the Creative Commons Attribution 4.0 License.
the Creative Commons Attribution 4.0 License.
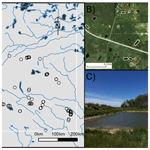
Regulation of carbon dioxide and methane in small agricultural reservoirs: optimizing potential for greenhouse gas uptake
Jackie R. Webb
Peter R. Leavitt
Gavin L. Simpson
Helen M. Baulch
Heather A. Haig
Kyle R. Hodder
Kerri Finlay
Small farm reservoirs are abundant in many agricultural regions across the globe and have the potential to be large contributing sources of carbon dioxide (CO2) and methane (CH4) to agricultural landscapes. Compared to natural ponds, these artificial waterbodies remain overlooked in both agricultural greenhouse gas (GHG) inventories and inland water global carbon (C) budgets. Improved understanding of the environmental controls of C emissions from farm reservoirs is required to address and manage their potential importance in agricultural GHG budgets. Here, we conducted a regional-scale survey (∼ 235 000 km2) to measure CO2 and CH4 surface concentrations and diffusive fluxes across 101 small farm reservoirs in Canada's largest agricultural area. A combination of abiotic, biotic, hydromorphologic, and landscape variables were modelled using generalized additive models (GAMs) to identify regulatory mechanisms. We found that CO2 concentration was estimated by a combination of internal metabolism and groundwater-derived alkalinity (66.5 % deviance explained), while multiple lines of evidence support a positive association between eutrophication and CH4 production (74.1 % deviance explained). Fluxes ranged from −21 to 466 and 0.14 to 92 mmol m−2 d−1 for CO2 and CH4, respectively, with CH4 contributing an average of 74 % of CO2-equivalent (CO2-e) emissions based on a 100-year radiative forcing. Approximately 8 % of farm reservoirs were found to be net CO2-e sinks. From our models, we show that the GHG impact of farm reservoirs can be greatly minimized with overall improvements in water quality and consideration to position and hydrology within the landscape.
- Article
(3658 KB) -
Supplement
(985 KB) - BibTeX
- EndNote
The expansion of agriculture and urban land use has introduced a new type of lentic system that remains relatively unexplored – small artificial waterbodies (Clifford and Heffernan, 2018). These artificial aquatic systems have been created through human modification of the hydrological landscape and include small farm reservoirs and urban ponds. Farm reservoirs are earthen excavations designed to store water for later use (BC Ministry of Agriculture, 2013). The global abundance of these systems remains uncertain (Verpoorter et al., 2014), but statistical extrapolation suggest there may be around 16 million artificial reservoirs worldwide (Lehner et al., 2011). Regional-scale inventories indicate that collectively upwards of 8 million farm reservoirs exist in the USA (Brunson, 1999; Smith et al., 2002), China (Chen et al., 2019), India (Anbumozhi et al., 2001), South Africa (Mantel et al., 2017), and Australia alone (Lowe et al., 2005; MDBA, 2008; Grinham et al., 2018a). The density of farm reservoirs can exceed 30 % of agricultural area in some regions such as China where food demand is high (Chen et al., 2019). Small agricultural reservoirs are estimated to cover 77 000 km2 globally and are being created at rates up to 60 % of existing reservoirs per annum in some regions (Downing et al., 2008). Given their abundance, these artificial systems may contribute substantially to landscape biogeochemical cycles, including fluxes of GHG. In particular, very little is known of the capability of these systems to act as GHG sinks to partially offset the otherwise strong carbon efflux associated with intensive agriculture (Robertson et al., 2000).
Small waterbodies have recently been recognized as substantial contributors to global carbon emissions from inland waters. Current assessments estimate that diffusive CO2 and CH4 emissions from small ponds (<0.001 km2) account for 15 % and 40 % of global emissions from lakes, respectively (Holgerson and Raymond, 2016). Other estimates suggest emissions from small lakes and impoundments (0.001 to 0.01 km2) could constitute 40 % of global CO2 emissions and 20 % of global CH4 emissions from lentic ecosystems (DelSontro et al., 2018). Extreme CO2 and CH4 supersaturation is characteristic of small waterbodies due to greater contact with the sediment and littoral zone (Downing et al., 2008; Holgerson, 2015), often making them disproportionately important in landscape carbon (C) budgets (Hamilton et al., 1994; Premke et al., 2016; Kuhn et al., 2018). Conversely, ponds may have the capacity to store landscape-significant amounts of carbon, with burial rates 20–30 times higher than wetlands and large lakes (Gilbert et al., 2014; Taylor et al., 2019). While these assessments have stimulated a growing area of research on small waterbodies, much work is still needed to revise estimates of their carbon emissions due to limited knowledge on their regional distribution and variability, as well as their overall global extent (Verpoorter et al., 2014). This is particularly true for greenhouse gas (GHG) emissions from human-created small waterbodies.
Understanding the controls and rates of carbon fluxes from small artificial waterbodies is the first step required to understand their landscape and eventually global importance. Further, estimates of CO2 and CH4 flux are complicated by high variation among reservoirs and regions in the importance of groundwater, littoral macrophytes, and local land use practises (Pennock et al., 2010; Badiou et al., 2019). Artificial reservoirs have the potential to be potent sources of CO2 and CH4 (Downing et al., 2008; Holgerson and Raymond, 2016). This role can be demonstrated by a carbon budget estimate from an urban pond where carbon emissions (both diffusive and ebullitive for CH4) offset carbon burial by >1000 % (van Bergen et al., 2019). The recent 2019 IPCC Refinement has assigned a CH4 emission factor of 183 kg ha−1 yr−1 to constructed waterbodies; however, data are greatly limited, both geographically and in number (n=68), such that climatic-zone emission factors cannot be estimated (IPCC, 2019). Currently, only three studies have assessed C fluxes from small agricultural reservoirs at regional scales, and these support the notion that they are important landscape sources of GHGs (Panneer Selvam et al., 2014; Grinham et al., 2018a; Ollivier et al., 2019). All studies found large fractions of CH4 being released and large mean CO2 emissions on the order of 24 and 99 mmol m−2 d−1, comparable to the global average flux rate of very small natural ponds (35 mmol m−2 d−1, Holgerson and Raymond, 2016). However, carbon fluxes from farm reservoirs remain unaccounted for in agricultural GHG inventories and global inland water carbon budgets. To facilitate their inclusion in agricultural and global budgets, we need to further constrain flux rates and mechanisms across a broad geographic area.
Here, we present a large-scale assessment of CO2 and CH4 concentrations from small farm reservoirs in the Northern Great Plains, the largest agricultural region in Canada. This study builds on from our previous farm reservoir GHG research which found an unexpected nitrous oxide (N2O) sink in 67 % of reservoirs (Webb et al., 2019). The hydroclimate, lithology and edaphic features are vastly different compared to previous studies of agricultural areas (Australia, India, USA), with factors that favour CO2 uptake by alkaline surface waters (Finlay et al., 2009, 2015) and lead to high variability in CH4 fluxes from regional wetlands (Pennock et al., 2010; Badiou et al., 2019). Our aim was to identify the key environmental conditions regulating CO2 and CH4 fluxes, as well as to evaluate these baseline data in the context of emission mitigation strategies. To achieve this goal, we carried out an extensive survey of CO2 and CH4 concentrations across 101 farm reservoirs and used generalized additive models (GAMs) to assess the effects of abiotic, biotic, hydromorphological, and land use properties. Our findings show that farm dams were not always strong sources of carbon emissions and in certain cases can be carbon neutral or sinks in terms of CO2-equivalent (CO2-e) emissions. By identifying the driving characteristics of farm dams that support reduced C emissions, our findings provide the first step to developing management strategies to help minimize farm carbon emissions.
2.1 Study site
Farm sites were surveyed across the agricultural region of Saskatchewan, Canada (Fig. 1). This region covers an area of 235 000 km2 in the southern half of the province, where agriculture accounts for ∼80 % of land use. The region has a sub-humid to semi-arid climate (Köppen Dfb classification), with short warm summers (∼18 ∘C) and long winters ( ∘C) resulting in 4.5 to 5.5 months of ice cover on surface waters (Finlay et al., 2015). Average annual precipitation in the area ranges from 354 to 432 mm.
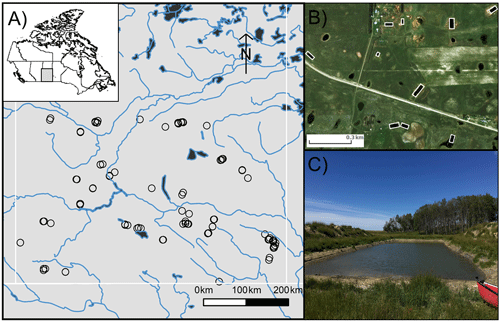
Figure 1(a) Map of southern Saskatchewan in Canada showing the distribution of studied farm reservoirs, (b) aerial image showing 10 farm reservoirs delineated by white rectangles within a 1 km2 area, and (c) general size and shape of farm reservoirs with two characteristic side mounds of excavated materials.
Small farm reservoirs (known locally as “dugouts”) are a prominent feature of the landscape, with densities of up to 10 km−2 (Fig. 1b). Up until 1985, over 110 000 farm reservoirs had been constructed in Saskatchewan (Gan, 2000), although subsequent densities are unknown. We sampled 101 farm reservoirs between July and August 2017, ranging in surface area from 158 to 13 900 m2 (Table 1), including basins in pasture (n=18), pastures with livestock (n=62), and cropland (n=21) sites. Each site was sampled once during this period, between the daylight hours of 10:00 and 15:00 Central Standard Time (CST; or GMT−6). Saskatchewan farm reservoirs are typically uniform in shape and morphometry, dug to a depth of 4 to 6 m with steep sides (1.5 : 1 slopes). Most shallow wetlands and lakes in the region exhibit water balances dominated by evaporation and limited inflow from winter precipitation or groundwater (Conly and van der Kamp, 2001; Pham et al., 2009). Farm reservoirs differ from small natural waterbodies in that they have a higher ratio of water volume to surface area, designed to minimize evaporation losses. Despite this feature, arid conditions persisted during the sampling year, with reduced (34 %–65 %) annual rainfall such that many reservoirs were only half their designed depth. Natural waterbodies also tend to be high-pH hard-water systems, owing to the soils which consist of glacial till high in carbonates (Last and Ginn, 2005). The same was observed for the majority of farm reservoirs, with an average pH of 8.75 (Table 1).
2.2 CO2 and CH4 measurements
Dissolved gas samples were collected using the in-field headspace extraction method (Webb et al., 2019). Briefly, water was collected from ∼30 cm below the surface using a submersible pump which filled a 1.2 L glass-serum bottle, ensuring the bottle overflowed and no air bubbles were present. The bottle was sealed with a rubber stopper fitted with two three-way stopcock valves. Using two 60 mL airtight syringes, atmospheric air was added to the bottle whilst simultaneously extracting 60 mL of water. The bottle was then shaken for 2 min to ensure gas equilibration in the headspace. Two analytical replicates were extracted and stored in 12 mL evacuated Exetainer vials with double-wadded caps. Headspace concentrations of CO2 and CH4 were measured using gas chromatography with a Scion 456 gas chromatograph (Bruker Ltd.) and calculated using standard curves. Dry molar fractions were corrected for dilution and converted to concentrations according to solubility coefficients (Weiss, 1974; Yamamoto et al., 1976).
To compare with the literature and assess the source/sink behaviour of the reservoirs, diffusive fluxes of carbon dioxide and methane fluxes were estimated for each waterbody. Given that the focus of the study was to investigate drivers of CO2 and CH4 concentrations across farm reservoirs, ebullition events were not measured during this survey and as such total CH4 fluxes are likely underestimated. Diffusive fluxes were estimated using water-column concentrations (Cwater) and average farm reservoir gas transfer velocity (kc) using the following equation:
where fC is the flux of CO2 or CH4 (mmol m−2 d−1) and Cair is the ambient air concentration. The average global mixing ratios for the sampling period of 406 and 1.85 µatm were used for ambient concentrations for CO2 and CH4, respectively (Mauna Loa NOAA station, June to August 2017). Site-specific gas transfer velocity (kc) was determined from 30 individual floating-chamber (area = 0.23 m2, volume = 0.046 m3) measurements carried out on a subset of 10 reservoirs. During each 10 min deployment, changes in gas concentrations were measured at 2.5 min intervals by taking samples using syringes and dispensing gases into pre-evacuated 12 mL vials. The flux (mmol m−2 d−1) was calculated from the observed rate of change in the dry mole fraction of the respective gas (Lorke et al., 2015). The gas transfer velocity normalized to a Schmidt number of 600 (k600) for each respective gas was then determined using measured flux, in situ gas concentrations, atmospheric concentration, Henry's constant, and Schmidt numbers, assuming a Schmidt exponent of 0.67. The average k600 calculated from the floating-chamber deployments was 1.50±1.34 and 1.64±1.14 m d−1 for CO2 and CH4, respectively (Table 1).
For comparing CO2-equivalent fluxes, CH4 fluxes were converted using the 100-year sustained-flux global warming potential (SGWP, Neubauer and Megonigal, 2015). This metric offers a more attainable measure of ecosystem climatic forcing, assuming gas flux persists over time instead of occurring as a single pulse as quantified using traditional global warming potentials (GWP, Myhre et al., 2013). Here, a SGWP multiplier of 45 was applied to all CH4 fluxes in the literature comparison, which is slightly higher than the traditional GWP of 32 over a 100-year time frame (Myhre et al., 2013).
2.3 Abiotic and biotic variables
A range of abiotic and biotic parameters were measured at each site. Water quality variables including temperature (∘C), pH, dissolved O2 (DO; % saturation), conductivity (µS cm−2), and salinity were measured at 0.5 m intervals from the surface to the bottom using a YSI (Yellow Springs Instruments, OH, USA) multi-probe meter. Surface (0.5 m) samples for water chemistry were collected using a submersible pump. Upon collection, samples for dissolved nitrogen (NO3+NO2, NH4, total dissolved N; µg N L−1), soluble reactive phosphorus (SRP; µg P L−1) and total dissolved P (TDP; µg P L−1), dissolved organic and inorganic carbon (DOC, DIC; mg C L−1), alkalinity (; mg L−1 as CaCO3), and water isotopes (δ2H, δ18O; ‰) were filtered through a 0.45 µm pore membrane filter. Nutrient and dissolved carbon samples were stored in a dark bottle at 4 ∘C until analysis. Chlorophyll a (Chl a) samples were collected on GF/C glass-fibre filters (nominal pore size 1.2 µm) and frozen (−10 ∘C) until analysis. Sediment samples were collected at the centre of each reservoir, with the uppermost 10 cm using an Ekman grab sampler, and were frozen at −10 ∘C until analysis.
Most analyses were carried out at the University of Regina Institute of Environmental Change and Society (IECS). Water nutrient and dissolved carbon concentrations were measured on a Lachat QuikChem 8500 and Shimadzu model 5000A total carbon analyzer, following standard analytical procedures, respectively (Patoine et al., 2006; Finlay et al., 2009). Alkalinity was measured using standard methods of the US Environmental Protection Agency (EPA) on a SmartChem 200 Discrete Analyzer (WestCo) and estimated as the concentration of CaCO3 (EPA, 1974). Chl a was analysed using standard trichromatic methods (Finlay et al., 2009). The total carbon and nitrogen content (% dry weight) of freeze-dried sediment samples were determined on a NC2500 Elemental Analyzer (ThermoQuest, CE Instruments).
2.4 Hydromorphology
Morphometric parameters of reservoirs were estimated for each site. The depth of each farm reservoir was measured during using a portable ultrasonic depth sounder, taken at the deepest section in the centre of the reservoir. Surface area was determined using Google Earth satellite imagery. Reservoir volume was calculated using the formula for a prismoid by assuming that all sites maintained their original shape, including slopes of 1.5 : 1 ratio (Andresen et al., 2015). From these measurements, an Index of Basin Permanence (IBP) was calculated (Kerekes, 1977).
The degree of water-column mixing or vertical stratification was determined by calculating the squared Brunt–Väisälä buoyancy frequency (N2, s−2). The strongest density gradient was calculated based on vertical temperature measurements at 0.5 m depth intervals using the package rLakeAnalyzer (Read et al., 2012) in R (version 3.5.2; R Core Team, 2018).
The hydrology of farm reservoirs was estimated through analysis of δ18O and δ2H isotope values of water. Samples were collected from 0.5 m below the surface, filtered (0.45 µm pore) and stored in amber borosilicate jars at 4 ∘C until analysis using a Picarro L2120-I cavity ring-down spectrometer (CRDS). Hydrological parameters, including evaporation-to-inflow ratio (E∕I), residence time (years), and inflow volume (m3), deuterium (2H) excess (d-excess), and δ18O inflow (δI) values, were calculated using the coupled isotope tracer method (Yi et al., 2008) and conventional isotopic water-balance methods (Gibson et al., 2001). All methods assumed that reservoirs were headwater systems in a hydrological steady state (Yi et al., 2008). Model inputs included information about the local meteoric water line (LMWL), the trajectory of evaporation along a local evaporative line (LEL), and regional meteorological conditions. From here, the water mass balance of a given waterbody can be quantified based on its relative position along the LEL (Gibson et al., 2001).
Briefly, the isotopic inflow values were estimated by the intercept between the LMWL and site-specific LEL as determined by the δ18O evaporation value (δE) and δ18O reservoir water value at each site (Yi et al., 2008). The E∕I ratio was calculated by using headwater isotopic models of the water mass balance (). Hydrologic residence time was estimated from the reservoir volume and the water isotopic values of waterbodies, inflow, and evaporation. Deuterium excess (d-excess ‰ = ) was calculated as an additional indicator of evaporation losses, where lower values ( ‰) indicate isotopic enrichment from precipitation (Brooks et al., 2014).
2.5 Landscape properties
Landscape soil data were obtained from the National Soil DataBase, Government of Canada (http://sis.agr.gc.ca/cansis/nsdb/dss/v3/index.html, last access: 31 October 2019), using ArcGIS to extract the soil attributes at each site. Extracted variables included soil salinity; soil pH; soil organic carbon content; saturated hydraulic conductivity (Ksat); cation exchange capacity (CEC); and the total composition of soil from sand, silt, and clay fractions (%). Reservoir elevation (m a.s.l.) was determined using ArcGIS and the Canadian Digital Elevation Model (CDEM, v1.1). Local land use in the immediate area surrounding each reservoir was categorized into three types based on local observations at the time of sampling. Categories included pasture land used for either livestock grazing or hay harvesting, pasture where livestock have direct access to the waterbody, and crop fields.
2.6 Statistical analyses
Environmental variables were selected based on known or presumed influence on CO2 and CH4 concentrations in lakes and small waterbodies. Both biotic and abiotic predictors that influence production or consumption of CO2 and CH4 were selected, including DO, alkalinity, NOx (NO2+NO3), NH4, dissolved inorganic nitrogen (DIN), total dissolved nitrogen (TDN), TDP, Chl a, DOC, conductivity, pH, and sediment organic C:N ratio. The influence of reservoir hydrology and morphology was also examined, including measures of surface area, basin permanence, hydrologic regime (E∕I), water source (δI), and degree of mixing (or stratification). Finally, potential effects of the surrounding terrestrial landscape were estimated in models using soil properties, elevation, and land use practises to account for any localized landscape drivers. Before testing relationships, all predictors were transformed as needed using either log10 or square root to remove skewness.
The relationships between covariates and CO2 and CH4 were estimated using generalized additive models (GAMs). GAMs provide an ideal approach to model non-linear associations between predictor variables and responses, using the sum of unspecified smooth functions to estimate trends. GAMs are not constrained by prescribed assumptions associated with parametric models such as linearity of link-scale effects in generalized linear models. Instead, the functional form of the partial relationships between covariates and the response is determined from the data. The more flexible modelling approach is useful where the effects of covariates on the response are non-linear and has been applied to complex aquatic datasets assessing GHGs (Wiik et al., 2018; Webb et al., 2019). GAMs were developed with a gamma distribution for the response and the log link function. Each model included covariates that represented hydromorphological, abiotic and biotic, and landscape controls. To avoid multicollinearity, correlation coefficients and statistical significance (p<0.05) between pairs from Pearson linear correlation tests were used to guide covariate choice before model fitting (Tables S1–S3 in the Supplement). Candidate variables were then selected for each model to test which variables best estimate variability in CO2 and CH4 concentrations. All model coefficients were estimated using restricted marginal likelihood with the mgcv package (Wood, 2011; Wood et al., 2016) for R (version 3.5.2; R Core Team, 2018).
The region experienced a drier-than-average year during sampling, with recorded average annual precipitation ∼ 60 % less than the long-term climate average of 390 mm in Regina, Saskatchewan (Government of Canada, https://weather.gc.ca, last access: 31 October 2019). Consequently, while most farm reservoirs were constructed to ∼5 m depth the mean water-column depth was 2.1 m (0.2–5.1, Table 1). Despite this, isotopic analysis of water revealed that 93 % of waterbodies exhibited an , suggesting that reservoirs were gaining more water than was lost via evaporation. In general, water residence time (WRT) was ∼8 months, although the range in this value was large (29 d to 2.5 years). Estimates of inflow δ18O (δI) indicated variable water sources, with 79 % derived from rain ( ‰), 6 % from snowmelt or groundwater ( ‰), and 15 % intermediate between sources (−17.9 ‰ to −15.6 ‰).
Carbon dioxide and methane concentrations spanned 3 orders of magnitude across surveyed reservoirs, with concentrations ranging between 1.3 and 326.1 µM and between 0.1 and 54.5 µM for CO2 and CH4, respectively (Fig. 2). Most waterbodies were alkaline, with a mean pH of 8.8 (7.0 to 10.2) and carbonate alkalinity between 71 and 755 mg L−1 (Table 1). Many waters were highly eutrophic, with means for Chl a of 99 µg L−1 (range 2 to 344 µg L−1), total nitrogen of >3000 µg N L−1 (418 to 14 280), and total phosphorus of 285 µg P L−1 (9 to 648). Dissolved O2 in the surface layer varied by 3 orders of magnitude among basins with 32 % exhibiting oversaturation (>100 %).
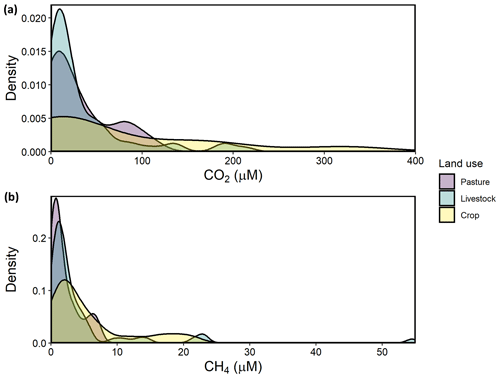
Figure 2Kernel density estimates of CO2 and CH4 concentrations measured in 101 farm reservoirs grouped by land use.
3.1 Models
Regional variation in CO2 concentrations were best estimated in a GAM including pH alone, with 86.3 % of deviance explained and a strongly declining CO2 at pH above 8 (Fig. S1 in the Supplement). Exclusive of the model with pH, the detailed mechanistic GAM for estimating CO2 concentrations across farm reservoirs included a combination of DO saturation, alkalinity, NOx, thermal stratification (buoyancy frequency), basin hydrology (the interaction between δI and WRT), and landscape features (soil CEC, elevation, soil salinity) (Fig. 3). Overall, the model explained 66.5 % of deviance in CO2 concentrations (Table S4, Fig. S2). All covariates had a significant effect except soil salinity, with DO, alkalinity, and the interaction between δI and WRT being the strongest predictors (p<0.001). CO2 concentrations displayed a positive response with increasing alkalinity, NOx, buoyancy frequency, and soil CEC, with a generally negative response to increasing DO and elevation. The effect of DO on CO2 was particularly distinct between 25 %–100 % O2 saturation (Fig. 3a). The interactive effect of hydrology parameters suggests that sites with elevated rain inflows ( ‰) and longer WRT will exhibit undersaturated CO2 concentrations.
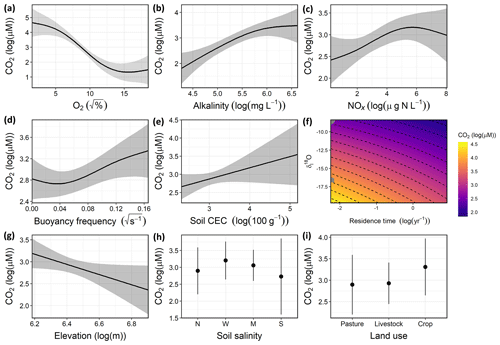
Figure 3Response patterns of farm reservoir CO2 concentrations with abiotic, biotic, hydromorphological, and landscape variables based on GAMs. CO2 was best estimated by a combination of (a) DO saturation, (b) alkalinity, (c) NOx, (d) buoyancy frequency, (e) interaction between δI and WRT, (f) soil CEC, (g) and elevation, with soil salinity (non-saline, N; weakly saline, W; moderately saline, M; strongly saline, S) (h) and land use (i) not significant. Model deviance explained was 66.5 %. The response patterns shown are the partial effect splines from the GAM (solid line), and the shaded area indicates 95 % credible intervals. See Table S4 and Fig. S2 for summary of model statistics and model fit with observed data.
Variation in CH4 concentrations among waterbodies was explained by a combination of DO saturation, sediment C∕N ratio, DIN, conductivity, the interaction between δI and WRT, and local land use (Fig. 4), with buoyancy frequency, soil Ksat, and elevation not significant. Overall, the GAM explained 74.1 % of the deviance in CH4 (Table S5, Fig. S3). Concentrations of CH4 increased with sediment C∕N and DIN and decreased with conductivity. The significant unimodal relationship with DO indicates that the highest observed CH4 concentrations occurred under both anoxic and supersaturated O2 environments (Fig. 4a), while low CH4 levels were seen when inflow was more composed of snowmelt or groundwater (depleted isotope values) and WRT was long (Fig. 4f). In contrast to the CO2 model, soil properties and elevation were not significant drivers, yet local land use was significant, with crop sites having significantly higher CH4 compared to pastures.
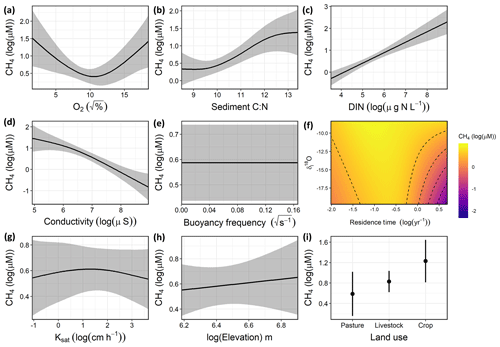
Figure 4Response patterns of farm reservoir CH4 concentrations with abiotic, biotic, hydromorphological, and landscape variables based on generalized additive models (GAMs). CH4 was explained by a combination of (a) DO saturation, (b) sediment C∕N, (c) DIN, (d) conductivity, (e) buoyancy frequency (not significant), (f) interaction between δI and WRT, (g) soil Ksat (not significant), (h) elevation (not significant), and (i) local land use. Model deviance explained was 74.1 %. The response patterns shown are the partial effect splines from the GAM (solid line), and the shaded area indicates 95 % credible intervals. See Table S5 and Fig. S3 for summary of model statistics and model fit with observed data.
Our comprehensive spatial analysis revealed wide variations among CO2 and CH4 concentrations between farm reservoirs (Fig. 2). Significant modelled environmental drivers suggested CO2 was primarily controlled by pH, with strong independent models indicating mechanisms associated with primary productivity, the hydrological regime, and landscape elevation. In contrast, CH4 was most correlated with internal abiotic and biotic mechanisms. We discuss these potential drivers in detail and from our evidence suggest management strategies that may help reduce the net GHG effect of these farm reservoirs.
4.1 Environmental drivers of CO2 concentrations
As seen in other hard-water ecosystems, variations in CO2 were strongly coupled to differences among sites in water-column pH (Finlay et al., 2015; Müller et al., 2016). We demonstrate this relation with the strong correlation observed between CO2 and pH in a separate GAM of only water pH as a covariate, explaining 86.3 % of deviance (Fig. S1). As expected, the role of pH in regulating CO2 content is most pronounced at values between 8.6 and 9.0, the transition point where the predominant species of DIC shifts from free CO2 to (Duarte et al., 2008; Finlay et al., 2015). Above this value, carbonate buffering increasingly regulates pH and restricts CO2 to only trace fractions of total DIC (Stumm and Morgan, 1970). However, direct changes in CO2 concentrations can also alter water-column pH, such as biological metabolism (Talling, 2010). Therefore, given the direct chemical relationship between pH and CO2 concentrations (Stumm and Morgan, 1970), we opted to leave pH out of our model to further investigate the underlying biological, chemical, hydrological, and land use mechanisms.
The detailed GAM showed that variance in CO2 concentrations among farm reservoirs was estimated (66.5 % of deviance) by a combination of predictors related to water-column productivity and microbial metabolism (DO saturation, alkalinity, NOx), thermal stratification (buoyancy frequency), basin hydrology (the interaction between δI and WRT), and landscape features (soil CEC, elevation) (Fig. 3) but not local soil salinity. This pattern was shown by the DO, alkalinity, δI, and WRT covariates having the most significant effect at p<0.001, while CO2 concentrations did not vary significantly between different soil salinity levels (Table S4, Fig. 3).
Carbon dioxide and dissolved oxygen are closely linked by biological metabolism in aquatic systems and diverge when other chemical or physical processes occur. Here, we see evidence for both linked and divergent processes (Fig. 3a). The tight linear relationship between CO2 and O2 at 25 % to 100 % saturation indicates close coupling between the gases. This likely represents control via metabolic processes such as net ecosystem production (NEP) or chemical oxidation of reduced species (Stets et al., 2017). In contrast, relationships between CO2 and O2 were less well defined a both high and low oxygen saturations, conditions which may indicate a greater contribution from anaerobic production of CO2 (Torgersen and Branco, 2008; Holgerson, 2015). Alternatively, alkalinity buffering can mediate the effect of NEP on CO2 concentrations at both extreme ranges of the DO spectrum (Marcé et al., 2015). Alkalinity buffering is most likely to affect CO2–DO relationships in waters where alkalinity is >2000 µeq L−1 (Stets et al., 2017), which was the case for ∼90 % of our sites (Table 1; Fig. 3).
Stratification can also weaken the impact of DO as a driver for CO2 by regulating the effect of sediment respiration on epilimnetic chemistry (Huotari et al., 2009; Holgerson, 2015). Our model shows that those sites that were most stratified (elevated buoyancy frequency) exhibited higher CO2 concentrations (Fig. 3d). This pattern contrasts those observed in other small lentic systems where elevated epilimnetic CO2 concentrations were observed during and after the breakdown of water-column stratification (Huotari et al., 2009; Glaz et al., 2016). Preliminary seasonal studies of some farm reservoirs in 2018 show that stratification is strong and persistent throughout the summer, with no obvious diurnal mixing events. Such strong stratification can maintain anoxic conditions throughout most of the water column, which supports intense anaerobic respiration and CO2 production.
The positive association between NOx and CO2 found in our reservoirs is consistent with similar patterns seen with dissolved inorganic N species in other artificial waterbodies (Ollivier et al., 2019; Peacock et al., 2019) and regional prairie lakes (Wiik et al., 2018). In some lakes, high N loading favoured elevated heterotrophy, despite simultaneous boosts in primary production, which draw down free CO2 (Huttunen et al., 2003; Cole et al., 2000). The effect of a high N influx on CO2 may be heightened in smaller or shallow lentic waters which are more influenced by sedimentary processes (Torgersen and Branco, 2008). Further, high N availability can increase algal biomass and the deposition of fresh organic matter (OM) made increasingly available for bacterial respiration (Cole et al., 2000). As a result, the effect of increased benthic respiration offsets CO2 uptake by primary producers, while extremely high influx of dissolved N can also favour microbial processes such as denitrification which increase CO2 evolution (Bogard et al., 2017).
Hydrological controls were found to be important regulators of CO2 concentrations in these farm reservoirs. Sites which received most of their inflow from snowmelt or groundwater, and which had short WRT, supported supersaturated CO2 concentrations (Fig. 3f). Such patterns may reflect increased inputs of groundwater which are typically supersaturated with CO2 (Macpherson, 2009). Long WRT is associated with larger, deeper systems. These sites are usually less influenced by the terrestrial–aquatic interface, take longer to concentrate the effect of any catchment-derived solutes (Junger et al., 2019), and have higher biotic assimilation of nutrients (Devito and Dillon, 1993; Fairchild and Velinsky, 2006). Larger waterbodies may also be able to better mediate stream or groundwater C inputs through longer chemical processing times and transformations. For example, agricultural reservoirs with the highest WRTs tended to be hydrologically closed systems () and any watershed-derived DIC delivered from previous water sources is likely to be consumed by primary production, which encourages atmospheric CO2 uptake (Macrae et al., 2004). Additionally, smaller waterbodies with shorter WRT can support higher rates of internal CO2 production due to higher rates of allochthonous DOC mineralization (Weyhenmeyer et al., 2015; Vachon et al., 2017).
Groundwater delivery of DIC-rich porewater is the most likely hydrological source resulting in CO2 enrichment of small farm reservoirs. This mechanism is also suggested by the observation that higher reservoir CO2 concentrations are predicted in high-CEC soils. Alkaline high-CEC soils retain more calcium ions within clay particles, which releases carbonates and bicarbonates into soil porewater (Kelley and Brown, 1934). Although regional snowmelt and groundwater have similar isotopic signatures (Pham et al., 2009; Jasechko et al., 2017), the positive correlation of CO2 with alkalinity suggests groundwater as the main source. Edaphic sources of inorganic carbon can result in farm waterbodies accumulating dissolved CO2, bicarbonates, and carbonates, and therefore alkalinity, from the surrounding soils via groundwater discharge (Miller et al., 1985). Other studies have found strong evidence for groundwater inputs driving CO2 supersaturation in small lentic systems (Perkins et al., 2015; Peacock et al., 2019) and watershed-derived alkalinity driving CO2 supersaturation in lakes (Marcé et al., 2015).
Finally, landscape elevation had a significant external effect on reservoir CO2 and may represent diverse weak controls related to landscape setting. Lower CO2 concentrations at higher elevations are common in perched ecosystems with smaller contributing catchment areas (Diem et al., 2012) and low rates of allochthonous carbon influx (Rose et al., 2015). Conversely, waterbodies low in the landscape may receive more watershed C via groundwater influx due to topographical gradient (Winter and LaBaugh, 2003; van der Kamp and Hayashi, 2009). The effect of elevation could also be related to changes in vegetation composition within the local landscape, with the lowest lying catchments exhibiting higher abundance of marginal wetland vegetation (Zhang et al., 2010), which favours higher inputs of terrestrial C (Magnuson et al., 2006; Abril et al., 2014).
4.2 Environmental drivers of CH4 concentrations
The GAM suggested that CH4 concentrations were primarily related to internal biogeochemical processes and the influence of the hydrological regime. For example, factors related to water-column productivity (DO, sediment C∕N, DIN, conductivity) had the most significant effect (p<0.01), while some of the broader landscape features such as soil Ksat and elevation had no significant effect on CH4 levels. The nutrient status of waterbodies is often a primary driver of high CH4 emissions in lakes, impoundments, and ponds (Deemer et al., 2016; Beaulieu et al., 2019; Peacock et al., 2019). Consequently, high nutrient availability is likely fuelling elevated values in both O2 saturation and CH4 (Fig. 4a). High CH4 concentrations at low O2 saturation reflect the development of anoxic habitats which favour methanogenesis (Huttunen et al., 2003; Bastviken et al., 2004). This is likely the result of rapid biomass production which both enriches epilimnion with O2 and depletes O2 in the hypolimnion by providing fresh labile organic matter for decomposition.
In support of eutrophication-driven CH4 production, our model indicated that high proportions of autochthonous organic matter in sediments were associated with elevated concentrations of CH4 (Fig. 4b). Overall, sedimentary C∕N ratios were in the range (8.5 to 13.4) expected for both phytoplankton and submerged macrophytes (Liu et al., 2018). This suggests that in situ rather than terrestrial organic matter was likely the main source of C fuelling methanogenesis in these reservoirs, although increasing CH4 concentrations with C∕N may also represent a larger contribution of terrestrial OM. Strong associations of labile autochthonous C and CH4 production in sediments (Due et al., 2010; Crowe et al., 2011) also suggests a direct link between eutrophication and CH4 production in small farm waterbodies.
Thermal stratification of the water column did not significantly influence surface CH4 concentrations in small farm reservoirs (Fig. 4e). This finding contrasts with observations from other small waterbodies where limited mixing favours CH4 accumulation (Kankaala et al., 2013). Although some small systems exhibit diurnal mixing patterns with turnover at night (Glaz et al., 2016), the wide range of buoyancy frequency values (0.00 to 0.16) suggests that at least some farm reservoirs are continuously stratified, particularly in deeper ponds (Kankaala et al., 2013), as noted for CO2 distributions (see above and Fig. 3d). Taken together, our findings suggest that variability in the biological production of CH4 likely exerts a stronger influence over CH4 concentrations across farm reservoirs than does physical mixing, and this further supports the hypothesis that the prevailing sediment and water chemistry are the primary controls of CH4 concentrations.
Although the hydrological regime of small water bodies is rarely measured, we find that water source (rain, snow/groundwater) and reservoir retention time interact to influence CH4 concentrations (Fig. 4f). In particular, CH4 concentrations were lowest when WRT was long (>1 year) and water was derived mainly from snow or groundwater sources (δ18O depleted). This may be due to a combination of reasons, including the prevalence of sulfate delivered from groundwater (Pennock et al., 2010), dilution of waterbody from snowmelt inflow, and sediments depleted in labile carbon due to longer biogeochemical processing times in the dams. The potential effect of sulfate limiting methanogenesis is in agreement with the strong negative relationship found between CH4 and conductivity in our model (Fig. 4d). Sulfate makes up a large portion of the ionic composition of groundwater in the Prairie Pothole Region due to pyrite oxidation (Goldhaber et al., 2014). Evidently, the biological influence on CH4 concentrations appears less pronounced in these larger, low-flow dams.
In contrast to the external drivers found for CO2, local land use had a significant effect on CH4 concentrations in farm reservoirs (Fig. 4i), with significantly higher CH4 levels in cropland waterbodies than those in pasture. Catchment land use regulates the physico-chemical properties of ponds (Novikmec et al., 2016) by influencing the degree of local vegetative cover and associated influx of allochthonous C to waterbodies (Whitfield et al., 2011). Similarly, regions with crops undergo more intensive agricultural modification, with fertilization, crop rotations, and mechanical disturbance of soil, which all lead to greater nutrient runoff and soil erosion. Our finding contrasts with those from Australian farm reservoirs where diffusive CH4 fluxes were 250 % higher in reservoirs with livestock compared to crops, although the mechanisms responsible for observed differences were inconclusive (Ollivier et al., 2019). This difference could be the result of the intensity of agricultural production, where farm reservoirs supporting high intensity grazing may also experience high CH4 production as demonstrated by a couple of high CH4 concentrations observed in our livestock pasture reservoirs (Fig. 2). In this case it is likely that CH4 levels are more influenced by nutrient loading from the landscape which stimulates eutrophication (Huttunen et al., 2003), as suggested by the biotic variables in our model (Fig. 4). The intensity of agricultural production under different land use types should be an area of further exploration for external controls on farm reservoir GHG production.
4.3 Emissions from farm reservoirs compared to other small waterbodies
To date, small waterbodies on farms have been shown to be large emitters of both CO2 and CH4 (Fig. 5). However, in our study we show that this is not always the case. Diffusive fluxes varied −21 to 466 and 0.14 to 92 mmol m−2 d−1 for CO2 and CH4, respectively. These findings are consistent with other small artificial waterbodies which are strong CH4 sources that exhibit a large range of variability from 0.02 to 33 mmol m−2 d−1 (Grinham et al., 2018a; Ollivier et al., 2019). Average CH4 fluxes from our farm reservoirs correspond to 417 kg CH4 ha−1 yr−1, which is greater than the current IPCC emission factor estimate of 183 kg CH4 ha−1 yr−1 (IPCC, 2019). Considering the skewness of our CH4 data, our median value of 184 kg CH4 ha−1 yr−1 agrees with the emission factor of other artificial ponds.
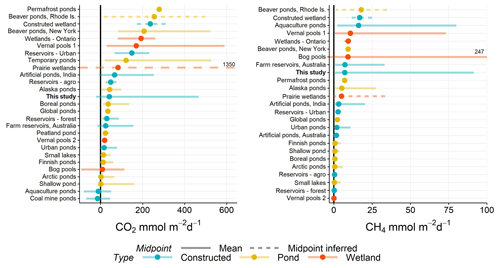
Figure 5Range of CO2 and CH4 (diffusive) fluxes observed in natural and constructed small (<0.01 km2) waterbodies, including this study (farm reservoirs). Dots represent the mean reported in each study and error bars the range. If no mean value was reported, then the midpoint was inferred as the middle of range (dashed lines). Solid black line distinguished between positive and negative fluxes. All data are from the published literature and references can be found in the Table S6.
The negative fluxes observed in our farm dams represents one of the few studied small waterbodies that exhibit CO2 sink behaviour, with most showing net heterotrophy (Fig. 5). Although other studies have noted CO2 sink behaviour in artificial ponds and reservoirs (Peacock et al., 2019; Ollivier et al., 2019), this is the first study to capture such a high proportion (>52 %) of CO2 uptake in such systems, with negative fluxes estimated to range between −21 and −0.1 mmol m−2 d−1 (mean −12 mmol m−2 d−1) for CO2 (Table 1). These flux ranges compare to CO2 uptake of −1 to −11 mmol m−2 d−1 in agricultural eutrophic lakes of North America (Finlay et al., 2010; Pacheco et al., 2014). Studies have shown the importance of eutrophication, leading to net autotrophy, in enhancing CO2 uptake and reversing carbon fluxes in lakes (Pacheco et al., 2014). However, a global analysis of GHG fluxes from lakes and reservoirs revealed that the consequence of increased CH4 emissions with increasing trophic status often outweighs the impact of negative CO2 fluxes (Deemer et al., 2016). Here, our model shows the potential importance of reservoir placement within the landscape as a way of reducing CO2 emissions via hydrological and geochemical controls without the added consequence of increased CH4 emissions.
When CO2 and CH4 fluxes from small artificial waterbodies are compared with natural small waterbodies, no apparent trend exists in which group produces more or less carbon emissions (Fig. 5). Natural ponds and constructed waterbodies have a similar range in variability of mean fluxes for both gases, while wetlands exhibit some of the greatest within-study variability. Constructed waterbodies often have lower net CO2 efflux, suggesting that these systems more often switch between net autotrophy and heterotrophy than do small natural systems. Small artificial waterbodies have disproportionately higher CO2 and CH4 emissions than other natural waterbodies due to the direct impact of agricultural and urban land use (Wang et al., 2017). However, analysis of the limited literature shows that is not the case. We suggest that the lack of a clear distinction between constructed and naturally occurring small water bodies arises because of geographical variation in the relative importance of the diverse factors regulating carbon metabolism (Figs. 3, 4).
When assessing the GHG impact of constructed waterbodies, it is important to consider the relative contribution to CO2-equivalent (CO2-e) fluxes between CO2 and CH4. Here, CH4 fluxes were converted to CO2-e fluxes using the sustained-flux global warming potential over 100 years (Neubauer and Megonigal, 2015). On average, 8 % of farm reservoirs were acting as CO2-e sinks on the range of −0.6 to 79 g CO2 m−2 d−1 during the time of sampling. This number offers a snapshot of the potential for farm reservoirs to act as a net CO2-e sink, and it is important to consider how seasonal variation influences the GHG sink/source status. Preliminary data on seasonal variation in CO2 and CH4 concentrations from a smaller number of farm reservoirs indicate variation (represented as the standard deviation related to the mean) ranging between 20 % and 200 % and between 40 % and 200 % for CO2 and CH4, respectively. Here, this variation represents monthly sampling between the periods of ice melt and ice formation on water bodies in Saskatchewan. Applying the average observed seasonal variation of 78 % and 93 % to our current spatial dataset suggests that CO2-e emissions from farm reservoirs may vary between −1.7 and 150 g CO2 m−2 d−1, or 0 % to 44 %, as acting net CO2-e sinks. Further study into the consistency of potential farm reservoir CO2 sinks on the temporal scale is required to better assess the overall GHG impact.
Small natural ponds and wetlands have some of the highest CO2-e emission rates, with particular importance of contributions from CH4 (Fig. 6). On average our farm reservoirs had one of the highest CH4 contribution to CO2-e fluxes (74 %), in agreement with the one other farm reservoir study (83 %) of CH4 contribution (Ollivier et al., 2019). This large contribution from CH4 is similar to patterns recorded from lakes and impoundments globally, where large freshwater bodies contribute to 75 % of all CO2-e efflux (DelSontro et al., 2018). Fortunately, because the factors that regulate CH4 emissions are becoming better identified (Fig. 4), there exists the possibility that artificial wetlands can be constructed to minimize CH4-related CO2-e emissions and mitigate the overall large rate of CO2-e emissions from agriculture (Robertson et al., 2000).
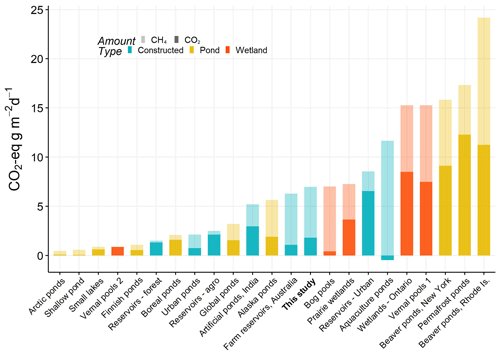
Figure 6Total average CO2 equivalent fluxes of CO2 and CH4 (diffusive) measured in natural and artificial small waterbodies (<0.01 km2). CO2-e fluxes were calculated based on 100-year sustained-flux global warming potentials in Neubauer and Megonigal (2015). Relative proportions of each gas are indicated by shading, and waterbody type is given by colour. All data are from the published literature and references can be found in the Table S6.
4.4 Minimizing emissions: potential management solutions
A combination of factors, including landscape position, construction, and management, could optimize features to minimize carbon emissions from reservoirs and potentially enhance the carbon storage on farms. From our models, we suggest that key variables including the degree of water-column stratification (buoyancy frequency), WRT, water source, land use, and elevation are all suitable parameters for management – for example, strategizing landscape positioning to favour groundwater influx of sulfate to reduce methanogenesis. Increasing WRT by creating deeper reservoirs may promote primary production through increased water clarity (Dirnberger and Weinberger, 2005), facilitate CH4 oxidation through the water column (Bastviken et al., 2008), and reduce the impact of watershed-derived solutes, terrestrial OM, and benthic respiration. Additionally, deeper and larger artificial waterbodies tend to have lower nutrient concentrations due to longer processing times (Chiandet and Xenopoulos, 2016). Finally, modest increases in pH may further enhance CO2 capture (Supplement), while having limited effect on CH4 fluxes (Fig. 4).
Agricultural and urban waterbodies are highly susceptible to nutrient enrichment due to their direct proximity to intensified land uses. Reducing nutrient loading from the landscape will likely have one of the greatest impacts in minimizing C emissions from farm dams given that both CO2 and CH4 were strongly predicted by inorganic N species. In Australian farm reservoirs, for example, a 25 % reduction of nitrates can reduce CO2-e emissions by 50 % (Ollivier et al., 2019). Similarly, removing direct livestock access to farm waterbodies will improve water quality overall through reducing direct DIN inputs and dam infilling.
Nitrogen loading can also have a direct influence on nitrous oxide (N2O), the third most potent greenhouse gas that can contribute substantially to CO2-e emissions in farm systems (Robertson et al., 2000). The flux of N2O was constrained in our earlier study (Webb et al., 2019), which found a small CO2-e sink (−89 to −3 mg CO2 m−2 d−1) for the majority of these farm reservoirs despite high N concentrations. Similar to our CO2 model, stratification and primary production were important regulators in driving N2O uptake (Webb et al., 2019). Therefore, the potential to achieve net GHG sinks weighs mostly on the ability to reduce CH4 emissions in these systems.
Studies have also shown the importance of emergent vegetation plant species in sequestering carbon in sediments. Emergent vegetation was found to contribute significantly to the soil carbon pool of stormwater ponds compared to allochthonous sources (Moore and Hunt, 2012). However, in our CH4 model, the significant effect of sediment C:N ratios suggested that an autochthonous organic matter source from either phytoplankton or submerged macrophytes supports greater CH4 production in farm reservoirs. The ability of farm reservoirs to have a negative climate forcing will rely on the balance between GHG fluxes and sediment carbon accumulation. The effect different plant species and other aquatic primary producers have on both these processes needs to be evaluated in future studies as the current design of farm dams within the study area minimizes growth of emergent vegetation through steep sides and slopes.
It is important to note that the CH4 contribution to CO2-e emissions is likely underestimated here as ebullition emissions were not measured. In farm reservoirs, ebullition flux can contribute >90 % of total CH4 emissions and is often highest in the smallest size classes (Grinham et al., 2018a). However, the sporadic nature of this pathway remains difficult to constrain for one single type of waterbody and may be a minor contributor in reservoirs and ponds > 3–5 m deep (Joyce and Jewell, 2003; DelSontro et al., 2016). This reinforces that design and management strategies that focus on reducing all pathways of CH4 emissions will be most effective in curbing total CO2-e emissions. Deeper farm dams with steep side slopes will likely be effective in reducing ebullition events due to a limited macrophytes, reduced bottom water temperature in summer, and suppressed bubble release with higher water pressure (Joyce and Jewell, 2003; Natchimuthu et al., 2014; Grinham et al., 2018b).
Until recently, carbon emissions from small farm reservoirs have been an overlooked yet potentially important source of CO2 and CH4 emissions within agricultural carbon budgets. To date, development of management strategies to reduce GHG emissions from waterbodies has been limited by the lack of knowledge about the mechanisms regulating CO2 and CH4 production in these systems. By utilizing adaptive modelling techniques across a broad range of environmental variables (abiotic, biotic, hydromorphological, landscape properties), we were able to explain a high degree of deviance in reservoir CO2 and CH4 concentrations. We found that in situ water chemistry and local hydrological regime had the strongest impact on CO2 and CH4 concentrations. In agreement with previous studies, CH4 fluxes were the largest contributor to CO2-e emissions. However, in 19 reservoirs the net CO2-e emissions were found to be sinks. We suggest that, with optimal reservoir design and management, the climatic impact of farm reservoir C-emissions has the potential to be a carbon net sink. To further develop farm reservoir management practices that are locally effective, we express a need for more widespread farm waterbody GHG measurements across the globe to cover other continents and land uses.
All data used in the models are freely available on GitHub (https://github.com/JackieRWebb/Dugouts-CO2-CH4, last access: 31 October 2019) and Zenodo (https://doi.org/10.5281/zenodo.3475628; Webb and Simpson, 2019).
The supplement related to this article is available online at: https://doi.org/10.5194/bg-16-4211-2019-supplement.
JRW, GLS, PRL, HMB, and KF designed the research; JRW performed the research and wrote the paper; HMB contributed the new reagents/analytic tools and managed gas analyses; HAH, PRL, GLS, and KF contributed towards ideas and data analysis; KRH performed the GIS analysis; and GLS developed the models. All authors edited and approved the paper.
The authors declare that they have no conflict of interest.
We thank Jessica Bos, Corey McCowan, Lauren Thies, Ryan Rimas, and Nathanael Bergbusch for fieldwork assistance and all landowners for their generous cooperation in volunteering their reservoirs for this research.
This research has been supported by the Government of Saskatchewan (grant no. 200160015), Natural Science and Engineering Research Council of Canada Discovery grants (to Kerri Finlay, Gavin L. Simpson, Helen M. Baulch, and Peter R. Leavitt), the Canada Research Chairs Program, and the Canada Foundation for Innovation, University of Regina and the Province of Saskatchewan.
This paper was edited by Ji-Hyung Park and reviewed by two anonymous referees.
Abril, G., Martinez, J.-M., Artigas, L. F., Moreira-Turcq, P., Benedetti, M. F., Vidal, L., Meziane, T., Kim, J.-H., Bernardes, M. C., and Savoye, N.: Amazon River carbon dioxide outgassing fuelled by wetlands, Nature, 505, 395–398, 2014.
Anbumozhi, V., Matsumoto, K., and Yamaji, E.: Towards Improved Performance of Irrigation Tanks in Semi-Arid Regions of India: Modernization Opportunities and Challenges, Irrigation and Drainage Systems, 15, 293–309, https://doi.org/10.1023/a:1014420822465, 2001.
Andresen, B., Buchanan, B., Corkal, D., Fairley, B., Fortin, R., Hilliard, C., Kidd, J., Pasquill, R., Sketchell, J., and Thompson, T.: Quality Farm Dugouts, Forestry, A. A. a., Alberta Government, Alberta, 2015.
Badiou, P., Page, B., and Ross, L.: A comparison of water quality and greenhouse gas emissions in constructed wetlands and conventional retention basins with and without submerged macrophyte management for storm water regulation, Ecol. Eng., 127, 292–301, https://doi.org/10.1016/j.ecoleng.2018.11.028, 2019.
Bastviken, D., Cole, J., Pace, M., and Tranvik, L.: Methane emissions from lakes: Dependence of lake characteristics, two regional assessments, and a global estimate, Global Biogeochem. Cy., 18, GB4009, https://doi.org/10.1029/2004GB002238, 2004.
Bastviken, D., Cole, J. J., Pace, M. L., and Van de Bogert, M. C.: Fates of methane from different lake habitats: Connecting whole-lake budgets and CH4 emissions, J. Geophys. Res.-Biogeo., 113, G02024, https://doi.org/10.1029/2007JG000608, 2008.
BC Ministry of Agriculture: British Columbia Farm Water Dugouts, edited by: Petersen, A., available at: https://www2.gov.bc.ca/assets/gov/farming-natural-resources-and-industry/agriculture-and-seafood/agricultural-land-and-environment/water/drought/510400-1_british_columbia_farm_water_dugouts.pdf (last access: 31 October 2019), 2013.
Beaulieu, J. J., DelSontro, T., and Downing, J. A.: Eutrophication will increase methane emissions from lakes and impoundments during the 21st century, Nat. Commun., 10, 1375, https://doi.org/10.1038/s41467-019-09100-5, 2019.
Bogard, M. J., Finlay, K., Waiser, M. J., Tumber, V. P., Donald, D. B., Wiik, E., Simpson, G. L., del Giorgio, P. A., and Leavitt, P. R.: Effects of experimental nitrogen fertilization on planktonic metabolism and CO2 flux in a hypereutrophic hardwater lake, PLOS One, 12, e0188652, https://doi.org/10.1371/journal.pone.0188652, 2017.
Brooks, J. R., Gibson, J. J., Birks, S. J., Weber, M. H., Rodecap, K. D., and Stoddard, J. L.: Stable isotope estimates of evaporation: inflow and water residence time for lakes across the United States as a tool for national lake water quality assessments, Limnol. Oceanogr., 59, 2150–2165, https://doi.org/10.4319/lo.2014.59.6.2150, 2014.
Brunson, M. W.: Managing Mississippi farm ponds and small lakes, 1999.
Chen, W., He, B., Nover, D., Lu, H., Liu, J., Sun, W., and Chen, W.: Farm ponds in southern China: Challenges and solutions for conserving a neglected wetland ecosystem, Sci. Total Environ., 659, 1322–1334, https://doi.org/10.1016/j.scitotenv.2018.12.394, 2019.
Chiandet, A. S. and Xenopoulos, M. A.: Landscape and morphometric controls on water quality in stormwater management ponds, Urban Ecosyst., 19, 1645–1663, https://doi.org/10.1007/s11252-016-0559-8, 2016.
Clifford, C. and Heffernan, J.: Artificial Aquatic Ecosystems, Water, 10, 1096, https://doi.org/10.3390/w10081096, 2018.
Cole, J. J., Pace, M. L., Carpenter, S. R., and Kitchell, J. F.: Persistence of net heterotrophy in lakes during nutrient addition and food web manipulations, Limnol. Oceanogr., 45, 1718–1730, https://doi.org/10.4319/lo.2000.45.8.1718, 2000.
Conly, F. M. and van der Kamp, G.: Monitoring the Hydrology of Canadian Prairie Wetlands to Detect the Effects of Climate Change and Land Use Changes, Environ. Monit. Assess., 67, 195–215, https://doi.org/10.1023/a:1006486607040, 2001.
Crowe, S., Katsev, S., Leslie, K., Sturm, A., Magen, C., Nomosatryo, S., Pack, M., Kessler, J., Reeburgh, W., and Roberts, J.: The methane cycle in ferruginous Lake Matano, Geobiology, 9, 61–78, 2011.
Deemer, B. R., Harrison, J. A., Li, S., Beaulieu, J. J., DelSontro, T., Barros, N., Bezerra-Neto, J. F., Powers, S. M., dos Santos, M. A., and Vonk, J. A.: Greenhouse gas emissions from reservoir water surfaces: a new global synthesis, BioScience, 66, 949–964, https://doi.org/10.1093/biosci/biw117, 2016.
DelSontro, T., Boutet, L., St-Pierre, A., del Giorgio, P. A., and Prairie, Y. T.: Methane ebullition and diffusion from northern ponds and lakes regulated by the interaction between temperature and system productivity, Limnol. Oceanogr., 61, S62–S77, https://doi.org/10.1002/lno.10335, 2016.
DelSontro, T., Beaulieu, J. J., and Downing, J. A.: Greenhouse gas emissions from lakes and impoundments: Upscaling in the face of global change, Limnol. Oceanogr. Lett., 3, 64–75, https://doi.org/10.1002/lol2.10073, 2018.
Devito, K. J. and Dillon, P. J.: Importance of Runoff and Winter Anoxia to the P and N Dynamics of a Beaver Pond, Can. J. Fish. Aquat. Sci., 50, 2222–2234, https://doi.org/10.1139/f93-248, 1993.
Diem, T., Koch, S., Schwarzenbach, S., Wehrli, B., and Schubert, C. J.: Greenhouse gas emissions (CO2, CH4, and N2O) from several perialpine and alpine hydropower reservoirs by diffusion and loss in turbines, Aquat. Sci., 74, 619–635, https://doi.org/10.1007/s00027-012-0256-5, 2012.
Dirnberger, J. M. and Weinberger, J.: Influences of lake level changes on reservoir water clarity in Allatoona Lake, Georgia, Lake Reserv. Manage., 21, 24–29, 2005.
Downing, J. A., Cole, J. J., Middelburg, J. J., Striegl, R. G., Duarte, C. M., Kortelainen, P., Prairie, Y. T., and Laube, K. A.: Sediment organic carbon burial in agriculturally eutrophic impoundments over the last century, Global Biogeochem. Cy., 22, GB1018, https://doi.org/10.1029/2006gb002854, 2008.
Duarte, C. M., Prairie, Y. T., Montes, C., Cole, J. J., Striegl, R., Melack, J., and Downing, J. A.: CO2 emissions from saline lakes: A global estimate of a surprisingly large flux, J. Geophys. Res.-Biogeo., 113, G04041, https://doi.org/10.1029/2007jg000637, 2008.
Due, N. T., Crill, P., and Bastviken, D.: Implications of temperature and sediment characteristics on methane formation and oxidation in lake sediments, Biogeochemistry, 100, 185–196, 2010.
EPA: Method 310.2: Alkalinity (Colorimetric, Automated, Methyl Orange) by Autoanalyzer, Agency, U. S. E. P., 1974.
Fairchild, G. W. and Velinsky, D. J.: Effects of Small Ponds on Stream Water Chemistry, Lake Reserv. Manage., 22, 321–330, https://doi.org/10.1080/07438140609354366, 2006.
Finlay, K., Leavitt, P. R., Wissel, B., and Prairie, Y. T.: Regulation of spatial and temporal variability of carbon flux in six hard-water lakes of the northern Great Plains, Limnol. Oceanogr., 54, 2553–2564, https://doi.org/10.4319/lo.2009.54.6_part_2.2553, 2009.
Finlay, K., Leavitt, P. R., Patoine, A., Patoine, A., and Wissel, B.: Magnitudes and controls of organic and inorganic carbon flux through a chain of hard‐water lakes on the northern Great Plains, Limnol. Oceanogr., 55, 1551–1564, https://doi.org/10.4319/lo.2010.55.4.1551, 2010.
Finlay, K., Vogt, R. J., Bogard, M. J., Wissel, B., Tutolo, B. M., Simpson, G. L., and Leavitt, P. R.: Decrease in CO2 efflux from northern hardwater lakes with increasing atmospheric warming, Nature, 519, 215–218, https://doi.org/10.1038/nature14172, 2015.
Gan, T. Y.: Reducing Vulnerability of Water Resources of Canadian Prairies to Potential Droughts and Possible Climatic Warming, Water Resour. Manage., 14, 111–135, https://doi.org/10.1023/a:1008195827031, 2000.
Gibson, J., Vincent, W., and Pienitz, R.: Hydrologic control and diurnal photobleaching of CDOM in a subarctic lake, Arch. Hydrobiol., 152, 143–159, 2001.
Gilbert, P. J., Taylor, S., Cooke, D. A., Deary, M., Cooke, M., and Jeffries, M. J.: Variations in sediment organic carbon among different types of small natural ponds along Druridge Bay, Northumberland, UK, Inland Waters, 4, 57–64, https://doi.org/10.5268/IW-4.1.618, 2014.
Glaz, P., Bartosiewicz, M., Laurion, I., Reichwaldt, E. S., Maranger, R., and Ghadouani, A.: Greenhouse gas emissions from waste stabilisation ponds in Western Australia and Quebec (Canada), Water Res., 101, 64–74, https://doi.org/10.1016/j.watres.2016.05.060, 2016.
Goldhaber, M. B., Mills, C. T., Morrison, J. M., Stricker, C. A., Mushet, D. M., and LaBaugh, J. W.: Hydrogeochemistry of prairie pothole region wetlands: Role of long-term critical zone processes, Chem. Geol., 387, 170–183, https://doi.org/10.1016/j.chemgeo.2014.08.023, 2014.
Grinham, A., Albert, S., Deering, N., Dunbabin, M., Bastviken, D., Sherman, B., Lovelock, C. E., and Evans, C. D.: The importance of small artificial water bodies as sources of methane emissions in Queensland, Australia, Hydrol. Earth Syst. Sci., 22, 5281–5298, https://doi.org/10.5194/hess-22-5281-2018, 2018a.
Grinham, A., Dunbabin, M., and Albert, S.: Importance of sediment organic matter to methane ebullition in a sub-tropical freshwater reservoir, Sci. Total Environ., 621, 1199–1207, https://doi.org/10.1016/j.scitotenv.2017.10.108, 2018b.
Hamilton, J. D., Kelly, C. A., Rudd, J. W. M., Hesslein, R. H., and Roulet, N. T.: Flux to the atmosphere of CH4 and CO2 from wetland ponds on the Hudson Bay lowlands (HBLs), J. Geophys. Res.-Atmos., 99, 1495–1510, https://doi.org/10.1029/93JD03020, 1994.
Holgerson, M. A.: Drivers of carbon dioxide and methane supersaturation in small, temporary ponds, Biogeochemistry, 124, 305–318, https://doi.org/10.1007/s10533-015-0099-y, 2015.
Holgerson, M. A. and Raymond, P. A.: Large contribution to inland water CO2 and CH4 emissions from very small ponds, Nat. Geosci., 9, 222, https://doi.org/10.1038/ngeo2654, 2016.
Huotari, J., Ojala, A., Peltomaa, E., Pumpanen, J., Hari, P., and Vesala, T.: Temporal variations in surface water CO2 concentration in a boreal humic lake based on high-frequency measurements, Boreal Environ. Res., 14, 48–60, 2009.
Huttunen, J. T., Alm, J., Liikanen, A., Juutinen, S., Larmola, T., Hammar, T., Silvola, J., and Martikainen, P. J.: Fluxes of methane, carbon dioxide and nitrous oxide in boreal lakes and potential anthropogenic effects on the aquatic greenhouse gas emissions, Chemosphere, 52, 609–621, https://doi.org/10.1016/S0045-6535(03)00243-1, 2003.
IPCC: 2019 Refinement to the 2006 Guidelines for National Greenhouse Gas Inventories, Chapter 7; Wetlands, available at: https://www.ipcc-nggip.iges.or.jp/public/2019rf/index.html (last access: 31 October 2019), 2019.
Jasechko, S., Wassenaar, L. I., and Mayer, B.: Isotopic evidence for widespread cold-season-biased groundwater recharge and young streamflow across central Canada, Hydrol. Process., 31, 2196–2209, https://doi.org/10.1002/hyp.11175, 2017.
Joyce, J. and Jewell, P. W.: Physical controls on methane ebullition from reservoirs and lakes, Environ. Eng. Geosci., 9, 167–178, 2003.
Junger, P. C., Dantas, F. d. C. C., Nobre, R. L. G., Kosten, S., Venticinque, E. M., Araújo, F. d. C., Sarmento, H., Angelini, R., Terra, I., Gaudêncio, A., They, N. H., Becker, V., Cabral, C. R., Quesado, L., Carneiro, L. S., Caliman, A., and Amado, A. M.: Effects of seasonality, trophic state and landscape properties on CO2 saturation in low-latitude lakes and reservoirs, Sci. Total Environ., 664, 283–295, https://doi.org/10.1016/j.scitotenv.2019.01.273, 2019.
Kankaala, P., Huotari, J., Tulonen, T., and Ojala, A.: Lake-size dependent physical forcing drives carbon dioxide and methane effluxes from lakes in a boreal landscape, Limnol. Oceanogr., 58, 1915–1930, https://doi.org/10.4319/lo.2013.58.6.1915, 2013.
Kelley, W. and Brown, S.: Principles governing the reclamation of alkali soils, Hilgardia, 8, 149–177, 1934.
Kerekes, J.: The index of lake basin permanence, Int. Rev. Ges. Hydrobio. Hydrogr., 62, 291–293, 1977.
Kuhn, M., Lundin, E. J., Giesler, R., Johansson, M., and Karlsson, J.: Emissions from thaw ponds largely offset the carbon sink of northern permafrost wetlands, Sci. Rep.-UK, 8, 9535, https://doi.org/10.1038/s41598-018-27770-x, 2018.
Last, W. M. and Ginn, F. M.: Saline systems of the Great Plains of western Canada: an overview of the limnogeology and paleolimnology, Saline systems, 1, 1–38, https://doi.org/10.1186/1746-1448-1-10, 2005.
Lehner, B., Liermann, C. R., Revenga, C., Vörösmarty, C., Fekete, B., Crouzet, P., Döll, P., Endejan, M., Frenken, K., Magome, J., Nilsson, C., Robertson, J. C., Rödel, R., Sindorf, N., and Wisser, D.: High-resolution mapping of the world's reservoirs and dams for sustainable river-flow management, Front. Ecol. Environ., 9, 494–502, https://doi.org/10.1890/100125, 2011.
Liu, W., Li, X., Wang, Z., Wang, H., Liu, H., Zhang, B., and Zhang, H.: Carbon isotope and environmental changes in lakes in arid Northwest China, Sci. China Earth Sci., 62, 1–14, 2018.
Lorke, A., Bodmer, P., Noss, C., Alshboul, Z., Koschorreck, M., Somlai-Haase, C., Bastviken, D., Flury, S., McGinnis, D. F., Maeck, A., Müller, D., and Premke, K.: Technical note: drifting versus anchored flux chambers for measuring greenhouse gas emissions from running waters, Biogeosciences, 12, 7013–7024, https://doi.org/10.5194/bg-12-7013-2015, 2015.
Lowe, L., Nathan, R., and Morden, R.: Assessing the impact of farm dams on streamflows, Part II: Regional characterisation, Australasian Journal of Water Resources, 9, 13–26, 2005.
Macpherson, G. L.: CO2 distribution in groundwater and the impact of groundwater extraction on the global C cycle, Chem. Geol., 264, 328–336, https://doi.org/10.1016/j.chemgeo.2009.03.018, 2009.
Macrae, M. L., Bello, R. L., and Molot, L. A.: Long-term carbon storage and hydrological control of CO2 exchange in tundra ponds in the Hudson Bay Lowland, Hydrol. Process., 18, 2051–2069, https://doi.org/10.1002/hyp.1461, 2004.
Magnuson, J. J., Kratz, T. K., and Benson, B. J.: Long-term dynamics of lakes in the landscape: long-term ecological research on north temperate lakes, Oxford University Press on Demand, 2006.
Mantel, S. K., Rivers-Moore, N., and Ramulifho, P.: Small dams need consideration in riverscape conservation assessments, Aquat. Conserv., 27, 748–754, 2017.
Marcé, R., Obrador, B., Morguí, J.-A., Lluís Riera, J., López, P., and Armengol, J.: Carbonate weathering as a driver of CO2 supersaturation in lakes, Nat. Geosci., 8, 107, https://doi.org/10.1038/ngeo2341, 2015.
MDBA: Mapping the growth, location, surface area and age of man made water bodies, including farm dams, in the Murray-Darling Basin, Murray-Darling Basin Commission, Canberra, MDBC Publication, 2008.
Miller, J. J., Acton, D. F., and St. Arnaud, R. J.: The effect of groundwater on soil formation in a morainal landscape in Saskatchewan, Can. J. Soil Sci., 65, 293–307, https://doi.org/10.4141/cjss85-033, 1985.
Moore, T. L. and Hunt, W. F.: Ecosystem service provision by stormwater wetlands and ponds – a means for evaluation?, Water Resour., 15, 6811–6823, https://doi.org/10.1016/j.watres.2011.11.026, 2012.
Müller, B., Meyer, J. S., and Gächter, R.: Alkalinity regulation in calcium carbonate-buffered lakes, Limnol. Oceanogr., 61, 341–352, 2016.
Myhre, G., Shindell, D., Bréon, F. M., Collins, W., Fuglestvedt, J., Huang, J., Koch, D., Lamarque, J. F., Lee, D., Mendoza, B., Nakajima, T., Robock, A., Stephens, G., Takemura, T., and Zhang, H.: Anthropogenic and natural radiative forcing, edited by: Stocker, T. F., Qin, D., Plattner, G. K., Tignor, M. M. B., Allen, S. K., Boschung, J., Nauels, A., Xia, Y., Bex, V., and Midgley, P. M., Cambridge University Press, Cambridge, UK, 2013.
Natchimuthu, S., Panneer Selvam, B., and Bastviken, D.: Influence of weather variables on methane and carbon dioxide flux from a shallow pond, Biogeochemistry, 119, 403–413, https://doi.org/10.1007/s10533-014-9976-z, 2014.
Neubauer, S. C. and Megonigal, J. P.: Moving Beyond Global Warming Potentials to Quantify the Climatic Role of Ecosystems, Ecosystems, 18, 1–14, 2015.
Novikmec, M., Hamerlík, L., Kočický, D., Hrivnák, R., Kochjarová, J., Ot'ahel'ová, H., Pal'ove-Balang, P., and Svitok, M.: Ponds and their catchments: size relationships and influence of land use across multiple spatial scales, Hydrobiologia, 774, 155–166, https://doi.org/10.1007/s10750-015-2514-8, 2016.
Ollivier, Q. R., Maher, D. T., Pitfield, C., and Macreadie, P. I.: Punching above their weight: Large release of greenhouse gases from small agricultural dams, Glob. Change Biol., 25, 721–732, https://doi.org/10.1111/gcb.14477, 2019.
Pacheco, F. S., Roland, F., and Downing, J. A.: Eutrophication reverses whole-lake carbon budgets, Inland Waters, 4, 41–48, https://doi.org/10.5268/IW-4.1.614, 2014.
Panneer Selvam, B., Natchimuthu, S., Arunachalam, L., and Bastviken, D.: Methane and carbon dioxide emissions from inland waters in India-Implications for large scale greenhouse gas balances, Glob. Change Biol., 11, 3397–3407, https://doi.org/10.1111/gcb.12575, 2014.
Patoine, A., Graham, M. D., and Leavitt, P. R.: Spatial variation of nitrogen fixation in lakes of the northern Great Plains, Limnol. Oceanogr., 51, 1665–1677, https://doi.org/10.4319/lo.2006.51.4.1665, 2006.
Peacock, M., Audet, J., Jordan, S., Smeds, J., and Wallin, M. B.: Greenhouse gas emissions from urban ponds are driven by nutrient status and hydrology, Ecosphere, 10, e02643, https://doi.org/10.1002/ecs2.2643, 2019.
Pennock, D., Yates, T., Bedard-Haughn, A., Phipps, K., Farrell, R., and McDougal, R.: Landscape controls on N2O and CH4 emissions from freshwater mineral soil wetlands of the Canadian Prairie Pothole region, Geoderma, 155, 308–319, https://doi.org/10.1016/j.geoderma.2009.12.015, 2010.
Perkins, A. K., Santos, I. R., Sadat-Noori, M., Gatland, J. R., and Maher, D. T.: Groundwater seepage as a driver of CO2 evasion in a coastal lake (Lake Ainsworth, NSW, Australia), Environ. Earth Sci., 74, 779–792, 2015.
Pham, S. V., Leavitt, P. R., McGowan, S., Wissel, B., and Wassenaar, L. I.: Spatial and temporal variability of prairie lake hydrology as revealed using stable isotopes of hydrogen and oxygen, Limnol. Oceanogr., 54, 101–118, https://doi.org/10.4319/lo.2009.54.1.0101, 2009.
Premke, K., Attermeyer, K., Augustin, J., Cabezas, A., Casper, P., Deumlich, D., Gelbrecht, J., Gerke, H. H., Gessler, A., Grossart, H. P., Hilt, S., Hupfer, M., Kalettka, T., Kayler, Z., Lischeid, G., Sommer, M., and Zak, D.: The importance of landscape diversity for carbon fluxes at the landscape level: small-scale heterogeneity matters, WIRES Water, 3, 601–617, https://doi.org/10.1002/wat2.1147, 2016.
Psenner, R. and Catalan, J.: Chemical composition of lakes in crystalline basins: a combination of atmospheric deposition, geologic background, biological activity and human action, in: Limnology Now: A Paradigm of Planetary Problems, edited by: Margalef, R., Elsevier, New York, 533 pp., 1994.
R Core Team: A language and environment for statistical computing, R Foundation for Statistical Computing, Vienna, Austria, 2018.
Read, J. S., Hamilton, D. P., Desai, A. R., Rose, K. C., MacIntyre, S., Lenters, J. D., Smyth, R. L., Hanson, P. C., Cole, J. J., Staehr, P. A., Rusak, J. A., Pierson, D. C., Brookes, J. D., Laas, A., and Wu, C. H.: Lake-size dependency of wind shear and convection as controls on gas exchange, Geophys. Res. Lett., 39, L09405, https://doi.org/10.1029/2012GL051886, 2012.
Robertson, G. P., Paul, E. A., and Harwood, R. R.: Greenhouse Gases in Intensive Agriculture: Contributions of Individual Gases to the Radiative Forcing of the Atmosphere, Science, 289, 1922–1925, https://doi.org/10.1126/science.289.5486.1922, 2000.
Rose, K. C., Williamson, C. E., Kissman, C. E. H., and Saros, J. E.: Does allochthony in lakes change across an elevation gradient?, Ecology, 96, 3281–3291, 2015.
Smith, S. V., Renwick, W. H., Bartley, J. D., and Buddemeier, R. W.: Distribution and significance of small, artificial water bodies across the United States landscape, Sci. Total Environ., 299, 21–36, https://doi.org/10.1016/S0048-9697(02)00222-X, 2002.
Stets, E. G., Butman, D., McDonald, C. P., Stackpoole, S. M., DeGrandpre, M. D., and Striegl, R. G.: Carbonate buffering and metabolic controls on carbon dioxide in rivers, Global Biogeochem. Cy., 31, 663–677, https://doi.org/10.1002/2016GB005578, 2017.
Stumm, W. and Morgan, J. J.: Aquatic chemistry; an introduction emphasizing chemical equilibria in natural waters, Wiley, 583 pp., 1970.
Talling, J. F.: pH, the CO2 System and Freshwater Science, BIOONE, 2, 133–146, 2010.
Taylor, S., Gilbert, P. J., Cooke, D. A., Deary, M. E., and Jeffries, M. J.: High carbon burial rates by small ponds in the landscape, Front. Ecol. Environ., 17, 25–31, https://doi.org/10.1002/fee.1988, 2019.
Torgersen, T. and Branco, B.: Carbon and oxygen fluxes from a small pond to the atmosphere: Temporal variability and the CO2∕O2 imbalance, Water Resour. Res., 44, W02417, https://doi.org/10.1029/2006WR005634, 2008.
Vachon, D., Prairie, Y. T., Guillemette, F., and del Giorgio, P. A.: Modeling Allochthonous Dissolved Organic Carbon Mineralization Under Variable Hydrologic Regimes in Boreal Lakes, Ecosystems, 20, 781–795, https://doi.org/10.1007/s10021-016-0057-0, 2017.
van Bergen, T. J. H. M., Barros, N., Mendonça, R., Aben, R. C. H., Althuizen, I. H. J., Huszar, V., Lamers, L. P. M., Lürling, M., Roland, F., and Kosten, S.: Seasonal and diel variation in greenhouse gas emissions from an urban pond and its major drivers, Limnol. Oceanogr., 64, 2129–2139, https://doi.org/10.1002/lno.11173, 2019.
van der Kamp, G. and Hayashi, M.: Groundwater-wetland ecosystem interaction in the semiarid glaciated plains of North America, Hydrogeol. J., 17, 203–214, https://doi.org/10.1007/s10040-008-0367-1, 2009.
Verpoorter, C., Kutser, T., Seekell, D. A., and Tranvik, L. J.: A global inventory of lakes based on high-resolution satellite imagery, Geophys. Res. Lett., 41, 6396–6402, https://doi.org/10.1002/2014GL060641, 2014.
Wang, X., He, Y., Yuan, X., Chen, H., Peng, C., Yue, J., Zhang, Q., Diao, Y., and Liu, S.: Greenhouse gases concentrations and fluxes from subtropical small reservoirs in relation with watershed urbanization, Atmos. Environ., 154, 225–235, https://doi.org/10.1016/j.atmosenv.2017.01.047, 2017.
Webb, J. R. and Simpson, G.: Farm dam carbon dioxide and methane data, Zenodo, https://doi.org/10.5281/zenodo.3475628, 2019.
Webb, J. R., Hayes, N. M., Simpson, G. L., Leavitt, P. R., Baulch, H. M., and Finlay, K.: Widespread nitrous oxide undersaturation in farm waterbodies creates an unexpected greenhouse gas sink, P. Natl. Acad. Sci. USA, 116, 9814–9819, https://doi.org/10.1073/pnas.1820389116, 2019.
Weiss, R. F.: Carbon dioxide in water and seawater: the solubility of a non-ideal gas, Mar. Chem., 2, 203–215, https://doi.org/10.1016/0304-4203(74)90015-2, 1974.
Weyhenmeyer, G. A., Kosten, S., Wallin, M. B., Tranvik, L. J., Jeppesen, E., and Roland, F.: Significant fraction of CO2 emissions from boreal lakes derived from hydrologic inorganic carbon inputs, Nat. Geosci., 8, 933, https://doi.org/10.1038/ngeo2582, 2015.
Whitfield, C. J., Aherne, J., and Baulch, H. M.: Controls on greenhouse gas concentrations in polymictic headwater lakes in Ireland, Sci. Total Environ., 410, 217–225, https://doi.org/10.1016/j.scitotenv.2011.09.045, 2011.
Wiik, E., Haig, H. A., Hayes, N. M., Finlay, K., Simpson, G. L., Vogt, R. J., and Leavitt, P. R.: Generalized Additive Models of Climatic and Metabolic Controls of Subannual Variation in pCO2 in Productive Hardwater Lakes, J. Geophys. Res.-Biogeo., 123, 1940–1959, https://doi.org/10.1029/2018jg004506, 2018.
Winter, T. C. and LaBaugh, J. W.: Hydrologic considerations in defining isolated wetlands, Wetlands, 23, 532, https://doi.org/10.1672/0277-5212(2003)023[0532:HCIDIW]2.0.CO;2, 2003.
Wood, S. N.: Fast stable restricted maximum likelihood and marginal likelihood estimation of semiparametric generalized linear models, J. R. Stat. Soc. B, 73, 3–36, https://doi.org/10.1111/j.1467-9868.2010.00749.x, 2011.
Wood, S. N., Pya, N., and Säfken, B.: Smoothing Parameter and Model Selection for General Smooth Models, J. Am. Stat. Assoc., 111, 1548–1563, https://doi.org/10.1080/01621459.2016.1180986, 2016.
Yamamoto, S., Alcauskas, J. B., and Crozier, T. E.: Solubility of methane in distilled water and seawater, J. Chem. Eng. Data, 21, 78–80, 1976.
Yi, Y., Brock, B. E., Falcone, M. D., Wolfe, B. B., and Edwards, T. W. D.: A coupled isotope tracer method to characterize input water to lakes, J. Hydrol., 350, 1–13, https://doi.org/10.1016/j.jhydrol.2007.11.008, 2008.
Zhang, J., Ma, K., and Fu, B.: Wetland loss under the impact of agricultural development in the Sanjiang Plain, NE China, Environ. Monit. Assess., 166, 139–148, https://doi.org/10.1007/s10661-009-0990-x, 2010.