the observed trend towards earlier bud burst were stronger in the northern boreal zone compared to the southern boreal zone for the period 1997–2006 (Pudas et al., 2008).
Table 4The observed albedo trends of regions based on the vegetation zones. Values for the slope and the coefficient of determination are shown both for annual data and moving averages of 5 years. The cases for which the moving average of 5 years shows a significant coefficient of determination are indicated with bold fonts.
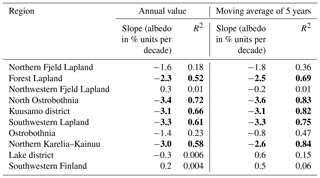
3.3 Albedo before onset of snowmelt
The surface albedo at the melt onset shows a decreasing trend in several northern regions based on the vegetation zones (Table 4, Fig. 9). This can be explained by the increase in the stem volume, as the albedo model results agree with the observed albedo trends of the forest-centre-based regions (Table 5, Fig. 10). Data showed meaningful trends (R2 > 0.5) for 5-year average albedo values only, when the fraction of the forested area exceeded 0.7 and the stem volume was smaller than 75 m3 ha−1. For those areas (Southern Lapland, Kainuu, Northern Ostrobothnia, Lapland and North Karelia), the albedo trend is negative. It is well known that the wintertime albedo depends strongly on the LAI (and thus stem volume of a larger area) when the LAI is relatively small (Manninen and Stenberg, 2009). For larger LAI values, the albedo more or less saturates, and a further increase in LAI does not show up markedly. As expected, no clear albedo trend after the snowmelt was observed. The reason is that the difference between the canopy and forest floor vegetation albedo is small, whereas the snow and canopy albedo values differ markedly. Hence, the accumulating stem volume in the northern part of Finland causes darkening of the winter- and springtime albedo, but does not have a significant effect on the albedo value right after the snowmelt.
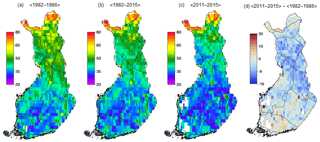
Figure 9The surface albedo (%) at the time of the melt onset in Finland. The average value during 1982–1986 (a), during 1982–2015 (b) and during 2011–2015 (c). The difference between the last and first 5 years' average value is shown as well (d). The white pixels on land represent cases when the melting has already started before the albedo could be retrieved, i.e. when the sun zenith angle was larger than 70∘.
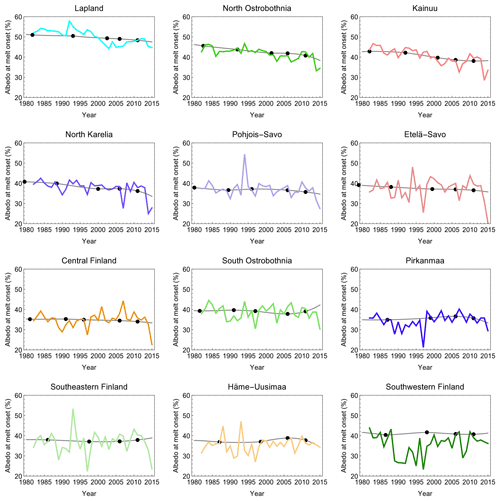
Figure 10Comparison of observed albedo variation (colour solid curves) of the forest centre areas (Fig. 1, Table 2) during 1982–2015 and corresponding albedo model results based on stem volume measurements (points). The interpolation curves (grey solid curves) of the modelled annual values are shown as well. The colours match those of the map in Fig. 1b, except for Lapland, which is treated as one area (15 and 16).
Table 5The observed albedo trends of the regions based on the forest centres. Values for the slope and the coefficient of determination are shown both for annual data and moving averages of 5 years. The region Lapland is divided into northern and southern parts as shown in Fig. 1. The cases for which the moving average of 5 years shows a significant coefficient of determination are indicated with bold fonts.
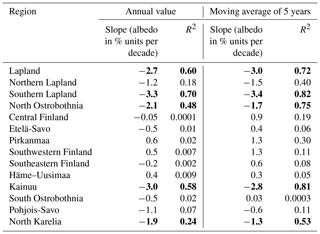
Surface albedo is determined by the quantity and type of land vegetation, the seasonal cycle of leaf development and the existence of snow cover. The snow conditions also depend on the vegetation cover. Seasonal dynamics of snow and vegetation are driven by temperature and precipitation. The air temperatures in the Arctic and boreal regions have increased and the Arctic sea ice extent has reduced, and their influence can be seen in the Finnish temperature and precipitation records. The trends are not self-evident, although as a whole the Arctic and boreal region is changing towards a warmer climate. The large variation in the climate can be seen in both observed and modelled snowmelt onset. The observed trends are clearly negative only in Northern Karelia–Kainuu and Southwestern Lapland. The year-to-year variation in the modelled snowmelt onset is not as large as in the observed snowmelt onset data, but the multi-year variation is similar, with the model acting as a smoother of observations. In many cases, both positive and negative tendencies appear during the study period, most significantly around year 2000, when the snowmelt showed a transition from earlier onset to later onset in Southwestern Finland, Lake district and Ostrobothnia. Also in a previous analysis using a 35-year data set from the passive microwave radiometer snow clearance data set, Metsämäki et al. (2018) concluded that the snow melt-off at northern latitudes in Europe has advanced 3–5 days per decade in boreal forests and tundra. The MODIS FSC-based snow-melt-off maps of this study gave a similar signal.
The trends of the end of the snowmelt are insignificant everywhere. However, combined with the negative snowmelt onset trend in Northern Karelia–Kainuu and Southwestern Lapland, we obtain an increase in the length of the snowmelt period in those regions. Generally, in northern latitudes, many studies have shown persistent negative trends in snowmelt onset and positive trends in early spring vegetation activity (Myneni et al., 1997; Pulliainen et al., 2017). A recent study indicated an increase in the length of the snowmelt period (Contosta, 2017), which could have both negative and positive impacts for soil water availability, nitrogen cycling, and ecosystem carbon and energy balance and thus induce complex perturbation of land-surface–climate feedbacks.
Snow has a very complex role in the forest ecosystems, and many of the snow-related effects could be revealed and many analyses could be deepened by including albedo and snow cover in the analyses. The snow cover directly affects the greatest soil frost depth in winter. Hence, changes in snow cover are expected to affect also the amount of wind throw. In particular, late autumn, winter and early spring storms will be more dangerous for the canopy if the soil is not frozen as it used to be in the past (Peltola et al., 1999). The trafficability of the forested terrain may reduce with earlier snowmelt (Kellomäki et al., 2010). In addition, frost affects the roots of the trees via the soil frost, rendering it as a potential factor influencing growth of the trees (Sutinen et al., 2014). Henttonen et al. (2017) studied forest growth changes in Finland in 1971–2010 and concluded that 37 % of the observed growth increase was associated with environmental factors, which are probably related to the climate. Further research is required about the effects of climate factors on growth and particularly about the effects of insulating snow cover and soil temperature.
The timber resources in Finnish forests have grown since the 1950s more than has been removed by logging (National Forest Inventory, 2013). In northern Finland the share of stout timber has traditionally been high, so the increase comes mainly from small-diameter timber. While carbon storage in forests has increased, Finland has darkened, which shows in the negative trend of albedo preceding the snowmelt season. The effect of canopy on snow-covered terrain albedo is largest for small LAI values (Manninen and Jääskeläinen, 2018); hence it is understandable that the marked decreasing trend of albedo before the snowmelt season is observed in northern Finland. In southern Finland, the share of stout timber has more than quadrupled since the 1950s, yet no trend in pre-melt albedo during 1982–2016 is observed there. Hence, the idea to increase carbon sinks in forests by increasing the timber resources of existing forests by causing trees to grow more densely and become stouter (Pihlainen et al., 2014; Baul et al., 2017a, b; Pingoud et al., 2018) suggests a possibility to combat climate change in regions of already medium-size canopy without the negative albedo feedback.
The surface albedo proved to be a suitable indicator of the snowmelt onset and end of the melting season. Overall agreement was found with corresponding dates determined using the JSBACH land ecosystem model snow depth data. The operationally provided end of the permanent snow cover date based on in situ data corresponded to about the day when the surface albedo value reached the lower 20 % of its dynamic range during the melting season. The end of the snowmelt determined using the satellite-based fractional snow cover data and the JSBACH land ecosystem model snow depth data also agreed well with the corresponding operationally produced date. The day when the surface albedo has decreased to the lower 1 % of its dynamic range is well in line with the day corresponding to completely snow-free open areas. In addition, the greening-up date followed on average 5–13 days later.
According to the albedo data, the melt onset date has advanced by about 17 and 21 days, i.e. 5 and 6 days per decade, respectively, in two regions in northern Finland (Southwestern Lapland and Northern Karelia–Kainuu). Similarly, in those areas the length of the melting season has increased by 23–24 days, i.e. 7 days per decade. The albedo data also show darkening albedo values (2 %–3 % per decade in absolute albedo percentage) preceding the snowmelt onset in North Ostrobothnia, Northern Karelia, Kainuu and Lapland, especially in Southern Lapland, which is coniferous-species dominated. This albedo decrease could be explained by the observed increase in stem volume in those areas.
The surface albedo data (https://doi.org/10.5676/EUM_SAF_CM/CLARA_AVHRR/V002) are available at the web user interface of the CM SAF project: https://wui.cmsaf.eu/safira/action/viewProduktSearch;jsessionid=5137E8E5FEF904478BBC386333FD8EB0.ku_2 (last access: 14 January 2019). The data on the start of the growing season (greening up of deciduous vegetation) for the period 2001–2016 can be viewed online in a web map application (http://syke.maps.arcgis.com, last access: 14 January 2019) and are available for download at the open-data service of SYKE (http://www.syke.fi/en-US/Open_information/Spatial_datasets\#P, last access: 14 January 2019). The original FSC data are available from the CryoLand portal (http://neso1.cryoland.enveo.at/cryoclient/, last access: 14 January 2019). Forest inventory data are not publicly available, but aggregated values are (http://kartta.luke.fi/index-en.html, last access: 14 January 2019).
TeM performed the albedo data analysis, comparison with other data sets and albedo modelling. TiM and AL carried out the ecosystem model calculations and analysis of those results. TA planned and led the climate-related work and interpreted the simulation results. MP compiled the forest inventory data, KB provided the greening-up data, SM provided the FSC-based yearly melt-off date data, and KA provided the albedo data and PP provided the FMI snowmelt data. AN coordinated the MONIMET project and everybody participated in the scientific discussions. All authors participated in the writing of the paper.
The authors declare that they have no conflict of interest.
This work was carried out in the Life+ project MONIMET (grant agreement
LIFE12 ENV/FI000409), for which the authors wish to express their gratitude
to EU. The authors are grateful for EUMETSAT for financial support in generating
the CLARA-A2 SAL time series in the CM SAF project. The authors are
grateful to Achim Drebs for discussions related to snowmelt. The
authors wish to thank also Maria Holmberg and Katri Rankinen for their
co-operation at various phases of the project and for providing
the vegetation zone map and Antti Ihalainen for inventory reports. We
thank the Academy of Finland Center of Excellence (307331) and the Academy
of Finland projects OPTICA (295874) and CARB-ARC (285630) for support.
Edited by: Martin De Kauwe
Reviewed by: Richard L. H. Essery and one anonymous referee
Aalto, J., Pirinen, P., Heikkinen, J., and Venäläinen, A: Spatial interpolation of monthly climate data for Finland: comparing the performance of kriging and generalized additive models, Theor. Appl. Climatol., 112, 99–111, 2013.
Anttila, K., Jääskeläinen, E., Riihelä, A., Manninen, T., Andersson, K., and Hollman, R.: Algorithm Theoretical Basis Document: CM SAF Cloud, Albedo, Radiation data record ed. 2 – Surface Albedo, 85 pp., https://doi.org/10.5676/EUM_SAF_CM/CLARA_AVHRR/V002, 2016a.
Anttila, K., Jääskeläinen, E., Riihelä, A., Manninen, T., Andersson, K., and Hollman, R.: Validation Report: CM SAF Cloud, Albedo, Radiation data record ed. 2 – Surface Albedo, 54 pp., 2016b.
Anttila, K., Manninen, T., Jääskeläinen, E., Riihelä, A., and Lahtinen, P.: The Role of Climate and Land Use in the Changes in Surface Albedo Prior to Snow Melt and the Timing of Melt Season of Seasonal Snow in Northern Land Areas of 40 N–80 N during 1982–2015, Remote Sensing, 10, 1619, https://doi.org/10.3390/rs10101619, 2018.
Arslan, A., Tanis, C., Metsämäki, S., Aurela, M., Böttcher, K., Linkosalmi, M., and Peltoniemi, M.: Automated Webcam Monitoring of Fractional Snow Cover in Northern Boreal Conditions, Geosciences, 7, 55, https://doi.org/10.3390/geosciences7030055, 2017.
Baul, T. K., Alam, A., Ikonen, A., Strandman, H., Asikainen, A., Peltola, H., and Kilpeläinen, A.: Climate Change Mitigation Potential in Boreal Forests: Impacts of Management, Harvest Intensity and Use of Forest Biomass to Substitute Fossil Resources, Forests, 8, 455, https://doi.org/10.3390/f8110455, 2017a.
Baul, T. K. Alam, A., Strandman, H., and Kilpeläinen, A.: Net climate impacts and economic profitability of forest biomass production and utilization in fossil fuel and fossil-based material substitution under alternative forest management, Biomass Bioenerg., 98, 291–305, 2017b.
Betts, R. A.: Offset of the potential carbon sink from boreal forestation by decreases in surface albedo, Nature, 408, 187–190, 2000.
Bonan, G. B., Pollard, D., and Thompson, S. L.: Effects of boreal forest vegetation on global climate, Nature, 359, 716–718, 1992.
Böttcher, K., Aurela, M., Kervinen, M., Markkanen, T., Mattila, O.-P., Kolari, P., Metsämäki, S., Aalto, T., Arslan, A. N., and Pulliainen, J.: MODIS time-series-derived indicators for the beginning of the growing season in boreal coniferous forest – A comparison with CO2 flux measurements and phenological observations in Finland, Remote Sens. Environ., 140, 625–638, 2014.
Böttcher, K., Markkanen, T., Thum, T., Aalto, T., Aurela, M., Reick, C., Kolari, P., Arslan, A., and Pulliainen, J.: Evaluating biosphere model estimates of the start of the vegetation active season in boreal forests by satellite observations, Remote Sensing, 8, 580, 2016.
Christensen, J. H., Boberg, F., Christensen, O. B., and Lucas-Picher, P.: On the need for bias correction of regional climate change projections of temperature and precipitation, Geophys. Res. Lett., 35, L20709, https://doi.org/10.1029/2008GL035694, 2008.
Cohen, J., Foster J., Barlow, M., Saito, K., and Jones, J.: Winter 2009–2010: a case study of an extreme Arctic Oscillation event, Geophys. Res. Lett., 37, L17707, https://doi.org/10.1029/2010GL044256, 2010.
Cohen, J. L., Furtado, J. C., Barlow, M. A., Alexeev, V. A., and Cherry, J. E.: Arctic warming, increasing snow cover and widespread boreal winter cooling, Environ. Res. Lett., 7, 014007, https://doi.org/10.1088/1748-9326/7/1/014007, 2012.
Contosta, A. R., Adolph, A., Burchsted, D., Burakowski, E., Green, M., Guerra, D., Albert, M., Dibb, J., Martin, M., McDowell, W. H., Routhier, M., Wake, C., Whitaker, R., and Wollheim, W.: A longer vernal window: the role of winter coldness and snowpack in driving spring transitions and lags, Glob. Change Biol., 23, 1610–1625, https://doi.org/10.1111/gcb.13517, 2017.
Davin, E. L. and de Noblet-Ducoudré, N.: Climatic impact of global-scale deforestation: Radiative versus nonradiative processes, J. Climate, 23, 97–112, 2010.
Dee, D. P., Uppala, S. M., Simmons, A. J., Berrisford, P., Poli, P., Kobayashi, S., Andrae, U., Balmaseda, M. A., Balsamo, G., Bauer, P., Bechtold, P., Beljaars, A. C. M., van de Berg, L., Bidlot, J., Bormann, N., Delsol, C., Dragani, R., Fuentes, M., Geer, A. J., Haimberger, L., Healy, S. B., Hersbach, H., Hólm, E. V., Isaksen, L., Kållberg, P., Köhler, M., Matricardi, M., McNally, A. P., Monge-Sanz, B. M., Morcrette, J.-J., Park, B.-K., Peubey, C., de Rosnay, P., Tavolato, C., Thépaut, J.-N., and Vitart, F.: The ERA-Interim reanalysis: configuration and performance of the data assimilation system, Q. J. Roy. Meteor. Soc., 137, 553–597, 2011.
Delbart, N., Le Toan, T., Kergoat, L., and Fedotova, V.: Remote sensing of spring phenology in boreal regions: A free of snow-effect method using NOAA-AVHRR and SPOT-VGT data (1982–2004), Remote Sens. Environ., 101, 52–62, 2006.
Derksen, C. and Brown, R.: Spring snow cover extent reductions in the 2008 – 2012 period exceeding climate model projections, Geophys. Res. Lett., 39, L19504, https://doi.org/10.1029/2012GL053387, 2012.
Gao, B.-C.: NDWI-A normalized difference water index for remote sensing of vegetation liquid water from space, Remote Sens. Environ., 58, 257–266, 1996.
Gao, Y., Weiher, S., Markkanen, T., Pietikäinen, J.-P., Gregow, H., Henttonen, H. M., Jacob, D., and Laaksonen, A.: Implementation of the CORINE land use classification in the regional climate model REMO, Boreal Environ. Res., 20, 261–282, 2015.
Handorf, D., Jaiser, R., Dethloff, K., Rinke, A., and Cohen, J.: Impacts of Arctic sea ice and continental snow cover changes on atmospheric winter teleconnections, Geophys. Res. Lett., 42, 2367–2377, https://doi.org/10.1002/2015GL063203, 2015.
Henttonen, H. M., Nöjd, P., and Mäkinen, H.: Environment-induced growth changes in the Finnish forests during 1971–2010 – An analysis based on National Forest Inventory, Forest Ecol. Manag., 386, 22–36, https://doi.org/10.1016/j.foreco.2016.11.044, 2017.
Jacob, D. and Podzun, R.: Sensitivity studies with the regional climate model REMO, Meteorol. Atmos. Phys., 63, 119–129, 1997.
Jacob, D., Van den Hurk, B. J. J. M., Andrae, U., Elgered, G., Fortelius, C., Graham, L. P., Jackson, S. D., Karstens, U., KoÈpken, Chr., Lindau, R., Podzun, R., Rockel, B., Rubel, F., Sass, B. H., Smith, R. N. B., and Yang, X.: A comprehensive model inter-comparison study investigating the water budget during the BALTEX-PIDCAP period, Meteorol. Atmos. Phys., 77, 19–43, 2001.
Jylhä, K., Ruosteenoja, K., Räisänen, J., and Fronzek, S.: Ilmasto, in: Miten väistämättömään ilmastonmuutokseen voidaan varautua? – yhteenveto suomalaisesta sopeutumistutkimuksesta eri toimialoilla, edited by: Ruuhela, R., Maa- ja metsätalousministeriö, Helsinki, MMM:n julkaisuja 6/2011, 16–23, 2012 (in Finnish).
Karlsson, K.-G., Anttila, K., Trentmann, J., Stengel, M., Meirink, J. F., Devasthale, A., Hanschmann, T., Kothe, S., Jääskeläinen, E., Sedlar, J., Benas, N., van Zadelhoff, G.-J., Schlundt, C., Stein, D., Finkensieper, S., and Håkansson, N.: GCOS: The Global Observing System for Climate: Implementation needs, Reference Number GCOS-200, WMO, 315 pp., 2016.
Karlsson, K.-G., Anttila, K., Trentmann, J., Stengel, M., Fokke Meirink, J., Devasthale, A., Hanschmann, T., Kothe, S., Jääskeläinen, E., Sedlar, J., Benas, N., van Zadelhoff, G.-J., Schlundt, C., Stein, D., Finkensieper, S., Håkansson, N., and Hollmann, R.: CLARA-A2: the second edition of the CM SAF cloud and radiation data record from 34 years of global AVHRR data, Atmos. Chem. Phys., 17, 5809–5828, https://doi.org/10.5194/acp-17-5809-2017, 2017.
Kellomäki, S., Maajärvi, M., Strandman, H., Kilpeläinen, A., and Peltola, H.: Model computations on the climate change effects on snow cover, soil moisture and soil frost in the boreal conditions over Finland, Silva Fenn., 44, 213–233, 2010.
Kersalo, J. and Pirinen, P. (Eds.): Suomen maakuntien ilmasto, Finnish Meteorological Institute Reports, 2009, 192 pp., 2009 (in Finnish with English summary).
Korhonen, K. T., Tomppo, E., Henttonen, H., Ihalainen, A., and Tonteri, T.: Hämeen-Uudenmaan metsäkeskuksen alueen metsävarat 1965–99, Metsätieteen aikakauskirja 3B/2000, 489–566, 2000a (in Finnish).
Korhonen, K. T., Tomppo, E., Henttonen, H., Ihalainen, A., Tonteri, T., and Tuomainen, T.: Pirkanmaan metsäkeskuksen alueen metsävarat 1965–1999, Metsätieteen aikakauskirja 4B/2000, 661–739, 2000b (in Finnish).
Korhonen, K. T., Tomppo, E., Henttonen, H., Ihalainen, A., Tonteri, T., and Tuomainen, T.: Pohjois-Karjalan metsäkeskuksen alueen metsävarat 1966–2000, Metsätieteen aikakauskirja 3B/2001, 495–576, 2001 (in Finnish).
Korhonen, K. T., Ihalainen, A., Viiri, H., Heikkinen, J., Henttonen, H. M., Hotanen, J.-P., Mäkelä, H., Nevalainen, S., and Pitkänen, J.: Suomen metsät 2004–2008 ja niiden kehitys 1921–2008, Metsätieteen aikakauskirja 3, 269–608, 2013 (in Finnish).
Korhonen, K. T., Ihalainen, A., Ahola, A., Heikkinen, J., Henttonen, H. M., Hotanen, J.-P., Nevalainen, S., Pitkänen, J., Strandström, M., and Viiri, H.: (2017-09) Suomen metsät 2009–2013 ja niiden kehitys 1921–2013, Luonnonvara- ja biotalouden tutkimus 59/2017, 86 pp., 2017 (in Finnish).
Linkosalmi, M., Aurela, M., Tuovinen, J.-P., Peltoniemi, M., Tanis, C. M., Arslan, A. N., Kolari, P., Böttcher, K., Aalto, T., Rainne, J., Hatakka, J., and Laurila, T.: Digital photography for assessing the link between vegetation phenology and CO2 exchange in two contrasting northern ecosystems, Geosci. Instrum. Method. Data Syst., 5, 417–426, https://doi.org/10.5194/gi-5-417-2016, 2016.
Luyssaert S., Jammet, M., Stoy, P. C., Estel, S., Pngratz, J., Ceschia, E., Churkina, G., Don, A., Erb, K. H., Ferlicoq, M., Gielen, B., Grünwald, T., Houghton., R. A., Klumpp, K., Knohl, A., Kolb, T., Kuemmerle, T., Laurila, T., Lohila, A., Loustau, D., McGrath, M., Meyfroidt, P., Moors, E. J., Naudts, K., Novick, K., Otto, J., Pilegaard, K., Pio, C. A., Rambal, S., Rebmann, C., Ryder, J., Suyker, A. E., Varlagin, A., Wattenbach, M., and Dolman, J.: Land management and land cover change have impacts of similar magnitude on surface temperature, Nature Clim. Change, 4, 389–393, https://doi.org/10.1038/nclimate2196, 2014.
Manninen, T. and Jääskeläinen, E.: The effect of boreal forest canopy on snow covered terrain broadband albedo, submitted, Geophysica 53, 7–27, 2018.
Manninen, T. and Stenberg, P.: Simulation of the effect of snow covered forest floor on the total forest albedo, Agr. Forest Meteorol., 149, 303–319, 2009.
Metsämäki, S., Anttila, S., Huttunen, M., and Vepsäläinen, J.: A feasible method for fractional snow cover mapping in boreal zone based on a reflectance model, Remote Sens. Environ., 95, 77–95, 2005.
Metsämäki, S., Mattila, O.-P., Pulliainen, J., Niemi, K., Luojus, K., and Böttcher, K.: An optical reflectance model-based method for fractional snow cover mapping applicable to continental scale, Remote Sens. Environ., 123, 508–521, 2012.
Metsämäki, S., Böttcher, K., Pulliainen, J., Luojus, K., Cohen, J., Takala, M., Mattila, O.-P., Schwaizer, G., Derksen, C., and Koponen, S.: The accuracy of snow melt-off day derived from optical and microwave radiometer data – A study for Europe, Remote Sens. Environ., 211, 1–12, 2018.
Myneni, R. B., Keeling, C. D., Tucker, C. J., Asrar, G., and Nemani, R. R.: Increased plant growth in the northern high latitudes from 1981 to 1991, Nature, 386, 698–702, 1997.
National Forest Inventory, Finnish Forest Research Institute, 2013.
Nagler, T., Bippus, G., Schiller, C., Metsämäki, S., Mattila, O.-P., Luojus, K., Malnes, E., Solberg, R., Diamandi, A., Larsen, H. E., Wiesmann, A., and Gustafsson, D.: CryoLand – Copernicus Service Snow and Land Ice: Final Report, pp. 46, available at: http://enveo.at (last access: 14 January 2019), 2015.
Osborn, T. J.: Winter 2009/2010 temperatures and a record-breaking North Atlantic Oscillation index, Weather, 66, 19–21, 2011.
Parry, M. L., Canziani, O. F., Palutikof, J. P., van der Linden, P. J., and Hanson, C. E.: IPCC: Climate Change 2007: Impacts, Adaptation and Vulnerability, Contribution of Working Group II to the Fourth Assessment Report of the Intergovernmental Panel on Climate Change, Cambridge University Press, Cambridge, London, UK, 976 pp., 2007.
Peltola, H., Kellomäki, S., and Väisänen, H.: Climatic Change, 41, 17–36, https://doi.org/10.1023/A:1005399822319, 1999.
Peltoniemi, M., Aurela, M., Böttcher, K., Kolari, P., Loehr, J., Karhu, J., Linkosalmi, M., Tanis, C. M., Tuovinen, J.-P., and Arslan, A. N.: Webcam network and image database for studies of phenological changes of vegetation and snow cover in Finland, image time series from 2014 to 2016, Earth Syst. Sci. Data, 10, 173–184, https://doi.org/10.5194/essd-10-173-2018, 2018a.
Peltoniemi, M., Aurela, M., Böttcher, K., Kolari, P., Loehr, J., Hokkanen, T., Karhu, J., Linkosalmi, M., Tanis, C. M., Metsämäki, S., Tuovinen, J., Vesala, T., and Arslan, A. N.: Networked web-cameras monitor congruent seasonal development of birches with phenological field observations, Agr. Forest Meteorol., 249, 335–347, https://doi.org/10.1016/j.agrformet.2017.10.008, 2018b.
Petoukhov, V. and Semenov, V. A.: A link between reduced Barents-Kara sea ice and cold winter extremes over northern continents, J. Geophys. Res., 115, D21111, https://doi.org/10.1029/2009JD013568, 2010.
Pietikäinen, J.-P., O'Donnell, D., Teichmann, C., Karstens, U., Pfeifer, S., Kazil, J., Podzun, R., Fiedler, S., Kokkola, H., Birmili, W., O'Dowd, C., Baltensperger, U., Weingartner, E., Gehrig, R., Spindler, G., Kulmala, M., Feichter, J., Jacob, D., and Laaksonen, A.: The regional aerosol-climate model REMO-HAM, Geosci. Model Dev., 5, 1323–1339, https://doi.org/10.5194/gmd-5-1323-2012, 2012.
Pihlainen, S., Tahvonen, O., and Niinimäki, S.: The economics of timber and bioenergy production and carbon storage in Scots pine stands, Can. J. Forest Res., 44, 1091–1102, https://doi.org/10.1139/cjfr-2013-0475, 2014.
Pingoud, K., Ekholm, T., Sievänen, T., Huuskonen, S., and Hynynen, J.: Trade-offs between forest carbon stocks and harvests in a steady state A multi-criteria analysis, J. Environ. Manage., 210, 96–103, 2018.
Pudas, E., Leppälä, M., Tolvanen, A., Poikolainen, J., Venäläinen, A., and Kubin, E.: Trends in phenology of Betula pubescens across the boreal zone in Finland, Int. J. Biometeorol., 52, 251–259, 2008.
Pulliainen, J., Aurela, M., Laurila, T., Aalto, T., Takala, M., Salminen, M., Kulmala, M., Barr, A., Heimann, M., Lindroth, A., Laaksonen, A., Derksen, C., Mäkelä, A., Markkanen, T., Lemmetyinen, J., Susiluoto, J., Dengel, S., Mammarella, I., Tuovinen, J.-P., and Vesala, T.: Early snowmelt significantly enhances boreal springtime carbon uptake, P. Natl. Acad. Sci. USA, 2017, 201707889, https://doi.org/10.1073/pnas.1707889114, 2017.
Raddatz, T. J., Reick, C. H., Knorr, W., Kattge, J., Roeckner, E., Schnur, R., Schnitzler, K. G., Wetzel, P., and Jungclaus, J.: Will the tropical land biosphere dominate the climate-carbon cycle feedback during the twenty-first century?, Clim. Dynam., 29, 565–574, 2007.
Räisänen, J. and Räty, O.: Projections of daily mean temperature variability in the future: cross-validation tests with ENSEMBLES regional climate simulations, Clim. Dynam., 41, 1553–1568, 2013.
Räisänen, P., Luomaranta, A., Järvinen, H., Takala, M., Jylhä, K., Bulygina, O. N., Luojus, K., Riihelä, A., Laaksonen, A., Koskinen, J., and Pulliainen, J.: Evaluation of North Eurasian snow-off dates in the ECHAM5.4 atmospheric general circulation model, Geosci. Model Dev., 7, 3037–3057, https://doi.org/10.5194/gmd-7-3037-2014, 2014.
Randerson, J. T., Liu, H., Flanner, M. G., Chambers, S. D., Jin, Y., Hess, P. G., Pfister, G., Mack, M. C., Treseder, K. K., Welp, L. R., Chapin, F. S., Harden, J. W., Goulden, M. L., Lyons, E., Neff, J. C., Schuur, E. A. G., and Zender, C. S.: The Impact of Boreal Forest Fire on Climate Warming, Science, 314, 1130–1132, 2006.
Räty, O., Räisanen, J., and Ylhäisi, J. S.: Evaluation of delta change and bias correction methods for future daily precipitation: intermodel cross-validation using ENSEMBLES simulations, Clim. Dynam., 42, 2287–2303, 2014.
Reick, C. H., Raddatz, T., Brovkin, V., and Gayler, V.: Representation of natural and anthropogenic land cover change in MPI-ESM, J. Adv. Model. Earth Sy., 5, 459–482, 2013.
Rinne, J., Aurela, M., and Manninen, T.: A Simple Method to Determine the Timing of Snow Melt by Remote Sensing with Application to the CO2 Balances of Northern Mire and Heath Ecosystems, Remote Sensing, 1, 1097–1107, 2009.
Roesch, A., Wild, M., Gilgen, H., and Ohmura, A.: A new snow cover fraction parameterization for the ECHAM4 GCM, Clim. Dynam., 17, 933–946, 2001.
Ruosteenoja, K.: Maailmanlaajuisiin ilmastomalleihin perustuvia lämpötila- ja sademääräskenaarioita. Sektoritutkimusohjelman ilmastoskenaariot (SETUKLIM) 1. osahanke, Ilmatieteen laitos, 15 pp., 2013 (in Finnish).
Ruosteenoja, K., Jylhä, K., and Kämäräinen, M.: Climate projections for Finland under the RCP forcing scenarios, Geophysica, 51, 17–50, 2016a.
Ruosteenoja, K., Räisänen, J., Venäläinen, A., Kämäräinen, M., and Pirinen, P.: Terminen kasvukausi lämpenevässä ilmastossa, Terra, 128, 3–15, 2016b (in Finnish with English summary).
Salminen, S.: Eteläisimmän Suomen metsävarat 1986–1988, Folia Forestalia, 825, 1–116, 1993 (in Finnish).
Salminen, S. and Salminen, O.: Metsävarat keskisessä Suomessa 1988–92 sekä koko Etelä-Suomessa 1986–92, Metsäntutkimuslaitoksen tiedonantoja 710, 1998 (in Finnish).
Shutova, E., Wielgolaski, F. E., Karlsen, S. R., Makarova, O., Berlina, N., Filimonova, T., Haraldsson, E., Aspholm, P. E., Flø, L., and Høgda, K. A.: Growing seasons of Nordic mountain birch in northernmost Europe as indicated by long-term field studies and analyses of satellite images, Int. J. Biometeorol., 51, 155–166, 2006.
Solantie, R., Drebs, A., Hellsten, E., and Saurio, P.: Lumipeitteen tulo-, lähtö- ja kestoajoista Suomessa talvina 1960/1961 – 1992/1993, Finnish Meteorological Institute, Meteorological publications No. 34, 159 pp., 1996 (in Finnish with English summary).
Solantie, R.: Productivity of boreal forests in relation to climate and vegetation zones, Boreal Environ. Res., 10, 275–297, 2005.
Stevens, B., Giorgetta, M., Esch, M., Mauritsen, T., Crueger, T., Rast, S., Salzmann, M., Schmidt, H., Bader, J., Block, K., Brokopf, R., Fast, I., Kinne, S., Kornblueh, L., Lohmann, U., Pincus, R., Reichler, T., and Roeckner, E.: Atmospheric component of the MPI-M Earth System Model: ECHAM6, J. Adv. Model. Earth Sy., 5, 146–172, https://doi.org/10.1002/jame.20015, 2013.
Sutinen, S., Roitto, M., Lehto, T., and Repo, T.: Simulated snowmelt and infiltration into frozen soil affected root growth, needle structure and physiology of Scots pine saplings, Boreal Environ. Res., 19, 281–294, 2014.
Tanis, C. M, Peltoniemi, M., Linkosalmi, M., Aurela, M., Böttcher, K., Manninen, T., and Arslan, A. N.: A system for acquisition, processing and visualization of image time series from multiple camera networks, Data, 3, 23, https://doi.org/10.3390/data3030023, 2018.
Teutschbein, C. and Siebert, J.: Bias correction of regional climate model simulations for hydrological climate-change impact studies: Review and evaluation of different methods, J. Hydrol., 456–457, 12–29, 2012.
Thackeray, S. J., Fletcher, C. G., Mudryk, L. R., and Derksen, C.: Quantifying the Uncertainty in Historical and Future Simulations of Northern Hemisphere Spring Snow Cover, J. Climate, 29, 8647–8663, 2016.
Tomppo, E., Henttonen, H., Korhonen, K. T., Aarnio, A., Ahola, A., Heikkinen, J., Ihalainen, A., Mikkelä, H., Tonteri, T., and Tuomainen, T.: Etelä-Pohjanmaan metsäkeskuksen alueen metsävarat ja niiden kehitys 1968–97, Metsätieteen aikakauskirja – Folia Forestalia 2B/1998, 293–374, 1998 (in Finnish).
Tomppo, E., Henttonen, H., Ihalainen, A., Tonteri, T., and Tuomainen, T.: Etelä-Savon metsäkeskuksen alueen metsävarat 1966–2000, Metsätieteen aikakauskirja 2B/2001, 309–388, 2001 (in Finnish).
Tomppo, E., Tuomainen, T., Henttonen, H., Ihalainen, A., and Tonteri, T.: Kainuun metsäkeskuksen alueen metsävarat 1969–2001, Metsätieteen aikakauskirja 2B/2003, 169–256, 2003 (in Finnish).
Tomppo, E., Tuomainen, T., Henttonen, H., Ihalainen, A., Korhonen, K. T., Mäkelä, H., and Tonteri, T.: Pohjois-Pohjanmaan metsäkeskuksen alueen metsävarat 1968–2002, Metsätieteen aikakauskirja 3B/2004, 339–418, 2004 (in Finnish).
Tomppo, E., Tuomainen, T., Heikkinen, J., Henttonen, H., Ihalainen, A., Korhonen, K. T., Mäkelä, H., and Tonteri, T.: Lapin metsäkeskuksen alueen metsävarat 1970–2003, Metsätieteen aikakauskirja 2B/2005, 199–287, 2005 (in Finnish).
Tomppo, E., Heikkinen, J., Henttonen, H. M., Ihalainen, A., Katila, M., Mäkelä, H., Tuomainen, H., and Vainikainen, N.: Designing and Conducting a Forest Inventory-case: 9th National Forest Inventory of Finland: Designing and Conducting a Forest Inventory-Case: 9Th National Forest Inventory of Finland, Springer, 2011.
Vihma, T.: Effects of Arctic Sea Ice Decline on Weather and Climate: A Review, Surv. Geophys., 35, 1175–1214, https://doi.org/10.1007/s10712-014-9284-0, 2014.