the Creative Commons Attribution 4.0 License.
the Creative Commons Attribution 4.0 License.
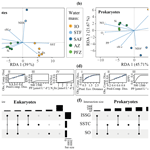
Hydrographic fronts shape productivity, nitrogen fixation, and microbial community composition in the southern Indian Ocean and the Southern Ocean
Eric J. Raes
Pier Luigi Buttigieg
Claire Lo Monaco
Anya M. Waite
Biogeochemical cycling of carbon (C) and nitrogen (N) in the ocean depends on both the composition and activity of underlying biological communities and on abiotic factors. The Southern Ocean is encircled by a series of strong currents and fronts, providing a barrier to microbial dispersion into adjacent oligotrophic gyres. Our study region straddles the boundary between the nutrient-rich Southern Ocean and the adjacent oligotrophic gyre of the southern Indian Ocean, providing an ideal region to study changes in microbial productivity. Here, we measured the impact of C and N uptake on microbial community diversity, contextualized by hydrographic factors and local physico-chemical conditions across the Southern Ocean and southern Indian Ocean. We observed that contrasting physico-chemical characteristics led to unique microbial diversity patterns, with significant correlations between microbial alpha diversity and primary productivity (PP). However, we detected no link between specific PP (PP normalized by chlorophyll-a concentration) and microbial alpha and beta diversity. Prokaryotic alpha and beta diversity were correlated with biological N2 fixation, which is itself a prokaryotic process, and we detected measurable N2 fixation to 60∘ S. While regional water masses have distinct microbial genetic fingerprints in both the eukaryotic and prokaryotic fractions, PP and N2 fixation vary more gradually and regionally. This suggests that microbial phylogenetic diversity is more strongly bounded by physical oceanographic features, while microbial activity responds more to chemical factors. We conclude that concomitant assessments of microbial diversity and activity are central to understanding the dynamics and complex responses of microorganisms to a changing ocean environment.
Please read the corrigendum first before continuing.
-
Notice on corrigendum
The requested paper has a corresponding corrigendum published. Please read the corrigendum first before downloading the article.
-
Article
(1853 KB)
- Corrigendum
-
Supplement
(12450 KB)
-
The requested paper has a corresponding corrigendum published. Please read the corrigendum first before downloading the article.
- Article
(1853 KB) - Full-text XML
- Corrigendum
-
Supplement
(12450 KB) - BibTeX
- EndNote
The Southern Ocean (SO), in particular its sub-Antarctic zone, is a major sink for atmospheric CO2 (Constable et al., 2014). The SO is separated from the Indian South Subtropical Gyre (ISSG) by the South Subtropical Convergence province (SSTC), comprising the Subtropical Front (STF) and the Subantarctic Front (SAF). The SSTC is a zone of deep mixing and thus elevated nutrient concentrations (Longhurst, 2007). Further, the SSTC has been shown to act as a transition zone both numerically and taxonomically for dominant populations of marine bacterioplankton (Baltar et al., 2016).
In this dynamic context, a key driver of microbial productivity is nutrient availability, especially through tightly coupled carbon (C) and nitrogen (N) cycles. The constant availability of nutrients through vertical mixing in frontal zones, such as the STF, enhances primary productivity (Le Fèvre, 1987) and chlorophyll-a (chl a) concentrations (Belkin and O'Reilly, 2009). Primary productivity (PP) and specific primary productivity (PB, meaning primary productivity per unit chl a) are reflected in the relative abundance of different phytoplankton size classes whose productivity values are, in turn, stimulated by nutrient injections via shallowing of mixed layer depth (MLD) at the SO fronts (Strass et al., 2002); decreasing the possibility of N limitation. However, N limitation can also biologically be alleviated through N2 fixation mediated by diazotrophs, significantly contributing to the N pool in oligotrophic regions (Tang et al., 2019). In high-latitude regions, biological N2 fixation could potentially have a large impact on productivity (Sipler et al., 2017). However, large disagreements exist between models of high-latitude N2 fixation and its coupling to microbial diversity due to sparse sampling in these regions (Tang et al., 2019).
Due to the dynamics of the region, conflicting observations, and climate-driven changes, resolving the coupling of microbial productivity and diversity is particularly important across the strong environmental gradients crossing the ISSG, through the SSTC into the SO. Indeed, climate variability has been shown to impact ocean productivity and thus influences the provision of resources to sustain ocean life (Behrenfeld et al., 2006). To date, observations of climate-change-related effects in this region of the SO have been synthesized only based on long-term nutrient concentration and physical (temperature and salinity) changes (Lo Monaco et al., 2010); however, these typically lack a microbial dimension. Microbial composition, activity, and C export may all be impacted by climate-driven changes in ocean dynamics (Evans et al., 2011) such as MLD shallowing, eddy formation, and poleward shifts of ocean fronts (Chapman et al., 2020). For a more holistic ecosystem-based understanding of this region, concomitant assessments of (1) steady-state biogeochemical processes through rate measurements of key elements (such as C and N) and (2) the microbial diversity that underpins it are essential enhancements to such long-term investigations.
Here, we measure the impact of C and N uptake on microbial community diversity, alongside the effects of hydrography (e.g., dispersal limitation) and local physico-chemical conditions across the Southern Ocean and southern Indian Ocean. We focused our investigation on surface communities, aiming to resolve horizontal surface variation. We used our observation to assess whether the following relationships – previously observed in related systems – hold in our study region:
-
Microbial diversity increases with increasing primary productivity (PP). Previous work has claimed that more resources support higher species richness until intermediate rates of PP (Fig. 1; Vallina et al., 2014) within ocean provinces (Raes et al., 2018).
-
Frontal systems are discrete ecological transition zones between regions that provide perspectives on the findings of Baltar et al. (2016; see above). These systems often separate water masses with distinct trophic structures (e.g., Albuquerque et al., 2021).
-
Microbial alpha and beta diversity are impacted by N2 fixation, which is itself correlated with the presence of other available sources of N and/or temperature; this is to provide more evidence on the role of N2 fixation to the N budget in high latitudes (see e.g., Shiozaki et al., 2018; Sipler et al., 2017).
To our knowledge, there are no concomitant evaluations of how surface gradients, microbial activity, and community composition relate to one another in this region. Here, we provide perspectives on these key relationships across the Indian South Subtropical Gyre (ISSG), the Subtropical Front (STF), and Subantarctic Front (SAF), and the SO comprising the Polar Front (PF) and Antarctic Zone (AZ).
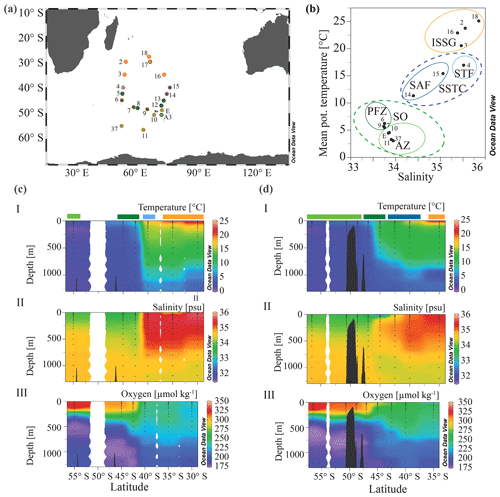
Figure 1(a) The MD206 transect and OISO stations. Stations are colored according to water masses and encircled by sampling extent: black circles indicate stations where only CTD (conductivity, temperature, depth) data are provided, and stations encircled in red denote where additional samples for C, N, and community composition were taken. (b) A plot of potential temperature (in degrees Celsius (∘C) and salinity (in practical salinity units)) using sea surface (7 m) data of the stations used in further microbial and analyses. The yellow circle highlights the Indian South Subtropical Gyre (ISSG), light blue circle the Subtropical Front (STF), blue circle the Subantarctic Front (SAF), dark green circle the Polar Front Zone (PFZ) and the light green circle indicates the Antarctic Zone (AZ); dashed lines indicate water masses clustered within ocean provinces: the blue line marks the South Subtropical Convergence province (SSTC), and the green line marks the Southern Ocean (SO); panels (c) and (d) show depth profiles of temperature, oxygen, and salinity along two transects of the OISO stations. Colored bars indicate water masses according to (b). Panel (c) shows the western transect covering OISO stations 2, 3, 4, 5, 6, and 37 around 53±1∘ E longitude; panel (d) shows the eastern transect of OISO stations 10, 11, 12, 13, 14, 15, 16, and E around 68±5∘ E.
2.1 Study region, background data, and sample collection
Our study region ranged from Réunion in the Indian South Subtropical Gyre (ISSG) to south of the Kerguelen Islands in the Southern Ocean (56.5∘ S, 63.0∘ E; Fig. 1a) as part of a larger repeated “OISO” sampling program – (Océan Indien Service d'Observations; Metzl and Lo Monaco, 1998; https://doi.org/10.17600/17009700). Samples were collected as part of the VT153/OISO27 (MD206) cruise aboard the R/V Marion Dufresne from 6 January to 7 February 2017. Physical and biogeochemical data, as well as metadata, were collected from a rosette equipped with Niskin bottles and a conductivity, temperature, depth sensor (CTD) (Sea-Bird SBE32) equipped with a SBE43 O2 sensor and a Chelsea Aqua tracker fluorometer. OISO long-term data, starting in 1998, were used as a backdrop to our data collected in 2018 and allowed us to monitor changes in physical and chemical oceanographic properties over time (Supplement File A).
2.2 Province delineation after Longhurst
We identified three main clusters (i.e., ocean provinces) and five subclusters (i.e., water masses) on a temperature–salinity plot (Fig. 1b). As an overview, we used CTD depth profiles to validate the vertical extent of water masses in our samples (Fig. 1c, d) and checked the horizontal extent of the identified clusters using remote sensing data of sea surface temperature (Fig. S2 in the Supplement). Additionally, we checked the horizontal boundaries of these clusters for matches in strong chl a concentration gradients as an approximate for biological component of ocean provinces, following the concept of Longhurst (2007). Satellite data were acquired from MODIS (https://neo.sci.gsfc.nasa.gov/, last access: 16 June 2021), with images processed by NASA Earth Observations (NEO) in collaboration with Gene Feldman and Norman Kuring, i.e., NASA OceanColor Group (Fig. S3). We calculated the geodesic distance between sites from latitude and longitude coordinates using the geodist package in R (v0.0.4; Padgham et al., 2020).
2.3 Nutrient analysis
Dissolved inorganic nutrient concentrations, including phosphate (PO), silicate (denoted Si), mono-nitrogen oxides (NOx), nitrite (NO), and ammonium (NH), were assayed on a QuAAtro39 continuous segmented flow analyzer (Seal Analytical) following widely used colorimetric methods (Armstrong, 1951; Murphy and Riley, 1962; Wood et al., 1967) with adaptations to particular needs for Seal Analytical QuAAtro autoanalyzer. NH was measured using the fluorometric method of Kérouel and Aminot (1997). Detection limits of these methods were 0.1 µmol L−1 for PO, 0.3 µmol L−1 for Si, 0.03 µmol L−1 for NOx, and 0.05 µmol L−1 for NH.
2.4 Dissolved inorganic nitrogen and carbon assimilation
At each CTD station, water samples to measure primary productivity (PP) and N2 fixation were taken from the underway flow-through system (intake at 7 m). As the ship was moving during sampling, the distance between samples of the same station can range up to ∼ 15 km. Incubations were performed in acid-washed polycarbonate bottles on deck at ambient light conditions. All polycarbonate incubation bottles were rinsed prior to sampling with 10 % HCl (3×), deionized H2O (3×), and sampling water (2×). In order to obtain the natural abundance of particulate nitrogen (PN) and particulate organic carbon (POC), which we used as a t0 value to calculate the assimilation rates, 4 L of water was filtered onto a 25 mm pre-combusted GF/F filter for each station.
N2 fixation experiments were carried out in triplicate for each station. We used the combination of the bubble approach (Montoya et al., 1996) and the dissolution method (Mohr et al., 2010) proposed by Klawonn et al. (2015). The 4.5 L bottles were filled up headspace free. All incubations were initialized by adding a 15N2 gas bubble with a volume of 10 mL. We used 15N2-labeled gas provided by Cambridge Isotope Laboratories (Tewksbury, MA). Bottles were gently rocked for 15 min. Finally, the remaining bubble was removed to avoid further equilibration between gas and the aqueous phase. After 24 h, a water subsample was transferred to a 12 mL exetainer® and preserved with 100 µL HgCl2 solution for later determination of exact 15N–15N concentration in solution. Natural 15N2 was determined using membrane inlet mass spectrometry (MIMS; GAM200, IPI) for each station with an average enrichment of 3.8±0.007 at. % 15N2 (mean ± SD; n=104). Primary productivity was measured by adding Na13CO3 at a final 13C concentration of 200 µmol L−1.
Incubation bottles were incubated on board at ambient sea surface temperature (SST; water intake at 7 m) using a continuous-flow-through system. Temperature of both incubation bins was continuously measured. After 24 h, the C and N2 fixation experiments were terminated by collecting the suspended particles from each bottle by gentle vacuum filtration through a 25 mm pre-combusted GF/F filter (<10 kPa). Filters were snap-frozen in liquid nitrogen and stored at −80 ∘C while at sea. Filters with enriched (T24) and unenriched (T0) samples were acidified and dried overnight at 60 ∘C. Analysis of 15N and 13C incorporated was carried out by the isotopic laboratory at the University of California, Davis, California campus, using an Elementar Vario EL Cube or MICRO cube elemental analyzer (Elementar Analysensysteme GmbH, Hanau, Germany).
Carbon assimilation rates were calculated according to Knap et al. (1996), excluding the 14C–12C conversion factor, and N2 fixation was calculated according to Montoya et al. (1996). The minimum quantifiable rate was calculated according to Gradoville et al. (2017).
2.5 Pigment analysis
For pigment analyses, 4 L of seawater was filtered (< 10 kPa) on a 47 mm Whatman GF/F filter and stored at −80 ∘C until further analysis. High-performance liquid chromatography (HPLC) was carried out as described in Kilias et al. (2013) with the following modifications: 150 µL of the internal standard canthaxanthin was included to each sample. Samples were dissolved in 4 mL acetone and disrupted with glass beads using a Precellys 24 tissue homogenizer (Bertin Technologies, France) at 7200 rpm for 20 s. Detection of the sample at 440 nm absorbance was performed using an HPLC analyzer (Varian Microsorb-MV 100-3 C8). We used chl a concentration to estimate phytoplankton biomass. Pigment concentrations were calculated according to Kilias et al. (2013) and quality controlled according to Aiken et al. (2009) (Supplement File A).
HPLC output data were analyzed using diagnostic pigments for the different taxa and phytoplankton functional types (PFTs) after Hirata et al. (2011) (Supplement File A, Table S2). This approach can be used to reveal dominant trends of the phytoplankton community and size structure at the regional and seasonal scales (Ras et al., 2008). Furthermore, diagnostic pigments were used to delineate three different size classes (pico-, nano-, and microplankton) according to Vidussi et al. (2001). The relative proportion of each phytoplankton size class (PSC) was calculated based on the linear regression model proposed by Uitz et al. (2006). We investigated the patterns of PSCs with a second-order polynomial fit (S1_code_archive/pigment_HPLC/diaganostic_ pigments.R L143:153).
2.6 DNA analysis
Two liters of seawater from the shipboard underway system from each station were filtered through a 0.22 µm Sterivex® filter cartridge for DNA isolation, snap-frozen in liquid nitrogen, and stored at −80 ∘C. DNA was extracted using a DNeasy® Plant Mini Kit (QIAGEN, Valencia, CA, USA, catalog no. or ID 69106) following the manufacturer's instructions. Sterivex cartridges were gently cracked open, and filters were removed and transferred into a new and sterile screw-cap tube. Approximately 0.3 g of pre-combusted glass beads (diameter 0.1 mm; 11079101 Bio Spec Products) and 400 µL buffer AP1 were added to the filter, followed by a bead beating step using a Precellys 24 tissue homogenizer (Bertin Technologies, France), with two times at 5500 rpm for 20 s with 2 min on ice in between and a final bead beating step at 5000 rpm for 15 s. DNA concentrations were quantified by the Quantus™ fluorometer and normalized to 2 ng µL−1.
2.6.1 Amplicon 16S and 18S rRNA gene PCR and sequencing
Amplicons of the bacterial 16S rRNA gene and eukaryotic 18S rRNA gene (using primers from 27F–519R; Parada et al., 2016, TA-Reuk454FWD1 – TAReukREV3; Stoeck et al., 2010, respectively) were generated following standard protocols of amplicon library preparation (16S Metagenomic Sequencing Library Preparation, Illumina, part no. 15044223 Rev. B; Supplement File B). The 16S and 18S rRNA gene PCR products were sequenced using 250 bp paired-end sequencing with a MiSeq sequencer (Illumina) at the European Molecular Biology Laboratory (EMBL) in Heidelberg (Germany) and at the Leibniz Institute on Aging (FLI) in Jena (Germany), respectively.
2.6.2 Amplicon sequence data analysis
For both 16S rRNA gene and 18S rRNA gene amplicon sequences, we used the DADA2 R package, v1.15.1 (Callahan et al., 2016) to construct Amplicon sequence variant (ASV) tables by the following steps: prefiltering “filterandtrim” function with truncL = 50 and default parameters (S1_code_archive/dada2). Primer sequences were cut using the Cutadapt software implementation (v1.18) in the DADA2 pipeline, removing a fixed number of bases matching the 16S forward (515F-Y, 19 bp) and reverse (926R, 20 bp) primers and the 18S forward (TA-Reuk454FWD1, 20 bp) and reverse (TAReukREV3, 21 bp) primers (S1_code_archive/dada2/dada2_16S.R L88:104; S1_code_archive/dada2/dada2_18S.R L92:104). Primer-trimmed fastq files were quality trimmed with a minimum sequence length of 50 bp and checked by inspection of the average sequence length distribution (for both the 16S rRNA gene and 18S rRNA gene sequences). Samples within forward and reverse fastq files were dereplicated and merged with a minimum overlap of 20 bp. ASV tables were constructed, and potential chimeras were identified de novo and removed using the “removeBimeraDenovo” command. Sequencing statistics for removed reads and sequences in each step can be found in Table S3. Taxonomic assignment was performed using the SilvaNGS (v1.4; Quast et al., 2013) pipeline for 16S rRNA gene data with the similarity threshold set to 1. Reads were aligned using SINA v1.2.10 (Pruesse et al., 2012) and classified using BLASTn (v2.2.30; Camacho et al., 2009) with the Silva database (v132) as a reference database (Supplement File C). For taxonomic assignment of 18S rRNA gene amplicons, we used the plugin “feature-classifier” (from package “q2-feature-classifier”, v2019.7.0) in QIIME2 (Bokulich et al., 2018) and the pr2 database (v4.12; Guillou et al., 2013). We removed ASVs annotated to mitochondria and chloroplasts from 16S rRNA gene ASV tables and ASVs annotated as metazoans from 18S rRNA gene ASV tables (S1_code_archive/import/import_16S.R L35:38; S1_code_archive/import/import_18S.R L29). ASV tables of 16S rRNA gene amplicon (Table S4) and 18S rRNA gene amplicons (Table S5) were used for further statistical analyses.
2.7 Ecological data and statistical analysis
A combination of temperature, salinity, dissolved oxygen concentrations, and dissolved inorganic nutrient concentrations (NO, NO, NH, Si, and PO were used to characterize the physical and biogeochemical environment of the study region.
All statistical tests were performed in R version 3.6.3 (R Core Team, 2020).
Statistical documentation, package citations, and scripts are available in
S1. Microbial alpha diversity was calculated with Hill numbers (richness,
Shannon entropy, inverse Simpson, q = 0–2;
Chao et al., 2014) using
the iNEXT package v2.0.20 in R with confidence set to 0.95 and bootstrap = 100 (S1_code_archive/alpha_diversity). Accordingly, rarefaction curves are shown in Fig. S6. Pearson
correlations between microbial richness (q=0), inverse Simpson diversity
(q=2), environmental parameters, and biological rates were calculated
and plotted (ggplot2) (Fig. S7). The p values were adjusted for multiple testing
using Holm adjustment (Holm, 1979), and residuals were checked for
normal distribution (Fig. S8). For comparability and statistical downstream
analyses, we performed the following transformations to the ASV table and
the environmental metadata: to account for the compositionality of
sequencing data (see Gloor et al., 2017), we
performed a centered log ratio (CLR)-transformation for redundancy analysis (RDA). We used
Hellinger transformation (decostand()
function in vegan) of the ASV pseudocount data
(minimum pseudocount per ASV cutoff was 3) for PERMANOVA analyses.
Environmental data were z scored for comparable metadata analysis
(S1_code_archive/transformations). For
multivariate analyses of microbial beta diversity and environmental
parameters, we performed redundancy analyses (RDA) of the CLR-transformed
ASV tables (S1_code_archive/RDA). Differences
of microbial beta diversity (based on Hellinger-transformed ASV tables),
phytoplankton community composition (based on pigment concentrations), and
water masses were tested with permutational ANOVA (PERMANOVA;
Anderson, 2001) using the adonis2()
function in
vegan along with a beta dispersion test to evaluate the homogeneity of
the dispersion (Fig. S9). To investigate where differences of environmental
variables have an impact on microbial community dissimilarity, we performed
a general dissimilarity model (GDM) of the community dissimilarity and
environmental variables, and we checked for the influence of geographic
distance based on spline magnitude (gdm package; S1_code_archive/GDM).
As differences in microbial beta diversity were significant in PERMANOVA between provinces and water masses, we performed a similarity percentage (SIMPER) analysis in R using the vegan package to assess which ASVs contribute most to the observed variance of microbial community composition (Table S6; S1_code_archive/taxonomy_analyses). To determine the number of ASVs shared between provinces (or unique to certain provinces), we transformed ASV pseudocount tables into binary tables and calculated shared and unique ASVs using the upsetR package in R (v.4, Conway et al., 2017; S1_code_archive/upsetR). We calculated the percentage of all within-sample-observed ASVs within the merged samples of a province (Table S7).
3.1 Delimitation of regional water masses
Through our analysis of temperature, salinity, oxygen, and dissolved inorganic nutrient (N, P, Si) concentrations, we identified five distinct water masses, fronts, and frontal zones: the ISSG, STF, SAF, PFZ, and AZ, which broadly aligned with three oceanographic provinces (ISSG, SSTC, and SO; Fig. 1a). Within the Southern Ocean (SO), we identified four water masses in our transect including the Antarctic Zone (AZ) and three distinct frontal systems: (1) the Polar Front (PF), (2) the Subantarctic Front (SAF), and (3) the Subtropical Front (STF; Fig. 1). In our analysis, stations 6, 7, and 9 were placed within the Polar Front Zone (PFZ), which is between the SAF and PF. Due to the bathymetrically driven convergence of the STF and SAF around Kerguelen island, we consider the SAF as part of the convergence zone between the SO and Indian Ocean (IO), i.e., the South Subtropical Convergence province (SSTC), rather than as a Southern Ocean frontal system. At 7 m depth, we noted clear shifts in temperature (SST), salinity, and dissolved inorganic nutrient (NO, PO, Si) concentrations when crossing the STF. The STF is described as a circumpolar frontal zone creating the boundary between our measurements of the warm (20–25 ∘C), saline (>35), and oligotrophic (NO < 0.03 µM; PO: 0.04–0.21 µM) subtropical waters (STW) of the Indian South Subtropical Gyre (ISSG) and the cold (3–6 ∘C) macronutrient-rich SO (NO: 19.2–24.9 µM; PO: 1.43–1.71 µM) (Figs. 1, 2, S3). In the context of this study, STW and ISSG could be used interchangeably; we henceforth refer to it as ISSG.
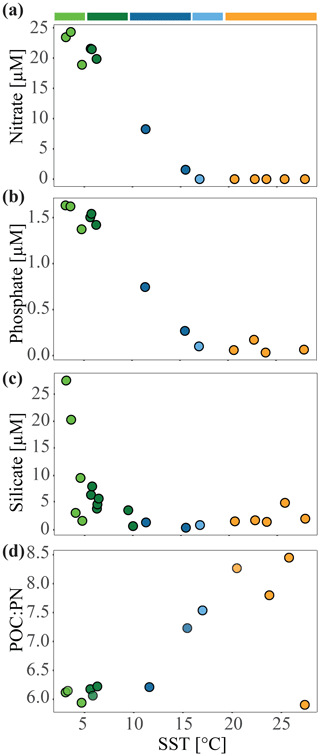
Figure 2Nutrient concentrations (µmol L−1) and molar ratios of particulate organic carbon (POC) to particulate nitrogen (PN) during the MD206 expedition against sea surface temperature (∘C): (a) nitrate, (b) phosphate, (c) silicate, and (d) POC : PN ratio. Colored bars indicate water masses according to their sea surface temperature: yellow bar highlights the Indian South Subtropical Gyre (ISSG), light blue bar highlights the Subtropical Front (STF), blue bar highlights the Subantarctic Front (SAF), dark green bar highlights the Polar Front Zone (PFZ), and light green bar highlights the Antarctic Zone (AZ).
3.2 Primary productivity (PP)
Maximum primary productivity (PP) values within our dataset were measured near the Kerguelen Plateau in the Polar Front Zone (PFZ) at station 9 (3236.8 and 3553.3 µmol C L−1 d−1, respectively) and station E (2212.4–2688.1 µmol C L−1 d−1, n=6). Comparing all PP measurements across water masses, we found relatively high PP in other stations of the PFZ (stations 6, 7; Fig. 3a; Table 1) and in the Subantarctic Front (SAF) (stations 4, 15). Lowest PP values (190.4–642.6 µmol C L−1 d−1) were measured at the stations in the Indian South Subtropical Gyre (ISSG). While stations in the ISSG showed very little variations within one station (e.g., 226.09–371.07 µmol C L−1 d−1, n=6, station 18), variation within SO stations was relatively high (e.g., 587.42–1875.58 µmol C L−1 d−1, n=6, station 37; Table 1).
Table 1Sampling stations visited during the MD206 cruise, including chlorophyll-a concentrations, primary productivity (PP), specific primary productivity (PB), and N2 fixation. Mixed layer depth (MLD) was calculated using Δd=0.03 kg m−3 compared to a surface reference depth of 7 m. NA indicates no data. Ranges and mean for sample replicates of N2 fixation and PP are given (n=3 for stations 3, 9, 11, 15; n=6 for stations E, 37, 2, 4, 6, 7, 14, 16, 18).
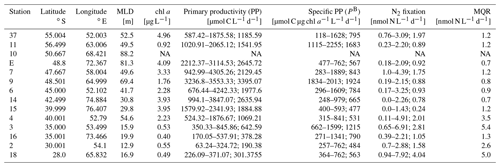
Overall, the variation of specific primary productivity (PB) did not show great variations between provinces, with maximum rates at station 11 (Table 1; Fig. 3b). We did not find a significant correlation between mixed layer depth and PB (Pearson correlation: r=0.21, p=0.47, n=12).
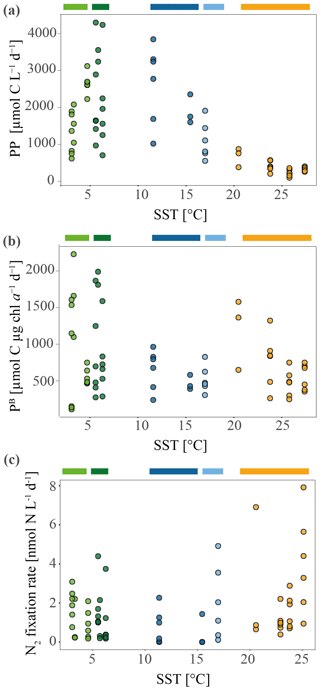
Figure 3Primary productivity (PP) and specific primary productivity (PB) measured during the MD206 cruise. (a) PP in micromole carbon per liter per day against sea surface temperature (SST) in degrees Celsius (∘C). (b) PB, normalized by chl a concentration. (c) Nitrogen fixation rates against sea surface temperature (SST) in degrees Celsius measured during the MD206 cruise. Rates are shown in nanomole nitrogen per liter per day. Colored bars indicate water masses: yellow bar highlights the Indian South Subtropical Gyre (ISSG), light blue bar highlights the Subtropical Front (STF), dark blue bar highlights the Subantarctic Front (SAF), dark green bar highlights the Polar Front Zone (PFZ), and light green bar marks the Antarctic Zone (AZ).
3.3 N2 fixation
Di-nitrogen (N2) fixation was above the minimum quantifiable rate (MQR) at all stations (Table 1). N2 fixation measurements did not show a clear temperature-dependent trend (Fig. 3), and neither were they directly associated with low dissolved inorganic nutrient (DIN) values (Fig. S10). N2 fixation in the warm oligotrophic waters of the Indian South Subtropical Gyre (ISSG) was up to 7.93 nmol N L−1 d−1 (station 18; Fig. 3c; Table 1). Lowest N2 fixations were measured in the productive zone of the STF and SAF (0.24–2.01 nmol N L−1 d−1, n=3). In the AZ, N2 fixation ranged between 0.89 and 1.97 nmol N L−1 d−1. The variation between replicates was high; e.g., rates ranged between 0.9 and 7.9 nmol N L−1 d−1 at station 18 (Table 1). Across provinces, we did not find notable differences in N2 fixation.
3.4 Phytoplankton pigment analyses
Photosynthetic pigment concentrations showed a clear separation between the oligotrophic ISSG and the nutrient-rich SO (Fig. S5). Chlorophyll-a concentrations were relatively low in the warmer water stations of the ISSG than in the SSTC and SO (Table 1). The relative proportion of phytoplankton biomass to the total organic matter was estimated by calculating the ratio of PN : chl a and showed a strong increase in the ISSG (11.5–29.7 PN : chl a, n=4) in comparison to the SSTC (2.7–7.2 PN : chl a, n=3) and SO (2.8–15.3 PN : chl a, n=6; Fig. S4).
The phytoplankton community composition was significantly and markedly different across provinces (PERMANOVA; permutations = 999, R2=0.76, p<0.001; n=14) and water masses (PERMANOVA; permutations = 999, p=0.002; R2=0.81, n=14). The pigment concentration of prokaryote-specific pigment zeaxanthin was high in the ISSG (0.03–0.06 mg m−3, n=4; Fig. S5a). Zeaxanthin still occurred in the STF and SAF (0.03–0.04 mg m−3, n=3) but disappeared in the SO (<0.01 mg m−3, n=6). Prochlorococcus was distinctly identified through its diagnostic pigment, divinyl chl a, and showed a relatively high pigment concentration in the ISSG (0.02–0.03 mg m−3, n=4; Fig. S5a). We found concentrations of diatom-specific fucoxanthin (except station 18) ranging from 0.021 mg m−3 in the ISSG (station 16) to 0.34 mg m−3 in the SO (station 37; Fig. S5a). Across water masses, fucoxanthin concentration was slightly higher in the AZ (0.06–0.5 mg m−3, n=4) than in all other water masses (0–0.13 mg m−3, n=10).
The distribution of potential phytoplankton size classes (PSCs; pico- nano- and microplankton), calculated from diagnostic pigments (Supplement File A), showed a clear pattern over temperature variations (Fig. S5b). The pigment data suggested that picoplankton dominated warm water in the ISSG, and picoplankton abundance sharply decreased (second-order polynomial fit: R2=0.98, p<0.001, n=14) at lower values of SST. Pigment data also suggested that microplankton showed a contrary trend to the relative fraction of picoplankton, having high abundance in cold water and decreasing at higher values of SST, with a minimum at 20 ∘C SST and a slight increase (14 % microplankton of all phytoplankton size classes) towards 25 ∘C SST (second-order polynomial fit: R2=0.77, p<0.001, n=14). Nanoplankton showed a maximum at 12 ∘C SST and decreased both towards warmer and colder waters (second-order polynomial fit, R2=0.58, p<0.01, n=14).
3.5 Eukaryotic planktonic community composition
For each station, except station 4, the V4 region of the small subunit ribosomal RNA gene (18S rRNA) was amplified and sequenced to determine the community composition of micro-, nano-, and pico-eukaryotes in all three oceanic provinces. We recovered a total of 2618 ASVs. After removing sequences annotated to metazoans, 2501 ASVs remained (4.4 % of ASVs removed).
We found a strong correlation between both eukaryotic richness and diversity (inverse Simpson index) with SST (Pearson correlations: r=0.85, p<0.001 for richness and r=0.82, p=0.001 for inverse Simpson, n=12; Fig. S7a, c). Overall, eukaryotic diversity was negatively correlated with PP (, p=0.02, n=12; Fig. S7e) and significantly and positively associated with N2 fixation (r=0.74, p=0.01, n=12; Fig. S7g). However, a strong correlation between rate measurements (PP, N2 fixation) and eukaryotic diversity was only apparent in the ISSG, and no significant trend across other provinces (Pearson correlation after removal of ISSG samples from dataset: for PP r=0.47, p=0.24 and for N2 fixation , p=0.23, n=8).
Our RDA constrained 81 % of the variance in the ASV table, with a p value of 0.095 (permutations = 999, n=12). Sites were well separated between Longhurst provinces along the first two RDA axes (capturing 52.67 % constrained variance, Fig. 4a). Our PERMANOVA, which tested the province-based separation, produced moderate but significant results (permutations = 999, R2=0.54, p=0.001, n=12). An additional PERMANOVA grouping sites by water masses produced similar results (permutations = 999, R2=0.67, p=0.001, n=12; Fig. 4a). We found that more ASVs only occurred in one province rather than in two or more provinces (Fig. 4e). Sites within the ISSG province were associated with SST and N2 fixation. Sites in the SSTC were associated with high NH concentrations. Sites belonging to the SO were associated with dissolved inorganic nutrients (NO, PO, Si), dissolved oxygen, and chl a concentrations as well as high PP. Linear relationships between beta diversity and rates were only weak for PP (PERMANOVA; permutations = 999, R2=0.27, p=0.004, n=12) and both weak and insignificant between beta diversity and N2 fixation (PERMANOVA; permutations = 999, R2=0.13, p=0.14, n=12).
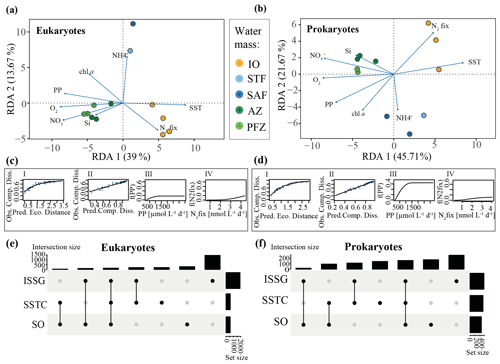
Figure 4(a) Eukaryotic and (b) prokaryotic community structures of different water masses measured during the MD206 cruise. Redundancy analysis (RDA) of 18S and 16S rRNA gene ASV tables as response variables and environmental metadata as explanatory variables; environmental metadata are represented as arrows. Constrained analyses were performed by water mass. There were significant relationships between water masses and community dissimilarities (PERMANOVA, 999 permutations; p<0.001, R2=0.67 for eukaryotes and p<0.001, R2=0.74 for prokaryotes). Colors indicate major water masses according to the legend: yellow bar highlights the Indian South Subtropical Gyre (ISSG), light blue bar highlights the Subtropical Front (STF), blue bar highlights the Subantarctic Front (SAF), dark green bar highlights the Polar Front Zone (PFZ), and light green bar highlights the Antarctic Zone (AZ). Eukaryotic (c) and prokaryotic (d) general dissimilarity model (GDM) with (I) observed compositional dissimilarity against predicted ecological distance, calculated from temperature + dissolved oxygen + NO + NH + Si + chl a + PP + N2 fixation; (II) observed compositional dissimilarity against predicted compositional dissimilarity to test the model fit; and contribution of (III) PP and (IV) N2 fixation to community dissimilarity expressed as a function of the environmental parameter (f(PP) and f(N2fix), respectively). For all functional plots of environmental data of the GDM analysis, see Fig. S11. Eukaryotic (e) and prokaryotic (f) UpSet plots of ASV intersections between Longhurst provinces. Analyses are based on binary tables (presence or absence) and the sum of all ASVs found across samples within one province. Intersection size shows number of ASVs shared between provinces (black dots, associated) and ASVs only found in one province (only black dot). Set size shows number of ASVs found in a specific Longhurst province.
Investigating whether and at which magnitude environmental parameters have an effect on microbial community dissimilarity, our general dissimilarity model (GDM) showed the expected curvilinear relationship between the predicted ecological distance and community dissimilarity (Fig. 4c I). Based on I-spline magnitudes of all tested environmental variables, geographic distance had little effect on community dissimilarity (Fig. S11a). Community dissimilarity changed most notably in response to variability in low magnitudes of PP (i.e., ISSG and STF; 17 % of total community dissimilarity, n=12) and plateaued with PP above 1100 µmol C L−1 d−1 (Fig. 4c III). A community dissimilarity change occurred most notably when N2 fixation rates were above 2 nmol N L−1 d−1 (∼ 19 % of change in total community dissimilarity associated with changes in N2 fixation rates) (Fig. 4c IV). Among all tested environmental parameters, our I-spline results showed that community dissimilarity increased most in response to variability in MLD and PO concentrations (49 % of change in total community dissimilarity associated with MLD variability and 63 % with PO variability, n=12; Fig. S11a).
Significant differences in community dissimilarity structure between Longhurst provinces were associated with high-pseudocount taxa, dominated by dinoflagellates (Dinophyceae) and diatoms (Bacillariophyta; SIMPER analysis; Table S6). The pseudocount of ASVs belonging to the phylum Ochrophyta (Bacillariophyta_X) contributed to differences between ocean provinces (contributing to at least 9.51 % of the differences in community dissimilarity between the SO and ISSG). Moreover, 4.79 % of the differences in community dissimilarity between the SO and the SSTC were associated with a higher ASV count of Bacillariophyta_X ASVs in the SO. Further, we identified 10 ASVs belonging to the phylum Dinophyceae, contributing 2.1 % to the community dissimilarity structure between the SO and ISSG and 5.79 % to the community dissimilarity structure between the SSTC and ISSG. This was further supported by relatively high concentrations of the photosynthetic pigments chl c3 and peridinin (both indicative pigments for dinoflagellates) in the SO and SAF. We found a relatively high number of ASV94 and ASV23 (Chloroparvula pacifica) in the SSTC, contributing 3.07 % to the community dissimilarity between the SSTC and the ISSG.
3.6 Prokaryotic community composition
From each of the 14 stations, a fragment of the small subunit ribosomal RNA gene (16S rRNA) was amplified and sequenced to obtain insights into the diversity and community composition of prokaryotes. A total of 1308 ASVs were recovered from which we removed 267 ASVs annotated as chloroplasts and 68 ASVs annotated as mitochondria. Prokaryotic richness increased with increasing sea surface temperature (Pearson correlation: r=0.65, p value = 0.03, n=11; Fig. S7a). Maximum alpha diversity (inverse Simpson) estimate was found in the SAF (81.92, station 15; Fig. S7d). Prokaryotic alpha diversity (inverse Simpson) was positively (but not significantly) linked to primary productivity (r=0.36, p=0.55, n=11; Fig. S7f) but showed a significant negative correlation with N2 fixation (, p=0.05, n=11; Fig. S7h).
Our RDA of the prokaryotic ASV table captured 90 % of the total variance with a p value of 0.06 (permutations = 999, n=11). Sites clustered into Longhurst provinces along the first two RDA axes (62.48 % of variance constrained; Fig. 4b). This was also shown in the PERMANOVA solution for Longhurst provinces (permutations = 999, R2=0.62, p<0.001, n=11) and our PERMANOVA grouping into water masses (permutations = 999, R2=0.74, p<0.001, n=11; Fig. 4b). We found more ASVs occurring in either the ISSG or the SO provinces rather than across all provinces (Fig. 4f). Further, the ISSG and the SO shared the least ASVs (Fig. 4f). In the RDA, sites within the ISSG province were positively associated with SST and N2 fixation. Sites belonging to the SO were positively associated with dissolved inorganic nutrients (NO, PO, Si), dissolved oxygen, and chl a concentrations as well as high PP (Fig. 4b). The community composition within the SSTC (STF and SAF) was distinct from that of the ISSG and SO along the second RDA axis (21.67 % variance constrained) and positively associated with NH concentrations (Fig. 4b). Linear relationships between beta diversity and rates were weak for PP (PERMANOVA; permutations = 999, R2=0.31, p=0.007, n=11) and N2 fixation (PERMANOVA; permutations = 999, R2=0.2, p=0.05, n=11).
Investigating whether and at which magnitude environmental parameters have an effect on prokaryotic microbial community dissimilarity, our general dissimilarity model (GDM) showed the expected curvilinear relationship (Fig. 4d I). Based on I-spline magnitude, geographic distance had little effect on community dissimilarity. The largest magnitude in community dissimilarity could be observed between 190–1200 µmol C L−1 d−1 (Fig. 4d III). Community dissimilarity changed most notably in response to variability in low magnitudes of N2 fixation and did not change in samples with highest average N2 fixation measurements (2.8 nmol N L−1 d−1 station 3, and 4.0 nmol N L−1 d−1 station 18). Largest magnitudes of community dissimilarity were associated with dissolved oxygen concentrations (Fig. S11b).
Taxonomically, based on analysis of the CLR-transformed ASV table, the prokaryotic community was dominated by Proteobacteria, Cyanobacteria, and Bacteroidetes, which are all typical clades for surface water samples (e.g., Biers et al., 2009). The greatest community differences occurred between stations of the Southern Ocean (SO) and the Indian South Subtropical Gyre (ISSG) provinces. Structure in community dissimilarity between the ISSG and SO were mostly associated with the number of Flavobacteriaceae (11.52 % of total community dissimilarity, SIMPER analysis, Table S6) and Planktomarina (Alphaproteobacteria) (5.69 % of the total difference in community dissimilarity, SIMPER analysis, Table S6). Further, the SO had distinct ASVs belonging to the SUP-05 cluster, contributing 2.56 % (ASV_12) to the difference between SO and SSTC. The ISSG was characterized by a high number of Cyanobacteria and some Actinobacteria. The cyanobacterial fraction was dominated by Prochlorococcus and Synechococcus.
Within the class level, all stations were dominated by Alpha- and Gammaproteobacteria, Bacteroidia, Oxyphotobacteria (Cyanobacteria), and Verrucomicrobia. Within the Alphaproteobacteria, we found a great dominance of ecotypes I, II, and IV of SAR11 clade throughout all samples (Table S4). The relative number of pseudocounts of bacteria belonging to the phylum Bacteroidetes decreased towards warmer SST in the ISSG, with significant differences between the SO and ISSG (Welch two-sample t test t=4.58, p<0.001, n1 = 341, n2 = 151). The phylum Bacteroidetes was largely dominated by the order Flavobacteriales (90.98 % of annotated ASVs). Cyanobacteria mainly occurred in the SSTC and in the ISSG, which were dominated by Prochlorococcus in the ISSG and Synechococcus in the SSTC. Cyanobacterial pseudocounts were significantly lower in the SO in comparison to the SSTC (Welch two-sample t test, , p value < 0.001, n1 = 17, n2 = 31) and to the ISSG (Welch two-sample t test, , p<0.001, n1 = 17, n2 = 45). Atelocyanobacteria (UCYN-A) ASVs occurred in the SAF (station 14) and ISSG (stations 2, 3).
Each water mass in our study had a distinct microbial fingerprint, including unique communities in frontal regions. We highlight clear relationships between microbial diversity, primary productivity, and N2 fixation (high linear and nonlinear covariability) in the southern Indian Ocean Gyre (ISSG), the Southern Ocean (SO), and their frontal transition zone. Below, we discuss how this clear provincialism of microbial diversity is disconnected from regional gradients in primary productivity (PP) and N2 fixation across our transect. This could suggest that microbial phylogenetic diversity is more strongly bounded by physical oceanographic boundaries, while microbial activity (and thus, perhaps, their functional diversity, not assessed here) responds more to chemical properties that changed more gradually between the low- and high-nutrient provinces we sampled.
4.1 N2 fixation and associated microbial diversity display distinct regional variations
Overall, our N2 fixation (up to 4.4 ± 2.5 nmol N L−1 d−1) was comparable to N2 fixation measured by González et al. (2014) above the Kerguelen Plateau (up to 10.27 ± 7.5 nmol N L−1 d−1) and showed a similar latitudinal trend as N2 fixation further east in the Indian Ocean, although with around 10-fold lower absolute rates (0.8–7 vs. 34–113 nmol N L−1 d−1; Raes et al., 2014). We note that the localized rates reported by González et al. (2014) are to date the only published N2 fixation measurements in this region, likely to be close to the annual maxima because of high irradiance; however, further investigations across seasonal changes within the study area are needed to confirm our observations. Our regional data are therefore important in closing the gaps in N2 fixation measurements in the Southern Ocean, especially considering that large disagreements exist between models of high-latitude N2 fixation rates (Tang et al., 2019).
N2 fixation measurements often show high basin-wide variability as well as high variability between samples at the same site, being sensitive to details of experimental design, incubation, and sea-state conditions (Mohr et al., 2010). In aggregate, these issues are best accounted for by calculating the minimum quantifiable rate (MQR; Gradoville et al., 2017). We observed high heterogeneity of biological samples taken from the underway flow-through system 5 min apart (separated by ∼ 15 km) within the same water mass. Similar variability in absolute measurements of N2 fixation (2.6–10.3 nmol N L−1 d−1 ± 7.5 nmol N L−1 d−1) were reported by González et al. (2014) close to our sampling site around Kerguelen island. This could imply a sub-mesoscale variability or influence of other unmeasured parameters.
As oligotrophic gyres extend and displace southwards under climate change (Yang et al., 2020), the biogeochemical and physical characteristics of the SO are changing (Caldeira and Wickett, 2005; Swart et al., 2018), and biological regional N2 fixation might become an important N source for productivity. Our data showed maximal N2 fixation in the oligotrophic waters of the ISSG; however, notably, measurable N2 fixation occurred well into the SO, to 56∘ S, suggesting that N2 fixation contributes to the regional N pool, despite other available sources of N (Shiozaki et al., 2018; Sipler et al., 2017). Similarly, we found a negative N* in the SO, which potentially indicates a P excess supporting N2 fixation (Knapp, 2012). Noteworthy is a slight increase in N2 fixation in the Antarctic Zone (AZ). High-latitude measurements in northern polar regions (Bering Sea) reached 10–11 nmol N L−1 d−1 (Shiozaki et al., 2017), substantially higher than our measurements of the SO (0.8–1.9 nmol N L−1 d−1), potentially supported by the close proximity to the coast or other factors such as day length, seasonality, diazotroph community, or trace metal concentrations.
Our results suggest that regional N2 fixation was not limited by the presence of other sources of bioavailable N (Fig. S10); this is a conclusion also reached in a number of studies including culture experiments (Boatman et al., 2018; Eichner et al., 2014; Knapp, 2012), as well as in situ measurements in the South Pacific (Halm et al., 2012); off the coast of Chile and Peru with rates up to 190 µmol N m−2 d−2 (Fernandez et al., 2011); and across the eastern Indian Ocean (Raes et al., 2015). This evidence counters the hypothesis of Breitbarth et al. (2007) that N2 fixation occurs only when other sources of N are limited. The contribution of N2 fixation to the N pool – and thus to productivity – varies strongly with ecosystem structure: in the SO, despite the local N2-fixation measurements, N2 fixation remains likely a very minor contributor to the N required by the microbial community for primary productivity.
Our results also strongly suggest that prokaryotic community structure and composition (beta diversity) were strongly impacted by the presence of biological N2 fixation, which is itself a prokaryotic process (Karl et al., 2002). For example, the N2-fixing Atelocyanobacteria (UCYN-A) occurred in the SAF and ISSG; however, to gain a clear insight into the community and N2 fixation, the diazotrophic community would need to be further resolved by amplicon analysis of functional (nifH) genes (Luo et al., 2012) as shown in other high-latitude studies (Fernández-Méndez et al., 2016; Raes et al., 2020).
4.2 Total and specific primary productivity differentially affect microbial diversity
We found PP was highest in the PFZ and decreased towards higher latitudes in the SO (Fig. 3a). Strass et al. (2002) showed that frontal maxima of PP are expected, and the observed decrease was probably due to Fe limitation in the SO (Blain et al., 2008). Primary productivity can also be limited by Si concentration and light availability when the mixed layer deepens (Boyd et al., 2000), but in our data Si concentrations were high in the surface water samples, and light levels were close to maximum in austral summer. The measured maximum PP above the Kerguelen Plateau (station E) was likely stimulated by Fe inputs (Blain et al., 2007).
Our results did not support prior observations that frontal regions (SAF and STF) supported higher specific primary productivity (PB) (as reported in the Antarctic Atlantic sector; Laubscher et al., 1993). While phytoplankton community composition, phytoplankton size distribution, and nutrient concentrations were strikingly different between the ISSG and SO, we found little difference in PB, with some slightly lower values observed within the SSTC (Fig. 3b). Differences in PB usually arise from physiological changes due to variabilities in irradiance (Geider, 1987), nutrient concentrations (Behrenfeld et al., 2008; Chalup and Laws, 1990), or differences in phytoplankton community structure, where cyanobacteria have the highest PP efficiency and diatoms the lowest (Talaber et al., 2018). Thus, our observations suggest that either (1) there is a lack of selective pressure on photosynthetic efficiency between provinces or (2) mechanisms driving PB are different between provinces, and the sum of beneficial (e.g., increased nutrient concentrations in the SO) and detrimental mechanisms (e.g., low irradiance and photoinhibition through deep vertical mixing, reported from the Antarctic circumpolar current (ACC); Alderkamp et al., 2011) result in similar PB. The slight variation around the frontal system is hard to interpret, as the complex interplay between factors may result in stochasticity.
Primary productivity can be an important driver for (phylogenetic) microbial alpha diversity (Vallina et al., 2014), especially within ocean provinces (Raes et al., 2018). While our observational study only has a small number of samples within and between oceanic provinces (n=12, nISSG=4, nSSTC=3, nSO=4), it did suggest that further validation of this assumption is needed. We observed that PP changed gradually across the sampling region and that local variability in PP was high between samples taken ∼ 15 km apart within the SSTC and SO (Fig. 3a). These local variabilities can arise from complex physico-chemical interactions between the STF, SAF, and SO (Mongin et al., 2008). Counter to Vallina et al. (2014) and Raes et al. (2018), we found a significant negative correlation between eukaryotic alpha diversity and PP within the ISSG. Further, we found no correlation between eukaryotic diversity and PP within the SSTC and SO and none between prokaryotic alpha diversity across all provinces (Fig. S7).
In terms of beta diversity, we observed a structuring effect of PP for pigment, 16S rRNA gene, and 18S rRNA gene-derived diversity profiles (Figs. 4a, b, S5). Pigment analysis revealed that photosynthetic prokaryotic diversity is strongly impacted by the relative abundance of Prochlorococcus, which does not generally occur in cold high-latitude waters (>40∘ S/N; Fig. S5) (Partensky et al., 1999) and, if so, only in low abundance (reviewed in Wilkins et al., 2013). Our 16S rRNA gene analyses confirm these observations showing that (1) picoplankton – and specifically Prochlorococcus – had relatively high proportions in the ISSG but very low in the SSTC, (2) Synechococcus dominated the Cyanobacterial fraction in the SSTC, and (3) both Prochlorococcus and Synechococcus were not detected in the SO (Tables S4, S6). In the SSTC and SO, phytoplankton communities had high proportions of dinoflagellates (Dinophyceae) and diatoms (Bacillariophyta) (up to 74 % of diatom diagnostic pigment concentrations), which are known as essential contributors to marine PP and microbial diversity (Malviya et al., 2016) and known to dominate the phytoplankton fraction within the Polar Frontal Zone (PFZ), especially as the blooming season progresses (Brown and Landry, 2001).
Further, our results show that phytoplankton community structure appears to be tightly coupled to the occurrence of specific heterotrophic organisms (Table S6) and thus may mediate an indirect effect of PP through microbial food webs (as also noted in, e.g., Sarmento and Gasol, 2012). For example, in areas of relatively high diatom concentrations, we found increased proportions of Flavobacteria. These bacteria specialize on successive decomposition of algal-derived organic matter (Teeling et al., 2012) and are known associates of diatoms (Pinhassi et al., 2004). Further, Planktomarina belonging to the Roseobacter clade affiliated (RCA) subgroup had relatively high proportions in the SO and is generally suggested to occur in colder environments (Giebel et al., 2009) and previously detected in the Polar Front (Wilkins et al., 2013b). The RCA subgroup is known for dimethylsulfoniopropionate (DMSP) degradation in phytoplankton blooms (Han et al., 2020). In addition to bacteria known to be associated with phytoplankton, we also observed those which symbiose with other organisms (e.g., Georgieva et al., 2020), such as the sulfur oxidizing Thioglobaceae (SUP-05 cluster), previously found in symbiosis with Myctophidae fish near Kerguelen Islands (Gallet et al., 2019). While beyond the scope of this study, we encourage further investigations of such trans-kingdom functional interactions as they themselves may offer regional insights.
4.3 Implications for microbial regionality
Microbial diversity was regionally constrained independent of geographical distance (GDM analysis), but it was partitioned into ocean provinces as repeatedly described for other ocean basins such as the Pacific (Raes et al., 2018) and the Atlantic Ocean (Milici et al., 2016). This supports the classical concept of microbial biogeography (Martiny et al., 2006). Further, we found that microbial beta diversity was even better resolved by individual water masses, highlighting the importance of including oceanographic boundaries that limit cross-front dispersal (Hanson et al., 2012; Hernando-Morales et al., 2017; Wilkins et al., 2013a).
Our beta diversity analysis confirmed the findings by Baltar and Arístegui (2017), who found unique environmental sorting and/or selection of microbial populations in the SAF and STF. Further, we were able to link these communities to high NH4 concentrations. This suggests high recycling of nitrogen sources within the microbial loop and potentially favoring nitrification in this area (Sambrotto and Mace, 2000). We also found increased dinoflagellate concentrations (PFT) which have been described to grow well under NH4 conditions (Townsend and Pettigrew, 1997). Despite our small sample size within the SAF and STF, we were able to detect these characteristics, supporting the call from Baltar et al. (2016) for better integrating frontal zones in our understanding of microbial biogeography.
Different trade-offs such as nutrient limitation and grazing can shape the microbial seascape (Acevedo-Trejos et al., 2018). In our study, the deviation between PN : chl a was large between the SO and IO with high PN : chl a ratios in the ISSG (Fig. S4), which has been used as an indicator of a relatively high abundance of heterotrophic microbes and protists over autotrophic organisms (Crawford et al., 2015; Hager et al., 1984; Waite et al., 2007). This would suggest that grazers formed a higher fraction of total biomass in the ISSG than in the SO. However, we did not measure zooplankton biomass or grazing rates, so this remains speculative.
Our study leads us to conclude that simultaneous assessment of microbial diversity, biogeochemical rates, and the physical partitioning of the ocean (provincialism) is central to the understanding of microbial oceanography.
Each water mass in our study had a distinct microbial fingerprint, including unique communities in frontal regions. Microbial alpha diversity and community dissimilarity correlated with biogeochemical rate measurements; however, mechanisms driving this association need further investigation through high-resolution sampling across spatial and temporal scales. Our results also indicate that high-latitude N2 fixation could meaningfully contribute to the global and regional N pool (as reported for Arctic N2 fixation by Sipler et al., 2017), which may become especially significant as global stratification (and concomitant restrictions in deep water replenishment of nutrients) intensifies.
While our sampling is too limited to conclude the point, our observations that phylogenetic diversity is constrained by hydrographic properties and province boundaries but that biogeochemical rates and nutrient concentrations are changing more gradually suggest that trans-province functional redundancy is present despite strong biogeographic separation in phylogenetic terms. As an outlook, we therefore encourage examining both phylogenetic and functional diversity to assess how functional groups and guilds contribute to the major biogeochemical (C, N) cycles across provinces and other biogeographic regions. Coordinated studies across ocean provinces are key to establishing the baselines we need to monitor the rapidly changing properties of the southern high latitudes in the face of rising temperature, acidification, and perturbations in regional currents.
All code is available under S1_code_archive.zip in the Supplement and additionally publicly archived under https://doi.org/10.5281/zenodo.5000001 (Hörstmann, 2021).
All HPLC data; environmental and rate measurement data, including PN, MIMS data, PP, and N2 fixation; and minimum quantification rate calculations are stored in the PANGAEA database (Hörstmann et al., 2018). All sequences are archived in the European Nucleotide Archive (primary accession: PRJEB29488).
The supplement related to this article is available online at: https://doi.org/10.5194/bg-18-3733-2021-supplement.
CH did the post-voyage processing and analysis of all samples and wrote the article. EJR conducted the fieldwork, designed the experiments, and contributed to data analysis and writing of the article. PLB contributed to data analysis, ecological interpretation, and writing of the article. CLM provided the historic physical and chemical data and contributed to the write-up. UJ helped with the DNA sequencing and writing of the article. AMW contributed to the design of the experiments, data analysis, and writing of the article.
The authors declare that they have no conflict of interest.
We thank Nicolas Metzel as well as the captain and crew of the Marion Dufresne. We thank Gaute Lavik from the Max Planck Institute of Marine Microbiology in Bremen for the guidance and for allowing us to use membrane inlet mass spectrometry. We thank Stefan Neuhaus for his knowledge on the bioinformatics pipeline. We thank Vladimir Benes and his team from the Genomics Core Facility, European Molecular Biology Laboratory, Heidelberg, Germany, for their kind guidance and support with the 16S rRNA gene sequencing. We thank the Leibniz Institute on Aging (FLI) in Jena (Germany) for their support in 18S rRNA gene sequencing. We thank Allison Fong and Matthias Ullrich for their comments on this study.
The article processing charges for this open-access publication were covered by the Alfred Wegener Institute, Helmholtz Centre for Polar and Marine Research (AWI).
This paper was edited by Koji Suzuki and reviewed by two anonymous referees.
Acevedo-Trejos, E., Maran, E., and Merico, A.: Phytoplankton size diversity and ecosystem function relationships across oceanic regions, P. Roy. Soc. B-Biol. Sci., 285, 20180621, https://doi.org/10.1098/rspb.2018.0621, 2018.
Aiken, J., Pradhan, Y., Barlow, R., Lavender, S., Poulton, A., Holligan, P., and Hardman-mountford, N.: Phytoplankton pigments and functional types in the Atlantic Ocean: A decadal assessment, 1995–2005, Deep-Sea Res. Pt. II, 56, 899–917, https://doi.org/10.1016/j.dsr2.2008.09.017, 2009.
Albuquerque, R., Bode, A., González-Gordillo, J. I., Duarte, C. M., and Queiroga, H.: Trophic Structure of Neuston Across Tropical and Subtropical Oceanic Provinces Assessed With Stable Isotopes, Front. Mar. Sci., 7, 606088, https://doi.org/10.3389/fmars.2020.606088, 2021.
Alderkamp, A. C., Garcon, V., de Baar, H. J. W., and Arrigo, K. R.: Short-term photoacclimation effects on photoinhibition of phytoplankton in the Drake Passage (Southern Ocean), Deep-Sea Res. Pt. I, 58, 943–955, https://doi.org/10.1016/j.dsr.2011.07.001, 2011.
Anderson, M. J.: A new method for non-parametric multivariate analysis of variance, Austral Ecol., 26, 32–46, https://doi.org/10.1046/j.1442-9993.2001.01070.x, 2001.
Armstrong, F. A. J.: The determination of silicate in sea water, J. Mar. Biol. Assoc. UK, 30, 149–160, https://doi.org/10.1017/S0025315400012649, 1951.
Baltar, F. and Arístegui, J.: Fronts at the Surface Ocean Can Shape Distinct Regions of Microbial Activity and Community Assemblages Down to the Bathypelagic Zone: The Azores Front as a Case Study, Front. Mar. Sci., 4, 1–13, https://doi.org/10.3389/fmars.2017.00252, 2017.
Baltar, F., Currie, K., Stuck, E., Roosa, S., and Morales, S. E.: Oceanic fronts: Transition zones for bacterioplankton community composition, Environ. Microbiol. Rep., 8, 132–138, https://doi.org/10.1111/1758-2229.12362, 2016.
Behrenfeld, M. J., O'Malley, R. T., Siegel, D. A., McClain, C. R., Sarmiento, J. L., Feldman, G. C., Milligan, A. J., Falkowski, P. G., Letelier, R. M., and Boss, E. S.: Climate-driven trends in contemporary ocean productivity, Nature, 444, 752–755, https://doi.org/10.1038/nature05317, 2006.
Behrenfeld, M. J., Halsey, K. H., and Milligan, A. J.: Evolved physiological responses of phytoplankton to their integrated growth environment, Philos. T. Roy. Soc. B, 363, 2687–2703, https://doi.org/10.1098/rstb.2008.0019, 2008.
Belkin, I. M. and O'Reilly, J. E.: An algorithm for oceanic front detection in chlorophyll and SST satellite imagery, J. Marine Syst., 78, 319–326, https://doi.org/10.1016/j.jmarsys.2008.11.018, 2009.
Biers, E. J., Sun, S., and Howard, E. C.: Prokaryotic genomes and diversity in surface ocean waters: Interrogating the global ocean sampling metagenome, Appl. Environ. Microb., 75, 2221–2229, https://doi.org/10.1128/AEM.02118-08, 2009.
Blain, S., Quéguiner, B., Armand, L., Belviso, S., Bombled, B., Bopp, L., Bowie, A., Brunet, C., Brussaard, C., Carlotti, F., Christaki, U., Corbière, A., Durand, I., Ebersbach, F., Fuda, J.-L., Garcia, N., Gerringa, L., Griffiths, B., Guigue, C., Guillerm, C., Jacquet, S., Jeandel, C., Laan, P., Lefèvre, D., Lo Monaco, C., Malits, A., Mosseri, J., Obernosterer, I., Park, Y.-H., Picheral, M., Pondaven, P., Remenyi, T., Sandroni, V., Sarthou, G., Savoye, N., Scouarnec, L., Souhaut, M., Thuiller, D., Timmermans, K., Trull, T., Uitz, J., van Beek, P., Veldhuis, M., Vincent, D., Viollier, E., Vong, L., and Wagener, T.: Effect of natural iron fertilization on carbon sequestration in the Southern Ocean, Nature, 446, 1070–1074, https://doi.org/10.1038/nature05700, 2007.
Blain, S., Sarthou, G., and Laan, P.: Distribution of dissolved iron during the natural iron-fertilization experiment KEOPS (Kerguelen Plateau, Southern Ocean), Deep-Sea Res. Pt. II, 55, 594–605, https://doi.org/10.1016/j.dsr2.2007.12.028, 2008.
Boatman, T. G., Davey, P. A., Lawson, T., and Geider, R. J.: The physiological cost of diazotrophy for Trichodesmium erythraeum IMS101, PLoS One, 3, 1–24, 2018.
Bokulich, N. A., Kaehler, B. D., Rideout, J. R., Dillon, M., Bolyen, E., Knight, R., Huttley, G. A., and Gregory Caporaso, J.: Optimizing taxonomic classification of marker-gene amplicon sequences with QIIME 2's q2-feature-classifier plugin, Microbiome, 6, 1–17, https://doi.org/10.1186/s40168-018-0470-z, 2018.
Boyd, P. W., Watson, A. J., Law, C. S., Abraham, E. R., Trull, T., Murdoch, R., Bakker, D. C. E., Bowie, A. R., Buesseler, K. O., Chang, H., Charette, M., Croot, P., Downing, K., Frew, R., Gall, M., Hadfield, M., Hall, J., Harvey, M., Jameson, G., LaRoche, J., Liddicoat, M., Ling, R., Maldonado, M. T., McKay, R. M., Nodder, S., Pickmere, S., Pridmore, R., Rintoul, S., Safi, K., Sutton, P., Strzepek, R., Tanneberger, K., Turner, S., Waite, A., and Zeldis, J.: A mesoscale phytoplankton bloom in the polar Southern Ocean stimulated by iron fertilization, Nature, 407, 695–702, https://doi.org/10.1038/35037500, 2000.
Breitbarth, E., Oschlies, A., and LaRoche, J.: Physiological constraints on the global distribution of Trichodesmium – effect of temperature on diazotrophy, Biogeosciences, 4, 53–61, https://doi.org/10.5194/bg-4-53-2007, 2007.
Brown, S. L. and Landry, M. R.: Mesoscale variability in biological community structure and biomass in the Antarctic Polar Front region at 170∘ W during austral spring 1997, J. Geophys. Res.-Ocean., 106, 13917–13930, https://doi.org/10.1029/1999JC000188, 2001.
Caldeira, K. and Wickett, M.: Ocean model predictions of chemistry changes from carbon dioxide emissions to the atmosphere and ocean, J. Geophys. Res.-Ocean., 110, 1–12, https://doi.org/10.1029/2004JC002671, 2005.
Callahan, B. J., McMurdie P. J., Rosen, M. J., Han, A. W., Johnson, A. J. A., and Holmes, S. P.: DADA2: High resolution sample inference from Illumina amplicon data, Nat. Methods, 13, 581–583, https://doi.org/10.1038/nmeth.3869, 2016.
Camacho, C., Coulouris, G., Avagyan, V., Ma, N., Papadopoulos, J., Bealer, K., and Madden, T. L.: BLAST +: architecture and applications, BMC Bioinformatics, 9, 1–9, https://doi.org/10.1186/1471-2105-10-421, 2009.
Chalup, M. S. and Laws, E. A.: A test of the assumptions and predictions of recent microalgal growth models with the marine phytoplankter Pavlova lutheri, Limnol. Oceanogr., 35, 583–596, https://doi.org/10.4319/lo.1990.35.3.0583, 1990.
Chao, A., Gotelli, N. J., Hsieh, T. C., Sander, E. L., Colwell, R. K., and Ellison, A. M.: Rarefaction and Extrapolation with Hill Numbers: A Framework for Sampling and Estimation in Species Diversity Studies, Ecol. Monogr., 84, 45–67, https://doi.org/10.1890/13-0133.1, 2014.
Chapman, C. C., Lea, M. A., Meyer, A., Sallée, J. B., and Hindell, M.: Defining Southern Ocean fronts and their influence on biological and physical processes in a changing climate, Nat. Clim. Change, 10, 209–219, https://doi.org/10.1038/s41558-020-0705-4, 2020.
Constable, A. J., Melbourne-Thomas, J., Corney, S. P., Arrigo, K. R., Barbraud, C., Barnes, D. K. A., Bindoff, N. L., Boyd, P. W., Brandt, A., Costa, D. P., Davidson, A. T., Ducklow, H. W., Emmerson, L., Fukuchi, M., Gutt, J., Hindell, M. A., Hofmann, E. E., Hosie, G. W., Iida, T., Jacob, S., Johnston, N. M., Kawaguchi, S., Kokubun, N., Koubbi, P., Lea, M. A., Makhado, A., Massom, R. A., Meiners, K., Meredith, M. P., Murphy, E. J., Nicol, S., Reid, K., Richerson, K., Riddle, M. J., Rintoul, S. R., Smith, W. O., Southwell, C., Stark, J. S., Sumner, M., Swadling, K. M., Takahashi, K. T., Trathan, P. N., Welsford, D. C., Weimerskirch, H., Westwood, K. J., Wienecke, B. C., Wolf-Gladrow, D., Wright, S. W., Xavier, J. C. and Ziegler, P.: Climate change and Southern Ocean ecosystems I: How changes in physical habitats directly affect marine biota, Glob. Change Biol., 20, 3004–3025, https://doi.org/10.1111/gcb.12623, 2014.
Conway, J. R., Lex, A., and Gehlenborg, N.: UpSetR: An R package for the visualization of intersecting sets and their properties, Bioinformatics, 33, 2938–2940, https://doi.org/10.1093/bioinformatics/btx364, 2017.
Crawford, D. W., Wyatt, S. N., Wrohan, I. A., Cefarelli, A. O., Giesbrecht, K. E., Kelly, B., and Varela, D. E.: Low particulate carbon to nitrogen ratios in marine surface waters of the Arctic, Global Biogeochem. Cy., 29, 2021–2033, https://doi.org/10.1002/2015GB005200, 2015.
Eichner, M., Kranz, S. A., and Rost, B.: Combined effects of different CO2 levels and N sources on the diazotrophic cyanobacterium Trichodesmium, Physiol. Plant., 152, 316–330, https://doi.org/10.1111/ppl.12172, 2014.
Evans, C., Thomson, P. G., Davidson, A. T., Bowie, A. R., van den Enden, R., Witte, H., and Brussaard, C. P. D.: Potential climate change impacts on microbial distribution and carbon cycling in the Australian Southern Ocean, Deep-Sea Res. Pt. II, 58, 2150–2161, https://doi.org/10.1016/j.dsr2.2011.05.019, 2011.
Fernández-Méndez, M., Turk-Kubo, K. A., Buttigieg, P. L., Rapp, J. Z., Krumpen, T., Zehr, J. P., and Boetius, A.: Diazotroph diversity in the sea ice, melt ponds, and surface waters of the eurasian basin of the Central Arctic Ocean, Front. Microbiol., 7, 1–18, https://doi.org/10.3389/fmicb.2016.01884, 2016.
Fernandez, C., Farías, L., and Ulloa, O.: Nitrogen fixation in denitrified marine waters, PLoS One, 6, 20539, https://doi.org/10.1371/journal.pone.0020539, 2011.
Gallet, A., Koubbi, P., Léger, N., Scheifler, M., Ruiz-Rodriguez, M., Suzuki, M. T., Desdevises, Y., and Duperron, S.: Low-diversity bacterial microbiota in Southern Ocean representatives of lanternfish genera Electrona, Protomyctophum and Gymnoscopelus (family Myctophidae), PLoS One, 14, 1–17, https://doi.org/10.1371/journal.pone.0226159, 2019.
Geider, R. J.: Light and Temperature Dependence of the Carbon to Chlorophyll a Ratio in Microalgae and Cyanobacteria: Implications for Physiology and Growth of Phytoplankton, New Phytol., 106, 1–34, https://doi.org/10.1111/j.1469-8137.1987.tb04788.x, 1987.
Georgieva, M. N., Taboada, S., Riesgo, A., Díez-Vives, C., De Leo, F. C., Jeffreys, R. M., Copley, J. T., Little, C. T. S., Ríos, P., Cristobo, J., Hestetun, J. T., and Glover, A. G.: Evidence of Vent-Adaptation in Sponges Living at the Periphery of Hydrothermal Vent Environments: Ecological and Evolutionary Implications, Front. Microbiol., 11, 1636, https://doi.org/10.3389/fmicb.2020.01636, 2020.
Giebel, H. A., Brinkhoff, T., Zwisler, W., Selje, N., and Simon, M.: Distribution of Roseobacter RCA and SAR11 lineages and distinct bacterial communities from the subtropics to the Southern Ocean, Environ. Microbiol., 11, 2164–2178, https://doi.org/10.1111/j.1462-2920.2009.01942.x, 2009.
Gloor, G. B., Macklaim, J. M., Pawlowsky-Glahn, V., and Egozcue, J. J.: Microbiome datasets are compositional: And this is not optional, Front. Microbiol., 8, 1–6, https://doi.org/10.3389/fmicb.2017.02224, 2017.
González, M. L., Molina, V., Florez-Leiva, L., Oriol, L., Cavagna, A. J., Dehairs, F., Farias, L., and Fernandez, C.: Nitrogen fixation in the Southern Ocean: a case of study of the Fe-fertilized Kerguelen region (KEOPS II cruise), Biogeosciences Discuss., 11, 17151–17185, https://doi.org/10.5194/bgd-11-17151-2014, 2014.
Gradoville, M. R., Bombar, D., Crump, B. C., Letelier, R. M., Zehr, J. P., and White, A. E.: Diversity and activity of nitrogen-fixing communities across ocean basins, Limnol. Oceanogr., 62, 1895–1909, https://doi.org/10.1002/lno.10542, 2017.
Guillou, L., Bachar, D., Audic, S., Bass, D., Berney, C., Bittner, L., Boutte, C., Burgaud, G., De Vargas, C., Decelle, J., Del Campo, J., Dolan, J. R., Dunthorn, M., Edvardsen, B., Holzmann, M., Kooistra, W. H. C. F., Lara, E., Le Bescot, N., Logares, R., Mahé, F., Massana, R., Montresor, M., Morard, R., Not, F., Pawlowski, J., Probert, I., Sauvadet, A. L., Siano, R., Stoeck, T., Vaulot, D., Zimmermann, P., and Christen, R.: The Protist Ribosomal Reference database (PR2): A catalog of unicellular eukaryote Small Sub-Unit rRNA sequences with curated taxonomy, Nucleic Acids Res., 41, 597–604, https://doi.org/10.1093/nar/gks1160, 2013.
Hager, S. W., Harmon, D. D., and Alpine, A. E.: Chemical Determination of Particulate Nitrogen in San Francisco Bay. Nitrogen Chlorophyll a Ratios in Plankton, Estuar. Coast. Shelf S., 19, 193–204, https://doi.org/10.1016/0272-7714(84)90064-7, 1984.
Halm, H., Lam, P., Ferdelman, T. G., Lavik, G., Dittmar, T., Laroche, J., D'Hondt, S., and Kuypers, M. M. M.: Heterotrophic organisms dominate nitrogen fixation in the south pacific gyre, ISME J., 6, 1238–1249, https://doi.org/10.1038/ismej.2011.182, 2012.
Han, D., Kang, H. Y., Kang, C. K., Unno, T., and Hur, H. G.: Seasonal Mixing-Driven System in Estuarine–Coastal Zone Triggers an Ecological Shift in Bacterial Assemblages Involved in Phytoplankton-Derived DMSP Degradation, Microb. Ecol., 79, 12–20, https://doi.org/10.1007/s00248-019-01392-w, 2020.
Hanson, C. A., Fuhrman, J. A., Horner-Devine, M. C., and Martiny, J. B. H.: Beyond biogeographic patterns: Processes shaping the microbial landscape, Nat. Rev. Microbiol., 10, 497–506, https://doi.org/10.1038/nrmicro2795, 2012.
Hernando-Morales, V., Ameneiro, J., and Teira, E.: Water mass mixing shapes bacterial biogeography in a highly hydrodynamic region of the Southern Ocean, Environ. Microbiol., 19, 1017–1029, https://doi.org/10.1111/1462-2920.13538, 2017.
Hirata, T., Hardman-Mountford, N. J., Brewin, R. J. W., Aiken, J., Barlow, R., Suzuki, K., Isada, T., Howell, E., Hashioka, T., Noguchi-Aita, M., and Yamanaka, Y.: Synoptic relationships between surface Chlorophyll-a and diagnostic pigments specific to phytoplankton functional types, Biogeosciences, 8, 311–327, https://doi.org/10.5194/bg-8-311-2011, 2011.
Hörstmann, C.: CoraHoerstmann/MD206_Microbes: (Version 2.0), Zenodo, https://doi.org/10.5281/zenodo.5000001, 2021.
Hörstmann, C., Raes, E. J., and Waite, A. M.: Nitrogen and carbon processes in the South Indian Ocean and the French Southern and Antarctic Lands, PANGAEA, https://doi.org/10.1594/PANGAEA.885896, 2018.
Holm, S.: Board of the Foundation of the Scandinavian Journal of Statistics, Scand. J. Stat., 6, 65–70, 1979.
Karl, D., Michaels, A., Bergman, B., Capone, D. G., Carpenter, E. J., Letelier, R., Lipschultz, F., Paerl, H., Sigman, D., and Stal, L.: Dinitrogen fixation in the world's oceans, Biogeochemistry, 57–58, 47–98, https://doi.org/10.1023/A:1015798105851, 2002.
Kérouel, R. and Aminot, A.: Fluorometric determination of ammonia in sea and estuarine waters by direct segmented flow analysis, Mar. Chem., 57, 265–275, https://doi.org/10.1016/S0304-4203(97)00040-6, 1997.
Kilias, E., Wolf, C., Nöthig, E. M., Peeken, I., and Metfies, K.: Protist distribution in the Western Fram Strait in summer 2010 based on 454-pyrosequencing of 18S rDNA, J. Phycol., 49, 996–1010, https://doi.org/10.1111/jpy.12109, 2013.
Klawonn, I., Lavik, G., Böning, P., Marchant, H. K., Dekaezemacker, J., Mohr, W., and Ploug, H.: Simple approach for the preparation of 15−15N2-enriched water for nitrogen fixation assessments: Evaluation, application and recommendations, Front. Microbiol., 6, 1–11, https://doi.org/10.3389/fmicb.2015.00769, 2015.
Knap, A., Michaels, A., Close, A., Ducklow, H., and Dickson, A.: Protocols for the Joint Global Ocean Flux Study (JGFOS) Core Measurements, JGOFS Reoprt Nr. 19, vi+170 pp., (Reprint of IOC MAnuals and Guides 29, UNESCO 1994), 198, http://hdl.handle.net/11329/220 (last access: 20 June 2021), 1996.
Knapp, A. N.: The sensitivity of marine N2 fixation to dissolved inorganic nitrogen, Front. Microbiol., 3, 1–14, https://doi.org/10.3389/fmicb.2012.00374, 2012.
Laubscher, R. K., Perissinotto, R., and McQuaid, C. D.: Phytoplankton Production and Biomass at Frontal Zones in the Atlantic Sector of the Southern-Ocean, Polar Biol., 13, 471–481, https://doi.org/10.1007/BF00233138, 1993.
Le Fèvre, J.: Aspects of the Biology of Frontal Systems, in: Advances in Marine Biology, Vol. 23, edited by: Blaxter, J. H. S. and Southward, A. J., 163–299, Academic Press INC. (London) LTD, https://doi.org/10.1016/S0065-2881(08)60109-1, 1987.
Lo Monaco, C., Álvarez, M., Key, R. M., Lin, X., Tanhua, T., Tilbrook, B., Bakker, D. C. E., van Heuven, S., Hoppema, M., Metzl, N., Ríos, A. F., Sabine, C. L., and Velo, A.: Assessing the internal consistency of the CARINA database in the Indian sector of the Southern Ocean, Earth Syst. Sci. Data, 2, 51–70, https://doi.org/10.5194/essd-2-51-2010, 2010.
Longhurst, A.: Ecological Geography of the sea, 2nd Edn., Academic Press, London, 2007.
Luo, Y.-W., Doney, S. C., Anderson, L. A., Benavides, M., Berman-Frank, I., Bode, A., Bonnet, S., Boström, K. H., Böttjer, D., Capone, D. G., Carpenter, E. J., Chen, Y. L., Church, M. J., Dore, J. E., Falcón, L. I., Fernández, A., Foster, R. A., Furuya, K., Gómez, F., Gundersen, K., Hynes, A. M., Karl, D. M., Kitajima, S., Langlois, R. J., LaRoche, J., Letelier, R. M., Marañón, E., McGillicuddy Jr., D. J., Moisander, P. H., Moore, C. M., Mouriño-Carballido, B., Mulholland, M. R., Needoba, J. A., Orcutt, K. M., Poulton, A. J., Rahav, E., Raimbault, P., Rees, A. P., Riemann, L., Shiozaki, T., Subramaniam, A., Tyrrell, T., Turk-Kubo, K. A., Varela, M., Villareal, T. A., Webb, E. A., White, A. E., Wu, J., and Zehr, J. P.: Database of diazotrophs in global ocean: abundance, biomass and nitrogen fixation rates, Earth Syst. Sci. Data, 4, 47–73, https://doi.org/10.5194/essd-4-47-2012, 2012.
Malviya, S., Scalco, E., Audic, S., Vincent, F., Veluchamy, A., Poulain, J., Wincker, P., Iudicone, D., de Vargas, C., Bittner, L., Zingone, A., and Bowler, C.: Insights into global diatom distribution and diversity in the world's ocean, P. Natl. Acad. Sci. USA, 113, E1516–E1525, https://doi.org/10.1073/pnas.1509523113, 2016.
Martiny, J. B. H., Bohannan, B. J. M., Brown, J. H., Kane, M., Krumins, J. A., Kuske, C. R., Morin, P. J., Naeem, S., Øvreås, L., Reysenbach, A., and Smith, V. H.: Microbial biogeography: putting microorganisms on the map, Nature, 4, 102–112, https://doi.org/10.1038/nrmicro1341, 2006.
Metzl, N. and Lo Monaco, C.: VT 153/OISO-27 cruise, RV Marion Dufresne, https://doi.org/10.17600/17009700, 2017.
Milici, M., Tomasch, J., Wos-Oxley, M. L., Decelle, J., Jáuregui, R., Wang, H., Deng, Z. L., Plumeier, I., Giebel, H. A., Badewien, T. H., Wurst, M., Pieper, D. H., Simon, M., and Wagner-Döbler, I.: Bacterioplankton biogeography of the Atlantic ocean: A case study of the distance-decay relationship, Front. Microbiol., 7, 1–15, https://doi.org/10.3389/fmicb.2016.00590, 2016.
Mohr, W., Großkopf, T., Wallace, D. W. R., and LaRoche, J.: Methodological underestimation of oceanic nitrogen fixation rates, PLoS One, 5, 1–7, https://doi.org/10.1371/journal.pone.0012583, 2010.
Mongin, M., Molina, E., and Trull, T. W.: Seasonality and scale of the Kerguelen plateau phytoplankton bloom: A remote sensing and modeling analysis of the influence of natural iron fertilization in the Southern Ocean, Deep-Sea Res. Pt. II, 55, 880–892, https://doi.org/10.1016/j.dsr2.2007.12.039, 2008.
Montoya, J. P., Voss, M., Kahler, P., and Capone, D. G.: A Simple, High-Precision, High-Sensitivity Tracer Assay for N2 Fixation, Appl. Environ. Microb., 62, 986–993, https://doi.org/10.1128/AEM.62.3.986-993.1996, 1996.
Murphy, J. and Riley, J.: A modified single solution method for the determination of phosphate in natural waters, Anal. Chem. ACTA, 27, 31–36, https://doi.org/10.1016/S0003-2670(00)88444-5, 1962.
Padgham, M., Sumner, M. D., and Karney, C. F. F.: geodist R pacakge version 0.0.4, GitHub, https://github.com/hypertidy/geodist (last access: 20 June 2021), 2020.
Parada, A. E., Needham, D. M., and Fuhrman, J. A.: Every base matters: Assessing small subunit rRNA primers for marine microbiomes with mock communities, time series and global field samples, Environ. Microbiol., 18, 1403–1414, https://doi.org/10.1111/1462-2920.13023, 2016.
Partensky, F., Blanchot, J., and Vaulot, D.: Differential distribution and ecology of Prochlorococcus and Synechococcus in oceanic waters: a review, Bull. l'Institut océanographique, 19, 457–475, 1999.
Pinhassi, J., Sala, M. M., Havskum, H., Peters, F., Guadayol, Ò., Malits, A., and Marrasé, C.: Changes in bacterioplankton composition under different phytoplankton regimens, Appl. Environ. Microb., 70, 6753–6766, https://doi.org/10.1128/AEM.70.11.6753-6766.2004, 2004.
Pruesse, E., Peplies, J., Glöckner, F. O., Editor, A., and Wren, J.: SINA: Accurate high-throughput multiple sequence alignment of ribosomal RNA genes, 28, 1823–1829, https://doi.org/10.1093/bioinformatics/bts252, 2012.
Quast, C., Pruesse, E., Yilmaz, P., Gerken, J., Schweer, T., Glo, F. O., and Yarza, P.: The SILVA ribosomal RNA gene database project: improved data processing and web-based tools, Nucleic Acids Res., 41, D590–D596, https://doi.org/10.1093/nar/gks1219, 2013.
Raes, E. J., Waite, A. M., McInnes, A. S., Olsen, H., Nguyen, H. M., Hardman-Mountford, N., and Thompson, P. A.: Changes in latitude and dominant diazotrophic community alter N2 fixation, Mar. Ecol.-Prog. Ser., 516, 85–102, https://doi.org/10.3354/meps11009, 2014.
Raes, E. J., Thompson, P. A., McInnes, A. S., Nguyen, H. M., Hardman-mountford, N., and Waite, A. M.: Sources of new nitrogen in the Indian Ocean, Global Biogeochem. Cy., 935, 1283–1297, https://doi.org/10.1002/2015GB005194, 2015.
Raes, E. J., Bodrossy, L., Kamp, J. Van De, Bissett, A., Ostrowski, M., and Brown, M. V: Oceanographic boundaries constrain microbial diversity gradients in the South Pacific Ocean, P. Natl. Acad. Sci. USA, 115, E8266–E8275, https://doi.org/10.1073/pnas.1719335115, 2018.
Raes, E. J., Kamp, J. Van De, Bodrossy, L., Fong, A. A., Riekenberg, J., Holmes, B. H., Erler, D. V, Eyre, B. D., Weil, S., and Waite, A. M.: N 2 Fixation and New Insights Into Nitrification From the Ice-Edge to the Equator in the South Pacific Ocean, 7, 1–20, https://doi.org/10.3389/fmars.2020.00389, 2020.
Ras, J., Claustre, H., and Uitz, J.: Spatial variability of phytoplankton pigment distributions in the Subtropical South Pacific Ocean: comparison between in situ and predicted data, Biogeosciences, 5, 353–369, https://doi.org/10.5194/bg-5-353-2008, 2008.
R Core Team: R: A language and environment for statistical computing. R Foundation for Statistical Computing, Vienna, Austria, available at: https://www.R-project.org/ (last access: 20 June 2021), 2020.
Sambrotto, R. N. and Mace, B. J.: Coupling of biological and physical regimes across the Antarctic Polar Front as reflected by nitrogen production and recycling, Deep-Sea Res. Pt. II, 47, 3339–3367, https://doi.org/10.1016/S0967-0645(00)00071-0, 2000.
Sarmento, H. and Gasol, J. M.: Use of phytoplankton-derived dissolved organic carbon by different types of bacterioplankton, Environ. Microbiol., 14, 2348–2360, https://doi.org/10.1111/j.1462-2920.2012.02787.x, 2012.
Shiozaki, T., Bombar, D., Riemann, L., Hashihama, F., Takeda, S., Yamaguchi, T., Ehama, M., Hamasaki, K., and Furuya, K.: Basin scale variability of active diazotrophs and nitrogen fixation in the North Pacific, from the tropics to the subarctic Bering Sea, Global Biogeochem. Cy., 31, 996–1009, https://doi.org/10.1002/2017GB005681, 2017.
Shiozaki, T., Fujiwara, A., Ijichi, M., Harada, N., Nishino, S., Nishi, S., Nagata, T., and Hamasaki, K.: Diazotroph community structure and the role of nitrogen fixation in the nitrogen cycle in the Chukchi Sea (western Arctic Ocean), Limnol. Oceanogr., 63, 2191–2205, https://doi.org/10.1002/lno.10933, 2018.
Sipler, R. E., Gong, D., Baer, S. E., Sanderson, M. P., Roberts, Q. N., Mulholland, M. R., and Bronk, D. A.: Preliminary estimates of the contribution of Arctic nitrogen fixation to the global nitrogen budget, Limnol. Oceanogr. Lett., 159–166, https://doi.org/10.1002/lol2.10046, 2017.
Stoeck, T., Bass, D., Nebel, M., Christen, R., and Meredith, D.: Multiple marker parallel tag environmental DNA sequencing reveals a highly complex eukaryotic community in marine anoxic water, Mol. Ecol., 19, 21–31, https://doi.org/10.1111/j.1365-294X.2009.04480.x, 2010.
Strass, V. H., Naveira Garabato, A. C., Pollard, R. T., Fischer, H. I., Hense, I., Allen, J. T., Read, J. F., Leach, H., and Smetacek, V.: Mesoscale frontal dynamics: Shaping the environment of primary production in the Antarctic Circumpolar Current, Deep-Sea Res. Pt. II, 49, 3735–3769, https://doi.org/10.1016/S0967-0645(02)00109-1, 2002.
Swart, N. C., Gille, S. T., Fyfe, J. C., and Gillett, N. P.: Recent Southern Ocean warming and freshening driven by greenhouse gas emissions and ozone depletion, Nat. Geosci., 11, 836–841, https://doi.org/10.1038/s41561-018-0226-1, 2018.
Talaber, I., Francé, J., Flander-Putrle, V., and Mozetič, P.: Primary production and community structure of coastal phytoplankton in the Adriatic Sea: Insights on taxon-specific productivity, Mar. Ecol.-Prog. Ser., 604, 65–81, https://doi.org/10.3354/meps12721, 2018.
Tang, W., Li, Z., and Cassar, N.: Machine Learning Estimates of Global Marine Nitrogen Fixation, J. Geophys. Res.-Biogeo., 2012, 717–730, https://doi.org/10.1029/2018JG004828, 2019.
Teeling, H., Fuchs, B. M., Becher, D., Klockow, C., Gardebrecht, A., Bennke, C. M., Kassabgy, M., Huang, S., Mann, A. J., Waldmann, J., Weber, M., Klindworth, A., Otto, A., Lange, J., Bernhardt, J., Reinsch, C., Hecker, M., Peplies, J., Bockelmann, F. D., Callies, U., Gerdts, G., Wichels, A., Wiltshire, K. H., Glöckner, F. O., Schweder, T., and Amann, R.: Substrate-Controlled Succession of Marine Bacterioplankton Populations Induced by a Phytoplankton Bloom, Science, 336, 608–611, https://doi.org/10.1126/science.1218344, 2012.
Townsend, D. W. and Pettigrew, N. R.: Nitrogen limitation of secondary production on Georges Bank, J. Plankton Res., 19, 221–235, 1997.
Uitz, J., Claustre, H., Morel, A., and Hooker, S. B.: Vertical distribution of phytoplankton communities in open ocean: An assessment based on surface chlorophyll, J. Geophys. Res.-Ocean., 111, C08005, https://doi.org/10.1029/2005JC003207, 2006.
Vallina, S. M., Follows, M. J., Dutkiewicz, S., Montoya, J. M., Cermeno, P. and Loreau, M.: Global relationship between phytoplankton diversity and productivity in the ocean, Nat. Commun., 5, 1–10, https://doi.org/10.1038/ncomms5299, 2014.
Vidussi, F., Claustre, H., Manca, B. B., Luchetta, A., and Marty, J.-C.: Phytoplankton pigment distribution in relation to upper thermocline circulation in the eastern Mediterranean Sea during winter, J. Geophys. Res., 106, 939–956, https://doi.org/10.1029/1999JC000308, 2001.
Waite, A. M., Muhling, B. A., Holl, C. M., Beckley, L. E., Montoya, J. P., Strzelecki, J., Thompson, P. A., and Pesant, S.: Food web structure in two counter-rotating eddies based on δ15N and δ13C isotopic analyses, Deep-Sea Res. Pt. II, 54, 1055–1075, https://doi.org/10.1016/j.dsr2.2006.12.010, 2007.
Wilkins, D., Lauro, F. M., Williams, T. J., Demaere, M. Z., Brown, M. V., Hoffman, J. M., Andrews-Pfannkoch, C., Mcquaid, J. B., Riddle, M. J., Rintoul, S. R., and Cavicchioli, R.: Biogeographic partitioning of Southern Ocean microorganisms revealed by metagenomics, Environ. Microbiol., 15, 1318–1333, https://doi.org/10.1111/1462-2920.12035, 2013a.
Wilkins, D., Yau, S., Williams, T. J., Allen, M. A., Brown, M. V., Demaere, M. Z., Lauro, F. M., and Cavicchioli, R.: Key microbial drivers in Antarctic aquatic environments, FEMS Microbiol. Rev., 37, 303–335, https://doi.org/10.1111/1574-6976.12007, 2013b.
Wood, E. D., Armstrong, F. A. J., and Richards, F. A.: Determination of nitrate in sea water by cadmium-copper reduction to nitrite, J. Mar. Biol. Assoc. UK, 47, 23–31, https://doi.org/10.1017/S002531540003352X, 1967.
Yang, H., Lohmann, G., Krebs-Kanzow, U., Ionita, M., Shi, X., Sidorenko, D., Gong, X., Chen, X., and Gowan, E. J.: Poleward Shift of the Major Ocean Gyres Detected in a Warming Climate, Geophys. Res. Lett., 47, e2019GL085868, https://doi.org/10.1029/2019GL085868, 2020.
The requested paper has a corresponding corrigendum published. Please read the corrigendum first before downloading the article.
- Article
(1853 KB) - Full-text XML
- Corrigendum
-
Supplement
(12450 KB) - BibTeX
- EndNote