the Creative Commons Attribution 4.0 License.
the Creative Commons Attribution 4.0 License.
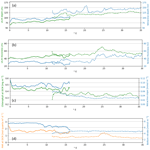
Deep chlorophyll maximum and nutricline in the Mediterranean Sea: emerging properties from a multi-platform assimilated biogeochemical model experiment
Giorgio Bolzon
Laura Feudale
Gianpiero Cossarini
Data assimilation has led to advancements in biogeochemical modelling and scientific understanding of the ocean. The recent operational availability of data from BGC-Argo (biogeochemical Argo) floats, which provide valuable insights into key vertical biogeochemical processes, stands to further improve biogeochemical modelling through assimilation schemes that include float observations in addition to traditionally assimilated satellite data. In the present work, we demonstrate the feasibility of joint multi-platform assimilation in realistic biogeochemical applications by presenting the results of 1-year simulations of Mediterranean Sea biogeochemistry. Different combinations of satellite chlorophyll data and BGC-Argo nitrate and chlorophyll data have been tested, and validation with respect to available independent non-assimilated and assimilated (before the assimilation) observations showed that assimilation of both satellite and float observations outperformed the assimilation of platforms considered individually. Moreover, the assimilation of BGC-Argo data impacted the vertical structure of nutrients and phytoplankton in terms of deep chlorophyll maximum depth, intensity, and nutricline depth. The outcomes of the model simulation assimilating both satellite data and BGC-Argo data provide a consistent picture of the basin-wide differences in vertical features associated with summer stratified conditions, describing a relatively high variability between the western and eastern Mediterranean, with thinner and shallower but intense deep chlorophyll maxima associated with steeper and narrower nutriclines in the western Mediterranean.
- Article
(2090 KB) - Full-text XML
- BibTeX
- EndNote
In recent years, biogeochemical modelling has significantly contributed to the knowledge of key aspects of marine ecosystem processes at both local and global scales (Fennel et al., 2019). While quality assessment advancements have improved our confidence in model results (Hipsey et al., 2020), intrinsic limitations still exist because of unrepresented processes and uncertainty in parameterization and numerical approximation (Dowd et al., 2014). On the other hand, emerging observation systems have provided valuable information on the biogeochemical state and processes in the ocean (Chai et al., 2020; Claustre et al., 2020; Groom et al., 2019; Muller-Karger et al., 2018; Roemmich et al., 2019). However, observations can be sparse and unevenly distributed in time and space (in situ), limited to the ocean surface (satellite remote sensing) and generally affected by calibration and measurement errors (Bittig et al., 2019; Xing et al., 2020). Data assimilation (DA) aims to increase knowledge and representation of processes by integrating models with information obtained from observations.
In ocean biogeochemical modelling, the assimilation of satellite ocean-colour observations has been successfully applied in research and operational applications at both global and regional scales (Fennel et al., 2019; Groom et al., 2019). Chlorophyll concentration is the most commonly assimilated variable since the first applications of ocean biogeochemical DA (Ciavatta et al., 2016; Dorofeyev and Sukhikh, 2018; Ford and Barciela, 2017; Ford, 2020; Gehlen et al., 2015; Mattern et al., 2017; Pradhan et al., 2019; Ratheesh et al., 2016; Santana-Falcón et al., 2020; Song et al., 2016; Teruzzi et al., 2018; Tsiaras et al., 2017). However, assimilation of the ocean-colour diffuse attenuation coefficient, phytoplankton functional types, particulate organic carbon, and inherent optical properties has been suggested as promising alternative to chlorophyll assimilation (Ciavatta et al., 2019, 2018, 2014; Dutkiewicz et al., 2019; Jones et al., 2016; Pradhan et al., 2020; Shulman et al., 2013; Skákala et al., 2018; Xiao and Friedrichs, 2014).
Ocean-colour observation assimilation takes advantage of the frequent, large-scale satellite observations related to the microbial biology of the upper ocean. However, the information includes the ocean surface layers only. In the context of data assimilation, the application of this information to deeper ocean layers requires approximations and assumptions. Vertical covariance must be parameterized by synthetic precalculated vertical profiles in variational schemes (Teruzzi et al., 2018), while EnKF-like (ensemble Kalman filter) schemes may have limitations in effectively impacting deeper ocean layers (Fontana et al., 2013; Hu et al., 2012). Indeed, some EnKF-like applications introduce limitation to the increments in subsurface layers through localization in the vertical direction to address spurious correlations (Goodliff et al., 2019; Pradhan et al., 2019).
In situ observations provide information on processes occurring in the ocean interior (e.g. deep chlorophyll maximum, vertical fluxes of nutrients or organic matter). An operational framework of biogeochemical observations has been recently introduced by BGC-Argo (biogeochemical Argo) floats, which routinely deliver biogeochemical observations for the open sea (typically chlorophyll, oxygen, nitrate concentrations, optical properties, and pH) with a profiling frequency ranging from 5 to 10 d (Bittig et al., 2019; Chai et al., 2020; Claustre et al., 2020; D'Ortenzio et al., 2020; Organelli et al., 2017). Data from BGC-Argo floats provide valuable insights into key vertical biogeochemical processes, such as the seasonal progression of stratified and mixed conditions and their impacts on the dynamics of phytoplankton and nutrients (e.g. Barbieux et al., 2019; D'Ortenzio et al., 2020, 2014; Pasqueron de Fommervault et al., 2015; Lavigne et al., 2013; Mayot et al., 2017; Mignot et al., 2014). The first examples of the assimilation of float observations into biogeochemical models have improved the estimates of the vertical variability in biogeochemical variables (Cossarini et al., 2019; Verdy and Mazloff, 2017). Moreover, the potential benefits of integrating BGC-Argo observations with satellite data and biogeochemical modelling have been demonstrated by recent observing system simulation experiments (OSSEs) and parameter optimization studies (Ford, 2021; Germineaud et al., 2019; Wang et al., 2020).
In the present work, we demonstrate the feasibility of joint multi-platform assimilation in realistic biogeochemical applications by presenting the results of 1-year simulations of Mediterranean Sea biogeochemistry using the MedBFM (Mediterranean biogeochemical flux model) system that includes the OGSTM and the low-trophic-level BFM (Salon et al., 2019) offline coupled with the NEMO-OceanVar Mediterranean Forecasting System (MFS) model (Oddo et al., 2014, 2009). Different combinations of satellite chlorophyll data and BGC-Argo nitrate and chlorophyll data have been assimilated using an upgraded version of the 3DVarBio variational assimilation scheme that was previously applied in single-platform assimilation (Cossarini et al., 2019; Teruzzi et al., 2019, 2018, 2014). The relatively high number of deployed BGC-Argo floats in the Mediterranean Sea and the specific seasonal variability in phytoplankton–nutrient dynamics, and the noticeable west–east gradient of the deep chlorophyll maximum (DCM) depth of the Mediterranean Sea (Lavigne et al., 2013) make this basin a suitable location for the implementation and verification of a multi-platform assimilation system. The simulations carried out in the present work have been validated with respect to available assimilated and non-assimilated (before the assimilation) observations and have been investigated in terms of assimilation impact. Moreover, since a good simulation skill was demonstrated by the validation, the model outcomes have been used to explore the basin-wide differences in the vertical features associated with summer stratified conditions when the DCM and nutricline are well established over the whole Mediterranean Sea (Barbieux et al., 2019; Lavigne et al., 2013; Lazzari et al., 2012; Mignot et al., 2014).
Section 2 describes the observation datasets used for the assimilation and the biogeochemical model and assimilation scheme set-up. The results and discussion are provided in Sects. 3 and 4, respectively.
The Mediterranean Sea biogeochemistry was simulated for 1 year (2015) with four different assimilation set-ups and a reference run without assimilation using the MedBFM model system that is operationally implemented in the Copernicus Marine Environment Monitoring Service (CMEMS) and provides nominal biogeochemical products for the Mediterranean Sea (Bolzon et al., 2020; Salon et al., 2019).
2.1 Observations
2.1.1 Satellite chlorophyll
The surface chlorophyll data used for assimilation included both open-sea and coastal observations (Teruzzi et al., 2018) and were obtained from the satellite multi-sensor product OCEANCOLOUR_MED_CHL_L3_NRT_OBSERVATIONS_009_ 040 (i.e. a merged product of MODIS-AQUA, NOAA20-VIIRS, NPP-VIIRS, and Sentinel3A-OLCI sensors; https://resources.marine.copernicus.eu/?option=com_csw&view=details&product_id=OCEANCOLOUR_MED_CHL_L3_NRT_OBSERVATIONS_009_040, (last access: 17 November 2021). Original products, provided at a daily frequency and a horizontal spatial resolution of 1 km, were weekly averaged and interpolated at the model grid resolution to be used in the assimilation and validation following the strategy previously implemented in Teruzzi et al. (2014, 2018). Two levels of quality check were performed on observations. The first quality check was made independently from data assimilation and consisted in removing observations whose anomalies were higher than 3 times the daily climatology standard deviation in order to remove spikes. A second pre-data-assimilation check rejected satellite observations not suitable for assimilation, excluding observations whose mismatch value with respect to the model was higher than 10 mg m−3 to keep the range of model–observation differences consistent with the assumed uncertainty levels. The threshold was calibrated by a statistical analysis of the model–observation mismatches of the REF (reference simulation without assimilation) run. Through the pre-assimilation criterion, about 2 % of satellite chlorophyll values were considered not suitable for the assimilation and rejected. With the described satellite chlorophyll data pre-processing, the yearly mean weekly coverage of surface chlorophyll was equal to 91 % over the Mediterranean Sea with coverage higher than 95 % in some sub-basins (ALB, SWM1, NWM, TYR1, ION1, and LEV2; names of sub-basins provided in Fig. 1). As expected, the highest coverage occurred in summer (nearly 95 % between May and August).
2.1.2 BGC-Argo floats
The BGC-Argo data used in the present study included vertical profiles of chlorophyll, nitrate, and oxygen. The data were obtained from the Coriolis Data Assembly Centre and processed with the BGC-Argo community procedures (Bittig et al., 2019; Johnson et al., 2018; Schmechtig et al., 2018, 2015; Thierry et al., 2018). As for satellite observations, two levels of quality check were applied on float observations. In the first check, which excluded unrealistic values, an expert judgement procedure was applied because the relative novelty of BGC-Argo datasets may lead to a higher occurrence of poorly reliable observations. In particular, nitrate profiles were rejected if the surface value was higher than 3 mmol m−3, chlorophyll profiles were checked for negative values (rejection of single negative observations), and quenching correction was performed by imposing a constant chlorophyll value in the mixed layer. The exclusion of nitrate values higher than 3 mmol m−3 in the surface layers was based on the analysis of climatological values provided in the EMODnet dataset (Buga et al., 2018). In the pre-data-assimilation check (second quality check phase) observations were rejected when the mismatch with respect to the model was higher than 5 mg m−3 and 2 mmol m−3 for chlorophyll and nitrate, respectively. As for satellite, these values were based on a statistical analysis of the model–observation mismatches in REF. The pre-assimilation check excluded nearly 3 % and less than 1 % of the float nitrate and chlorophyll observations, respectively. The number of profiles used for assimilation and validation were 1484 for chlorophyll, 718 for nitrate, and 794 for oxygen. Float profiles covered the whole Mediterranean Sea but with a larger sampling in the western regions than in the other regions (Fig. 1). In particular, the southern part of the Ionian Sea lacks float measurements. Since BGC-Argo floats are focused on and designed to operate in pelagic areas, shallow basins (northern Adriatic and Aegean seas) were not sampled.
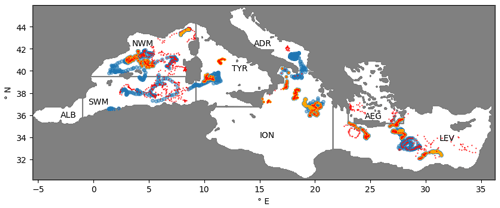
Figure 1Positions of BGC-Argo floats equipped with sensors to provide chlorophyll (blue), nitrate (orange), and oxygen (red) concentrations and limits of the sub-basins: Alboran (ALB), southwestern Mediterranean (SWM), northwestern Mediterranean (NWM), Tyrrhenian (TYR), Ionian (ION), Adriatic (ADR), Aegean (AEG), and Levantine (LEV) seas.
2.2 Model system
The MedBFM system used in this study is the biogeochemical component of the Mediterranean CMEMS model system aimed at providing short-term forecasts and multi-annual reanalysis, and it consists of the coupled physical–biogeochemical OGSTM-BFM model and the 3DVarBio assimilation scheme (Salon et al., 2019). The OGSTM-BFM is designed with a transport model (OGSTM) and a biogeochemical reactor featuring the biogeochemical flux model (BFM). The OGSTM transport model, which is a modified version of the OPA 8.1 transport model (Lazzari et al., 2010), was recently upgraded to resolve the free surface and variable volume-layer effects on the transport of tracers and is fully consistent with the off-line coupling of the NEMO3.2 version (Salon et al., 2019). The BFM is a medium-complexity low-trophic-level ecosystem model designed to describe energy and material fluxes through both “classical food-chain” and “microbial food-web” pathways (Thingstad and Rassoulzadegan, 1995). This model includes nine plankton functional types (four phytoplankton, four zooplankton, and one bacteria) and takes into account the co-occurring effects of multi-nutrient interactions (Lazzari et al., 2012). The BFM was recently developed with a carbonate system (Cossarini et al., 2015) and revised phytoplankton nutrient uptake processes (Lazzari et al., 2016). Moreover, the BFM has been implemented in the Mediterranean Sea in a wide range of applications, including 1D and 3D configurations aimed at studying the interaction between optics and biogeochemistry (Terzić et al., 2021, 2019), conducting climate scenario simulations (Lazzari et al., 2014), estimating carbon sequestration services (Melaku Canu et al., 2015), simulating high-resolution coastal dynamics in marginal seas (Cossarini et al., 2017), analysing temporal scales of multi-decadal variability (Di Biagio et al., 2019), and studying CDOM (chromophoric-dissolved organic matter) spatiotemporal variability (Lazzari et al., 2021). 3DVarBio is the data assimilation scheme for the correction of phytoplankton biomass and nutrient concentrations (i.e. nitrate and phosphate) using surface chlorophyll from satellite observations and vertical profiles of chlorophyll and nitrate from the BGC-Argo floats.
Model set-up
In the current application, the MedBFM was forced offline with outputs from the NEMO3.2 model of the Mediterranean CMEMS model system (Simoncelli et al., 2016), which provides daily mean physical dynamics (i.e. horizontal and vertical current velocities, vertical eddy diffusivity, potential temperature, salinity, and sea surface height in addition to surface data for solar shortwave irradiance and wind stress). The mesh grid was based on a ∘ longitudinal scale factor and a ∘ cos (φ) latitudinal scale factor. The vertical mesh grid accounted for 70 vertical z levels: 25 in the first 200 m of depth, 31 between 200 and 2000 m, and 14 below 2000 m.
Initial conditions were provided by a 2-year spin-up simulation forced by 2015 physical fields in perpetual mode. The 2-year spin-up was initialized by profiles of biogeochemical variables as provided by the EMODnet_int climatology, which merges the in situ EMODnet data collections (Buga et al., 2018) and the datasets listed in Lazzari et al. (2016) and Cossarini et al. (2015). In the Atlantic area (i.e. west of the Strait of Gibraltar to the 9∘ longitude) tracer concentrations were relaxed to seasonally varying climatological profiles. Seasonal profiles of phosphate, nitrate, silicate, and dissolved oxygen were derived from an analysis of the climatological World Ocean Atlas 2018 data (Garcia et al., 2019) and the EMODnet_int dataset. The biogeochemical open-boundary conditions at the Dardanelles strait were obtained through a Dirichlet-type scheme that uses climatological values from the literature (Salon et al., 2019). Atmospheric deposition rates of inorganic nitrogen and phosphorus were set according to the synthesis proposed by Ribera d'Alcalà et al. (2003), while terrestrial inputs of nutrients (nitrogen and phosphorous) from 39 rivers were obtained from the PERSEUS FP7-287600 project dataset (deliverable D4.6, http://www.perseus-net.eu/assets/media/PDF/deliverables/3321.6_Final.pdf, (last access: 17 November 2021). The terrestrial nutrient discharge rates were climatological (average of the 2000–2015 period) and took into account seasonal variability at a monthly scale based on varying monthly water discharge.
2.3 3D variational assimilation
In 3DVarBio, assimilation is performed through the minimization of a cost function that is defined on the basis of Bayes' theorem (Lorenc, 1986) as the weighted sum of the square mismatches between the model background state xb (the model state before the assimilation) and the analysis xa (the assimilation result) and the observations y. Each square mismatch is weighted according to its accuracy estimations, meaning that xa−xb is weighted by the background error covariance matrix B, while (y−H(xb)) is weighted by the observation error covariance matrix R:
In Eq. (1) y−H(xb) is usually named innovation and H the observational operator that maps the values of the model background state xb in the observation space. In our application H(xb) are model values of the variables observed by satellite or floats at observation locations. Through the minimization of the cost function 1, the assimilation provides the analysis xa, i.e. the optimal weighted distance from both y and xb. According to Weaver et al. (2003) and Dobricic and Pinardi (2008), the solution of the assimilation step (i.e. the increment xa−xb) is calculated by defining a control variable v and a transformation matrix V such that and B=VVT. Moreover, the matrix V, which transforms increments from the control space to the model space, is decomposed into a sequence of operators that characterize different aspects of the error covariances: the vertical error covariance (VV), the horizontal error covariance (VH), and the biogeochemical state variable error covariance (VB) (Teruzzi et al., 2014). Recent developments of the scheme include the upgrade of VH to become anisotropic to address the assimilation of satellite coastal observations (Teruzzi et al., 2018), the upgrade of H and VV to address the assimilation of BGC-Argo float profiles (Cossarini et al., 2019), and the parallel recoding of the horizontal filter applied in VH (Teruzzi et al., 2019). In the present study, the 3DVarBio assimilation scheme was adapted to assimilate float nitrate data and both satellite and float chlorophyll data and to provide corrections on all the phytoplankton variables and on nitrate and phosphate concentrations. In particular, in addition to the covariance between the assimilated chlorophyll and the 17 BFM variables describing the phytoplankton functional types (Teruzzi et al., 2014), the VB operator now includes the covariance between nitrate and phosphate calculated on a validated 20-year simulation of the MedBFM system (Teruzzi et al., 2016). Observation error covariance matrix R has been assumed to be diagonal, and it is unchanged with respect to previous applications for satellite and float chlorophyll observations (Cossarini et al., 2019; Teruzzi et al., 2018), while a uniform and constant error has been assigned to float nitrate observations (0.24 mmol m−3) according to triple-collocation error estimates (Mignot et al., 2019).
2.3.1 Assimilation set-ups
Provided that single-sensor chlorophyll data assimilation has already been demonstrated (Teruzzi et al., 2018; Cossarini et al., 2019), satellite (Sc) and float (Fc) chlorophyll data assimilation simulations in 2015, together with a reference simulation without assimilation (REF), were used as benchmarks to evaluate the relative improvement in the joint data assimilation simulations of float chlorophyll and nitrate (Fcn) observations and float nitrate and both float and satellite chlorophyll observations (ScFcn; Table 1). Oxygen profiles, available from the BGC-Argo floats, were not assimilated but were used as independent observations for validation.
The weekly averaged maps of satellite chlorophyll concentration (Sect. 2.1.1) were assimilated once per week, while the assimilation of available vertical in situ profiles of chlorophyll and nitrate concentrations (Sect. 2.1.2) was performed every day. When satellite and float observations were both available, the assimilation was performed separately with satellite information assimilation occurring first since the different data densities impacted the effectiveness of the multi-platform assimilation. Through the VB operator of 3DVarBio, the assimilation of chlorophyll data provided increments for the four phytoplankton functional groups (17 state variables) for chlorophyll data assimilation, as well as for nitrate and phosphate for nitrate data assimilation. The application of simultaneous increments of nitrate and phosphate was a key element in the Mediterranean Basin, where both nutrients can act as limiting factors of phytoplankton growth (Lazzari et al., 2016). Adopting a conservative approach, other nutrients were not updated by DA because of their less relevant role as a limiting factor in the Mediterranean Sea.
Table 1Assimilation set-ups and names assigned to the simulations. Assimilated variables can be satellite chlorophyll (Sat CHL), float chlorophyll (Float CHL), and float nitrate (Float NIT). DA updates can be applied to the 17 phytoplankton variables (phyto) and to nitrate and phosphate concentrations (nutrients). The covariance between biogeochemical variables applied by VB is that between chlorophyll and phytoplankton variables (CHL-Phyto) and between nitrate and phosphate (NIT-PHO).
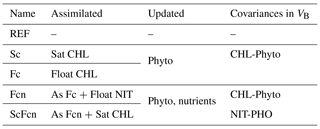
3.1 Validation with observations
The performance of the different assimilation set-ups (Table 1) was evaluated by comparing available assimilated observations with model outputs before the assimilation and non-assimilated observations with daily mean model outputs. The root mean square difference (RMSD) in four macro sub-basins and at eight different layers (Fig. 1 and Table 2) was calculated for the BGC-Argo observations and in two seasons for the surface satellite chlorophyll observations.
3.1.1 Validation with the BGC-Argo floats
Considering the BGC-Argo float chlorophyll data, the RMSD values of the REF simulation were lower in the eastern sub-basins than in the other sub-basins, with values higher than 0.1 mg m−3 only in the NWM sub-basin (Fig. 2). In general, subsurface layers had a higher RMSD than the surface with a west–east increase in the depth of the maximum RMSD, which matches the west–east gradient of the deep chlorophyll maximum (DCM) in the Mediterranean Sea. The assimilation of satellite chlorophyll (Sc) data alone did not clearly affect the model skill with respect to float chlorophyll data. Indeed, the RMSD values with respect to the REF decreased by almost 10 % in the surface layers of ION and LEV and increased by almost 10 %–15 % in TYR and in the subsurface L4 layer of ION and LEV.
On the other hand, and as expected, the assimilation of float chlorophyll data significantly reduced the RMSD values with respect to chlorophyll in all sub-basins in the Fc, Fcn, and ScFcn simulations. RMSD reductions occurred especially in layers with the highest RMSD in the REF simulation, ranging between −13 % and −20 %, with the highest values in the western sub-basins. Differences in RMSD reductions between the Fc and Fcn simulations were not appreciable, meaning that the assimilation of nitrate did not decrease the benefit of float chlorophyll data assimilation. The joint assimilation of satellite chlorophyll data and float chlorophyll data and nitrate data caused variations in the RMSD with respect to float chlorophyll data that were both positive and negative according to the effect on RMSD with satellite chlorophyll data assimilation alone. The resulting RMSDs were lower or slightly higher than the REF RMSDs everywhere, with the unique exception of layer L4 in LEV.
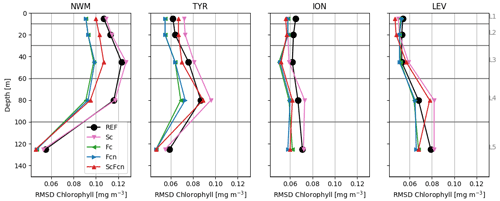
Figure 2RMSD between model simulations and BGC-Argo chlorophyll values in four sub-basins (Fig. 1). Grey lines indicate the limits of layers L1–L5 (Table 2) used to calculate the chlorophyll RMSD.
Higher RMSD values with respect to float nitrate values (Fig. 3) were registered in the subsurface and deep layers (L5–L8) of the REF simulation in all the sub-basins except NWM. The RMSD values increased with depth following the vertical increase in nitrate concentration (Salon et al., 2019), while the RMSD values higher than 1 mmol m−3 in the L1 and L2 layers in the NWM were related to an overestimation of surface nitrate during summer. Satellite data assimilation in the Sc simulation impacted nitrate indirectly through model dynamics after the DA increments in phytoplankton biomass, resulting in lower RMSD values with respect to REF in the surface layers (L1–L4) in the NWM (−20 %) and the opposite in the TYR (+20 %). When float data were assimilated, a 5 % reduction in nitrate RMSD occurred in the surface layers in the NWM and TYR in the Fc simulation, while a general reduction of up to 30 % in several layers in the western sub-basins and up to 20 % in ION and LEV was achieved with the assimilation of nitrate profiles in both the Fcn and ScFcn simulations.
Considering the joint assimilation in ScFcn, it is worth noting that an increase in the nitrate RMSD in L3 in the TYR resulted from the superimposition of the increase due to satellite chlorophyll data assimilation and the reduction due to float nitrate data assimilation. In general, the effects on RMSD with respect to nitrate in the joint satellite–float assimilation showed that the RMSD variations were almost additive: the RMSD reductions in the Sc simulation were reflected in more intense reductions in ScFcn than those in the Fcn simulation, while the opposite occurred in the case of the RMSD increases in the Sc simulation.
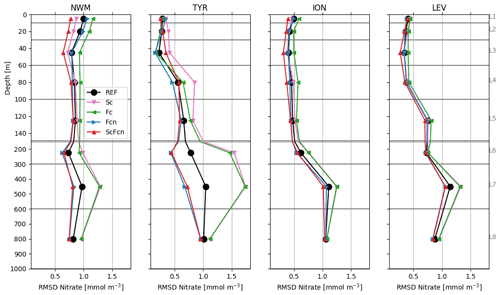
Figure 3RMSD between model simulations and BGC-Argo nitrate data in four sub-basins (Fig. 1). Grey lines indicate the limits of layers L1–L8 (Table 2) used to calculate the RMSD. The depth scale is different above and below 150 m (thick grey line).
The RMSD between the float oxygen data and REF simulation (Fig. 4) increased from the surface (approximately 5–15 mmol m−3) to the subsurface and deeper layers (approximately 15–20 mmol m−3) in the NWM and TYR sub-basins, while it was almost uniform along the vertical in the eastern sub-basins with ranges of 2–10 and 7–17 mmol m−3 in ION and LEV, respectively. In particular, RMSDs very slightly differed among simulations, especially in the eastern sub-basins. In particular, the float assimilation had very little effect on oxygen RMSDs, and the effect of satellite chlorophyll assimilation is not univocal since RMSDs are both slightly reduced (TYR) and increased (NWM) in the Sc simulation. On the other hand, the float assimilation had very little effect on oxygen RMSDs.
3.1.2 Validation with satellite chlorophyll data
The RMSDs with respect to the satellite chlorophyll data (Fig. 5) in the REF simulation ranged between 0.07 and 0.11 mg m−3 in winter and between 0.013 and 0.035 mg m−3 in summer, consistent with the seasonal variation in the surface chlorophyll concentration in the Mediterranean Sea. Assimilation of float data alone had negligible effects on the RMSD calculated with satellite data (i.e. RMSD reductions between 0 % and 3 % in all the sub-basins except SWM in Fcn), whereas the assimilation of satellite chlorophyll data in the Sc and ScFcn simulations significantly reduced the satellite chlorophyll RMSD with a gradient of reduction intensity from west to east. In fact, relative reductions were more intense than or close to 50 % in ION and LEV and almost equal to 20 % and 30 % in SWM in winter and summer, respectively.
The skill performance analysis (Figs. 2–5) clearly showed that joint assimilation of satellite chlorophyll data and float chlorophyll and nitrate data (ScFcn simulation) significantly reduced the RMSD with respect to all the assimilated variables and did not induce any degradation of float oxygen observations, outperforming all the simulations with single-stream assimilation.
3.2 Data assimilation impact
Several factors (e.g. the number and position of available observations, the innovation values, the spatial covariances, and those between the biogeochemical variables) influence the spatial extension and temporal persistence of the assimilation impact on biogeochemical dynamics. Several methods have been tested to measure the impact of observations on models, including conducting OSSEs (e.g. Ford, 2021; Germineaud et al., 2019) and introducing data impact indicators (e.g. Raicich and Rampazzo, 2003). To assess the impact of satellite and BGC-Argo float observations, the following indicator was evaluated:
where ScFcn(t) and REF(t) indicate results at date t of the ScFcn and REF simulations, respectively, and |ScFcn(t)−REF(t)| is their absolute difference. The subscript 200 represents the integral over the 0–200 m layer, while the overbar represents the average over the whole Mediterranean and over seasonal periods. The impact indicator Ixy(t) was calculated for each assimilation date and each grid point and was then statistically analysed and summarized on a seasonal basis. The indicator Ixy(t) quantifies how much an assimilated run deviates from the REF simulation; thus it is higher where and when the simulation deviates from REF and is closer than REF to the assimilated observations. The seasonal median of the impact index values masked scattered assimilations (in particular in summer for chlorophyll and in winter for nitrate; Figs. 6 and 7) showing that satellite data, given their high observational density, almost always had a relevant impact over the whole basin. On the other hand, the 95th percentile of the Ixy(t) distribution allowed us to highlight the areas where at least one assimilation markedly reduced the model mismatch.
In almost the whole (i.e. 97 %) Mediterranean Sea the Ixy(t) 95th percentile for chlorophyll concentration was greater than 0.3 in winter (Fig. 6), while in summer, it was greater than 0.3 % in 26 % of the Mediterranean Sea and mainly in areas surrounding the float trajectories (Fig. 6, bottom map). The impact indicator calculated for the single data-stream assimilation runs (Fcn and Sc, not shown) confirmed that summer local DA effects were mainly due to float assimilation, while the relevant impact of ScFcn over the whole basin in winter was mainly related to satellite chlorophyll data assimilation.
Chlorophyll profiles averaged over the areas with Ixy(t) 95th percentiles higher than 0.3 for REF and ScFcn revealed that DA impacts were different in winter and summer (Fig. 6, left panels). In winter, when impacts were mainly related to satellite observations, assimilation generally induced chlorophyll concentration reductions that were vertically homogeneous in the surface layer and then vanished almost at the bottom of the euphotic layer. On the other hand, the float-related assimilation impacts of summer were vertically localized around DCM and affected its depth and intensity.
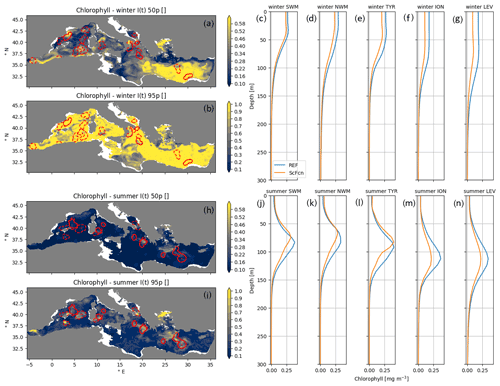
Figure 6Maps of Ixy(t) 50th and 95th percentiles for chlorophyll in winter and summer (a, b, h, and i); red contours identify the areas within three correlation radii from the float positions (dashed lines for floats without nitrate observations). Mean profiles at time and location with Ixy(t) higher than 0.3 in five sub-basins for the ScFcn and REF simulations (c, d, e, f, g, j, k, l, m, and n) in winter (c–g) and summer (j–n).
The data assimilation impact on the nitrate 3D field was less intense than that for chlorophyll, and relatively high values of I(t) were located mainly in float trajectory convolution areas (Fig. 7 maps). Satellite and float chlorophyll data in both single-stream and joint assimilation (not shown) had relatively low impacts on nitrate, and local patches of large assimilation impacts occurred mainly where float nitrate data were assimilated.
Analysing the seasonal distribution of the spatial data impact, the Ixy(t) 95th percentile was higher than 0.3 only in 3 % of the Mediterranean Sea in winter, while the percentage increased to 12 % in summer. Moreover, in summer, the Ixy(t) 95th percentile was slightly higher in the basin outside the areas affected by float observations. The seasonal differences in the Ixy(t) spatial distribution were due to two concurring effects: the lower number of floats equipped with nitrate sensors in winter and the persistence of nitrate changes, as indicated by the enhancement in Ixy(t) spatial homogeneity over time (not shown).
Data assimilation impacts on nitrate profile shapes (Fig. 5) were nearly homogeneous along the vertical with negligible effects on nitracline depth and slope in ION in winter (positive ScFcn and REF differences), in NWM in winter, and in ION and LEV in summer (negative ScFcn and REF differences). In contrast, nitracline depth and slope were significantly affected by vertically non-uniform assimilation impacts (e.g. NWM and TYR in summer). Below 300 m (not shown), differences between REF and ScFcn propagate nearly constantly until 500 m and then tend to vanish between 500 and 600 m.
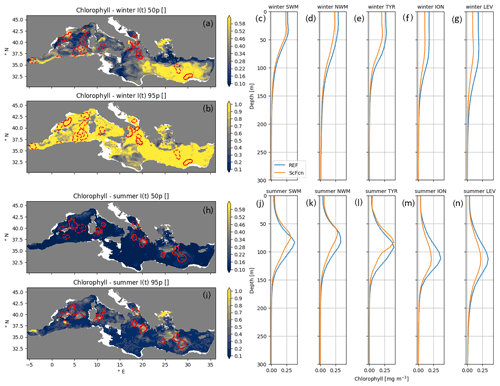
Figure 7Maps of the Ixy(t) 50th and 95th percentiles for nitrate in winter and summer (a, b, h, and i); red contours identify the areas within three correlation radii from the float position (dashed lines for floats without nitrate observations). Mean profiles at time and location with Ixy(t) higher than 0.3 in five sub-basins for the ScFcn and REF simulations (c, d, e, f, g, j, k, l, m, and n) in winter (c–g) and summer (j–n).
3.3 Nitracline and deep chlorophyll maximum
The good skill of the analysis simulation that integrates satellite and BGC-Argo observations to reproduce 3D fields of phytoplankton and nutrients (ScFcn simulation) supported the use of the simulation outputs to investigate some basin-wide biogeochemical features in summer, when processes in the vertical direction are dominated by stratified conditions. In particular, deep chlorophyll maximum (DCM) and nutricline dynamics were investigated by calculating several indexes that identified the vertical displacement and the profile shape (Table 5) and provided the representation of their west-to-east variability in the Mediterranean Sea during summer (Figs. 8 and 9).
Consistent with previous findings (Lavigne et al., 2013; Lazzari et al., 2012; Mignot et al., 2014), the simulated DCM depth exhibited a west–east gradient (Fig. 8) ranging from nearly 80 m in the western basins to values higher than 100 m in the eastern basins. Moreover, in the ScFcn simulation, the DCM and DBM (deep phytoplankton biomass maximum) depths mostly coincided with a correlation coefficient higher than 0.9. The DCM thickness and the chlorophyll concentration at the DCM also showed spatial gradients over the Mediterranean Sea (Fig. 9): moving eastward, as the DCM deepens, the DCM thickness increases, while its intensity (chlorophyll concentration at DCM) decreases.
According to the paradigm that the DBM is a layer where both light and nutrients are co-limiting factors for phytoplankton (Cullen, 2015), the DCM and nitracline depths were spatially correlated in the Mediterranean Sea (Fig. 9). Indeed, similar to the DCM depth, the nitracline depth showed a west-to-east gradient, and given the proposed definitions (Table 5), the nitracline was located slightly above the DCM, with a nearly constant 15 m gap. As shown for the DCM, moving eastward, the nitracline thickness varied in the same way as its depth. Indeed, the nitracline thickness was higher in the eastern (50 m) than in the western sub-basins (30 m; Fig. 9). The nitracline slope gradient exhibited an opposite sign moving eastward, with values ranging between 0.08–0.12 and 0.02–0.5 mmol m−4 in the western and eastern Mediterranean, respectively (Fig. 9). Even if the Mediterranean Sea is a small marginal sea, it exhibits a wide range of summer vertical conditions: a 25 % thinner and shallower but intense DCM associated with a steeper (more than double) and 33 % narrower nitracline in the western Mediterranean than in the more oligotrophic eastern Mediterranean.
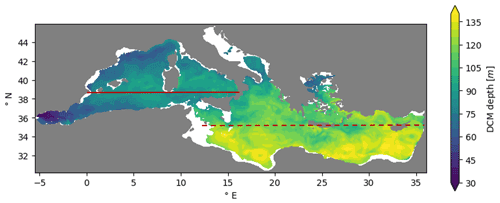
Figure 8Mean DCM depth [m] calculated over summer in areas with water depths lower than 200 m. Western and eastern meridional averages of Fig. 9 are calculated along red continuous and dashed lines, respectively, excluding the Adriatic (ADR) and Aegean (AEG) seas.
The simulated values of PAR (photosynthetically active radiation) at the DCM depth (Fig. 9) ranged between 1.5 and 2 mol quanta m−2 d−1 moving westward from the Sicilian Channel and between 0.6 and 1 mol quanta m−2 d−1 in the eastern Mediterranean, with higher PAR-at-DCM values occurring between 24 and 26∘ E only. The west-to-east decreasing values of PAR at DCM are documented in a study based on BGC-Argo observation (Mignot et al., 2014), even if the reported values were slightly lower than in Fig. 9.
The DBM can be considered a nutrient trap layer where all the nutrient fluxes from the bottom layer are consumed by phytoplankton. Thus, since it is confined upward by nutrient depletion and downward by the absence of light, higher irradiance at the DBM (equivalent to DCM in our case) can indicate a higher rate of nutrient uptake by phytoplankton and related higher production (Cullen, 2015). In our results, this hypothesis was confirmed by higher nutrient uptakes in the western sub-basins than in the eastern sub-basins at the DCM depth: the nitrate and phosphate uptake ranges were equal to 1.0– and 1.0– mmol m−3 d−1 in the western Mediterranean, while in the eastern Mediterranean, they decreased to 0.5– and 0.4– mmol m−3 d−1, respectively. The spatial variability in nutrient uptake was correlated with the phytoplankton primary production gradient simulated in the DCM layer. In fact, the simulated primary production maximum in the DCM layer was equal on average to 4 mg C m−3 d−1 in the western Mediterranean and to 2.5 mg C m−3 d−1 in the eastern Mediterranean (a detailed primary production scenario simulated by the same BFM model with a very similar set-up is presented in Fig. 2.2.2 of Schuckmann et al., 2020). The higher rate of biological activity produced a sharper transition from the surface depleted zone to the deeper nutrient-rich zone in the western Mediterranean. Additionally, the higher nitrate concentration in the mesopelagic layer contributed to increasing the nitracline steepness in the western sub-basins (Fig. 7) and hence the vertical supply of nutrients and higher nutrient consumption and production in the DBM layer.
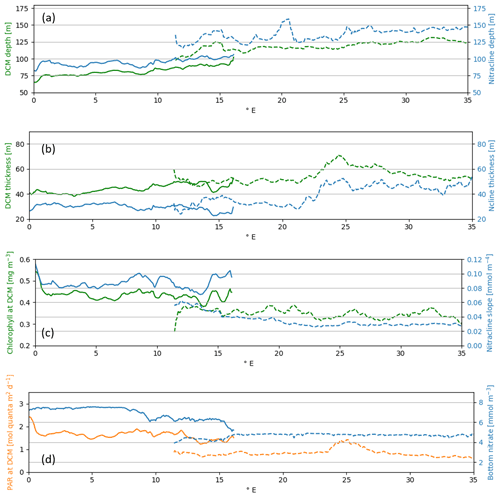
Figure 9Meridional averages of summer water column indexes (Table 5) along red lines in Fig. 8 for western (continuous line) and eastern (dashed line) sub-basins: DCM (green) and nitracline (blue) depth [m] (a); DCM (green) and nitracline (blue) thickness [m] (b); DCM intensity (green) as chlorophyll concentration [mg m−3] and nitracline slope (blue) [mmol m−4] (c); PAR-at-DCM depth (orange) [mol quanta m−2 d−1] and bottom nitrate concentration (blue) [mmol m−3] (d).
The results of the present work demonstrate the feasibility of assimilating multi-stream biogeochemical observations in real ecosystem simulations, showing the potential and the impact of emerging observation systems such as the BGC-Argo network. Moreover, using assimilation set-ups that differently combine satellite chlorophyll and BGC-Argo float chlorophyll and nitrate observations, the reciprocal effects of each observation source showed that the impact of two or more data streams resulted in an almost superimposition of the assimilation effects on the simulation results. Even if the significantly different observed densities of the two data streams considered here (i.e. much higher in the satellite data than in the float data) could lead to the suppression of the impact of the less populated data stream, it is also true that the satellite surface observations and float profiles were shown to act mostly in different seasons (winter versus summer, respectively) and at different pelagic layers, leading to an additive superimposition of single assimilation effects. These results were obtained by adopting a sequence of assimilation events for the different data streams and letting the model dynamically adjust the increments. In particular, the assimilation frequency was set to weekly and daily for satellites and floats, respectively, in accordance with previous studies on single-stream assimilation (Cossarini et al., 2019; Teruzzi et al., 2018, 2014). However, alternative strategies can mitigate the effects of higher observation densities, excessively reducing the impact of the other more sparse and more scarce observations. For instance, an error in the spatial covariance component can be included in the observation error covariance matrix (Moore et al., 2019) to take into consideration the spatial correlation of neighbouring high-density observations (e.g. satellite observations). Keeping the observation error covariance matrix diagonal, another option can be to increase satellite observation errors close to the locations of the assimilated float profiles. Either considering the off-diagonal element of the observation error matrix or dynamically adjusting its diagonal elements close to float positions requires considerable work to revise the formulation scheme and tune the errors.
On the other hand, as shown in a recent OSSE (Ford et al., 2020), the availability of a large and homogeneous float coverage can generate a full-domain impact of float assimilation. The foreseen increase in the BGC-Argo network (Bittig et al., 2019; Claustre et al., 2020) will move in that direction. However, the need to elongate life floats will probably result in a decrease in the sampling cycle frequency from 5 to 10 d (Roemmich et al., 2019). Moreover, the potential effectiveness of the present BGC-Argo missions in covering the Mediterranean bioregions has been demonstrated at least for chlorophyll (D'Ortenzio et al., 2020). Thus, while it is desirable to increase the number of nitrate sensors, it must be acknowledged that the density of BGC-Argo could not increase indefinitely in the future. In the Mediterranean Sea, a season-dependent sampling frequency that is higher during winter and spring surface blooms and lower during summer slow-dynamic conditions could compensate for the battery-saving needs and the maximization of float impact in joint float–satellite assimilation. In addition, to increase the spatial impact of data assimilation, future operational implementations can be based on pseudo profiles reconstructed by a neural network approach such as CANYON-MED that uses the larger coverage of the Argo network and oxygen sensors (Fourrier et al., 2020).
The present results of the joint assimilation of multi-stream chlorophyll data showed a mitigation of the increase in the RMSD of chlorophyll with respect to the non-assimilated chlorophyll dataset in the single-stream assimilation simulations (Figs. 2 and 5). The RMSD increase with respect to the non-assimilated chlorophyll data in the single-stream assimilation can be ascribed to discrepancies between the two datasets obtained by different measurement methods. Provided that florescence-derived methods and reflectance-based models have different sensitivity and calibration patterns whose investigation is out of the scope of the present work, it is relevant to highlight that discrepancies have a seasonal distribution: higher-than-float satellite values occur in summer, and the opposite occurs in winter (Fig. 10). As a consequence of these discrepancies and of the propagation of information through the prescribed DA vertical covariance, the change in chlorophyll profiles due to satellite assimilation either reduced or increased the distance between modelled chlorophyll and BGC-Argo chlorophyll profiles (Fig. 2). On the other hand, considering RMSDs with respect to satellite chlorophyll (Fig. 5), the effect of inconsistency between satellite and float chlorophyll was highlighted by the slight increase in RMSDs in simulations with float chlorophyll assimilation. In perspective, when the inconsistency between satellite and float will be solved, the multi-platform assimilation will provide improvements over large areas thanks to the relevant spatial coverage of satellite observations. In the meantime, the model, acting as a dynamical filter, integrates both sources of information together with the vertical covariance VV operator implemented in the assimilation scheme. Indeed, the vertical covariance is a key element that allows us to integrate surface with subsurface information. In our case the VV operator was derived at a monthly scale from the simulation results averaged over sub-basins (Teruzzi et al., 2018). However, by adopting a higher temporal and spatial resolution for the definition of VV, local information (single satellite observation and float profile) can be better integrated through the assimilation. Indeed, in physical oceanography, point-to-point varying VV or a combination of seasonal pre-calculated and flow-dependent parts have been successfully tested in assimilation applications (Dobricic et al., 2015; Storto et al., 2018; Storto and Oddo, 2019).
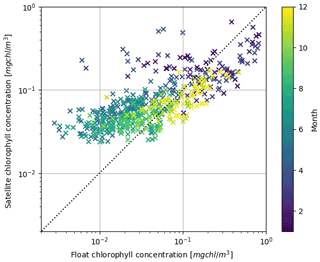
Figure 10Scatter plot on a logarithmic scale of float and satellite observations of chlorophyll concentration.
The different assimilation set-ups investigated in the present work showed the reciprocal effects of the assimilation of different data streams and how they combined in the multi-stream assimilation. Considering effects on nitrate, the assimilation of satellite chlorophyll reduced the nitrate RMSDs (computed on BGC-Argo data) with respect to the REF simulation in all the sub-basins with the exception of TYR (Fig. 3). The rather persistent and broad DA increments during late winter and early spring were acting to reduce the overestimation of the bloom maxima, resulting in nearly uniform reductions in the phytoplankton biomass in the euphotic layer. In turn, by reacting to the new phytoplankton concentration, less nitrogen was eventually re-mineralized to nitrate. Thus, the nitrate concentration was modified in the direction of reducing the REF nitrate overestimation in the upper layer. An analogous mechanism has been investigated in previous applications of satellite chlorophyll assimilation in the MedBFM system (Teruzzi et al., 2018, 2014). In the case of BGC-Argo chlorophyll assimilation, the changes in the phytoplankton profiles were less uniform (Cossarini et al., 2019), often alternating between positive and negative increments along the same profile. It follows that the effects on nitrate profiles (through new uptake or release after mortality and exudation) were non-linear and not uniform with relatively small impacts on the overall nitrate RMSDs. Concerning the assimilation effects on the non-assimilated oxygen variable, the non-degradation of the oxygen RMSDs was a positive aspect of the assimilated simulations, while the limited and non-univocal effects of the assimilation on oxygen RMSDs were related to the interaction of a number of trophic processes (e.g. phytoplankton production and respiration, zooplankton and bacteria respiration) after the assimilation increments on phytoplankton biomass. Effects on non-assimilated biogeochemical variables are discussed in a number of works (e.g. Ciavatta et al., 2014; Ford, 2020; Mattern et al., 2017; Santana-Falcón et al., 2020; Simon et al., 2015; Skákala et al., 2021; Teruzzi et al., 2018; Tsiaras et al., 2017; Yu et al., 2018) in which the non-degradation of non-assimilated variables is considered already a good result of the assimilation process. Moreover, the model-assimilation system acts as a filter so that, even if the performance of the multi-platform assimilation is lower than any one of the single assimilations, it produces a balanced solution with respect to all the available information.
The VB operator (biogeochemical covariance operator) plays a key role in the accuracy of results of the assimilated and non-assimilated variables. In this work, VB was based on the actual physiological status at each grid point for the phytoplankton components, while the nitrate–phosphate covariance was pre-calculated using a multi-annual simulation and varied monthly and at sub-basin scale. Our results suggest that the relevant variability in the DCM and nutricline conditions across the Mediterranean Sea would require a VB including finer temporal- and spatial-scale covariances between phytoplankton and nutrients. An available option to extend the number of variables considered in VB and to increase its capability to represent covariance at finer scales consists of Kalman-filter-based methods, among which recently emerging hybrid schemes (Carrassi et al., 2018) could be particularly relevant since they can combine pre-calculated covariance with the one estimated by an ensemble Kalman filter approach. In the present application the covariance operator VB has an impact on all the phytoplankton variables, as well as on two nutrients (phosphate and nitrate), which can act as limiting factors of phytoplankton growth in the Mediterranean Sea (Lazzari et al., 2016). In perspective VB could be developed to include other variables; however, considering silicate, it should be noted that in OGST-BFM applications in the Mediterranean Sea silicate limitation is less relevant compared to nitrate or phosphate.
Recent literature highlighted the relevant role of assimilating vertical observations from BGC-Argo to improve the simulation of key biogeochemical processes (Ford, 2021; Germineaud et al., 2019; Wang et al., 2020). Our analysis of the assimilation impact showed that the description of several biogeochemical features of the euphotic layer (such as the DCM depth and intensity and nutricline depth) benefited from BGC-Argo chlorophyll and nitrate data. Furthermore, the results of the simulation that integrated float and satellite observations provided a validated 3D description of Mediterranean Sea biogeochemistry. In particular, we investigated the DCM layer during summer-stratified conditions. The results were quantitatively consistent with previous estimations of DCM depth over the Mediterranean Sea (Lavigne et al., 2013; Lazzari et al., 2012; Mignot et al., 2014) and qualitatively with the results of studies that investigated the variability in the main DCM and nutricline features according to different nutrient and light availability regimes (e.g. Aksnes et al., 2007; Barbieux et al., 2019; Beckmann and Hense, 2007; Cossarini et al., 2019; Cullen, 2015; Gong et al., 2017; Terzić et al., 2019). In particular, we showed that the DCM is shallower, more intense, and less thick and occurs at higher light intensity with higher nutrient uptake by phytoplankton in the western Mediterranean than in the eastern Mediterranean. Correspondingly, the nitracline and the phosphocline (not shown) are shallower, steeper, and narrower. Moving eastward, the DCM and nutricline features change by as much as 50 % (Fig. 9), which indicates that the Mediterranean Sea has relatively variable conditions despite being a small semi-enclosed basin (Bethoux et al., 1999; Malanotte-Rizzoli et al., 2014; Schroeder et al., 2016). Several factors contribute to the east–west differences in the DCM and nutricline properties. For instance, a higher light extinction factor and higher nutrient concentrations in the bottom layer than in the other layers have been associated with shallower DCM (or DBM) and nutricline depths and a steeper nutricline (Aksnes et al., 2007; Beckmann and Hense, 2007; Gong et al., 2017; Mellard et al., 2011; Mignot et al., 2014). Moreover, winter deep mixing events can create conditions for a shallower DCM since a larger availability of nutrients in the subsurface layer is made available by winter mixing in the northwestern Mediterranean (Mignot et al., 2014). In our simulations, both the higher bottom-layer nutrients and typical strong mixing events in the western Mediterranean were consistently reproduced, and they sustained the east–west gradient of DCM depth. As in our simulation results, BGC-Argo observations in the Mediterranean Sea (Barbieux et al., 2019; Pasqueron de Fommervault et al., 2015) showed a shallower, more intense, and narrower DCM and a shallower and steeper nitracline in the western Mediterranean, but nitracline depths were closer to the DCM in the eastern Mediterranean, in contrast to the almost constant difference between the DCM and nitracline depths observed in our simulation. However, this discrepancy can be ascribed to the different methods used to define a nitracline. According to Salon et al. (2019), we defined nitracline as the layer where the slope was larger, corresponding to the interface between high and depleted nutrient concentrations. In fact, results more similar to those of Barbieux et al. (2019) have been obtained by applying the same definition of nitracline depth to our simulation results (not shown). In a previous study based on BGC-Argo observations, the relation between the DCM and DBM in the Mediterranean Sea was also investigated (Barbieux et al., 2019; Mignot et al., 2014). As an emergent property of the BFM formulation and simulation set-ups, our results show that the subsurface depths of chlorophyll and biomass maxima coincided (similar to the findings of Mignot et al., 2014). On the other hand, the results of Barbieux et al. (2019) highlight that the two depths can be non-coincident under oligotrophic conditions typical of the eastern Mediterranean basins.
In addition to confirming previous findings on the spatial variability in the DCM layer characteristics in the Mediterranean Sea, the assimilated simulation presented in this work provides a full 3D and time-varying description of a number of biogeochemical variables, allowing us to further investigate potential relations between the vertical distributions of phytoplankton, nutrients, light availability, and other physical forcings. The use of the results of assimilated simulations that include along-depth observations can integrate investigations on the DCM and its dynamics that to date have been based on observations, which can be sparse and not evenly distributed in time (Navarro and Ruiz, 2013; Ricour et al., 2021).
In this work, we presented the results of a set of simulations of Mediterranean Sea biogeochemistry that integrated BGC-Argo chlorophyll and nitrate observations and satellite chlorophyll observations using different assimilation set-ups. The results show that the assimilation of all the data streams outperformed the single-source assimilation when validated with respect to available observations, indicating that the assimilation of BGC-Argo observations has relevant (even if local) impacts on the vertical structure of nutrients and phytoplankton. The impacts of multi-variate profile assimilation are directly linked to the sampling frequency and dimension of the BGC-Argo network, which should increase to match the consolidated importance and relevance of satellite observation assimilation. Thus, in a perspective view, the multi-platform assimilation can improve model representation of both large-scale (hundreds to thousands of kilometres) to mesoscale features and be beneficial for robust reconstruction in global and regional reanalysis. The results of the simulation with multi-platform assimilation provided a 3D description of the basin-wide gradient of DCM and nitracline dynamics through specifically developed metrics (e.g. DCM depth and intensity, as well as nitracline depth and steepness) and highlighted the role played by light and nutrient availability in the western and eastern Mediterranean Sea. Even if the Mediterranean Sea is a small marginal sea, it exhibits a wide range of summer vertical conditions of the DCM and nutricline with thinner and shallower but intense DCM associated with a steeper and narrower nutricline in the western Mediterranean Sea, while the opposite occurs in the eastern Mediterranean Sea.
The BFM biogeochemical model and its documentation can be downloaded at the following address: http://bfm-community.eu/ (Vichi et al., 2015). The variational assimilation code has been published in Teruzzi et al. (2019), and the version used in the present work is available at https://doi.org/10.5281/zenodo.5723738 (Di Cerbo et al., 2021). The quality-controlled databases used in the present paper are publicly available from the SEANOE (SEA scieNtific Open data Edition) publisher at https://doi.org/10.17882/42182 (Argo, 2021).
AT and GC conceived the study. GB and AT developed the model code and performed the simulations. LF and GB prepared the observation datasets. AT conducted the analysis of the simulation results and wrote the first draft. GC and AT discussed and reviewed the manuscript. LF and GC participated in the final manuscript editing.
The contact author has declared that neither they nor their co-authors have any competing interests.
Publisher’s note: Copernicus Publications remains neutral with regard to jurisdictional claims in published maps and institutional affiliations.
This article is part of the special issue “Biogeochemistry in the BGC-Argo era: from process studies to ecosystem forecasts (BG/OS inter-journal SI)”. It is not associated with a conference.
This study has been conducted using EU Copernicus Marine Service Information. We thank Sarah Schlunegger and the other anonymous reviewer for their constructive comments.
This research has been supported by CMEMS, which is implemented by Mercator Ocean International within the framework of a delegation agreement with the European Union (grant no. 74-CMEMS-MFC-MED).
This paper was edited by Marilaure Grégoire and reviewed by Sarah Schlunegger and one anonymous referee.
Aksnes, D. L., Ohman, M. D., and Rivière, P.: Optical effect on the nitracline in a coastal upwelling area, Limnol. Oceanogr., 52, 1179–1187, https://doi.org/10.4319/lo.2007.52.3.1179, 2007.
Argo: Argo float data and metadata from Global Data Assembly Centre (Argo GDAC), SEANOE [data set], https://doi.org/10.17882/42182, 2021.
Barbieux, M., Uitz, J., Gentili, B., Pasqueron de Fommervault, O., Mignot, A., Poteau, A., Schmechtig, C., Taillandier, V., Leymarie, E., Penkerc'h, C., D'Ortenzio, F., Claustre, H., and Bricaud, A.: Bio-optical characterization of subsurface chlorophyll maxima in the Mediterranean Sea from a Biogeochemical-Argo float database, Biogeosciences, 16, 1321–1342, https://doi.org/10.5194/bg-16-1321-2019, 2019.
Beckmann, A. and Hense, I.: Beneath the surface: Characteristics of oceanic ecosystems under weak mixing conditions – A theoretical investigation, Prog. Oceanogr., 75, 771–796, https://doi.org/10.1016/j.pocean.2007.09.002, 2007.
Bethoux, J. P., Gentili, B., Morin, P., Nicolas, E., Pierre, C., and Ruiz-Pino, D.: The Mediterranean Sea: a miniature ocean for climatic and environmental studies and a key for the climatic functioning of the North Atlantic, Prog. Oceanogr., 44, 131–146, https://doi.org/10.1016/S0079-6611(99)00023-3, 1999.
Bittig, H. C., Maurer, T. L., Plant, J. N., Schmechtig, C., Wong, A. P. S., Claustre, H., Trull, T. W., Udaya Bhaskar, T. V. S., Boss, E., Dall'Olmo, G., Organelli, E., Poteau, A., Johnson, K. S., Hanstein, C., Leymarie, E., Le Reste, S., Riser, S. C., Rupan, A. R., Taillandier, V., Thierry, V., and Xing, X.: A BGC-Argo Guide: Planning, Deployment, Data Handling and Usage, Front. Mar. Sci., 6, 502, https://doi.org/10.3389/fmars.2019.00502, 2019.
Buga, L., Sarbu, G., Fryberg, L., Magnus, W., Wesslander, K., Gatti, J., Leroy, D., Iona, S., Larsen, M., Koefoed Rømer, J., Østrem, A. K., Lipizer, M., and Giorgietti, A.: EMODnet Chemistry Eutrophication and Acidity aggregated datasets v2018, EMODnet Thematic Lot n° 4/SI2.749773, https://doi.org/10.6092/EC8207EF-ED81-4EE5-BF48-E26FF16BF02E, 2018.
Carrassi, A., Bocquet, M., Bertino, L., and Evensen, G.: Data assimilation in the geosciences: An overview of methods, issues, and perspectives, WIREs Clim. Change, 9, e535, https://doi.org/10.1002/wcc.535, 2018.
Chai, F., Johnson, K. S., Claustre, H., Xing, X., Wang, Y., Boss, E., Riser, S., Fennel, K., Schofield, O., and Sutton, A.: Monitoring ocean biogeochemistry with autonomous platforms, Nat. Rev. Earth Environ., 1, 315–326, https://doi.org/10.1038/s43017-020-0053-y, 2020.
Ciavatta, S., Torres, R., Martinez-Vicente, V., Smyth, T., Dall'Olmo, G., Polimene, L., and Allen, J. I.: Assimilation of remotely-sensed optical properties to improve marine biogeochemistry modelling, Prog. Oceanogr., 127, 74–95, https://doi.org/10.1016/j.pocean.2014.06.002, 2014.
Ciavatta, S., Kay, S., Saux-Picart, S., Butenschoen, M., and Allen, J. I.: Decadal reanalysis of biogeochemical indicators and fluxes in the North West European shelf-sea ecosystem, J. Geophys. Res.-Oceans, 121, 1824–1845, https://doi.org/10.1002/2015JC011496, 2016.
Ciavatta, S., Brewin, R. J. W., Skákala, J., Polimene, L., Mora, L., de Artioli, Y., and Allen, J. I.: Assimilation of Ocean-Color Plankton Functional Types to Improve Marine Ecosystem Simulations, J. Geophys. Res.-Oceans, 123, 834–854, https://doi.org/10.1002/2017JC013490, 2018.
Ciavatta, S., Kay, S., Brewin, R. J. W., Cox, R., Cicco, A. D., Nencioli, F., Polimene, L., Sammartino, M., Santoleri, R., Skákala, J., and Tsapakis, M.: Ecoregions in the Mediterranean Sea Through the Reanalysis of Phytoplankton Functional Types and Carbon Fluxes, J. Geophys. Res.-Oceans, 124, 6737–6759, https://doi.org/10.1029/2019JC015128, 2019.
Claustre, H., Johnson, K. S., and Takeshita, Y.: Observing the Global Ocean with Biogeochemical-Argo, Annu. Rev. Mar. Sci., 12, 23–48, https://doi.org/10.1146/annurev-marine-010419-010956, 2020.
Cossarini, G., Lazzari, P., and Solidoro, C.: Spatiotemporal variability of alkalinity in the Mediterranean Sea, Biogeosciences, 12, 1647–1658, https://doi.org/10.5194/bg-12-1647-2015, 2015.
Cossarini, G., Querin, S., Solidoro, C., Sannino, G., Lazzari, P., Di Biagio, V., and Bolzon, G.: Development of BFMCOUPLER (v1.0), the coupling scheme that links the MITgcm and BFM models for ocean biogeochemistry simulations, Geosci. Model Dev., 10, 1423–1445, https://doi.org/10.5194/gmd-10-1423-2017, 2017.
Cossarini, G., Mariotti, L., Feudale, L., Mignot, A., Salon, S., Taillandier, V., Teruzzi, A., and D'Ortenzio, F.: Towards operational 3D-Var assimilation of chlorophyll Biogeochemical-Argo float data into a biogeochemical model of the Mediterranean Sea, Ocean Model., 133, 112–128, https://doi.org/10.1016/j.ocemod.2018.11.005, 2019.
Cullen, J. J.: Subsurface Chlorophyll Maximum Layers: Enduring Enigma or Mystery Solved?, Annu. Rev. Mar. Sci., 7, 207–239, https://doi.org/10.1146/annurev-marine-010213-135111, 2015.
Di Biagio, V., Cossarini, G., Salon, S., Lazzari, P., Querin, S., Sannino, G., and Solidoro, C.: Temporal scales of variability in the Mediterranean Sea ecosystem: Insight from a coupled model, J. Mar. Syst., 197, 103176, https://doi.org/10.1016/j.jmarsys.2019.05.002, 2019.
Di Cerbo, P., Pascolo, E., and Bolzon, G.: inogs/3dVarBio: Multivariate version (release-4.0), Zenodo [code], https://doi.org/10.5281/zenodo.5723738, 2021.
Dobricic, S., Wikle, C. K., Milliff, R. F., Pinardi, N., and Berliner, L. M.: Assimilation of oceanographic observations with estimates of vertical background-error covariances by a Bayesian hierarchical model, Q. J. Roy. Meteor. Soc., 141, 182–194, https://doi.org/10.1002/qj.2348, 2015.
Dorofeyev, V. and Sukhikh, L.: A model for monitoring the evolution of the Black Sea ecosystem on the basis of remote sensing data assimilation, Int. J. Remote Sens., 39, 9339–9355, https://doi.org/10.1080/01431161.2018.1523589, 2018.
D'Ortenzio, F., Lavigne, H., Besson, F., Claustre, H., Coppola, L., Garcia, N., Laës-Huon, A., Reste, S. L., Malardé, D., Migon, C., Morin, P., Mortier, L., Poteau, A., Prieur, L., Raimbault, P., and Testor, P.: Observing mixed layer depth, nitrate and chlorophyll concentrations in the northwestern Mediterranean: A combined satellite and NO3 profiling floats experiment, Geophys. Res. Lett., 41, 6443–6451, https://doi.org/10.1002/2014GL061020, 2014.
D'Ortenzio, F., Taillandier, V., Claustre, H., Prieur, L. M., Leymarie, E., Mignot, A., Poteau, A., Penkerc'h, C., and Schmechtig, C. M.: Biogeochemical Argo: The Test Case of the NAOS Mediterranean Array, Front. Mar. Sci., 7, 120, https://doi.org/10.3389/fmars.2020.00120, 2020.
Dowd, M., Jones, E., and Parslow, J.: A statistical overview and perspectives on data assimilation for marine biogeochemical models, Environmetrics, 25, 203–213, https://doi.org/10.1002/env.2264, 2014.
Dutkiewicz, S., Hickman, A. E., Jahn, O., Henson, S., Beaulieu, C., and Monier, E.: Ocean colour signature of climate change, Nat. Commun., 10, 578, https://doi.org/10.1038/s41467-019-08457-x, 2019.
Fennel, K., Gehlen, M., Brasseur, P., Brown, C. W., Ciavatta, S., Cossarini, G., Crise, A., Edwards, C. A., Ford, D., Friedrichs, M. A. M., Gregoire, M., Jones, E., Kim, H.-C., Lamouroux, J., Murtugudde, R., Perruche, C., and the GODAE OceanView Marine Ecosystem Analysis and Prediction Task Team: Advancing Marine Biogeochemical and Ecosystem Reanalyses and Forecasts as Tools for Monitoring and Managing Ecosystem Health, Front. Mar. Sci., 6, 89, https://doi.org/10.3389/fmars.2019.00089, 2019.
Fontana, C., Brasseur, P., and Brankart, J.-M.: Toward a multivariate reanalysis of the North Atlantic Ocean biogeochemistry during 1998–2006 based on the assimilation of SeaWiFS chlorophyll data, Ocean Sci., 9, 37–56, https://doi.org/10.5194/os-9-37-2013, 2013.
Ford, D.: Assimilating synthetic Biogeochemical-Argo and ocean colour observations into a global ocean model to inform observing system design, Biogeosciences, 18, 509–534, https://doi.org/10.5194/bg-18-509-2021, 2021.
Ford, D. A.: Assessing the role and consistency of satellite observation products in global physical–biogeochemical ocean reanalysis, Ocean Sci., 16, 875–893, https://doi.org/10.5194/os-16-875-2020, 2020.
Ford, D. and Barciela, R.: Global marine biogeochemical reanalyses assimilating two different sets of merged ocean colour products, Remote Sens. Environ., 203, 40–54, https://doi.org/10.1016/j.rse.2017.03.040, 2017.
Fourrier, M., Coppola, L., Claustre, H., D'Ortenzio, F., Sauzède, R., and Gattuso, J.-P.: A Regional Neural Network Approach to Estimate Water-Column Nutrient Concentrations and Carbonate System Variables in the Mediterranean Sea: CANYON-MED, Front. Mar. Sci., 7, 620, https://doi.org/10.3389/fmars.2020.00620, 2020.
Gehlen, M., Barciela, R., Bertino, L., Brasseur, P., Butenschön, M., Chai, F., Crise, A., Drillet, Y., Ford, D., Lavoie, D., Lehodey, P., Perruche, C., Samuelsen, A., and Simon, E.: Building the capacity for forecasting marine biogeochemistry and ecosystems: recent advances and future developments, J. Oper. Oceanogr., 8, s168–s187, https://doi.org/10.1080/1755876X.2015.1022350, 2015.
Germineaud, C., Brankart, J.-M., and Brasseur, P.: An Ensemble-Based Probabilistic Score Approach to Compare Observation Scenarios: An Application to Biogeochemical-Argo Deployments, J. Atmos. Ocean. Tech., 36, 2307–2326, https://doi.org/10.1175/JTECH-D-19-0002.1, 2019.
Gong, X., Jiang, W., Wang, L., Gao, H., Boss, E., Yao, X., Kao, S.-J., and Shi, J.: Analytical solution of the nitracline with the evolution of subsurface chlorophyll maximum in stratified water columns, Biogeosciences, 14, 2371–2386, https://doi.org/10.5194/bg-14-2371-2017, 2017.
Goodliff, M., Bruening, T., Schwichtenberg, F., Li, X., Lindenthal, A., Lorkowski, I., and Nerger, L.: Temperature assimilation into a coastal ocean-biogeochemical model: assessment of weakly and strongly coupled data assimilation, Ocean Dyn., 69, 1217–1237, https://doi.org/10.1007/s10236-019-01299-7, 2019.
Groom, S., Sathyendranath, S., Ban, Y., Bernard, S., Brewin, R., Brotas, V., Brockmann, C., Chauhan, P., Choi, J., Chuprin, A., Ciavatta, S., Cipollini, P., Donlon, C., Franz, B., He, X., Hirata, T., Jackson, T., Kampel, M., Krasemann, H., Lavender, S., Pardo-Martinez, S., Mélin, F., Platt, T., Santoleri, R., Skakala, J., Schaeffer, B., Smith, M., Steinmetz, F., Valente, A., and Wang, M.: Satellite Ocean Colour: Current Status and Future Perspective, Front. Mar. Sci., 6, 485, https://doi.org/10.3389/fmars.2019.00485, 2019.
Hipsey, M. R., Gal, G., Arhonditsis, G. B., Carey, C. C., Elliott, J. A., Frassl, M. A., Janse, J. H., de Mora, L., and Robson, B. J.: A system of metrics for the assessment and improvement of aquatic ecosystem models, Environ. Modell. Softw., 128, 104697, https://doi.org/10.1016/j.envsoft.2020.104697, 2020.
Hu, J., Fennel, K., Mattern, J. P., and Wilkin, J.: Data assimilation with a local Ensemble Kalman Filter applied to a three-dimensional biological model of the Middle Atlantic Bight, J. Mar. Syst., 94, 145–156, https://doi.org/10.1016/j.jmarsys.2011.11.016, 2012.
Johnson, K., Pasqueron De Fommervault, O., Serra, R., D'Ortenzio, F., Schmechtig, C., Claustre, H., and Poteau, A.: Processing Bio-Argo nitrate concentration at the DAC Level, IFREMER for Argo Data Management, https://doi.org/10.13155/46121, 2018.
Jones, E. M., Baird, M. E., Mongin, M., Parslow, J., Skerratt, J., Lovell, J., Margvelashvili, N., Matear, R. J., Wild-Allen, K., Robson, B., Rizwi, F., Oke, P., King, E., Schroeder, T., Steven, A., and Taylor, J.: Use of remote-sensing reflectance to constrain a data assimilating marine biogeochemical model of the Great Barrier Reef, Biogeosciences, 13, 6441–6469, https://doi.org/10.5194/bg-13-6441-2016, 2016.
Lavigne, H., D'Ortenzio, F., Migon, C., Claustre, H., Testor, P., d'Alcalà, M. R., Lavezza, R., Houpert, L., and Prieur, L.: Enhancing the comprehension of mixed layer depth control on the Mediterranean phytoplankton phenology, J. Geophys. Res.-Oceans, 118, 3416–3430, https://doi.org/10.1002/jgrc.20251, 2013.
Lazzari, P., Teruzzi, A., Salon, S., Campagna, S., Calonaci, C., Colella, S., Tonani, M., and Crise, A.: Pre-operational short-term forecasts for Mediterranean Sea biogeochemistry, Ocean Sci., 6, 25–39, https://doi.org/10.5194/os-6-25-2010, 2010.
Lazzari, P., Solidoro, C., Ibello, V., Salon, S., Teruzzi, A., Béranger, K., Colella, S., and Crise, A.: Seasonal and inter-annual variability of plankton chlorophyll and primary production in the Mediterranean Sea: a modelling approach, Biogeosciences, 9, 217–233, https://doi.org/10.5194/bg-9-217-2012, 2012.
Lazzari, P., Mattia, G., Solidoro, C., Salon, S., Crise, A., Zavatarelli, M., Oddo, P., and Vichi, M.: The impacts of climate change and environmental management policies on the trophic regimes in the Mediterranean Sea: Scenario analyses, J. Mar. Syst., 135, 137–149, https://doi.org/10.1016/j.jmarsys.2013.06.005, 2014.
Lazzari, P., Solidoro, C., Salon, S., and Bolzon, G.: Spatial variability of phosphate and nitrate in the Mediterranean Sea: A modeling approach, Deep Sea Res. Part I Oceanogr. Res. Pap., 108, 39–52, https://doi.org/10.1016/j.dsr.2015.12.006, 2016.
Lazzari, P., Álvarez, E., Terzić, E., Cossarini, G., Chernov, I., D'Ortenzio, F., and Organelli, E.: CDOM Spatiotemporal Variability in the Mediterranean Sea: A Modelling Study, J. Mar. Sci.-Eng., 9, 176, https://doi.org/10.3390/jmse9020176, 2021.
Lorenc, A. C.: Analysis methods for numerical weather prediction, Q. J. Roy. Meteor. Soc., 112, 1177–1194, https://doi.org/10.1002/qj.49711247414, 1986.
Malanotte-Rizzoli, P., Artale, V., Borzelli-Eusebi, G. L., Brenner, S., Crise, A., Gacic, M., Kress, N., Marullo, S., Ribera d'Alcalà, M., Sofianos, S., Tanhua, T., Theocharis, A., Alvarez, M., Ashkenazy, Y., Bergamasco, A., Cardin, V., Carniel, S., Civitarese, G., D'Ortenzio, F., Font, J., Garcia-Ladona, E., Garcia-Lafuente, J. M., Gogou, A., Gregoire, M., Hainbucher, D., Kontoyannis, H., Kovacevic, V., Kraskapoulou, E., Kroskos, G., Incarbona, A., Mazzocchi, M. G., Orlic, M., Ozsoy, E., Pascual, A., Poulain, P.-M., Roether, W., Rubino, A., Schroeder, K., Siokou-Frangou, J., Souvermezoglou, E., Sprovieri, M., Tintoré, J., and Triantafyllou, G.: Physical forcing and physical/biochemical variability of the Mediterranean Sea: a review of unresolved issues and directions for future research, Ocean Sci., 10, 281–322, https://doi.org/10.5194/os-10-281-2014, 2014.
Mattern, J. P., Song, H., Edwards, C. A., Moore, A. M., and Fiechter, J.: Data assimilation of physical and chlorophyll a observations in the California Current System using two biogeochemical models, Ocean Model., 109, 55–71, https://doi.org/10.1016/j.ocemod.2016.12.002, 2017.
Mayot, N., D'Ortenzio, F., Taillandier, V., Prieur, L., de Fommervault, O. P., Claustre, H., Bosse, A., Testor, P., and Conan, P.: Physical and Biogeochemical Controls of the Phytoplankton Blooms in North Western Mediterranean Sea: A Multiplatform Approach Over a Complete Annual Cycle (2012–2013 DEWEX Experiment), J. Geophys. Res.-Oceans, 122, 9999–10019, https://doi.org/10.1002/2016JC012052, 2017.
Melaku Canu, D., Ghermandi, A., Nunes, P. A. L. D., Lazzari, P., Cossarini, G., and Solidoro, C.: Estimating the value of carbon sequestration ecosystem services in the Mediterranean Sea: An ecological economics approach, Global Environ. Chang., 32, 87–95, https://doi.org/10.1016/j.gloenvcha.2015.02.008, 2015.
Mellard, J. P., Yoshiyama, K., Litchman, E., and Klausmeier, C. A.: The vertical distribution of phytoplankton in stratified water columns, J. Theor. Biol., 269, 16–30, https://doi.org/10.1016/j.jtbi.2010.09.041, 2011.
Mignot, A., Claustre, H., Uitz, J., Poteau, A., D'Ortenzio, F., and Xing, X.: Understanding the seasonal dynamics of phytoplankton biomass and the deep chlorophyll maximum in oligotrophic environments: A Bio-Argo float investigation, Global Biogeochem. Cy., 28, 856–876, https://doi.org/10.1002/2013GB004781, 2014.
Mignot, A., D'Ortenzio, F., Taillandier, V., Cossarini, G., and Salon, S.: Quantifying Observational Errors in Biogeochemical-Argo Oxygen, Nitrate, and Chlorophyll a Concentrations, Geophys. Res. Lett., 46, 4330–4337, https://doi.org/10.1029/2018GL080541, 2019.
Moore, A. M., Martin, M. J., Akella, S., Arango, H. G., Balmaseda, M., Bertino, L., Ciavatta, S., Cornuelle, B., Cummings, J., Frolov, S., Lermusiaux, P., Oddo, P., Oke, P. R., Storto, A., Teruzzi, A., Vidard, A., and Weaver, A. T.: Synthesis of Ocean Observations Using Data Assimilation for Operational, Real-Time and Reanalysis Systems: A More Complete Picture of the State of the Ocean, Front. Mar. Sci., 6, 90, https://doi.org/10.3389/fmars.2019.00090, 2019.
Muller-Karger, F. E., Miloslavich, P., Bax, N. J., Simmons, S., Costello, M. J., Sousa Pinto, I., Canonico, G., Turner, W., Gill, M., Montes, E., Best, B. D., Pearlman, J., Halpin, P., Dunn, D., Benson, A., Martin, C. S., Weatherdon, L. V., Appeltans, W., Provoost, P., Klein, E., Kelble, C. R., Miller, R. J., Chavez, F. P., Iken, K., Chiba, S., Obura, D., Navarro, L. M., Pereira, H. M., Allain, V., Batten, S., Benedetti-Checchi, L., Duffy, J. E., Kudela, R. M., Rebelo, L.-M., Shin, Y., and Geller, G.: Advancing Marine Biological Observations and Data Requirements of the Complementary Essential Ocean Variables (EOVs) and Essential Biodiversity Variables (EBVs) Frameworks, Front. Mar. Sci., 5, 211, https://doi.org/10.3389/fmars.2018.00211, 2018.
Navarro, G. and Ruiz, J.: Hysteresis conditions the vertical position of deep chlorophyll maximum in the temperate ocean, Global Biogeochem. Cy., 27, 1013–1022, https://doi.org/10.1002/gbc.20093, 2013.
Oddo, P., Adani, M., Pinardi, N., Fratianni, C., Tonani, M., and Pettenuzzo, D.: A nested Atlantic-Mediterranean Sea general circulation model for operational forecasting, Ocean Sci., 5, 461–473, https://doi.org/10.5194/os-5-461-2009, 2009.
Oddo, P., Bonaduce, A., Pinardi, N., and Guarnieri, A.: Sensitivity of the Mediterranean sea level to atmospheric pressure and free surface elevation numerical formulation in NEMO, Geosci. Model Dev., 7, 3001–3015, https://doi.org/10.5194/gmd-7-3001-2014, 2014.
Organelli, E., Barbieux, M., Claustre, H., Schmechtig, C., Poteau, A., Bricaud, A., Boss, E., Briggs, N., Dall'Olmo, G., D'Ortenzio, F., Leymarie, E., Mangin, A., Obolensky, G., Penkerc'h, C., Prieur, L., Roesler, C., Serra, R., Uitz, J., and Xing, X.: Two databases derived from BGC-Argo float measurements for marine biogeochemical and bio-optical applications, Earth Syst. Sci. Data, 9, 861–880, https://doi.org/10.5194/essd-9-861-2017, 2017.
Pasqueron de Fommervault, O. P., D'Ortenzio, F., Mangin, A., Serra, R., Migon, C., Claustre, H., Lavigne, H., d'Alcalà, M. R., Prieur, L., Taillandier, V., Schmechtig, C., Poteau, A., Leymarie, E., Dufour, A., Besson, F., and Obolensky, G.: Seasonal variability of nutrient concentrations in the Mediterranean Sea: Contribution of Bio-Argo floats, J. Geophys. Res.-Oceans, 120, 8528–8550, https://doi.org/10.1002/2015JC011103, 2015.
Pradhan, H. K., Völker, C., Losa, S. N., Bracher, A., and Nerger, L.: Assimilation of Global Total Chlorophyll OC-CCI Data and Its Impact on Individual Phytoplankton Fields, J. Geophys. Res.-Oceans, 124, 470–490, https://doi.org/10.1029/2018JC014329, 2019.
Pradhan, H. K., Völker, C., Losa, S. N., Bracher, A., and Nerger, L.: Global Assimilation of Ocean-Color Data of Phytoplankton Functional Types: Impact of Different Data Sets, J. Geophys. Res.-Oceans, 125, e2019JC015586, https://doi.org/10.1029/2019JC015586, 2020.
Raicich, F. and Rampazzo, A.: Observing System Simulation Experiments for the assessment of temperature sampling strategies in the Mediterranean Sea, Ann. Geophys., 21, 151–165, https://doi.org/10.5194/angeo-21-151-2003, 2003.
Ratheesh, S., Chakraborty, A., Sharma, R., and Basu, S.: Assimilation of satellite chlorophyll measurements into a coupled biophysical model of the Indian Ocean with a guided particle filter, Remote Sens. Lett., 7, 446–455, https://doi.org/10.1080/2150704X.2016.1143985, 2016.
Ribera d'Alcalà, M., Civitarese, G., Conversano, F., and Lavezza, R.: Nutrient ratios and fluxes hint at overlooked processes in the Mediterranean Sea, J. Geophys. Res.-Oceans, 108, 8106, https://doi.org/10.1029/2002JC001650, 2003.
Ricour, F., Capet, A., D'Ortenzio, F., Delille, B., and Grégoire, M.: Dynamics of the deep chlorophyll maximum in the Black Sea as depicted by BGC-Argo floats, Biogeosciences, 18, 755–774, https://doi.org/10.5194/bg-18-755-2021, 2021.
Roemmich, D., Alford, M. H., Claustre, H., Johnson, K., King, B., Moum, J., Oke, P., Owens, W. B., Pouliquen, S., Purkey, S., Scanderbeg, M., Suga, T., Wijffels, S., Zilberman, N., Bakker, D., Baringer, M., Belbeoch, M., Bittig, H. C., Boss, E., Calil, P., Carse, F., Carval, T., Chai, F., Conchubhair, D. Ó., d'Ortenzio, F., Dall'Olmo, G., Desbruyeres, D., Fennel, K., Fer, I., Ferrari, R., Forget, G., Freeland, H., Fujiki, T., Gehlen, M., Greenan, B., Hallberg, R., Hibiya, T., Hosoda, S., Jayne, S., Jochum, M., Johnson, G. C., Kang, K., Kolodziejczyk, N., Körtzinger, A., Traon, P.-Y. L., Lenn, Y.-D., Maze, G., Mork, K. A., Morris, T., Nagai, T., Nash, J., Garabato, A. N., Olsen, A., Pattabhi, R. R., Prakash, S., Riser, S., Schmechtig, C., Schmid, C., Shroyer, E., Sterl, A., Sutton, P., Talley, L., Tanhua, T., Thierry, V., Thomalla, S., Toole, J., Troisi, A., Trull, T. W., Turton, J., Velez-Belchi, P. J., Walczowski, W., Wang, H., Wanninkhof, R., Waterhouse, A. F., Waterman, S., Watson, A., Wilson, C., Wong, A. P. S., Xu, J., and Yasuda, I.: On the Future of Argo: A Global, Full-Depth, Multi-Disciplinary Array, Front. Mar. Sci., 6, 439, https://doi.org/10.3389/fmars.2019.00439, 2019.
Salon, S., Cossarini, G., Bolzon, G., Feudale, L., Lazzari, P., Teruzzi, A., Solidoro, C., and Crise, A.: Novel metrics based on Biogeochemical Argo data to improve the model uncertainty evaluation of the CMEMS Mediterranean marine ecosystem forecasts, Ocean Sci., 15, 997–1022, https://doi.org/10.5194/os-15-997-2019, 2019.
Santana-Falcón, Y., Brasseur, P., Brankart, J. M., and Garnier, F.: Assimilation of chlorophyll data into a stochastic ensemble simulation for the North Atlantic Ocean, Ocean Sci., 16, 1297–1315, https://doi.org/10.5194/os-16-1297-2020, 2020.
Schmechtig, C., Poteau, A., Claustre, H., D'Ortenzio, F., and Boss, E.: Processing bio-Argo chlorophyll-A concentration at the DAC level, IFREMER for Argo Data Management, https://doi.org/10.13155/39468, 2015.
Schmechtig, C., Poteau, A., Claustre, H., D'Ortenzio, F., Dall'Olmo, G., and Boss, E.: Processing Bio-Argo particle backscattering at the DAC level, IFREMER for Argo Data Management, https://doi.org/10.13155/39459, 2018.
Schroeder, K., Chiggiato, J., Bryden, H. L., Borghini, M., and Ben Ismail, S.: Abrupt climate shift in the Western Mediterranean Sea, Sci. Rep., 6, 23009, https://doi.org/10.1038/srep23009, 2016.
Shulman, I., Frolov, S., Anderson, S., Penta, B., Gould, R., Sakalaukus, P., and Ladner, S.: Impact of bio-optical data assimilation on short-term coupled physical, bio-optical model predictions, J. Geophys. Res.-Oceans, 118, 2215–2230, https://doi.org/10.1002/jgrc.20177, 2013.
Simon, E., Samuelsen, A., Bertino, L., and Mouysset, S.: Experiences in multiyear combined state–parameter estimation with an ecosystem model of the North Atlantic and Arctic Oceans using the Ensemble Kalman Filter, J. Mar. Syst., 152, 1–17, https://doi.org/10.1016/j.jmarsys.2015.07.004, 2015.
Skákala, J., Ford, D., Brewin, R. J. W., McEwan, R., Kay, S., Taylor, B., de Mora, L., and Ciavatta, S.: The Assimilation of Phytoplankton Functional Types for Operational Forecasting in the Northwest European Shelf, J. Geophys. Res.-Oceans, 123, 5230–5247, https://doi.org/10.1029/2018JC014153, 2018.
Skákala, J., Ford, D., Bruggeman, J., Hull, T., Kaiser, J., King, R. R., Loveday, B., Palmer, M. R., Smyth, T., Williams, C. A. J., and Ciavatta, S.: Towards a Multi-Platform Assimilative System for North Sea Biogeochemistry, J. Geophys. Res.-Oceans, 126, e2020JC016649, https://doi.org/10.1029/2020JC016649, 2021.
Song, H., Edwards, C. A., Moore, A. M., and Fiechter, J.: Data assimilation in a coupled physical-biogeochemical model of the California current system using an incremental lognormal 4-dimensional variational approach: Part 3–Assimilation in a realistic context using satellite and in situ observations, Ocean Model., 106, 159–172, https://doi.org/10.1016/j.ocemod.2016.06.005, 2016.
Storto, A. and Oddo, P.: Optimal Assimilation of Daytime SST Retrievals from SEVIRI in a Regional Ocean Prediction System, Remote Sens., 11, 2776, https://doi.org/10.3390/rs11232776, 2019.
Storto, A., Oddo, P., Cipollone, A., Mirouze, I., and Lemieux-Dudon, B.: Extending an oceanographic variational scheme to allow for affordable hybrid and four-dimensional data assimilation, Ocean Model., 128, 67–86, https://doi.org/10.1016/j.ocemod.2018.06.005, 2018.
Teruzzi, A., Dobricic, S., Solidoro, C., and Cossarini, G.: A 3-D variational assimilation scheme in coupled transport-biogeochemical models: Forecast of Mediterranean biogeochemical properties, J. Geophys. Res.-Oceans, 119, 200–217, https://doi.org/10.1002/2013JC009277, 2014.
Teruzzi, A., Bolzon, G., Salon, S., Lazzari, P., Solidoro, C., and Cossarini, G.: Assimilation of coastal and open sea biogeochemical data to improve phytoplankton simulation in the Mediterranean Sea, Ocean Model., 132, 46–60, https://doi.org/10.1016/j.ocemod.2018.09.007, 2018.
Teruzzi, A., Di Cerbo, P., Cossarini, G., Pascolo, E., and Salon, S.: Parallel implementation of a data assimilation scheme for operational oceanography: The case of the MedBFM model system, Comput. Geosci., 124, 103–114, https://doi.org/10.1016/j.cageo.2019.01.003, 2019.
Terzić, E., Lazzari, P., Organelli, E., Solidoro, C., Salon, S., D'Ortenzio, F., and Conan, P.: Merging bio-optical data from Biogeochemical-Argo floats and models in marine biogeochemistry, Biogeosciences, 16, 2527–2542, https://doi.org/10.5194/bg-16-2527-2019, 2019.
Terzić, E., Salon, S., Cossarini, G., Solidoro, C., Teruzzi, A., Miró, A., and Lazzari, P.: Impact of interannually variable diffuse attenuation coefficients for downwelling irradiance on biogeochemical modelling, Ocean Model., 161, 101793, https://doi.org/10.1016/j.ocemod.2021.101793, 2021.
Thierry, V., Bittig, H., Gilbert, D., Kobayashi, T., Kanako, S., and Schmid, C.: Processing Argo oxygen data at the DAC level,IFREMER for Argo Data Management, https://doi.org/10.13155/39795, 2018.
Thingstad, T. F. and Rassoulzadegan, F.: Nutrient limitations, microbial food webs and “biological C-pumps”: suggested interactions in a P-limited Mediterranean, Mar. Ecol. Prog. Ser., 117, 299–306, 1995.
Tsiaras, K. P., Hoteit, I., Kalaroni, S., Petihakis, G., and Triantafyllou, G.: A hybrid ensemble-OI Kalman filter for efficient data assimilation into a 3-D biogeochemical model of the Mediterranean, Ocean Dyn., 67, 673–690, https://doi.org/10.1007/s10236-017-1050-7, 2017.
Verdy, A. and Mazloff, M. R.: A data assimilating model for estimating Southern Ocean biogeochemistry, J. Geophys. Res.-Oceans, 122, 6968–6988, https://doi.org/10.1002/2016JC012650, 2017.
Vichi, M., Lovato, T., Lazzari, P., Cossarini, G., Gutierrez Mlot, E., Mattia, G., Masina, S., McKiver, W. J., Pinardi, N., Solidoro, C., Tedesco, L., and Zavatarelli, M.: The BiogeochemicalFlux Model (BFM): Equation Description and User Manual. BFMversion 5.1, BFM Reportseries N. 1, Release 1.1 [code], Bologna, Italy, 104 pp., available at: http://bfm-community.eu (last access: 17 November 2021), 2015.
von Schuckmann, K., Traon, P.-Y. L., Smith, N., Pascual, A., Djavidnia, S., Gattuso, J.-P., Grégoire, M., Nolan, G., Aaboe, S., Fanjul, E. Á., Aouf, L., Aznar, R., Badewien, T. H., Behrens, A., Berta, M., Bertino, L., Blackford, J., Bolzon, G., Borile, F., Bretagnon, M., Brewin, R. J. W., Canu, D., Cessi, P., Ciavatta, S., Chapron, B., Chau, T. T. T., Chevallier, F., Chtirkova, B., Ciliberti, S., Clark, J. R., Clementi, E., Combot, C., Comerma, E., Conchon, A., Coppini, G., Corgnati, L., Cossarini, G., Cravatte, S., de Alfonso, M., de Montégut, C. B., Fernández, C. D. L., de los Santos, F. J., Denvil-Sommer, A., de Collar, Á. P., Nunes, P. A. L. D., Biagio, V. D., Drudi, M., Embury, O., Falco, P., d'Andon, O. F., Ferrer, L., Ford, D., Freund, H., León, M. G., Sotillo, M. G., García-Valdecasas, J. M., Garnesson, P., Garric, G., Gasparin, F., Gehlen, M., Genua-Olmedo, A., Geyer, G., Ghermandi, A., Good, S. A., Gourrion, J., Greiner, E., Griffa, A., González, M., Hernández-Carrasco, I., Isoard, S., Kennedy, J. J., Kay, S., Korosov, A., Laanemäe, K., Land, P. E., Lavergne, T., Lazzari, P., Legeais, J.-F., Lemieux, B., Levier, B., Llovel, W., Lyubartsev, V., Traon, P.-Y. L., Lien, V. S., Lima, L., Lorente, P., Mader, J., Magaldi, M. G., Maljutenko, I., Mangin, A., Mantovani, C., Marinova, V., Masina, S., Mauri, E., Meyerjürgens, J., Mignot, A., McEwan, R., Mejia, C., Melet, A., Menna, M., Meyssignac, B., Mouche, A., Mourre, B., Müller, M., Notarstefano, G., Orfila, A., Pardo, S., Peneva, E., Pérez-Gómez, B., Perruche, C., Peterlin, M., Poulain, P.-M., Pinardi, N., Quilfen, Y., Raudsepp, U., Renshaw, R., Révelard, A., Reyes-Reyes, E., Ricker, M., Rodríguez-Rubio, P., Rotllán, P., Royo Gelabert, E., Rubio, A., Ruiz-Parrado, I., Sathyendranath, S., She, J., Solidoro, C., Stanev, E. V., Staneva, J., Storto, A., Su, J., Tajalli Bakhsh, T., Tilstone, G.H., Tintoré, J., Toledano, C., Tournadre, J., Tranchant, B., Uiboupin, R., Valcarcel, A., Valcheva, N., Verbrugge, N., Vrac, M., Wolff, J.-O., Zambianchi, E., Zielinski, O., Zinck, A.-S., and Zunino, S.: Copernicus Marine Service Ocean State Report, Issue 4, J. Oper. Oceanogr., 13, S1–S172, https://doi.org/10.1080/1755876X.2020.1785097, 2020.
Wang, B., Fennel, K., Yu, L., and Gordon, C.: Assessing the value of biogeochemical Argo profiles versus ocean color observations for biogeochemical model optimization in the Gulf of Mexico, Biogeosciences, 17, 4059–4074, https://doi.org/10.5194/bg-17-4059-2020, 2020.
Xiao, Y. and Friedrichs, M. A. M.: The assimilation of satellite-derived data into a one-dimensional lower trophic level marine ecosystem model, J. Geophys. Res.-Oceans, 119, 2691–2712, https://doi.org/10.1002/2013JC009433, 2014.
Xing, X., Boss, E., Zhang, J., and Chai, F.: Evaluation of Ocean Color Remote Sensing Algorithms for Diffuse Attenuation Coefficients and Optical Depths with Data Collected on BGC-Argo Floats, Remote Sens., 12, 2367, https://doi.org/10.3390/rs12152367, 2020.
Yu, L., Fennel, K., Bertino, L., Gharamti, M. E., and Thompson, K. R.: Insights on multivariate updates of physical and biogeochemical ocean variables using an Ensemble Kalman Filter and an idealized model of upwelling, Ocean Model., 126, 13–28, https://doi.org/10.1016/j.ocemod.2018.04.005, 2018.