the Creative Commons Attribution 4.0 License.
the Creative Commons Attribution 4.0 License.
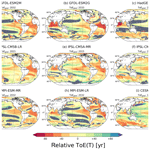
Is deoxygenation detectable before warming in the thermocline?
Thomas L. Frölicher
Juliette Mignot
Fortunat Joos
Anthropogenic greenhouse gas emissions cause ocean warming and oxygen depletion, with adverse impacts on marine organisms and ecosystems. Warming is one of the main indicators of anthropogenic climate change, but, in the thermocline, changes in oxygen and other biogeochemical tracers may emerge from the bounds of natural variability prior to warming. Here, we assess the time of emergence (ToE) of anthropogenic change in thermocline temperature and thermocline oxygen within an ensemble of Earth system model simulations from the fifth phase of the Coupled Model Intercomparison Project. Changes in temperature typically emerge from internal variability prior to changes in oxygen. However, in about a third (35±11 %) of the global thermocline deoxygenation emerges prior to warming. In these regions, both reduced ventilation and reduced solubility add to the oxygen decline. In addition, reduced ventilation slows the propagation of anthropogenic warming from the surface into the ocean interior, further contributing to the delayed emergence of warming compared to deoxygenation. Magnitudes of internal variability and of anthropogenic change, which determine ToE, vary considerably among models leading to model–model differences in ToE. We introduce a new metric, relative ToE, to facilitate the multi-model assessment of ToE. This reduces the inter-model spread compared to the traditionally evaluated absolute ToE. Our results underline the importance of an ocean biogeochemical observing system and that the detection of anthropogenic impacts becomes more likely when using multi-tracer observations.
- Article
(18981 KB) - Full-text XML
-
Supplement
(108689 KB) - BibTeX
- EndNote
Carbon emissions from human activities are causing ocean warming (Rhein et al., 2013) and ocean deoxygenation, i.e. a decrease in the oceanic oxygen (O2) concentration (Sarmiento et al., 1998; Bopp et al., 2002; Matear and Hirst, 2003; Battaglia and Joos, 2018). Both warming and deoxygenation adversely affect marine organisms, ecosystems and the services they provide (e.g. Pörtner et al., 2014; Deutsch et al., 2015; Gattuso et al., 2015; Magnan et al., 2016).
All major ocean basins have experienced significant warming over the last few decades. Warming is generally strongest at the surface and weaker at deeper layers, indicative of heat penetrating from the surface towards the deep ocean as expected from atmospheric greenhouse gas forcing. The strongest warming in the top 2000 m has been observed in the Southern Ocean (Roemmich et al., 2015) and the tropical/subtropical Pacific and Atlantic Ocean (Cheng et al., 2017). On regional to local scales, the anthropogenic warming signal may be masked by natural interannual to multi-decadal variability. For example, decadal-scale cooling trends in the tropical Pacific and Indian oceans may arise from natural El Niño–Southern Oscillation and/or Indian Ocean dipole variability (Han et al., 2014). Similarly, decadal variability in the Atlantic meridional overturning is observed to modulate temperature and heat content change in the North Atlantic (Chen and Tung, 2018).
Observation-based studies indicate that the global ocean oxygen content has decreased since 1960 (e.g. Schmidtko et al., 2017). Increased ocean surface temperature reduces oxygen solubility, limiting atmospheric oxygen dissolution into the upper ocean. In subsurface waters, oxygen concentration is also affected by changes in ventilation and the remineralisation of organic matter. In the contemporary ocean, oxygen decreases in the interior are mostly dominated by a reduction in ventilation with a smaller role for changes related to the production of organic matter, O2 solubility and air–sea equilibration of O2 in surface waters (Bopp et al., 2002, 2017; Plattner et al., 2002; Tjiputra et al., 2018; Hameau et al., 2019). The largest oxygen declines are located in the Pacific Ocean (Equator and Northern Hemisphere) and the Southern Ocean. However, observations are relatively sparse and only start in the second half of the 20th century. Therefore, it is challenging to distinguish human-caused trends from natural variations in the observational record of ocean O2.
Global climate models, such as the Earth system models that participated in phase 5 of the Coupled Model Intercomparison Project (CMIP5), reproduce the long-term trend in global ocean heat content over the last 50 years when uncertainties of observation-based estimates and internally generated natural variability are taken into account (Frölicher and Paynter, 2015; Cheng et al., 2019). Modelling studies agree on the sign of oceanic O2 changes but likely underestimate the magnitude of loss (Bopp et al., 2013; Cocco et al., 2013; Oschlies et al., 2017). In particular in the tropical regions, models are not able to reproduce observed O2 decrease in equatorial low-oxygen zones (Stramma et al., 2008; Cocco et al., 2013; Cabré et al., 2015).
It is expected that ocean warming and deoxygenation, and the combination thereof, increase the risk of adverse impacts on marine organisms and ecosystem services (Pörtner et al., 2014). Warming of the ocean influences the physiology and ecology of almost all marine organisms. Reduced oceanic O2 concentrations can disrupt marine ecosystems by pushing organisms to their species-specific limits of hypoxic tolerance, below which the species are no longer able to meet their metabolic O2 demand. The species-specific metabolic demand of O2 is also a function of temperature, as warmer temperatures increase metabolic rates and oxygen requirements (Deutsch et al., 2015). At the same time, higher ocean temperatures also decrease oxygen supply through reduced ventilation, enlarging the regions with limited O2 concentrations and thus shifting ecosystem distribution (Cheung et al., 2011).
Beyond the combined impact of physical and biogeochemical changes, an interesting question is whether anthropogenic changes in the ocean interior are first detectable in variables that are routinely and frequently measured such as temperature (T) or in variables with a relatively low observational coverage but potentially high impact for ecosystems such as O2 (Joos et al., 2003). The answer may have implications for measurement strategies to detect anthropogenic changes in subsurface waters as well as for the impacts of physical and biogeochemical change on marine life. For the surface ocean, earlier studies (Keller et al., 2014; Rodgers et al., 2015; Frölicher et al., 2016; Schlunegger et al., 2019) showed that the anthropogenic signals of pH and pCO2 emerge earlier than sea surface temperature and O2 change earlier than productivity. Changes in surface O2 are tightly coupled to temperature-driven solubility changes and O2 varies hand in hand with sea surface temperature and the two signals emerge typically concomitantly. Regarding the ocean interior, the sequence of emergence for O2 and T is less clear. Global warming increases surface ocean temperature, which tends to reduce O2. However, O2 is also influenced by non-thermal processes, such as respiration and the redistribution by ocean circulation and mixing. Respiration of organic matter in the ocean interior may have a larger influence on O2 change than temperature-driven solubility change in a more stratified and less ventilated ocean. One could therefore expect that, under global warming, the combined effect of increased O2 consumption and decreased O2 solubility will accelerate the O2 depletion in subsurface waters and that O2 may be detectable before the warming reaches that layer.
The concept of time of emergence (ToE; Christensen et al., 2007; Hawkins and Sutton, 2012) is often used to determine the point in time when the anthropogenic signal becomes larger than the range of natural variability. ToE has been broadly used in climate change detection for physical climate variables (e.g. surface temperature: Hawkins and Sutton, 2012; Frame et al., 2017), land carbon fluxes (Lombardozzi et al., 2014) or marine biogeochemical variables (e.g. pH, alkalinity, dissolved inorganic carbon (DIC), pCO2: Hauri et al., 2013; Keller et al., 2014; marine biological productivity: Henson et al., 2016). A limited number of studies addressed anthropogenic deoxygenation detection in the subsurface layers (Rodgers et al., 2015; Frölicher et al., 2016; Henson et al., 2016, 2017; Long et al., 2016; Hameau et al., 2019). One study, Hameau et al. (2019), uses a single model (Community Earth System Model; CESM), to investigate ToE of temperature and oxygen in the thermocline, finding that anthropogenic ocean warming emerges much earlier than the O2 signal in low-latitude and midlatitude regions. Delayed emergence of changes in O2 is due to the opposing effects of O2 solubility and O2 consumption. In the high latitudes and the Pacific subtropical gyres, deoxygenation emerges before ocean warming in CESM. This occurs because decreases in oxygen solubility are reinforced by increased O2 consumption, leading to strong O2 depletion. However, it is unknown if this single-model result is robust across a suite of different Earth system model simulations. Here, we conduct a multi-model study to more broadly test the hypothesis that anthropogenic deoxygenation in the thermocline emerges prior to anthropogenic warming. Since the primary objective is to test the consistency across models of the order of emergence (deoxygenation prior to warming) within a single model, we introduce a relative ToE to conduct the intercomparison, rather than the absolute year of ToE. We define relative ToE as a deviation relative to the model mean ToE for improved model intercomparison.
In this study, we analyse and compare the relative ToE(T) and ToE(O2) in the thermocline (200–600 m) using nine different CMIP5 Earth system models. We also assess the impact of using the relative ToE in comparison to the classical approach using absolute ToE. In addition, we discuss the magnitude of background internal variability and anthropogenic signal, and their translation into ToE. Finally, we analyse the role of solubility, ventilation and respiration for the emergence of anthropogenic changes in oxygen and temperature.
Smith et al., 2010(Moore et al., 2002, 2004)(Hurrell et al., 2013)Danabasoglu et al., 2011(Griffies et al., 2011)(Dunne et al., 2013)(Hallberg, 1997)(Dunne et al., 2012, 2013)(Palmer and Totterdell, 2001)(Collins et al., 2011)(Collins et al., 2011)(Madec et al., 2017)(Aumont and Bopp, 2006)(Dufresne et al., 2013)(Jungclaus et al., 2013)(Ilyina et al., 2013)(Giorgetta et al., 2013)2.1 Earth system models
We use output from eight different configurations of four Earth system models (ESMs) that participated in CMIP5 (Taylor et al., 2012): GFDL-ESM2M, GFDL-ESM2G, HadGEM2-CC, IPSL-CM5A-LR, IPSL-CM5A-MR, IPSL-CM5B-LR, MPI-ESM-LR and MPI-ESM-MR (Table 1). In order to extend the multi-model ensemble from four to five family models, we also included the output from simulations performed with CESM1.0 conducted at the Swiss Supercomputing Centre. The horizontal ocean model resolution is about 1° in both the Geophysical Fluid Dynamics Laboratory (GFDL) models and CESM1.0. Hadley Centre Global Environment Model version 2 – Carbon Cycle (HadGEM2-CC) and Institut Pierre Simon Laplace (IPSL) models have a horizontal resolution of about 2° and the MPI models have a horizontal resolution of about 0.4° (medium resolution; MR) and 1.5° (low resolution; LR). Of the nine models, all but one (GFDL-ESM2G, isopycnal vertical coordinate) use a pressure-based vertical coordinate. For additional information on the individual model setups, the reader is referred to the references listed in Table 1.
Both the CMIP5 ESMs and the CESM1.0 were run under prescribed anthropogenic and natural greenhouse gas and aerosol forcing. All simulations span the historical 1861–2005 period and the 2006–2100 period following the Representative Concentration Pathway 8.5 (RCP8.5) scenario. The RCP8.5 represents a high emission scenario with a radiative forcing of 8.5 W m−2 in the year 2100 (Riahi et al., 2011). These simulations are complemented with output from corresponding control runs with constant preindustrial forcing. The CESM1.0 simulations differ from the CMIP5 simulations only with regard to the spin-up procedure. The CMIP5 model simulations are branched off from preindustrial control simulations, whereas the CESM1.0 simulation is an extension of a last-millennium simulation run under 850 CE conditions (Lehner et al., 2015). For this study, all CMIP5 models are used for which the three-dimensional output of oxygen, temperature and salinity for all simulations was available on the Earth System Grid. We regridded all model output onto a regular grid. Even though the model drift in the control simulations is relatively small in the thermocline ( mmol m−3 yr−1 for trend in global mean oxygen concentration and ∘C yr−1 for trend in global mean temperature averaged over 200–600 m), we detrended all model output with a linear trend obtained from the preindustrial control simulation in each grid cell. The CESM1.0 simulation also shows some model drift. Therefore, an exponential curve was fitted to the annual output of its associated control simulation at each grid cell. The detrending procedure is described in detail in Hameau et al. (2019).
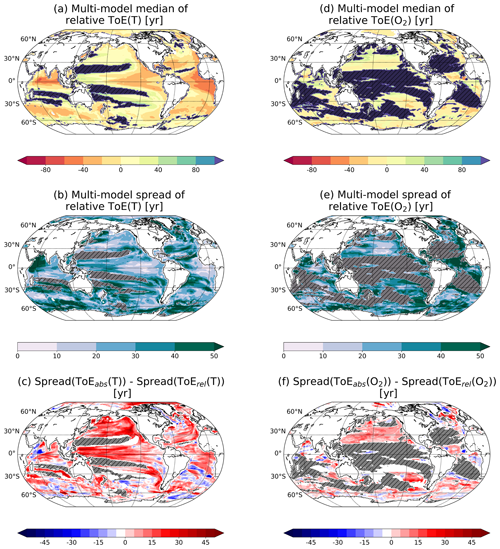
Figure 1Multi-model median (a, d) and spread (b, e) of relative ToE for temperature (a, b, c) and dissolved oxygen (d, e, f) for the thermocline (200–600 m). The spread is computed as the interquartile range. Difference (c, f) between the multi-model spread of absolute ToE estimates with the multi-model spread of relative ToE estimates for (c) temperature and (f) dissolved oxygen. The hatched areas show regions with no emergence for at least three models. The relative ToE estimates are shown for each model in Figs. 2 and 3 and the absolute estimates in Figs. S1 and S2 in the Supplement.
2.2 Multi-model analysis methods
We use the concept of ToE (e.g. Hawkins and Sutton, 2012) to compare anthropogenic changes in O2 and temperature (signal; S) with internal variations (background noise; N). Here, ToE represents the moment in time at which the ocean state becomes distinct from the preindustrial state. Appendix Fig. A1 provides a graphical illustration of the method used to compute ToE.
We define the absolute ToE as the first year when the anthropogenic signal S becomes equal to or larger than twice the noise of internal variability N (Eq. 1; following Hameau et al., 2019; Fig. A1). The threshold is set to 2 in order to distinguish the signal from the noise at 95 % confidence level. Annual O2 and T data are first averaged over the thermocline (200–600 m) at each grid point of the horizontal grid, and local S and N are computed from these depth-averaged values for each model, variable and (horizontal) grid point. Annual anomalies are calculated relative to the preindustrial period (1860–1959).
The background noise, N, is computed as 1 standard deviation (SD) of O2 and of T from the annual preindustrial control output. The entire duration of the control simulation is considered for each model to estimate the background noise.
N represents the noise due to the internal chaotic variability of the climate system. Note that this definition of the noise differs from Hameau et al. (2019), who used internal plus externally forced natural variability from a last-millennium simulation to assess the standard background noise.
The annual output of the forced, transient simulation (1860–2099) is smoothed by a low-pass spline filter (Enting, 1987) to estimate S for each (horizontal) grid point in the thermocline. The cut-off period of the spline is set to 80 years to remove decadal to multi-decadal variations (e.g. associated with internal variability). The signal S is then defined as the value of the spline at each point in time.
To ensure that S indeed detects anthropogenic trends, we also apply a criterion for the sign of S to define ToE: S needs to have the same sign as the difference between the last 30 years of the future simulation and the preindustrial average for the corresponding variable and grid point.
In order to minimise inter-model differences and to highlight the common spatial patterns of ToE, we introduce a new metric, the relative ToE (ToErel). It is defined as the absolute ToE (ToEabs) minus the global area-averaged ToE (ToEglob; Eq. 2).
S, N, ToE and ToErel are first computed from the annual output for each model and at each (horizontal) grid cell. Then, multi-model median and spread (interquartile range) of the multi-model estimations are computed from the model ensemble. The median represents a “best” estimate and the interquartile range a measure of model uncertainty. Uniform weights are applied to each model configuration to compute those statistics. Tests have been performed using a weighted median as several simulations stem from the same model family (CESM × 1; GFDL × 0.5; HadGEM2 × 1; IPSL × 0.3; MPI × 0.5). However, the median and interquartile range of the multi-model ensemble are not sensitive to the weighting scheme applied (not shown). Because an anthropogenic signal may not emerge before the end of the simulation in the year 2100, ToE can be undefined. We therefore require that ToE values are defined for at least seven out of nine models to compute the multi-model statistics (median and spread). If more than two models have an undefined ToE, we mask the grid points in maps of the multi-model median and of the multi-model spread.
2.3 Separating mechanisms of oxygen change
To diagnose processes driving the simulated changes in ocean O2, the direct thermal/solubility component of change (O2,sol) can be isolated from the total O2 change. The residual, apparent oxygen utilisation (AOU), represents the summation of all non-thermal changes, including those resulting from changes in ventilation and remineralisation.
The solubility component for each model is computed following Garcia and Gordon (1992), which requires local salinity and temperature output. The solubility depends mostly on temperature with a small contribution of salinity. The non-thermal component ([-AOU]) is deduced from the difference between O2,sol and O2 following Eq. (3). In Sect. 3.4, we will use changes in [-AOU] as a proxy for changes in water mass age and ventilation. Output of an ideal age tracer is not available for most models. A decrease in water exchange between the surface ocean and the thermocline typically leads to an increase in water mass age in the thermocline. Therefore, changes in ventilation affect the balance between the rate of supply of O2-rich waters from the surface and the rate of O2 consumption by remineralisation of organic matter. It has been demonstrated in earlier studies (e.g. Gnanadesikan et al., 2012; Bopp et al., 2017; Hameau et al., 2019) that a decrease in [-AOU] typically corresponds to a decrease in ventilation and an increase in water mass age, as simulated changes in the remineralisation rates of organic material and in associated O2 consumption are relatively small over the 21st century.
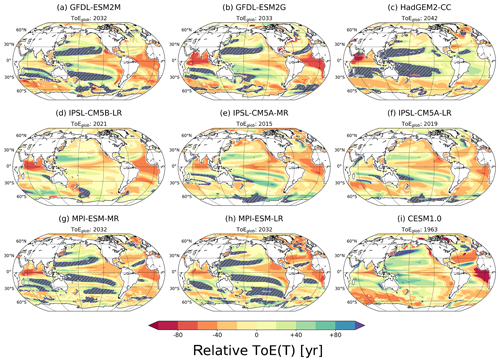
Figure 2ToE of T in the thermocline (200–600 m) relative to the averaged ToE in that layer for each simulation. The hatched areas show regions with no emergence by the end of the 21st century. The values of the global average ToE, ToEglob, are given above each panel. The absolute ToE estimates are shown in Fig. S1.
3.1 Relative time of emergence
We start by discussing the multi-model median and spread of relative ToE estimates for potential temperature (Fig. 1a, b) and dissolved oxygen (Fig. 1d, e) changes in the thermocline (200–600 m). An analysis of the roles of internal variability and anthropogenic change ToE and why anthropogenic change is detectable early or late is presented in Sect. 3.3.
3.1.1 Anthropogenic warming
ToErel(T) shows early emergence in low latitudes and between 30 and 60∘ S, and late emergence in the western tropical Pacific, in the Atlantic subpolar gyre and the subtropical gyres of the Indian and Pacific oceans (Fig. 1a). The northern Indian Ocean and the eastern equatorial Atlantic stand out as the regions with earliest emergence in anthropogenic warming, i.e. 70 years (median of nine ToErel(T)) before the global average ToE. No emergence of warming by the end of the 21st century (for at least three models; see Sect. 2.2) is simulated in the subtropical gyres of the Indian and Pacific oceans, south of Greenland and locally south of 60∘ S.
The multi-model spread in ToErel(T) is generally small in regions with early emergence (Fig. 1b). This is the case in many regions of the Pacific and the Southern Ocean (±15 years). However, in the Atlantic subtropical gyres and in the Arabian Sea, the early ToErel(T) estimates are associated with a wider spread across models (±25 to ±45 years). Large inter-model spread is also found in the Kuroshio extension and in the Indian and Atlantic region of the Southern Ocean (±50 years). These regional differences in the multi-model spread could not be explained by the multi-model median of ToE, the anthropogenic signal nor the internal variability amplitude for both O2 and T. Scatter plots of individual grid cell values of the multi-model spread in ToErel versus those of the multi-model median of ToE, the anthropogenic signal or the internal variability amplitude do not show a clear relationship (not shown). On average, the multi-model spread for ToErel(T) is about 25 years.
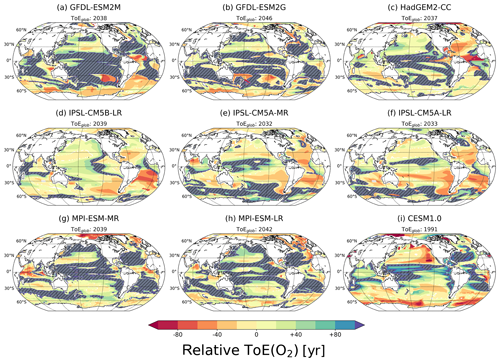
Figure 3ToE of O2 in the thermocline (200–600 m) relative to the averaged ToE in that layer for each simulation. The hatched areas show regions with no emergence by the end of the 21st century. The absolute ToE estimates are shown in Fig. S2. The global average ToE, ToEglob, is shown for each model.
ToE values for individual horizontal grid cells are globally averaged to obtain an area-weighted global mean ToE for the thermocline and each model. These global mean values range between the years 1963 and 2033 for the nine models (see subtitles in Fig. 2). The patterns of ToErel(T) for each individual model are shown in Fig. 2. As described previously, low-latitude regions and parts of the Southern Ocean show earlier emergence compared to mid- and other high-latitude regions. The HadGEM2-CC model (Fig. 2c) is an exception in that respect as temperature emerges later (+30 to +50 years) than the global average in the tropical Atlantic and Pacific. In the Pacific and Indian subtropical gyre regions, the models show late (IPSL family) or no emergence. And finally, CESM and the IPSL family models are the only models that show emergence before the end of the 21st century in the subtropical gyres of the Pacific.
3.1.2 Anthropogenic deoxygenation
In contrast to ToErel(T), most of the thermocline shows no emergence of the anthropogenic O2 change by the end of the 21st century (Fig. 1d). In the remaining regions, ToErel(O2) varies by about ±40 years. Early emergence is found in the subtropical gyre of the North Pacific, the northern North Atlantic, the Atlantic sector of the Southern Ocean and generally south of 60∘ S. No emergence is simulated in 47 % of the ocean area by the end of the 21st century including large parts of the tropics and the subtropical gyres of the Atlantic Ocean and the Indian Ocean.
The multi-model spread for ToErel(O2) is 20 years in the global average and thus somewhat smaller than for ToErel(T). The models show a high spread for ToErel(O2) (±50 years) at low latitudes, such as in the southern Arabian Sea or in the equatorial Atlantic, whereas high model agreement is found in parts of the central North Pacific and the northern Indian Ocean (spread of ±15 years) (Fig. 1e). In the eastern tropical Atlantic, the spread for ToErel(O2) is, despite a smaller global mean spread, larger than for ToErel(T). In summary, even though the median pattern of ToErel(O2) is relatively uniform in comparison to ToErel(T), the spread for ToErel(O2) varies between regions as for ToErel(T).
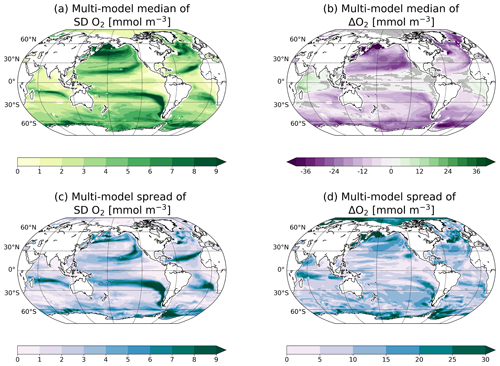
Figure 5Median (a, b) and spread (c, d) of multi-model natural variability (standard deviation of control simulations; a, c) and changes by the end of the 21st century (b, d) of O2 between 200 and 600 m. The hatched areas in panel (b) show regions where at least 70 % of the models do not agree on ΔO2 sign. The individual responses for each model are shown in Figs. S5 and S6.
The multi-model median O2 signal does not emerge in 47 % of the global thermocline as noted above. Midlatitudes and low latitudes show no emergence by the end of the 21st century in most of the models (Fig. 3). However, the exact regions of no emergence differ between models. This regional mismatch, in combination with the requirement that at least seven out of nine models need to show an emerging signal (Sect. 2.2), explains why in the multi-model analysis many grid cells are masked, indicating no emergence in the median (Fig. 1d–f). The area fraction with no emerging O2 signal is smaller in individual models than in the multi-model median and ranges between 10 % and 30 %.
As for temperature, a large range in absolute ToE is found with globally averaged ToE(O2) ranging between the years 1991 and 2046 for the nine models (see subtitles in Fig. 3). The analysis of ToErel(O2) for individual models reveals some additional notable differences (Fig. 3). GFDL-ESM2M, GFDL-ESM2G, HadGEM2-CC and CESM1.0 simulate early emergence in the Southern Ocean, but the IPSL models project no emergence of deoxygenation in this region by the end of the 21st century. In addition, the IPSL models and the CESM1.0 model show relatively early emergence in many grid cells of the western tropical Pacific, a region with no emergence in other models. ToErel(O2) also diverges across the models in the Atlantic subtropical gyres: in the HadGEM2 and IPSL simulations, oxygen changes are simulated to emerge relatively early (ToErel(O2) ∼40 to 60 years), whereas in the GFDL, MPI and CESM simulations, the changes are not yet detectable by the end of the 21st century.
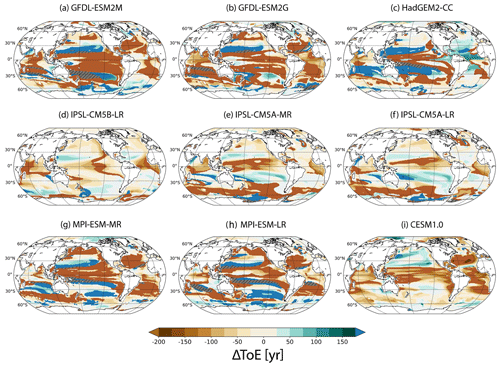
Figure 6ΔToE defined as ToE(T) minus ToE(O2) for each simulation in the thermocline. Blueish colours indicate earlier emergence of oxygen. Brownish colours indicate earlier emergence of temperature. The saturated colours mean that one of the variables has not emerged by 2099. No emergence in both T and O2 is shown by the hatched areas.
3.2 Relative versus absolute ToE
Mapping ToErel for different models is intended to emphasise common patterns across models by removing the global mean bias between models, while model–model differences in ToEabs are indicative of an overall model uncertainty.
The multi-model spread for ToEabs is on average larger than the multi-model spread for ToErel for temperature (Fig. 1c) and oxygen (Fig. 1f), while spatial patterns are similar for ToErel and ToEabs.
On a global average, the spread is reduced from ±30 years for ToEabs(T) to ±23 years for ToErel(T) and from ±20 years for ToEabs(O2) to ±17 years for ToErel(O2). Regionally, the reduction can be larger. For example, in the equatorial regions, the Atlantic and the Southern Ocean, the spread is reduced by 20 to 50 years when computed for ToErel(T) instead for ToEabs(T). Similarly, the spread in ToE(O2) is reduced from ±35 to ±5 years in parts the North Pacific.
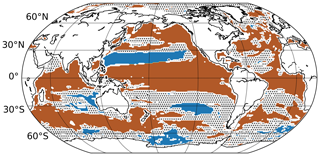
Figure 7Summary map showing the regions where oxygen changes emerge before temperature changes (blue areas; ΔToE >0 with ΔToE = ToE(T) − ToE(O2)) and where temperature changes emerge before oxygen changes (brown areas; ΔToE <0) for at least seven out of nine models. The dashed areas show the regions where more than three models differ in the sign of ΔToE.
3.3 Internal variability and anthropogenic signals
The ToE allows for a comparison across climate models, by combining the amplitude of the climate response to anthropogenic forcing and the amplitude of natural variability in one metric. The magnitude and the spatial patterns of the internal variability and of the anthropogenic signal for both thermocline temperature and oxygen are discussed next.
The multi-model median of internal variability for thermocline temperature fluctuates with an amplitude typically ±0.1 ∘C in the tropics and the Arctic Ocean, and ±0.5 ∘C in mid-to-high latitudes (Fig. 4a). SD(T) is the largest (up to ±0.9 ∘C) in the western boundary currents such as the Kuroshio Current and the Gulf Stream. The internally generated variability is also relatively large along the equatorward flanks of the subtropical gyres. It is also in these regions where SD(T) differs most among models (up to ±0.5 ∘C along the North Atlantic Current; Fig. 4c).
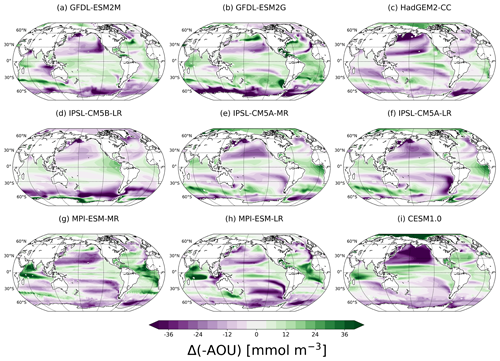
Figure 8Anthropogenic changes (2070–2099 CE minus 1861–1959 CE) in [-AOU] in the thermocline for each model.
In the multi-model median, temperature in the thermocline is projected to increase on global average by 1.2±0.7 ∘C (Fig. 4b) by the end of the 21st century under the RCP8.5 scenario relative to the period 1861–1959, in accordance with published observational studies (Levitus et al., 2009, 2012; Bilbao et al., 2019). Large warming of more than 4.0±0.7 ∘C is projected in the northern North Atlantic and around the subantarctic water in the Indian and Atlantic oceans (Figs. 4b and S4). We note that these projections are also characterised with the largest inter-model spread (±1.5 ∘C; Figs. 4d and S4), and uncertainties in these regional warming projections are large. Finally, disagreement among models in simulating changes in thermocline temperature is also large in the Arctic Ocean, possibly related to different simulated changes in sea ice cover (Stroeve et al., 2012; Wang and Overland, 2012).
The combination of a strong signal and small variability results in early detection of the changes. This is the case in the Southern Ocean at 45∘ S (in the Atlantic and Indian regions; Fig. 1a), where the anthropogenic warming is strong (up to 4 ∘C; Fig. 4b) but the internal variability is relatively small (0.1 to 0.3 ∘C; Fig. 4a). However, early emergence of anthropogenic changes can also occur when the signal is relatively small, if the variability is even smaller. This is the case in the tropical oceans such as in the Arabian Sea, the equatorial Atlantic and the western equatorial Pacific, where water masses warm modestly (up to 1.5 ∘C) but vary naturally between 0.1 and 0.2 ∘C only. It is also the case in the eastern equatorial Pacific, where the early emergence arises from the very weak internal variability in the thermocline, although the temperature increase (∼0.80 ∘C) is also relatively weak. In this region, the substantial variability in O2 and T is largely confined to the top 200 m. No emergence by the end of the 21st century, such as that simulated in the subtropical gyres of the Indian and Pacific oceans, results from a relatively weak signal combined with a relatively strong variability in these regions.
Internal variability of dissolved oxygen concentrations is particularly large in the northern North Pacific and North Atlantic, the Southern Ocean and along the equatorward boundaries of the subtropical gyres with SD(O2) of up to 10 mmol m−3 (Fig. 5a). The multi-model spread of SD(O2) (Fig. 5c) is about equally large as the median of SD(O2) (Fig. 5a) along the equatorward boundaries of the subtropical gyres. Looking at the individual model responses, the O2 internal variability shows a wide range of different patterns (Fig. S5). The GFDL and MPI models simulate high internal variability of oxygen in the entire thermocline, whereas CESM, HadGEM2 and IPSL models show high variability regionally.
The O2 concentration in the thermocline (Fig. 5b) is projected to decrease under global warming, in accordance with previous model studies (e.g. Sarmiento et al., 1998; Cocco et al., 2013; Bopp et al., 2017). The anthropogenic decrease in O2 is large in the Southern Ocean, in the North Pacific subtropical gyre and in the North Atlantic subpolar gyre. In tropical regions, the changes are projected to be small, except for the western Indian Ocean, where more than 70 % of the models project an increase of O2 concentration. The simulated O2 changes differ most across models in high latitudes and in the subpolar gyres, as well as in the equatorial Indian Ocean (Fig. 5d).
Despite differences in the simulated magnitude of O2 changes and internal variability patterns of O2 between the different models, the resulting ToErel(O2) are robust across models. For example, the decrease in O2 spans from −12 to −40 mmol m−3 (Fig. S6) and SD(O2) spans from ±5 to ±15 mmol m−3 (Fig. S5) in the central North Pacific. Moreover, the spatial locations of the maximum O2 depletion differ across the models. However, ToErel(O2) in this region is within ∼10 years (Fig. 1d), with a relatively low spread (±10 years) compared to ToEabs(O2) (±30 years). Another example is the CESM model. The very early detection of anthropogenic changes (for temperature and oxygen) in the CESM model described in Sect. 3.1, results from a particularly weak internal variability (Figs. S3i and S5i; see also Hameau et al., 2019) combined with a high climate sensitivity of the model (Figs. S4i and S6i). The ToErel allows the comparison of ToE resulting from CESM output with the results from the eight models in spite of these model–model differences (Figs. 2 and 3).
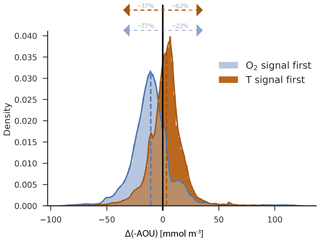
Figure 9Density distribution of [-AOU] changes by 2099 for the grid points where the O2 signal emerges first (blue) and where the temperature signal emerges first (brown) in the thermocline for the ensemble of nine models. Each distribution is centred around the median (dashed blue: −10.8 mmol m−3; dashed brown: 3.3 mmol m−3).
3.4 Comparison of ToE(O2) with ToE(T)
In general, temperature changes are detectable before O2 changes in around 64±11 % of the thermocline (yellow to brown colours in Fig. 6). As discussed in Sect. 3.1, the anthropogenic O2 signal emerges late or not at all in many low-latitude regions, while the anthropogenic warming signal is emerging in most regions and typically early around the Equator. However, there are also areas where anthropogenic deoxygenation is detectable earlier than anthropogenic warming in all models (green to blue colours in Fig. 6). These cover 35±11 % of the global thermocline in the nine models. They are mainly located in the midlatitudes, especially between ∼15 and 30∘ N in the North Pacific, around Antarctica (including the Ross and Weddell seas), along the Western Australian Current and the Pacific southern subtropical gyre region. Model results for the Atlantic subtropical gyres are mixed. Some models suggest O2 changes to be detectable earlier than T changes (HadGEM2 and the IPSL family), whereas in other models the O2 signal does not even emerge.
The exact locations of relatively early emergence of O2 differ across models. Hence, the regions where at least seven out of the nine models show consistently an earlier emergence of O2 than T is smaller and amounts to 17 % of the global thermocline area. As shown in Fig. 7 (blue areas), the O2 signal emerges consistently in at least seven models before the T signal in parts of the Pacific subtropical gyres, the Southern Ocean and the southeast Indian Ocean.
A mechanistic explanation of early or late emergence of the O2 signal relative to the temperature signal is not straightforward as two ratios (S∕N) are involved. Nevertheless, changes in apparent oxygen utilisation (Δ[-AOU]; Fig. 8) provide some insight into underlying mechanisms. We use Δ[-AOU] as a proxy for changes in water mass age and ventilation, as noted in Sect. 2.2.
Regions with early emergence of anthropogenic O2 compared to T show typically a decrease in [-AOU] (Fig. 6 versus Fig. 8), whereas regions with early emergence of T compared to O2 show typically an increase in [-AOU]. For example, [-AOU] is decreasing in 77±8 % of the areas with early emergence of O2, while only 22±8 % of these regions show an increase in [-AOU] (Fig. 9; blue). In most regions where T is emerging before O2 (Fig. 9; brown), [-AOU] is increasing (62±12 %). A decreasing trend in [-AOU] is indicative of a reduced ventilation induced by upper ocean warming and increased stratification (e.g. Capotondi et al., 2012). A more sluggish ventilation slows the supply of O2 from the surface to the ocean interior. Consequently, thermocline [O2] and [-AOU] are both decreasing. This leads to a strong and thus early detectable anthropogenic deoxygenation. In addition, a more sluggish ventilation slows the penetration of the anthropogenic warming signal from the surface to the interior and similarly the penetration of the thermally driven O2 signal ([O2,sol]). The detection of the temperature changes is thus delayed compared to AOU and O2. There are some exceptions to this relationship between [-AOU] and the earlier emergence of O2 than T. For example, O2 change emerges before warming in the GFDL model around 30∘ S and 120∘ W, although [-AOU] is increasing in this region. However, warming is emerging very late as the GFDL models simulate weak warming and even some cooling (Fig. S4) in this part of the thermocline. Thus, in this special case, the early emergence of O2 relative to T is due to the absence of large warming in a region with notable temperature internal variability.
Regions where the warming signal is detectable before the deoxygenation are typically associated with an increase in [-AOU]. Such increase counteracts the decrease in [O2,sol], leading to relatively smaller changes in [O2], which are thus often not detectable. There are again a few exceptions. For example, the IPSL models simulate a decrease in [-AOU] in the northern North Pacific but an earlier ToE for T than for O2 in this region.
In summary, anthropogenic change in temperature is detectable earlier than anthropogenic change in O2 in most of the global ocean. However, there are large ocean regions where anthropogenic O2 changes are detectable earlier in the thermocline in all models. Early emergence of deoxygenation relative to warming is typically detected in regions where thermocline ventilation and [-AOU] are decreasing over the simulation and late emergence of O2 changes where ventilation and [-AOU] are increasing.
We analysed the time of emergence (ToE) of human-induced changes in oxygen (O2) concentrations and temperature (T) in the thermocline (200–600 m) using nine Earth system model simulations of the climate over the historical and the future period. Using ToE as a metric allows for the assessment of anthropogenic changes by comparing the magnitude of the human-induced changes with the magnitude of internal variability. Both the magnitude of anthropogenic change and internal variability are model dependent, rendering the absolute year of ToE strongly model dependent. Evaluating differences in absolute year of ToE, however, can obscure important model agreement upon the spatial patterns and progression of emergence within a multi-variable framework. We therefore introduce a new metric, the relative ToE (ToErel), to better compare ToE across different models and variables. ToErel is computed by subtracting the global mean ToE from the ToE field. Absolute years of emergence are thus not considered by this metric and it only illustrates whether a signal emerges relatively early or late for a given model. We investigated whether anthropogenic T or O2 changes emerge first and link patterns of ToE(T)–ToE(O2) to changes in apparent oxygen utilisation (Δ[-AOU]) and ventilation of the thermocline. In addition, we also identified the processes for earlier/later detection in O2 changes compared to temperature changes.
This multi-model study relies only on results from only four different model families (GFDL-ESM, HadGEM2-CC, IPSL, MPI-ESM and CESM), applied in nine model configurations. All model configurations available from CMIP5 that provide three-dimensional fields for O2 and T for the control, historical and future RCP8.5 scenario simulations have been incorporated into the analysis. Nevertheless, using a larger model ensemble would increase confidence in our results (Knutti and Sedláček, 2013).
A limitation of our study is that all the Earth system models included have a relatively coarse resolution for simulating the complex processes in the O2 minimum zones (Margolskee et al., 2019). Earth system models diverge in projecting physical and biogeochemical changes in these regions (Brandt et al., 2015; Cabré et al., 2015). Some models used in this study project a large increase in [-AOU] (Fig. 8) and considerable warming (Fig. S6) in the eastern tropical Atlantic, likely indicative of a reduced upwelling (Gnanadesikan et al., 2007). Observations show a decrease in O2 and an expansion of hypoxia in the tropics (Stramma et al., 2008, 2012) over recent decades, contradicting the long-term projections from some models. However, these observed trends in the tropics may also be a result of natural variability acting on multi-decadal timescales associated with the Pacific Decadal Oscillation.
Comparing ToE estimates from different studies is delicate due to the model and method dependencies of ToE. Although the generic definition of ToE is under consensus, the methodologies applied to estimate ToE differ in the published literature, as mentioned in the introduction (e.g. IPCC, 2019). Depending on the spatial and temporal scale of a given variable, the threshold for which emergence is defined and the reference period applied, the absolute value of ToE can differ. In addition, the ToE also depends on the definition of the background variability, here acting as noise (Hameau et al., 2019). Estimating the background noise as the standard deviation (SD) of the internal chaotic variability from the control simulation (Frölicher et al., 2016) or as the SD of the variability from the industrial period (after removing anthropogenic trends; Keller et al., 2015; Henson et al., 2016) results in earlier ToE for both O2 and T as when estimating the noise from the total (internal and externally forced) natural variability over the last millennium. Yet, the finding that anthropogenic O2 change emerges before anthropogenic warming in large ocean regions is robust across investigated choices. The anthropogenic signal is frequently computed as a linear trend over a few decades (Rodgers et al., 2015; Henson et al., 2017; Tjiputra et al., 2018). However, the resulting slope depends on the time window used to calculate the linear trend. Hameau et al. (2019) use a low-pass-filtered output to estimate the signal. They showed that ideally the noise (N) component of ToE should be estimated from simulations that include natural variability forced by explosive volcanic eruptions and changes in total solar irradiance, especially when assessing regional- to global-scale ToE estimates. However, these authors also find that on a grid cell scale, internal variability is typically the dominant contribution to overall natural variability during the last millennium. Therefore, estimating the noise from control simulations that include internal variability only, as done in this study, appears justified.
Another limitation of our study lies in the assumption that the anthropogenic signal emerges from interannual to multidecadal internal variability. The anthropogenic signal S and the noise N are estimated by smoothing the model output with a multi-decadal spline filter. Any potential natural centennial variations are retained in the signal S and removed from the noise N. Results from a forced simulation over the past millennium with CESM1.0 show that potential biases in ToE arising from the neglect of long-term natural variability are small for this model (Hameau et al., 2019). However, our multi-model analysis reveals centennial variations in some grid cells and models causing multiple emergence of the signal from the noise (Fig. A2). This may bias the detection of the anthropogenic signal towards early emergence. Here, we constrained detection to partly circumvent problems with re-emerging signals; we require that the trend of the signal at the time of emergence must have the same sign as the change between the last and first 30 model years. Re-emerging signals are found in only a few grid cells, except in HadGEM2, and centennial natural variability appears to play a minor role in these simulations. We expect therefore that our estimates of ToE are reliable for the model ensemble.
Published studies addressing the detection of anthropogenic ocean warming focus on temperature at sea surface. To our knowledge, only a single study (Hameau et al., 2019) using output from a single model is assessing ToE(T) in the thermocline. Yet, the thermocline is a habitat for many fish and other species. Warming in combination with other stressors, such as deoxygenation, ocean acidification and hypocapnia, may reduce the habitat suitability of marine ecosystems in a future climate (e.g. Deutsch et al., 2015; Gattuso et al., 2015; Breitburg et al., 2018; Cheung et al., 2018). Multi-tracer analyses contribute to a better understanding of the potential impact on marine ecosystems in a changing ocean.
We find that thermocline anthropogenic warming emerges first in low latitudes, followed by the Southern Ocean and the high northern latitudes. No emergence is detected in parts of the subtropical gyres of the Pacific and Indian oceans. The rapid emergence at low latitudes is explained by the small internal variability but moderate to strong warming signals. Exceptions are the subtropical gyres in the Atlantic, where it takes approximately two additional decades to detect the temperature changes, mainly because of the relatively large internal variability there. The warming in mid- to high-latitude thermocline emerges approximately 60 to 80 years later than in low latitudes. No emergence is simulated for the Pacific and Indian subtropical gyres, because the changes in temperature are relatively small and the internal variability relatively high there (in accordance with Hameau et al., 2019). For comparison, surface temperature changes emerge at first in low latitudes and then in midlatitudes (Henson et al., 2017).
The time of emergence spatial pattern of thermocline oxygen changes is almost opposite to the one of temperature. Rapid emergence for O2 is simulated at midlatitudes, whereas low latitudes generally do not experience emergence of the O2 signal by the end of the 21st century (Rodgers et al., 2015; Frölicher et al., 2016; Long et al., 2016).
Although internal variability is low in the tropical regions, the O2 signal does not emerge by 2100. This is because the projected changes are also small. This is due to the opposite responses of O2 components. The thermal component is simulated to decrease (due to temperature increase), but [-AOU] is on average projected to increase, counteracting the O2,sol trend (Frölicher et al., 2009; Cocco et al., 2013; Bopp et al., 2017). Some regions show similar relative ToE but for different reasons. For example, in the North Pacific subtropical gyre and the Southern Ocean, both the oxygen depletion and the internal variability are relatively strong. In the Arabian Sea, internal variability and anthropogenic response are both rather weak. Nevertheless, the S∕N ratio results in very similar relative ToE for all these regions.
The transient climate response of the individual models and therefore the ocean heat uptake, thermocline warming and deoxygenation can substantially differ among models (Bopp et al., 2013). The simulated internal variability also differs considerably across models (e.g. Resplandy et al., 2015; Frölicher et al., 2016). ToE values computed from CESM1.0 projections, for example, differ by many decades in absolute values from other CMIP5 models, mostly due to a very weak internal variability. Nijsse et al. (2019) suggest that the magnitude of simulated decadal variability and climate sensitivity might be correlated. They suggest that models with a high climate sensitivity tend to simulate a high decadal variability. This may imply a compensation between the simulated signal and noise on the decadal scale. To extract valuable insights as to the relative spatial and temporal features of emergence across models and variables, we introduced a new metric, the relative time of emergence. Normalising the ToE using the globally averaged ToE as reference allows for a more direct comparison with the other models. As a result, the patterns and time of emergence of anthropogenic changes in O2 and warming in CESM1.0 are more coherent with the other models for ToErel than for the absolute ToE.
Following Hameau et al. (2019), we compared the ToE(T) with the ToE(O2) in nine models. We find that the anthropogenic decline in O2 emerges before anthropogenic warming in a significant part of the thermocline. On average across the nine models, an area covering 35±11 % of the global thermocline shows emergence in O2 change before temperature change. Yet, the exact locations of these patterns differ across models. Only 17 % of the global thermocline show agreement (seven out of the nine models) on earlier emergence of O2 changes prior to T changes; thus, our multi-model analysis confirms earlier findings using output from a single model only (Hameau et al., 2019). The early emergence of O2 suggests that the monitoring of biogeochemical variables would be particularly useful to detect early signals of anthropogenic change in the ocean interior (Joos et al., 2003). Multi-tracer observations of both physical and biogeochemical variables may enable an earlier detection of potential changes than temperature-only data (Keller et al., 2015) in specific regions and for specific processes.
Hameau et al. (2019) established a direct link between the early emergence in O2 with a slowdown of ventilation. A weaker ventilation leads to a decrease in [-AOU], and therefore a reduction in O2, with a minor role for organic matter export changes in their simulation. We used [-AOU] as a ventilation age proxy for our model ensemble and concluded that the slowdown of the ventilation induces O2 changes to be detectable before T changes in many regions. A slower ventilation seems to shift the balance between O2 supply from the surface and O2 consumption by organic matter remineralisation. Moreover, a more stratified upper ocean delays the propagation of the temperature signal from the surface into the subsurface waters. Note that the exact locations of early O2 emergence and reductions in [-AOU] and ventilation diverge among the models. This is partly due to model biases in terms of ocean dynamics. In addition, the use of depth coordinates to define a thermocline layer from 200 to 600 m may lead in our analysis to the inclusion of different water masses for different models. Another approach would be to perform the analysis on isopycnal levels instead on depth levels.
To conclude, normalising ToE across models (relative ToE) or estimating ToE in relation to another variable (ToE(T) – ToE(O2)) reduces the multi-model spread arising from method and model dependencies. We find that in about 35 % of the thermocline anthropogenic O2 depletion emerges before anthropogenic warming. This relative early emergence of O2 is linked to a more sluggish ventilation of these subsurface waters under global warming. Our study also suggests that temperatures in the thermocline have already left the bounds of internal variability in much of the tropical ocean and that temperatures will have left these bounds in most of the thermocline by 2100 under unabated global warming.
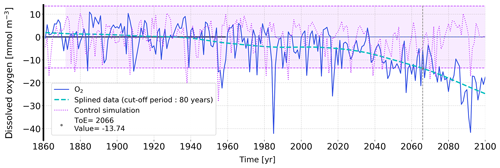
Figure A1Illustration of the ToE method by using simulated oxygen concentration of the MPI-ESM-LR model averaged over 200–600 m in one grid cell in the western North Pacific. The dark blue line shows that temporal evolution of oxygen anomalies from 1860 to 2099 relative to preindustrial concentrations (1860–1959). The two pink lines indicate the magnitude of the noise, N, which is computed as 2 standard deviations from annual output of the control simulation. The anthropogenic signal, S, is the simulated oxygen concentration over 1860 to 2099 from the forced simulation splined with a 80-year cut-off period (dashed cyan). Here, the resulting ToE is the intersection between the lower limit of the noise and the splined signal (vertical dashed line).
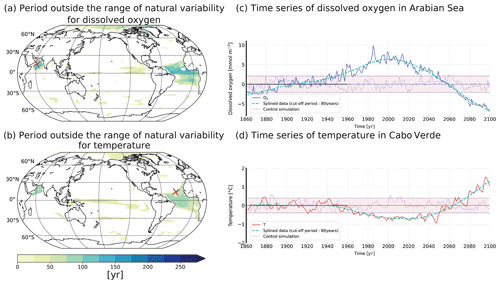
Figure A2Period outside the range of natural variability for (a) oxygen concentration and (b) temperature in the thermocline for the model HadGEM2. The time series show two examples of the temporal evolution of the oxygen concentration (c) and temperature (d) for a single grid point: red crosses in panels (a, b): Arabian Sea (c) and equatorial Atlantic (d).
The CMIP5 simulations are available on https://esgf-node.ipsl.upmc.fr (last access: June 2019, Taylor et al., 2012). Results from the CESM1.0 simulations are available upon request.
The supplement related to this article is available online at: https://doi.org/10.5194/bg-17-1877-2020-supplement.
All authors contributed to the discussion and the writing of the paper.
The authors declare that they have no conflict of interest.
This article is part of the special issue “Ocean deoxygenation: drivers and consequences – past, present and future (BG/CP/OS inter-journal SI)”. It is a result of the International Conference on Ocean Deoxygenation, Kiel, Germany, 3–7 September 2018.
Angélique Hameau, Fortunat Joos and Thomas L. Frölicher thank Christoph Raible and Flavio Lehner for providing CESM output. We also thank the World Climate Research Programme's Working Group on Coupled Modelling, which is responsible for CMIP5, and the climate modelling groups for producing and making available their model output. We thank the anonymous referee, Sarah Schlunegger and Ming Li for their time and effort.
This research has been supported by the Oeschger Center for Climate Change Research, the Swiss National Science Foundation (nos. 200020_172476 and PP00P2_170687) and the CSCS Swiss National Supercomputing Center, providing computing resources. This publication has received funding from the European Union’s Horizon 2020 research and innovation programme under grant agreement no. 820989 (project COMFORT, Our common future ocean in the Earth system – quantifying coupled cycles of carbon, oxygen, and nutrients for determining and achieving safe operating spaces 30 with respect to tipping points). The work reflects only the authors’ view; the European Commission and their executive agency are not responsible for any use that may be made of the information the work contains.
This paper was edited by Kenneth Rose and reviewed by Sarah Schlunegger, Ming Li, and one anonymous referee.
Aumont, O. and Bopp, L.: Globalizing results from ocean in situ iron fertilization studies, Global Biogeochem. Cy., 20, GB2017, https://doi.org/10.1029/2005GB002591, 2006. a
Battaglia, G. and Joos, F.: Hazards of decreasing marine oxygen: the near-term and millennial-scale benefits of meeting the Paris climate targets, Earth Syst. Dynam., 9, 797–816, https://doi.org/10.5194/esd-9-797-2018, 2018. a
Bilbao, R. A. F., Gregory, J. M., Bouttes, N., Palmer, M. D., and Stott, P.: Attribution of ocean temperature change to anthropogenic and natural forcings using the temporal, vertical and geographical structure, Clim. Dynam., 53, 5389–5413, https://doi.org/10.1007/s00382-019-04910-1, 2019. a
Bopp, L., Le Quéré, C., Heimann, M., Manning, A. C., and Monfray, P.: Climate-induced oceanic oxygen fluxes: Implications for the contemporary carbon budget, Global Biogeochem. Cy., 16,https://doi.org/10.1029/2001GB001445, 2002. a, b
Bopp, L., Resplandy, L., Orr, J. C., Doney, S. C., Dunne, J. P., Gehlen, M., Halloran, P., Heinze, C., Ilyina, T., Séférian, R., Tjiputra, J., and Vichi, M.: Multiple stressors of ocean ecosystems in the 21st century: projections with CMIP5 models, Biogeosciences, 10, 6225–6245, https://doi.org/10.5194/bg-10-6225-2013, 2013. a, b
Bopp, L., Resplandy, L., Untersee, A., Mezo, P. L., and Kageyama, M.: Ocean (de)oxygenation from the Last Glacial Maximum to the twenty-first century: insights from Earth System models, Philos. T. Roy. Soc. A, 375, 20160323, https://doi.org/10.1098/rsta.2016.0323, 2017. a, b, c, d
Brandt, P., Bange, H. W., Banyte, D., Dengler, M., Didwischus, S.-H., Fischer, T., Greatbatch, R. J., Hahn, J., Kanzow, T., Karstensen, J., Körtzinger, A., Krahmann, G., Schmidtko, S., Stramma, L., Tanhua, T., and Visbeck, M.: On the role of circulation and mixing in the ventilation of oxygen minimum zones with a focus on the eastern tropical North Atlantic, Biogeosciences, 12, 489–512, https://doi.org/10.5194/bg-12-489-2015, 2015. a
Breitburg, D., Levin, L. A., Oschlies, A., Grégoire, M., Chavez, F. P., Conley, D. J., Garçon, V., Gilbert, D., Gutiérrez, D., Isensee, K., Jacinto, G. S., Limburg, K. E., Montes, I., Naqvi, S. W. A., Pitcher, G. C., Rabalais, N. N., Roman, M. R., Rose, K. A., Seibel, B. A., Telszewski, M., Yasuhara, M., and Zhang, J.: Declining oxygen in the global ocean and coastal waters, Science, 359, 6371, https://doi.org/10.1126/science.aam7240, 2018. a
Cabré, A., Marinov, I., Bernardello, R., and Bianchi, D.: Oxygen minimum zones in the tropical Pacific across CMIP5 models: mean state differences and climate change trends, Biogeosciences, 12, 5429–5454, https://doi.org/10.5194/bg-12-5429-2015, 2015. a, b
Capotondi, A., Alexander, M. A., Bond, N. A., Curchitser, E. N., and Scott, J. D.: Enhanced upper ocean stratification with climate change in the CMIP3 models, J. Geophys. Res.-Oceans, 117, C04031, https://doi.org/10.1029/2011JC007409, 2012. a
Chen, X. and Tung, K.-K.: Global surface warming enhanced by weak Atlantic overturning circulation, Nature, 559, 387–391, https://doi.org/10.1038/s41586-018-0320-y, 2018. a
Cheng, L., Trenberth, K. E., Fasullo, J., Boyer, T., Abraham, J., and Zhu, J.: Improved estimates of ocean heat content from 1960 to 2015, Sci. Adv., 3, 3, https://doi.org/10.1126/sciadv.1601545, 2017. a
Cheng, L., Abraham, J., Hausfather, Z., and Trenberth, K. E.: How fast are the oceans warming?, Science, 363, 128–129, https://doi.org/10.1126/science.aav7619, 2019. a
Cheung, W. W., Jones, M. C., Reygondeau, G., and Frölicher, T. L.: Opportunities for climate-risk reduction through effective fisheries management, Global Change Biol., 24, 5149–5163, 2018. a
Cheung, W. W. L., Dunne, J., Sarmiento, J. L., and Pauly, D.: Integrating ecophysiology and plankton dynamics into projected maximum fisheries catch potential under climate change in the Northeast Atlantic, ICES J. Mar. Sci., 68, 1008–1018, https://doi.org/10.1093/icesjms/fsr012, 2011. a
Christensen, J., Hewitson, B., Busuioc, A., Chen, A., Gao, X., Held, I., Jones, R., Kolli, R. K., Kwon, W.-T., Laprise, R., Magaña Rueda, V., Mearns, L., Menéndez, C. G., Räisänen, J., Rinke, A., Sarr, A., and Whetton, P.: Regional Climate Projections, in: Climate Change 2007: The Physical Science Basis, Contribution of Working Group I to the Fourth Assessment Report of the Intergovernmental Panel on Climate Change, Cambridge University Press, Cambridge, United Kingdom and New York, NY, USA, 848–940, 2007. a
Cocco, V., Joos, F., Steinacher, M., Frölicher, T. L., Bopp, L., Dunne, J., Gehlen, M., Heinze, C., Orr, J., Oschlies, A., Schneider, B., Segschneider, J., and Tjiputra, J.: Oxygen and indicators of stress for marine life in multi-model global warming projections, Biogeosciences, 10, 1849–1868, https://doi.org/10.5194/bg-10-1849-2013, 2013. a, b, c, d
Collins, W. J., Bellouin, N., Doutriaux-Boucher, M., Gedney, N., Halloran, P., Hinton, T., Hughes, J., Jones, C. D., Joshi, M., Liddicoat, S., Martin, G., O'Connor, F., Rae, J., Senior, C., Sitch, S., Totterdell, I., Wiltshire, A., and Woodward, S.: Development and evaluation of an Earth-System model – HadGEM2, Geosci. Model Dev., 4, 1051–1075, https://doi.org/10.5194/gmd-4-1051-2011, 2011. a, b
Danabasoglu, G., Bates, S. C., Briegleb, B. P., Jayne, S. R., Jochum, M., Large, W. G., Peacock, S., and Yeager, S. G.: The CCSM4 Ocean Component, J. Climate, 25, 1361–1389, https://doi.org/10.1175/JCLI-D-11-00091.1, 2011. a
Deutsch, C., Ferrel, A., Seibel, B., Pörtner, H.-O., and Huey, R. B.: Climate change tightens a metabolic constraint on marine habitats, Science, 348, 1132–1135, https://doi.org/10.1126/science.aaa1605, 2015. a, b, c
Dufresne, J.-L., Foujols, M.-A., Denvil, S., Caubel, A., Marti, O., Aumont, O., Balkanski, Y., Bekki, S., Bellenger, H., Benshila, R., Bony, S., Bopp, L., Braconnot, P., Brockmann, P., Cadule, P., Cheruy, F., Codron, F., Cozic, A., Cugnet, D., de Noblet, N., Duvel, J.-P., Ethé, C., Fairhead, L., Fichefet, T., Flavoni, S., Friedlingstein, P., Grandpeix, J.-Y., Guez, L., Guilyardi, E., Hauglustaine, D., Hourdin, F., Idelkadi, A., Ghattas, J., Joussaume, S., Kageyama, M., Krinner, G., Labetoulle, S., Lahellec, A., Lefebvre, M.-P., Lefevre, F., Levy, C., Li, Z. X., Lloyd, J., Lott, F., Madec, G., Mancip, M., Marchand, M., Masson, S., Meurdesoif, Y., Mignot, J., Musat, I., Parouty, S., Polcher, J., Rio, C., Schulz, M., Swingedouw, D., Szopa, S., Talandier, C., Terray, P., Viovy, N., and Vuichard, N.: Climate change projections using the IPSL-CM5 Earth System Model: from CMIP3 to CMIP5, Clim. Dynam., 40, 2123–2165, https://doi.org/10.1007/s00382-012-1636-1, 2013. a
Dunne, J. P., John, J. G., Adcroft, A. J., Griffies, S. M., Hallberg, R. W., Shevliakova, E., Stouffer, R. J., Cooke, W., Dunne, K. A., Harrison, M. J., Krasting, J. P., Malyshev, S. L., Milly, P. C. D., Phillipps, P. J., Sentman, L. T., Samuels, B. L., Spelman, M. J., Winton, M., Wittenberg, A. T., and Zadeh, N.: GFDL's ESM2 Global Coupled Climate-Carbon Earth System Models, Part I: Physical Formulation and Baseline Simulation Characteristics, J. Climate, 25, 6646–6665, https://doi.org/10.1175/JCLI-D-11-00560.1, 2012. a
Dunne, J. P., John, J. G., Shevliakova, E., Stouffer, R. J., Krasting, J. P., Malyshev, S. L., Milly, P. C. D., Sentman, L. T., Adcroft, A. J., Cooke, W., Dunne, K. A., Griffies, S. M., Hallberg, R. W., Harrison, M. J., Levy, H., Wittenberg, A. T., Phillips, P. J., and Zadeh, N.: GFDL’s ESM2 Global Coupled Climate-Carbon Earth System Models, Part II: Carbon System Formulation and Baseline Simulation Characteristics, J. Climate, 26, 2247–2267, https://doi.org/10.1175/JCLI-D-12-00150.1, 2013. a, b
Enting, I. G.: On the use of smoothing splines to filter CO2 data, J. Geophys. Res.-Atmos., 92, 10977–10984, https://doi.org/10.1029/JD092iD09p10977, 1987. a
Frame, D., Joshi, M., Hawkins, E., Harrington, L. J., and de Roiste, M.: Population-based emergence of unfamiliar climates, Nat. Clim. Change, 7, 407–411, https://doi.org/10.1038/nclimate3297, 2017. a
Frölicher, T. L. and Paynter, D. J.: Extending the relationship between global warming and cumulative carbon emissions to multi-millennial timescales, Environ. Res. Lett., 10, 075002, https://doi.org/10.1088/1748-9326/10/7/075002, 2015. a
Frölicher, T. L., Joos, F., Plattner, G.-K., Steinacher, M., and Doney, S. C.: Natural variability and anthropogenic trends in oceanic oxygen in a coupled carbon cycle–climate model ensemble, Global Biogeochem. Cy., 23, GB1003, https://doi.org/10.1029/2008GB003316, 2009. a
Frölicher, T. L., Rodgers, K. B., Stock, C. A., and Cheung, W. W. L.: Sources of uncertainties in 21st century projections of potential ocean ecosystem stressors, Global Biogeochem. Cy., 30, 1224–1243, https://doi.org/10.1002/2015GB005338, 2016. a, b, c, d, e
Garcia, H. E. and Gordon, L. I.: Oxygen solubility in seawater: Better fitting equations, Limnol. Oceanogr., 37, 1307–1312, https://doi.org/10.4319/lo.1992.37.6.1307, 1992. a
Gattuso, J.-P., Magnan, A., Billé, R., Cheung, W. W. L., Howes, E. L., Joos, F., Allemand, D., Bopp, L., Cooley, S. R., Eakin, C. M., Hoegh-Guldberg, O., Kelly, R. P., Pörtner, H.-O., Rogers, A. D., Baxter, J. M., Laffoley, D., Osborn, D., Rankovic, A., Rochette, J., Sumaila, U. R., Treyer, S., and Turley, C.: Contrasting futures for ocean and society from different anthropogenic CO2 emissions scenarios, Science, 349, 6243, https://doi.org/10.1126/science.aac4722, 2015. a, b
Giorgetta, M. A., Jungclaus, J., Reick, C. H., Legutke, S., Bader, J., Böttinger, M., Brovkin, V., Crueger, T., Esch, M., Fieg, K., Glushak, K., Gayler, V., Haak, H., Hollweg, H.-D., Ilyina, T., Kinne, S., Kornblueh, L., Matei, D., Mauritsen, T., Mikolajewicz, U., Mueller, W., Notz, D., Pithan, F., Raddatz, T., Rast, S., Redler, R., Roeckner, E., Schmidt, H., Schnur, R., Segschneider, J., Six, K. D., Stockhause, M., Timmreck, C., Wegner, J., Widmann, H., Wieners, K.-H., Claussen, M., Marotzke, J., and Stevens, B.: Climate and carbon cycle changes from 1850 to 2100 in MPI-ESM simulations for the Coupled Model Intercomparison Project phase 5, J. Adv. Model. Earth. Sy., 5, 572–597, https://doi.org/10.1002/jame.20038, 2013. a
Gnanadesikan, A., Russell, J. L., and Fanrong Zeng: How does ocean ventilation change under global warming?, Ocean Sci., 3, 43–53, https://doi.org/10.5194/os-3-43-2007, 2007. a
Gnanadesikan, A., Dunne, J. P., and John, J.: Understanding why the volume of suboxic waters does not increase over centuries of global warming in an Earth System Model, Biogeosciences, 9, 1159–1172, https://doi.org/10.5194/bg-9-1159-2012, 2012. a
Griffies, S. M., Winton, M., Donner, L. J., Horowitz, L. W., Downes, S. M., Farneti, R., Gnanadesikan, A., Hurlin, W. J., Lee, H.-C., Liang, Z., Palter, J. B., Samuels, B. L., Wittenberg, A. T., Wyman, B. L., Yin, J., and Zadeh, N.: The GFDL CM3 Coupled Climate Model: Characteristics of the Ocean and Sea Ice Simulations, J. Climate, 24, 3520–3544, https://doi.org/10.1175/2011JCLI3964.1, 2011. a
Hallberg, R.: Some aspects of the circulation in ocean basins with isopycnals intersecting sloping boundaries, University of Washington, https://books.google.ch/books?id=sdbENwAACAAJ, 488 pp., 1997. a
Hameau, A., Mignot, J., and Joos, F.: Assessment of time of emergence of anthropogenic deoxygenation and warming: insights from a CESM simulation from 850 to 2100 CE, Biogeosciences, 16, 1755–1780, https://doi.org/10.5194/bg-16-1755-2019, 2019. a, b, c, d, e, f, g, h, i, j, k, l, m, n, o, p
Han, W., Vialard, J., McPhaden, M. J., Lee, T., Masumoto, Y., Feng, M., and de Ruijter, W. P.: Indian Ocean Decadal Variability: A Review, B. Am. Meteorol. Soc., 95, 1679–1703, https://doi.org/10.1175/BAMS-D-13-00028.1, 2014. a
Hauri, C., Gruber, N., McDonnell, A. M. P., and Vogt, M.: The intensity, duration, and severity of low aragonite saturation state events on the California continental shelf, Geophys. Res. Lett., 40, 3424–3428, https://doi.org/10.1002/grl.50618, 2013. a
Hawkins, E. and Sutton, R.: Time of emergence of climate signals, Geophys. Res. Lett., 39, L01702, https://doi.org/10.1029/2011GL050087, 2012. a, b, c
Henson, S. A., Beaulieu, C., and Lampitt, R.: Observing climate change trends in ocean biogeochemistry: when and where, Global Change Biol., 22, 1561–1571, https://doi.org/10.1111/gcb.13152, 2016. a, b, c
Henson, S. A., Beaulieu, C., Ilyina, T., John, J. G., Long, M., Séférian, R., Tjiputra, J., and Sarmiento, J. L.: Rapid emergence of climate change in environmental drivers of marine ecosystems, Nat. Commun., 8, 14682, https://doi.org/10.1038/ncomms14682, 2017. a, b, c
Hurrell, J. W., Holland, M. M., Gent, P. R., Ghan, S., Kay, J. E., Kushner, P. J., Lamarque, J.-F., Large, W. G., Lawrence, D., Lindsay, K., Lipscomb, W. H., Long, M. C., Mahowald, N., Marsh, D. R., Neale, R. B., Rasch, P., Vavrus, S., Vertenstein, M., Bader, D., Collins, W. D., Hack, J. J., Kiehl, J., and Marshall, S.: The Community Earth System Model: A Framework for Collaborative Research, B. Am. Meteorol. Soc., 94, 1339–1360, https://doi.org/10.1175/BAMS-D-12-00121.1, 2013. a
Ilyina, T., Six, K. D., Segschneider, J., Maier‐Reimer, E., Li, H., and Núñez‐Riboni, I.: Global ocean biogeochemistry model HAMOCC: Model architecture and performance as component of the MPI-Earth system model in different CMIP5 experimental realizations, J. Adv. Model. Earth Sy., 5, 287–315, https://doi.org/10.1029/2012MS000178, 2013. a
IPCC: Summary for policymakers, in: IPCC Special Report on the Ocean and Cryosphere in a Changing Climate, edited by: Pörtner, H.-O., Roberts, D., Masson-Delmotte, V., Zhai, P., Tignor, M., Poloczanska, E., Mintenbeck, K., Nicolai, M., Okem, A., Petzold, J., Rama, B., and Weyer, N., https://www.ipcc.ch/srocc/home/, in press, 2019. a
Joos, F., Plattner, G.-K., Stocker, T. F., Körtzinger, A., and Wallace, D. W. R.: Trends in marine dissolved oxygen: Implications for ocean circulation changes and the carbon budget, EOS T. Am. Geophys. Un., 84, 197–201, https://doi.org/10.1029/2003EO210001, 2003. a, b
Jungclaus, J. H., Fischer, N., Haak, H., Lohmann, K., Marotzke, J., Matei, D., Mikolajewicz, U., Notz, D., and von Storch, J. S.: Characteristics of the ocean simulations in the Max Planck Institute Ocean Model (MPIOM) the ocean component of the MPI-Earth system model, J. Adv. Model. Earth Sy., 5, 422–446, https://doi.org/10.1002/jame.20023, 2013. a
Keller, K. M., Joos, F., and Raible, C. C.: Time of emergence of trends in ocean biogeochemistry, Biogeosciences, 11, 3647–3659, https://doi.org/10.5194/bg-11-3647-2014, 2014. a, b
Keller, K. M., Joos, F., Lehner, F., and Raible, C. C.: Detecting changes in marine responses to ENSO from 850 to 2100 CE, Insights from the ocean carbon cycle, Geophys. Res. Lett., 42, https://doi.org/10.1002/2014GL062398, 2015. a, b
Knutti, R. and Sedláček, J.: Robustness and uncertainties in the new CMIP5 climate model projections, Nat. Clim. Change, 3, 369–373, https://doi.org/10.1038/nclimate1716, 2013. a
Lehner, F., Joos, F., Raible, C. C., Mignot, J., Born, A., Keller, K. M., and Stocker, T. F.: Climate and carbon cycle dynamics in a CESM simulation from 850 to 2100 CE, Earth Syst. Dynam., 6, 411–434, https://doi.org/10.5194/esd-6-411-2015, 2015. a
Levitus, S., Antonov, J. I., Boyer, T. P., Locarnini, R. A., Garcia, H. E., and Mishonov, A. V.: Global ocean heat content 1955–2008 in light of recently revealed instrumentation problems, Geophys. Res. Lett., 36, L07608, https://doi.org/10.1029/2008gl037155, 2009. a
Levitus, S., Antonov, J. I., Boyer, T. P., Baranova, O. K., Garcia, H. E., Locarnini, R. A., Mishonov, A. V., Reagan, J. R., Seidov, D., Yarosh, E. S., and Zweng, M. M.: World ocean heat content and thermosteric sea level change (0–2000 m), 1955–2010, Geophys. Res. Lett., 39, L10603, https://doi.org/10.1029/2012GL051106, 2012. a
Lombardozzi, D., Bonan, G. B., and Nychka, D. W.: The emerging anthropogenic signal in land-atmosphere carbon-cycle coupling, Nat. Clim. Change, 4, 796–800, https://doi.org/10.1038/nclimate2323, 2014. a
Long, M. C., Deutsch, C., and Ito, T.: Finding forced trends in oceanic oxygen, Global Biogeochem. Cy., 30, 381–397, https://doi.org/10.1002/2015GB005310, 2016. a, b
Madec, G., Bourdallé-Badie, R., Bouttier, P.-A., Bricaud, C., Bruciaferri, D., Calvert, D., Chanut, J., Clementi, E., Coward, A., Delrosso, D., Ethé, C., Flavoni, S., Graham, T., Harle, J., Iovino, D., Lea, D., Lévy, C., Lovato, T., Martin, N., Masson, S., Mocavero, S., Paul, J., Rousset, C., Storkey, D., Storto, A., and Vancoppenolle, M.: NEMO ocean engine, available at: https://zenodo.org/record/3248739/#.XmYhkmbTW71 (last access: November 2019), 2017. a
Magnan, A. K., Colombier, M., Billé, R., Joos, F., Hoegh-Guldberg, O., Pörtner, H.-O., Waisman, H., Spencer, T., and Gattuso, J.-P.: Implications of the Paris agreement for the ocean, Nat. Clim. Change, 6, 732–735, https://doi.org/10.1038/nclimate3038, 2016. a
Margolskee, A., Frenzel, H., Emerson, S., and Deutsch, C.: Ventilation Pathways for the North Pacific Oxygen Deficient Zone, Global Biogeochem. Cy., 33, 875–890, https://doi.org/10.1029/2018GB006149, 2019. a
Matear, R. J. and Hirst, A. C.: Long-term changes in dissolved oxygen concentrations in the ocean caused by protracted global warming, Global Biogeochem. Cy., 17, 1125, https://doi.org/10.1029/2002GB001997, 2003. a
Moore, J. K., Doney, S. C., Kleypas, J. A., Glover, D. M., and Fung, I. Y.: An intermediate complexity marine ecosystem model for the global domain, Deep-Sea Res. Pt. II, 49, 403–462, https://doi.org/10.1016/S0967-0645(01)00108-4, 2002. a
Moore, J. K., Doney, S. C., and Lindsay, K.: Upper ocean ecosystem dynamics and iron cycling in a global three-dimensional model, Global Biogeochem. Cy., 18, GB4028, https://doi.org/10.1029/2004GB002220, 2004. a
Nijsse, F. J. M. M., Cox, P. M., Huntingford, C., and Williamson, M. S.: Decadal global temperature variability increases strongly with climate sensitivity, Nat. Clim. Change, 9, 598–601, https://doi.org/10.1038/s41558-019-0527-4, 2019. a
Oschlies, A., Duteil, O., Getzlaff, J., Koeve, W., Landolfi, A., and Schmidtko, S.: Patterns of deoxygenation: sensitivity to natural and anthropogenic drivers, Philos. T. Roy. Soc. A, 375, 2102, https://doi.org/10.1098/rsta.2016.0325, 2017. a
Palmer, J. R. and Totterdell, I. J.: Production and export in a global ocean ecosystem model, Deep-Sea Res. Pt. I, 48, 1169–1198, https://doi.org/10.1016/S0967-0637(00)00080-7, 2001. a
Plattner, G.-K., Joos, F., and Stocker, T. F.: Revision of the global carbon budget due to changing air-sea oxygen fluxes, Global Biogeochem. Cy., 16, 1096, https://doi.org/10.1029/2001GB001746, 2002. a
Pörtner, H.-O., Karl, D. M., Boyd, P. W., Cheung, W., Lluch-Cota, S. E., Nojiri, Y., Schmidt, D. N., Zavialov, P. O., Alheit, J., and Aristegui, J.: Ocean systems, in: Climate change 2014: Impacts, adaptation, and vulnerability, Part A: global and sectoral aspects, Contribution of working group II to the fifth assessment report of the intergovernmental panel on climate change, Cambridge University Press, 411–484, 2014. a, b
Resplandy, L., Séférian, R., and Bopp, L.: Natural variability of CO2 and O2 fluxes: What can we learn from centuries-long climate models simulations?, J. Geophys. Res.-Oceans, 120, 384–404, https://doi.org/10.1002/2014JC010463, 2015. a
Rhein, M., Rintoul, S., Aoki, S., Campos, E., Chambers, D., Feely, R., Gulev, S., Johnson, G., Josey, S., Kostianoy, A., Mauritzen, C., Roemmich, D., Talley, L., and Wang, F.: Observations: Ocean, in: Climate Change 2013: The Physical Science Basis, Contribution of Working Group I to the Fifth Assessment Report of the Intergovernmental Panel on Climate Change, edited by: Stocker, T., Qin, D., Plattner, G.-K., Tignor, M., Allen, S., Boschung, J., Nauels, A., Xia, Y., Bex, V., and Midgley, P., Cambridge University Press, Cambridge, United Kingdom and New York, NY, USA, 255–316, https://doi.org/10.1017/CBO9781107415324.010, 2013. a
Riahi, K., Rao, S., Krey, V., Cho, C., Chirkov, V., Fischer, G., Kindermann, G., Nakicenovic, N., and Rafaj, P.: RCP 8.5 – A scenario of comparatively high greenhouse gas emissions, Clim. Change, 109, 33, https://doi.org/10.1007/s10584-011-0149-y, 2011. a
Rodgers, K. B., Lin, J., and Frölicher, T. L.: Emergence of multiple ocean ecosystem drivers in a large ensemble suite with an Earth system model, Biogeosciences, 12, 3301–3320, https://doi.org/10.5194/bg-12-3301-2015, 2015. a, b, c, d
Roemmich, D., Church, J., Gilson, J., Monselesan, D., Sutton, P., and Wijffels, S.: Unabated planetary warming and its ocean structure since 2006, Nat. Clim. Change, 5, 240–245, https://doi.org/10.1038/nclimate2513, 2015. a
Sarmiento, J. L., Hughes, T. M. C., Stouffer, R. J., and Manabe, S.: Simulated response of the ocean carbon cycle to anthropogenic climate warming, Nature, 393, 245, https://doi.org/10.1038/30455, 1998. a, b
Schlunegger, S., Rodgers, K. B., Sarmiento, J. L., Frölicher, T. L., Dunne, J. P., Ishii, M., and Slater, R.: Emergence of anthropogenic signals in the ocean carbon cycle, Nat. Clim. Change, 9, 719–725, https://doi.org/10.1038/s41558-019-0553-2, 2019. a
Schmidtko, S., Stramma, L., and Visbeck, M.: Decline in global oceanic oxygen content during the past five decades, Nature, 542, 335–339, https://doi.org/10.1038/nature21399, 2017. a
Smith, R., Jones, P., Briegleb, P., Bryan, O., Danabasoglu, G., Dennis, M., Dukowicz, J., Eden, C., Fox-Kemper, B., Gent, R., Hecht, M., Jayne, S., Jochum, M., Large, G., Lindsay, K., Maltrud, M., Norton, J., Peacock, L., Vertenstein, M., and Yeager, S.: The Parallel Ocean Program (POP) reference manual: Ocean component of the Community Climate System Model (CCSM), Tech. Rep., Los Alamos National Laboratory (LANL), available at: https://opensky.ucar.edu/islandora/object/manuscripts%3A825/ (last access: November 2019), 2010. a
Stramma, L., Johnson, G. C., Sprintall, J., and Mohrholz, V.: Expanding Oxygen-Minimum Zones in the Tropical Oceans, Science, 320, 655–658, https://doi.org/10.1126/science.1153847, 2008. a, b
Stramma, L., Prince, E. D., Schmidtko, S., Luo, J., Hoolihan, J. P., Visbeck, M., Wallace, D. W. R., Brandt, P., and Körtzinger, A.: Expansion of oxygen minimum zones may reduce available habitat for tropical pelagic fishes, Nat. Clim. Change, 2, 33–37, https://doi.org/10.1038/nclimate1304, 2012. a
Stroeve, J. C., Kattsov, V., Barrett, A., Serreze, M., Pavlova, T., Holland, M., and Meier, W. N.: Trends in Arctic sea ice extent from CMIP5, CMIP3 and observations, Geophys. Res. Lett., 39, L16502, https://doi.org/10.1029/2012GL052676, 2012. a
Taylor, K. E., Stouffer, R. J., and Meehl, G. A.: An Overview of CMIP5 and the Experiment Design, B. Am. Meteorol. Soc., 93, 485–498, https://doi.org/10.1175/BAMS-D-11-00094.1, 2012. a, b
Tjiputra, J. F., Goris, N., Lauvset, S. K., Heinze, C., Olsen, A., Schwinger, J., and Steinfeldt, R.: Mechanisms and Early Detections of Multidecadal Oxygen Changes in the Interior Subpolar North Atlantic, Geophys. Res. Lett., 45, 4218–4229, https://doi.org/10.1029/2018GL077096, 2018. a, b
Wang, M. and Overland, J. E.: A sea ice free summer Arctic within 30 years: An update from CMIP5 models, Geophys. Res. Lett., 39, L18501, https://doi.org/10.1029/2012GL052868, 2012. a