the Creative Commons Attribution 4.0 License.
the Creative Commons Attribution 4.0 License.
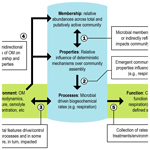
Disturbance triggers non-linear microbe–environment feedbacks
Sarah J. Fansler
Rosalie K. Chu
Robert E. Danczak
Vanessa A. Garayburu-Caruso
Lupita Renteria
Hyun-Seob Song
Jason Toyoda
Jacqueline Hager
James C. Stegen
Conceptual frameworks linking microbial community membership, properties, and processes with the environment and emergent function have been proposed but remain untested. Here we refine and test a recent conceptual framework using hyporheic zone sediments exposed to wetting–drying transitions. Our refined framework includes relationships between cumulative properties of a microbial community (e.g., microbial membership, community assembly properties, and biogeochemical rates), environmental features (e.g., organic matter thermodynamics), and emergent ecosystem function. Our primary aim was to evaluate the hypothesized relationships that comprise the conceptual framework and contrast outcomes from the whole and putatively active bacterial and archaeal communities. Throughout the system we found threshold-like responses to the duration of desiccation. Membership of the putatively active community – but not the whole bacterial and archaeal community – responded due to enhanced deterministic selection (an emergent community property). Concurrently, the thermodynamic properties of organic matter (OM) became less favorable for oxidation (an environmental component), and respiration decreased (a microbial process). While these responses were step functions of desiccation, we found that in deterministically assembled active communities, respiration was lower and thermodynamic properties of OM were less favorable. Placing the results in context of our conceptual framework points to previously unrecognized internal feedbacks that are initiated by disturbance and mediated by thermodynamics and that cause the impacts of disturbance to be dependent on the history of disturbance.
- Article
(1391 KB) - Full-text XML
-
Supplement
(597 KB) - BibTeX
- EndNote
1.1 Conceptual foundations
Given the influence of microbes over ecosystem function, deeper knowledge of microbe–environment relationships is needed to improve ecosystem models (Bier et al., 2015). In turn, there is strong interest in quantifying and predicting microbe–environment relationships such as defining microbial life history strategies as traits in ecosystem models (Malik et al., 2020), assessing microbial biomass stoichiometry distributions in response to changing resource environments (Manzella et al., 2019), and evaluating the extent of microbial adaptation to changing environments and their role in biogeochemical processes (Wallenstein and Hall, 2012). To enhance and synthesize understanding of microbe–environment interactions, it is useful to develop conceptual frameworks based on linkages among microbial characteristics and ecosystem processes. Previous work has used such frameworks to improve mechanistic representation and predictive capacity of microbe–environment interactions in ecosystem models (Wieder et al., 2015).
A recently developed framework by Hall et al. (2018) poses a series of concepts that collectively define the intersection between microbial and ecosystem ecology. Their framework draws attention to causal relationships between microbial characteristics (microbial membership influences community properties and microbial processes), which in turn regulate ecosystem fluxes. These components can be further modified by environmental variation, leading to cumulative ecosystem processes with the potential to incorporate relevant mechanistic links into predictive ecosystem models. Hall et al. (2018) particularly draws attention to the need to understand how microorganisms influence their environment by separating microbial community properties based on community-aggregated traits (those which can be predicted using constituent taxa) and emergent properties (properties unable to be explained by constituent taxa). A powerful element of the framework is that it applies to diverse systems spanning natural (Gilbert et al., 2018), host-associated (Lloyd-Price et al., 2019), and built (Fu et al., 2020) environments as well as across spatiotemporal scales (König et al., 2018). While potentially very useful, the Hall et al. (2018) framework has seen little direct use in terms of explicitly defining and evaluating the linkages within specific study systems (but see Manzella et al., 2019). To make full use of and continually improve the framework, it is necessary to consider different realizations and interpretations of the proposed linkages. In the following paragraphs we detail a modified interpretation of the framework (Fig. 1) to enable its application to microbial communities and biogeochemistry associated with hyporheic zone sediments experiencing hydrological disturbance. In turn, we use data from a controlled laboratory experiment to evaluate key linkages within the modified framework.
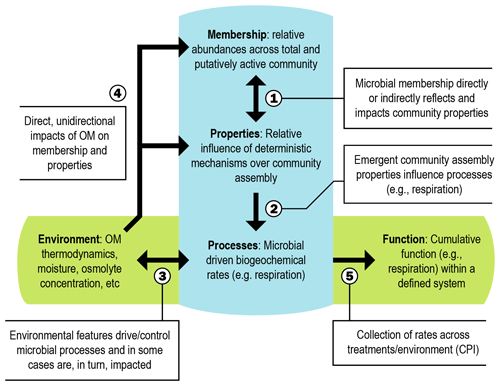
Figure 1Integrated conceptual framework. The conceptual figure (modified from Hall et al., 2018) details relationships (indicated by numbered arrows) between cumulative properties of the microbial community (e.g., microbial membership, community assembly properties, biogeochemical rates), environmental features (e.g., organic matter (OM) thermodynamics), and emergent ecosystem function (e.g., control point influence (CPI)). Double-headed arrows indicate feedbacks.
Our modified framework aims to refine the link between microbial communities and ecosystem functions. As detailed below, a couple critical elements of our modification include (i) defining and evaluating the relative influence of community assembly processes as an emergent community property and (ii) proposing bi-directional links between environment and microbial processes to indicate that environmental conditions drive microbial processes, which in turn influence the environment, and these cumulative environment-microbial processes ultimately drive ecosystem function.
1.2 Conceptual framework development
As in Hall et al. (2018) we consider microbial membership to be directly influenced by environmental conditions (arrow 4, Fig. 1) and to underlie community-level properties (arrow 1, Fig. 1). Determining microbial membership is relatively straightforward and uses culture-independent (Behrens et al., 2012; Norland et al., 1995; Thompson et al., 2017; Wagner, 2009) and culture-dependent (Bartelme et al., 2020) techniques. Sequence-based assays using phylogenetic markers are routine, with DNA-based (total community members) and RNA-based (putatively active community members) (Barnard et al., 2015; Blazewicz et al., 2013; Cardoso et al., 2017; Kearns et al., 2016; Shu et al., 2019; Wisnoski et al., 2020) approaches providing the foundation to study community properties.
While community membership is relatively straightforward, the identification of community properties that are relevant to a given system and function is open to broader interpretation. Here we propose using the relative influences of deterministic and stochastic community assembly processes (Stegen et al., 2012) as emergent properties of microbial communities that have implications for biogeochemical function (Graham and Stegen, 2017) including in ecosystems experiencing environmental disturbance. Deterministic mechanisms are associated with systematic differences in reproductive success imposed by the biotic and/or abiotic environment, while stochastic mechanisms are associated with passive spatial movements of organisms and birth and death events that are not due to systematic differences across taxa in reproductive success (Dini-Andreote et al., 2015; Stegen et al., 2015). The relative contributions of determinism and stochasticity can be inferred by coupling microbial community membership and phylogeny to ecological null models (Stegen et al., 2012, 2013, 2015; Zhou and Ning, 2017).
We propose that the relative contributions of determinism and stochasticity are emergent properties that are greater than the sum of the individual components (i.e., taxa) and that are complementary to the community properties proposed by Hall et al. (2018), such as biomass and gene expression. Furthermore, we propose that there are feedbacks between assembly processes and microbial membership whereby assembly processes influence which taxa are found in which abundances, but biotic interactions also influence assembly processes. In turn, we modified the framework whereby arrow 1 is bidirectional (Fig. 1).
It is important to recognize that the ecological processes of community assembly are distinct from “microbial processes” associated with biogeochemical reactions. As an emergent property, the relative influence of determinism and stochasticity is the result of complex biotic and abiotic interactions (Grilli et al., 2017) and also shapes cumulative microbial processes that impact ecosystem biogeochemical functions (Graham and Stegen, 2017) (arrow 2, Fig. 1). For example, a stronger influence of determinism over community assembly is hypothesized to cause higher respiration rates (a microbial processes) due to a larger contribution of well-adapted taxa (Graham and Stegen, 2017), though the respiration response may vary depending on the existing community composition and the deterministic forces exerted.
Analyses of microbial community assembly have been widely employed across environments including soil (Bottos et al., 2018; Dini-Andreote et al., 2015; Feng et al., 2018; Jurburg et al., 2017; Sengupta et al., 2019b), sediment (Graham et al., 2017a; Stegen et al., 2013, 2016, 2018b), marine (Starnawski et al., 2017; Wu et al., 2018), riverine (Chen et al., 2019), gut (Martínez et al., 2015), and engineered (Ofiţeru et al., 2010; Zhou et al., 2013) systems. Previous work has focused primarily on using DNA-derived membership and phylogenetic data to study whole-community assembly. In contrast, recent studies have also used an RNA-based approach to study the relative influence of stochasticity over the assembly of the putatively active portion of microbial communities (Jia et al., 2020; Jurburg et al., 2017). This RNA-based approach is complementary to the DNA-based approach and may provide additional insights into shorter-term dynamic linkages between emergent community properties and microbial processes. Such linkages have not, however, been previously evaluated.
The Hall et al. (2018) framework proposes that microbial processes (e.g., respiration rate) are influenced by both microbial community properties and environmental factors. Here we propose a revision of this structure that includes bidirectional links between the environment and microbial processes (arrow 3, Fig. 1). Such bi-directional links between microbes and their environment are common (Daly et al., 2016; Leventhal et al., 2019; Ratzke et al., 2018; Stegen et al., 2018a) and in hyporheic zone sediments may be particularly tied to thermodynamic properties of organic matter (Graham et al., 2018) and influenced by hydrological disturbances that are common in such environments. For example, preferential use of OM by microbial communities has potential to alter the thermodynamic properties of organic matter pools (Graham et al., 2017a). This microbe-driven shift in the environment could then feedback to impact microbial metabolism due to the strong influence of OM thermodynamics on biogeochemical rates (Boye et al., 2017; Garayburu-Caruso et al., 2020; Song et al., 2020; Stegen et al., 2018b). Bi-directional feedback between environmental factors and microbial processes is, therefore, likely important to the link between microbial communities and ecosystem function. Fundamental knowledge of these feedbacks and how they are modulated by hydrologic disturbances in hyporheic zone sediments is largely unknown, however.
Within ecosystems, mechanistic associations between environmental factors, microbial properties, and microbial processes underlie spatial and temporal distributions of biogeochemical rates (Fig. 1, arrow 5). The resulting distributions (e.g., of respiration rates) define cumulative system function and can be used to understand key phenomena such as biogeochemical hot spots and hot moments (McClain et al., 2003). Developing concepts and models to predict the influences of biogeochemical hot spots/moments is a major outstanding challenge. To facilitate progress, Bernhardt et al. (2017) proposed grouping hot spots/moments into the concept of ecosystem control points that exert a disproportionate influence on ecosystem function.
While not called out explicitly in Bernhardt et al. (2017), the control point concept is based on the distribution of biogeochemical rates through space and/or time. Focusing on the shape of rate distributions allows the notion of control points to be extended to the concept of control point influence (CPI; Fig. 1, arrow 5). The CPI is a quantitative measurement of the contribution of elevated biogeochemical rates in space and/or time to the net aggregated rate within a defined system (Arora et al., 2020). While proposed conceptually and studied via simulation in Arora et al. (2020), empirical measurements of CPI are lacking. More generally, incorporating CPI into a modified version of the Hall et al. (2018) framework (Fig. 1) provides an integrated conceptualization for how environmental factors, microbial properties, and microbial processes contribute to emergent system function.
Some elements of our modified framework (Fig. 1) are generalizable across systems (e.g., CPI), while others (e.g., OM thermodynamics) may have different levels of relevance across different ecosystem types. Here we aim to generate fundamental knowledge of the linkages between microbial community and ecosystem function as well as reveal how hydrologic disturbance may modify these linkages. While relationships between microbial community assembly and function have been evaluated, integrating the concepts of microbial structure, function, assembly, and environment interactions into one coherent framework has the potential to advance our understanding of the feedbacks between microbial communities and the environment.
1.3 Study objectives
Our primary objective was to study the modified conceptual framework in the context of variably inundated hyporheic zone sediments exposed to different drying and wetting dynamics. Hyporheic zones are biogeochemically active subsurface domains in river corridors through which surface water flows and can mix with groundwater (Bernhardt et al., 2017; Boano et al., 2014; McClain et al., 2003). These zones can have disproportionate biogeochemical impacts on river corridors (Boano et al., 2014; Burrows et al., 2017; Demars, 2019; Fischer et al., 2005; Kaufman et al., 2017). Within variably inundated streams (Larned et al., 2010; Romaní et al., 2006), hyporheic zones experience extreme changes in environmental conditions, but the consequences of this variability for microbe–ecosystem linkages is poorly known.
To mimic natural disturbances, we subjected sediments to wetting–drying transitions and focused on a series of analyses tied to our modified framework. We specifically evaluated relationships between (i) the relative influence of stochastic assembly as a community property and respiration rates as a microbial process (Fig. 1, arrow 2) as well as (ii) environmental features and both microbial properties (Fig. 1, arrow 4) and processes (Fig. 1, arrow 3) that underlie aggregate system function (Fig. 1, arrow 5). We evaluated relationships between cumulative properties of the microbial community (membership, assembly, biogeochemical rates), environmental features, and emergent ecosystem function by hypothesizing that (i) stronger influences of determinism result in well-adapted microbes that will generate higher respiration rates; (ii) longer duration in an inundated state will result in greater influences of stochastic assembly – due to weaker ecological selection – and lower respiration rates following re-inundation due to relatively consistent abiotic conditions (Birch, 1964); (iii) microbial processes are facilitated by OM that is thermodynamically more favorable for oxidation, leading to an association between respiration rates and OM thermodynamics; and (iv) more wet–dry transitions will increase among-replicate heterogeneity (e.g., in microbial membership), thereby increasing CPI by increasing within-treatment variability in respiration rates.
2.1 Study site and sediment collection
Hyporheic sediments were collected from the Columbia River shoreline (approximately 46.372411∘ N, 119.271695∘ W) in eastern Washington state (Fig. S1 in the Supplement) (Arntzen, 2006; Goldman et al., 2017; Graham et al., 2016; Slater et al., 2010; Stegen et al., 2018b; Zachara et al., 2013) within the Hanford Site on 14 January 2019 at 09:00 Pacific standard time. Samples were aseptically collected to a depth of 10 cm at five sub-sampling locations within a meter to form a composite sample that was sieved on site through a 2 mm sieve into a clean glass beaker. Sieved sediment was stored on blue ice for 30 min while being transported back to the laboratory. Once back at the laboratory sediment was stored at 4 ∘C until processing into incubation vials (see below).
The sediments were subjected to increasing temporal environmental variance (as a function of periodic wetting and drying transitions) and evaluated for associations between microbial membership, microbial properties, microbial community assembly, OM chemistry, absolute respiration rate (represented in this study as O2 consumption rates), and cumulative respiration rates represented as CPI. Aerobic respiration was chosen as the biogeochemical process since it influences global-scale energy and material fluxes (Fatichi et al., 2019) and because the hyporheic zone within the field system is predominantly aerobic (Graham et al., 2016, 2017b). Detailed experimental design and methods are provided in the following paragraphs.
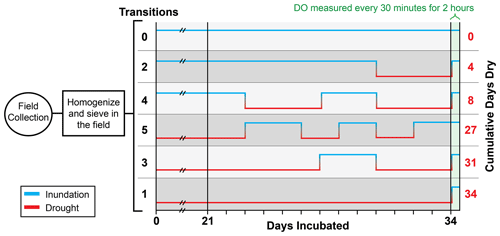
Figure 2Experimental design of batch reactor incubations subjected to six treatment regimes of inundated (blue line) and dry (red line) conditions. Black values on the left indicate the number of inundated–dry transitions, including the final inundation that all treatments experienced immediately prior to the measurement of respiration. Red values on the right indicate the number of cumulative dry days (e.g., treatments with one or three transitions experienced 34 or 31 cumulative dry days, respectively. Transitions between inundated and dry conditions started on day 24. All treatments were held at either an inundated or dry state prior to day 24. Treatments are ordered by the number of days dry.
2.2 Experimental design
Sediments used in the batch reactors were sourced from one homogenized sediment pool. In turn, 10 g of sediment from the homogenized pool was added to each reactor vial. The sample set was then split into two groups, one inundated and the other allowed to desiccate. Desiccation was achieved within 15–17 d. Sediments were periodically weighed to allow the inundated samples to be maintained at a constant water content and to monitor the remaining samples for desiccation. Inundated sediments were placed on ceramic porous plates saturated with deionized water for weight adjustments. These conditions were maintained for 23 d prior to the start of dynamic moisture manipulation, from 29 January to 21 February 2019. This initial “preconditioning” period was used to avoid measuring the immediate impacts of sampling disturbance and to allow time for desiccation. All replicate reactors were maintained in the dark, shaking at 100 rpm, at 21 ∘C and were covered with a gas-permeable Breathe-Easy (MilliporeSigma, Burlington, MA) membrane that allowed for gas exchange and drying. After the preconditioning period in which sediments were consistently inundated or allowed to continuously desiccate, the transition regimes (Fig. 2) were applied to the reactors starting on 22 February 2019. We refer to the time from 22 February 2019 onwards as the “transition period” for all treatments, even though some did not experience transitions between being inundated and dry. Each treatment had six to seven replicates (detailed below). These regimes were designed around the number of wet–dry transitions experienced by sediments within a given treatment. Treatment regimes also caused variation in the cumulative number of days sediments were in a drying state. We imposed six different experimental treatment regimes (Fig. 2) as follows.
-
Zero transitions and 0 d of desiccation. Sediments were maintained at field moisture conditions for the preconditioning and transition periods. This treatment had six replicates.
-
One transition and 34 d of desiccation. Sediments were dry during the preconditioning and transition periods and transitioned once to the field moisture level prior to respiration estimation. This treatment had six replicates.
-
Two transitions and 4 d of desiccation. Sediments were held at field moisture levels for the preconditioning period and then for the first 7 d of the transition period, then transitioned to a dried state for 4 d, and transitioned to field moisture conditions prior to respiration estimation. This treatment had seven replicates.
-
Three transitions and 31 d of desiccation. Sediments were dry during the preconditioning period and the first 4 d of the transition period, then transitioned to field moisture levels for 3 d, then transitioned to 4 d in a dried state, and transitioned again to field moisture levels prior to respiration estimation. This treatment had seven replicates.
-
Four transitions and 8 d of desiccation. Sediments were held at field moisture levels for the preconditioning period and transitioned to a dried state for the first 4 d of the transition period, then transitioned to field moisture levels for 3 d, then transitioned to 4 d in a dried state, and transitioned to field moisture levels prior to respiration estimation. This treatment had seven replicates.
-
5 Transitions and 27 d of desiccation: Sediments were dry during the preconditioning period and then transitioned to field moisture levels for the first 3 d of the transitions period, transitioned to a dried state for 2 d, transitioned to field moisture levels for 2 d, transitioned to a dried state for 2 d, and transitioned to field moisture levels for 3 d prior to respiration estimation. This treatment had seven replicates.
To avoid modifying electrical conductivity across experimental treatments, sterile deionized water was added to reactors to achieve/maintain field moisture levels according to the defined wet–dry regimes detailed above. For reactors with sediments that were below field moisture levels, deionized water was added to achieve field moisture levels prior to respiration rate estimation. Changes in the total mass of reactors and volumes of water added during the course of the experiment are provided in Table S1.
2.3 Respiration rate measurements
Laboratory incubations were performed in batch reactors to quantify dissolved oxygen consumption rates. Borosilicate glass vials (20 mL) (I-Chem™ clear VOA glass vials, Thermo-Fisher, Waltham, MA) served as incubator reactors. Factory-calibrated oxygen sensor spots (Part# 200001875, diameter=0.5 cm, detection limit 15 ppb, 0 %–100 % oxygen; PreSens GmbH, Regensburg, Germany) were adhered to the inner vials of the reactor prior to sediment addition. Detailed description of sensor adhesion and non-destructive measurements of DO consumption using these sensors are provided in Garayburu-Caruso et al. (2020). Vials were grouped into six treatment regimes (explained in the previous section) representing inundation–drought transitions.
Sample processing and incubations were performed in a laboratory at 21±1 ∘C. The reactors were monitored for 2 h, with measurement of dissolved oxygen (DO) concentration (µmol L−1) every 30 min. DO concentration in each bioreactor was measured with an oxygen optical meter (Fibox 3; PreSens GmbH) connected to a 2 mm polymer optical fiber lined up to sense the sensor dot every 30 min. A few samples were discarded due to sensor dots detaching from the glass surface. Respiration rates () were estimated as the slope of the linear regression between DO concentration and incubation time for each sample. Some non-linearity was observed in the relationship between DO concentration and time such that only the first four data points – time zero to 2 h – were used to fit a linear function. The slope of the linear function was taken as an estimate of respiration rate.
2.4 Microbial analysis
Post-incubation, the sediment slurry was transferred to centrifuge tubes (Item#28-108 Genesee Scientific) and centrifuged for 5 min at 3200 rcf and 20 ∘C. The supernatant was removed and reserved for biogeochemistry analyses, and sediment aliquots for DNA and RNA extraction were flash-frozen in liquid N2 and stored at −80 ∘C. The extraction, purification, and sequencing of sediment microbial gDNA were performed according to published protocol (Bottos et al., 2018). The extraction of RNA was performed using the Qiagen PowerSoil RNA extraction kit (Qiagen, Germantown, MD). RNA was treated with DNase and quantified with a Qubit RNA kit (Thermo Fisher, Waltham, MA). An aliquot of the RNA extraction was used to generate cDNA using the SuperScript™ IV First-Strand Synthesis System (Thermo Fisher Scientific, Waltham, MA). The 16S rRNA gene sequencing – for both gDNA and cDNA – followed the established protocol by The Earth Microbiome Project (Caporaso, 2018). Sequence pre-processing, operational taxonomic unit (OTU) assignment, and phylogenetic tree building were performed using an in-house pipeline, HUNDO (Brown et al., 2018). Sequences were deposited at NCBI's Sequence Read Archive PRJNA641165. The final sample count of gDNA and cDNA, respectively, for each treatment regime, after dropping samples following quality filtering and rarefaction, was 5 and 5 (zero transition), 4 and 3 (one transition), 5 and 4 (two transitions), 7 and 6 (three transitions), 7 and 6 (four transitions), and 7 and 5 (five transitions). Rarefaction levels are provided below in the Statistics section.
2.5 Biogeochemistry
Reserved supernatant was filtered through a 0.22 µm polyethersulfone membrane filter (Millipore Sterivex), and an aliquot was immediately removed for non-purgeable organic carbon (NPOC), and the remainder was stored at −20 ∘C until further OM high-resolution analysis was conducted (see below). NPOC was determined by acidifying an aliquot of sample with 15 % by volume of 2N ultra-pure HCL (Optima grade, Fisher#A466-500). The acidified sample was sparged with carrier gas (zero air, Oxarc# X32070) for 5 min to remove the inorganic carbon component. The sparged sample was then injected into the TOC-L furnace of the Shimadzu combustion carbon analyzer TOC-L CSH/CSN E100V with an ASI-L autosampler at 680 ∘C using 150 µL injection volumes. The best four out of five injection replicates were averaged to get the final result. The NPOC standard was made from potassium hydrogen phthalate solid (Nacalai Tesque, lot M7M4380). The calibration range was 0 to 70 ppm NPOC as carbon.
Fourier transform ion cyclotron resonance mass spectrometry (FTICR-MS) of post-incubation sediment slurry was conducted as per Danczak et al. (2020). Sample processing; injection; and data acquisition, processing, and analysis were performed as per scripts provided in Danczak et al. (2020), with “Start tolerance” in Formularity changed to 8. Ten samples were dropped due to poor calibration, resulting in five replicates for zero transitions, four replicates for one transition, five replicates for two transitions, six replicates for three transitions, and five replicates each for four and five transition regimes.
From the FTICR-MS data, as in previous work (Garayburu-Caruso et al., 2020; Graham et al., 2018; Sengupta et al., 2019b; Stegen et al., 2018b), we followed LaRowe and Van Cappellen (2011) to calculate the Gibbs free energy for the half reaction of organic carbon oxidation under standard conditions (). This calculation is based on elemental stoichiometries associated with molecular formulae assigned to individual molecules observed in the FTICR-MS data. The formulae assignments are part of the processing scripts described in Danczak et al. (2020). As in previous work (Garayburu-Caruso et al., 2020; Graham et al., 2018; Sengupta et al., 2019b; Stegen et al., 2018b), we interpret larger values of to indicate OM that is thermodynamically less favorable for oxidation by microbes. That is, larger values of indicate OM that provides less net energy to a microbial cell per oxidation event, assuming all else is equal. Given the large numbers of assigned formulae within each sample, this resulted in thousands of estimates within each sample, from which we estimated mean for each sample.
2.6 Estimating influences of community assembly processes
The relative influences of community assembly processes impacting microbial community membership are emergent properties that cannot be calculated/inferred directly from knowledge of membership. To evaluate assembly processes as a link between membership and microbial processes (refer Fig. 1), it is necessary to quantitatively estimate the relative influences of these processes. To do so we use a well-established null modeling framework based on phylogenetic relationships among microbial taxa (Dini-Andreote et al., 2015; Stegen et al., 2012, 2015; Zhou and Ning, 2017). We refer the reader to these previous studies for details. In brief, randomizations were used to generate estimates of phylogenetic associations among microbial taxa for scenarios in which microbial communities were stochastically assembled. These stochastic (i.e., null) expectations were compared quantitatively to observed phylogenetic associations to estimate the β-nearest taxon index (βNTI) (Stegen et al., 2012). We used cDNA sequences rarefied to 27 227 and gDNA sequences rarefied to 15 106 sequences per sample to determine putatively active community and whole-community βNTI values, respectively. Samples falling below these sequence counts were removed as indicated above in Sect. 2.4 Microbial Analysis. A βNTI value of 0 indicates no deviation between the stochastic expectation and the observed phylogenetic associations, thereby indicating the dominance of stochastic assembly processes. As βNTI deviates further from 0, there is an increasing influence of deterministic assembly processes that drive community membership away from the stochastic expectation. βNTI values below −2 or above +2 indicate statistical significance, with negative and positive values indicating less than or more than expected shifts in membership. βNTI is a pairwise metric measured between any two communities and/or samples, such that shifts in membership are related to changes between the pair of communities being evaluated. We used βNTI to study all pairwise community comparisons within each experimental treatment. Each community from a given reactor is therefore associated with multiple βNTI values due to being compared to communities associated with other replicate reactors. In turn, the average βNTI was calculated for each reactor. As in Stegen et al. (2015), this provides a community-specific value for βNTI and thus an estimate of the relative influences of stochastic and deterministic processes causing deviations between a given community and all other communities within the same experimental conditions (Sengupta et al., 2019b). That is, the larger the absolute value of βNTI for a given community, the stronger the influence of deterministic assembly processes acting on that community (Stegen et al., 2015). In turn, these community-specific estimates were related to reactor-specific measurements. For example, respiration rates were regressed against βNTI to evaluate the link between emergent properties (i.e., ecological assembly) and microbial processes (i.e., respiration rates).
2.7 Evaluating relationships between microbial characteristics and environment
Respiration rate distributions, absolute βNTI values, and were summarized as box plots. A pairwise Mann–Whitney test was performed to evaluate statistical differences between reactor-specific measurements (e.g., respiration rates and thermodynamic properties) and treatment groups (cumulative dry and inundated days). Continuous bivariate relationships were evaluated with ordinary least-squares regression. Prior to regression analyses, respiration rates were log-transformed due to non-linearities resulting from respiration being constrained to be at or above zero. Prior to log-transformation, half the smallest non-zero rate was added to each rate to enable inclusion of rate estimates with a value of zero.
2.8 Control point influence calculation
To characterize respiration rate distributions, we used the control point influence (CPI) metric. CPI was recently developed (Arora et al., 2020) and is defined as the fraction of cumulative function (Rtot; e.g., total respiration rate) within a defined system that is contributed by individual rates that are above the system's median rate (Rmed). To define cumulative function one must first define the system being evaluated. In our study, all replicate batch reactors within a given experimental treatment were conceptualized as a representative set of samples from a larger system experiencing the experimental conditions. Rtot for each treatment was therefore estimated as the sum of respiration rates across a treatment's replicate reactors. CPI was estimated as the sum of respiration rates that fell above the median rate for a given treatment (Rabove) divided by Rtot for that treatment. That is, , where Ri denotes respiration rates from individual reactors that fell above Rmed, and .
An important feature of CPI is that it makes no assumptions of distribution normality and can be estimated for rate distributions of any form (e.g., unimodal, multimodal, Gaussian, skewed). In most cases, CPI is constrained to have a minimum value of 0.5 (for a perfectly normal distribution with no outliers) and asymptotically approach 1 as a maximum value (e.g., for heavily skewed distributions with a small number of very high rates). CPI therefore quantitatively estimates the biogeochemical contribution of places in space or points in time that have elevated biogeochemical rates.
Understanding how CPI varies through space, time, and with environmental conditions (e.g., disturbance frequency) provides an opportunity to deepen our understanding of factors controlling hot spot/moment behavior. Lower values of CPI (i.e., closer to 0.5) can arise through any mechanism that constrains biogeochemical rates to be consistent through space or time. For example, if respiration rates were measured in multiple locations across a well-mixed water body, we may expect a Gaussian rate distribution with little variation. The cumulative respiration of the water body should not be influenced significantly by hot spots, which would be reflected in low CPI. Conversely, higher values of CPI (i.e., closer to 1) can arise through any mechanism that increases the probability of positive outliers within a given rate distribution. Spatial variation in the temporal dynamics of disturbances (e.g., more frequent disturbances in some locations) is an example that is closely aligned with our experimental treatments. In this case the probability of rate outliers (i.e., hot spots) may vary as a function of disturbance frequency. For example, in spatial domains that experience more wetting–drying dynamics we might expect a higher probability of rate outliers. This is because each time sediments go dry the exact spatial distribution of water films that remain will vary across locations within the broader wetted–dried spatial domain. In turn, we would expect higher values of CPI in spatial domains that are more frequently wetted and dried. Regardless of whether this specific hypothesis is rejected or not, we contend that using CPI enables development of a priori quantitative hypotheses spanning space, time, environmental conditions (e.g., disturbance frequency), and scales. This provides new opportunities to more systematically elucidate factors governing the influence of hot spots/moments.
To link microbial membership to emergent microbial community properties (Fig. 1, arrow 1), we used null modeling to estimate the contributions of stochastic and deterministic community assembly. Results from the null models indicate a relatively balanced mixture of stochasticity and determinism for both the whole community (gDNA-based) and putatively active community (rRNA-based) (Fig. S2 in the Supplement). More specifically, stochasticity and determinism each governed 50 % of turnover in microbial membership for the whole community and 33 % and 67 %, respectively, for the putatively active community. The relative contributions of the two deterministic components (homogeneous and variable selection) were strongly imbalanced. Homogeneous selection was responsible for 94 % and 91 % of the deterministic component for the whole and putatively active communities, respectively. The contributions of homogeneous and variable selection to the deterministic component must sum to 1, such that the variable selection was responsible for 6 % and 9 % of the deterministic component for the whole and putatively active communities, respectively.
As shown in Fig. 1 (arrow 2), we hypothesized a link between microbial community properties and microbial processes realized as a relationship between the strength of determinism and respiration rates. Such a relationship was not observed for the whole community (Fig. 3a), but we did observe a non-linear decreasing relationship between respiration rates and the absolute value of βNTI for the putatively active community (Fig. 3b). The direction of this relationship (negative) was opposite of that expected, and the relationship was clearly structured by among-treatment shifts in both respiration rate and βNTI (Fig. 3b).
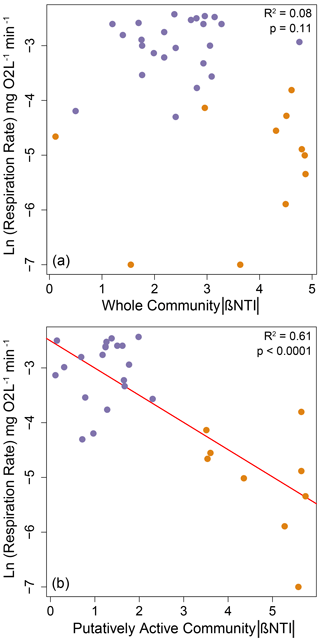
Figure 3Natural-log-transformed respiration rates (i.e., O2 consumption) as a function of the absolute value of βNTI for (a) whole communities or (b) putatively active communities. Larger absolute values of βNTI indicate stronger influences of deterministic assembly. Nonlinearity was observed because the respiration rate has a lower limit of 0 such that its relationship with βNTI was fit as a negative exponential function. The significant regression model is shown as a red line, and statistics are provided on each panel. Treatments with 0, 4, 8, or 27 d dry are shown in purple. Treatments with 31 or 34 d dry are shown in orange.
In our conceptual framework there are multiple ways in which connections among the environment, microbial properties, and microbial processes may be realized, in part due to the environment having multiple components relevant to our study (Fig. 1, arrows 3, 4). More specifically, the environment was characterized here in terms of both disturbance (number of dry days, imposed by the experimental manipulation) and OM thermodynamics (; this is an emergent aspect of the environment).
Disturbance influenced both microbial properties and processes. These influences appeared to be non-linear with experimental treatments associated with the two largest number of dry days (31 and 34) causing decreases in respiration rates (Fig. 4a) and stronger influences of deterministic homogeneous selection for the putatively active community (Fig. 4b). Disturbance had no clear influence on community assembly for the whole community (Figs. S3 and S4a in the Supplement). Given the apparent binary nature of these results, we evaluated statistical significance by combining respiration rate data from treatments with 0–27 cumulative dry days and separately combining data from treatments with 31 or 34 cumulative dry days (Fig. S5 in the Supplement). Respiration rates were significantly depressed in the treatments associated with 31 or 34 cumulative dry days (W=5, p<0.001). The βNTI data are non-independent due to being based on all pairwise comparisons within a treatment. Standard statistical tools are therefore not applicable for assigning statistical significance when comparing βNTI distributions. However, as shown in Figs. 4b and S4b in the Supplement, there is an obvious shift to lower βNTI values for the putatively active community in the treatments with 31 or 34 cumulative dry days.
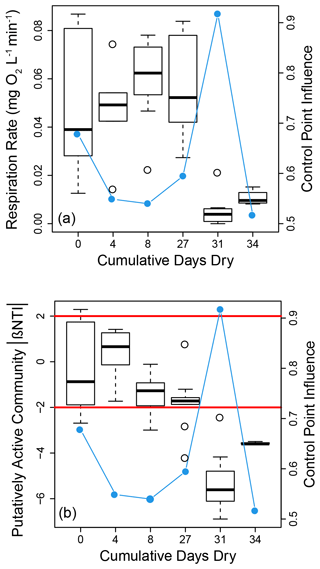
Figure 4Boxplot representations of respiration rate (a) and putatively active community βNTI (b) distributions as a function of the cumulative number of days reactors were in a dried state. Each value along the horizontal axis represents a different experimental treatment. On both panels the right hand axis provides estimates of control point influence (blue circles and lines) across the treatments. Horizontal red lines in (b) indicate significance thresholds; values below −2 indicate deterministic homogenous selection, values above +2 indicate deterministic variable selection, and values between −2 and +2 indicate stochastic assembly.
The other aspect of the environment examined here (i.e., OM thermodynamics) also had significant relationships with both microbial processes (Fig. 1, arrow 3) and properties (Fig. 1, arrow 4). More specifically, respiration rates decreased significantly as a negative exponential function of increasing (R2=0.34; p=0.001; Fig. 5a). This indicates a decrease in respiration rate as OM thermodynamic properties shifted towards lower favorability for oxidation (i.e., larger values of ). Similarly, we found that the strength of deterministic assembly associated with the putatively active community increased linearly with (R2=0.40; p=0.001; Fig. 5b). The relationships were clearly structured by among-treatment shifts in respiration rate, βNTI, and favorability of organic matter (). The strength of deterministic assembly associated with the whole community was unrelated to (p=0.64) (Fig. S6 in the Supplement).
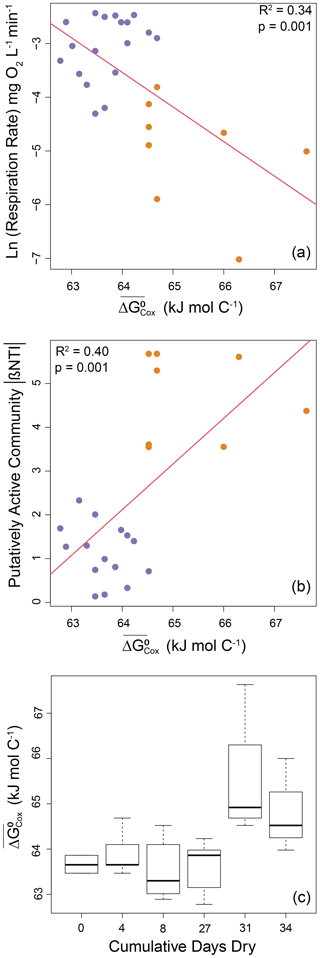
Figure 5Microbial processes and properties as a function of OM thermodynamics as well as impacts of disturbance on OM thermodynamics. (a) Respiration rates (natural log transformed) decreased with decreasing favorability for oxidation (larger values of ). (b) The strength of deterministic selection measured as the absolute value of βNTI increased with decreasing favorability for oxidation. Regression models are shown as red lines, and statistics are provided on each panel. (c) Boxplot representations of the distributions of OM thermodynamics across experimental treatments. Significant increases were observed for treatments with 31 or 34 cumulative dry days. See text and Fig. S7 for a description of statistics. Treatments with 0, 4, 8, or 27 d dry are shown in purple. Treatments with 31 or 34 d dry are shown in orange.
The conceptual model described in Fig. 1 focuses primarily on connections among environmental and/or microbial attributes, but there are potentially important relationships within attribute categories. In particular, within the environmental category there is the potential for an influence of disturbance on OM thermodynamics. Such an effect was found for OM thermodynamics as measured by (Fig. 5c). Using the same approach as for analyses described above, we combined data for treatments with 0–27 cumulative dry days and compared that distribution to data combined across treatments with 31 or 34 cumulative dry days. A Mann–Whitney test comparing these distributions confirmed a significant change in the distribution (W=189, ) (Fig. S7 in the Supplement).
The last component of the conceptual model considered here is the connection between microbial processes occurring in a given location and cumulative system function that aggregates across locations (Fig. 1, arrow 5). It is at the system level that the influence of biogeochemical hot spots (or hot moments) can be evaluated. We conceptualized an aggregate system as the collection of replicate batch reactors within a given experimental treatment. Based on this definition, we estimated control point influence (CPI) as a measurement for the influence of biogeochemical hot spots. We observed a large amount of variation in CPI across experimental treatments, but there was no clear, direct influence of the treatments on CPI (Fig. 4). The largest value of CPI observed (>0.9) was associated with the treatment that imposed 31 cumulative dry days. This treatment also had the lowest median respiration rates across all treatments (Fig. 4). We did not find any significant relationship (p>0.70) between within-treatment community compositions (whole and putatively active) and CPI, suggesting that within-treatment beta-dispersion of the community is not a better predictor of CPI.
Mechanistic evaluation of microbe–environment interactions is fundamental to understanding microbe-mediated ecosystem function. Inspired by a microbe–environment–ecosystem framework proposed by Hall et al. (2018), we proposed and evaluated a modified framework linking microbial characteristics (membership, emergent properties, processes), the environment (disturbance, OM thermodynamics), and cumulative ecosystem function (CPI) of hyporheic zone sediments. Our results provide clear support for the overall conceptual framework and further point to an iterative loop among OM thermodynamics, respiration rates, and microbial community assembly that can be initiated by externally imposed disturbance. Furthermore, our results indicate that the iterative thermodynamics–assembly–respiration loop may be initiated through threshold-like impacts of disturbance that were observed only after 31 or more cumulative days of desiccation.
We first evaluated emergent community properties as a function of microbial membership by studying the relative influences of stochasticity and determinism over community assembly. Taking this approach, we found fully balanced stochastic–deterministic influences over the whole community, in which each contributed to 50 % of the variation in community composition. The relative influences of stochasticity and determinism have been quantified for many microbial systems and the estimates are highly variable (Tripathi et al., 2018; Wang et al., 2013). In addition, within the deterministic component of assembly, homogeneous selection had a far greater influence than variable selection. Previous work has also observed a broad range of contributions from homogeneous and variable selection (Fillinger et al., 2019; Graham et al., 2016; Li et al., 2019; Sengupta et al., 2019a; Whitman et al., 2018). As such, the assembly-associated outcomes observed here for the whole community are not unexpected relative to previous work. Very few studies, however, have examined the relative influences of different assembly components over putatively active microbial communities. This focus is critical in our framework since many microorganisms are inactive while the active community remains sensitive to abiotic stresses and contributes to ecosystem function.
For the putatively active communities we found that across all treatments both stochasticity and determinism were important, though deterministic assembly had greater influence. This deviates quantitatively from the whole community in which the influences of stochasticity and determinism were more balanced. The difference in assembly influences in whole and putatively active communities is likely driven by the duration of the imposed experimental treatments (2 weeks). This time period may not be sufficient for birth and death events to restructure the community composition. Instead, physiological responses as a function of decreases and increases in microbial activity (changes in cDNA signatures) are more likely. A stronger disturbance (e.g., imposing the treatments for a longer period of time) may provide further insights into the impacts of disturbance on the whole community (e.g., evident as changes in gDNA signatures). Consistent with the whole-community results, however, was the dominance of homogeneous selection within the deterministic component of assembly.
The strong influence of homogeneous selection is likely due to selection-based constraints imposed by aspects of the experimental system that did not vary across treatments. For example, mineralogy is known to strongly influence microbial communities (Boyd et al., 2007; Carson et al., 2009; Doetterl et al., 2018; Fauvel et al., 2019; Mauck and Roberts, 2007; Stegen et al., 2016) and was homogenized across the experimental batch reactors, thereby potentially imposing homogeneous selection on both the whole and putatively active communities.
Our study uniquely evaluates null-model outcomes of putatively active community assemblies in hyporheic zone sediments, where homogeneous selection was further enhanced by our experimentally imposed hydrologic disturbances. Increased homogeneous selection in response to disturbance is consistent with previous work in aquatic (Chase, 2007) and soil systems. For example, in a soil system, disturbance led to an immediate increase in homogeneous selection for the putatively active community (Jurburg et al., 2017). The strong influence of homogeneous selection on the putatively active community is not always observed, however, suggesting it may be tied to acute disturbance. That is, Jia et al. (2020) recently found that within a natural soil chronosequence, variable selection was stronger for putatively active communities while homogeneous selection influenced the whole community assembly. Our results combined with these previous studies indicate that community assembly of putatively active members may be more closely linked to short-term environmental change than assembly of the whole community.
In addition to being more sensitive to disturbance, we find that assembly of the putatively active community was more strongly tied to microbial processes (i.e., respiration rate) than was the whole community. A strong link between biogeochemical rates and the putatively active community is consistent with previous studies (Freedman et al., 2015; Levy-Booth et al., 2019). More specifically, we observed a negative relationship between respiration rate and absolute values of βNTI for the putatively active community but no relationship for the whole community. The direction of this relationship is opposite to our hypothesis. While stronger selection should remove mal-adapted individuals, leading to higher biogeochemical rates (Graham and Stegen, 2017), increased selection in our experiment was imposed by disturbance that appeared to directly suppress respiration rates due to desiccation (Baldwin and Mitchell, 2000; Manzoni et al., 2012). The simultaneous suppression of respiration and imposition of stronger selection in treatments with 31 or 34 d of dry conditions (Fig. 3b) led to the negative relationship between respiration and the strength of selection. The lack of such relationships when considering the whole community indicates that a greater focus on assembly dynamics of putatively active communities could reveal new insights into the multi-component linkages among microbes, the environment, and function.
Disturbance also impacted OM thermodynamics and respiration rates, potentially initiating an iterative loop among microbial assembly, microbial processes, and the abiotic environment. In this iterative loop the direction of causation between OM thermodynamics and microbial processes (Fig. 1, arrow 3) is not clear due to feedbacks, though we interpret a direction of causation from OM thermodynamics to microbial properties in terms of community assembly (Fig. 1, arrow 4). As such, there may be a loop between OM thermodynamics and microbial processes (i.e., respiration) embedded in a larger loop that also includes microbial properties (i.e., community assembly). Such feedbacks are inherent in complex systems and often lead to non-linear dynamics as observed here in terms of the threshold-like impact of desiccation on multiple system components (Pérez Castro et al., 2019; Prosser and Martiny, 2020).
As key elements of the inferred system of feedbacks, the links among OM thermodynamic properties, respiration, and desiccation found here are consistent with recent work tied to the same field system. That is, Garayburu-Caruso et al. (2020) also showed decreasing aerobic respiration with decreasing favorability for oxidation (i.e., larger values of ) using sediments sourced ∼2 years previously from the same field system. In addition, the impacts of desiccation found here are similar to Goldman et al. (2017) after re-inundation. This impact of desiccation on respiration contrasts with the Birch effect (Birch and Friend, 1956) in soils whereby desiccation followed by re-wetting leads to enhanced respiration. The consistency across hyporheic zone studies and deviation from classical soil phenomena point to potential consistency in governing processes within the hyporheic zone that deviate from processes operating in soil systems. Further evaluation is needed across additional hyporheic zone systems to rigorously evaluate this inference, however.
In addition to linkages between the environment and microbial aspects of the system, our study revealed connections within the environmental components of the conceptual framework. That is, greater cumulative desiccation caused an increase in , indicating a significant change in OM thermodynamics (Fig. 5c). While our data cannot pinpoint governing mechanism(s), we hypothesize that the response may have been tied to increased ion concentration following desiccation. For example, OM chemistry may have been altered due to changes in abiotic sorption, limitations of microbially accessible C due to water potential constraints, and/or osmolyte production and formation of extracellular polymeric substances (Fierer et al., 2003; Gionchetta et al., 2020; Homyak et al., 2018).
Irrespective of mechanisms, the shift in OM thermodynamics in response to desiccation was associated with a decline in respiration. We infer a causal connection between OM thermodynamics and respiration, potentially triggered by desiccation-driven shifts in OM chemistry and/or microbial physiology. This causal connection is supported by recent work (Garayburu-Caruso et al., 2020) and the observation of a continuous function between and respiration rate that transcended experimental treatments. Desiccation therefore likely influences and may even initiate an iterative loop among OM thermodynamics, microbial assembly, and biogeochemistry that underlies cumulative system function.
Cumulative system function can often be driven by ecosystem control points (Bernhardt et al., 2017), but we observed relatively little indication of such behavior. That is, estimates of control point influence (CPI) were relatively low across most treatments. CPI is theoretically constrained to range from 0.5–1, with lower values indicating smaller influences of control points. In our study, all but one treatment had CPI between ∼0.5 and 0.7. The associated distributions of respiration rates did not contain obvious outliers such that we interpret CPI values in the 0.5–0.7 range to be relatively low and not strongly influenced by control points or biogeochemical hot spots/moments (McClain et al., 2003). The treatment with 31 cumulative days of desiccation diverged from the rest in having a CPI value of ∼0.9. This large CPI was due to a single outlier (Fig. 5a) such that most of the cumulative respiration across reactors was contributed by that single reactor. We interpret that single reactor as a biogeochemical hot spot or control point within that experimental treatment. It is unclear, however, what led to such behavior as disturbance did not have any systematic influence on CPI.
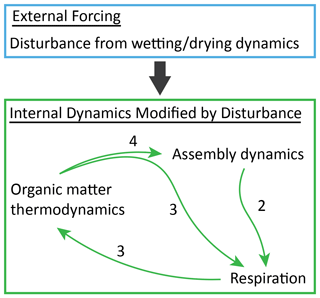
Figure 6Integrated conceptual interpretation of results from this study. Collectively, our results indicate that the external forcing imposed by disturbance leads to feedback between assembly of the putatively active community and respiration rates that is modulated by coupled dynamics in organic matter thermodynamics. Relative to Fig. 1, here external and internal aspects of the environment are separated. The arrows within the internal dynamics component are analogous to arrows 2, 3, and 4 in Fig. 1. The arrow from external to internal is not considered in Fig. 1 and represents the impact of external forcing on all aspects of the internal system. These impacts are both direct effects of disturbance and indirect effects mediated through the internal feedback that collectively lead to impacts of re-wetting that are contingent on desiccation history.
A strength of CPI as a metric is that it allows for direct quantitative comparisons across studies, systems, and scales. Ours is the first study to estimate CPI, however, such that we cannot yet make comparisons to previous work. Through future comparisons it will be possible to evaluate the strengths, weaknesses, and behavior of CPI. We expect that some patterns may emerge such as CPI having a greater likelihood to reach very high values (near 1) in systems with relatively low rates on average. In these conditions, even a modest quantitative increase in biogeochemical rates can lead to a large proportional change such that most cumulative function is from a single point in space and/or time, resulting in large CPI. We also expect that some biogeochemical processes will show greater variation in CPI than others, potentially due to variation in degree of functional redundancy (Louca et al., 2018). For example, processes such as respiration can be performed by numerous microbial taxa (i.e., there is high functional redundancy), while others are more constrained to a relatively small number of taxa (e.g., ammonia oxidation). We hypothesize that CPI may be lower on average and less variable across systems and scales for biogeochemical processes with greater functional redundancy. Additional work will be needed to test this hypothesis.
In this study we coupled intrinsic characteristics of natural hyporheic zone sediments with imposed constraints in the form of desiccation to evaluate an a priori conceptual framework modified from Hall et al. (2018). Our results demonstrated strong and often non-linear connections among desiccation, OM thermodynamics, assembly of the putatively active microbial community, and respiration rates. Collating our results points to further modification of the framework into an a posteriori conceptual model containing nested feedback loops (Fig. 6). This conceptual model is consistent with the recently proposed unification of microbial ecology around the concepts of external forcing, internal dynamics, and historical contingencies (Stegen et al., 2018a). That is, we hypothesize that external forcing imposed by desiccation initiates multiple internal loops that drive biological and chemical dynamics that, in turn, underlie respiration responses to re-wetting that are contingent on desiccation history. The development of conceptual models such as this is key to incorporating additional mechanistic detail into predictive simulation models (e.g., reactive transport codes). We encourage further evaluation and improvement of both our a priori and a posteriori concepts across environmentally divergent conditions to generate knowledge that is transferable across systems.
The R scripts used in this study are hosted on ESS-DIVE in the data package found at https://data.ess-dive.lbl.gov/view/doi:10.15485/1807580 (last access: 12 July 2021). Null modeling scripts were based on those available at https://github.com/stegen/Stegen_etal_ISME_2013 (last access: 25 February 2015).
Data are available on the ESS-DIVE archive at the following link https://data.ess-dive.lbl.gov/view/doi:10.15485/1807580 (last access: 12 July 2021). Sequence data are available on NCBI's Sequence Read Archive PRJNA641165.
The supplement related to this article is available online at: https://doi.org/10.5194/bg-18-4773-2021-supplement.
JCS and SF conceptualized and designed the study; JT, JH, LR, RC, SF, and VAG-C performed the experiment; AS, JCS, RED, and SF analyzed data; AS, JCS, and SF drafted the manuscript, and all authors contributed to further writing.
The authors declare that they have no conflict of interest.
We thank Amy Goldman and Nathan Johnson for developing graphics.
Publisher's note: Copernicus Publications remains neutral with regard to jurisdictional claims in published maps and institutional affiliations.
The initial experimental stages of this work were supported by the PREMIS Initiative (grant 71053) at the Pacific Northwest National Laboratory (PNNL) with funding from the Laboratory Directed Research and Development Program at PNNL, a multiprogram national laboratory operated by Battelle for the US Department of Energy under contract DE-AC05-76RL01830. The later stages of this work (e.g., data analysis, conceptual interpretation, manuscript development) were supported by the US Department of Energy BER program, as part of an Early Career Award (grant 74193) to JCS at PNNL. A portion of the research was performed using the Environmental Molecular Sciences Laboratory (user proposal 51180), a DOE Office of Science User Facility sponsored by the Office of Biological and Environmental Research.
This paper was edited by Denise Akob and reviewed by Stephanie Jurburg and one anonymous referee.
Arntzen, E.: Effects of fluctuating river flow on groundwater/surface water mixing in the hyporheic zone of a regulated, large cobble bed river – River Research and Applications – Wiley Online Library, https://doi.org/10.1002/rra.947, 2006.
Arora, B., Briggs, M. A., Zarnetske, J., Stegen, J. C., Gomez-Velez, J., Dwivedi, D., and Steefel, C. I.: Hot Spots and Hot Moments in the Critical Zone: Identification of and Incorporation into Reactive Transport Models, in: Biogeochemistry of the Critical Zone, Wymore, A., Yang, W., Silver, W., McDowell, B., and Chorover, J. (Eds.), Springer-Nature, in press, 2020.
Baldwin, D. S. and Mitchell, A. M.: The effects of drying and re-flooding on the sediment and soil nutrient dynamics of lowland river–floodplain systems: a synthesis, Regul. River., 16, 457–467, https://doi.org/10.1002/1099-1646(200009/10)16:5<457::AID-RRR597>3.0.CO;2-B, 2000.
Barnard, R. L., Osborne, C. A., and Firestone, M. K.: Changing precipitation pattern alters soil microbial community response to wet-up under a Mediterranean-type climate, ISME J., 9, 946–957, https://doi.org/10.1038/ismej.2014.192, 2015.
Bartelme, R. P., Custer, J. M., Dupont, C. L., Espinoza, J. L., Torralba, M., Khalili, B., and Carini, P.: Influence of Substrate Concentration on the Culturability of Heterotrophic Soil Microbes Isolated by High-Throughput Dilution-to-Extinction Cultivation, mSphere, 5, e00024-20, https://doi.org/10.1128/mSphere.00024-20, 2020.
Behrens, S., Kappler, A., and Obst, M.: Linking environmental processes to the in situ functioning of microorganisms by high-resolution secondary ion mass spectrometry (NanoSIMS), and scanning transmission X-ray microscopy (STXM), Environ. Microbiol., 14, 2851–2869, https://doi.org/10.1111/j.1462-2920.2012.02724.x, 2012.
Bernhardt, E. S., Blaszczak, J. R., Ficken, C. D., Fork, M. L., Kaiser, K. E., and Seybold, E. C.: Control Points in Ecosystems: Moving Beyond the Hot Spot Hot Moment Concept, Ecosystems, 20, 665–682, https://doi.org/10.1007/s10021-016-0103-y, 2017.
Bier, R. L., Bernhardt, E. S., Boot, C. M., Graham, E. B., Hall, E. K., Lennon, J. T., Nemergut, D. R., Osborne, B. B., Ruiz-González, C., Schimel, J. P., Waldrop, M. P., and Wallenstein, M. D.: Linking microbial community structure and microbial processes: an empirical and conceptual overview, FEMS Microbiol. Ecol., 91, fiv113, https://doi.org/10.1093/femsec/fiv113, 2015.
Birch, H. F.: Mineralisation of plant nitrogen following alternate wet and dry conditions, Plant Soil, 20, 43–49, https://doi.org/10.1007/BF01378096, 1964.
Birch, H. F. and Friend, M. T.: Humus Decomposition in East African Soils, Nature, 178, 500–501, https://doi.org/10.1038/178500a0, 1956.
Blazewicz, S. J., Barnard, R. L., Daly, R. A., and Firestone, M. K.: Evaluating rRNA as an indicator of microbial activity in environmental communities: limitations and uses, ISME J., 7, 2061–2068, https://doi.org/10.1038/ismej.2013.102, 2013.
Boano, F., Harvey, J. W., Marion, A., Packman, A. I., Revelli, R., Ridolfi, L., and Wörman, A.: Hyporheic flow and transport processes: Mechanisms, models, and biogeochemical implications, Rev. Geophys., 52, 603–679, https://doi.org/10.1002/2012RG000417, 2014.
Bottos, E. M., Kennedy, D. W., Romero, E. B., Fansler, S. J., Brown, J. M., Bramer, L. M., Chu, R. K., Tfaily, M. M., Jansson, J. K., and Stegen, J. C.: Dispersal limitation and thermodynamic constraints govern spatial structure of permafrost microbial communities, FEMS Microbiol. Ecol., 94, fiy110, https://doi.org/10.1093/femsec/fiy110, 2018.
Boyd, E. S., Cummings, D. E., and Geesey, G. G.: Mineralogy Influences Structure and Diversity of Bacterial Communities Associated with Geological Substrata in a Pristine Aquifer, Microb. Ecol., 54, 170–182, https://doi.org/10.1007/s00248-006-9187-9, 2007.
Boye, K., Noël, V., Tfaily, M. M., Bone, S. E., Williams, K. H., Bargar, J. R., and Fendorf, S.: Thermodynamically controlled preservation of organic carbon in floodplains, Nat. Geosci., 10, 415–419, https://doi.org/10.1038/ngeo2940, 2017.
Brown, J., Zavoshy, N., Brislawn, C. J., and McCue, L. A.: Hundo: a Snakemake workflow for microbial community sequence data, PeerJ Inc., 2018.
Burrows, R. M., Rutlidge, H., Bond, N. R., Eberhard, S. M., Auhl, A., Andersen, M. S., Valdez, D. G., and Kennard, M. J.: High rates of organic carbon processing in the hyporheic zone of intermittent streams, Sci. Rep., 7, 1–11, https://doi.org/10.1038/s41598-017-12957-5, 2017.
Caporaso, J. G.: EMP 16S Illumina Amplicon Protocol, https://doi.org/10.17504/protocols.io.nuudeww, 2018.
Cardoso, D. C., Sandionigi, A., Cretoiu, M. S., Casiraghi, M., Stal, L., and Bolhuis, H.: Comparison of the active and resident community of a coastal microbial mat, Sci. Rep., 7, 1–10, https://doi.org/10.1038/s41598-017-03095-z, 2017.
Carson, J. K., Campbell, L., Rooney, D., Clipson, N., and Gleeson, D. B.: Minerals in soil select distinct bacterial communities in their microhabitats, FEMS Microbiol. Ecol., 67, 381–388, https://doi.org/10.1111/j.1574-6941.2008.00645.x, 2009.
Chase, J. M.: Drought mediates the importance of stochastic community assembly, P. Natl. Acad. Sci. USA, 104, 17430–17434, https://doi.org/10.1073/pnas.0704350104, 2007.
Chen, W., Ren, K., Isabwe, A., Chen, H., Liu, M., and Yang, J.: Stochastic processes shape microeukaryotic community assembly in a subtropical river across wet and dry seasons, Microbiome, 7, 138, https://doi.org/10.1186/s40168-019-0749-8, 2019.
Daly, R. A., Borton, M. A., Wilkins, M. J., Hoyt, D. W., Kountz, D. J., Wolfe, R. A., Welch, S. A., Marcus, D. N., Trexler, R. V., MacRae, J. D., Krzycki, J. A., Cole, D. R., Mouser, P. J., and Wrighton, K. C.: Microbial metabolisms in a 2.5-km-deep ecosystem created by hydraulic fracturing in shales, Nat. Microbiol., 1, 1–9, https://doi.org/10.1038/nmicrobiol.2016.146, 2016.
Danczak, R. E., Goldman, A. E., Chu, R. K., Toyoda, J. G., Garayburu-Caruso, V. A., Tolić, N., Graham, E. B., Morad, J. W., Renteria, L., Wells, J. R., Herzog, S. P., Ward, A. S., and Stegen, J. C.: Ecological theory applied to environmental metabolomes reveals compositional divergence despite conserved molecular properties, bioRxiv, 2020.02.12.946459, https://doi.org/10.1101/2020.02.12.946459, 2020.
Demars, B. O. L.: Hydrological pulses and burning of dissolved organic carbon by stream respiration, Limnol. Oceanogr., 64, 406–421, https://doi.org/10.1002/lno.11048, 2019.
Dini-Andreote, F., Stegen, J. C., Elsas, J. D. van, and Salles, J. F.: Disentangling mechanisms that mediate the balance between stochastic and deterministic processes in microbial succession, Proc. Natl. Acad. Sci. USA, 112, E1326–E1332, https://doi.org/10.1073/pnas.1414261112, 2015.
Doetterl, S., Berhe, A. A., Arnold, C., Bodé, S., Fiener, P., Finke, P., Fuchslueger, L., Griepentrog, M., Harden, J. W., Nadeu, E., Schnecker, J., Six, J., Trumbore, S., Van Oost, K., Vogel, C., and Boeckx, P.: Links among warming, carbon and microbial dynamics mediated by soil mineral weathering, Nat. Geosci., 11, 589–593, https://doi.org/10.1038/s41561-018-0168-7, 2018.
Fatichi, S., Manzoni, S., Or, D., and Paschalis, A.: A Mechanistic Model of Microbially Mediated Soil Biogeochemical Processes: A Reality Check, Global Biogeochem. Cy., 33, 620–648, https://doi.org/10.1029/2018GB006077, 2019.
Fauvel, B., Cauchie, H.-M., Gantzer, C., and Ogorzaly, L.: Influence of physico-chemical characteristics of sediment on the in situ spatial distribution of F-specific RNA phages in the riverbed, FEMS Microbiol. Ecol., 95, fiy240, https://doi.org/10.1093/femsec/fiy240, 2019.
Feng, Y., Chen, R., Stegen, J. C., Guo, Z., Zhang, J., Li, Z., and Lin, X.: Two key features influencing community assembly processes at regional scale: Initial state and degree of change in environmental conditions, Mol. Ecol., 27, 5238–5251, https://doi.org/10.1111/mec.14914, 2018.
Fierer, N., Allen, A. S., Schimel, J. P., and Holden, P. A.: Controls on microbial CO2 production: a comparison of surface and subsurface soil horizons, Glob. Change Biol., 9, 1322–1332, https://doi.org/10.1046/j.1365-2486.2003.00663.x, 2003.
Fillinger, L., Zhou, Y., Kellermann, C., and Griebler, C.: Non-random processes determine the colonization of groundwater sediments by microbial communities in a pristine porous aquifer, Environ. Microbiol., 21, 327–342, https://doi.org/10.1111/1462-2920.14463, 2019.
Fischer, H., Kloep, F., Wilzcek, S., and Pusch, M. T.: A River's Liver – Microbial Processes within the Hyporheic Zone of a Large Lowland River, Biogeochemistry, 76, 349–371, https://doi.org/10.1007/s10533-005-6896-y, 2005.
Freedman, Z. B., Romanowicz, K. J., Upchurch, R. A., and Zak, D. R.: Differential responses of total and active soil microbial communities to long-term experimental N deposition, Soil Biol. Biochem., 90, 275–282, https://doi.org/10.1016/j.soilbio.2015.08.014, 2015.
Fu, X., Li, Y., Meng, Y., Yuan, Q., Zhang, Z., Norbäck, D., Deng, Y., Zhang, X., and Sun, Y.: Derived ecological niches of indoor microbes are crucial for asthma symptoms in university dormitories, bioRxiv, 2020.01.05.893529, https://doi.org/10.1101/2020.01.05.893529, 2020.
Garayburu-Caruso, V. A., Stegen, J. C., Song, H.-S., Renteria, L., Wells, J., Garcia, W., Resch, C. T., Goldman, A. E., Chu, R. K., Toyoda, J., and Graham, E. B.: Carbon Limitation Leads to Thermodynamic Regulation of Aerobic Metabolism, Environ. Sci. Technol. Let., 7, 517–524, https://doi.org/10.1021/acs.estlett.0c00258, 2020.
Gilbert, J. A., Jansson, J. K., and Knight, R.: Earth Microbiome Project and Global Systems Biology, mSystems, 3, e00217-17, https://doi.org/10.1128/mSystems.00217-17, 2018.
Gionchetta, G., Oliva, F., Romani, A. M., and Baneras, L.: Hydrological variations shape diversity and functional responses of streambed microbes, Sci. Total Environ., 714, 136838, https://doi.org/10.1016/j.scitotenv.2020.136838, 2020.
Goldman, A. E., Graham, E. B., Crump, A. R., Kennedy, D. W., Romero, E. B., Anderson, C. G., Dana, K. L., Resch, C. T., Fredrickson, J. K., and Stegen, J. C.: Biogeochemical cycling at the aquatic–terrestrial interface is linked to parafluvial hyporheic zone inundation history, Biogeosciences, 14, 4229–4241, https://doi.org/10.5194/bg-14-4229-2017, 2017.
Graham, E. B. and Stegen, J. C.: Dispersal-Based Microbial Community Assembly Decreases Biogeochemical Function, Processes, 5, 65, https://doi.org/10.3390/pr5040065, 2017.
Graham, E. B., Crump, A. R., Resch, C. T., Fansler, S., Arntzen, E., Kennedy, D. W., Fredrickson, J. K., and Stegen, J. C.: Coupling Spatiotemporal Community Assembly Processes to Changes in Microbial Metabolism, Front. Microbiol., 7, 1949, https://doi.org/10.3389/fmicb.2016.01949, 2016.
Graham, E. B., Tfaily, M. M., Crump, A. R., Goldman, A. E., Bramer, L. M., Arntzen, E., Romero, E., Resch, C. T., Kennedy, D. W., and Stegen, J. C.: Carbon Inputs From Riparian Vegetation Limit Oxidation of Physically Bound Organic Carbon Via Biochemical and Thermodynamic Processes, J. Geophys. Res.-Biogeo., 122, 3188–3205, https://doi.org/10.1002/2017JG003967, 2017a.
Graham, E. B., Crump, A. R., Resch, C. T., Fansler, S., Arntzen, E., Kennedy, D. W., Fredrickson, J. K., and Stegen, J. C.: Deterministic influences exceed dispersal effects on hydrologically-connected microbiomes, Environ. Microbiol., 19, 1552–1567, https://doi.org/10.1111/1462-2920.13720, 2017b.
Graham, E. B., Crump, A. R., Kennedy, D. W., Arntzen, E., Fansler, S., Purvine, S. O., Nicora, C. D., Nelson, W., Tfaily, M. M., and Stegen, J. C.: Multi 'omics comparison reveals metabolome biochemistry, not microbiome composition or gene expression, corresponds to elevated biogeochemical function in the hyporheic zone, Sci. Total Environ., 642, 742–753, https://doi.org/10.1016/j.scitotenv.2018.05.256, 2018.
Grilli, J., Barabás, G., Michalska-Smith, M. J., and Allesina, S.: Higher-order interactions stabilize dynamics in competitive network models, Nature, 548, 210–213, https://doi.org/10.1038/nature23273, 2017.
Hall, E. K., Bernhardt, E. S., Bier, R. L., Bradford, M. A., Boot, C. M., Cotner, J. B., del Giorgio, P. A., Evans, S. E., Graham, E. B., Jones, S. E., Lennon, J. T., Locey, K. J., Nemergut, D., Osborne, B. B., Rocca, J. D., Schimel, J. P., Waldrop, M. P., and Wallenstein, M. D.: Understanding how microbiomes influence the systems they inhabit, Nat. Microbiol., 3, 977–982, https://doi.org/10.1038/s41564-018-0201-z, 2018.
Homyak, P. M., Blankinship, J. C., Slessarev, E. W., Schaeffer, S. M., Manzoni, S., and Schimel, J. P.: Effects of altered dry season length and plant inputs on soluble soil carbon, Ecology, 99, 2348–2362, https://doi.org/10.1002/ecy.2473, 2018.
Jia, X., Dini-Andreote, F., and Falcão Salles, J.: Comparing the Influence of Assembly Processes Governing Bacterial Community Succession Based on DNA and RNA Data, Microorganisms, 8, 798, https://doi.org/10.3390/microorganisms8060798, 2020.
Jurburg, S. D., Nunes, I., Stegen, J. C., Le Roux, X., Priemé, A., Sørensen, S. J., and Salles, J. F.: Autogenic succession and deterministic recovery following disturbance in soil bacterial communities, Sci. Rep., 7, 1–11, https://doi.org/10.1038/srep45691, 2017.
Kaufman, M. H., Cardenas, M. B., Buttles, J., Kessler, A. J., and Cook, P. L. M.: Hyporheic hot moments: Dissolved oxygen dynamics in the hyporheic zone in response to surface flow perturbations, Water Resour. Res., 53, 6642–6662, https://doi.org/10.1002/2016WR020296, 2017.
Kearns, P. J., Angell, J. H., Howard, E. M., Deegan, L. A., Stanley, R. H. R., and Bowen, J. L.: Nutrient enrichment induces dormancy and decreases diversity of active bacteria in salt marsh sediments, Nat. Commun., 7, 12881, https://doi.org/10.1038/ncomms12881, 2016.
König, S., Worrich, A., Banitz, T., Centler, F., Harms, H., Kästner, M., Miltner, A., Wick, L. Y., Thullner, M., and Frank, K.: Spatiotemporal disturbance characteristics determine functional stability and collapse risk of simulated microbial ecosystems, Sci. Rep., 8, 1–13, https://doi.org/10.1038/s41598-018-27785-4, 2018.
Larned, S. T., Datry, T., Arscott, D. B., and Tockner, K.: Emerging concepts in temporary-river ecology, Freshwater Biol., 55, 717–738, https://doi.org/10.1111/j.1365-2427.2009.02322.x, 2010.
LaRowe, D. E. and Van Cappellen, P.: Degradation of natural organic matter: A thermodynamic analysis, Geochim. Cosmochim. Ac., 75, 2030–2042, https://doi.org/10.1016/j.gca.2011.01.020, 2011.
Leventhal, G. E., Ackermann, M., and Schiessl, K. T.: Why microbes secrete molecules to modify their environment: the case of iron-chelating siderophores, J. R. Soc. Interface, 16, 20180674, https://doi.org/10.1098/rsif.2018.0674, 2019.
Levy-Booth, D. J., Giesbrecht, I. J. W., Kellogg, C. T. E., Heger, T. J., D'Amore, D. V., Keeling, P. J., Hallam, S. J., and Mohn, W. W.: Seasonal and ecohydrological regulation of active microbial populations involved in DOC, CO2, and CH4 fluxes in temperate rainforest soil, ISME J., 13, 950–963, https://doi.org/10.1038/s41396-018-0334-3, 2019.
Li, Y., Gao, Y., Zhang, W., Wang, C., Wang, P., Niu, L., and Wu, H.: Homogeneous selection dominates the microbial community assembly in the sediment of the Three Gorges Reservoir, Sci. Total Environ., 690, 50–60, https://doi.org/10.1016/j.scitotenv.2019.07.014, 2019.
Lloyd-Price, J., Arze, C., Ananthakrishnan, A. N., Schirmer, M., Avila-Pacheco, J., Poon, T. W., Andrews, E., Ajami, N. J., Bonham, K. S., Brislawn, C. J., Casero, D., Courtney, H., Gonzalez, A., Graeber, T. G., Hall, A. B., Lake, K., Landers, C. J., Mallick, H., Plichta, D. R., Prasad, M., Rahnavard, G., Sauk, J., Shungin, D., Vázquez-Baeza, Y., White, R. A., Braun, J., Denson, L. A., Jansson, J. K., Knight, R., Kugathasan, S., McGovern, D. P. B., Petrosino, J. F., Stappenbeck, T. S., Winter, H. S., Clish, C. B., Franzosa, E. A., Vlamakis, H., Xavier, R. J., and Huttenhower, C.: Multi-omics of the gut microbial ecosystem in inflammatory bowel diseases, Nature, 569, 655–662, https://doi.org/10.1038/s41586-019-1237-9, 2019.
Louca, S., Polz, M. F., Mazel, F., Albright, M. B. N., Huber, J. A., O'Connor, M. I., Ackermann, M., Hahn, A. S., Srivastava, D. S., Crowe, S. A., Doebeli, M., and Parfrey, L. W.: Function and functional redundancy in microbial systems, Nat. Ecol. Evol., 2, 936–943, https://doi.org/10.1038/s41559-018-0519-1, 2018.
Malik, A. A., Martiny, J. B. H., Brodie, E. L., Martiny, A. C., Treseder, K. K., and Allison, S. D.: Defining trait-based microbial strategies with consequences for soil carbon cycling under climate change, ISME J., 14, 1–9, https://doi.org/10.1038/s41396-019-0510-0, 2020.
Manzella, M., Geiss, R., and Hall, E. K.: Evaluating the stoichiometric trait distributions of cultured bacterial populations and uncultured microbial communities, Environ. Microbiol., 21, 3613–3626, https://doi.org/10.1111/1462-2920.14684, 2019.
Manzoni, S., Schimel, J. P., and Porporato, A.: Responses of soil microbial communities to water stress: results from a meta-analysis, Ecology, 93, 930–938, https://doi.org/10.1890/11-0026.1, 2012.
Martínez, I., Stegen, J. C., Maldonado-Gómez, M. X., Eren, A. M., Siba, P. M., Greenhill, A. R., and Walter, J.: The Gut Microbiota of Rural Papua New Guineans: Composition, Diversity Patterns, and Ecological Processes, Cell Rep., 11, 527–538, https://doi.org/10.1016/j.celrep.2015.03.049, 2015.
Mauck, B. S. and Roberts, J. A.: Mineralogic Control on Abundance and Diversity of Surface-Adherent Microbial Communities, Geomicrobiol. J., 24, 167–177, https://doi.org/10.1080/01490450701457162, 2007.
McClain, M. E., Boyer, E. W., Dent, C. L., Gergel, S. E., Grimm, N. B., Groffman, P. M., Hart, S. C., Harvey, J. W., Johnston, C. A., Mayorga, E., McDowell, W. H., and Pinay, G.: Biogeochemical Hot Spots and Hot Moments at the Interface of Terrestrial and Aquatic Ecosystems, Ecosystems, 6, 301–312, https://doi.org/10.1007/s10021-003-0161-9, 2003.
Norland, S., Fagerbakke, K. M., and Heldal, M.: Light element analysis of individual bacteria by x-ray microanalysis, Appl. Environ. Microbiol., 61, 1357–1362, https://doi.org/10.1128/AEM.61.4.1357-1362.1995, 1995.
Ofiţeru, I. D., Lunn, M., Curtis, T. P., Wells, G. F., Criddle, C. S., Francis, C. A., and Sloan, W. T.: Combined niche and neutral effects in a microbial wastewater treatment community, Proc. Natl. Acad. Sci. USA, 107, 15345–15350, https://doi.org/10.1073/pnas.1000604107, 2010.
Pérez Castro, S., Cleland, E. E., Wagner, R., Sawad, R. A., and Lipson, D. A.: Soil microbial responses to drought and exotic plants shift carbon metabolism, ISME J., 13, 1776–1787, https://doi.org/10.1038/s41396-019-0389-9, 2019.
Prosser, J. I. and Martiny, J. B. H.: Conceptual challenges in microbial community ecology, Philos. T. R. Soc. B, 375, 20190241, https://doi.org/10.1098/rstb.2019.0241, 2020.
Ratzke, C., Denk, J., and Gore, J.: Ecological suicide in microbes, Nat. Ecol. Evol., 2, 867–872, https://doi.org/10.1038/s41559-018-0535-1, 2018.
Romaní, A. M., Vázquez, E., and Butturini, A.: Microbial Availability and Size Fractionation of Dissolved Organic Carbon After Drought in an Intermittent Stream: Biogeochemical Link Across the Stream–Riparian Interface, Microb. Ecol., 52, 501–512, https://doi.org/10.1007/s00248-006-9112-2, 2006.
Sengupta, A., Stegen, J. C., Neto, A. A. M., Wang, Y., Neilson, J. W., Tatarin, T., Hunt, E., Dontsova, K., Chorover, J., Troch, P. A., and Maier, R. M.: Assessing Microbial Community Patterns During Incipient Soil Formation From Basalt, J. Geophys. Res.-Biogeo., 124, 941–958, https://doi.org/10.1029/2017JG004315, 2019a.
Sengupta, A., Indivero, J., Gunn, C., Tfaily, M. M., Chu, R. K., Toyoda, J., Bailey, V. L., Ward, N. D., and Stegen, J. C.: Spatial gradients in the characteristics of soil-carbon fractions are associated with abiotic features but not microbial communities, Biogeosciences, 16, 3911–3928, https://doi.org/10.5194/bg-16-3911-2019, 2019b.
Shu, D., Guo, J., Zhang, B., He, Y., and Wei, G.: rDNA- and rRNA-derived communities present divergent assemblage patterns and functional traits throughout full-scale landfill leachate treatment process trains, Sci. Total Environ., 646, 1069–1079, https://doi.org/10.1016/j.scitotenv.2018.07.388, 2019.
Slater, L. D., Ntarlagiannis, D., Day-Lewis, F. D., Mwakanyamale, K., Versteeg, R. J., Ward, A., Strickland, C., Johnson, C. D., and Lane, J. W.: Use of electrical imaging and distributed temperature sensing methods to characterize surface water–groundwater exchange regulating uranium transport at the Hanford 300 Area, Washington, Water Resour. Res., 46, W10533, https://doi.org/10.1029/2010WR009110, 2010.
Song, H.-S., Stegen, J. C., Graham, E. B., Lee, J.-Y., Garayburu-Caruso, V. A., Nelson, W. C., Chen, X., Moulton, J. D., and Scheibe, T. D.: Representing Organic Matter Thermodynamics in Biogeochemical Reactions via Substrate-Explicit Modeling, bioRxiv, 2020.02.27.968669, https://doi.org/10.1101/2020.02.27.968669, 2020.
Starnawski, P., Bataillon, T., Ettema, T. J. G., Jochum, L. M., Schreiber, L., Chen, X., Lever, M. A., Polz, M. F., Jørgensen, B. B., Schramm, A., and Kjeldsen, K. U.: Microbial community assembly and evolution in subseafloor sediment, Proc. Natl. Acad. Sci. USA, 114, 2940–2945, https://doi.org/10.1073/pnas.1614190114, 2017.
Stegen, J. C., Lin, X., Konopka, A. E., and Fredrickson, J. K.: Stochastic and deterministic assembly processes in subsurface microbial communities, ISME J., 6, 1653–1664, https://doi.org/10.1038/ismej.2012.22, 2012.
Stegen, J. C., Lin, X., Fredrickson, J. K., Chen, X., Kennedy, D. W., Murray, C. J., Rockhold, M. L., and Konopka, A.: Quantifying community assembly processes and identifying features that impose them, ISME J., 7, 2069–2079, https://doi.org/10.1038/ismej.2013.93, 2013.
Stegen, J. C., Lin, X., Fredrickson, J. K., and Konopka, A. E.: Estimating and mapping ecological processes influencing microbial community assembly, Front. Microbiol., 6, 370, https://doi.org/10.3389/fmicb.2015.00370, 2015.
Stegen, J. C., Konopka, A., McKinley, J. P., Murray, C., Lin, X., Miller, M. D., Kennedy, D. W., Miller, E. A., Resch, C. T., and Fredrickson, J. K.: Coupling among Microbial Communities, Biogeochemistry and Mineralogy across Biogeochemical Facies, Sci. Rep., 6, 1–14, https://doi.org/10.1038/srep30553, 2016.
Stegen, J. C., Bottos, E. M., and Jansson, J. K.: A unified conceptual framework for prediction and control of microbiomes, Curr. Opin. Microbiol., 44, 20–27, https://doi.org/10.1016/j.mib.2018.06.002, 2018a.
Stegen, J. C., Johnson, T., Fredrickson, J. K., Wilkins, M. J., Konopka, A. E., Nelson, W. C., Arntzen, E. V., Chrisler, W. B., Chu, R. K., Fansler, S. J., Graham, E. B., Kennedy, D. W., Resch, C. T., Tfaily, M., and Zachara, J.: Influences of organic carbon speciation on hyporheic corridor biogeochemistry and microbial ecology, Nat. Commun., 9, 1–11, https://doi.org/10.1038/s41467-018-02922-9, 2018b.
Thompson, L. R., Sanders, J. G., McDonald, D., Amir, A., Ladau, J., Locey, K. J., Prill, R. J., Tripathi, A., Gibbons, S. M., Ackermann, G., Navas-Molina, J. A., Janssen, S., Kopylova, E., Vázquez-Baeza, Y., González, A., Morton, J. T., Mirarab, S., Zech Xu, Z., Jiang, L., Haroon, M. F., Kanbar, J., Zhu, Q., Jin Song, S., Kosciolek, T., Bokulich, N. A., Lefler, J., Brislawn, C. J., Humphrey, G., Owens, S. M., Hampton-Marcell, J., Berg-Lyons, D., McKenzie, V., Fierer, N., Fuhrman, J. A., Clauset, A., Stevens, R. L., Shade, A., Pollard, K. S., Goodwin, K. D., Jansson, J. K., Gilbert, J. A., and Knight, R.: A communal catalogue reveals Earth's multiscale microbial diversity, Nature, 551, 457–463, https://doi.org/10.1038/nature24621, 2017.
Tripathi, B. M., Stegen, J. C., Kim, M., Dong, K., Adams, J. M., and Lee, Y. K.: Soil pH mediates the balance between stochastic and deterministic assembly of bacteria, ISME J., 12, 1072–1083, https://doi.org/10.1038/s41396-018-0082-4, 2018.
Wagner, M.: Single-cell ecophysiology of microbes as revealed by Raman microspectroscopy or secondary ion mass spectrometry imaging, Annu. Rev. Microbiol., 63, 411–429, https://doi.org/10.1146/annurev.micro.091208.073233, 2009.
Wallenstein, M. D. and Hall, E. K.: A trait-based framework for predicting when and where microbial adaptation to climate change will affect ecosystem functioning, Biogeochemistry, 109, 35–47, https://doi.org/10.1007/s10533-011-9641-8, 2012.
Wang, J., Shen, J., Wu, Y., Tu, C., Soininen, J., Stegen, J. C., He, J., Liu, X., Zhang, L., and Zhang, E.: Phylogenetic beta diversity in bacterial assemblages across ecosystems: deterministic versus stochastic processes, ISME J., 7, 1310–1321, https://doi.org/10.1038/ismej.2013.30, 2013.
Whitman, T., Neurath, R., Perera, A., Chu-Jacoby, I., Ning, D., Zhou, J., Nico, P., Pett-Ridge, J., and Firestone, M.: Microbial community assembly differs across minerals in a rhizosphere microcosm, Environ. Microbiol., 20, 4444–4460, https://doi.org/10.1111/1462-2920.14366, 2018.
Wieder, W. R., Allison, S. D., Davidson, E. A., Georgiou, K., Hararuk, O., He, Y., Hopkins, F., Luo, Y., Smith, M. J., Sulman, B., Todd-Brown, K., Wang, Y.-P., Xia, J., and Xu, X.: Explicitly representing soil microbial processes in Earth system models, Global Biogeochem. Cy., 29, 1782–1800, https://doi.org/10.1002/2015GB005188, 2015.
Wisnoski, N. I., Muscarella, M. E., Larsen, M. L., Peralta, A. L., and Lennon, J. T.: Metabolic insight into bacterial community assembly across ecosystem boundaries, Ecology, 101, e02968, https://doi.org/10.1002/ecy.2968, 2020.
Wu, W., Lu, H.-P., Sastri, A., Yeh, Y.-C., Gong, G.-C., Chou, W.-C., and Hsieh, C.-H.: Contrasting the relative importance of species sorting and dispersal limitation in shaping marine bacterial versus protist communities, ISME J., 12, 485–494, https://doi.org/10.1038/ismej.2017.183, 2018.
Zachara, J. M., Long, P. E., Bargar, J., Davis, J. A., Fox, P., Fredrickson, J. K., Freshley, M. D., Konopka, A. E., Liu, C., McKinley, J. P., Rockhold, M. L., Williams, K. H., and Yabusaki, S. B.: Persistence of uranium groundwater plumes: Contrasting mechanisms at two DOE sites in the groundwater–river interaction zone, J. Contam. Hydrol., 147, 45–72, https://doi.org/10.1016/j.jconhyd.2013.02.001, 2013.
Zhou, J. and Ning, D.: Stochastic Community Assembly: Does It Matter in Microbial Ecology?, Microbiol. Mol. Biol. R., 81, e00002-17, https://doi.org/10.1128/MMBR.00002-17, 2017.
Zhou, J., Liu, W., Deng, Y., Jiang, Y.-H., Xue, K., He, Z., Nostrand, J. D. V., Wu, L., Yang, Y., and Wang, A.: Stochastic Assembly Leads to Alternative Communities with Distinct Functions in a Bioreactor Microbial Community, mBio, 4, e00584-12, https://doi.org/10.1128/mBio.00584-12, 2013.