the Creative Commons Attribution 4.0 License.
the Creative Commons Attribution 4.0 License.
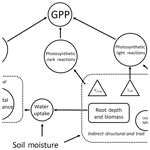
The importance of physiological, structural and trait responses to drought stress in driving spatial and temporal variation in GPP across Amazon forests
Sophie Flack-Prain
Patrick Meir
Yadvinder Malhi
Thomas Luke Smallman
Mathew Williams
The capacity of Amazon forests to sequester carbon is threatened by climate-change-induced shifts in precipitation patterns. However, the relative importance of plant physiology, ecosystem structure and trait composition responses in determining variation in gross primary productivity (GPP) remain largely unquantified and vary among models. We evaluate the relative importance of key climate constraints to GPP, comparing direct plant physiological responses to water availability and indirect structural and trait responses (via changes to leaf area index (LAI), roots and photosynthetic capacity). To separate these factors we combined the soil–plant–atmosphere model with forcing and observational data from seven intensively studied forest plots along an Amazon drought stress gradient. We also used machine learning to evaluate the relative importance of individual climate factors across sites. Our model experiments showed that variation in LAI was the principal driver of differences in GPP across the gradient, accounting for 33 % of observed variation. Differences in photosynthetic capacity (Vcmax and Jmax) accounted for 21 % of variance, and climate (which included physiological responses) accounted for 16 %. Sensitivity to differences in climate was highest where a shallow rooting depth was coupled with a high LAI. On sub-annual timescales, the relative importance of LAI in driving GPP increased with drought stress (R2=0.72), coincident with the decreased importance of solar radiation (R2=0.90). Given the role of LAI in driving GPP across Amazon forests, improved mapping of canopy dynamics is critical, opportunities for which are offered by new satellite-based remote sensing missions such as GEDI, Sentinel and FLEX.
- Article
(2009 KB) - Full-text XML
-
Supplement
(640 KB) - BibTeX
- EndNote
As the entry point for carbon into the biosphere, gross primary productivity (GPP) is central to the global carbon cycle. Tropical rainforests alone account for one-third of total terrestrial GPP, assimilating ∼41 Pg of carbon each year (Beer et al., 2010). Carbon fluxes across the tropics are tightly coupled to climate, and water availability is a principal driver of spatial and temporal variation in GPP (Fisher et al., 2007; Von Randow et al., 2013; Beer et al., 2010; Malhi et al., 2015; Guan et al., 2015). Across Amazon forests, GPP decreases linearly with increasing seasonal water deficit (Malhi et al., 2015). Shifts in precipitation patterns as a result of anthropogenic climate change are predicted to have a major impact on Amazon GPP (Phillips et al., 2009; Malhi et al., 2008; Meir and Woodward, 2010; Zhang et al., 2015; Meir et al., 2015a). Longer and more intense dry seasons are projected, together with an increased frequency and severity of drought events (Joetzjer et al., 2013; Boisier et al., 2015; Duffy et al., 2015). Given the biogeochemical influence of Amazon forests at regional and global scales (Liu et al., 2017), accurately predicting GPP response to drought stress is critical.
Dynamic global vegetation models (DGVMs) disagree on the effects of projected precipitation change on Amazon carbon dynamics. Galbraith et al. (2010) found future shifts in precipitation patterns had little effect on model estimates of biomass change (for two of the three models tested), reflecting poorly the observed sensitivity of Amazon forests to water availability illustrated by throughfall exclusion experiments and natural drought events (Rowland et al., 2015a; Nepstad et al., 2007; Phillips et al., 2009). Substantial progress has been made in model development to capture the impact of drought stress on plant physiology. By coupling stomatal conductance and plant hydraulic theory, models have proved better able to predict ecosystem functioning and mortality (Eller et al., 2018; Fisher et al., 2006, 2007, 2018; Bonan et al., 2014). However, the interactions between drought stress, ecosystem structure (e.g. canopy dynamics and rooting depth) and trait composition (e.g. Vcmax, Jmax, leaf lifespan and leaf mass per unit area (LMA)) are typically absent from models, despite having a major impact on simulated GPP (Fauset et al., 2012; Sakschewski et al., 2016; Lee et al., 2013). Furthermore, changes in canopy dynamics have been identified as a likely cause for the disparity between field observations and model predictions (Restrepo-Coupe et al., 2017; Powell et al., 2013).
The relative importance of plant physiology, ecosystem structure and trait composition responses in determining variation in GPP remain largely unquantified in data-constrained analysis (Meir et al., 2015b). Plant physiological responses to drought stress include stomatal conductance, which is limited by water availability and atmospheric demand. Stomatal conductance constrains GPP via changes in CO2 supply but is considered a short (varying on sub-hourly timescales) rather than long-term response to climate forcings (Sperry et al., 2002). Changes to both ecosystem structure and traits, such as leaf area index (LAI), rooting depth and carboxylation capacity, are expected to be more long-standing (Meir et al., 2015a).
Extensive evidence links spatial and temporal variation in drought stress with ecosystem structure (across sub-annual and annual timescales). LAI typically decreases with increasing drought stress (Iio et al., 2014; Meir et al., 2015b; Brando et al., 2008; Grier and Running, 1977; Wright et al., 2013). Across the wet–dry tropical forest transition, LAI declines on average ∼1.4 m2 m−2 (Iio et al., 2014). Brando et al. (2008) report a 21 %–26 % decline in LAI following 5 years of drought onset at the Amazon throughfall exclusion experiment at Tapajós National Forest, Pará, Brazil. Growth of near-surface root mass, length and surface area decline with seasonal drought stress (and increase during periods of high soil water availability to exploit available resources), whilst deep roots can support water supply during dry periods (Nepstad et al., 1994; Metcalfe et al., 2008). Root depth, mass and traits influence hydraulic supply and consequently stomatal conductance.
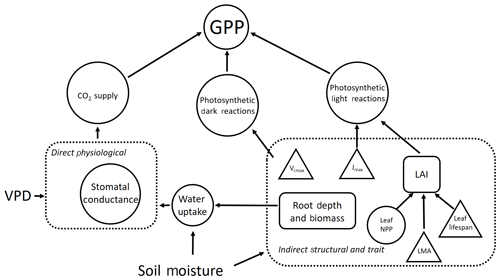
Figure 1A schematic of the direct and indirect effects of drought stress via soil moisture and VPD on GPP. Drought stress affects GPP directly via stomatal conductance and indirectly through its determinant effect on plant traits and structural properties. Plant processes are represented by circles, traits are represented by triangles and vegetation properties (i.e. ecosystem structure) are represented by rectangles. Dashed boxes identify interactions driving the direct and indirect pathways through which drought stress impacts GPP. We note that other climate forcings (e.g. shortwave radiation and temperature) impact GPP but are not included here.
Leaf traits similarly exhibit spatial and temporal variation with changing water availability. Leaf nitrogen content (per unit mass) and light- and CO2-saturated photosynthetic rates (per unit mass) increase with drought stress across tropical precipitation gradients, as ψ50 (the water potential at which 50 % of hydraulic conductivity is lost) declines (Wright et al., 2004; Santiago et al., 2004; Anderegg, 2015). Leaf traits affect GPP via photosynthetic capacity (Vcmax and Jmax) (Bahar et al., 2017; Fyllas et al., 2017) and, through their influence on canopy carbon economics, via leaf growth and maintenance costs (Bloom et al., 1985).
Field observations show variation in Amazon GPP is correlated with physiological, ecosystem structure and trait composition responses to climate (Restrepo-Coupe et al., 2013; Goulden et al., 2004; Hutyra et al., 2007; Wu et al., 2017; Wagner et al., 2017). Modelling approaches have similarly highlighted the role of canopy dynamics and leaf traits in driving spatial and temporal variation in GPP (Mercado et al., 2011; Castanho et al., 2013; Restrepo-Coupe et al., 2013; Rodig et al., 2018); however, their relative effects have not been explicitly isolated and quantified. Quantifying the direct effect of discrete photosynthetic drivers has been limited by the need for detailed field measurements of carbon fluxes, canopy dynamics and traits. A deserved research effort has focused on the importance of nutrient availability in driving spatial variation in GPP (Mercado et al., 2011; Castanho et al., 2013); however, the role of ecosystem responses to water availability has received less attention (Green et al., 2019). In light of projected changes in rainfall patterns across the basin, capturing responses to water availability in ecosystem models is critical to reducing current uncertainty around Amazon climate–vegetation feedbacks. We aim to reduce the uncertainty by assessing the relative effects of physiological, structural and trait responses to water availability on GPP across monthly to annual timescales.
We apply an ecosystem model to plots across the Amazon, spanning a large drought stress gradient (herein, the term drought stress refers to seasonal water deficit), and a range in forest types from moist equatorial to seasonally dry tropical forests. Process modelling allows the links between climate, ecosystem structure and leaf traits to be quantified explicitly and separated across timescales (Fig. 1). The soil–plant–atmosphere model (SPA) (Williams et al., 1996, 1998; Fisher et al., 2006, 2007; Rowland et al., 2015b) is well suited to this investigation given its prior use in accurately simulating carbon and water fluxes in Amazon tropical forests. We calibrate and validate the model using field data gathered over multiple years (2009–2010) on permanent sample plots from the Global Ecosystems Monitoring (GEM) network (Doughty et al., 2015; Malhi et al., 2015). The datasets comprise detailed measurements of carbon fluxes, carbon stocks and leaf traits. We simulate the effect of forest structure and leaf trait distributions along the drought stress gradient, and we explore the covariation of observed leaf traits (leaf N content (a proxy for photosynthetic capacity) and LMA) and those derived from model calibrations (leaf lifespan), before using SPA to address the following questions.
- Q1
Is spatial variation in GPP across the drought stress gradient principally driven by the direct effects of climate and soils, which include physiological responses to water availability via hydraulic transport and stomatal conductance? Alternatively, are indirect effects of climate, via structural and trait responses to water availability (LAI, rooting biomass, root depth and photosynthetic capacity, i.e. Vcmax and Jmax), more important?
- Q2
Does the sensitivity of GPP to differences in climate, LAI, photosynthetic capacity (Vcmax and Jmax) and rooting depth vary across the drought stress gradient?
- Q3
What drives seasonal variation in GPP across an Amazon forest drought stress gradient?
Linked to question one, we hypothesise that indirect effects of climate via structural and trait responses are more important than the direct effects (via physiological responses) in explaining spatial variation in GPP across the drought stress gradient (Fig. 1). We further posit that LAI is the principal driver of differences in GPP among Amazon forests, effected through the observed increase in leaf area with decreasing drought stress.
For question two, we predict that the sensitivity of GPP to differences in climate, LAI, photosynthetic capacity (Vcmax and Jmax) and rooting depth will vary depending on water demand (via LAI and stomatal conductance) and supply (climate and root depth and biomass; Fig. 1). We expect that forests under lower drought stress will be most sensitive to differences in LAI and photosynthetic capacity within the bounds of observations across the gradient. We predict that forests under higher drought stress will be more sensitive to differences in rooting depth. We expect forests with a high LAI but a shallow rooting depth will be most sensitive to differences in climate, due to their higher transpiration demand but low capacity for water supply.
For question three, we hypothesise that, on monthly timescales, climate will be more important than canopy dynamics in driving GPP. Across the drought stress gradient, we expect that solar radiation will be relatively more important during the wet season. Vapor pressure deficit (VPD) will be more important during the dry season, reflecting seasonal shifts in light and water availability. Due to differences in dry season length, we predict that for forests experiencing lower drought stress, solar radiation will be most important in driving sub-annual variation in GPP. For forests under higher drought stress, VPD will be the dominant driver.
By combining detailed plot-level time series data with a hydrodynamic terrestrial ecosystem model, we are able to use an innovative model experimentation approach to understand the drivers of spatial variation in GPP, beyond correlative effects. We are able to apportion variation in GPP to the direct and indirect effects of climate (Fig. 1), across sub-annual and annual timescales (Q1 and Q3). Furthermore, by performing a sensitivity analysis within the context of observed variation in parameters across the Amazon (Q2) we identify areas potentially more vulnerable to changes in precipitation regime.
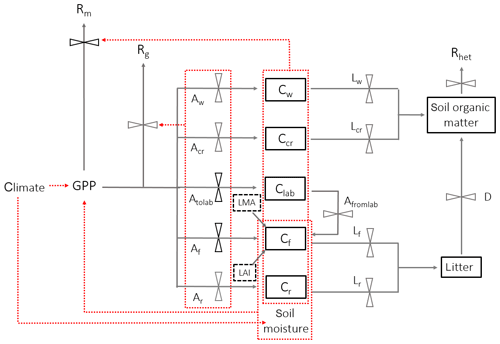
Figure 2A schematic of DALECcanopy, the carbon allocation sub-model integrated within the soil–plant–atmosphere model. Carbon moves between pools (solid boxes) via fluxes (solid arrows). Leaf carbon fluxes are constrained by field measurements (black dashed boxes). An effect of climate, carbon pools or fluxes on another carbon flux is shown by a red dashed arrow, whereby red dotted boxes indicate a collective impact of the contained carbon pools or fluxes. Black flux bars indicate that the carbon pathway is prioritised within the model above pathways from the same node. Climate is a model input, and soil moisture is simulated within SPA. Carbon pools (C), allocation (A) and litterfall (L) are separated by component: w represents wood, cr represents coarse roots, r represents fine roots, f represents foliage, and lab represents labile (or non-structural carbon), with to and from used for labile carbon.
Plot characteristics are summarised in Table 1 and detailed in full in the Supplement. We characterise plot water status using mean maximum climatological water deficit (MCWD) and not annual precipitation, as water deficit is more closely linked to the mechanisms constraining GPP than total water input. MCWD is the maximum cumulative water deficit reached within a year. A water deficit estimate for each month is calculated as the difference between precipitation and transpiration (which ground measurements estimate at ∼100 mm per month; see Aragao et al., 2007). Therefore, the forest is in water deficit if monthly precipitation falls below 100 mm. Maximum cumulative water deficit is calculated as the sum of sequential monthly water deficits (for equations see Supplement). More negative MCWD values indicate higher drought stress.
Table 1Amazon Forest Inventory Network (RAINFOR) site code and environmental characteristics of GEM network Amazon permanent sample plots across the MCWD gradient. Meteorological data are from local weather stations, gap filled with ERA-Interim data for the years 2009–2010 (Dee et al., 2011).
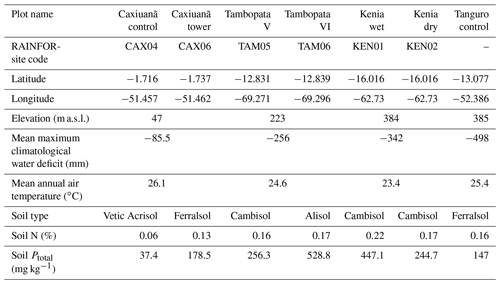
2.1 The soil–plant–atmosphere model (SPA)
The soil–plant–atmosphere model (SPA) is a hydrodynamic terrestrial ecosystem model, which has been calibrated and evaluated for moist tropical forests in Manaus and Caxiuanã (Williams et al., 1996, 1998; Fisher et al., 2007). In SPA, carbon and water fluxes are estimated through process-based modelling of radiative transfer, boundary layer and stomatal conductance; plant and leaf ecophysiology; and soil–plant energy and water balance (Smallman et al., 2013; Williams et al., 1996). Plant physiological responses to water availability are well represented in SPA due to the stomatal conductance algorithm being coupled directly to plant water use (Fisher et al., 2006). As a result, higher evaporative demand under increased LAI drives increased root water uptake and consequently a depletion in soil moisture. Within SPA, C allocation between structural tissue and the non-structural C (NSC) pool is executed via the sub-model DALECcanopy (Bloom and Williams, 2015) (Fig. 2). DALECcanopy was updated on daily time steps and, in this study, forced using LAI observation data. Constraining simulated LAI was integral to the model experiments conducted. It allowed the quantification of direct effects of different LAI time series on GPP under different plot conditions. However, the capacity of SPA to accurately simulate canopy dynamics is demonstrated by both López-Blanco et al. (2018) and Sus et al. (2010). To force modelled LAI, LMA (gC m−2) and daily LAI estimates were used to calculate the foliar C stock. Leaf net primary productivity (NPP) was calculated as the difference between the foliar C stock of the current and previous time step. Leaf NPP was calculated as the difference between the foliar C stock of the current and previous time step. Leaf NPP was allocated prior to other plant components, and if the leaf NPP requirement exceeded total NPP for the given time step, the non-structural C pool was drawn upon (where total NPP was calculated as the difference between simulated GPP and autotrophic respiration) (see Supplement). The NSC pool serves functions additional to the seasonal redistribution of C (e.g. phloem transport and osmoregulation; Dietze et al., 2014). As such, we assume the NSC pool is stable over time. If the NSC pool becomes depleted, a fraction of NPP is redirected towards NSC storage. Allocation towards NSC storage is executed in subsequent time steps when leaf NPP does not exceed total NPP. Root and wood NPP were calculated from the NPP remaining after leaf allocation. Leaf maintenance respiration was calculated as a function of leaf N content (Reich et al., 2008) and total leaf C stock (see Supplement). Within SPA, wood and fine root maintenance respiration were simulated as a function of component C stock and a plot-specific respiration coefficient. Growth respiration was calculated as the fixed fraction of net primary productivity (NPP; 0.28) (Waring and Schlesinger, 1985). Model inputs and outputs are listed in Table 2.
Table 2Summary of the relationship between model variables and field data. Values are either a SPA model parameter (input) or output. Model parameters may be initial conditions, a fixed value, or a time series, whereby the parameter value at each time point is prescribed to the model. Model outputs are generated on either an hourly or daily time step and are presented in the text as the mean annual sum (2009–2010), unless otherwise stated. Model outputs are calibrated or evaluated using field data. Values are specific to each of the seven GEM Amazonian permanent sample plots.
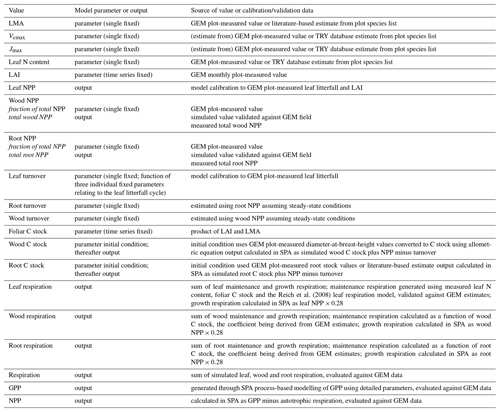
2.2 Model calibration
Following data collation to parameterise SPA, the model was calibrated and validated for each plot prior to conducting model experiments. Measurements used to parameterise SPA include soil texture; soil C stock; leaf N content; LMA; photosynthetic capacity; the fraction of NPP allocated to fine roots and wood; root depth; and foliar, wood and fine root C stocks (Table 2). Soil, wood and fine root C stocks (single point measurements, not time series) were the initial model inputs and were allowed to vary thereafter depending on simulated C dynamics. Plot-specific field measurements of leaf N content are presented in Fyllas et al. (2009), or where absent were retrieved from trait databases using plot species composition (Kattge et al., 2011; Poorter and Bongers, 2006). Photosynthetic capacity estimates (Vcmax and Jmax) were derived from leaf N content (Walker et al., 2014) or field measurements (Caxiuanã only). Wood and root respiration measurements were used together with component C stocks to estimate plot-specific wood and root respiration coefficients.
The model was driven using hourly meteorological data, retrieved from local weather stations. The number of missing hourly field meteorological measurements across the time series varied from 2 % to 40 % across sites, whilst the frequency of gaps varied from 2 to 99 yr−1. Gaps less than 6 h in length accounted for between 20 % and 100 % of total gaps across plots. Short gaps in air temperature, wind speed, shortwave radiation and vapour pressure deficit measurements (< 6 h) were filled by spline interpolation between existing data. Where local meteorological data were unavailable for a longer period of time, or for gaps in precipitation measurements, hourly spline-interpolated ERA-Interim data were used (Dee et al., 2011). The interpolation of solar radiation estimates accounted for the solar zenith angle. MCWD was calculated for the years 2009–2010 and was consistent with previously published estimates for all plots excluding Caxiuanã, which were calculated across different years (Malhi et al., 2015, Caxiuanã −203 mm, Tambopata −259 mm, Kenia −386 mm, Tanguro −482 mm; this study, Caxiuanã mm, Tambopata mm, Kenia 342±146 mm, Tanguro 451±73 mm).
The simulation of soil water drainage in SPA was calibrated against time series of field measurements of soil moisture. Initial investigations comparing modelled soil moisture to monthly field data highlighted an overestimation by SPA. Pre-calibration, SPA soil moisture estimates were on average 11 %–68 % higher than field measurements across plots. The difference between model and field soil moisture estimates increased significantly with MCWD (R2=0.69, p=0.04). The empirical model used in SPA to relate soil texture to water retention (Saxton et al., 1986, Eq. 10) was then calibrated by adjusting the slope of the interaction to better represent soil moisture across tropical soils (to within standard error estimates of mean annual soil moisture).
Leaf litterfall parameters (day of peak leaf fall, leaf fall period and leaf lifespan) were calibrated against field data to accurately simulate litterfall period and amplitude (within standard error estimates of annual litterfall). Wood and fine root biomass turnover rates were assumed to be proportional to NPP, given the maturity of stands and their disturbance history:
where i is wood or fine roots.
Local, monthly LAI estimates derived from hemispherical photographs were scaled to daily estimates via linear interpolation and used to force simulated LAI. The vertical distribution of leaf area is kept constant, as current field data are insufficient to provide an accurate depiction of how vertical distributions change with canopy density across the MCWD gradient.
We calculate model uncertainty as a result of input parameters. SPA was forced with the observed LAI time series plus and minus the standard error for each plot. Model uncertainty estimates were limited to that derived from LAI as the availability of uncertainty estimates for leaf traits, root depth and root biomass was variable and plot dependent, and there were no uncertainty estimates for hourly meteorological data or soil properties. Model structural uncertainty was not calculated, and we recognise that the model error estimates presented are therefore underestimated. With respect to model structural uncertainty, we highlight that the stomatal conductance algorithm embedded within SPA is consistent with leaf- and canopy-scale observations and surpasses the performance of the Ball–Berry model where soils experience moisture stress (Bonan et al., 2014). However, model (and empirical) uncertainty remains around the role of non-structural carbon in regulating water transport in large trees during drought periods (O'Brien et al., 2014). Furthermore, SPA does not account for hydraulic lift and redistribution of water through the soil profile, which is known to impact water fluxes across the soil–plant–atmosphere continuum in Amazon trees (Oliveira et al, 2005; Wang et al., 2011).
2.3 Model validation
Observation-constrained SPA simulations were validated against biometric field measurements of C fluxes (i.e. from infrared gas analysers, dendrometers, root ingrowth cores litterfall traps, etc.). Linear regression models were constructed to compare modelled estimates and independent field measurements of GPP, autotrophic respiration and total NPP. A comprehensive comparison of model estimates and independent field measurements of component NPP and respiration were also made. Validation of the SPA model against biometric data lent confidence to subsequent analyses, where the model was used to explore C fluxes under non-observed conditions.
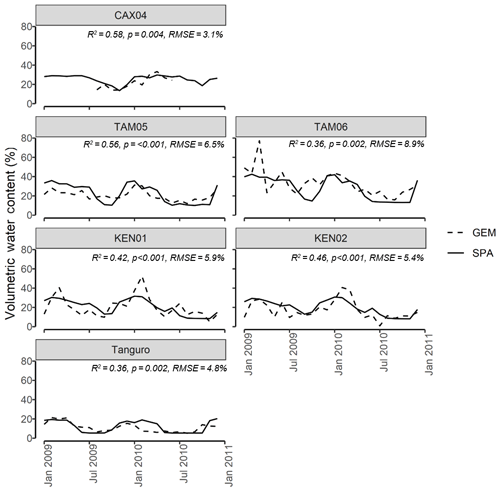
Figure 3SPA estimated soil volumetric water content compared to GEM measured values for six of the seven sample plots at four locations across the Amazon basin. Data presented are for the time period 2009–2010. Field data for CAX04 were limited to a shorter time period and were unavailable for CAX06. R2, p value and RMSE estimates presented are derived from linear regressions between monthly GEM measurements and SPA simulations.
2.4 Model experiments
Our aim was to isolate the direct effects of climate and soils (via physiological responses), as well as the indirect effects via ecosystem structure, and leaf traits, on simulated GPP. To avoid capturing the feedback effects of changing photosynthate supply (i.e. as a result of changes in climate, soils, ecosystem structure or traits) on ecosystem structure, model experiments were conducted in the absence of C cycle feedbacks. Thus, within model experiments, C stocks for each component (leaves, wood, fine root, coarse root) were constrained to observations unless otherwise stated.
2.4.1 Experiment 1: drivers of spatial variation in GPP
Through a series of model input alternations, we used SPA to quantify the effects of (i) climate, (ii) soil properties, (iii) LAI, (iv) root biomass and (v) rooting depth, as well as (vi) trait responses driven by photosynthetic capacity (Vcmax and Jmax), on simulated GPP. Model inputs for each driver were alternated at each plot to that of all other plots, and annual GPP values for each of the two years were retrieved. For example, plot CAX04 was simulated with the climate, soil properties, LAI, root biomass, root depth and photosynthetic capacity of CAX06, TAM05, TAM06, KEN01, KEN02 and Tanguro (Fig. S1 in the Supplement). SPA-simulated GPP for a total of 462 combinations (for climate, 7 plots × 3 alternations × 2 years plus, for the remaining drivers, 5 drivers × 7 plots × 6 alternations × 2 years) was combined with 14 annual GPP estimates from observation-constrained (control) runs (7 plots × 2 years). A factorial ANOVA was applied to the difference between GPP from each model run and its control simulation (n=476, i.e. 462 +14) (Galbraith et al., 2010). The proportions of variation in GPP explained by climate, soil properties, LAI, photosynthetic capacity, root biomass and rooting depth were then calculated as the conditional sum of square divided by the total sum of squares.
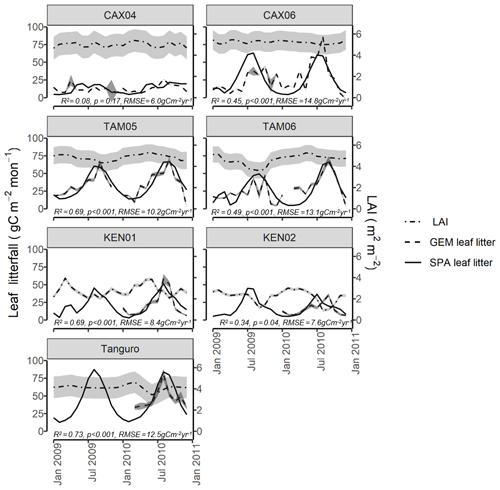
Figure 4Field estimated monthly LAI, leaf litterfall (GEM) and standard error, compared with SPA-simulated leaf litterfall for seven plots at four locations across the Amazon basin. SPA leaf litterfall was calibrated against GEM estimates to derive three fixed model drivers relating to the leaf cycle (peak leaf fall timing, leaf fall period and leaf lifespan). GEM leaf litterfall data were available for 2009–2010 for CAX04, CAX06, TAM05 and TAM06 and for 2010 only for KEN01, KEN02 and Tanguro. R2, p value and RMSE estimates presented are derived from linear regressions between monthly GEM measurements and SPA simulations.
2.4.2 Experiment 2: variation in forest sensitivity to drivers of GPP
We quantified how the relative sensitivity of GPP to differences in LAI, climate, photosynthetic capacity and rooting depth varied across the MCWD gradient. For example, we tested whether forests occupying lower drought stress zones were more sensitive to differences in LAI than forests in higher drought stress zones. We used model outputs generated in Experiment 1 to calculate the sensitivity of GPP to drivers at each plot, within the bounds of observations across the MCWD gradient. Root biomass and soil properties were not included in the analysis as across the MCWD gradient they explained little variation in GPP (Experiment 1, Table 6). The sensitivity of GPP to drivers at each plot was calculated as the absolute range in simulated GPP values under each driver alternation, i.e. the sensitivity of CAX04 to variation in LAI was calculated as the maximum GPP minus the minimum GPP simulated by alternating LAI with that of all other plots. Plots were grouped by location (Caxiuanã, Tambopata, Kenia and Tanguro) to compare how the sensitivity of GPP to LAI, climate, photosynthetic capacity and rooting depth varies across the MCWD gradient.
2.4.3 Experiment 3: drivers of sub-annual variation in GPP
We quantified the role of climate and LAI in explaining variation in sub-annual GPP. We used the random forest technique to compute the relative importance of LAI, VPD, solar radiation, precipitation and air temperature driving variation in monthly GPP (n=168; 7 plots × 24 months), where GPP estimates were derived from SPA simulations. To quantify the effects of LAI and climate variables on monthly GPP, we used the random forest machine learning technique applied by means of the Python scikit-learn module (Breiman, 2001; Pedregosa et al., 2011). The approach uses multiple mathematical decision tree predictors to describe a dependent variable as a function of selected independent variables. An importance value between 0 and 100 was assigned to each driver based on a tree-wise comparison of explanatory power (Moore et al., 2018; López-Blanco et al., 2017). We calculated the average relative importance of drivers at each plot to determine the principal drivers of variation in sub-annual GPP and investigated the seasonality of driver importance.
3.1 Model calibration
Calibrated SPA soil water content corresponded well to field measurements from the GEM network (Fig. 3). Simulated mean annual soil moisture estimates were within field measurement standard error for all plots. The timing of observed peak soil moisture was captured by SPA simulations (R2=0.98, p<0.001, RMSE = 1 month). A positive, but non-significant, correlation existed between model and field estimates of seasonal soil moisture range (R2=0.35, p=0.21, RMSE = 5 %).Notably, for some plots such as Kenia, the magnitudes of seasonal peak soil water fluxes were not captured by SPA simulations (up to 39 % lower than field estimates). For Tanguro, peak soil water lasted 3 months longer in SPA simulations than was measured in the field.
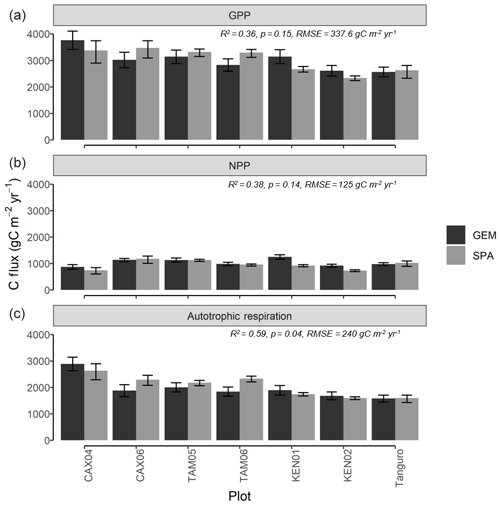
Figure 5Carbon flux estimates (gC m−2 yr−1) of (a) GPP, (b) NPP and (c) autotrophic respiration, derived from process-based modelling (SPA) and biometric methods (GEM) for seven permanent sample plots at four locations across the Amazon basin. Estimates are mean annual values representative of the years 2009–2010. GEM error bars represent the standard error from field carbon flux measurements. SPA error bars represent simulated C fluxes under the upper and lower field LAI standard error. R2, p values and RMSE represent the interaction between SPA and GEM C flux estimates.
SPA was also successfully calibrated to simulate local leaf litterfall accurately. The calibration of leaf fall cycle parameters in SPA using GEM leaf litterfall time series (Table 4), resulted in the magnitude and timing of leaf litterfall being well represented by the model for all plots (monthly leaf litterfall range for GEM measurements and SPA simulations: R2=0.54, p=0.009, RMSE = 11.2 gC m−2 yr−1; timing of leaf litterfall peak: R2=0.96, p<0.001, RMSE = 1.1 months) (Fig. 4). SPA-simulated mean annual leaf litterfall correlated significantly with GEM estimates (R2=0.99, p=<0.001, RMSE = 9.0 gC m−2 yr−1).
3.2 Model validation
Estimates of ecosystem-scale C fluxes from SPA model runs were validated against biometrically derived estimates from the GEM network. GPPSPA and GPPGEM estimates were correlated across plots, though not significantly (R2=0.36, p=0.15; Fig. 5a). Along the MCWD gradient, GPPSPA estimates varied across plots by 1137 gC m−2 yr−1, in line with GPPGEM estimates which varied by 1202 gC m−2 yr−1. Error bars overlap between GPPSPA and GPPGEM estimates for all plots except KEN01 and TAM06, though marginally (the difference for KEN01 is 115 gC m−2 yr−1 and for TAM06 it is 50 gC m−2 yr−1). GPPGEM error bars represent the field estimate standard error, and GPPSPA error bars represent the simulated GPP variance under the LAI standard error. Across plots, GPPSPA estimates were 0.57 % higher than GPPGEM estimates. The correlation between GPP and MCWD was similar for GPPSPA (R2=0.64, p=0.03, slope = 2.4) and GPPGEM estimates (R2=0.52, p=0.07, slope = 2.00).
NPPSPA estimates (the sum of model-simulated root and wood NPP and data-constrained leaf NPP) were also correlated with NPPGEM measurements across plots (R2=0.38, p=0.14), though not significantly due to differences in Kenia plots (on exclusion of Kenia plots: R2=0.92, p=0.01, RMSE = 42 gC m−2 yr−1) (Fig. 5b). NPPSPA estimates were 7.9 % lower than field measurements across plots on average. RaSPA values (the sum of predicted leaf respiration and parameterised root and wood respiration) were significantly correlated with biometric measurements (RaGEM) across plots (R2=0.59, p=0.04; Fig. 5c), though they were on average 5.3 % higher.
Table 3Field estimated mean annual leaf area index (LAI), leaf traits, maximum rooting depth and fine root biomass for Amazon permanent sample plots along a MCWD gradient. LAI estimates were derived from monthly hemispherical photographs. LAI, leaf trait and rooting depth estimates were used to constrain SPA model runs. Estimate standard errors are presented in brackets. Fine root C stock estimates were absent for Tanguro plots.
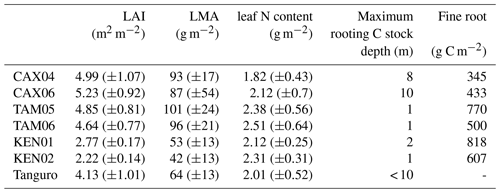
Table 4SPA calibrated leaf litterfall parameters for plots across an Amazon MCWD gradient. Peak leaf fall is the day of year leaf litterfall reaches its maximum, leaf lifespan reflects maximum lifespan of leaves and leaf fall period is the number of days over which systematic increases in leaf fall occur. Leaf litterfall parameters were calibrated against GEM field estimates to capture leaf litterfall and timing.
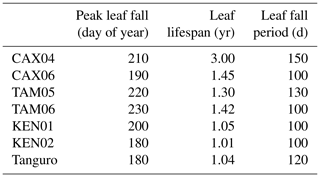
Leaf respiration estimates simulated as a function of leaf nitrogen content were correlated with field measurements, though not significantly (R2=0.47, p=0.09; Table 5). Parameterised wood and fine root respiration, together with fine root NPP, correlated significantly with field measurements. SPA estimates of wood NPP did not correlate significantly with GEM measurements due to underestimation at KEN01 (on exclusion: R2=0.78, p=0.02, RMSE = 7.5 gC m−2 yr−1). Further comparisons of SPA estimates and GEM measurements of component NPP and respiration are presented in Table 5.
3.3 LAI and leaf trait trends along the MCWD gradient
Field estimated mean annual LAI ranged from 2.2 to 5.2 m2 m−2 and increased (though not significantly) with MCWD across plots (R2=0.35, p=0.16; Table 3). A negative, non-significant correlation existed between calibrated leaf lifespan and MCWD (R2=0.50, p=0.08). Photosynthetic capacity (Vcmax and Jmax) estimates derived from measured leaf N content similarly exhibited a negative non-significant correlation with MCWD (R2=0.51, p=0.07 and R2=0.53, p=0.06). A positive non-significant correlation existed between model-calibrated leaf lifespan, measured LMA (log–log: R2=0.39, p=0.14) and LAI (R2=0.28, p=0.22). Model-calibrated leaf lifespan exhibited a negative, non-significant correlation with photosynthetic capacity estimates (Vcmax: R2=0.46, p=0.09; Jmax: R2=0.47, p=0.09). A significant positive correlation existed between mean annual LAI and LMA (R2=0.85, p=0.003).
Table 5A comparison of GEM field measurements and SPA process-based modelling estimates of component autotrophic respiration and NPP. We present the R2, p value, and root mean square error (RMSE) of the interaction between SPA and GEM annual estimates, together with the range in GEM biometrically derived estimates across seven sample plots at four locations in the Amazon basin.
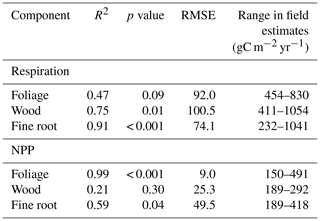
Table 6The proportion of variation in GPP across seven GEM Amazonian permanent sample plots explained by photosynthetic drivers in SPA. Model drivers were alternated individually at each plot with that of all other plots and the resultant change in GPP retrieved. Proportion of variance explained was calculated as the conditional sum of squares divided by the total sum of squares (n=476; where the conditions were LAI, photosynthetic capacity, rooting depth, root biomass, climate and soil).
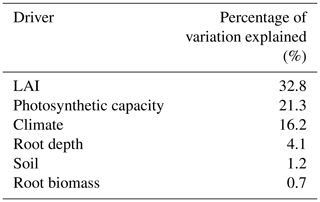
3.4 Model experiments
3.4.1 Experiment 1: drivers of spatial variation in GPP
Structural and trait responses to water availability explained more variation in GPP across the MCWD gradient than did climate. LAI accounted for the largest proportion of variance in mean annual GPP across plots (32.8 %, Table 6). Differences in photosynthetic capacity explained 21.3 % of variance. Photosynthetic capacity increased with decreasing MCWD; this relationship partially offset the decrease in GPP linked to declining LAI. The direct effects of climate on GPP (which included physiological responses to water availability including stomatal conductance) accounted for 16.2 % of plot variation in mean annual GPP. Rooting depth did not vary directionally with MCWD and consequently only had a small effect on GPP (4.1 %). Soil properties and root biomass accounted for a very small fraction of variance (< 2 %).
3.4.2 Experiment 2: variation in forest sensitivity to drivers of GPP
The relative sensitivity of GPP to drivers varied across the MCWD gradient (Fig. 6). GPP was most sensitive to changes in LAI (per unit m−2 leaf area) for plots located at Caxiuanã, which experience the least negative MCWD and have a large rooting depth. The sensitivity of GPP to LAI exhibited a positive, non-significant correlation with MCWD (R2=0.88, p=0.06). Reflecting LAI trends, the sensitivity of GPP to differences in photosynthetic capacity (per unit µmol C g s−1) was similarly highest at Caxiuanã and decreased linearly (though not significantly) across the MCWD gradient (R2=0.83, p=0.09). Tambopata plots, which have a high LAI but a shallow rooting depth, were most sensitive to differences in climate (per unit MCWD mm). Kenia plots, which have a similarly shallow rooting depth but a low LAI, were the least sensitive. The sensitivity of GPP to differences in rooting depth (per metre rooting depth) was highest at Tanguro and Tambopata and lowest at Caxiuanã and Kenia.
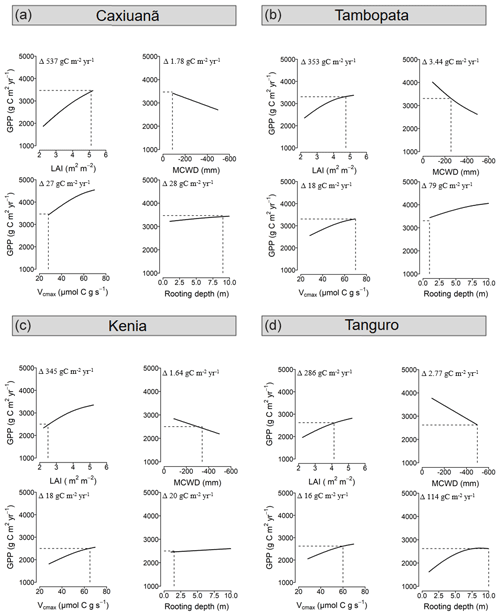
Figure 6The sensitivity of GPP to model driver alternations in SPA at each location. Model drivers LAI, climate (characterised by MCWD), photosynthetic capacity (characterised by Vcmax) and rooting depth, derived from field observations, were alternated individually at each plot with that of all other plots and the resultant GPP retrieved. Solid lines represent SPA-simulated GPP under the named driver alternations, and the dashed line represents the simulated value under observed conditions. SPA GPP estimates presented are location averages. Climate and LAI were input to the model as time series. Photosynthetic capacity and rooting depth were fixed values. Plots are ordered to reflect soil moisture stress which increases from Caxiuanã to Tambopata to Kenia and to Tanguro. The range in GPP estimates under each set of driver alternations for each location is presented (i.e. Δ values).
3.4.3 Experiment 3: drivers of sub-annual variation in GPP
In contrast to drivers of spatial variation in GPP, on a sub-annual timescale LAI had less explanatory power than climate (Tables 6 and 7). The relative importance of solar radiation in driving monthly GPP increased significantly with MCWD (R2=0.90, p=<0.001), as the relative importance of LAI declined (R2=0.72, p=0.015). The relative importance of VPD did not vary directionally across the MCWD gradient (R2=0.10, p=0.49). Both precipitation and air temperature had little effect on monthly GPP, though it is noted that a significant interaction existed between both precipitation and VPD (p<0.001) and air temperature and shortwave radiation (p<0.001). Furthermore, temperature varied least across plots in comparison to other climate forcings (standard deviation as a percentage of the mean; temperature 9.8 %, VPD 73 %, precipitation 192 %, shortwave radiation 34 %). As such, seasonal changes in the relative importance of temperature and precipitation were not investigated further. The relative importance of LAI, VPD and solar radiation shifted seasonally, reflecting changes in the availability of light and water. Solar radiation was typically the most important driver of monthly GPP during the wet season, whilst VPD was more important during the dry season (Fig. 7). The relative importance of LAI forcings peaked before dry season onset for forests under lower drought stress (Caxiuanã and Tambopata) and during the dry season for forests under higher drought stress (Kenia and Tanguro). Notably, LAI was also more important during the dry season at KEN02, which occupies shallow soil (< 1 m) in comparison to KEN01.
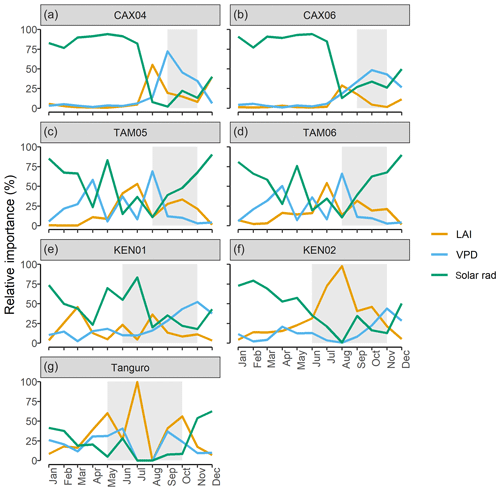
Figure 7The relative importance (%) of LAI, vapour pressure deficit (VPD) and solar radiation (solar rad) in driving SPA estimated monthly photosynthesis at permanent sample plots across an Amazon MCWD gradient. Relative importance was calculated using random forest machine learning. Shaded regions represent the dry season, where monthly precipitation was below 100 mm. Plots are ordered to reflect drought stress which increased from Caxiuanã to Tambopata to Kenia and to Tanguro.
4.1 LAI and leaf traits along the MCWD gradient
Leaf trait parameters retrieved from SPA litterfall calibrations suggest a wide range of potential leaf lifespans across the MCWD gradient (∼1–3 years) and are in accordance with estimates for Amazon tree species reported by Reich et al. (1991) of between 2 months and 2 years (Table 4). Leaf trait estimates co-varied across the MCWD gradient, in line with leaf economic theory (Wright et al., 2004). However, the interactions were often not significant. We suggest that, in instances where R2 values indicate a large proportion of variation is explained, high p values may have occurred as a result of a small sample size (i.e. 7 plots). As drought stress increased, a shift towards deciduous strategies resulted in reduced leaf lifespan but higher photosynthetic capacity. The co-variation of leaf traits along the MCWD gradient shapes both the rate of carbon assimilation (via photosynthetic capacity) and the carbon economics of canopy dynamics (via LMA, leaf lifespan and metabolic rate). Coincident with changes in leaf traits, mean annual LAI increased with decreasing drought stress. Research efforts have focused on mapping LAI (Iio et al., 2014) and leaf trait (Kattge et al., 2011; Asner et al., 2015) distributions across climatic gradients; however, their covariance has not yet been explored. Given the role of leaf traits in shaping canopy carbon economics, the mechanisms underpinning LAI and leaf trait distributions across the resource availability gradient could prove important in understanding the effect of changes in precipitation regime on future Amazon carbon dynamics.
4.2 Drivers of spatial variation in GPP
Indirect effects of climate via ecosystem structure and long-term trait responses to water availability accounted for 54 % of variation in GPP (Q1; Fig. 1). Direct effects of climate (which included physiological responses to water availability) accounted for only 16 % of observed variance (Table 6). Our results are consistent with previous reports on the importance of ecosystem structure and traits in determining spatial variation in GPP (Rodig et al., 2018; van de Weg et al., 2013; Reichstein et al., 2014) but go further to quantify the direct contribution of discrete drivers to observed variation in carbon assimilation. LAI explained the greatest proportion of variation in GPP, followed by photosynthetic capacity. Root and soil properties had little explanatory power.
Evidence of changes in LAI in response to precipitation regime has been presented across multiple ecosystems and over time (Grier and Running, 1977; Schleppi et al., 2011; Iio et al., 2014; Dobbertin et al., 2010; Wright et al., 2013). Amazonian forest throughfall exclusion experiments identified a decline in LAI with the onset of reduced soil water (Fisher et al., 2007; Meir et al., 2008; Brando et al., 2008). At Caxiuanã, over a 4-year period, observed leaf area was 20 %–30 % lower than the control stand (Meir et al., 2009), with long-term reductions estimated at between 10 % and 15 % (Rowland et al., 2015a). Investigations show that declines in LAI are not caused by increased leaf turnover due to drought stress but instead are the result of lower leaf production (Nepstad et al., 2002; Schuldt et al., 2011), suggesting an active response of plant allocation strategy to water availability. Concurrently, after 15 years under throughfall exclusion, Rowland et al. (2018) found that leaf litterfall still remained consistently lower. Reported trends in canopy dynamics are therefore in accordance with our findings and indicate that LAI is a key response mechanism to precipitation regime. Other studies such as da Costa et al. (2018) have similarly pointed towards structural responses as the principal determinant of variation in GPP; however, they identify changes in sapwood area as the main driver rather than LAI. We suggest that whilst sapwood area may be more important in shaping the response to short-term changes in precipitation, over longer timescales emergent canopy properties (LAI) drive GPP trends.
Photosynthetic capacity also proved an important driver of spatial variation in GPP across the MCWD gradient. Our results are consistent with a number of Amazon-based studies linking leaf traits to productivity (Aragao et al., 2009; Cleveland et al., 2011; Castanho et al., 2013). Interestingly, the observed shifts in photosynthetic capacity along the gradient had a compensatory effect on the GPP–MCWD interaction. Reductions in GPP under high drought stress were alleviated by higher photosynthetic capacitance. Similarly, shifts in photosynthetic capacity in response to temperature have been reported to reduce spatial variation in GPP across a tropical elevation gradient (Bahar et al., 2017; van de Weg et al., 2013). Consistent with Fyllas et al. (2017), our results also show that the effect of climatic forcings on carbon fluxes can be successfully captured through spatial variation in canopy dynamics and leaf traits. However, as we have focused on the role of leaf traits in the absence of carbon cycle feedbacks, we do not take into account the effect of concurrent shifts in LMA and leaf lifespan, which together influence canopy carbon economics (Wright et al., 2004; Osnas et al., 2013; McMurtrie and Dewar, 2011).
Root depth, root biomass and soil properties had little direct effect on spatial variation in GPP. We recognise that the difficulty in measuring root depth and biomass (Metcalfe et al., 2007) adds uncertainty to our results; however, the findings do not reflect the importance of belowground functioning highlighted by other studies (Fisher et al., 2007; Metcalfe et al., 2008; Baker et al., 2008; Phillips et al., 2009, Ichii et al., 2007). Notably, a number of GEM plots had hard pan layers (Quesada et al., 2012), so they may be acclimated to operate in shallow rooting zones and are therefore not necessarily representative of other Amazon forests under the same precipitation regime. Differences in root depth and biomass can alleviate water constraints to photosynthesis via the direct physiological pathway (i.e. stomatal conductance). But in the absence of C cycle feedbacks, changes in root depth and biomass do not drive changes in emergent canopy properties (i.e. LAI), which proved most important in determining GPP. It is therefore likely that if feedbacks were enabled within analyses, root and soil properties would prove to have a stronger effect.
4.3 Variation in forest sensitivity to drivers of GPP
The sensitivity of GPP to differences in LAI, climate, photosynthetic capacity and rooting depth varied across the MCWD gradient with evaporative potential and water uptake capacity (Q2; Fig. 6). As the model experiment was conducted in the absence of carbon cycle feedbacks, sensitivities reflect shorter rather than long-term effects of changes in forcings. The sensitivity of GPP to differences in LAI and photosynthetic capacity was greatest for forests occupying the lowest drought stress zone and declined with increasing drought stress. Our results are in agreement with findings from Wright et al. (2013), who reported that GPP was most sensitive to decreases in leaf area when water availability was highest. Forests with a high LAI (and therefore high evaporative potential) but a shallow rooting depth were most sensitive to differences in climate. Our results suggest that, where rooting depth is relatively shallow, and unable to ameliorate the effects of drought stress as seen elsewhere (Nepstad et al., 2007; Malhi et al., 2009), forests with a high LAI could be more vulnerable to reduced precipitation. Investigations into the vulnerability of Amazon forests to drought have put a deservedly large emphasis on the role of physiological responses (Choat et al., 2012; Phillips et al., 2009; Bennett et al., 2015; Corlett, 2016). However, our results indicate that the role of ecosystem structure could also prove important and that forests with a high evaporative potential (high LAI) but low water uptake capacity (shallow rooting depth) should be a focus for future studies.
4.4 Drivers of sub-annual variation in GPP
Seasonal (i.e. sub-annual) variation in GPP was driven by changes in solar radiation, VPD and LAI. The relative importance of these factors was dependent on MCWD (Q3; Fig. 7). Shortwave radiation was the dominant driver of sub-annual variation in GPP across plots, but its relative importance was greater for moister forests (Table 7). The relative importance of LAI in driving sub-annual GPP increased with drought stress. In accordance with our findings, a number of studies report that for Amazon forests in higher rainfall zones GPP increases in line with solar radiation and for forests subject to significantly low annual rainfall GPP declines with increased VPD (Von Randow et al., 2013; Goulden et al., 2004; Hutyra et al., 2007; Saleska et al., 2003; Rowland et al., 2014; Carswell et al., 2002). Our results suggest that LAI is not the principal driver of sub-annual variation in GPP, in contrast to its role in driving spatial variation across the MCWD gradient. However, while other studies agree that leaf area alone does not drive variation in sub-annual GPP (Wu et al., 2016, 2017; Brando et al., 2010; Restrepo-Coupe et al., 2013; Bi et al., 2015), we do not account for potential shifts in photosynthetic capacity with leaf age. The coordination of leaf age (via leaf flushing and new leaf emergence) with climatic drivers such as solar radiation is thought to exceed the effects of LAI in non-water limited forests (Myneni et al., 2007). We further recognise the uncertainty introduced through using leaf N content to derive photosynthetic capacity estimates (for five of the seven plots), given the distribution of leaf N between photosynthetic and non-photosynthetic proteins is not fixed (Onoda et al., 2017). However, notwithstanding temporal variation in photosynthetic capacity, we demonstrate that the relative importance of LAI dynamics and climatic forcing driving variation in GPP shifts with light and water availability.
Table 7The relative importance of LAI, VPD, solar radiation, precipitation and air temperature (Tair) in driving monthly variation in GPP (%). Monthly GPP estimates are derived from calibrated SPA simulations for seven permanent sample plots across an Amazon MCWD gradient, constrained using monthly field LAI measurements. Relative importance values were derived from analyses using the random forest technique (n=168).
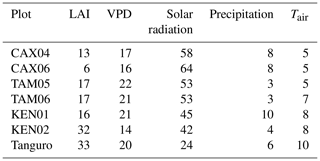
Our results indicate that, with respect to drought stress, annual GPP is constrained via indirect pathways (i.e. ecosystem structure and traits) across spatial scales but is limited via direct pathways (i.e. physiology) across sub-annual timescales (Fig. 1). In a study on net ecosystem exchange (NEE), Richardson et al. (2007) found that indirect pathways became progressively more important in driving NEE as the period of integration was lengthened (for a spruce-dominated forest in Maine, USA). The authors reasoned that the shift from direct to indirect pathways (as the period of integration transitions through day–week–month–seasonal–annual) reflected the timescales over which these processes operate. Stomata vary at hourly timescales with meteorology and soil conditions. The shift in direct to indirect pathways driving GPP variance reported here can similarly be explained by the difference in timescales over which responses to drought stress operate. LAI varies over monthly timescales, constrained by C and nutrient investment requirements over years. As a result, over monthly timescales, up to one-third of variation in GPP was explained by indirect effects of climate (i.e. LAI; Table 7), but direct effects (via physiological responses) remained the dominant driver (consistent with Richardson et al., 2007). Across the drought stress gradient, structural and trait responses to water availability (across annual to decadal timescales) result in indirect pathways dominating the GPP response, and the direct effects of climate are less important.
4.5 Limitations and opportunities
As nutrient dynamics are not directly accounted for in SPA, we are unable to quantify the impact of soil nutrients on the GPP–MCWD interaction. Soil nutrient availability varied widely across plots (Table 1). We recognise that nutrient limitation likely impacts GPP across the MCWD gradient, effected through both nutrient availability and plant acquisition capacity (which is dependent on moisture stress). However, there was no significant interaction between GPP and soil phosphorous (GEM: R2=0.1, p=0.48; SPA: R2=0.01, p=0.81) or soil nitrogen (GEM: R2=0.37, p=0.14; SPA: R2=0.31, p=0.19). Furthermore, we expect to capture soil nutrient effects via the inclusion of site-specific leaf nutrient estimates as a model inputs (which influence simulated photosynthetic and metabolic rate).
We recognise that the lack of significant correlation between SPA and GEM GPP estimates could impact the interpretation of our results. However, we argue that five of the seven plot estimates were within the error bounds of field measurements and that the inferential statistics used were limited by our small sample size (n=7). We also note that GEM GPP error estimates (calculated as the propagated standard error of component NPP and respiration measurements) do not account for assumptions used in scaling biometric measurements to plot level (e.g. uncertainty in using estimated total woody surface area to scale stem CO2 efflux measurements).
Given the importance of LAI in driving variation in GPP, data on canopy dynamics are critical to constrain carbon flux estimates across the Amazon basin. Our approach utilised field estimates of LAI from hemispherical photographs to constrain model simulations. The accuracy and spatial validity of indirect estimates of LAI has been questioned at higher leaf areas (Bréda, 2003; Jonckheere et al., 2004; Weiss et al., 2004). In this study, we expect that if field measurements of LAI were underestimated at higher leaf areas, the proportion of spatial variation in GPP explained by LAI would increase, as a result of increased variation in both field-measured and model-simulated GPP. Yet, our highest estimates of LAI (Caxiuanã 5.11±1.41 m2 m−2) align with destructive sampling measurements from a terra-firme Amazon forest (McWilliam et al., 1993 5.7±0.5 m2 m−2). Furthermore, a comparison of LAI estimation approaches (Asner et al., 2003) suggested that indirect methods were appropriate for broadleaved forests, and it presented no statistical difference between destructive harvesting and indirect methods. However, the use of ground measurements is limited to smaller spatial scales, and LAI estimates across the basin are needed to constrain carbon flux estimates. Though the interpretation of forest responses to drought stress through remote sensing approaches has caused controversy (Asner and Alencar, 2010; Saleska et al., 2007; Samanta et al., 2010), an increase in canopy mapping through satellite missions could be instrumental to efforts aiming to better understand LAI dynamics. Current and upcoming satellite missions including FLEX (Fluorescence Explorer), GEDI (Global Ecosystem Dynamics Investigation) and Sentinel will offer opportunity for new insights into changes in leaves in situ, vertical canopy structure and temporal variability via repeat measurements (Morton, 2016; Drusch et al., 2017; Pettorelli et al., 2018). Efforts to map trait distributions will also prove important (Kattge et al., 2011; Asner et al., 2015) given their role in driving variation in GPP.
We show that indirect effects of climate (via ecosystem structure and trait responses) exceed direct effects (via physiological responses) in driving spatial variation in GPP across an Amazon MCWD gradient (Q1). Conversely, across sub-annual timescales, the reverse was true (Q3). The relative sensitivity of GPP to changes in direct and indirect forcings shifted across the MCWD gradient and was dependent on water availability, demand and acquisition potential (Q2). We identify the potential vulnerability of forests with a high evaporative potential (i.e. high LAI), but low water uptake capacity (i.e. shallow rooting depth), to changes in precipitation regime. Given the role of LAI in driving GPP across the drought stress gradient, we highlight a requisite for improved mapping of canopy dynamics (via remote sensing). We propose that ecosystem model development should focus on the integration of structural and trait responses to drought stress (alongside physiological responses). The inclusion of both direct and indirect effects of climate in ecosystem models would reduce current uncertainty in predicted annual and sub-annual GPP for tropical forests.
Field data used in model calibration and validation are available at https://doi.org/10.1038/nature14213 (Doughty et al., 2015). Model runs used in experimental analysis can be found in this paper: https://doi.org/10.7488/ds/2710 (Flack-Prain et al., 2019).
The supplement related to this article is available online at: https://doi.org/10.5194/bg-16-4463-2019-supplement.
SFP, MW and PM conceived the research questions. Data used in model calibration and validation were collected by YM and associates. Model experiments were designed and conducted by SFP with contributions from MW and TLS. SFP and MW prepared the manuscript with active contributions from all co-authors.
The authors declare that they have no conflict of interest.
The authors would like to thank the Global Ecosystems Monitoring network team for the field data used in this study, collected through funding from NERC and the Gordon and Betty Moore Foundation, and an ERC Advanced Investigator Award to YM (GEM-TRAIT). The authors would also like to thank the PhD project funding body, the UK Natural Environment Research Council E3 DTP, the National Centre for Earth Observation, the UKSA project Forests 2020 and the Newton CSSP Brazil. The TRY trait database is thanked for the data used in model parameterisation. The authors would also like to thank the handling editor Andreas Ibrom and two anonymous reviewers for their helpful comments.
This research has been supported by the UK Natural Environment Research Council E3 DTP, the National Centre for Earth Observation, the UKSA project Forests 2020 and the Newton CSSP Brazil.
This paper was edited by Andreas Ibrom and reviewed by two anonymous referees.
Anderegg, W. R. L.: Spatial and temporal variation in plant hydraulic traits and their relevance for climate change impacts on vegetation, New Phytol., 205, 1008–1014, 2015.
Aragao, L. E. O. C., Malhi, Y., Roman-Cuesta, R. M., Saatchi, S., Anderson, L. O., and Shimabukuro, Y. E.: Spatial patterns and fire response of recent Amazonian droughts, Geophys. Res. Lett., 34, L07701, https://doi.org/10.1029/2006GL028946, 2007.
Aragao, L. E. O. C., Malhi, Y., Metcalfe, D. B., Silva-Espejo, J. E., Jiménez, E., Navarrete, D., Almeida, S., Costa, A. C. L., Salinas, N., Phillips, O. L., Anderson, L. O., Alvarez, E., Baker, T. R., Goncalvez, P. H., Huamán-Ovalle, J., Mamani-Solórzano, M., Meir, P., Monteagudo, A., Patiño, S., Peñuela, M. C., Prieto, A., Quesada, C. A., Rozas-Dávila, A., Rudas, A., Silva Jr., J. A., and Vásquez, R.: Above- and below-ground net primary productivity across ten Amazonian forests on contrasting soils, Biogeosciences, 6, 2759–2778, https://doi.org/10.5194/bg-6-2759-2009, 2009.
Asner, G. P. and Alencar, A.: Drought impacts on the Amazon forest: the remote sensing perspective, New Phytol., 187, 569–578, 2010.
Asner, G. P., Scurlock, J. M. O., and Hicke, J. A.: Global synthesis of leaf area index observations: implications for ecological and remote sensing studies, Global Ecol. Biogeogr., 12, 191–205, 2003.
Asner, G. P., Martin, R. E., Anderson, C. B., and Knapp, D. E.: Quantifying forest canopy traits: Imaging spectroscopy versus field survey, Remote Sens. Environ., 158, 15–27, 2015.
Bahar, N. H., Ishida, F. Y., Weerasinghe, L. K., Guerrieri, R., O'Sullivan, O. S., Bloomfield, K. J., Asner, G. P., Martin, R. E., Lloyd, J., Malhi, Y., Phillips, O. L., Meir, P., Salinas, N., Cosio, E. G., Domingues, T. F., Quesada, C. A., Sinca, F., Escudero Vega, A., Zuloaga Ccorimanya, P. P., Del Aguila-Pasquel, J., Quispe Huaypar, K., Cuba Torres, I., Butron Loayza, R., Pelaez Tapia, Y., Huaman Ovalle, J., Long, B. M., Evans, J. R., and Atkin, O. K.: Leaf-level photosynthetic capacity in lowland Amazonian and high-elevation Andean tropical moist forests of Peru, New Phytol., 214, 1002–1018, 2017.
Baker, I. T., Prihodko, L., Denning, A. S., Goulden, M., Miller, S., and da Rocha, H. R.: Seasonal drought stress in the Amazon: Reconciling models and observations, J. Geophys. Res.-Biogeo., 113, G00B01, https://doi.org/10.1029/2007JG000644, 2008.
Beer, C., Reichstein, M., Tomelleri, E., Ciais, P., Jung, M., Carvalhais, N., Rödenbeck, C., Arain, M. A., Baldocchi, D., and Bonan, G. B.: Terrestrial gross carbon dioxide uptake: global distribution and covariation with climate, Science, 329, 834–838, 2010.
Bennett, A. C., McDowell, N. G., Allen, C. D., and Anderson-Teixeira, K. J.: Larger trees suffer most during drought in forests worldwide, Nat. Plants, 1, 15139, https://doi.org/10.1038/nplants.2015.139, 2015.
Bi, J., Knyazikhin, Y., Choi, S. H., Park, T., Barichivich, J., Ciais, P., Fu, R., Ganguly, S., Hall, F., Hilker, T., Huete, A., Jones, M., Kimball, J., Lyapustin, A. I., Mottus, M., Nemani, R. R., Piao, S. L., Poulter, B., Saleska, S. R., Saatchi, S. S., Xu, L., Zhou, L. M., and Myneni, R. B.: Sunlight mediated seasonality in canopy structure and photosynthetic activity of Amazonian rainforests, Environ. Res. Lett., 10, 064014, https://doi.org/10.1088/1748-9326/10/6/064014, 2015.
Bloom, A. A. and Williams, M.: Constraining ecosystem carbon dynamics in a data-limited world: integrating ecological “common sense” in a model-data fusion framework, Biogeosciences, 12, 1299–1315, https://doi.org/10.5194/bg-12-1299-2015, 2015.
Bloom, A. J., Chapin, F. S., and Mooney, H. A.: Resource Limitation in Plants – an Economic Analogy, Annu. Rev. Ecol. Syst., 16, 363–392, 1985.
Boisier, J. P., Ciais, P., Ducharne, A., and Guimberteau, M.: Projected strengthening of Amazonian dry season by constrained climate model simulations, Nat. Clim. Change, 5, 656–660, 2015.
Bonan, G. B., Williams, M., Fisher, R. A., and Oleson, K. W.: Modeling stomatal conductance in the earth system: linking leaf water-use efficiency and water transport along the soil-plant-atmosphere continuum, Geosci. Model Dev., 7, 2193–2222, https://doi.org/10.5194/gmd-7-2193-2014, 2014.
Brando, P. M., Nepstad, D. C., Davidson, E. A., Trumbore, S. E., Ray, D., and Camargo, P.: Drought effects on litterfall, wood production and belowground carbon cycling in an Amazon forest: results of a throughfall reduction experiment, Philos. T. R. Soc. B, 363, 1839–1848, 2008.
Brando, P. M., Goetz, S. J., Baccini, A., Nepstad, D. C., Beck, P. S., and Christman, M. C.: Seasonal and interannual variability of climate and vegetation indices across the Amazon, P. Natl. Acad. Sci. USA, 107, 14685–14690, 2010.
Bréda, N. J. J.: Ground-based measurements of leaf area index: a review of methods, instruments and current controversies, J. Exp. Bot., 54, 2403–2417, 2003.
Breiman, L.: Random forests, Mach. Learn., 45, 5–32, 2001.
Carswell, F. E., Costa, A. L., Palheta, M., Malhi, Y., Meir, P., Costa, J. D. R., Ruivo, M. D., Leal, L. D. M., Costa, J. M. N., Clement, R. J., and Grace, J.: Seasonality in CO2 and H2O flux at an eastern Amazonian rain forest, J. Geophys. Res.-Atmos, 107, D208076, https://doi.org/10.1029/2000JD000284, 2002.
Castanho, A. D. A., Coe, M. T., Costa, M. H., Malhi, Y., Galbraith, D., and Quesada, C. A.: Improving simulated Amazon forest biomass and productivity by including spatial variation in biophysical parameters, Biogeosciences, 10, 2255–2272, https://doi.org/10.5194/bg-10-2255-2013, 2013.
Choat, B., Jansen, S., Brodribb, T. J., Cochard, H., Delzon, S., Bhaskar, R., Bucci, S. J., Feild, T. S., Gleason, S. M., Hacke, U. G., Jacobsen, A. L., Lens, F., Maherali, H., Martinez-Vilalta, J., Mayr, S., Mencuccini, M., Mitchell, P. J., Nardini, A., Pittermann, J., Pratt, R. B., Sperry, J. S., Westoby, M., Wright, I. J., and Zanne, A. E.: Global convergence in the vulnerability of forests to drought, Nature, 491, 752–755, 2012.
Cleveland, C. C., Townsend, A. R., Taylor, P., Alvarez-Clare, S., Bustamante, M. M. C., Chuyong, G., Dobrowski, S. Z., Grierson, P., Harms, K. E., and Houlton, B. Z.: Relationships among net primary productivity, nutrients and climate in tropical rain forest: a pan-tropical analysis, Ecol. Lett., 14, 939–947, 2011.
Corlett, R. T.: The Impacts of Droughts in Tropical Forests, Trends Plant Sci., 21, 584–593, 2016.
da Costa, A. C. L., Rowland, L., Oliveira, R. S., Oliveira, A. A. R., Binks, O. J., Salmon, Y., Vasconcelos, S. S., Junior, J. A. S., Ferreira, L. V., Poyatos, R., Mencuccini, M., and Meir, P.: Stand dynamics modulate water cycling and mortality risk in droughted tropical forest, Glob. Change Biol., 24, 249–258, 2018.
Dee, D. P., Uppala, S. M., Simmons, A. J., Berrisford, P., Poli, P., Kobayashi, S., Andrae, U., Balmaseda, M. A., Balsamo, G., and Bauer, P.: The ERA-Interim reanalysis: Configuration and performance of the data assimilation system, Q. J. Roy. Meteor. Soc., 137, 553–597, 2011.
Dietze, M. C., Sala, A., Carbone, M. S., Czimczik, C. I., Mantooth, J. A., Richardson, A. D., and Vargas, R.: Nonstructural carbon in woody plants, Annu. Rev. Plant Biol., 65, 667–687, 2014.
Dobbertin, M., Eilmann, B., Bleuler, P., Giuggiola, A., Graf Pannatier, E., Landolt, W., Schleppi, P., and Rigling, A.: Effect of irrigation on needle morphology, shoot and stem growth in a drought-exposed Pinus sylvestris forest, Tree Physiol., 30, 346–360, 2010.
Doughty, C. E., Metcalfe, D. B., Girardin, C. A., Amezquita, F. F., Cabrera, D. G., Huasco, W. H., Silva-Espejo, J. E., Araujo-Murakami, A., da Costa, M. C., Rocha, W., Feldpausch, T. R., Mendoza, A. L., da Costa, A. C., Meir, P., Phillips, O. L., and Malhi, Y.: Drought impact on forest carbon dynamics and fluxes in Amazonia, Nature, 519, 78–82, https://doi.org/10.1038/nature14213, 2015.
Drusch, M., Moreno, J., Del Bello, U., Franco, R., Goulas, Y., Huth, A., Kraft, S., Middleton, E. M., Miglietta, F., and Mohammed, G.: The FLuorescence EXplorer Mission Concept – ESA's Earth Explorer 8, IEEE T. Geosci. Remote, 55, 1273–1284, 2017.
Duffy, P. B., Brando, P., Asner, G. P., and Field, C. B.: Projections of future meteorological drought and wet periods in the Amazon, P. Natl. Acad. Sci. USA, 112, 13172–13177, 2015.
Eller, C. B., Rowland, L., Oliveira, R. S., Bittencourt, P. R. L., Barros, F. V., da Costa, A. C. L., Meir, P., Friend, A. D., Mencuccini, M., Sitch, S., and Cox, P.: Modelling tropical forest responses to drought and El Nino with a stomatal optimization model based on xylem hydraulics, Philos. T. R. Soc. B., 373, 20170315, https://doi.org/10.1098/rstb.2017.0315, 2018.
Fauset, S., Baker, T. R., Lewis, S. L., Feldpausch, T. R., Affum-Baffoe, K., Foli, E. G., Hamer, K. C., and Swaine, M. D.: Drought-induced shifts in the floristic and functional composition of tropical forests in Ghana, Ecol. Lett., 15, 1120–1129, 2012.
Fisher, R. A., Williams, M., Do, V., Lobo, R., Da Costa, A. L., and Meir, P.: Evidence from Amazonian forests is consistent with isohydric control of leaf water potential, Plant Cell Environ., 29, 151–165, 2006.
Fisher, R. A., Williams, M., Da Costa, A. L., Malhi, Y., Da Costa, R. F., Almeida, S., and Meir, P.: The response of an Eastern Amazonian rain forest to drought stress: results and modelling analyses from a throughfall exclusion experiment, Glob. Change Biol., 13, 2361–2378, 2007.
Fisher, R. A., Koven, C. D., Anderegg, W. R. L., Christoffersen, B. O., Dietze, M. C., Farrior, C. E., Holm, J. A., Hurtt, G. C., Knox, R. G., Lawrence, P. J., Lichstein, J. W., Longo, M., Matheny, A. M., Medvigy, D., Muller-Landau, H. C., Powell, T. L., Serbin, S. P., Sato, H., Shuman, J. K., Smith, B., Trugman, A. T., Viskari, T., Verbeeck, H., Weng, E., Xu, C., Xu, X., Zhang, T., and Moorcroft, P. R.: Vegetation demographics in Earth System Models: A review of progress and priorities, Glob. Change Biol., 24, 35–54, 2018.
Flack-Prain, S., Meir, P., Malhi, Y., Smallman, T., and Williams, M.: The importance of physiological, structural and trait responses to drought stress in driving spatial and temporal variation in GPP across Amazon forests, University of Edinburgh, School of Geosciences, https://doi.org/10.7488/ds/2710, 2019.
Fyllas, N. M., Patiño, S., Baker, T. R., Bielefeld Nardoto, G., Martinelli, L. A., Quesada, C. A., Paiva, R., Schwarz, M., Horna, V., Mercado, L. M., Santos, A., Arroyo, L., Jiménez, E. M., Luizão, F. J., Neill, D. A., Silva, N., Prieto, A., Rudas, A., Silviera, M., Vieira, I. C. G., Lopez-Gonzalez, G., Malhi, Y., Phillips, O. L., and Lloyd, J.: Basin-wide variations in foliar properties of Amazonian forest: phylogeny, soils and climate, Biogeosciences, 6, 2677–2708, https://doi.org/10.5194/bg-6-2677-2009, 2009.
Fyllas, N. M., Bentley, L. P., Shenkin, A., Asner, G. P., Atkin, O. K., Diaz, S., Enquist, B. J., Farfan-Rios, W., Gloor, E., Guerrieri, R., Huasco, W. H., Ishida, Y., Martin, R. E., Meir, P., Phillips, O., Salinas, N., Silman, M., Weerasinghe, L. K., Zaragoza-Castells, J., and Malhi, Y.: Solar radiation and functional traits explain the decline of forest primary productivity along a tropical elevation gradient, Ecol. Lett., 20, 730–740, 2017.
Galbraith, D., Levy, P. E., Sitch, S., Huntingford, C., Cox, P., Williams, M., and Meir, P.: Multiple mechanisms of Amazonian forest biomass losses in three dynamic global vegetation models under climate change, New Phytol., 187, 647–665, 2010.
Goulden, M. L., Miller, S. D., da Rocha, H. R., Menton, M. C., de Freitas, H. C., Figueira, A. M. E. S., and de Sousa, C. A. D.: Diel and seasonal patterns of tropical forest CO2 exchange, Ecol. Appl., 14, S42–S54, 2004.
Green, J. K., Seneviratne, S. I., Berg, A. M., Findell, K. L., Hagemann, S., Lawrence, D. M., and Gentine, P.: Large influence of soil moisture on long-term terrestrial carbon uptake, Nature, 565, 476–479, 2019.
Grier, C. C. and Running, S. W.: Leaf Area of Mature Northwestern Coniferous Forests – Relation to Site Water-Balance, Ecology, 58, 893–899, 1977.
Guan, K., Pan, M., Li, H., Wolf, A., Wu, J., Medvigy, D., Caylor, K. K., Sheffield, J., Wood, E. F., Malhi, Y., and Liang, M.: Photosynthetic seasonality of global tropical forests constrained by hydroclimate, Nat. Geosci., 8, 284–289, 2015.
Hutyra, L. R., Munger, J. W., Saleska, S. R., Gottlieb, E., Daube, B. C., Dunn, A. L., Amaral, D. F., De Camargo, P. B., and Wofsy, S. C.: Seasonal controls on the exchange of carbon and water in an Amazonian rain forest, J. Geophys. Res.-Biogeo., 112, G03008, https://doi.org/10.1029/2006JG000365, 2007.
Ichii, K., Hashimoto, H., White, M. A., Potters, C., Hutyra, L. R., Huete, A. R., Myneni, R. B., and Nemanis, R. R.: Constraining rooting depths in tropical rainforests using satellite data and ecosystem modeling for accurate simulation of gross primary production seasonality, Glob. Change Biol., 13, 67–77, 2007.
Iio, A., Hikosaka, K., Anten, N. P. R., Nakagawa, Y., and Ito, A.: Global dependence of field-observed leaf area index in woody species on climate: a systematic review, Global Ecol. Biogeogr., 23, 274–285, 2014.
Joetzjer, E., Douville, H., Delire, C., and Ciais, P.: Present-day and future Amazonian precipitation in global climate models: CMIP5 versus CMIP3, Clim. Dynam., 41, 2921–2936, 2013.
Jonckheere, I., Fleck, S., Nackaerts, K., Muys, B., Coppin, P., Weiss, M., and Baret, F.: Review of methods for in situ leaf area index determination – Part I, Theories, sensors and hemispherical photography, Agr. Forest Meteorol., 121, 19–35, 2004.
Kattge, J., Diaz, S., Lavorel, S., Prentice, I. C., Leadley, P., Bönisch, G., Garnier, E., Westoby, M., Reich, P. B., and Wright, I. J.: TRY–a global database of plant traits, Glob. Change Biol., 17, 2905–2935, 2011.
Lee, J. E., Frankenberg, C., van der Tol, C., Berry, J. A., Guanter, L., Boyce, C. K., Fisher, J. B., Morrow, E., Worden, J. R., Asefi, S., Badgley, G., and Saatchi, S.: Forest productivity and water stress in Amazonia: observations from GOSAT chlorophyll fluorescence, P. R. Soc. B., 280, 20130171, https://doi.org/10.1098/rspb.2013.0171, 2013.
Liu, J., Bowman, K. W., Schimel, D. S., Parazoo, N. C., Jiang, Z., Lee, M., Bloom, A. A., Wunch, D., Frankenberg, C., and Sun, Y.: Contrasting carbon cycle responses of the tropical continents to the 2015–2016 El Niño, Science, 358, eaam5690, https://doi.org/10.1126/science.aam5690, 2017.
López-Blanco, E., Lund, M., Williams, M., Tamstorf, M. P., Westergaard-Nielsen, A., Exbrayat, J.-F., Hansen, B. U., and Christensen, T. R.: Exchange of CO2 in Arctic tundra: impacts of meteorological variations and biological disturbance, Biogeosciences, 14, 4467–4483, https://doi.org/10.5194/bg-14-4467-2017, 2017.
Malhi, Y., Roberts, J. T., Betts, R. A., Killeen, T. J., Li, W., and Nobre, C. A.: Climate change, deforestation, and the fate of the Amazon, Science, 319, 169–172, 2008.
Malhi, Y., Aragao, L. E., Galbraith, D., Huntingford, C., Fisher, R., Zelazowski, P., Sitch, S., McSweeney, C., and Meir, P.: Exploring the likelihood and mechanism of a climate-change-induced dieback of the Amazon rainforest, P. Natl. Acad. Sci. USA, 106, 20610–20615, 2009.
Malhi, Y., Doughty, C. E., Goldsmith, G. R., Metcalfe, D. B., Girardin, C. A. J., Marthews, T. R., del Aguila-Pasquel, J., Aragao, L. E. O. C., Araujo-Murakami, A., Brando, P., da Costa, A. C. L., Silva-Espejo, J. E., Amezquita, F. F., Galbraith, D. R., Quesada, C. A., Rocha, W., Salinas-Revilla, N., Silverio, D., Meir, P., and Phillips, O. L.: The linkages between photosynthesis, productivity, growth and biomass in lowland Amazonian forests, Glob. Change Biol., 21, 2283–2295, 2015.
McMurtrie, R. E. and Dewar, R. C.: Leaf-trait variation explained by the hypothesis that plants maximize their canopy carbon export over the lifespan of leaves, Tree Physiol., 31, 1007–1023, 2011.
McWilliam, A. L., Roberts, J. M., Cabral, O. M. R., Leitao, M., De Costa, A. C. L., Maitelli, G. T., and Zamparoni, C.: Leaf area index and above-ground biomass of terra firme rain forest and adjacent clearings in Amazonia, Funct. Ecol., 7, 310–317, 1993.
Meir, P. and Woodward, F. I.: Amazonian rain forests and drought: response and vulnerability, New Phytol., 187, 553–557, 2010.
Meir, P., Metcalfe, D. B., Costa, A. C., and Fisher, R. A.: The fate of assimilated carbon during drought: impacts on respiration in Amazon rainforests, Philos. T. R. Soc. B, 363, 1849–1855, 2008.
Meir, P., Brando, P. M., Nepstad, D., Vasconcelos, S., Costa, A. C. L., Davidson, E., Almeida, S., Fisher, R. A., Sotta, E. D., and Zarin, D.: The effects of drought on Amazonian rain forests, Amazonia and Global Change, 186, 429–449, 2009.
Meir, P., Mencuccini, M., and Dewar, R. C.: Drought-related tree mortality: addressing the gaps in understanding and prediction, New Phytol., 207, 28–33, 2015a.
Meir, P., Wood, T. E., Galbraith, D. R., Brando, P. M., Da Costa, A. C., Rowland, L., and Ferreira, L. V.: Threshold Responses to Soil Moisture Deficit by Trees and Soil in Tropical Rain Forests: Insights from Field Experiments, Bioscience, 65, 882–892, 2015b.
Mercado, L. M., Patino, S., Domingues, T. F., Fyllas, N. M., Weedon, G. P., Sitch, S., Quesada, C. A., Phillips, O. L., Aragao, L. E., Malhi, Y., Dolman, A. J., Restrepo-Coupe, N., Saleska, S. R., Baker, T. R., Almeida, S., Higuchi, N., and Lloyd, J.: Variations in Amazon forest productivity correlated with foliar nutrients and modelled rates of photosynthetic carbon supply, Philos. T. R. Soc. B, 366, 3316–3329, 2011.
Metcalfe, D. B., Meir, P., Aragao, L., Malhi, Y., Da Costa, A. C. L., Braga, A., Gonçalves, P. H. L., de Athaydes, J., De Almeida, S. S., and Williams, M.: Factors controlling spatio-temporal variation in carbon dioxide efflux from surface litter, roots, and soil organic matter at four rain forest sites in the eastern Amazon, J. Geophys. Res.-Biogeo., 112, G04001, https://doi.org/10.1029/2007JG000443, 2007.
Metcalfe, D. B., Meir, P., Aragao, L. E. O. C., da Costa, A. C. L., Braga, A. P., Goncalves, P. H. L., Silva, J. D., de Almeida, S. S., Dawson, L. A., Malhi, Y., and Williams, M.: The effects of water availability on root growth and morphology in an Amazon rainforest, Plant Soil, 311, 189–199, 2008.
Moore, C. E., Beringer, J., Donohue, R. J., Evans, B., Exbrayat, J. F., Hutley, L. B., and Tapper, N. J.: Seasonal, interannual and decadal drivers of tree and grass productivity in an Australian tropical savanna, Glob. Change Biol., 24, 2530–2544, 2018.
Morton, D. C.: FOREST CARBON FLUXES A satellite perspective, Nat. Clim. Change, 6, 346–348, 2016.
Myneni, R. B., Yang, W., Nemani, R. R., Huete, A. R., Dickinson, R. E., Knyazikhin, Y., Didan, K., Fu, R., Negron Juarez, R. I., Saatchi, S. S., Hashimoto, H., Ichii, K., Shabanov, N. V., Tan, B., Ratana, P., Privette, J. L., Morisette, J. T., Vermote, E. F., Roy, D. P., Wolfe, R. E., Friedl, M. A., Running, S. W., Votava, P., El-Saleous, N., Devadiga, S., Su, Y., and Salomonson, V. V.: Large seasonal swings in leaf area of Amazon rainforests, P. Natl. Acad. Sci. USA, 104, 4820–4823, 2007.
Nepstad, D. C., Decarvalho, C. R., Davidson, E. A., Jipp, P. H., Lefebvre, P. A., Negreiros, G. H., Dasilva, E. D., Stone, T. A., Trumbore, S. E., and Vieira, S.: The Role of Deep Roots in the Hydrological and Carbon Cycles of Amazonian Forests and Pastures, Nature, 372, 666–669, 1994.
Nepstad, D. C., Moutinho, P., Dias, M. B., Davidson, E., Cardinot, G., Markewitz, D., Figueiredo, R., Vianna, N., Chambers, J., Ray, D., Guerreiros, J. B., Lefebvre, P., Sternberg, L., Moreira, M., Barros, L., Ishida, F. Y., Tohlver, I., Belk, E., Kalif, K., and Schwalbe, K.: The effects of partial throughfall exclusion on canopy processes, aboveground production, and biogeochemistry of an Amazon forest, J. Geophys. Res.-Atmos, 107, D208085, https://doi.org/10.1029/2001JD000360, 2002.
Nepstad, D. C., Tohver, I. M., Ray, D., Moutinho, P., and Cardinot, G.: Mortality of large trees and lianas following experimental drought in an Amazon forest, Ecology, 88, 2259–2269, 2007.
O'Brien, M. J., Leuzinger, S., Philipson, C. D., Tay, J., and Hector, A.: Drought survival of tropical tree seedlings enhanced by non-structural carbohydrate levels, Nat. Clim. Change, 4, 710–740, 2014.
Oliveira, R. S., Dawson, T. E., Burgess, S. S., and Nepstad, D. C.: Hydraulic redistribution in three Amazonian trees, Oecologia, 145, 354–363, 2005.
Onoda, Y., Wright, I. J., Evans, J. R., Hikosaka, K., Kitajima, K., Niinemets, Ü., Poorter, H., Tosens, T., and Westoby, M.: Physiological and structural tradeoffs underlying the leaf economics spectrum, New Phytol., 214, 1447–1463, 2017.
Osnas, J. L., Lichstein, J. W., Reich, P. B., and Pacala, S. W.: Global leaf trait relationships: mass, area, and the leaf economics spectrum, Science, 340, 741–744, 2013.
Pedregosa, F., Varoquaux, G., Gramfort, A., Michel, V., Thirion, B., Grisel, O., Blondel, M., Prettenhofer, P., Weiss, R., Dubourg, V., Vanderplas, J., Passos, A., Cournapeau, D., Brucher, M., Perrot, M., and Duchesnay, E.: Scikit-learn: Machine Learning in Python, J. Mach. Learn Res., 12, 2825–2830, 2011.
Pettorelli, N., Buhne, H. S. T., Tulloch, A., Dubois, G., Macinnis-Ng, C., Queiros, A. M., Keith, D. A., Wegmann, M., Schrodt, F., Stellmes, M., Sonnenschein, R., Geller, G. N., Roy, S., Somers, B., Murray, N., Bland, L., Geijzendorffer, I., Kerr, J. T., Broszeit, S., Leitao, P. J., Duncan, C., El Serafy, G., He, K. S., Blanchard, J. L., Lucas, R., Mairota, P., Webb, T. J., and Nicholson, E.: Satellite remote sensing of ecosystem functions: opportunities, challenges and way forward, Remote Sens. Ecol. Conserv., 4, 71–93, 2018.
Phillips, O. L., Aragao, L. E., Lewis, S. L., Fisher, J. B., Lloyd, J., Lopez-Gonzalez, G., Malhi, Y., Monteagudo, A., Peacock, J., Quesada, C. A., van der Heijden, G., Almeida, S., Amaral, I., Arroyo, L., Aymard, G., Baker, T. R., Banki, O., Blanc, L., Bonal, D., Brando, P., Chave, J., de Oliveira, A. C., Cardozo, N. D., Czimczik, C. I., Feldpausch, T. R., Freitas, M. A., Gloor, E., Higuchi, N., Jimenez, E., Lloyd, G., Meir, P., Mendoza, C., Morel, A., Neill, D. A., Nepstad, D., Patino, S., Penuela, M. C., Prieto, A., Ramirez, F., Schwarz, M., Silva, J., Silveira, M., Thomas, A. S., Steege, H. T., Stropp, J., Vasquez, R., Zelazowski, P., Alvarez Davila, E., Andelman, S., Andrade, A., Chao, K. J., Erwin, T., Di Fiore, A., Honorio, C. E., Keeling, H., Killeen, T. J., Laurance, W. F., Pena Cruz, A., Pitman, N. C., Nunez Vargas, P., Ramirez-Angulo, H., Rudas, A., Salamao, R., Silva, N., Terborgh, J., and Torres-Lezama, A.: Drought sensitivity of the Amazon rainforest, Science, 323, 1344–1347, 2009.
Poorter, L. and Bongers, F.: Leaf traits are good predictors of plant performance across 53 rain forest species, Ecology, 87, 1733–1743, 2006.
Powell, T. L., Galbraith, D. R., Christoffersen, B. O., Harper, A., Imbuzeiro, H. M., Rowland, L., Almeida, S., Brando, P. M., da Costa, A. C., Costa, M. H., Levine, N. M., Malhi, Y., Saleska, S. R., Sotta, E., Williams, M., Meir, P., and Moorcroft, P. R.: Confronting model predictions of carbon fluxes with measurements of Amazon forests subjected to experimental drought, New Phytol., 200, 350–365, 2013.
Quesada, C. A., Phillips, O. L., Schwarz, M., Czimczik, C. I., Baker, T. R., Patiño, S., Fyllas, N. M., Hodnett, M. G., Herrera, R., Almeida, S., Alvarez Dávila, E., Arneth, A., Arroyo, L., Chao, K. J., Dezzeo, N., Erwin, T., di Fiore, A., Higuchi, N., Honorio Coronado, E., Jimenez, E. M., Killeen, T., Lezama, A. T., Lloyd, G., López-González, G., Luizão, F. J., Malhi, Y., Monteagudo, A., Neill, D. A., Núñez Vargas, P., Paiva, R., Peacock, J., Peñuela, M. C., Peña Cruz, A., Pitman, N., Priante Filho, N., Prieto, A., Ramírez, H., Rudas, A., Salomão, R., Santos, A. J. B., Schmerler, J., Silva, N., Silveira, M., Vásquez, R., Vieira, I., Terborgh, J., and Lloyd, J.: Basin-wide variations in Amazon forest structure and function are mediated by both soils and climate, Biogeosciences, 9, 2203–2246, https://doi.org/10.5194/bg-9-2203-2012, 2012.
Reich, P. B., Uhl, C., Walters, M. B., and Ellsworth, D. S.: Leaf lifespan as a determinant of leaf structure and function among 23 Amazonian tree species, Oecologia, 86, 16–24, 1991.
Reich, P. B., Tjoelker, M. G., Pregitzer, K. S., Wright, I. J., Oleksyn, J., and Machado, J. L.: Scaling of respiration to nitrogen in leaves, stems and roots of higher land plants, Ecol. Lett., 11, 793–801, 2008.
Reichstein, M., Bahn, M., Mahecha, M. D., Kattge, J., and Baldocchi, D. D.: Linking plant and ecosystem functional biogeography, P. Natl. Acad. Sci. USA, 111, 13697–13702, 2014.
Restrepo-Coupe, N., da Rocha, H. R., Hutyra, L. R., da Araujo, A. C., Borma, L. S., Christoffersen, B., Cabral, O. M. R., de Camargo, P. B., Cardoso, F. L., and da Costa, A. C. L.: What drives the seasonality of photosynthesis across the Amazon basin? A cross-site analysis of eddy flux tower measurements from the Brasil flux network, Agr. Forest Meteorol., 182, 128–144, 2013.
Restrepo-Coupe, N., Levine, N. M., Christoffersen, B. O., Albert, L. P., Wu, J., Costa, M. H., Galbraith, D., Imbuzeiro, H., Martins, G., and Araujo, A. C.: Do dynamic global vegetation models capture the seasonality of carbon fluxes in the Amazon basin? A data-model intercomparison, Glob. Change Biol., 23, 191–208, 2017.
Richardson, A. D., Hollinger, D. Y., Aber, J. D., Ollinger, S. V., and Braswell, B. H.: Environmental variation is directly responsible for short-but not long-term variation in forest-atmosphere carbon exchange, Glob. Change Biol., 13, 788–803, 2007.
Rodig, E., Cuntz, M., Rammig, A., Fischer, R., Taubert, F., and Huth, A.: The importance of forest structure for carbon fluxes of the Amazon rainforest, Environ. Res. Lett., 13, 054013, https://doi.org/10.1088/1748-9326/aabc61, 2018.
Rowland, L., Hill, T. C., Stahl, C., Siebicke, L., Burban, B., Zaragoza-Castells, J., Ponton, S., Bonal, D., Meir, P., and Williams, M.: Evidence for strong seasonality in the carbon storage and carbon use efficiency of an Amazonian forest, Glob. Change Biol., 20, 979–991, 2014.
Rowland, L., Da Costa, A. C. L., Galbraith, D. R., Oliveira, R. S., Binks, O. J., Oliveira, A. A. R., Pullen, A. M., Doughty, C. E., Metcalfe, D. B., and Vasconcelos, S. S.: Death from drought in tropical forests is triggered by hydraulics not carbon starvation, Nature, 528, 119–122, 2015a.
Rowland, L., Harper, A., Christoffersen, B. O., Galbraith, D. R., Imbuzeiro, H. M. A., Powell, T. L., Doughty, C., Levine, N. M., Malhi, Y., Saleska, S. R., Moorcroft, P. R., Meir, P., and Williams, M.: Modelling climate change responses in tropical forests: similar productivity estimates across five models, but different mechanisms and responses, Geosci. Model Dev., 8, 1097–1110, https://doi.org/10.5194/gmd-8-1097-2015, 2015b.
Rowland, L., da Costa, A. C. L., Oliveira, A. A. R., Almeida, S. S., Ferreira, L. V., Malhi, Y., Metcalfe, D. B., Mencuccini, M., Grace, J., and Meir, P.: Shock and stabilisation following long-term drought in tropical forest from 15 years of litterfall dynamics, J. Ecol., 106, 1673–1682, 2018.
Sakschewski, B., von Bloh, W., Boit, A., Poorter, L., Pena-Claros, M., Heinke, J., Joshi, J., and Thonicke, K.: Resilience of Amazon forests emerges from plant trait diversity, Nat. Clim. Change, 6, 1032–1036, 2016.
Saleska, S. R., Miller, S. D., Matross, D. M., Goulden, M. L., Wofsy, S. C., da Rocha, H. R., de Camargo, P. B., Crill, P., Daube, B. C., de Freitas, H. C., Hutyra, L., Keller, M., Kirchhoff, V., Menton, M., Munger, J. W., Pyle, E. H., Rice, A. H., and Silva, H.: Carbon in Amazon forests: unexpected seasonal fluxes and disturbance-induced losses, Science, 302, 1554–1557, 2003.
Saleska, S. R., Didan, K., Huete, A. R., and da Rocha, H. R.: Amazon forests green-up during 2005 drought, Science, 318, p. 612, 2007.
Samanta, A., Ganguly, S., Hashimoto, H., Devadiga, S., Vermote, E., Knyazikhin, Y., Nemani, R. R., and Myneni, R. B.: Amazon forests did not green-up during the 2005 drought, Geophys. Res. Lett., 37, L05401, https://doi.org/10.1029/2009GL042154, 2010.
Santiago, L. S., Goldstein, G., Meinzer, F. C., Fisher, J. B., Machado, K., Woodruff, D., and Jones, T.: Leaf photosynthetic traits scale with hydraulic conductivity and wood density in Panamanian forest canopy trees, Oecologia, 140, 543–550, 2004.
Saxton, K. E., Rawls, W. J., Romberger, J. S., and Papendick, R. I.: Estimating generalized soil-water characteristics from texture 1, Soil Sci. Soc. Am. J., 50, 1031–1036, 1986.
Schleppi, P., Thimonier, A., and Walthert, L.: Estimating leaf area index of mature temperate forests using regressions on site and vegetation data, Forest Ecol. Manag., 261, 601–610, 2011.
Schuldt, B., Leuschner, C., Horna, V., Moser, G., Köhler, M., van Straaten, O., and Barus, H.: Change in hydraulic properties and leaf traits in a tall rainforest tree species subjected to long-term throughfall exclusion in the perhumid tropics, Biogeosciences, 8, 2179–2194, https://doi.org/10.5194/bg-8-2179-2011, 2011.
Smallman, T. L., Moncrieff, J. B., and Williams, M.: WRFv3.2-SPAv2: development and validation of a coupled ecosystem–atmosphere model, scaling from surface fluxes of CO2 and energy to atmospheric profiles, Geosci. Model Dev., 6, 1079–1093, https://doi.org/10.5194/gmd-6-1079-2013, 2013.
Sperry, J. S., Hacke, U. G., Oren, R., and Comstock, J. P.: Water deficits and hydraulic limits to leaf water supply, Plant Cell Environ., 25, 251–263, 2002.
Sus, O., Williams, M., Bernhofer, C., Béziat, P., Buchmann, N., Ceschia, E., Doherty, R., Eugster, W., Grünwald, T., Kutsch, W., and Smith, P.: A linked carbon cycle and crop developmental model: Description and evaluation against measurements of carbon fluxes and carbon stocks at several European agricultural sites, Agr. Ecosys. Environ., 139, 402–418, 2010.
van de Weg, M. J., Shaver, G. R., and Salmon, V. G.: Contrasting effects of long term versus short-term nitrogen addition on photosynthesis and respiration in the Arctic, Plant Ecol., 214, 1273–1286, 2013.
Von Randow, C., Zeri, M., Restrepo-Coupe, N., Muza, M. N., de Gonçalves, L. G. G., Costa, M. H., Araujo, A. C., Manzi, A. O., da Rocha, H. R., and Saleska, S. R.: Inter-annual variability of carbon and water fluxes in Amazonian forest, Cerrado and pasture sites, as simulated by terrestrial biosphere models, Agr. Forest Meteorol., 182, 145–155, 2013.
Wagner, F. H., Herault, B., Rossi, V., Hilker, T., Maeda, E. E., Sanchez, A., Lyapustin, A. I., Galvao, L. S., Wang, Y., and Aragao, L.: Climate drivers of the Amazon forest greening, PLoS One, 12, e0180932, https://doi.org/10.1371/journal.pone.0180932, 2017.
Walker, A. P., Beckerman, A. P., Gu, L., Kattge, J., Cernusak, L. A., Domingues, T. F., Scales, J. C., Wohlfahrt, G., Wullschleger, S. D., and Woodward, F. I.: The relationship of leaf photosynthetic traits – Vcmax and Jmax – to leaf nitrogen, leaf phosphorus, and specific leaf area: a meta-analysis and modeling study, Ecol. Evol., 4, 3218–3235, 2014.
Wang, G., Alo, C., Mei, R., and Sun, S.: Droughts, hydraulic redistribution, and their impact on vegetation composition in the Amazon forest, Plant Ecol., 212, 663–673, 2011.
Waring, R. H. and Schlesinger, W. H.: Forest ecosystems. Concepts and management, Academic Press, 7–37, 1985.
Weiss, M., Baret, F., Smith, G. J., Jonckheere, I., and Coppin, P.: Review of methods for in situ leaf area index (LAI) determination Part II, Estimation of LAI, errors and sampling, Agr. Forest Meteorol., 121, 37–53, 2004.
Williams, M., Rastetter, E. B., Fernandes, D. N., Goulden, M. L., Wofsy, S. C., Shaver, G. R., Melillo, J. M., Munger, J. W., Fan, S. M., and Nadelhoffer, K. J.: Modelling the soil-plant-atmosphere continuum in a Quercus–Acer stand at Harvard Forest: the regulation of stomatal conductance by light, nitrogen and soil ∕ plant hydraulic properties, Plant Cell Environ., 19, 911–927, 1996.
Williams, M., Malhi, Y., Nobre, A. D., Rastetter, E. B., Grace, J., and Pereira, M. G. P.: Seasonal variation in net carbon exchange and evapotranspiration in a Brazilian rain forest: a modelling analysis, Plant Cell Environ., 21, 953–968, 1998.
Wright, I. J., Reich, P. B., Westoby, M., Ackerly, D. D., Baruch, Z., Bongers, F., Cavender-Bares, J., Chapin, T., Cornelissen, J. H., Diemer, M., Flexas, J., Garnier, E., Groom, P. K., Gulias, J., Hikosaka, K., Lamont, B. B., Lee, T., Lee, W., Lusk, C., Midgley, J. J., Navas, M. L., Niinemets, U., Oleksyn, J., Osada, N., Poorter, H., Poot, P., Prior, L., Pyankov, V. I., Roumet, C., Thomas, S. C., Tjoelker, M. G., Veneklaas, E. J., and Villar, R.: The worldwide leaf economics spectrum, Nature, 428, 821–827, 2004.
Wright, J. K., Williams, M., Starr, G., McGee, J., and Mitchell, R. J.: Measured and modelled leaf and stand-scale productivity across a soil moisture gradient and a severe drought, Plant Cell Environ., 36, 467–483, 2013.
Wu, J., Albert, L. P., Lopes, A. P., Restrepo-Coupe, N., Hayek, M., Wiedemann, K. T., Guan, K., Stark, S. C., Christoffersen, B., Prohaska, N., Tavares, J. V., Marostica, S., Kobayashi, H., Ferreira, M. L., Campos, K. S., da Silva, R., Brando, P. M., Dye, D. G., Huxman, T. E., Huete, A. R., Nelson, B. W., and Saleska, S. R.: Leaf development and demography explain photosynthetic seasonality in Amazon evergreen forests, Science, 351, 972–976, 2016.
Wu, J., Guan, K. Y., Hayek, M., Restrepo-Coupe, N., Wiedemann, K. T., Xu, X. T., Wehr, R., Christoffersen, B. O., Miao, G. F., da Silva, R., de Araujo, A. C., Oliviera, R. C., Camargo, P. B., Monson, R. K., Huete, A. R., and Saleska, S. R.: Partitioning controls on Amazon forest photosynthesis between environmental and biotic factors at hourly to interannual timescales, Glob. Change Biol., 23, 1240–1257, 2017.
Zhang, K., Castanho, A. D. D., Galbraith, D. R., Moghim, S., Levine, N. M., Bras, R. L., Coe, M. T., Costa, M. H., Malhi, Y., Longo, M., Knox, R. G., McKnight, S., Wang, J. F., and Moorcroft, P. R.: The fate of Amazonian ecosystems over the coming century arising from changes in climate, atmospheric CO2, and land use, Glob. Change Biol., 21, 2569–2587, 2015.