the Creative Commons Attribution 4.0 License.
the Creative Commons Attribution 4.0 License.
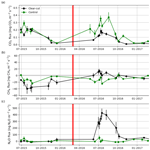
Greenhouse gas and energy fluxes in a boreal peatland forest after clear-cutting
Juha-Pekka Tuovinen
Timo Penttilä
Sakari Sarkkola
Paavo Ojanen
Kari Minkkinen
Juuso Rainne
Tuomas Laurila
Annalea Lohila
The most common forest management method in Fennoscandia is rotation forestry, including clear-cutting and forest regeneration. In clear-cutting, stem wood is removed and the logging residues are either removed or left on site. Clear-cutting changes the microclimate and vegetation structure at the site, both of which affect the site's carbon balance. Peat soils with poor aeration and high carbon densities are especially prone to such changes, and significant changes in greenhouse gas exchange can be expected. We measured carbon dioxide (CO2) and energy fluxes with the eddy covariance method for 2 years (April 2016–March 2018) after clear-cutting a drained peatland forest. We observed a significant rise (23 cm) in the water table level and a large CO2 source (first year: 3086±148 g CO2 m−2 yr−1; second year: 2072±124 g CO2 m−2 yr−1). These large CO2 emissions resulted from the very low gross primary production (GPP) following the removal of photosynthesizing trees and the decline of ground vegetation, unable to compensate for the decomposition of logging residues and peat. During the second summer (June–August) after the clear-cutting, GPP had already increased by 96 % and total ecosystem respiration decreased by 14 % from the previous summer. The mean daytime ratio of sensible to latent heat flux decreased after harvesting from 2.6 in May 2016 to 1.0 in August 2016, and in 2017 it varied mostly within 0.6–1.0. In April–September, the mean daytime sensible heat flux was 33 % lower and latent heat flux 40 % higher in 2017, probably due to the recovery of ground vegetation that increased evapotranspiration and albedo of the site. In addition to CO2 and energy fluxes, we measured methane (CH4) and nitrous oxide (N2O) fluxes with manual chambers. After the clear-cutting, the site turned from a small CH4 sink into a small source and from N2O neutral to a significant N2O source. Compared to the large CO2 emissions, the 100-year global warming potential (GWP100) of the CH4 emissions was negligible. Also, the GWP100 due to increased N2O emissions was less than 10 % of that of the CO2 emission change.
- Article
(8610 KB) -
Supplement
(719 KB) - BibTeX
- EndNote
Northern peatlands cover approximately 3 % of the Earth's land surface (Clarke and Rieley, 2010), most of which is located in the boreal region (Fischlin et al., 2007). Boreal and subarctic peatlands are substantial reservoirs of carbon (C), storing 270–550 Pg C in total (Turunen et al., 2002; Yu, 2011). These reservoirs are affected by peatland drainage, which has been a common practice in northern countries. In Finland, more than half of the original peatland area of 100 000 km2 has been drained, mostly for forestry (Päivänen and Hånell, 2012). Drainage lowers water table level (WTL), which accelerates the peat decomposition rate due to the increased availability of oxygen (Drzymulska, 2016). This often leads to higher carbon dioxide (CO2) emissions from the soil (Maljanen et al., 2010; Ojanen et al., 2013). On the other hand, a well-performed drainage increases root aeration and nutrient availability, which enhances tree growth and CO2 uptake by trees (Minkkinen et al., 2001). In addition, methane (CH4) emissions decrease, and the soil may even turn into a net CH4 sink if the site is well-drained (Maljanen et al., 2010; Ojanen et al., 2010). This results from the thickening of the oxic peat layer, which enhances CH4 oxidation in the soil and from the disappearance of deep-rooted vascular plants, which in natural mires feed anaerobic microbes with fresh carbon and transport methane from the anoxic peat layer to atmosphere through their aerenchyma. In contrast to CH4, nitrous oxide (N2O) emissions often increase after drainage in nitrogen-rich minerotrophic peatlands (Maljanen et al., 2010; Ojanen et al., 2010, 2018) because nitrification (the process that produces nitrate and N2O as a by-product) needs nitrogen and oxic conditions.
The most common method of forest management in Finland is rotation forestry including clear-cutting and forest regeneration. In clear-cutting, stem wood is removed, while the logging residues, i.e. foliage biomass, branches, stumps and roots, are either removed or left on site. After clear-cutting, the site is prepared, e.g. by mounding or scalping, and a new tree generation is established by planting or sowing or naturally from surrounding seed trees. Removing the trees changes the local microclimate as more solar radiation reaches the soil surface, which increases soil temperature (Edwards and Ross-Todd, 1983; Londo et al., 1999) and its diel variation. This affects the carbon cycle, as higher soil temperature potentially increases soil respiration. In peatland forests, however, removing tree biomass raises the WTL by diminishing transpiration (Sarkkola et al., 2010), which in turn may decrease soil respiration and slow down the peat decomposition rate due to the reduced volume of aerated peat. On the other hand, photosynthesis is diminished due to the removal of trees and decline of ground vegetation. Mäkiranta et al. (2010) found that ground vegetation recovers rather fast in a peatland forest after clear-cutting; however, after 3 years the recovery was still insufficient to compensate for the high ecosystem respiration produced by the large amount of fresh organic matter. The increase in ecosystem respiration is accounted for by the decay of logging residues and possibly also by increased soil organic matter decomposition under these residues (Mäkiranta et al., 2012; Ojanen et al., 2017). As boreal forest grows slowly, it may take from 8 up to 20 years to turn the forest back to a net carbon sink (Fredeen et al., 2007; Kolari et al., 2004; Mäkiranta et al., 2010; Pypker and Fredeen, 2002; Rannik et al., 2002; Schulze et al., 1999).
The CO2 and energy fluxes between ecosystems and the atmosphere are commonly measured with the eddy covariance (EC) method (Aubinet et al., 2012). Previous work concerning clear-cutting in forests with mineral soil has involved both EC (Clark et al., 2004; Humphreys et al., 2005; Kolari et al., 2004; Kowalski et al., 2004, 2003; Machimura et al., 2005; Mamkin et al., 2016; Takagi et al., 2009; Williams et al., 2013) and soil chambers (Howard et al., 2004; Londo et al., 1999; Zerva and Mencuccini, 2005). Also, soil chambers have been used on clear-cut peatland forests (Mäkiranta et al., 2010; Pearson et al., 2012), whereas EC measurements have been lacking. In addition, most of this peatland forest gas exchange research has concentrated on carbon dynamics and overlooked the possibly important variations in energy and water fluxes.
Exchange of sensible and latent heat constitutes an important part of the surface energy balance, driving many local-, regional- and global-scale climatological processes. The available energy for these turbulent fluxes is determined predominantly by net radiation and the ground heat flux. The partitioning of available energy between the sensible and latent heat fluxes, which can be described in terms of the Bowen ratio, is strongly influenced by vegetation and soil properties (Betts et al., 2007). Also, release of latent heat to the atmosphere, i.e. evapotranspiration, is a key component of the water cycle in peatlands and forests, also affecting soil moisture and forest productivity, which further affect the GHG fluxes in a forest. In a temperate deciduous broadleaf forest, evapotranspiration has been found to recover rapidly after clear-cutting: the latent heat flux increased over the first 3 years, while the sensible heat flux declined correspondingly (Williams et al., 2013). It is unknown if this also happens in peatland forests.
In this study, we investigated the GHG and energy fluxes between the forest floor and the atmosphere and their environmental drivers after clear-cutting in a boreal peatland forest in southern Finland. The study was based on EC measurements made for 2 years (April 2016–March 2018) after clear-cutting and on soil chamber measurements performed during the period of June 2015–August 2017. Our specific aims were as follows:
-
to estimate the magnitude of CO2 fluxes and their environmental drivers after clear-cutting,
-
to quantify the development of surface heat fluxes after clear-cutting,
-
to investigate how soil CH4 and N2O fluxes change due to clear-cutting.
2.1 Measurement site
The measurements were set up in a nutrient-rich peatland forest called Lettosuo, which is located in southern Finland (60∘38′ N, 23∘57′ E). The site was drained with widely spaced, manually dug ditches probably during the 1930s, then drained more effectively in 1969 and fertilized with phosphorus and potassium. The distance between the ditches is on average 45 m, and they were dug ca. 1 m deep but have since been partially filled with vegetation. After drainage and before clear-cutting in 2016, the tree stand was dominated by Scots pine (Pinus sylvestris), with some pubescent birch (Betula pubescens). The understorey included mostly Norway spruce (Picea abies) and some small-sized pubescent birch. The tree stand was quite dense, which made the ground vegetation and moss layer patchy and variable due to irregular shading. Ground vegetation included herbs, such as Trientalis europaea and Dryopteris carthusiana, and dwarf shrubs, such as Vaccinium myrtillus (Bhuiyan et al., 2017). The moss layer was dominated by Pleurozium schreberi and Dicranum polysetum, with some Sphagnum mosses, such as Sphagnum capillifolium, S. angustifolium and S. russowii, in moist patches.
Before clear-cutting, the average soil organic carbon content at the site was 58 % and carbon distribution was rather uniform across different soil layers (Table 1). The total soil nitrogen content was lowest in the litter layer (1.7 %) and varied from 2.2 % up to 2.5 % among the other soil layers. The C:N ratio averaged at 27, which is typical for peatland forests with a fen history. The mean soil bulk density of the 0–40 cm layer was 0.14 g cm−3. The present-day peat thickness at Lettosuo varies mostly between 1.5 and 2.5 m. Assuming a thickness of 2 m results in soil organic carbon and nitrogen stock estimates of 156±72 kg C m−2 and 6.4±2.9 kg N m−2 (± SD), respectively.
Table 1Mean soil organic carbon, nitrogen, C:N ratio, bulk density and their standard deviation in different soil layers determined before the harvest (n = 3–33).
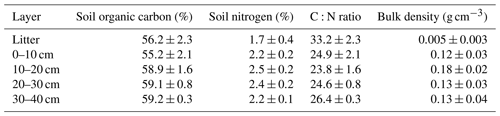
Clear-cutting was performed within a trapezoidal area of 23 500 m2 (Fig. 1) between 29 February and 16 March 2016. After clear-cutting, the logging residues were left at the site. The previous ground vegetation was almost totally destroyed in the harvesting operation and the following drastic increase in solar radiation. In the following summer, some species adapted to the open, well-lit conditions; for example, Rubus idaeus, Carex canescens and Dryopteris carthusiana were observed here and there within the clear-cut site. Mounding was performed on 1–2 August 2016, and spruce (Picea abies) seedlings were planted in 2017. In addition to the clear-cut site, we conducted GHG measurements on a similarly sized control site located southwest of the clear-cut site, where the forest was left in its original state with similar tree stand and vegetation composition to at the preharvest clear-cut site.
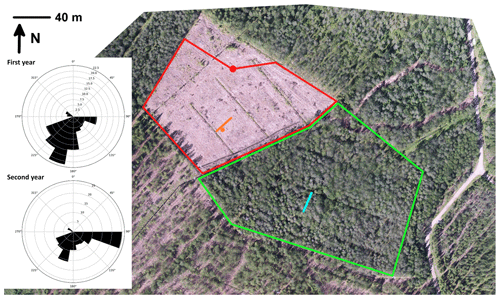
Figure 1Aerial view of the clear-cut site and the surrounding forest at Lettosuo. The red dot shows the location of the eddy covariance mast, and the red lines surround the target area (80–315∘). The meteorological measurements were conducted at the location indicated by the orange dot, while the orange line marks the water table, soil temperature and chamber measurements (4, 8, 12 and 22.5 m from the ditch). Similar measurements of greenhouse gas fluxes, water table and soil temperature within the control site, surrounded by the green lines, are shown with a light blue line. The wind roses on the left show the cumulative footprint contributions (in percentage) of the accepted flux data.
2.2 Measurement system
An EC system to measure turbulent CO2 and energy fluxes was set up in the northeastern part of the clear-cut (Fig. 1), and the measurements started on 8 April 2016, approximately 3 weeks after the clear-cutting had ended. The measurements continued until 7 April 2018. From this point on, the time periods of 8 April 2016–7 April 2017 and 8 April 2017–7 April 2018 are referred to as the first and second EC measurement year, respectively. The EC system included a three-axis sonic anemometer (uSonic-3 Scientific, METEK, Elmshorn, Germany) for wind speed and air temperature and a closed-path infrared gas analyser (LI-7000, Licor Biosciences, Lincoln, NE, USA) for CO2 and H2O mixing ratios. A sampling rate of 10 Hz was used for the EC system. The measurement height was 2.75 m, the flow rate was about 6 L min−1 and the length of the inlet tube (inner diameter 3.1 mm, Bevaline IV) was 8 m. The mouth of the inlet tube was positioned 15 cm below the sonic anemometer. Air with a zero CO2 concentration was used as the reference gas when calibrating the gas analyser. The micrometeorological sign convention is used throughout the paper: a positive flux indicates a flux from the ecosystem to the atmosphere (net emission) and a negative flux indicates a flux from the atmosphere into the ecosystem (net uptake).
Auxiliary meteorological measurements were installed at the centre of the clear-cut (80 m south of the EC mast) on 24 July 2015, i.e. before the clear-cutting. A similar system was installed 2 weeks earlier for the control site (180 m southeast of the EC mast). Air temperature and relative humidity were measured at 2 m height (HMP155, Vaisala Corporation, Vantaa, Finland) and soil temperature profile was measured at 5, 10, 20 and 30 cm depths (Pt100, Vaisala Corporation, Vantaa, Finland); the measurements also included net radiation (NR Lite2 Net Radiometer, Kipp & Zonen, Delft, The Netherlands), photosynthetically active photon flux density (PPFD) (PQS1 PAR Quantum Sensor, Kipp & Zonen, Delft, the Netherlands) and ground heat flux (HFP01, Hukseflux Thermal Sensors B.V., Delft, the Netherlands). In addition to these, global radiation (Pyranometer CMP3, Kipp & Zonen, Delft, The Netherlands) was measured from another EC mast above the canopy of the surrounding forest (250 m south from the clear-cut EC mast). The data were collected by data loggers (QML201C, Vaisala Corporation, Vantaa, Finland) as 30 min averages.
2.3 EC data processing
Half-hourly turbulent fluxes were calculated using standard EC methods (Aubinet et al., 2012). The 10 Hz raw data were block-averaged, and a double rotation of the coordinate system was applied (McMillen, 1988). The time lag between the gas analyser and sonic anemometer signals was determined by cross-correlation analysis for each flux variable and 30 min period. Water vapour fluctuations affecting flux measurements with LI-7000 were compensated for (Webb et al., 1980); a corresponding compensation is not necessary for temperature (Rannik et al., 1997). The fluxes were corrected for systematic losses due to block averaging and attenuation of the highest frequencies in the cospectra between vertical wind speed and mixing ratio; the transfer function method of Moore (1986) was used for this. For the high-frequency losses, the transfer functions describing the flux attenuation were determined separately for CO2 and water vapour fluxes (with half-power frequencies of 1.3 and 0.6 Hz, respectively) using temperature cospectra as the reference. These functions were convoluted with generic cospectral distributions (Kaimal and Finnigan, 1994) to calculate the flux correction as a function of wind speed and atmospheric stability.
The 30 min averaged data were screened according to the following acceptance criteria: relative stationarity (Foken and Wichura, 1996) <100 %, internal LI-7000 pressure >60 kPa, CO2 mixing ratio >350 ppm, wind direction within 80–315∘, number of spikes in the vertical wind speed and CO2 concentration data <150 of 18 000. Also, the periods of weak turbulence were discarded by applying a friction velocity (u∗) limit of 0.125 m s−1 (Fig. S1 in the Supplement). In addition, the footprint accumulated within the target area shown in Fig. 1 was required to exceed a limit of 0.75 to ensure that the measured flux originated predominantly from the clear-cut site. The footprints were calculated using the model developed by Kormann and Meixner (2001) and input data measured with the sonic anemometer.
In addition to the vertical turbulent fluxes, the CO2 fluxes associated with the storage of CO2 below the measurement height were calculated from the change in the mean CO2 concentration profile between consecutive half-hour periods. It was assumed that the CO2 concentration in the air column from ground level to the measurement height of 2.75 m was constant.
There were only two periods when a measurement gap in the data was longer than 5 d: 30 September–5 October 2016 and 28 April–4 May 2017. After applying all the data filters described above, 30 % of the 32 635 half-hour periods recorded within the measurement period were accepted for further analysis (Table S1, Fig. S2 in the Supplement). The gap-filling procedure and uncertainty analysis of the net ecosystem exchange of CO2 (NEE) are described in Appendices A and B.
2.4 Surface energy balance and Bowen ratio
The surface energy balance can be expressed as
where QH is sensible heat flux, QE is latent heat flux, QN is net radiation, QG is the ground heat flux, and QS is the sum of storage fluxes from other energy sinks and sources. In this study, we assumed that QS=0.
The Bowen ratio, defined as
is used to describe the partitioning of QN−QG to QH and QE at the surface. When β<1, more available energy at the surface is released to the atmosphere as latent heat than as sensible heat (and vice versa when β>1). In this study, we present monthly Bowen ratios that were calculated from the monthly mean daytime (10:00–16:00, UTC+2) fluxes of QH and QE.
Gap-filling of the energy fluxes is described in Appendix C.
2.5 Chamber measurements of GHG fluxes
For manual chamber measurements, sampling transects were set up within the clear-cut and control sites, where the GHG fluxes were measured between 29 June 2015 and 29 August 2017, mostly during the snow-free periods. The measurement interval varied between 1 week and 1 month, but there were longer gaps in autumn 2015 and spring 2016. The transect had two flux measurement plots at a distance of 4, 8, 12 and 22.5 m from the ditch, and at each distance there was an automatic WTL logger close to the flux measurement plots (see Sect. 2.7 for details). In addition, all the flux plots included a soil temperature data logger (iButton DS1921G, Maxim Integrated Products) at 5 cm depth and two of them (8 and 22.5 m from the ditch) also had a similar logger at 30 cm depth. Before starting the measurements, 2 cm deep grooves were carved into the soil surface for the chambers, and the grooves were occasionally renewed when necessary to keep the chamber-sealing adequate. It should be noted that, even though logging residues were left at the site, the measurement plots did not have any above-ground residues. The fluxes were measured using a closed-chamber system with an opaque cylindrical chamber (height 30.5 cm, diameter 31.5 cm) including a mixing fan. The measurements were made in two different ways: using (1) a portable analyser and (2) a stationary analyser. As a portable analyser, we employed a Gasmet DX4015 (Gasmet Technologies Oy, Helsinki, Finland), based on Fourier transform infrared spectroscopy, to measure CO2, CH4 and N2O mixing ratios every 5 s. At the clear-cut site, only the portable gas analyser was used. In this setup, the sampled air was circulated in a loop between the gas analyser and the chamber, and the closure time was 10–11 min. At the control site, the portable analyser was used in 2015 for all the gases, but from 2016 onwards the analyser was used only for measuring the N2O mixing ratios. Starting from 2016 at the control site, we connected the portable gas analyser in series with a Picarro G1130 cavity ring-down spectroscopy gas analyser (Picarro Inc., Santa Clara, CA, USA) to acquire more precise CO2 and CH4 mixing ratios. In this system, the gas analysers were stored in a cabin and connected to the chamber with 50 m long tubing. The instruments were compared against each other and observed to result in very similar flux estimates with the 10–11 min closure time. The error caused by not returning the sampled air back to the chamber was corrected for in the data analysis.
The fluxes were calculated the same way for all the gases (Korkiakoski et al., 2017). In short, both linear and exponential regression models were first fitted to the mixing ratio time series using the least-squares approach. The start and end points of the chamber closure were visually identified from the data for each closure. The first minute of each measurement was discarded to ensure that the sample air was properly mixed inside the chamber. After fitting, the mass flux (F) was calculated as
where is the mixing ratio change in time determined from a linear or exponential model at the beginning of the closure; M is the molecular mass of CO2, CH4, or N2O (44.01, 16.04 and 44.01 g mol−1, respectively); P is air pressure; R is the universal gas constant (8.314 J mol−1 K−1); T is the mean chamber headspace temperature during closure; and V and A are the volume and the base area of the chamber headspace, respectively. The snow depth and the height of mosses and other vegetation in the chamber headspace volume were taken into account, ignoring the pore space in the soil and snow. However, if the soil surface was frozen, the measurements were not made as it was not possible to properly seal the chamber. Finally, analyser-specific flux limits were determined to choose between the linear and exponential models (Korkiakoski et al., 2017). If the flux calculated with the linear model was smaller than the limit, then this estimate was considered more robust for the noisy data and adopted for the later analysis. These limits for the portable system were 5.6 µg CO2 m−2 s−1, 9.7 ng CH4 m−2 s−1 and 12.5 ng N2O m−2 s−1. We did not define a corresponding limit for the CO2 flux measured with the Picarro gas analyser at the control site as the fluxes were always sufficiently large for using exponential fitting, but for CH4 the limit was set to 0.7 ng CH4 m−2 s−1.
The CO2 flux measured with chambers represents forest floor respiration (Rff), which is defined as the sum of heterotrophic and autotrophic respiration. As clear-cutting affects soil processes, it should be noted that the Rff before and after clear-cutting consists of different components. Before the clear-cutting (the 2015 data), Rff includes ground vegetation and living roots of trees and ground vegetation, but after clear-cutting (2016–2017) it instead includes the survived and regrown ground vegetation and living and dead roots.
The summertime sum of Rff was calculated for each summer separately by using Eq. (A3) with hourly soil temperature at 5 cm depth. The uncertainty of this sum was estimated from the minimum and maximum sums based on the standard errors of model parameters (R0 and E). The minimum and maximum sums were slightly asymmetrical around the mean sum, so the largest deviation from the mean sum was adopted as a conservative uncertainty estimate.
2.6 Water table level measurements and analysis
The water table levels within both the clear-cut and non-managed control site were measured for 1 year before (2015) and 2 years after the clear-cutting (2016–2017). Four automatic monitoring plots consisting of dipwells (perforated plastic tubes 120 cm long and 3.5 cm in diameter) were set up at the centre of each site (Fig. 1), located at a distance of 4, 8, 12 and 22.5 m in a transect perpendicular to the ditch (ditch spacing was 45 m). In addition, in order to calibrate the automatic water table measurement data, manually monitored dipwells were installed close to the automatically monitored dipwells within the clear-cut and control sites. WTL was measured manually at weekly or fortnightly intervals during March–November. From the automated dipwells, WTL was recorded with automatic probes (TruTrack WT-HR-logger, Intech Instruments Ltd, Auckland, New Zealand; Odyssey Capacitance Water Level Logger, Dataflow Systems Limited, Christchurch, New Zealand) at hourly intervals. The recorded values were then calibrated with linear regression using the manually measured WTL data from both the control and clear-cut site.
2.7 Statistical analysis
The linear mixed-effect model was used for testing for differences in the daily mean CO2, CH4 and N2O fluxes between the clear-cut and control sites and between the years in the chamber measurement data. In both cases, the chamber plots were treated as a random effect. The fixed effects of the model were the site type (clear-cut or control) and the measurement year when comparing the measurements made at different sites and in different years, respectively. The linear mixed-effect model was carried out with the R programming language (R Core Team, 2018, version 3.5.0) using the “lme4” package. The normality of model residuals was visually checked using quantile–quantile plots. The differences were tested with Tukey's honestly significant difference post hoc test.
The analysis of the clear-cutting effects on WTL was based on the paired treatment approach (also called calibration period – control area method) (e.g. Kaila et al., 2014; Laurén et al., 2009). We first calculated linear regressions between the WTL within the control and clear-cut sites for the pretreatment period using the WTL logger data from 2015. Then we used this regression model and the post-treatment WTL data from the control site to predict WTL for the clear-cut site as if it had not been harvested. The clear-cut effect was calculated as the difference between the calibrated post-clear-cut WTL measurements and the predicted background WTL values in the clear-cut site after the harvest.
3.1 Meteorological and hydrological conditions
The long-term (1981–2010) mean annual, winter (DJF) and summer (JJA) air temperatures at the nearby (Jokioinen, 35 km northwest of Lettosuo) weather station were 4.6, −5.3 and 15.2 ∘C, respectively (Pirinen et al., 2012). The mean annual temperature before the clear-cutting in 2015 at Jokioinen was 6.2 ∘C. Also, the winter (2015–2016) before the clear-cutting was warmer (−3.4 ∘C), while summer 2015 was colder (14.4 ∘C) than the long-term mean. The mean post-clear-cut annual air temperatures at the EC site during the first and second EC measurement year were 5.6 and 4.4 ∘C, respectively (Fig. 2a); this difference was reflected in soil temperatures (Fig. 2b). Both post-clear-cut winters were warmer (2016–2017: −3.0 ∘C; 2017–2018: −3.4 ∘C) than the long-term average. However, the mean summer temperature at the EC site in 2016 was similar to (15.3 ∘C), and in 2017 was cooler (by 1.6 ∘C) than, in 1981–2010. In addition, compared to the precipitation at Jokioinen (long-term average 627 mm), the first EC measurement year was drier (502 mm) and the second year similar (656 mm) (Fig. 2c), while the pre-clear-cut year of 2015 was wetter (680 mm) than both post-clear-cut years. The autumn (SON) 2016 and winter 2016–2017 were especially dry, while the springs and summers were quite similar to the long-term average conditions.
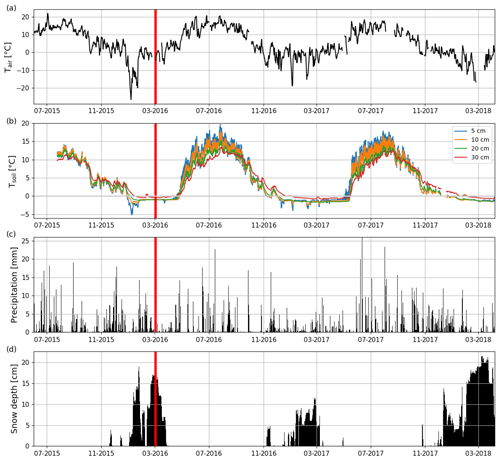
Figure 2Time series of (a) daily mean air temperature (Tair) and (b) hourly mean soil temperatures at 5, 10, 20, and 30 cm depths (Tsoil) measured at Lettosuo, and (c) daily precipitation sum and (d) daily mean snow level recorded at the Jokioinen observatory (35 km northwest of Lettosuo). The clear-cutting (red vertical line) was carried out in February–March 2016.
Both winters had a shallower snow cover than the long-term average annual maximum (28 cm; Pirinen et al., 2012). The maximum snow depths at Jokioinen were 12 and 22 cm in 2016–2017 and 2017–2018, respectively. Due to the especially shallow snow cover in the first winter and the high temperatures in March, all the snow had melted by 23 March 2017 (Fig. 2d). However, in 2018, the snow cover was still intact when the measurement period ended on 7 April.
The preharvest soil temperatures at 30 cm depth were similar within the clear-cutting and control sites, but clear-cutting increased their temporal variation (Fig. S3). After clear-cutting in late spring, the daily mean 30 cm soil temperature rose faster and was higher throughout the summer within the clear-cut than at the control site. Also, the 30 cm temperature within the clear-cut started to decline earlier in autumn and was lower during winter within the clear-cut than the control site. The monthly mean diel variation in soil temperature at 5 cm depth ranged from 0.8 to 1.3 ∘C in July–September 2015 before clear-cutting (Fig. S4). After clear-cutting, this variation increased to 2.3–2.9 and 2.1–3.7 ∘C in 2016 and 2017, respectively. On average the diel variation was 1.8 ∘C larger in July–September after the clear-cutting than before it (Figs. 2b and S4). Increases in the diel temperature range were also observed at the 10 cm (1.2 ∘C) and 20 cm (0.33 ∘C) depths but not at 30 cm.
The mean WTL in July–August 2015 was −33 cm within the clear-cut area, while at the control site it was −50 cm (Fig. 3). After clear-cutting, the WTL rose and remained continuously at a markedly higher level than the predicted background WTL and the measured WTL at the control site. At the clear-cut site, the WTL rose to −22 and −27 cm in July–August 2016 and 2017, respectively. On the other hand, the WTL at the control site sank to −55 cm during these months in both post-harvest years. The largest post-treatment rise occurred in July–October when the average WTL was −23 and −24 cm in the clear-cut and −46 and −49 cm in the background model, corresponding to a 23 and 25 cm rise in WTL in 2016 and 2017, respectively (Fig. 3). As a consequence of heavy rain episodes in summer and autumn in 2017, the amplitude of WTL variations was larger then than the previous year.
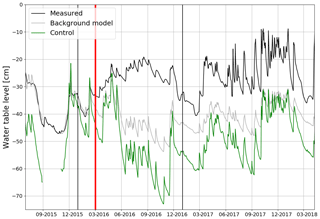
Figure 3Mean daily water table level at the control site (measured; green) and the clear-cut site with (measured; black line) and without (estimated; grey line) the harvesting effect averaged over all the measurement points (4, 8, 12 and 22.5 m from the ditch) from June 2015 to October 2017. The clear-cutting (red vertical line) was carried out in February–March 2016.
3.2 CO2 exchange on an ecosystem level
3.2.1 Seasonal and diel variations
When the measurements started at the beginning of April 2016, the daily mean NEE was mainly <0.08 mg CO2 m−2 s−1 but started to increase with temperature at the end of April, after the daily mean temperature exceeded 5 ∘C (Figs. 2a and 4). There was a substantial temporary drop in NEE in the first half of June after a cold spell when the daily mean temperature decreased from 20 to 10 ∘C. By the end of June, NEE stabilized at around 0.21 mg CO2 m−2 s−1. From the end of August, NEE decreased until reaching a stable level of 0.03 mg CO2 m−2 s−1 in January 2017. At the end of March 2017, NEE started to gradually increase, and in mid-May it quickly rose to 0.08 mg CO2 m−2 s−1. After this, NEE continued to increase, stabilizing at around 0.13 mg CO2 m−2 s−1 in August and then decreasing from the end of September onwards.
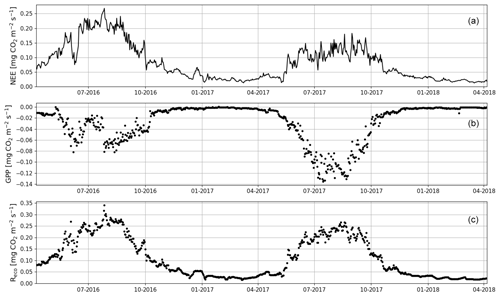
Figure 4The gap-filled time series of daily mean net ecosystem exchange (NEE, a) and the corresponding modelled gross primary production (GPP, b) and ecosystem respiration (Reco, c) based on Eqs. (A2) and (A3), respectively.
Even though all the big trees were removed in clear-cutting and most of the ground vegetation was destroyed, some small understorey trees were left at the site and weak photosynthesis could be observed simultaneously with the increased respiration in the end of April 2016 (Fig. 4). In May, the estimated magnitude of gross primary production (, Appendix A) increased to 0.05 mg CO2 m−2 s−1, which was about 25 % of the respiration rate at that time. However, decreased to 0.02 mg CO2 m−2 s−1 in June, after which it started to increase again, reaching its maximum at 0.08 g CO2 m−2 s−1 at the end of July and the beginning of August. From that point on, photosynthesis started to weaken, marking the ending of the growing season, and ceased in mid-October, about a month before the first snow. In 2017, increased from mid-May until July, when it stabilized at around 0.11 mg CO2 m−2 s−1. Similarly to in 2016, decreased from August onwards.
The correlation between the night-time NEE (i.e. Reco in Eq. A3) and the 5 cm soil temperature was stronger in summer 2017 than 2016 (Fig. 5a). The model fits (Eq. A3) indicate that the temperature response of ecosystem respiration (parameter E) was also stronger in 2017. However, the base respiration rate R0 (Reco at 10 ∘C) was larger in the first than the second summer (0.199 vs. 0.173 mg CO2 m−2 s−1). In addition, no correlation was found between the model residuals and WTL in either summer (Fig. S5). Like the temperature response of Reco, the light response of GPP was stronger in summer 2017 than 2016 (Fig. 5b). Also, the corresponding maximum gross photosynthesis rate (GPmax, Eq. A2) more than doubled (−0.226 vs. −0.100 mg CO2 m−2 s−1).
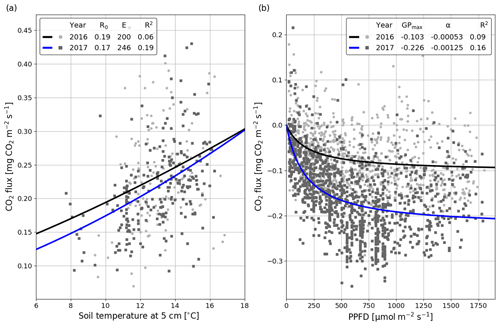
Figure 5Temperature (a; Eq. A3) and light responses (b; Eq. A2) of 30 min CO2 fluxes for the summers of (JJA) 2016 (black) and 2017 (blue). The temperature response equation was fitted to the night-time data (PPFD < 20 µmol m−2 s−1), and the light response equation was fitted to the daytime (PPFD > 20 µmol m−2 s−1) data.
The site was on average a CO2 source throughout the day during the summer as well. It should be noted, however, that there were still multiple half-hour periods, especially in August 2017, when the site acted as a CO2 sink. A noticeable diel variation in NEE was observed mainly from April to October (Fig. 6). Between April and August, the mean diel NEE cycle had a minimum during the morning hours (05:00–10:00, UTC+2) and the highest emissions during the evening and night (21:00–01:00). After August, the lowest emissions took place later, at around noon. On the other hand, the highest emissions were observed earlier, at 20:00 in September and at 18:00 in October. In October, the diel cycle of NEE was still noticeable; it vanished in November and reappeared in March 2017. In spite of the different mean fluxes, the amplitudes of their diel cycle were rather similar in 2016 and 2017, except from July to September when the amplitudes were much larger in 2017. Also, unlike in 2016, systematic diel variation was still obvious in November 2017. Even though such variation indicates significant photosynthesis during the midday hours, the monthly mean diel NEE cycle consistently showed positive fluxes.
3.2.2 CO2 balances
The annual CO2 balances of the first and the second year after clear-cutting were 3086±148 g CO2 m−2 (± uncertainty; see Appendix) and 2072±124 g CO2 m−2, respectively. About half of the annual CO2 emissions during both EC measurement years took place during the summer months (JJA), totalling 1558±99 g CO2 m−2 (Table 2, Fig. 7), and 73 % (2256±146 g CO2 m−2) of the annual total was accumulated during the May–September period. In 2017, however, these emissions decreased to 915±79 g CO2 m−2 (June–August) and 1401±109 g CO2 m−2 (May–September), i.e. by 41 % and 38 %, respectively. The effect of storage fluxes on the CO2 balance was negligible as the daily mean storage flux typically varied within ±0.009 mg CO2 m−2 s−1 during summer and within ±0.004 mg CO2 m−2 s−1 during the other seasons.
Table 2Annual and summertime (JJA) balances of net ecosystem exchange (NEE), modelled gross primary production (GPP), and modelled total ecosystem respiration (Reco), as well as the modelled summertime sums of forest floor respiration (Rff).
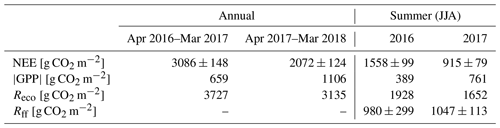
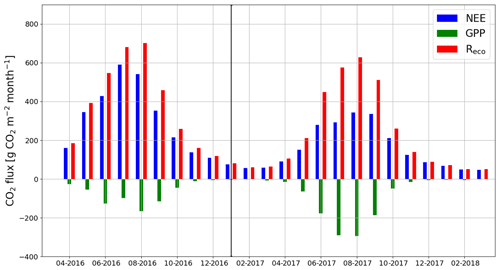
Figure 7The monthly sums of net ecosystem exchange (NEE), gross primary production (GPP) and ecosystem respiration (Reco) based on EC data in April 2016–March 2018.
The most significant period of CO2 uptake was from June to September (Fig. 7). Considering only the summer months (JJA), the integrated GPP was 389 and 761 g CO2 m−2 in 2016 and 2017, respectively (Table 2); i.e. the mean CO2 uptake in the second summer after clear-cutting increased by 96 % from the first summer. On the other hand, the total summertime Reco decreased by 14 %, from 1928 g CO2 m−2 in 2016 to 1652 g CO2 m−2 in 2017 (Table 2).
3.3 Soil GHG fluxes
The summertime (JJA) Rff measured at the soon-to-be clear-cut site in 2015 was 1611±191 g CO2 m−2 (± uncertainty; see Sect. 2.5), and the measured CO2 fluxes varied from 0.04 to 0.36 g CO2 m−2 s−1 (Fig. 8a). The CH4 flux averaged over all the measurement plots was ng CH4 m−2 s−1 before the clear-cutting. All the measured CH4 fluxes were negative (Fig. 8b) ranging from −103 to −2 ng CH4 m−2 s−1. N2O fluxes varied mostly from −17 to 33 ng N2O m−2 s−1 and averaged at 1±5 ng N2O m−2 s−1 (Fig. 8c). At the control site, the summertime fluxes of CO2 (from 0.03 to 0.39 g CO2 m−2 s−1) and N2O (mean: 11±9 ng N2O m−2 s−1) in 2015 were not significantly different from those at the soon-to-be clear-cut site. However, the CH4 fluxes (mean: ng CH4 m−2 s−1) at the control site were significantly (p<0.02) larger than at the soon-to-be clear-cut site.
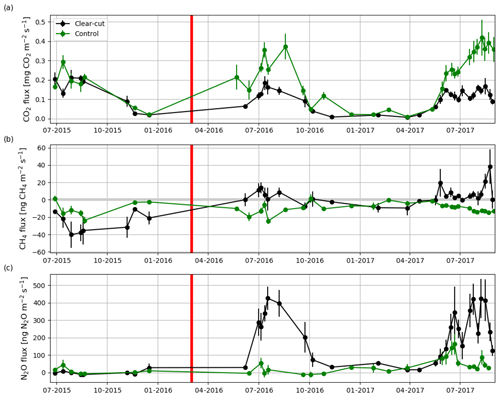
Figure 8Hourly mean fluxes measured with manual chambers of (a) CO2, (b) CH4 and (c) N2O averaged over all measurement points (4, 8, 12 and 22.5 m from the ditch) at the clear-cut (black) and control (green) sites from June 2015 to August 2017. The error bars show the standard error of the mean. The clear-cutting (red vertical line) was carried out in February–March 2016.
After the clear-cutting, the total summertime Rff decreased to 980±299 g CO2 m−2 in summer 2016 and remained at the same level (1047±113 g CO2 m−2) in 2017. These numbers were 51 % and 63 % of the Reco for the summers of 2016 and 2017, respectively (Fig. 9). Also, the temperature response of Rff got weaker, and the R0 parameter (Rff at 10 ∘C) decreased, while at the control site the responses remained quite similar over the years (Fig. 10). The strength of temperature response and R0 partly recovered in 2017 but were still weaker than before the clear-cutting. In contrast to the Rff at the clear-cut, the summertime Rff at the control site increased from 1852±1353 g CO2 m−2 in 2015 to 2280±346 g CO2 m−2 and 2438±225 g CO2 m−2 in 2016 and 2017, respectively. However, the increase was significant only when comparing the summers of 2015 and 2017 (p<0.05). The clear-cut and control sites were significantly different from each other in both summers (p<0.001) after clear-cutting.
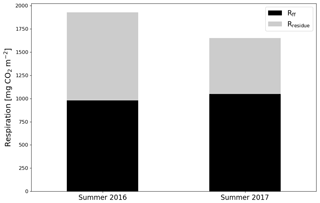
Figure 9The division of ecosystem respiration (Reco, based on EC measurements) to forest floor respiration Rff (chamber measurements) and respiration of logging residues () for the summers of 2016 and 2017.
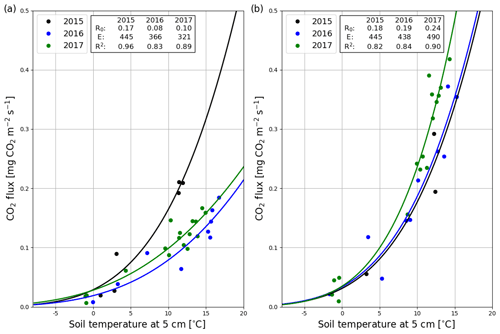
Figure 10Temperature response (Eq. A3) of the hourly mean CO2 fluxes measured with manual chambers at the clear-cut (a) and control (b) site in 2015 (black), 2016 (blue) and 2017 (green).
CH4 fluxes changed markedly after the clear-cutting, as the previously small mean CH4 sink turned into a small CH4 source in the first (4±3 ng CH4 m−2 s−1) and second (6±2 ng CH4 m−2 s−1) year after the clear-cutting (Fig. 8b); the changes were significant for both years (p<0.001). However, the post-clear-cut years were not significantly different from each other (p=0.85). The post-clear-cut fluxes varied mostly from −14 to 28 ng CH4 m−2 s−1, with some occasional emission peaks reaching up to 140 ng CH4 m−2 s−1. Most of the measured fluxes were positive (emission), even though a single measurement plot could act both as a source and a sink on different measurement days. The control site remained as a CH4 sink in 2016 and 2017 and neither of the mean summer fluxes were significantly different from the preharvest summer mean. However, the mean CH4 fluxes were significantly different (p<0.001) between the sites in both post-harvest summers.
Similarly to CH4, the post-clear-cut N2O fluxes were significantly higher than the pre-clear-cut ones (p<0.001), and the post-clear-cut years did not differ significantly from each other. After the clear-cutting, the mean annual fluxes were 228±26 and 212±21 ng N2O m−2 s−1 in 2016 and 2017, respectively (Fig. 8c). All the measurement plots turned to large N2O sources, and the largest measured emission was 1339 ng N2O m−2 s−1. All the measured fluxes were positive, except for a plot furthest from the ditch that acted as a temporary N2O sink in 2017, especially in the June–July period. The N2O fluxes at the control site remained low in summer 2016 (mean: 16±11 ng N2O m−2 s−1) but increased in summer 2017 (mean: 70±10 ng N2O m−2 s−1). However, the post-harvest emissions at the control site were significantly lower than those at the clear-cut site (p<0.001). Both the spatial and temporal variations were large: the largest emissions occurred in June while from July onwards the emissions decreased (Fig. 8c).
3.4 Energy fluxes
The daily sums of sensible heat (QH) were about 1 MJ m−2 d−1 on average at the start of the EC measurement period in April 2016 and increased to a maximum of 4.7 MJ m−2 d−1 in June (Fig. 11). In July, the daily QH sum was already decreasing with the decreasing net radiation (QN) and turned negative in October. On the other hand, the daily sums of latent heat flux (QE) were quite stable from April until August, averaging at 1.0±0.04 MJ m−2 d−1 (± standard error of mean). In September, QE started to decrease and reached zero at the end of October. The ground heat flux (QG) varied within 0–0.5 MJ m−2 d−1 from April to August, after which it varied mostly between −0.5 and 0 MJ m−2 d−1 until the end of April 2017.
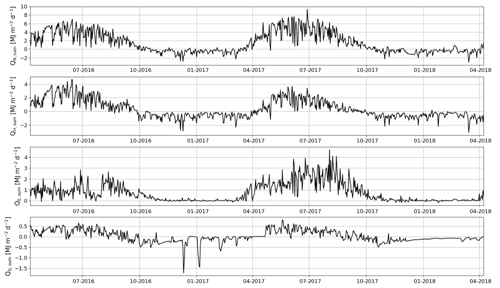
Figure 11Daily sums of net radiation (QN), sensible heat flux (QH), latent heat flux (QE) and ground heat flux (QG).
In 2017, the seasonal dynamics of the energy fluxes were similar to 2016, but the magnitude of fluxes changed considerably. The summertime average daily sum of QH decreased from 1.8 to 1.2 MJ m−2 d−1, while that of QE increased from 1.3 to 2.3 MJ m−2 d−1. Also, the monthly sums of these fluxes were markedly different in the second year after the clear-cutting. During the period of the highest fluxes (April–September), the QH sum was 33 % smaller in 2017 than 2016, while the QE and QN sums were higher by 40 % and 13 %, respectively. The changes in QG were minor.
The monthly mean midday QH in May and June 2016 were 206 and 165 W m−2, respectively (Fig. 12a). After June, the midday QH started to decrease with decreasing QN, and the night-time QH turned from zero to slightly negative. The midday QE also increased from April to June, reaching 88 W m−2, and remained quite stable until September. The resulting monthly mean daytime Bowen ratio (β), increased from 1.7 in April to 2.6 in May 2016 and then declined to 0.8 in August. In 2017, the mean midday QH was similar to that in 2016, except in July and September when the fluxes were 34 % and 23 % lower, respectively, than in 2016 (Fig. 12b). However, the daily maximum QE was either similar to or higher (up to 48 %) than QH in all months except May 2017. Correspondingly, β was lower than 1 (0.6–1.0) during the period of high fluxes in 2017, except in May when it was 1.7.
4.1 Dynamics of the CO2 fluxes and flux components
The study site was a large source of CO2 during the 2 EC measurement years after clear-cutting, but the emissions were 33 % smaller in the second than the first year. remained low and stable during the first summer after clear-cutting, reflecting the fact that neither vascular ground vegetation nor moss cover had developed substantially by that time. However, during the second summer, the total increased by 96 % from the first post-clear-cut summer, and the increase in the coverage of ground vegetation was already noticeable (although not directly measured). This relatively fast recovery of ground vegetation is similar to the leaf area index changes observed by Mäkiranta et al. (2010) after clear-cutting in a nutrient-poor peatland forest. Reco was larger in the first summer than in the second post-harvest year, which could be due to the first year being warmer or due to changes in the respiration of either residues or forest floor in the second year. The chamber measurements, which were not affected by aboveground logging residues, showed a 39 % and 35 % decrease in Rff after clear-cutting in the first and second summer after clear-cutting, respectively. Possible reasons for this decrease include reduced peat decomposition due to raised WTL, ceased autotrophic respiration of (the harvested) trees roots and decreased autotrophic respiration of ground vegetation, even though the latter slightly recovered during the summer as the ground vegetation started to regrow. The CO2 flux from the decomposing below-ground logging residues (roots) was not sufficiently large to compensate for this decrease in Rff. In summer 2016, Rff was 51 % of the Reco, suggesting that half of the Reco during that time originated from the above-ground logging residues, which were not included in the chamber measurements of Rff. In summer 2017, however, Reco decreased by 14 %, but Rff remained about the same, indicating that a larger proportion (63 %) of Reco originated from the forest floor respiration than in the previous summer. This could be due to the decrease in decomposition rate of the aboveground logging residues due to lower temperatures and because the residues had already partly decomposed during the previous summer. However, the lower temperatures would also decrease Rff, but this did not happen because in 2017 WTL was 5 cm lower, which enhanced peat decomposition. In addition, the NEE in summer 2017 was approximately equal to Rff, which means that the increase in was sufficient to balance the CO2 emissions from the logging residues but not from soil and plants.
After the removal of the canopy, more solar radiation reached the ground surface, heating it more during the daytime, while the heat transfer from the soil to the atmosphere was enhanced during the night; together these resulted in a higher diel variation in soil temperature. On the other hand, the insulating impact of logging residues may have partly dampened the amplitude of the diel temperature cycle, as observed by Ojanen et al. (2017). The higher soil temperatures during the daytime may have enhanced peat respiration in the layer closest to the surface. However, it has been shown in previous studies that the temperature response of respiration weakens when the soil gets dry, often resulting in lower respiration rates (Mäkiranta et al., 2009, 2010). Such weakening of the temperature response was also observed at Lettosuo, especially in the first summer after the harvest when the almost bare soil surface was exposed to more solar radiation after clear-cutting. Also, summer 2016 was warmer and drier than on average, which probably enhanced this effect. Moreover, the addition of logging residues may have increased Reco substantially, as demonstrated by Mäkiranta et al. (2012), who observed that the plots with logging residues had a CO2 efflux twice as high as the plots without them. However, a later study by Ojanen et al. (2017) did not show a consistent increase in soil organic matter decomposition rates at similar sites. In previous studies, a decrease has been observed in both Reco (Kowalski et al., 2004, 2003; Takagi et al., 2009) and Rff (Mäkiranta et al., 2010; Takagi et al., 2009; Zerva and Mencuccini, 2005) after clear-cutting in peatland and upland forests. However, also increasing Reco and Rff have also been reported (Kowalski et al., 2004; Londo et al., 1999). The differences between these results are most likely caused by a different effect of the harvest on soil microbial activity, which is mostly controlled by soil temperature, moisture and nutrient availability (Davidson et al., 1998; Fontaine et al., 2004, 2007; Lloyd and Taylor, 1994). Other possible reasons for this variability include differences in, for example, root respiration, the type and amount of logging residue, and WTL, especially in peatland forests.
In peat soils, the decomposition of organic matter is mainly controlled by soil temperature and WTL (Blodau et al., 2004; Mäkiranta et al., 2009; Silvola et al., 1996), but soil temperature is typically the most influential factor for explaining the temporal dynamics in Rff (e.g. Mäkiranta et al., 2008; Ojanen et al., 2010). At Lettosuo, the correlation between the half-hourly Reco and soil temperature was mostly weak. This could be due to logging residues, as their decomposition rate probably does not depend as much on soil temperature as on the temperature and moisture of the logging residues themselves. At times, especially when the temperature range was larger (in May and June), a significant relationship (correlation coefficient peaking at 0.57) was observed, and there was also an obvious temperature response that followed the annual cycle. Also, when WTL is closer ( cm) to the surface, it is usually a significant predictor of Rff in peat soils (Chimner and Cooper, 2003; Riutta et al., 2007). At Lettosuo after clear-cutting, WTL varied mostly within 20–30 cm below the soil surface, and there was no correlation between Reco and WTL after removing the effect of soil temperature on Reco (Fig. S5). Because Reco combines many respiration components in addition to Rff, it is likely that the high CO2 efflux from logging residues, for example, masks the possible WTL effect on Rff.
Our results show that, after clear-cutting, a peatland forest is a large source of CO2 to the atmosphere. Nutrient-rich drained peatland forests with a growing tree stand have generally been found to be CO2 neutral or small sinks of CO2 (Meyer et al., 2013; Ojanen et al., 2013; Uri et al., 2017), as the carbon accumulated by trees typically balances the carbon emissions caused by the decomposing peat layer. This suggests that Lettosuo was probably also close to a CO2-neutral state before the clear-cutting. Thus, the impact of clear-cutting from CO2 neutral to a source of 3086±148 g CO2 m−2 yr−1 in the first year after clear-cutting must be considered substantial. This also means that the site may need to be considered a net CO2 source in terms of the long-term balance even if the forest had been a CO2 sink at a particular growth stage (Hommeltenberg et al., 2014), depending on how long the post-clear-cut emissions continue. In this study, however, we only focus on the immediate impact of clear-cutting.
Mäkiranta et al. (2010) measured CO2 exchange in a clear-cut nutrient-poor peatland forest with soil chambers during a growing season (May–October) and reported slightly smaller NEE (1990 g CO2 m−2 season−1) than the NEE we measured at Lettosuo during a similar timeframe (2340 g CO2 m−2 season−1). Among mineral soil forests, on the other hand, the annual CO2 exchange measured with the EC method right after the clear-cutting varies a lot; the annual balances ranging from ca. 400 to 4700 g CO2 m−2 yr−1 (Amiro et al., 2010; Clark et al., 2004; Humphreys et al., 2005; Kowalski et al., 2004, 2003; Takagi et al., 2009; Williams et al., 2013). If considering only mineral soil forests, the forests in warmer climates typically emit more carbon after the harvest, but they also recover faster than the boreal forests (Amiro et al., 2010). Compared to the studies cited above, the clear-cut site at Lettosuo was the second largest source of CO2 immediately after clear-cutting, being second only to the slash pine plantation in Florida (Clark et al., 2004). The high CO2 emission at Lettosuo as compared to other clear-cut sites on mineral soil is apparently due to the decomposition of the oxic peat layer in this nutrient-rich forest, which are known to act as CO2 sources up to 1000 g CO2 m−2 yr−1 (Ojanen et al., 2013; Uri et al., 2017). Mineral soil forests do not have a peat layer or any other carbon storage that could cause CO2 emissions of such magnitude.
4.2 Soil CH4 and N2O fluxes
The CH4 flux in peat soils is mostly controlled by WTL (Martikainen et al., 1995; Roulet et al., 1993), which divides the peat column into anoxic and oxic layers in which CH4 production and oxidation occur, respectively. After clear-cutting, the WTL rose from −33 cm in 2015 to −22 cm in 2016 during July–August, and the highest estimated rise in WTL occurred in July–October when clear-cutting was estimated to raise WTL by 23 cm on average when compared to the background model. This made the topsoil oxic layer much thinner and the conditions less favourable for CH4 oxidation, allowing a smaller amount of the CH4 produced in the anoxic layer to be oxidized before reaching the atmosphere. The WTL rise was enough to turn the clear-cut site from a CH4 sink into a small CH4 source, while the control site remained a consistent CH4 sink. A similar turn of a clear-cut site from a CH4 sink to a source has previously been found for both peat (Zerva and Mencuccini, 2005) and mineral soils (Sundqvist et al., 2014). However, Huttunen et al. (2003) measured mostly net CH4 uptake in two drained peatland forest sites in southern Finland also after clear-cutting, and the difference between the control and clear-cut sites was not statistically significant in either case.
The N2O fluxes were highly variable both before and after clear-cutting. However, there was a strong increase from the mean pre-clear-cut average flux of 1±5 ng N2O m−2 s−1 to the post-clear-cut flux of 228±26 ng N2O m−2 s−1. On the other hand, the emissions were small at the control site in 2016 but increased in 2017, especially in June, even though the increase was markedly smaller than at the clear-cut site. This is probably due to higher precipitation in 2017, which increased soil moisture and enhanced denitrification. Increases in N2O emissions after clear-cutting have also been observed in peat and mineral soil forests by Huttunen et al. (2003) and Saari et al. (2009), but the fluxes at these sites were an order of magnitude smaller than at Lettosuo. It should be noted that the N2O measurements in this study do not include any above-ground logging residues, which are expected to raise N2O emissions further; Mäkiranta et al. (2012) found N2O emissions about 3 times as large from the plots with logging residues as those from the plots without them.
Compared to the change in NEE (i.e. CO2 fluxes) at the site, the change in CH4 emissions is negligible when considering its climatic impact in terms of the global warming potential over 100 years (GWP100 = 34; IPCC, 2013). Even though the change in N2O fluxes to the present level at our site was large, the climatic effect of N2O was only about 10 % of that due to the even larger change in NEE, when considering GWP over a period of up to 100 years (GWP100 = 298; IPCC, 2013).
4.3 Energy fluxes
The mean daily QE at Lettosuo during the spring and summer right after the clear-cutting varied mostly within 0.5–2.5 MJ m−2 d−1 and within 1.0–4.0 MJ m−2 d−1 during the second spring and summer. Compared to the values of 2–10 MJ m−2 d−1 measured in an upland forest in western Russia (Mamkin et al., 2016), our numbers are low. In the case of QH, the fluxes at Lettosuo were systematically lower than those reported by Mamkin et al. (2016), but the difference was not as large as for QE.
At Lettosuo, β was lower in the second summer after the clear-cutting as compared to the first summer: in summer 2016 β was >1 most of the time, whereas in summer 2017 it was <1 for the whole summer. Thus, the pattern was somewhat different from the results of Mamkin et al. (2016), who reported that β<1 already throughout the first summer after the clear-cutting. One reason for the smaller QE at Lettosuo could be the drier-than-normal summers in 2016 and 2017 and the drier surface layer of the peat.
In summer 2017 at Lettosuo, QH decreased slightly and QE almost doubled compared to 2016, suggesting a recovery of transpiration due to the gradual recovery of ground vegetation. This is in agreement with the results obtained by Williams et al. (2013) for a clear-cut Norway spruce plantation in the northeastern USA, where a recovery of evapotranspiration and a decrease in QH were observed across the years after the clear-cutting. Moreover, the recovered ground vegetation at Lettosuo most likely increased albedo and thus prevented the soil from heating as much as in 2016, resulting in a smaller QH over the whole summer. The clear-cut site at Lettosuo is small, but a larger-scale clear-cutting would probably affect local and regional climate due to changes in surface energy balance fluxes. This is because QH and QE affect the properties and growth rate of the planetary boundary layer, influencing convection and the long-range transport of heat and humidity.
Based on our measurements, we conclude that after clear-cutting a nutrient-rich peatland forest is a large CO2 source. This is due both to the decomposition of peat and logging residues and to the reduction of gross primary production as a result of the removal of photosynthesizing trees and the decline of ground vegetation and understorey. Removing the trees decreased transpiration, which caused the WTL to rise by 23 cm compared to the background model during July–October, which in turn likely decreased the peat decomposition rate due to the decreased volume of aerobic peat. Plant respiration also decreased as the plants were removed or destroyed. On the other hand, decomposition of logging residues increased CO2 emissions from the site; the emissions from the residues were estimated to be 49 % of the total ecosystem respiration in the first summer after clear-cutting. In the second summer, ground vegetation and its primary production recovered noticeably. On the other hand, the CO2 emissions from the logging residues decreased, as part of the residues had decomposed during the previous summer. In total, these changes reduced the net CO2 emissions of the site by 41 % compared to the first summer.
The soil turned from a small CH4 sink into a small source after clear-cutting due to the WTL rise. However, the radiative forcing related to this change was insignificant compared to that due to the change in NEE. Clear-cutting turned the soil into a substantial source of N2O. This change produced a 100-year GWP of about 10 % of that due to the increased NEE at the site.
The mean daytime latent heat flux almost doubled from the first to the second year after clear-cutting, suggesting that the transpiration of ground vegetation had recovered. The recovered ground vegetation most likely also increased albedo and thus prevented the soil from heating as much as in 2016, which resulted in a smaller sensible heat flux.
Overall, the results of this study show that clear-cutting peatland forests exerts a strong climatic warming effect through accelerated emissions of greenhouse gases. However, this study only demonstrates a short-term impact of 2 years, and more extensive measurements are required to gain knowledge of the long-term effects of clear-cutting in peatland forests.
The measured flux and meteorological data are available at Zenodo (https://doi.org/10.5281/zenodo.3384791, Korkiakoski et al., 2019).
To calculate seasonal and annual CO2 balances, full time series are needed and thus any gaps in the CO2 flux data need to be filled. For this reason and to analyse the components of the carbon balance, we partitioned the measured CO2 flux, i.e. net ecosystem exchange (NEE), into gross primary production (GPP) and total ecosystem respiration (Reco):
For gap-filling, we expressed GPP as a function of irradiance (e.g. Aurela et al., 2015):
where PPFD is the photosynthetic photon flux density, α is the initial slope of the NEE response to PPFD and GPmax is the asymptotic gross photosynthesis rate in optimal light conditions. Reco was assumed to follow the Arrhenius-type model described by Lloyd and Taylor (1994):
where R0 is the ecosystem respiration at 10 ∘C, E is the temperature sensitivity of the respiration, Tsoil is the measured soil temperature at 5 cm depth, T0=56.02 K and T1=227.13 K.
The parameters E and R0 were defined from the night-time data (PPFD <20 µmol m−2 s−1) in two parts. First, the parameter E, which was allowed to vary within 200–500 K, was determined with a 15 d moving window for each day, with the minimum number of observations set to 12. If there were not enough observations within a time window, then the window size was increased by 1 d both in the beginning and at the end until enough data were found. The resulting window size varied between 15 and 27 d, but only 22 gap-filled days had a longer window than 15 d. Next, all the E values that hit the allowed boundary values (200 and 500 K) were discarded and filled with 14 d moving medians. Finally, a 15 d moving window, similar to the one in the first part, was used to determine R0 by using the fixed E values. Also, using the same moving window, GPmax and α were determined from the daytime data. However, from 1 November 2016 until 8 March 2017, with no significant CO2 uptake, a 5 d moving average was used to fill the gaps in the measured NEE.
All the calculations and analyses were made with the Python programming language (Python Software Foundation, version 2.7, https://www.python.org, last access: 5 September 2019). For the fits, the least-squares method was used through the “polyfit” function of the NumPy (http://www.numpy.org/, last access: 5 September 2019) library for the linear regression and the “curve_fit” function of the SciPy (http://www.scipy.org/, last access: 5 September 2019) library for the nonlinear fits.
The CO2 balance obtained from EC measurements has multiple potential error sources due to instrumental, statistical and methodological uncertainties. We included the most significant, although not all, random error sources. The random error including the statistical measurement error (Emeas) inherent in EC measurements and the error caused by gap filling of missing data (Egap) was estimated as follows (Räsänen et al., 2017):
where NEEobs is the 30 min flux that passed all the filtering procedures, NEEmod is the corresponding fitted NEE (Eqs. A1–A3), and nmeas∕gap is the number of the measured or gap-filled data. This method provides a conservative estimate for Emeas (Aurela et al., 2002) and for Egap includes the effect of random variability on the model fits.
The annual systematic error caused by the friction velocity filtering (Eustar) was estimated by recalculating the annual balance with modified data sets that were screened with two different u∗ limits (0.075 and 0.175 m s−1). Eustar was calculated as the average difference between the annual balance calculated with the optimal u∗ threshold (0.125 m s−1) and with the annual balances calculated with the modified u∗ limits. Similarly, two additional different footprint limits (0.65 and 0.85) were adopted to estimate the annual error caused by the footprint filtering (Efp).
The total uncertainty of the annual balance (Etot) was calculated with the standard error propagation principle:
Energy fluxes were gap-filled in several steps following the procedure described by Kowalski et al. (2003). First, the gaps in daytime QH (QN>0) were filled with monthly linear regressions with net radiation. Next, the night-time (QN<0) gaps in QH were replaced by the corresponding QN. Finally, the daytime gaps in QE were filled in such a way that the monthly mean energy balance closure was achieved, while during the night the missing QE data were set to 0.
The supplement related to this article is available online at: https://doi.org/10.5194/bg-16-3703-2019-supplement.
TP, PO, KM, TL and AL designed the study. Field measurements and maintenance were carried out by MK, JR, TL and AL. JPT made spectral corrections and footprint analysis for the EC data. SS and PO corrected the WTL data and did the WTL background modelling. The rest of the data analysis was carried out by MK. MK wrote the paper with contributions from all co-authors.
The authors declare that they have no conflict of interest.
We are grateful for the financial support from the Maj and Tor Nessling foundation and from the Ministry of Transport and Communications through the Integrated Carbon Observing System (ICOS) research.
This research has been supported by the Maj ja Tor Nesslingin Säätiö (grant no. 201700450) and by the Ministry of Transport and Communications through the Integrated Carbon Observing System (ICOS) research.
This paper was edited by Frank Hagedorn and reviewed by three anonymous referees.
Amiro, B. D., Barr, A. G., Barr, J. G., Black, T. A., Bracho, R., Brown, M., Chen, J., Clark, K. L., Davis, K. J., Desai, A. R., Dore, S., Engel, V., Fuentes, J. D., Goldstein, A. H., Goulden, M. L., Kolb, T. E., Lavigne, M. B., Law, B. E., Margolis, H. A., Martin, T., McCaughey, J. H., Misson, L., Montes-Helu, M., Noormets, A., Randerson, J. T., Starr, G., and Xiao, J.: Ecosystem carbon dioxide fluxes after disturbance in forests of North America, J. Geophys. Res.-Biogeo., 115, G00K02, https://doi.org/10.1029/2010JG001390, 2010.
Aubinet, M., Vesala, T., and Papale, D. (Eds.): Eddy Covariance: a practical guide to measurement and data analysis, Springer Science & Business Media, 2012.
Aurela, M., Laurila, T., and Tuovinen, J.-P.: Annual CO2 balance of a subarctic fen in northern Europe: Importance of the wintertime efflux, J. Geophys. Res.-Atmos., 107, 1–12, https://doi.org/10.1029/2002JD002055, 2002.
Aurela, M., Lohila, A., Tuovinen, J.-P., Hatakka, J., Penttilä, T., and Laurila, T.: Carbon dioxide and energy flux measurements in four northern-boreal ecosystems at Pallas, Boreal Environ. Res., 20, 455–473, 2015.
Betts, A. K., Desjardins, R. L., and Worth, D.: Impact of agriculture, forest and cloud feedback on the surface energy budget in BOREAS, Agr. Forest Meteorol., 142, 156–169, https://doi.org/10.1016/j.agrformet.2006.08.020, 2007.
Bhuiyan, R., Minkkinen, K., Helmisaari, H.-S., Ojanen, P., Penttilä, T., and Laiho, R.: Estimating fine-root production by tree species and understorey functional groups in two contrasting peatland forests, Plant Soil, 412, 299–316, https://doi.org/10.1007/s11104-016-3070-3, 2017.
Blodau, C., Basiliko, N., and Moore, T.: Carbon turnover in peatland mesocosms exposed to different water table levels, Biogeochemistry, 67, 331–351, https://doi.org/10.1023/B:BIOG.0000015788.30164.e2, 2004.
Chimner, R. A. and Cooper, D. J.: Influence of water table levels on CO2 emissions in a Colorado subalpine fen: An in situ microcosm study, Soil Biol. Biochem., 35, 345–351, https://doi.org/10.1016/S0038-0717(02)00284-5, 2003.
Clark, K. L., Gholz, H. L., and Castro, M. S.: Carbon dynamics along a chronosequence of slash pine plantations in north Florida, Ecol. Appl., 14, 1154–1171, 2004.
Clarke, D. and Rieley, J.: Strategy for Responsible Peatland Management, edited by: Clarke, D. and Rieley, J., International Peat Society, Saarijärvi, Finland, 2010.
Davidson, E., Belk, E., and Boone, R. D.: Soil water content and temperature as independent or confounded factors controlling soil respiration in a temperate mixed hardwood forest, Glob. Change Biol., 4, 217–227, https://doi.org/10.1046/j.1365-2486.1998.00128.x, 1998.
Drzymulska, D.: Peat decomposition – Shaping factors, significance in environmental studies and methods of determination; A literature review, Geologos, 22, 61–69, https://doi.org/10.1515/logos-2016-0005, 2016.
Edwards, N. T. and Ross-Todd, B. M.: Soil Carbon Dynamics in a Mixed Deciduous Forest Following Clear-Cutting with and without Residue Removal, Soil Sci. Soc. Am. J., 47, 1014–1021, https://doi.org/10.2136/sssaj1983.03615995004700050035x, 1983.
Fischlin, A., Midgley, G. F., Price, J., Leemans, R., Gopal, B., Turley, C., Rounsevell, M., Dube, P., Tarazona, J., and Velichko, A.: Ecosystems, their properties, goods, and services, in: Climate Change 2007: Impacts, Adaptation and Vulnerability. Contribution of working group II to the Fourth Assessment Report of the Intergovernmental Panel on Climate Change, edited by: Parry, M. L., Canziani, O. F., Palutikof, J. P., van der Linden, P. J., and Hanson, C. E., Cambridge University Press, Cambridge, available at: http://www.treesearch.fs.fed.us/pubs/33102 (last access: 5 September 2019), 2007.
Foken, T. and Wichura, B.: Tools for quality assessment of surface-based flux measurements, Agr. Forest Meteorol., 78, 83–105, https://doi.org/10.1016/0168-1923(95)02248-1, 1996.
Fontaine, S., Bardoux, G., Abbadie, L., and Mariotti, A.: Carbon input to soil may decrease soil carbon content, Ecol. Lett., 7, 314–320, https://doi.org/10.1111/j.1461-0248.2004.00579.x, 2004.
Fontaine, S., Barot, S., Barré, P., Bdioui, N., Mary, B., and Rumpel, C.: Stability of organic carbon in deep soil layers controlled by fresh carbon supply, Nature, 450, 277–280, https://doi.org/10.1038/nature06275, 2007.
Fredeen, A. L., Waughtal, J. D., and Pypker, T. G.: When do replanted sub-boreal clearcuts become net sinks for CO2?, Forest Ecol. Manage., 239, 210–216, https://doi.org/10.1016/j.foreco.2006.12.011, 2007.
Hommeltenberg, J., Schmid, H. P., Drösler, M., and Werle, P.: Can a bog drained for forestry be a stronger carbon sink than a natural bog forest?, Biogeosciences, 11, 3477–3493, https://doi.org/10.5194/bg-11-3477-2014, 2014.
Howard, E. A., Gower, S. T., Foley, J. A., and Kucharik, C. J.: Effects of logging on carbon dynamics of a jack pine forest in Saskatchewan, Canada, Glob. Change Biol., 10, 1267–1284, https://doi.org/10.1111/j.1529-8817.2003.00804.x, 2004.
Humphreys, E. R., Black, T. A., Morgenstern, K., Li, Z., and Nesic, Z.: Net ecosystem production of a Douglas-fir stand for 3 years following clearcut harvesting, Glob. Change Biol., 11, 450–464, https://doi.org/10.1111/j.1365-2486.2005.00914.x, 2005.
Huttunen, J. T., Nykänen, H., Martikainen, P. J., and Nieminen, M.: Fluxes of nitrous oxide and methane from drained peatlands following forest clear-felling in southern Finland, Plant Soil, 255, 457–462, https://doi.org/10.1023/A:1026035427891, 2003.
IPCC: Climate Change 2013: The Physical Science Basis. Contribution of Working Group I to the Fifth Assessment Report of the Intergovernmental Panel on Climate Change, edited by: Stocker, T. F., Qin, D., Plattner, G.-K., Tignor, M., Allen, S. K., Boschung, J., Nauels, A., and Xia, Y., Cambridge University Press, Cambridge, UK and New York, NY, USA, 2013.
Kaila, A., Sarkkola, S., Laurén, A., Ukonmaanaho, L., Koivusalo, H., Xiao, L., O'Driscoll, C., Asam, Z. U. Z., Tervahauta, A., and Nieminen, M.: Phosphorus export from drained Scots pine mires after clear-felling and bioenergy harvesting, Forest Ecol. Manage., 325, 99–107, https://doi.org/10.1016/j.foreco.2014.03.025, 2014.
Kaimal, J. C. and Finnigan, J. J.: Atmospheric boundary layer flows: their structure and measurement, Oxford University Press, Oxford, 1994.
Kolari, P., Pumpanen, J., Rannik, Ü., Ilvesniemi, H., Hari, P., and Berninger, F.: Carbon balance of different aged Scots pine forests in Southern Finland, Glob. Change Biol., 10, 1106–1119, https://doi.org/10.1111/j.1529-8817.2003.00797.x, 2004.
Korkiakoski, M., Tuovinen, J.-P., Aurela, M., Koskinen, M., Minkkinen, K., Ojanen, P., Penttilä, T., Rainne, J., Laurila, T., and Lohila, A.: Methane exchange at the peatland forest floor – automatic chamber system exposes the dynamics of small fluxes, Biogeosciences, 14, 1947–1967, https://doi.org/10.5194/bg-14-1947-2017, 2017.
Korkiakoski, M., Tuovinen, J.-P., Penttilä, T., Sarkkola, S., Ojanen, P., Minkkinen, K., Rainne, J., Laurila, T., and Lohila, A.: Greenhouse gas and energy fluxes in a boreal peatland forest after clearcutting, Zenodo, https://doi.org/10.5281/zenodo.3384791, 2019.
Kormann, R. and Meixner, F. X.: An analytical footprint model for non-neutral stratification, Bound.-Lay. Meteorol., 99, 207–224, https://doi.org/10.1023/A:1018991015119, 2001.
Kowalski, A. S., Loustau, D., Berbigier, P., Manca, G., Tedeschi, V., Borghetti, M., Valentini, R., Kolari, P., Berninger, F., Rannik, Ü., Hari, P., Rayment, M., Mencuccini, M., Moncrieff, J., and Grace, J.: Paired comparisons of carbon exchange between undisturbed and regenerating stands in four managed forest in Europe, Glob. Change Biol., 10, 1707–1723, https://doi.org/10.1111/j.1365-2486.2004.00846.x, 2004.
Kowalski, S., Sartore, M., Burlett, R., Berbigier, P., and Loustau, D.: The annual carbon budget of a French pine forest (Pinus pinaster) following harvest, Glob. Change Biol., 9, 1051–1065, https://doi.org/10.1046/j.1365-2486.2003.00627.x, 2003.
Laurén, A., Heinonen, J., Koivusalo, H., Sarkkola, S., Tattari, S., Mattsson, T., Ahtiainen, M., Joensuu, S., Kokkonen, T., and Finér, L.: Implications of uncertainty in a pre-treatment dataset when estimating treatment effects in paired catchment studies: Phosphorus loads from forest clear-cuts, Water. Air. Soil Poll., 196, 251–261, https://doi.org/10.1007/s11270-008-9773-1, 2009.
Lloyd, J. and Taylor, J. A.: On the Temperature Dependence of Soil Respiration, Funct. Ecol., 8, 315–323, 1994.
Londo, A. J., Messina, M. G., and Schoenholtz, S. H.: Forest Harvesting Effects on Soil Temperature, Moisture, and Respiration in a Bottomland Hardwood Forest, Soil Sci. Soc. Am. J., 63, 637–644, https://doi.org/10.2136/sssaj1999.03615995006300030029x, 1999.
Machimura, T., Kobayashi, Y., Iwahana, G., Hirano, T., Lopez, L., Fukuda, M., and Fedorov, A. N.: Change of carbon dioxide budget during three years after deforestation in eastern siberian larch forest, J. Agr. Meteorol., 60, 653–656, 2005.
Mäkiranta, P., Minkkinen, K., Hytönen, J., and Laine, J.: Factors causing temporal and spatial variation in heterotrophic and rhizospheric components of soil respiration in afforested organic soil croplands in Finland, Soil Biol. Biochem., 40, 1592–1600, https://doi.org/10.1016/j.soilbio.2008.01.009, 2008.
Mäkiranta, P., Laiho, R., Fritze, H., Hytönen, J., Laine, J., and Minkkinen, K.: Indirect regulation of heterotrophic peat soil respiration by water level via microbial community structure and temperature sensitivity, Soil Biol. Biochem., 41, 695–703, https://doi.org/10.1016/j.soilbio.2009.01.004, 2009.
Mäkiranta, P., Riutta, T., Penttilä, T., and Minkkinen, K.: Dynamics of net ecosystem CO2 exchange and heterotrophic soil respiration following clearfelling in a drained peatland forest, Agr. Forest Meteorol., 150, 1585–1596, https://doi.org/10.1016/j.agrformet.2010.08.010, 2010.
Mäkiranta, P., Laiho, R., Penttilä, T., and Minkkinen, K.: The impact of logging residue on soil GHG fluxes in a drained peatland forest, Soil Biol. Biochem., 48, 1–9, https://doi.org/10.1016/j.soilbio.2012.01.005, 2012.
Maljanen, M., Sigurdsson, B. D., Guðmundsson, J., Óskarsson, H., Huttunen, J. T., and Martikainen, P. J.: Greenhouse gas balances of managed peatlands in the Nordic countries – present knowledge and gaps, Biogeosciences, 7, 2711–2738, https://doi.org/10.5194/bg-7-2711-2010, 2010.
Mamkin, V., Kurbatova, J., Avilov, V., Mukhartova, Y., Krupenko, A., Ivanov, D., Levashova, N., and Olchev, A.: Changes in net ecosystem exchange of CO2 , latent and sensible heat fluxes in a recently clear-cut spruce forest in western Russia: results from an experimental and modeling analysis, Environ. Res. Lett., 11, 125012, https://doi.org/10.1088/1748-9326/aa5189, 2016.
Martikainen, P. J., Nykänen, H., Alm, J., and Silvola, J.: Change in Fluxes of Carbon-Dioxide, Methane and Nitrous-Oxide Due To Forest Drainage of Mire Sites of Different Trophy, Plant Soil, 168, 571–577, https://doi.org/10.1007/bf00029370, 1995.
McMillen, R. T.: An eddy correlation technique with extended applicability to non-simple terrain, Bound.-Lay. Meteorol., 43, 231–245, https://doi.org/10.1007/BF00128405, 1988.
Meyer, A., Tarvainen, L., Nousratpour, A., Björk, R. G., Ernfors, M., Grelle, A., Kasimir Klemedtsson, Å., Lindroth, A., Räntfors, M., Rütting, T., Wallin, G., Weslien, P., and Klemedtsson, L.: A fertile peatland forest does not constitute a major greenhouse gas sink, Biogeosciences, 10, 7739–7758, https://doi.org/10.5194/bg-10-7739-2013, 2013.
Minkkinen, K., Laine, J., and Hökkä, H.: Tree stand development and carbon sequestration in drained peatland stands in Finland – a simulation study, Silva Fenn., 35, 55–69, https://doi.org/10.14214/sf.603, 2001.
Moore, C. J.: Frequency response corrections for eddy correlation systems, Bound.-Lay. Meteor., 37, 17–35, https://doi.org/10.1007/BF00122754, 1986.
Ojanen, P., Minkkinen, K., Alm, J., and Penttilä, T.: Soil-atmosphere CO2, CH4 and N2O fluxes in boreal forestry-drained peatlands, Forest Ecol. Manage., 260, 411–421, https://doi.org/10.1016/j.foreco.2010.04.036, 2010.
Ojanen, P., Minkkinen, K., and Penttilä, T.: The current greenhouse gas impact of forestry-drained boreal peatlands, Forest Ecol. Manage., 289, 201–208, https://doi.org/10.1016/j.foreco.2012.10.008, 2013.
Ojanen, P., Mäkiranta, P., Penttilä, T., and Minkkinen, K.: Do logging residue piles trigger extra decomposition of soil organic matter?, Forest Ecol. Manage., 405, 367–380, https://doi.org/10.1016/j.foreco.2017.09.055, 2017.
Ojanen, P., Minkkinen, K., Alm, J., and Penttilä, T.: Corrigendum to “Soil–atmosphere CO2, CH4 and N2O fluxes in boreal forestry-drained peatlands” [Forest Ecol. Manage., 260, 411–421, https://doi.org/10.1016/j.foreco.2010.04.036], Forest Ecol. Manage., 412, 95–96, https://doi.org/10.1016/j.foreco.2018.01.020, 2018.
Päivänen, J. and Hånell, B.: Peatland ecology and forestry?: a sound approach, University of Helsinki Department of Forest Sciences Publications 3, Helsinki, Finland, 2012.
Pearson, M., Saarinen, M., Minkkinen, K., Silvan, N., and Laine, J.: Short-term impacts of soil preparation on greenhouse gas fluxes: A case study in nutrient-poor, clearcut peatland forest, Forest Ecol. Manage., 283, 10–26, https://doi.org/10.1016/j.foreco.2012.07.011, 2012.
Pirinen, P., Simola, H., Aalto, J., Kaukoranta, J.-P., Karlsson, P., and Ruuhela, R.: Climatological statistics of Finland 1981–2010, Reports 2, Finnish Meteorological Institute, Helsinki, Finland, 2012.
Pypker, T. G. and Fredeen, A. L.: Ecosystem CO2 flux over two growing seasons for a sub-Boreal clearcut 5 and 6 years after harvest, Agr. Forest Meteorol., 114, 15–30, https://doi.org/10.1016/S0168-1923(02)00139-9, 2002.
Rannik, Ü., Vesala, T., and Keskinen, R.: On the damping of temperature fluctuations in a circular tube relevant to the eddy covariance measurement technique, J. Geophys. Res.-Atmos., 102, 12789–12794, 1997.
Rannik, Ü., Altimir, N., Raittila, J., Suni, T., Gaman, A., Hussein, T., Hölttä, T., Lassila, H., Latokartano, M., Lauri, A., Natsheh, A., Petäjä, T., Sorjamaa, R., Ylä-Mella, H., Keronen, P., Berninger, F., Vesala, T., Hari, P., and Kulmala, M.: Fluxes of carbon dioxide and water vapour over Scots pine forest and clearing, Agr. Forest Meteorol., 111, 187–202, https://doi.org/10.1016/S0168-1923(02)00022-9, 2002.
Räsänen, M., Aurela, M., Vakkari, V., Beukes, J. P., Tuovinen, J.-P., Van Zyl, P. G., Josipovic, M., Venter, A. D., Jaars, K., Siebert, S. J., Laurila, T., Rinne, J., and Laakso, L.: Carbon balance of a grazed savanna grassland ecosystem in South Africa, Biogeosciences, 14, 1039–1054, https://doi.org/10.5194/bg-14-1039-2017, 2017.
R Core Team: R: A language and Environment for Statistical Computing, available at: https://www.r-project.org (last access: 5 September 2019), 2018.
Riutta, T., Laine, J., and Tuittila, E.-S.: Sensitivity of CO2 exchange of fen ecosystem components to water level variation, Ecosystems, 10, 718–733, https://doi.org/10.1007/s10021-007-9046-7, 2007.
Roulet, N., Ash, R., Quinton, W., and Moore, T.: Methane flux from drained northern peatlands: Effect of a persistent water table lowering on flux, Global Biogeochem. Cy., 7, 749–769, 1993.
Saari, P., Saarnio, S., Kukkonen, J. V. K., Akkanen, J., Heinonen, J., Saari, V., and Alm, J.: DOC and N2O dynamics in upland and peatland forest soils after clear-cutting and soil preparation, Biogeochemistry, 94, 217–231, https://doi.org/10.1007/s10533-009-9320-1, 2009.
Sarkkola, S., Hökkä, H., Koivusalo, H., Nieminen, M., Ahti, E., Päivänen, J., and Laine, J.: Role of tree stand evapotranspiration in maintaining satisfactory drainage conditions in drained peatlands, Can. J. For. Res., 40, 1485–1496, https://doi.org/10.1139/X10-084, 2010.
Schulze, E.-D., Lloyd, J., Kelliher, F. M., Wirth, C., Rebmann, C., Luhker, B., Mund, M., Knohl, A., Milyukova, I. M., Schulze, W., Ziegler, W., Varlagin, A. B., Sogachev, A. F., Valentini, R., Dore, S., Grigoriev, S., Kolle, O., Panfyorov, M. I., Tchebakova, N., and Vygodskaya, N. N.: Productivity of forests in the eurosiberian boreal region and their potential to act as a carbon sink – a synthesis, Glob. Change Biol., 5, 703–722, https://doi.org/10.1046/j.1365-2486.1999.00266.x, 1999.
Silvola, J., Alm, J., Ahlholm, U., Nykänen, H., and Martikainen, P. J.: CO2 Fluxes from Peat in Boreal Mires under Varying Temperature and Moisture Conditions, J. Ecol., 84, 219–228, 1996.
Sundqvist, E., Vestin, P., Crill, P., Persson, T., and Lindroth, A.: Short-term effects of thinning, clear-cutting and stump harvesting on methane exchange in a boreal forest, Biogeosciences, 11, 6095–6105, https://doi.org/10.5194/bg-11-6095-2014, 2014.
Takagi, K., Fukuzawa, K., Liang, N., Kayama, M., Nomura, M., Hojyo, H., Sugata, S., Shibata, H., Fukazawa, T., Takahashi, Y., Nakaji, T., Oguma, H., Mano, M., Akibayashi, Y., Murayama, T., Koike, T., Sasa, K., and Fujinuma, Y.: Change in CO2 balance under a series of forestry activities in a cool-temperate mixed forest with dense undergrowth, Glob. Change Biol., 15, 1275–1288, https://doi.org/10.1111/j.1365-2486.2008.01795.x, 2009.
Turunen, J., Tomppo, E., Tolonen, K., and Reinikainen, A.: Estimating carbon accumulation rates of undrained mires in Finland–application to boreal and subarctic regions, Holocene, 12, 69–80, https://doi.org/10.1191/0959683602hl522rp, 2002.
Uri, V., Kukumägi, M., Aosaar, J., Varik, M., Becker, H., Morozov, G., and Karoles, K.: Ecosystems carbon budgets of differently aged downy birch stands growing on well-drained peatlands, Forest Ecol. Manage., 399, 82–93, https://doi.org/10.1016/j.foreco.2017.05.023, 2017.
Webb, E. K., Pearman, G. I., and Leuning, R.: Correction of flux measurements for density effects due to heat and water vapour transfer, Q. J. Roy. Meteor. Soc., 106, 85–100, https://doi.org/10.1002/qj.49710644707, 1980.
Williams, C., Vanderhoof, M., Khomik, M., and Ghimire, B.: Post-clearcut dynamics of carbon, water and energy exchanges in a midlatitude temperate, deciduous broadleaf forest environment, Glob. Change Biol., 20, 992–1007, https://doi.org/10.1111/gcb.12388, 2013.
Yu, Z.: Holocene carbon flux histories of the world's peatlands: Global carbon-cycle implications, Holocene, 21, 761–774, https://doi.org/10.1177/0959683610386982, 2011.
Zerva, A. and Mencuccini, M.: Short-term effects of clearfelling on soil CO2, CH4, and N2O fluxes in a Sitka spruce plantation, Soil Biol. Biochem., 37, 2025–2036, https://doi.org/10.1016/j.soilbio.2005.03.004, 2005.
- Abstract
- Introduction
- Materials and methods
- Results
- Discussion
- Conclusions
- Data availability
- Appendix A: Gap-filling CO2 data
- Appendix B: Uncertainty analysis of NEE
- Appendix C: Gap-filling energy fluxes
- Author contributions
- Competing interests
- Acknowledgements
- Financial support
- Review statement
- References
- Supplement
- Abstract
- Introduction
- Materials and methods
- Results
- Discussion
- Conclusions
- Data availability
- Appendix A: Gap-filling CO2 data
- Appendix B: Uncertainty analysis of NEE
- Appendix C: Gap-filling energy fluxes
- Author contributions
- Competing interests
- Acknowledgements
- Financial support
- Review statement
- References
- Supplement