the Creative Commons Attribution 4.0 License.
the Creative Commons Attribution 4.0 License.
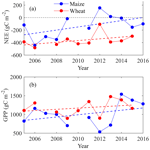
Decadal variation in CO2 fluxes and its budget in a wheat and maize rotation cropland over the North China Plain
Dawen Yang
Lihua Xiong
Beijing Fang
Carbon sequestration in agroecosystems has great potential to mitigate global greenhouse gas emissions. To assess the decadal trend of CO2 fluxes of an irrigated wheat–maize rotation cropland over the North China Plain, the net ecosystem exchange (NEE) with the atmosphere was measured by using an eddy covariance system from 2005 to 2016. To evaluate the detailed CO2 budget components of this representative cropland, a comprehensive experiment was conducted in the full 2010–2011 wheat–maize rotation cycle by combining the eddy covariance NEE measurements, plant carbon storage samples, and a soil respiration experiment that differentiated between heterotrophic and below-ground autotrophic respirations. Over the past decade (from 2005 to 2016), the cropland exhibited a statistically nonsignificant decreasing carbon sequestration capacity; the average of total NEE, gross primary productivity (GPP), and ecosystem respiration (ER), respectively, were −364, 1174, and 810 gC m−2 for wheat and −136, 1008, and 872 gC m−2 for maize. The multiple regression revealed that air temperature and groundwater depth showed pronounced correlations with the CO2 fluxes for wheat. However, in the maize season, incoming shortwave radiation and groundwater depth showed pronounced correlations with CO2 fluxes. For the full 2010–2011 agricultural cycle, the CO2 fluxes for wheat and maize were as follows: for NEE they were −438 and −239 gC m−2, for GPP 1078 and 780 gC m−2, for ER 640 and 541 gC m−2, for soil heterotrophic respiration 377 and 292 gC m−2, for below-ground autotrophic respiration 136 and 115 gC m−2, and for above-ground autotrophic respiration 128 and 133 gC m−2. The net biome productivity was 59 gC m−2 for wheat and 5 gC m−2 for maize, indicating that wheat was a weak CO2 sink and maize was close to CO2 neutral to the atmosphere for this agricultural cycle. However, when considering the total CO2 loss in the fallow period, the net biome productivity was −40 gC m−2 yr−1 for the full 2010–2011 cycle, implying that the cropland was a weak CO2 source. The investigations of this study showed that taking cropland as a climate change mitigation tool is challenging and that further studies are required for the CO2 sequestration potential of croplands.
The widely used eddy covariance technique (Aubinet et al., 2000; Baldocchi et al., 2001; Falge et al., 2002a, b) has enabled us to better understand the terrestrial CO2 exchange with the atmosphere, and has thereby fostered our understanding of the mechanisms through which terrestrial ecosystems contribute to mitigating ongoing climate change (Falkowski et al., 2000; Gray et al., 2014; Poulter et al., 2014; Forkel et al., 2016). Agroecosystems play an important role in regulating the global carbon balance (Lal, 2001; Bondeau et al., 2007; Özdoğan, 2011; Taylor et al., 2013; Gray et al., 2014) and are believed to have great potential to mitigate global carbon emissions through cropland management (Sauerbeck, 2001; Freibauer et al., 2004; Smith, 2004; Hutchinson et al., 2007; van Wesemael et al., 2010; Ciais et al., 2011; Schmidt et al., 2012). Furthermore, some studies proposed using agroecosystems as “natural climate solutions” to mitigate global carbon emissions (e.g., Griscom et al., 2017; Fargione et al., 2018). Field management practices (e.g., irrigation, fertilization and residue removal, etc.) impact the cropland CO2 fluxes (Baker and Griffis, 2005; Béziat et al., 2009; Ceschia et al., 2010; Eugster et al., 2010; Drewniak et al., 2015; de la Motte et al., 2016; Hunt et al., 2016; Vick et al., 2016), but their relative importance in determining the cropland CO2 budget remain unclear because of limited field observations (Kutsch et al., 2010), motivating comprehensive CO2 budget assessments across different cropland management styles.
Over the past 2 decades, CO2 investigations of agroecosystems have mainly focused on the variations in the net ecosystem exchange with the atmosphere (i.e., net ecosystem exchange, NEE) or its two derived components (i.e., gross primary productivity, GPP, and ecosystem respiration, ER) using the eddy covariance method. To date, these evaluations have been widely conducted for wheat (Gilmanov et al., 2003; Anthoni et al., 2004a; Moureaux et al., 2008; Béziat et al., 2009; Vick et al., 2016), maize (Verma et al., 2005), sugar beet (Aubinet et al., 2000; Moureaux et al., 2006), potato (Anthoni et al., 2004b; Fleisher et al., 2008), soybean–maize rotation cropland (Gilmanov et al., 2003; Hollinger et al., 2005; Suyker et al., 2005; Verma et al., 2005; Grant et al., 2007) and winter wheat–summer maize cropland (Zhang et al., 2008; Lei and Yang, 2010). However, the long-term variations in the cropland CO2 fluxes remain limited, leaving our knowledge of cropland potential as a future climate change mitigation tool incomplete.
The widely used eddy covariance technique has fostered our understanding of the integrated fluxes of NEE, GPP and ER but cannot provide detailed CO2 budget components, which consist of carbon assimilation (i.e., GPP), soil heterotrophic respiration (RH), above-ground autotrophic respiration (RAA), below-ground autotrophic respiration (RAB), lateral carbon export at harvest, and import at sowing or through organic fertilization (Ceschia et al., 2010). These different CO2 components result from different biological and biophysical processes (Moureaux et al., 2008) that may respond differently to climatic conditions, environmental factors and management strategies (Ekblad et al., 2005; Zhang et al., 2013). Differentiating among these components is a prerequisite for understanding the response of terrestrial ecosystems to changing environment (Heimann and Reichstein, 2008), thus the carbon budget evaluations have been reported for a few croplands (e.g., Moureaux et al., 2008; Ceschia et al., 2010; Wang et al., 2015; Demyan et al., 2016; Gao et al., 2017). In particular, to account for the literal carbon export, the net biome productivity (NBP) is often estimated by combining the eddy covariance technique and field carbon measurements associated with harvests and residue treatments (Ceschia et al., 2010; Kutsch et al., 2010). As a detailed CO2 budget might facilitate better predictions of agroecosystems' responses to climate change, CO2 budget evaluations in different croplands remain necessary.
The North China Plain (NCP) is one of the most important food production regions in China, and it guarantees national food security by providing more than 50 % and 33 % of the nation's wheat and maize, respectively (Kendy et al., 2003). Irrigation by diverting water from the Yellow River is common to alleviate water stress during spring in the NCP, resulting in a very shallow groundwater depth (usually range from 2 to 4 m) along the Yellow River (Cao et al., 2016) (Fig. 1). Wang et al. (2015) suggested that groundwater-fed cropland in the NCP had been losing carbon, and other studies also reported croplands in this region as carbon sources (e.g., Li et al., 2006; Luo et al., 2008). However, the long-term variations (e.g., >10 years) of the CO2 fluxes over the NCP remain lacking, leaving the trend of carbon sequestration capacity of this region unknown.
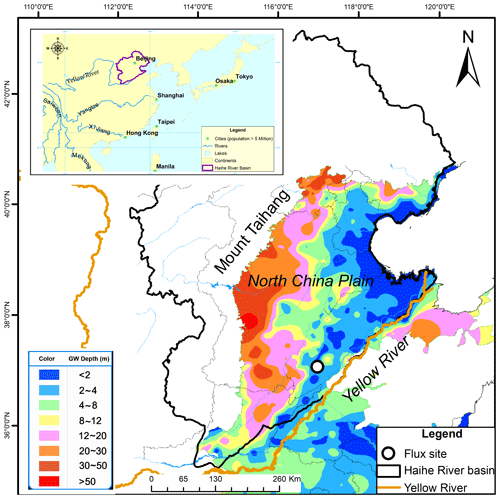
Figure 1Location of the experimental site. The background is the shallow groundwater depth in early September of 2011 provided by the © Water Information Center in the Ministry of Water Resources, P.R. China.
To this end, this study is designed to assess the long-term variation in CO2 fluxes and its budget of the representative wheat–maize rotation cropland in the NCP. The eddy covariance system was used to measure the CO2 exchange from 2005 to 2016. For the full 2010–2011 agricultural cycle, we measured soil respiration and sampled crops to quantify the detailed CO2 budget components. These measurements allow us to (1) investigate the decadal CO2 flux (NEE, GPP and ER) trend over this cropland; (2) provide detailed CO2 budget components; and (3) estimate the net primary productivity (NPP), net ecosystem productivity (NEP), and NBP.
2.1 Site description and field management
The experiment was conducted in a rectangular-shaped (460 m × 280 m) field of the representative cropland over the NCP (36∘39′ N, 116∘03′ E, Weishan site of Tsinghua University, Fig. 1). The soil is silt loam with a field capacity of 0.33 m3 m−3 and saturation point of 0.45 m3 m−3 for the top 5 cm of the soil. The mean annual precipitation is 532 mm and the mean air temperature is +13.3 ∘C. The winter wheat–summer maize rotation system is the representative cropping style in this region. On average, the winter wheat is sown around 17 October and harvested around 16 June of the following year with crop residues left on the field; summer maize is sown following the wheat harvest around 17 June and harvested around 16 October. Prior to sowing wheat of the next season, the field is thoroughly plowed to fully incorporate maize residues into the top 20 cm of the soil. The canopies of both wheat and maize are very uniform across the whole season. Nitrogen fertilizer is commonly applied at this site with the amount being 35 gN m−2 for wheat and 20 gN m−2 for maize. The crop density is 775 plants per square meter for wheat with a ridge spacing of 0.26 m and 4.9 plants per square meter for maize with a ridge spacing of 0.63 m on average. Wheat is commonly irrigated with water diverted from the Yellow River and the irrigation is about 150 mm every year; maize is rarely irrigated because of the high precipitation in the summer. During the 2010–2011 agricultural cycle, when CO2 budget components were evaluated, winter wheat was sown on 23 October 2010 and subsequently harvested on 10 June 2011 and summer maize was sown on 23 June 2011 and harvested on 30 September 2011. The entire year from 23 October 2010 to 22 October 2011 was studied for the annual CO2 budget evaluation.
2.2 Eddy covariance measurements
A flux tower was set up at the center of the experiment field in 2005 (Lei and Yang, 2010; Zhang et al., 2013). The NEE was measured at 3.7 m above ground with an eddy covariance system consisting of an infrared gas analyzer (LI-7500, LI-COR Inc., Lincoln, NE, USA) and a three-dimensional sonic anemometer (CSAT3, Campbell Scientific Inc., Logan, UT, USA). The 30 min averaged NEE was calculated from the 10 Hz raw measurements with TK2 (Mauder and Foken, 2004) from 2005 to 2012 and TK3 software package (Mauder and Foken, 2011) from 2013 to 2016. The storage flux was calculated by assuming a constant CO2 concentration profile. Nighttime measurements under stable atmospheric conditions with a friction velocity lower than 0.1 m s−1 were removed from the analysis (Lei and Yang, 2010). In the gap-filling procedure, gaps less than 2 h were filled using linear regression, while other short gaps were filled using the Mean Diurnal Variation (MDV) method (Falge et al., 2001); gaps longer than 4 weeks were not filled. NEE was further partitioned to derive GPP and ER using the nighttime method (Reichstein et al., 2005; Lei and Yang, 2010), which assumes that daytime and nighttime ER follow the same temperature response, which thereby estimates the daytime ER using the regression model derived from the nighttime measurements. In particular, this study adopted the method proposed by Reichstein et al. (2005) to quantify the short-term temperature sensitivity of ER from nighttime measurements as described by the van 't Hoff equation,
where TS is soil temperature, ERref is the reference respiration at 0 ∘C and b is a parameter associated with the commonly used temperature sensitivity coefficient Q10,
The long-term temperature sensitivity b of the season (either wheat or maize) was determined by averaging all the estimated short-term b in each of the 4 d windows with the inverse of the standard error as a weighing factor. The long-term temperature sensitivity b was then used to estimate the ERref parameter in each of the 4 d windows by fitting Eq. (1). Following this, ERref of each day was estimated by using the least-squares spline approximation (Lei and Yang, 2010).
To quantify the contribution of source areas to the CO2 flux measurement of the eddy covariance, we used an analytical footprint model (Hsieh et al., 2000),
where D=0.28 and P=0.59 are similarity constants for unstable condition (Hsieh et al., 2000), κ=0.4 is von Karman constant, χ represents the horizontal coordinate, L represents the Obukhov length, zm represents the measurement height, and zu represents the length scale expressed as follows:
where z0 represents the roughness height set to be 0.1 Hc (canopy height).
Note that the eddy covariance system failed from 23 October 2010 to 1 April 2011 during the wheat dormant season. To evaluate the seasonal CO2 budget of this rotation cycle, the flux gap of this period was filled by using the machine learning Support Vector Regression (SVR) algorithm (Cristianini and Shave-Taylor, 2000), which has been proved to be an appropriate tool for flux gap filling (e.g., Kang et al., 2019; Kim et al., 2019) (see Appendix A).
2.3 Meteorological and environmental condition measurements
The meteorological variables were measured at 30 min intervals by a standard meteorological station on the tower. Among these variables were the air temperature (Ta) and relative humidity (RH) (HMP45C, Vaisala Inc, Helsinki, Finland) at a height of 1.6 m and precipitation (P) (TE525MM, Campbell Scientific Inc), incoming shortwave radiation (Rsi) (CRN1, Kipp & Zonen, Delft, Netherlands), and photosynthetic photon flux density (PPFD) (LI-190SA, LI-COR Inc) at a height of 3.7 m. The 30 min interval edaphic measurements included soil temperature (TS) (109-L, Campbell Scientific Inc.) and volumetric soil moisture (θ) (CS616-L, Campbell Scientific Inc.) for the top 5 cm of the soil; soil matric potential (ψ) (257-L, Campbell Scientific Inc.) has been measured since 2010 at the same depth. The groundwater depth (WD) (CS420-L, Campbell Scientific Inc.) was measured at a location close to flux tower in 30 min intervals.
2.4 Biometric measurements and crop samples
To trace crop development and carbon storage, we measured canopy height (Hc), leaf area index (LAI), crop dry matter (DM) and carbon content of crop organs at an interval of 7–10 d in the footprint of eddy covariance. Due to inclement weather, measurement intervals were occasionally extended to 2 weeks or longer. The Hc was measured with a ruler, and LAI was measured with LAI-2000 (LI-COR Inc.) at 10 locations randomly distributed in the field. For crop samples, four locations were randomly selected at the start of the growing season, and crop samples were then collected close to these four locations throughout the experimental period. At each location, 10 crop samples were collected for wheat and 3 crop samples were collected from maize. To reduce the sample uncertainty at harvest, 200 crops and 5 crops were collected in each location for wheat and maize, respectively. The crop organs were separated and oven-dried at 105 ∘C for kill-enzyme torrefaction for 30 min and then oven-dried at 75 ∘C until a constant weight. The crop samples were used to estimate the average field biomass (Dry Matter). The carbon content was analyzed using the combustion–oxidation–titration method (National Standards of Environmental Protection of the People's Republic of China, 2013) to estimate carbon storage. The crop samples provided a direct estimate of the NPP.
2.5 Soil respiration measurements
Soil respiration was measured every day in the footprint of the eddy covariance between 13:00 and 15:00 UTC+8 from March to September 2011 using a portable soil respiration system LI-8100 (LI-COR Inc.). Below-ground autotrophic respiration and heterotrophic respiration were differentiated using the root exclusion method (Zhang et al., 2013). The total soil respiration (RS) and RH were measured at treatments with and without roots, respectively, and the corresponding difference is RAB. To reduce the uncertainty associated with spatial variability, we set three replicate pairs of comparative treatments (i.e., with root and without root) randomly in the field. The uniform field condition contributes to reducing the measurement uncertainty associated with the spatial variability (see Zhang et al., 2013). To assess the seasonal variations and total amount of soil respirations, the seasonal continuous RH was constructed using the Q10 model by incorporating soil moisture as follows (Zhang et al., 2013):
where θf is the field capacity. The parameters were inferred by fitting the RH and TS measurements by using the least-squares method (see Zhang et al., 2013), where A=1.16 µmol m−2 s−1, B=0.0503 ∘C−1 and (unitless) (see Zhang et al., 2013). Note that the plant biomass was negligible before 14 March, during which RH was set to equal to the ecosystem respiration and the RAB was assumed to be 0. RAB of other periods was estimated based on the RH measurement and the ratio of RAB to RS estimated previously (Zhang et al., 2013), and the continuous RAB∕RS ratio was interpolated from the daily records (Fig. 2). This estimation method is robust because the RAB∕RS ratio is nearly constant around its diurnal average (Zhang et al., 2015b).
2.6 Synthesis of the CO2 budget components
The CO2 budget components were derived by combining the eddy covariance measurements, soil respiration experiments and crop samples. Eddy-covariance-measured NEE is the difference between carbon assimilation (i.e., GPP) and carbon release (i.e., ER). The ER consists of RH, RAB (i.e., root respiration) and above-ground autotrophic respiration (RAA). The total soil respiration is the sum of RH and RAB,
The total autotrophic respiration (RA) is the difference between the eddy-covariance-derived ER and RH,
The above-ground autotrophic respiration (RAA) is the difference between the eddy-covariance-derived ER and RS in Eq. (6),
NPP is plant biomass carbon storage and can be quantified as the difference between GPP and RA,
where the subscript “EC” represents that the NPP is estimated from the eddy-covariance-derived GPP. In parallel, NPP can also be directly inferred from biomass samples as follows:
where the subscript “CS” indicates that NPP is based on crop samples and Ccro is the plant biomass carbon storage at harvest. We used the average of the two independent NPPs as the measurement for this site.
NEP is commonly estimated by the NEE measurement (NEPEC = −NEE). In this study, the crop samples and soil respiration measurements also provided an independent estimate as follows:
We used the average of the two NEPs as the measurement for this site.
At this site, there were no fire and insect disturbances and no manure fertilizer application. The carbon input from seeds was negligible, and all crop residues were returned to the field. Thus, NBP can be quantified as the difference between NEP and grain export carbon loss (Cgra),
3.1 Meteorological conditions and crop development
The interannual variations in major meteorological variables are shown in Fig. 3, and they showed no clear trend for both wheat and maize seasons. For the full 2010–2011 cycle with comprehensive experiments, the average Rsi and Ta were very close to other years; however, the P during maize season was a little higher than other years (Fig. 3c), leading to a shallow WD in the maize season (Fig. 3d). The intra-annual variations in field microclimates for the full 2010–2011 cycle are shown in Fig. 4. The seasonal maximum and minimum Ta occurred in July and January, respectively, and the variations in vapor pressure deficit (VPD) followed the Ta well. The WD mainly followed the irrigation events in winter and spring but followed P in summer and autumn. In particular, the WD varied from 0 to 3 m throughout the year. The wet soil conditions prohibited the field from experiencing water stress (Fig. 4d) because even the lowest soil matric potential (−187.6 kPa) remained a lot higher than the permanent wilting point of crops (around −1500.0 kPa).
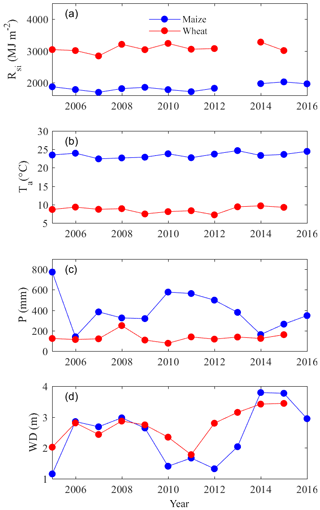
Figure 3The seasonal (a) total incoming shortwave radiation (Rsi), (b) average air temperature (Ta), (c) total precipitation (P) and (d) average groundwater depth (D) for both wheat and maize evaluated for the period from 2005 to 2016. Note that incoming shortwave radiation in the 2013 season is missing due to equipment malfunction.
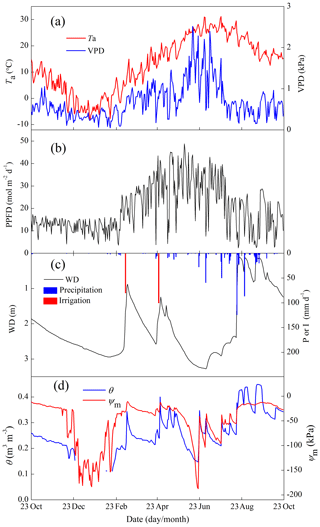
Figure 4Seasonal variations in the environmental variables of (a) air temperature (Ta) and vapor pressure deficit (VPD); (b) photosynthetic photon flux density (PPFD); (c) precipitation (P), irrigation (I), and groundwater depth (WD); and (d) volumetric soil moisture (θ) and soil matric potential (ψm).
Figure 5 shows the seasonal variations in Hc and LAI, reflecting the crop development for the full 2010–2011 cycle. The maximum LAI was 4.2 m2 m−2 for wheat and 3.6 m2 m−2 for maize. The variations in Hc and LAI distinguished the different stages of crop development. During the wheat season, the stages of regreening, jointing, booting, heading and maturity started approximately on 1 March, 20 April, 1 May, 7 May and 5 June, respectively. The seasonal variations in DM agreed well with the crop stages (Fig. 6), and the wheat biomass mainly accumulated in April and May, while maize biomass mainly accumulated in July and August. The total DM was 1718 g m−2 for wheat and 1262 g m−2 for maize at harvest. Upon harvest, the wheat DM was distributed as 3 % root, 43 % stem, 9 % leaf and 45 % grain, while the maize DM was distributed as 2 % root, 29 % stem, 7 % green leaf, 5 % dead leaf, 4 % bracket, 7 % cob and 46 % grain. The seasonal average carbon contents of the root, stem, green leaf, dead leaf, and grain were 410, 439, 486, 452, and 457 gC kg−1 DM for wheat and 408, 438, 477, 457, and 456 gC kg−1 DM for maize (see Table 1 for the seasonal variation).
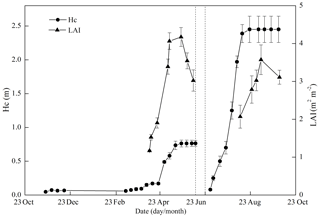
Figure 5Seasonal variations in canopy height (Hc) and leaf area index (LAI). The error bars denote 1 standard deviation of the 10 points.
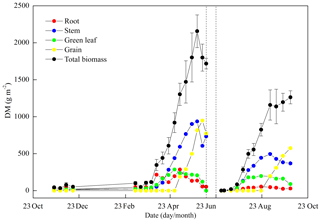
Figure 6Seasonal variations in the total dry biomass (DM) and its major components of root, stem, green leaf and grain. The error bars of total biomass denote 1 standard deviation of the four sample points.
3.2 The interannual variations in the NEE, GPP and ER
For the period from 2005 to 2016, if grain export was not considered, wheat was a consistent CO2 sink, as the seasonal total NEEs were consistently negative, and maize was a CO2 sink in most years, except for 2012 and 2013 when NEE was positive (Fig. 7a). NEEs of both wheat and maize fields became less negative during the past decade (though not in a statistically significant way), implying a progressive decline of the carbon sequestration potential of this cropland. The GPPs of both wheat and maize showed an increasing trend, though they were not statistically significant (Fig. 7b). The ERs of both wheat and maize also showed an increasing trend in these years, but only the trend of maize was significant (Fig. 7c). The decadal average of NEE, GPP, and ER were −364 (SD ± 98), 1174 (SD ± 189), and 810 (SD ± 161) gC m−2 for wheat and −136 (SD ± 168), 1008 (SD ± 297), and 872 (SD ± 284) gC m−2 for maize.
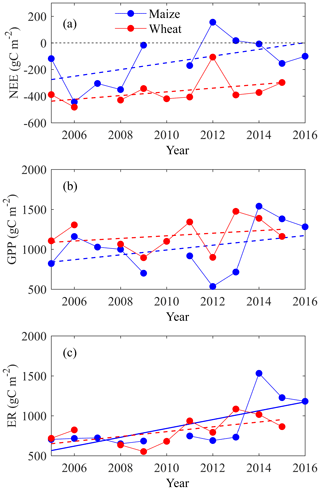
Figure 7The temporal trend of annual (a) net ecosystem exchange (NEE), (b) gross primary productivity (GPP) and (c) ecosystem respiration (ER) for both wheat and maize from 2005 to 2016. Note that though most gaps of carbon fluxes were filled, the wheat of 2007 was excluded as it had a large gap accounting for 26 % of annual records that we were unable to fill. Maize was not planted in the growing season of 2010. Note that the solid line shows where the temporal trend passes F test at p<0.05 significance level, while the dashed line shows where the temporal trend does not pass the F test at p<0.05 level.
The NEE, GPP and ER for both wheat and maize were correlated with the three main environmental variables of Rsi, Ta and WD using the multiple regression (see Appendix B for details). In the wheat season, Ta showed its relatively great importance (compared to Rsi and WD) to all three of the CO2 fluxes with a higher Ta increasing both GPP and ER and also enhancing NEE (more negative) (Fig. 8a). WD correlated negatively with GPP, thereby reducing net carbon uptake (less negative NEE). WD exhibited almost no effect on ER. Rsi exhibited almost no effect on all three CO2 fluxes. Therefore, Ta explained most of the interannual variations in NEE, GPP and ER, followed by WD. In the maize season, WD had good correlations with all three fluxes of GPP, ER and NEE, where a deeper WD contributed to lower both GPP and ER and also drove higher net carbon uptake (more negative NEE). Ta showed almost no effect on all three CO2 fluxes. Rsi had a positive correlation with ER but almost no correlation with GPP (Fig. 8b). Ultimately, higher Rsi in the maize season lowered the net carbon uptake (more positive NEE). Overall, Rsi and WD showed their great importance in influencing the interannual variation in maize NEE, with Rsi having a positive correlation and WD having a comparable negative correlation (Fig. 8b).
3.3 Intra-annual variations in the NEE, GPP and ER
The intra-annual variations in NEE, GPP and ER exhibited a bimodal curve corresponding with the two crop seasons (Fig. 9). All three CO2 fluxes were almost in phase, with peaks appearing at the start of May during the wheat season and in the middle of August during the maize season. During some of the winter season, the field still sequestered a small amount of CO2 because of the weak photosynthesis, which was confirmed by leaf level gas exchange measurement (data not shown). Net carbon emission happened during the fallow periods, in addition to the start of the maize season when the plant was small and high temperatures enhanced heterotrophic respiration. During the wheat season, two evident spikes appeared on 21 April and 8 May with positive NEE values (i.e., net carbon release). These spikes resulted from the radiation decline during the inclement weather (Fig. 4b), which suppressed the photosynthesis rate; similar phenomena also appeared during the maize season.
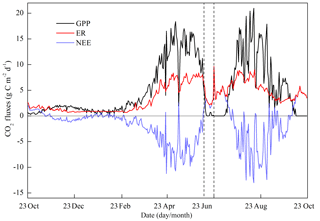
Figure 9Seasonal variations in gross primary productivity (GPP), net ecosystem exchange (NEE) and ecosystem respiration (ER) (those before 2 April were calculated with the SVR method).
Figure 10 shows the variations in ER and its components. During the wheat season, the variation in ER closely followed crop development and temperature, but there were two evident declines at the end of April and the start of May due to low temperatures associated with the inclement weather. During the early growing stage of maize, RH was the main component of ER. When waterlogging conditions occurred in late August and early September, both RH and RAB were suppressed to zero.
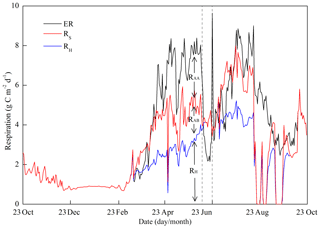
Figure 10Seasonal variations in the components of ecosystem respiration (ER), total soil respiration (RS) and soil heterotrophic respiration (RH). The difference between ER and RS denotes above-ground autotrophic respiration (RAA), and the difference between RS and RH denotes below-ground autotrophic respiration (RAB).
3.4 CO2 budget synthesis in the 2010–2011 agricultural cycle
CO2 budget analysis showed that this wheat–maize rotation cropland has the potential to uptake carbon from the atmosphere (Fig. 11). In the full 2010–2011 cycle, the total NEE, GPP, and ER values were −438, 1078, and 640 gC m−2 for wheat and −239, 780, and 541 gC m−2 for maize. The NPP values were 750 and 815 gC m−2 for wheat based on crop samples and the eddy covariance and that complemented with soil respiration measurements, respectively, and were 592 and 532 gC m−2 for maize based on the two methods. We used the average of these two methods for NPP measurements, which were 783 (SD ± 46) gC m−2 for wheat and 562 (SD ± 43) gC m−2 for maize. We also used the average of NEP from the two independent methods for the measurement, and the NEP was 406 gC m−2 for wheat and 269 gC m−2 for maize. Furthermore, when considering the carbon loss associated with the grain export, the NBP values were 59 gC m−2 for wheat and 5 gC m−2 for maize, respectively. Considering the net CO2 loss of −104 gC m−2 during the two fallow periods, NBP of the whole wheat–maize crop cycle was −40 gC m−2 yr−1, suggesting that the cropland was a weak carbon source to the atmosphere under these specific climatic conditions and field management practices.
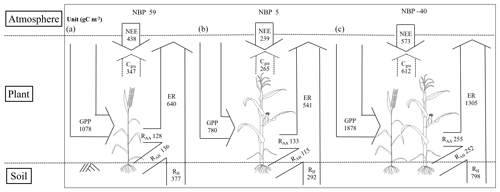
Figure 11Carbon budget of wheat (a), maize (b) and the full wheat–maize rotation cycle, with fallow periods included (c). Note that the absolute value of NEE is shown here; NBPs of wheat and maize are the average of two independent methods (i.e, an eddy covariance-based method and a crop-sample-based method).
This study investigated the decadal variations in the NEE, GPP and ER for an irrigated wheat–maize rotation cropland over the North China Plain, and the results exhibited a decreasing trend of the CO2 sink capacity during the past decade. The interannual variations in the carbon fluxes of wheat showed close dependence on temperature and groundwater depth, while those of maize were mostly regulated by solar radiation and groundwater depth. Furthermore, the detailed CO2 budget components were quantified for a full wheat–maize agricultural cycle. Investigating the decadal trend of the CO2 fluxes and quantifying the detailed CO2 budget components for this representative cropland will provide useful knowledge for regional greenhouse gas emission evaluation over the North China Plain.
4.1 Comparison with other croplands
The cropland has been reported as carbon neutral to the atmosphere (e.g., Ciais et al., 2010), as a carbon source (e.g., Anthoni et al., 2004a; Verma et al., 2005; Kutsch et al., 2010; Wang et al., 2015; Eichelmann et al., 2016) and also as a carbon sink (e.g., Kutsch et al., 2010). Such inconsistency probably results from the different crop types and management practices (residue removal, the use of organic manure, etc.), in addition to variations in the climatic conditions (Béziat et al., 2009; Smith et al., 2014) and fallow period length (Dold et al., 2017). Our results show that the fully irrigated wheat–maize rotation cropland with a shallow groundwater depth was a weak CO2 sink during both the wheat and maize seasons in the full 2010–2011 cycle, but the CO2 loss during the fallow period reversed the cropland from a sink into a weak source with an NBP of −40 gC m−2 yr−1. These results are consistent with previous studies that reported the wheat–maize rotation cropland as a carbon source (Li et al., 2006; Wang et al., 2015). However, the net CO2 loss was much lower at our site, most likely due to the shallow groundwater depth.
Field measurements of the long-term CO2 fluxes over croplands remain lacking, and we found the carbon sequestration capacity of this cropland has been progressively decreasing, though it was not statistically significant. The cropland has been widely suggested as a climate change mitigation tool (e.g., Lal, 2001), but the potential in the future is challenging. However, since cropland management greatly impacts the carbon balance of cropland (Béziat et al., 2009; Ceschia et al., 2010), it remains required investigating if the management adjustment can foster the cropland carbon sink capacity over the long term.
The annual total NPP of 1345 gC m−2 yr−1 at our site is approximately twice the average of the model-estimated NPP for Chinese croplands (714 gC m−2 yr−1) with a rotation index of 2 (i.e., two crop cycles within 1 year) (Huang et al., 2007), more than 3 times the value estimated by MODIS (400 gC m−2 yr−1) (Zhao et al., 2005) and slightly higher than the value of the same crop rotation at the Luancheng site (1144 gC m−2 yr−1) (Wang et al., 2015). The higher NPP at our site may partially result from the sufficient irrigation and fertilization (Huang et al., 2007; Smith et al., 2014).
The contrasting respiration partitioning of the same crop in different regions (Table 2) indicate that the respiration processes may also be subject to climatic conditions and management practices. Though the ratio of ecosystem respiration to GPP at our site is comparable to other studies, the ratio of autotrophic respiration to GPP is much lower at our site, and while the ratio of heterotrophic respiration to ecosystem respiration is greater at our site, these findings are different from those at the other sites with similar crop variety (Moureaux et al., 2008; Aubinet et al., 2009; Suleau et al., 2011; Wang et al., 2015; Demyan et al., 2016), as they showed that ecosystem respiration is usually dominated by below-ground and above-ground autotrophic respirations. The higher soil heterotrophic respiration at our site probably results from the full irrigation and shallow groundwater, which both alleviate soil water stress.
Table 2Various ratios associated with carbon fluxes in croplands.
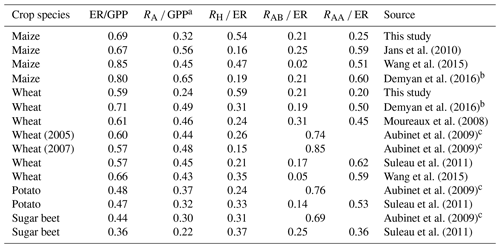
a The values in parentheses indicate that the value is calculated by the equation RA ∕ GPP = 1 − NPP∕GPP. b The data were from 2012, and the estimation is based on the average of the static and dynamic methods. c RA and RH are the averaged values of the two corresponding methods.
4.2 The effects of groundwater on carbon fluxes
The groundwater table at our site is much closer to the surface because of the irrigation by water diverted from the Yellow River. In contrast, the nearby Luancheng site (Wang et al., 2015) is groundwater-fed with a very deep groundwater depth (approximately 42 m) (Shen et al., 2013), and their CO2 budget components had some differences with our study. Comparing the net CO2 exchange of wheat, the GPP at our site is a little higher than the Luancheng site, implying the irrigation at our site may better sustain the photosynthesis rate for wheat; ER at our site is also a little higher than the Luancheng site. For maize, both sites are not irrigated due to the high summer precipitation. GPP and ER at our site were comparable to Luancheng site, implying that the irrigation method prior to the maize season had no discernible effect on the integrated CO2 fluxes for maize. However, the three components of ER in our study showed pronounced differences from the Luancheng site, where they reported the RAA was 411 gC m−2 for wheat and 428 gC m−2 for maize, 3 times the results of our study (128 gC m−2 for wheat and 133 gC m−2 for maize). However, their RAB for wheat (36 gC m−2) and maize (16 gC m−2) were less than a quarter of our results (136 gC m−2 for wheat and 115 gC m−2 for maize). Their RH of wheat (245 gC m−2) was less than our estimate (377 gC m−2), but RH of maize (397 gC m−2) was greater than our result (292 gC m−2). In general, the above-ground crop parts in our site respired more carbon than the Luancheng site, possibly because the shallow groundwater depth at our site increased the above-ground biomass allocation but lowered the root biomass allocation (Poorter et al., 2012). These independent cross-site comparisons demonstrate that carbon budget components may be subject to the specific groundwater depth influenced by the irrigation type, and even the same crop under similar climatic conditions can behave differently in carbon consumption.
Our site experienced a short period of waterlogging during the 2010–2011 cycle due to the combined effects of full irrigation and the high precipitation during the summer. This distinct field condition reduced soil carbon losses in the maize season, potentially maintaining the CO2 captured by the cropland. Waterlogging events were occasionally reported in upland croplands. For example, Terazawa et al. (1992) and Iwasaki et al. (2010) suggested that waterlogging causes damage to plants, resulting in a decline in GPP as reported by Dold et al. (2017) and our study. Our study further shows that waterlogging reduces ER to a greater degree than GPP, possibly because of the low soil oxygen conditions, and thereby reduces the overall cropland CO2 loss. However, the CH4 released over the short term may be pronounced in waterlogged soils. As CH4 emission in this kind of cropping system over the North China Plain cropland remains lacking, additional field experiments are required to understand how irrigation and water saturation field condition impact the overall carbon budget.
4.3 Uncertainty in the estimation and limitation of this study
In the comprehensive experiment period for the full 2010–2011 agricultural cycle, the NEE of the wheat season from 23 October 2010 to 1 April 2011 was calculated using a calibrated SVR model. The SVR model performs well for predicting GPP and ER with very high R2 of 0.95 and 0.97 and an acceptable uncertainty level of 22.9 % and 15.2 % for GPP and ER, respectively. Hence, these estimates should have a negligible effect on the seasonal total carbon evaluation. The footprint analysis showed that 90 % of the measured eddy flux comes from the nearest 420 and 166 m in wheat and maize crops under unstable conditions, respectively, confirming that both soil respiration experiments and crop samples paired well with the EC measurements .
Root biomass was difficult to measure, but the uncertainty should be low because the root ratio (the ratio of the root weight to the total biomass weight) accounts for 15 %–16 % of the crop for wheat and maize (Wolf et al., 2015), and our measurements are very close to these values; i.e., the averaged seasonal root ratio was 15 % for wheat and 10 % for maize at our site. However, the relatively low root ratios (3 % for wheat and 2 % for maize) at harvest probably result from the root decay associated with plant senescence. The estimates of annual soil respiration are based on the Q10 model validated by the field measurements that may generate some uncertainty in the soil respiration budget due to the hysteresis response of soil respiration to temperature (Phillips et al., 2011; Zhang et al., 2015a, 2018). However, the Q10 model remains robust in soil respiration estimations if it is well validated (Tian et al., 1999; Zhang et al., 2013; Latimer and Risk, 2016), allowing for confidence in the estimates.
During the wheat season, the cumulative curves of NPPEC and NPPCS were not perfectly consistent in the main growing season, as clear differences emerged during the dormant season of wheat from 15 December 2010 to 8 March 2011 (Fig. 12). These differences may result from the small wheat sample number. However, the sample number at harvest was sufficiently big, and no discernible difference was found between the two NPPs at harvest. These two independent estimates of NPP were similar throughout the maize season (Fig. 12).
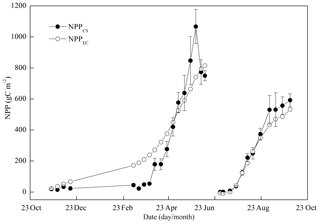
Figure 12Seasonal variations in the cumulative net primary productivity (NPP) with two independent methods of crop sampling (NPPCS) and eddy covariance (NPPEC) complemented with soil respiration measurements.
This study provides a comprehensive quantification of the CO2 budget components of the cropland, but it remains limited to a relatively wet year (see Fig. 3c and d). The integrated carbon fluxes (NEE, GPP and ER) have pronounced interannual variations, also suggesting further investigations are required on the interannual variations in the carbon budget components.
Based on the decadal measurements of CO2 fluxes over an irrigated wheat–maize rotation cropland over the North China Plain, we found the cropland was a strong CO2 sink if grain export was not considered. When considering the grain export, the cropland was a weak CO2 source, with an NBP of −40 gC m−2 yr−1 in the full 2010–2011 agricultural cycle. The net CO2 exchange during the past decade from 2005 to 2016 showed a statistically nonsignificant decreasing trend, implying a decreasing carbon sequestration capacity of this cropland, discouraging the potential of taking agroecosystems as the mitigation tool of climate change. In the wheat season, air temperature showed the best correlation with the CO2 fluxes followed by the groundwater depth, whereas in the maize season both shortwave radiation and groundwater depth showed good correlation with the CO2 fluxes. The comprehensive investigation showed most of the carbon sequestration occurred during the wheat season, while maize was close to being CO2 neutral. Soil heterotrophic respiration in this cropland contributes substantially to CO2 loss in both the wheat and maize seasons. This study provides detailed knowledge for estimating regional carbon emissions over the North China Plain.
A1 Support Vector Regression method
The Support Vector Regression (SVR) method is a machine learning technique-based regression, which transforms regression from nonlinear into linear by mapping the original low-dimensional input space to higher-dimensional space (Cristianini and Shave-Taylor, 2000). The SVR method has two advantages: (1) the model training always converges to global optimal solution, with only a few free parameters to adjust, and no experimentation is needed to determine the architecture of SVR. (2) The SVR method is robust to small errors in the training data (Ueyama et al., 2013). The Support Vector Machine (SVM) software package obtained from LIBSVM (Chang and Lin, 2005) is used in this study.
A2 Data processing and selection of explanatory variables
Gross primary productivity (GPP) is influenced by several edaphic, atmospheric and physiological variables, among which air temperature (Ta), relative humidity (RH), leaf area index (LAI), net photosynthetically active radiation (PAR) and soil moisture (θ) are the dominant factors. Hence, we select Ta, RH, LAI, PAR and θ as explanatory variables of GPP. Ecosystem respiration (ER) consists of total soil respiration and above-ground autotrophic respiration. The total soil respiration is largely influenced by soil temperature and soil moisture, while above-ground autotrophic respiration is largely influenced by air temperature and above-ground biomass. Therefore, we select Ta, soil temperature at 5 cm (TS), θ and LAI as explanatory variables of ER. LAI is estimated from the Wide Dynamic Range Vegetation Index derived from the MOD09Q1 reflectance data (250 m, 8 d average, https://ladsweb.modaps.eosdis.nasa.gov/missions-and-measurements/products/MOD09Q1/, last access: 15 May 2016; see Lei et al., 2013).
The three wheat seasons of 2005–2006, 2009–2010 and 2010–2011 are selected for model training, and the original half-hourly measurements of GPP and ER, together with the explanatory variables, are averaged to the daily scale, but we remove days missing more than 25 % of half-hourly data. We have GPP available from 466 d and ER from 483 d for model training. The explanatory variables for the equipment failure are also averaged into daily scale, which will be used to calculate GPP and ER with the trained model described in the following section.
A3 SVR model training and flux calculation
In order to eliminate the impact of variables with different absolute magnitudes, we rescale all the variables in the training data set to the [0, 1] range prior to SVR model training. In the training process, the radial basis function (RBF, a kernel function of SVR) is used and the width of insensitive error band is set as 0.01. The SVR model training follows these steps:
-
All training data samples are randomly divided into five nonoverlapping subsets, and four of them are selected as the training sets (also calibration set); the remaining subset is treated as the test set (or validation set). This process is repeated five times to ensure that every subset has a chance to be the test set.
-
For the selected training set, the SVR parameters (cost of errors c and kernel parameter σ) are determined using a grid search with a five-fold cross-validation training process. In this approach, the training set is further randomly divided into five nonoverlapping subsets. Training is performed on each of the four subsets within this training set, with the remaining subset reserved for calculating the root-mean-square error (RMSE), and model parameters (c and σ) yielding the minimum RMSE value are selected.
-
The SVR model is trained based on the training set from step (1) and initialized by the parameters (c and σ) derived from step (2).
-
The test set from the step (1) is used to evaluate the model obtained from the step (3) by using the coefficient of determination (R2) and RMSE.
-
The model is trained with all of the available samples that achieved good performance, as R2 are 0.95 and 0.97 for GPP and ER, respectively, and the mean RMSE is 1.28 and 0.44 gC m−2 d−1. The RMSE can be further used as a metric quantifying uncertainty, which accounts for 22.9 % and 15.2 % for the averaged GPP and ER, respectively. GPP and ER during the equipment failure period are then calculated with the trained model complemented with the observed explanatory variables, and NEE is derived as the difference of GPP and ER.
The flux of NEE, GPP or ER is correlated with incoming shortwave radiation (Rsi), air temperature (Ta) and groundwater depth (WD) as flux = , where flux is NEE, GPP or ER; a, b, c and d are regression parameters. All the variables are normalized to derive their z score before the regression, where the z score is calculated by subtracting the mean from the data and dividing the result by the standard deviation. The coefficient of each variable represents the relative importance of the corresponding variable in contributing to the dependent variable.
The data of this study are available to the public by request to the corresponding author (Huimin Lei).
QZ and HL designed the study and methodology with substantial input from all co-authors. QZ conducted the field experiment. BF conducted the SVR calculation for gap filling. All authors contributed to interpretation of the results. QZ drafted the manuscript, and all authors edited and approved the final manuscript.
The authors declare that they have no conflict of interest.
We thank editor Paul C. Stoy and reviewers Russell Scott and Seth Spawn for their constructive comments, which greatly improved this work. We also would like to thank two additional anonymous reviewers of the initial submission; we could not have achieved this without their constructive criticism. The financial support from the NSFC–NSF collaboration funding and NSFC are greatly appreciated.
This research has been supported by NSFC–NSF collaboration funding (P. R. China–U.S) (grant no. 51861125102) and the National Natural Science Foundation of China (project nos. 51509187, 51679120 and 51525902).
This paper was edited by Paul Stoy and reviewed by Russell Scott and Seth Spawn.
Anthoni, P. M., Freibauer, A., Kolle, O., and Schulze, E. D.: Winter wheat carbon exchange in Thuringia, Germany, Agr. Forest Meteorol., 121, 55–67, https://doi.org/10.1016/s0168-1923(03)00162-x, 2004a.
Anthoni, P. M., Knohl, A., Rebmann, C., Freibauer, A., Mund, M., Ziegler, W., Kolle, O., and Schulze, E. D.: Forest and agricultural land-use-dependent CO2 exchange in Thuringia, Germany, Glob. Change Biol., 10, 2005–2019, https://doi.org/10.1111/j.1365-2486.2004.00863.x, 2004b.
Aubinet, M., Grelle, A., Ibrom, A., Rannik, Ü., Moncrieff, J., Foken, T., Kowalski, A. S., Martin, P. H., Berbigier, P., Bernhofer, C., Clement, R., Elbers, J., Granier, A., Grunwald, T., Morgenstern, K., Pilegaard, K., Rebmann, C., Snijders, W., Valentini, R., and Vesala, T.: Estimates of the annual net carbon and water exchange of forests: The EUROFLUX methodology, Adv. Ecol. Res., 30, 113–175, 2000.
Aubinet, M., Moureaux, C., Bodson, B., Dufranne, D., Heinesch, B., Suleau, M., Vancutsem, F., and Vilret, A.: Carbon sequestration by a crop over a 4-year sugar beet/winter wheat/seed potato/winter wheat rotation cycle, Agr. Forest Meteorol., 149, 407–418, https://doi.org/10.1016/j.agrformet.2008.09.003, 2009.
Baker, J. M. and Griffis, T. J.: Examining strategies to improve the carbon balance of corn/soybean agriculture using eddy covariance and mass balance techniques, Agr. Forest Meteorol., 128, 163–177, https://doi.org/10.1016/j.agrformet.2004.11.005, 2005.
Baldocchi, D., Falge, E., Gu, L. H., Olson, R., Hollinger, D., Running, S., Anthoni, P., Bernhofer, C., Davis, K., Evans, R., Fuentes, J., Goldstein, A., Katul, G., Law, B., Lee, X. H., Malhi, Y., Meyers, T., Munger, W., Oechel, W., U, K. T. P., Pilegaard, K., Schmid, H. P., Valentini, R., Verma, S., Vesala, T., Wilson, K., and Wofsy, S.: FLUXNET: A new tool to study the temporal and spatial variability of ecosystem-scale carbon dioxide, water vapor, and energy flux densities, B. Am. Meteorol. Soc., 82, 2415–2434, 2001.
Béziat, P., Ceschia, E., and Dedieu, G.: Carbon balance of a three crop succession over two cropland sites in South West France, Agr. Forest Meteorol., 149, 1628–1645, https://doi.org/10.1016/j.agrformet.2009.05.004, 2009.
Bondeau, A., Smith, P. C., Zaehle, S., Schaphoff, S., Lucht, W., Cramer, W., Gerten, D., Lotze-Campen, H., Muller, C., Reichstein, M., and Smith, B.: Modelling the role of agriculture for the 20th century global terrestrial carbon balance, Glob. Change Biol., 13, 679–706, https://doi.org/10.1111/j.1365-2486.2006.01305.x, 2007.
Cao, G., Scanlon, B. R., Han, D., and Zheng, C.: Impacts of thickening unsaturated zone on groundwater recharge in the North China Plain, J. Hydrol., 537, 260–270, https://doi.org/10.1016/j.jhydrol.2016.03.049, 2016.
Ceschia, E., Béziat, P., Dejoux, J. F., Aubinet, M., Bernhofer, C., Bodson, B., Buchmann, N., Carrara, A., Cellier, P., Di Tommasi, P., Elbers, J. A., Eugster, W., Grunwald, T., Jacobs, C. M. J., Jans, W. W. P., Jones, M., Kutsch, W., Lanigan, G., Magliulo, E., Marloie, O., Moors, E. J., Moureaux, C., Olioso, A., Osborne, B., Sanz, M. J., Saunders, M., Smith, P., Soegaard, H., and Wattenbach, M.: Management effects on net ecosystem carbon and GHG budgets at European crop sites, Agr. Ecosyst. Environ., 139, 363–383, https://doi.org/10.1016/j.agee.2010.09.020, 2010.
Chang, C. C. and Lin, C. J.: LIBSVM-A library for Support Vector Machines, available at: http://www.csie.ntu.edu.tw/~cjlin/libsvm/ (last access: 15 March 2016), 2005.
Ciais, P., Wattenbach, M., Vuichard, N., Smith, P., Piao, S. L., Don, A., Luyssaert, S., Janssens, I. A., Bondeau, A., Dechow, R., Leip, A., Smith, P. C., Beer, C., van der Werf, G. R., Gervois, S., Van Oost, K., Tomelleri, E., Freibauer, A., Schulze, E. D., and Team, C. S.: The European carbon balance. Part 2: croplands, Glob. Change Biol., 16, 1409–1428, https://doi.org/10.1111/j.1365-2486.2009.02055.x, 2010.
Ciais, P., Gervois, S., Vuichard, N., Piao, S. L., and Viovy, N.: Effects of land use change and management on the European cropland carbon balance, Glob. Change Biol., 17, 320–338, https://doi.org/10.1111/j.1365-2486.2010.02341.x, 2011.
Cristianini, N. and Shawe-Taylor, J.: An Introduction to SupportVector Machines and Other Kernel-Based Learning Methods, Cambridge Univ. Press, Cambridge, UK, 189 pp., 2000.
Demyan, M. S., Ingwersen, J., Funkuin, Y. N., Ali, R. S., Mirzaeitalarposhti, R., Rasche, F., Poll, C., Muller, T., Streck, T., Kandeler, E., and Cadisch, G.: Partitioning of ecosystem respiration in winter wheat and silage maize-modeling seasonal temperature effects, Agr. Ecosyst. Environ., 224, 131–144, https://doi.org/10.1016/j.agee.2016.03.039, 2016.
de la Motte, L. G., Jérôme, E., Mamadou, O., Beckers, Y., Bodson, B., Heinesch, B., and Aubinet, M.: Carbon balance of an intensively grazed permanent grassland in southern Belgium, Agr. Forest Meteorol., 228–229, 370–383, https://doi.org/10.1016/j.agrformet.2016.06.009, 2016.
Dold, C., Büyükcangaz, H., Rondinelli, W., Prueger, J., Sauer, T., and Hatfield, J.: Long-term carbon uptake of agro-ecosystems in the Midwest, Agr. Forest Meteorol., 232, 128–140, https://doi.org/10.1016/j.agrformet.2016.07.012, 2017.
Drewniak, B. A., Mishra, U., Song, J., Prell, J., and Kotamarthi, V. R.: Modeling the impact of agricultural land use and management on US carbon budgets, Biogeosciences, 12, 2119–2129, https://doi.org/10.5194/bg-12-2119-2015, 2015.
Eichelmann, E., Wagner-Riddle, C., Warland, J., Deen, B., and Voroney, P.: Comparison of carbon budget, evapotranspiration, and albedo effect between the biofuel crops switchgrass and corn, Agr. Ecosyst. Environ., 231, 271–282, https://doi.org/10.1016/j.agee.2016.07.007, 2016.
Ekblad, A., Bostrom, B., Holm, A., and Comstedt, D.: Forest soil respiration rate and δ13C is regulated by recent above ground weather conditions, Oecologia, 143, 136–142, https://doi.org/10.1007/s00442-004-1776-z, 2005.
Eugster, W., Moffat, A. M., Ceschia, E., Aubinet, M., Ammann, C., Osborne, B., Davis, P. A., Smith, P., Jacobs, C., Moors, E., Le Dantec, V., Beziat, P., Saunders, M., Jans, W., Grunwald, T., Rebmann, C., Kutsch, W. L., Czerny, R., Janous, D., Moureaux, C., Dufranne, D., Carrara, A., Magliulo, V., Di Tommasi, P., Olesen, J. E., Schelde, K., Olioso, A., Bernhofer, C., Cellier, P., Larmanou, E., Loubet, B., Wattenbach, M., Marloie, O., Sanz, M. J., Sogaard, H., and Buchmann, N.: Management effects on European cropland respiration, Agr. Ecosyst. Environ., 139, 346–362, https://doi.org/10.1016/j.agee.2010.09.001, 2010.
Falkowski, P., Scholes, R. J., Boyle, E. E. A., Canadell, J., Canfield, D., Elser, J., Gruber, N., Hibbard, K., Högberg, P., Linder, S., and Mackenzie, F. T.: The global carbon cycle: a test of our knowledge of earth as a system, Science, 290, 291–296, https://doi.org/10.1126/science.290.5490.291, 2000.
Falge, E., Baldocchi, D., Olson, R., Anthoni, P., Aubinet, M., Bernhofer, C., Burba, G., Ceulemans, R., Clement, R., Dolman, H., Granier, A., Gross, P., Grunwald, T., Hollinger, D., Jensen, N. O., Katul, G., Keronen, P., Kowalski, A., Lai, C. T., Law, B. E., Meyers, T., Moncrieff, H., Moors, E., Munger, J. W., Pilegaard, K., Rannik, U., Rebmann, C., Suyker, A., Tenhunen, J., Tu, K., Verma, S., Vesala, T., Wilson, K., and Wofsy, S.: Gap filling strategies for defensible annual sums of net ecosystem exchange, Agr. Forest Meteorol., 107, 43–69, https://doi.org/10.1016/S0168-1923(00)00225-2, 2001.
Falge, E., Baldocchi, D., Tenhunen, J., Aubinet, M., Bakwin, P., Berbigier, P., Bernhofer, C., Burba, G., Clement, R., Davis, K. J., Elbers, J. A., Goldstein, A. H., Grelle, A., Granier, A., Guomundsson, J., Hollinger, D., Kowalski, A. S., Katul, G., Law, B. E., Malhi, Y., Meyers, T., Monson, R. K., Munger, J. W., Oechel, W., Paw, K. T., Pilegaard, K., Rannik, U., Rebmann, C., Suyker, A., Valentini, R., Wilson, K., and Wofsy, S.: Seasonality of ecosystem respiration and gross primary production as derived from FLUXNET measurements, Agr. Forest Meteorol., 113, 53–74, https://doi.org/10.1016/S0168-1923(02)00102-8, 2002a.
Falge, E., Tenhunen, J., Baldocchi, D., Aubinet, M., Bakwin, P., Berbigier, P., Bernhofer, C., Bonnefond, J. M., Burba, G., Clement, R., Davis, K. J., Elbers, J. A., Falk, M., Goldstein, A. H., Grelle, A., Granier, A., Grunwald, T., Gudmundsson, J., Hollinger, D., Janssens, I. A., Keronen, P., Kowalski, A. S., Katul, G., Law, B. E., Malhi, Y., Meyers, T., Monson, R. K., Moors, E., Munger, J. W., Oechel, W., U, K. T. P., Pilegaard, K., Rannik, U., Rebmann, C., Suyker, A., Thorgeirsson, H., Tirone, G., Turnipseed, A., Wilson, K., and Wofsy, S.: Phase and amplitude of ecosystem carbon release and uptake potentials as derived from FLUXNET measurements, Agr. Forest Meteorol., 113, 75–95, https://doi.org/10.1016/S0168-1923(02)00103-X, 2002b.
Fargione, J. E., Bassett, S., Boucher, T., Bridgham, S. D., Conant, R. T., Cook-Patton, S. C., Ellis, P. W., Falcucci, A., Fourqurean, J. W., Gopalakrishna, T., Gu, H., Henderson, B., Hurteau, M. D., Kroeger, K. D., Kroeger, T., Lark, T. J., Leavitt, S. M., Lomax, G., McDonald, R. I., Megonigal, J. P., Miteva, D. A., Richardson, C. J., Sanderman, J., Shoch, D., Spawn, S. A., Veldman, J. W., Williams, C. A., Woodbury, P. B., Zganjar, C., Baranski, M., Elias, P., Houghton, R. A., Landis, E., McGlynn, E., Schlesinger, W. H., Siikamaki, J. V., Sutton-Grier, A. E., and Griscom, B. W.: Natural climate solutions for the United States, Sci. Adv., 4, https://doi.org/10.1126/sciadv.aat1869, 2018.
Fleisher, D. H., Timlin, D. J., and Reddy, V. R.: Elevated carbon dioxide and water stress effects on potato canopy gas exchange, water use, and productivity, Agr. Forest Meteorol., 148, 1109–1122, https://doi.org/10.1016/j.agrformet.2008.02.007, 2008.
Forkel, M., Carvalhais, N., Rödenbeck, C., Keeling, R., Heimann, M., Thonicke, K., Zaehle, S., and Reichstein, M.: Enhanced seasonal CO2 exchange caused by amplified plant productivity in northern ecosystems, Science, 351, 696–699, https://doi.org/10.1126/science.aac4971, 2016.
Freibauer, A., Rounsevell, M. D. A., Smith, P., and Verhagen, J.: Carbon sequestration in the agricultural soils of Europe, Geoderma, 122, 1–23, https://doi.org/10.1016/j.geoderma.2004.01.021, 2004.
Gao, X., Gu, F., Hao, W., Mei, X., Li, H., Gong, D., and Zhang, Z.: Carbon budget of a rainfed spring maize cropland with straw returning on the Loess Plateau, China, Sci. Total Environ., 586, 1193–1203, https://doi.org/10.1016/j.scitotenv.2017.02.113, 2017.
Gilmanov, T. G., Verma, S. B., Sims, P. L., Meyers, T. P., Bradford, J. A., Burba, G. G., and Suyker, A. E.: Gross primary production and light response parameters of four Southern Plains ecosystems estimated using long-term CO2-flux tower measurements, Global Biogeochem. Cy., 17, 1071, https://doi.org/10.1029/2002gb002023, 2003.
Grant, R. F., Arkebauer, T. J., Dobermann, A., Hubbard, K. G., Schimelfenig, T. T., Suyker, A. E., Verma, S. B., and Walters, D. T.: Net biome productivity of irrigated and rainfed maize-soybean rotations: Modeling vs. measurements, Agron. J., 99, 1404–1423, https://doi.org/10.2134/agronj2006.0308, 2007.
Gray, J. M., Frolking, S., Kort, E. A., Ray, D. K., Kucharik, C. J., Ramankutty, N., and Friedl, M. A.: Direct human influence on atmospheric CO2 seasonality from increased cropland productivity, Nature, 515, 398–401, https://doi.org/10.1038/nature13957, 2014.
Griscom, B. W., Adams, J., Ellis, P. W., Houghton, R. A., Lomax, G., Miteva, D. A., Schlesinger, W. H., Shoch, D., Siikamaki, J. V., Smith, P., Woodbury, P., Zganjar, C., Blackman, A., Campari, J., Conant, R. T., Delgado, C., Elias, P., Gopalakrishna, T., Hamsik, M. R., Herrero, M., Kiesecker, J., Landis, E., Laestadius, L., Leavitt, S. M., Minnemeyer, S., Polasky, S., Potapov, P., Putz, F. E., Sanderman, J., Silvius, M., Wollenberg, E., and Fargione, J.: Natural climate solutions, P. Natl. Acad. Sci. USA, 114, 11645–11650, https://doi.org/10.1073/pnas.1710465114, 2017.
Heimann, M. and Reichstein, M.: Terrestrial ecosystem carbon dynamics and climate feedbacks, Nature, 451, 289–292, https://doi.org/10.1038/Nature06591, 2008.
Hollinger, S. E., Bernacchi, C. J., and Meyers, T. P.: Carbon budget of mature no-till ecosystem in North Central Region of the United States, Agr. Forest Meteorol., 130, 59–69, https://doi.org/10.1016/j.agrformet.2005.01.005, 2005.
Hsieh, C. I., Katul, G., and Chi, T.: An approximate analytical model for footprint estimation of scaler fluxes in thermally stratified atmospheric flows, Adv. Water Resour., 23, 765–772, https://doi.org/10.1016/S0309-1708(99)00042-1, 2000.
Huang, Y., Zhang, W., Sun, W. J., and Zheng, X. H.: Net primary production of Chinese croplands from 1950 to 1999, Ecol. Appl., 17, 692–701, https://doi.org/10.1890/05-1792, 2007.
Hunt, J. E., Laubach, J., Barthel, M., Fraser, A., and Phillips, R. L.: Carbon budgets for an irrigated intensively grazed dairy pasture and an unirrigated winter-grazed pasture, Biogeosciences, 13, 2927–2944, https://doi.org/10.5194/bg-13-2927-2016, 2016.
Hutchinson, J. J., Campbell, C. A., and Desjardins, R. L.: Some perspectives on carbon sequestration in agriculture, Agr. Forest Meteorol., 142, 288–302, https://doi.org/10.1016/j.agrformet.2006.03.030, 2007.
Iwasaki, H., Saito, H., Kuwao, K., Maximov, T. C., and Hasegawa, S.: Forest decline caused by high soil water conditions in a permafrost region, Hydrol. Earth Syst. Sci., 14, 301–307, https://doi.org/10.5194/hess-14-301-2010, 2010.
Jans, W. W. P., Jacobs, C. M. J., Kruijt, B., Elbers, J. A., Barendse, S., and Moors, E. J.: Carbon exchange of a maize (Zea mays L.) crop: Influence of phenology, Agr. Ecosyst. Environ., 139, 316–324, https://doi.org/10.1016/j.agee.2010.06.008, 2010.
Kang, M., Ichii, K., Kim, J., Indrawati, Y. M., Park, J., Moon, M., Lim, J. H., and Chun, J. H.: New gap-filling strategies for long-period flux data gaps using a data-driven approach, Atmosphere-Basel, 10, 568, https://doi.org/10.3390/Atmos10100568, 2019.
Kendy, E., Gerard-Marchant, P., Walter, M. T., Zhang, Y. Q., Liu, C. M., and Steenhuis, T. S.: A soil-water-balance approach to quantify groundwater recharge from irrigated cropland in the North China Plain, Hydrol. Process., 17, 2011–2031, https://doi.org/10.1002/hyp.1240, 2003.
Kim, Y., Johnson, M. S., Knox, S. H., Black, T. A., Dalmagro, H. J., Kang, M., Kim, J., and Baldocchi, D.: Gap-filling approaches for eddy covariance methane fluxes: A comparison of three machine learning algorithms and a traditional method with principal component analysis, Glob. Change Biol., 26, 1–20, https://doi.org/10.1111/gcb.14845, 2019.
Kutsch, W. L., Aubinet, M., Buchmann, N., Smith, P., Osborne, B., Eugster, W., Wattenbach, M., Schrumpf, M., Schulze, E. D., Tomelleri, E., Ceschia, E., Bernhofer, C., Beziat, P., Carrara, A., Di Tommasi, P., Grunwald, T., Jones, M., Magliulo, V., Marloie, O., Moureaux, C., Olioso, A., Sanz, M. J., Saunders, M., Sogaard, H., and Ziegler, W.: The net biome production of full crop rotations in Europe, Agr. Ecosyst. Environ., 139, 336–345, https://doi.org/10.1016/j.agee.2010.07.016, 2010.
Lal, R.: World cropland soils as a source or sink for atmospheric carbon, Adv. Agron., 71, 145–191, 2001.
Latimer, R. N. C. and Risk, D. A.: An inversion approach for determining distribution of production and temperature sensitivity of soil respiration, Biogeosciences, 13, 2111–2122, https://doi.org/10.5194/bg-13-2111-2016, 2016.
Lei, H. M. and Yang, D. W.: Seasonal and interannual variations in carbon dioxide exchange over a cropland in the North China Plain, Glob. Change Biol., 16, 2944–2957, https://doi.org/10.1111/j.1365-2486.2009.02136.x, 2010.
Lei, H. M., Yang, D. W., Cai, J. F., and Wang, F. J.: Long-term variability of the carbon balance in a large irrigated area along the lower Yellow River from 1984 to 2006, Sci. China Earth Sci., 56, 671–683, https://doi.org/10.1007/s11430-012-4473-5, 2013.
Li, J., Yu, Q., Sun, X. M., Tong, X. J., Ren, C. Y., Wang, J., Liu, E. M., Zhu, Z. L., and Yu, G. R.: Carbon dioxide exchange and the mechanism of environmental control in a farmland ecosystem in North China Plain, Sci. China Ser. D, 49, 226–240, https://doi.org/10.1007/s11430-006-8226-1, 2006.
Luo, Y., He, C. S., Sophocleous, M., Yin, Z. F., Ren, H. R., and Zhu, O. Y.: Assessment of crop growth and soil water modules in SWAT2000 using extensive field experiment data in an irrigation district of the Yellow River Basin, J. Hydrol., 352, 139–156, https://doi.org/10.1016/j.jhydrol.2008.01.003, 2008.
Mauder, M. and Foken, T.: Documentation and instruction manual of the eddy covariance software package TK2, Abt. Mikrometeorologie, Arbeitsergebnisse, Universität Bayreuth, 2004.
Mauder, M. and Foken, T.: Documentation and instruction manual of the eddy-covariance software package TK3, Abt. Mikrometeorologie, Arbeitsergebnisse, Universität Bayreuth, 2011.
Moureaux, C., Debacq, A., Bodson, B., Heinesch, B., and Aubinet, M.: Annual net ecosystem carbon exchange by a sugar beet crop, Agr. Forest Meteorol., 139, 25–39, https://doi.org/10.1016/j.agrformet.2006.05.009, 2006.
Moureaux, C., Debacq, A., Hoyaux, J., Suleau, M., Tourneur, D., Vancutsem, F., Bodson, B., and Aubinet, M.: Carbon balance assessment of a Belgian winter wheat crop (Triticum aestivum L.), Glob. Change Biol., 14, 1353–1366, https://doi.org/10.1111/j.1365-2486.2008.01560.x, 2008.
National Standards of Environmental Protection of the People's Republic of China: Soil–Determination of organic carbon – Combustion oxidation-titration method, HJ658-2013, Ministry of environmental protection, P.R. China, Beijing, 2013.
Özdoğan, M.: Exploring the potential contribution of irrigation to global agricultural primary productivity, Global Biogeochem. Cy., 25, GB3016, https://doi.org/10.1029/2009GB003720, 2011.
Phillips, C. L., Nickerson, N., Risk, D., and Bond, B. J.: Interpreting diel hysteresis between soil respiration and temperature, Glob. Change Biol., 17, 515–527, https://doi.org/10.1111/j.1365-2486.2010.02250.x, 2011.
Poorter, H., Niklas, K. J., Reich, P. B., Oleksyn, J., Poot, P., and Mommer, L.: Biomass allocation to leaves, stems and roots: meta-analyses of interspecific variation and environmental control, New Phytol., 193, 30–50, https://doi.org/10.1111/j.1469-8137.2011.03952.x, 2012.
Poulter, B., Frank, D., Ciais, P., Myneni, R. B., Andela, N., Bi, J., Broquet, G., Canadell, J. G., Chevallier, F., Liu, Y. Y., and Running, S. W.: Contribution of semi-arid ecosystems to interannual variability of the global carbon cycle, Nature, 509, 600–603, https://doi.org/10.1038/nature13376, 2014.
Reichstein, M., Falge, E., Baldocchi, D., Papale, D., Aubinet, M., Berbigier, P., Bernhofer, C., Buchmann, N., Gilmanov, T., Granier, A., Grunwald, T., Havrankova, K., Ilvesniemi, H., Janous, D., Knohl, A., Laurila, T., Lohila, A., Loustau, D., Matteucci, G., Meyers, T., Miglietta, F., Ourcival, J. M., Pumpanen, J., Rambal, S., Rotenberg, E., Sanz, M., Tenhunen, J., Seufert, G., Vaccari, F., Vesala, T., Yakir, D., and Valentini, R.: On the separation of net ecosystem exchange into assimilation and ecosystem respiration: review and improved algorithm, Glob. Change Biol., 11, 1424–1439, https://doi.org/10.1111/j.1365-2486.2005.001002.x, 2005.
Sauerbeck, D. R.: CO2 emissions and C sequestration by agriculture – perspectives and limitations, Nutr. Cycl. Agroecosys., 60, 253–266, https://doi.org/10.1023/A:1012617516477, 2001.
Schmidt, M., Reichenau, T. G., Fiener, P., and Schneider, K.: The carbon budget of a winter wheat field: An eddy covariance analysis of seasonal and inter-annual variability, Agr. Forest Meteorol., 165, 114–126, https://doi.org/10.1016/j.agrformet.2012.05.012, 2012.
Shen, Y., Zhang, Y., Scanlon, B. R., Lei, H., Yang, D., and Yang, F: Energy/water budgets and productivity of the typical croplands irrigated with groundwater and surface water in the North China Plain, Agr. Forest Meteorol., 181, 133–142, https://doi.org/10.1016/j.agrformet.2013.07.013, 2013.
Smith, P.: Carbon sequestration in croplands: the potential in Europe and the global context, Eur. J. Agron., 20, 229–236, https://doi.org/10.1016/j.eja.2003.08.002, 2004.
Smith, W. K., Cleveland, C. C., Reed, S. C., and Running, S. W.: Agricultural conversion without external water and nutrient inputs reduces terrestrial vegetation productivity, Geophys. Res. Lett., 41, 449–455, https://doi.org/10.1002/2013GL058857, 2014.
Suyker, A. E., Verma, S. B., Burba, G. G., and Arkebauer, T. J., Gross primary production and ecosystem respiration of irrigated maize and irrigated soybean during a growing season, Agr. Forest Meteorol., 31, 180–190, https://doi.org/10.1016/j.agrformet.2005.05.007, 2005.
Suleau, M., Moureaux, C., Dufranne, D., Buysse, P., Bodson, B., Destain, J. P., Heinesch, B., Debacq, A., and Aubinet, M.: Respiration of three Belgian crops: Partitioning of total ecosystem respiration in its heterotrophic, above- and below-ground autotrophic components, Agr. Forest Meteorol., 151, 633–643, https://doi.org/10.1016/j.agrformet.2011.01.012, 2011.
Taylor, A. M., Amiro, B. D., and Fraser, T. J.: Net CO2 exchange and carbon budgets of a three-year crop rotation following conversion of perennial lands to annual cropping in Manitoba, Canada, Agr. Forest Meteorol., 182–183, 67–75, https://doi.org/10.1016/j.agrformet.2013.07.008, 2013.
Terazawa, K., Maruyama, Y., and Morikawa, Y.: Photosynthetic and Stomatal Responses of Larix-Kaempferi Seedlings to Short-Term Waterlogging, Ecol. Res., 7, 193–197, https://doi.org/10.1007/Bf02348500, 1992.
Tian, H., Melillo, J., Kicklighter, D., McGuire, A., and Helfrich, J.: The sensitivity of terrestrial carbon storage to historical climate variability and atmospheric CO2 in the United States, Tellus B, 51, 414–452, 1999.
Ueyama, M., Ichii, K., Iwata, H., Euskirchen, E. S., Zona, D., Rocha, A. V., Harazono, Y., Iwama, C., Nakai, T., and Oechel, W. C.: Upscaling terrestrial carbon dioxide fluxes in Alaska with satellite remote sensing and support vector regression, J. Geophys. Res.-Biogeo., 118, 1266–1281, https://doi.org/10.1002/jgrg.20095, 2013.
van Wesemael, B., Paustian, K., Meersmans, J., Goidts, E., Barancikova, G., and Easter, M.: Agricultural management explains historic changes in regional soil carbon stocks, P. Natl. Acad. Sci. USA, 107, 14926–14930, https://doi.org/10.1073/pnas.1002592107, 2010.
Verma, S. B., Dobermann, A., Cassman, K. G., Walters, D. T., Knops, J. M., Arkebauer, T. J., Suyker, A. E., Burba, G. G., Amos, B., Yang, H. S., Ginting, D., Hubbard, K. G., Gitelson, A. A., and Walter-Shea, E. A.: Annual carbon dioxide exchange in irrigated and rainfed maize-based agroecosystems, Agr. Forest Meteorol., 131, 77–96, https://doi.org/10.1016/j.agrformet.2005.05.003, 2005.
Vick, E. S. K., Stoy, P. C., Tang, A. C. I., and Gerken, T.: The surface-atmosphere exchange of carbon dioxide, water, and sensible heat across a dryland wheat-fallow rotation, Agr. Ecosyst. Environ., 232, 129–140, https://doi.org/10.1016/j.agee.2016.07.018, 2016.
Wang, Y. Y., Hu, C. S., Dong, W. X., Li, X. X., Zhang, Y. M., Qin, S. P., and Oenema, O.: Carbon budget of a winter-wheat and summer-maize rotation cropland in the North China Plain, Agr. Ecosyst. Environ., 206, 33–45, https://doi.org/10.1016/j.agee.2015.03.016, 2015.
Wolf, J., West, T. O., Le Page, Y., Kyle, G. P., Zhang, X., Collatz, G. J., and Imhoff, M. L.: Biogenic carbon fluxes from global agricultural production and consumption, Global Biogeochem. Cy., 29, 1617–1639, https://doi.org/10.1002/2015gb005119, 2015.
Zhang, Q., Lei, H. M., and Yang, D. W.: Seasonal variations in soil respiration, heterotrophic respiration and autotrophic respiration of a wheat and maize rotation cropland in the North China Plain, Agr. Forest Meteorol., 180, 34–43, https://doi.org/10.1016/j.agrformet.2013.04.028, 2013.
Zhang, Q., Katul, G. G., Oren, R., Daly, E., Manzoni, S., and Yang, D. W.: The hysteresis response of soil CO2 concentration and soil respiration to soil temperature, J. Geophys. Res.-Biogeo., 120, 1605–1618, https://doi.org/10.1002/2015JG003047, 2015a.
Zhang, Q., Lei, H. M., Yang, D. W., Bo, H. B., and Cai, J. F.: On the diel characteristics of soil respiration over the North China Plain, J. Tsinghua University (Science and Technology), 55, 33–38, 2015b (in Chinese with English abstract).
Zhang, Q., Phillips, R. P., Manzoni, S., Scott, R. L., Oishi, A. C., Finzi, A., Daly, E., Vargas, R., and Novick, K. A.: Changes in photosynthesis and soil moisture drive the seasonal soil respiration-temperature hysteresis relationship, Agr. Forest Meteorol., 259, 184–195, https://doi.org/10.1016/j.agrformet.2018.05.005, 2018.
Zhang, Y. Q., Yu, Q., Jiang, J., and Tang, Y. H.: Calibration of Terra/MODIS gross primary production over an irrigated cropland on the North China Plain and an alpine meadow on the Tibetan Plateau, Glob. Change Biol., 14, 757–767, https://doi.org/10.1111/j.1365-2486.2008.01538.x, 2008.
Zhao, M. S., Heinsch, F. A., Nemani, R. R., and Running, S. W.: Improvements of the MODIS terrestrial gross and net primary production global data set, Remote Sens. Environ., 95, 164–176, https://doi.org/10.1016/j.rse.2004.12.011, 2005.
- Abstract
- Introduction
- Materials and methods
- Results
- Discussion
- Conclusion
- Appendix A: Flux calculation of the period with equipment failure
- Appendix B: Multiple regression for NEE, GPP and ER with microclimate variables
- Data availability
- Author contributions
- Competing interests
- Acknowledgements
- Financial support
- Review statement
- References
- Abstract
- Introduction
- Materials and methods
- Results
- Discussion
- Conclusion
- Appendix A: Flux calculation of the period with equipment failure
- Appendix B: Multiple regression for NEE, GPP and ER with microclimate variables
- Data availability
- Author contributions
- Competing interests
- Acknowledgements
- Financial support
- Review statement
- References