the Creative Commons Attribution 4.0 License.
the Creative Commons Attribution 4.0 License.
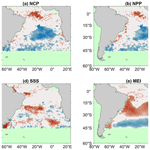
Identifying the biological control of the annual and multi-year variations in South Atlantic air–sea CO2 flux
Daniel J. Ford
Gavin H. Tilstone
Jamie D. Shutler
Vassilis Kitidis
The accumulation of anthropogenic CO2 emissions in the atmosphere has been buffered by the absorption of CO2 by the global ocean, which acts as a net CO2 sink. The CO2 flux between the atmosphere and the ocean, which collectively results in the oceanic carbon sink, is spatially and temporally variable, and fully understanding the driving mechanisms behind this flux is key to assessing how the sink may change in the future. In this study a time series decomposition analysis was applied to satellite observations to determine the drivers that control the sea–air difference of CO2 partial pressure (ΔpCO2) and the CO2 flux on seasonal and inter-annual timescales in the South Atlantic Ocean. Linear trends in ΔpCO2 and the CO2 flux were calculated to identify key areas of change.
Seasonally, changes in both the ΔpCO2 and CO2 flux were dominated by sea surface temperature (SST) in the subtropics (north of 40∘ S) and were correlated with biological processes in the subpolar regions (south of 40∘ S). In the equatorial Atlantic, analysis of the data indicated that biological processes are likely a key driver as a response to upwelling and riverine inputs. These results highlighted that seasonally ΔpCO2 can act as an indicator to identify drivers of the CO2 flux. Inter-annually, the SST and biological contributions to the CO2 flux in the subtropics were correlated with the multivariate El Niño–Southern Oscillation (ENSO) index (MEI), which leads to a weaker (stronger) CO2 sink in El Niño (La Niña) years.
The 16-year time series identified significant trends in ΔpCO2 and CO2 flux; however, these trends were not always consistent in spatial extent. Therefore, predicting the oceanic response to climate change requires the examination of CO2 flux rather than ΔpCO2. Positive CO2 flux trends (weakening sink for atmospheric CO2) were identified within the Benguela upwelling system, consistent with increased upwelling and wind speeds. Negative trends in the CO2 flux (intensifying sink for atmospheric CO2) offshore into the South Atlantic gyre were consistent with an increase in the export of nutrients from mesoscale features, which drives the biological drawdown of CO2. These multi-year trends in the CO2 flux indicate that the biological contribution to changes in the air–sea CO2 flux cannot be overlooked when scaling up to estimates of the global ocean carbon sink.
- Article
(6994 KB) - Full-text XML
- BibTeX
- EndNote
Since the industrial revolution, anthropogenic CO2 emissions have increased unabated and continue to raise atmospheric CO2 concentrations (IPCC, 2021). The global oceans have buffered the rise by acting as a sink for atmospheric CO2 at a rate of between 1 and 3.5 Pg C yr−1 (e.g. Friedlingstein et al., 2020; Landschützer et al., 2014; Watson et al., 2020). The strength of the ocean as a sink for CO2 appears to be increasing with time (Friedlingstein et al., 2020; Watson et al., 2020). Regionally this can vary hugely, however, and the ocean can oscillate between a source and sink of atmospheric CO2. The difference in the partial pressure of CO2 (pCO2) between the seawater and atmosphere (ΔpCO2) is used as an indicator or proxy for the net direction of air–sea CO2 flux during gas exchange.
In the open ocean, changes in physical and biogeochemical processes that control seawater pCO2 (pCO2 (sw)) also modify ΔpCO2 as the atmospheric pCO2 (pCO2 (atm)) is less variable (e.g. Henson et al., 2018; Landschützer et al., 2016). ΔpCO2 can therefore be controlled by changes in sea surface temperature (SST) because the pCO2 is proportional to the temperature. In addition, plankton net community production (NCP) modifies the concentration of CO2 in the seawater depending on the balance between net primary production (NPP; uptake of CO2 via photosynthesis) and respiration (release of CO2 into the water). The NCP describes the overall metabolic balance of the plankton community, where positive (negative) NCP indicates a drawdown (or release) of CO2 from (or into) the water contributing to a decrease (increase) in ΔpCO2. Physical processes, including riverine input (e.g. Ibánhez et al., 2016; Lefèvre et al., 2020; Valerio et al., 2021) and upwelling (e.g. González-Dávila et al., 2009; Lefèvre et al., 2008; Santana-Casiano et al., 2009), can alter pCO2 (sw) and ΔpCO2 directly through the entrainment of high-CO2 water or indirectly by modifying NCP through nutrient supply (enhancing photosynthesis) and/or organic material supply (enhancing respiration).
The air–sea CO2 flux is more precisely a function of the difference in CO2 concentrations across the mass boundary layer at the ocean's surface, with any turbulent exchange characterized by the gas transfer velocity. The CO2 concentration difference is determined by the pCO2 at the base (pCO2 (sw)) and top (pCO2 (atm)) of the mass boundary layer and the respective solubilities (Weiss, 1974), and it must be carefully calculated due to vertical thermo-haline gradients existing across the mass boundary layer (Woolf et al., 2016). The gas transfer velocity is usually parameterized as a function of wind speed (e.g. Ho et al., 2006; Nightingale et al., 2000; Wanninkhof, 2014) which accounts for ∼ 75 % of the variance in surface turbulent exchange (e.g. Dong et al., 2021; Ho et al., 2006). Therefore, both oceanographic and meteorological conditions are able to modify and control the seasonality, inter-annual variability, and multi-year trends of this flux.
Seasonal drivers of ΔpCO2 have been explored globally (Takahashi et al., 2002) and regionally in the Atlantic Ocean (Landschützer et al., 2013; Henson et al., 2018). Takahashi et al. (2002) used binned in situ pCO2 (sw) observations to a 4∘ by 5∘ global grid and found that SST drives ΔpCO2 in the subtropics and that non-SST processes (i.e. biological activity and ocean circulation) dominate in subpolar and equatorial regions. Landschützer et al. (2013) used a self-organizing map feed forward neural network (SOM-FNN) technique to extrapolate the in situ pCO2 (sw) observations and reported similar seasonal drivers in the Atlantic Ocean with one exception, which is that SST and non-SST processes compensated each other in the equatorial Atlantic. Henson et al. (2018) using binned in situ observations for the North Atlantic Ocean also indicated that the subtropics are driven by SST and that subpolar regions are correlated with biological activity.
The inter-annual drivers of ΔpCO2 are different compared to the seasonal drivers in the North Atlantic (Henson et al., 2018), which could be true of the South Atlantic Ocean, though this needs to be further investigated. Landschützer et al. (2016, 2014) postulated that the El Niño cycle may influence ΔpCO2 in the subtropical South Atlantic but did not explore the underlying processes. South of 35∘ S, Landschützer et al. (2015) indicated that atmospheric forcing could control the inter-annual variability in ΔpCO2 through changes in Ekman transport and upwelling. These inter-annual drivers of ΔpCO2 and the CO2 flux in the South Atlantic Ocean are poorly understood but have key implications for determining how the oceanic CO2 sink could be impacted by climate change and its evolution over inter-annual and decadal timescales.
In this study, we investigate the drivers of ΔpCO2 and the CO2 flux in the South Atlantic Ocean over both seasonal and inter-annual timescales using a time series decomposition approach. Trends in ΔpCO2 and the CO2 flux were calculated from 2002 to 2018, and regions in the South Atlantic Ocean showing the greatest change in the CO2 flux are investigated.
2.1 pCO2 data
Satellite estimates of pCO2 (sw) were retrieved from the South Atlantic Feed Forward Neural Network (SA-FNN) dataset (Ford et al., 2022, 2021a). Ford et al. (2022) showed that the SA-FNN improved on estimating the seasonal pCO2 (sw) variability in the South Atlantic Ocean compared to the current “state of the art” methodology (the SOM-FNN). The SA-FNN estimates pCO2 (sw) by clustering in situ monthly 1∘ gridded Surface Ocean CO2 Atlas (SOCAT) v2020 pCO2 (sw) observations (Bakker et al., 2016; Sabine et al., 2013), which have been reanalysed into a dataset configured using consistent depth and temperature fields (Goddijn-Murphy et al., 2015; Woolf et al., 2016; Reynolds et al., 2002), into eight static provinces in the South Atlantic Ocean (Fig. B1a). The use of eight static provinces allows the SA-FNN to more accurately reproduce the pCO2 (sw) variability. The nonlinear relationships between pCO2 (sw) and three environmental drivers (SST, NCP, and pCO2 (atm)) were constructed for each province with a feed forward neural network (FNN). The FNNs for each province were applied to produce spatially and temporally complete pCO2 (sw) fields on monthly 1∘ grids between July 2002 and December 2018, with uncertainties generated on a per pixel basis as described in Ford et al. (2022). These per pixel uncertainties are shown in Appendix B (Fig. B1).
Monthly 1∘ grids of pCO2 (atm) were extracted from v5.5 of the global estimates of the pCO2 (sw) dataset (Landschützer et al., 2017, 2016) which was calculated using the dry mixing ratio of CO2 from the NOAA Earth System Research Laboratories (ESRL) marine boundary layer reference (https://www.esrl.noaa.gov/gmd/ccgg/mbl/, last access: 25 September 2020), optimum interpolated SST (Reynolds et al., 2002), and sea level pressure following Dickson et al. (2007). ΔpCO2 was calculated from pCO2 (sw) and pCO2 (atm) as
2.2 Air–sea CO2 flux data
The air–sea CO2 flux (F) can be estimated using a bulk parameterization as
where k is the gas transfer velocity which was estimated from ERA5 monthly reanalysis wind speed (Hersbach et al., 2019) following the parameterization of Nightingale et al. (2000). αw and αs are the solubility of CO2 at the base and top of the mass boundary layer at the sea surface (Woolf et al., 2016). αw was calculated as a function of SST and sea surface salinity (SSS) (Weiss, 1974) using the monthly optimum interpolated SST (Reynolds et al., 2002) and SSS from the Copernicus Marine Environment Modelling Service global ocean physics reanalysis product (GLORYS12V1; CMEMS, 2021). αs was calculated using the same temperature and salinity datasets but included a gradient from the base to the top of the mass boundary layer of −0.17 K (Donlon et al., 1999) and +0.1 salinity units (Woolf et al., 2016). pCO2 (atm) was calculated using the dry mixing ratio of CO2 from the NOAA ESRL marine boundary layer reference, optimum interpolated SST (Reynolds et al., 2002) applying a cool skin bias (0.17 K; Donlon et al., 1999), and sea level pressure following Dickson et al. (2007).
All of these calculations along with the resulting monthly CO2 flux were carried out using the open-source FluxEngine toolbox (Holding et al., 2019; Shutler et al., 2016) for the period between July 2002 and December 2018, assuming “rapid” transfer (as described in Woolf et al., 2016).
2.3 Biological data
The 4 km resolution mean monthly chlorophyll a (Chl a) was calculated from Moderate Resolution Imaging Spectroradiometer on Aqua (MODIS-A) Level 1 granules, retrieved from the National Aeronautics Space Administration (NASA) Ocean Colour website (https://oceancolor.gsfc.nasa.gov/, last access: 10 December 2020), using SeaDAS v7.5 and applying the standard OC3-CI algorithm for Chl a (https://oceancolor.gsfc.nasa.gov/atbd/chlor_a/, last access: 15 December 2020). Monthly composites of MODIS-A SST (NASA OBPG, 2015) and photosynthetically active radiation (PAR; NASA OBPG, 2017b) were also downloaded from the NASA Ocean Colour website. Monthly NPP composites were generated from MODIS-A Chl a, SST, and PAR composites using the wavelength resolving model (Morel, 1991) with the look-up table described in Smyth et al. (2005). Coincident monthly composites of NCP using the algorithm NCP-D described in Tilstone et al. (2015) were generated using the NPP and SST data. Further details of the satellite algorithms are given in O'Reilly et al. (1998), O'Reilly and Werdell (2019), and Hu et al. (2012) for Chl a, Smyth et al. (2005) and Tilstone et al. (2005, 2009) for NPP, and Tilstone et al. (2015) for NCP. Monthly composites were generated between July 2002 and December 2018 and were re-gridded onto the same 1∘ grid as the pCO2 (sw) and flux data. Ford et al. (2021b) showed that these satellite algorithms for Chl a, NPP, NCP, and SST are accurate compared to in situ observations in the South Atlantic Ocean following an algorithm intercomparison which accounted for model, in situ, and input parameter uncertainties.
2.4 Seasonal and inter-annual driver analysis
The X-11 analytical econometric tool (Shiskin et al., 1967) was used to decompose the time series into seasonal, inter-annual, and residual components following the methodology of Pezzulli et al. (2005). In brief, the X-11 method comprises a three-step filtering algorithm. (1) The inter-annual component (Tt) is initially estimated using an annual centred running mean, which is subtracted from the initial time series (Xt), and a seasonal running mean applied to estimate the seasonal component (St). (2) Tt is revised by applying an annual centred running mean to the Xt minus St. The revised Tt is removed from Xt and the final St calculated with a seasonal running mean. (3) The final Tt is calculated by applying an annual centred running mean to Xt minus the revised St. The analysis has been shown to be effective in the decomposition of environmental time series (Pezzulli et al., 2005; Vantrepotte and Mélin, 2011; Henson et al., 2018), which allows the seasonal cycle to vary on a yearly basis, and it produces an inter-annual component that results in a robust representation of the longer-term changes in the time series.
The approach was applied to monthly 1∘ fields of ΔpCO2 that were estimated from pCO2 (atm) and SA-FNN pCO2 (sw) on a per pixel basis. The pCO2 (atm) and spatially and temporally varying pCO2 (sw) uncertainties (Table 1; Fig. B1) were propagated through the X-11 analysis, using a Monte Carlo uncertainty propagation approach. The input time series were randomly perturbed 1000 times within the uncertainties of each parameter, and Spearman correlations were calculated for each perturbation. The 95 % confidence interval was extracted from the resulting distribution of correlation coefficients, and results were deemed significant (α<0.05) where the confidence interval remained significant. Spatial autocorrelation was tested using the method of field significance (Wilks, 2006). The analysis was then conducted on the CO2 fluxes on a per pixel basis. The pCO2 (sw), pCO2 (atm), gas transfer velocity, SST, and SSS uncertainties (Table 1) were propagated through the flux calculations using the same Monte Carlo uncertainty propagation approach used for ΔpCO2.
The potential drivers tested were MODIS-A skin SST, NCP, and NPP alongside SSS from the CMEMS global reanalysis product (GLORYSV12; CMEMS, 2021) and two climate indices: multivariate El Niño–Southern Oscillation (ENSO) index (MEI) as an indicator of ENSO phases from https://www.esrl.noaa.gov/psd/enso/mei (last access: 19 December 2019) and Southern Annular Mode (SAM) data, which indicate the displacement of the westerly winds in the Southern Ocean and were downloaded from http://www.nerc-bas.ac.uk/icd/gjma/sam.html (last access: 19 December 2019).
2.5 Trend analysis
The linear trends in the inter-annual components of ΔpCO2 and the CO2 flux were calculated on a per pixel basis using the non-parametric Mann–Kendall test (Kendall, 1975; Mann, 1945) and Sen's slope estimates (Sen, 1968), which are less sensitive to outliers in the time series. The input parameter uncertainties (Table 1) were propagated within this trend analysis using a Monte Carlo uncertainty propagation (n=1000) to extract the 95 % confidence interval on the trends. The overall trend was deemed significant if 95 % of the trends were significant (α=0.05), and the uncertainties in these trends are displayed in Appendix B (Fig. B2).
2.6 Limitations
It should be noted that correlations between the ΔpCO2 and SST and NCP are expected since the SA-FNN estimates pCO2 (sw) (the major determinant of ΔpCO2 variability) using SST and NCP as input parameters which are subsequently interpreted as drivers here. By extension, but to a lesser extent, this also applies to correlations between CO2 flux and SST and NCP since pCO2 (sw) is included in the flux calculations. Different lines of evidence suggest that this is not a major limitation of our study. Firstly, any correlation between Δ flux and SST and NCP is not determined a priori but is an emerging property of the SA-FNN. Therefore, the driver analysis undertaken here represents an indirect decomposition of the SA-FNN drivers rather than a strict correlation analysis between independent variables. The accurate representation of seasonal pCO2 (sw) cycles across the South Atlantic Ocean (Ford et al., 2022) provides confidence in the SA-FNN. Secondly, conducting the analysis described by Henson et al. (2018) using in situ pCO2 (sw) to estimate ΔpCO2 on a per province basis (Longhurst, 1998) for the South Atlantic Ocean yielded similar seasonal drivers to the SA-FNN (Appendix A). The inter-annual drivers displayed some differences, however, which may be due to the spatial and temporal averaging that is required to construct the in situ time series.
3.1 Seasonal drivers of ΔpCO2 and CO2 flux
The X-11 analysis conducted on ΔpCO2 indicated significant seasonal correlations (Fig. 1) when the uncertainties are accounted for. The subtropics (10 to 40∘ S) showed positive correlations between ΔpCO2, SST, and SSS (Fig. 1c, d), as well as negative correlations between ΔpCO2, NCP, and NPP (Fig. 1a, b). In contrast the subpolar (south of 40∘ S) and equatorial regions (10∘ N to 10∘ S) displayed negative correlations between ΔpCO2 and SST (Fig. 1c). Correlations between ΔpCO2 and NCP were negative in the subpolar regions and were positive in the equatorial regions (Fig. 1a). There were no significant correlations observed between ΔpCO2 and MEI or SAM in any of the regions.
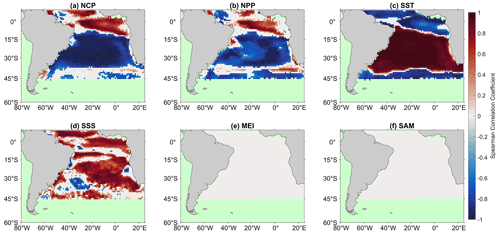
Figure 1Significant Spearman correlations between the ΔpCO2 seasonal component of the X-11 analysis and (a) net community production (NCP), (b) net primary production (NPP), (c) sea surface temperature (SST), (d) sea surface salinity (SSS), (e) multivariate ENSO index (MEI), and (f) Southern Annular Mode (SAM) seasonal components. White regions indicate no significant correlations, and green regions indicate no analysis was performed due to missing satellite data.
Regional deviations were observed in the Amazon plume, Benguela upwelling, the South American coast, and a band across 40∘ S. The region under the influence of the Amazon plume indicated negative correlations between ΔpCO2 and NCP in contrast to the surrounding waters which had positive correlations (Fig. 1a). The Benguela upwelling displayed positive correlations between ΔpCO2 and NCP (Fig. 1a), no significant correlations between ΔpCO2 and SST (Fig. 1c), and negative correlations between ΔpCO2 and SSS (Fig. 1e). The South American coast between 12 and 17∘ S displayed positive correlations between ΔpCO2 and NPP (Fig. 1b), along with negative correlations between ΔpCO2 and SSS (Fig. 1e). Negative correlation between ΔpCO2 and SSS and positive correlations between NCP, NPP, and ΔpCO2 were also observed in the southwestern Atlantic (Fig. 1e). Positive correlations between NCP, NPP, and ΔpCO2 were identified in a band across 40∘ S (Fig. 1a, b). Performing the X-11 analysis on the CO2 flux revealed similar and comparable correlations to ΔpCO2 (Fig. 2). Significant driver–flux correlations were observed over a larger area compared to ΔpCO2, however.
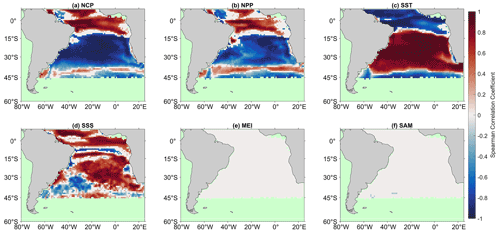
Figure 2Significant Spearman correlations between the air–sea CO2 flux seasonal component of the X-11 analysis and (a) net community production (NCP), (b) net primary production (NPP), (c) sea surface temperature (SST), (d) sea surface salinity (SSS), (e) multivariate ENSO index (MEI), and (f) Southern Annular Mode (SAM) seasonal components. White regions indicate no significant correlations, and green regions indicate no analysis was performed due to missing satellite data.
3.2 Inter-annual drivers of ΔpCO2 and CO2 flux
The X-11 analysis identified regionally significant inter-annual correlations between ΔpCO2 and SST, MEI, and to a lesser extent NCP and SSS (Fig. 3). The subtropics displayed positive correlations between SST and ΔpCO2, which extended across the basin from the South American coast (Fig. 3c). Positive correlations were also observed between the MEI and ΔpCO2 (Fig. 3e), with a similar geographic extent to the correlations with SST. In the central South Atlantic gyre spatially variable negative correlations between NCP and ΔpCO2 and positive correlations between SSS and ΔpCO2 were observed (Fig. 3a, d). The central equatorial Atlantic displayed spatially variable positive correlations between NCP and ΔpCO2, which extended southeast towards the African coast (Fig. 3a).
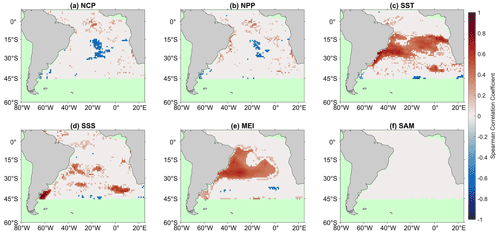
Figure 3Significant Spearman correlations between the ΔpCO2 inter-annual component of the X-11 analysis and (a) net community production (NCP), (b) net primary production (NPP), (c) sea surface temperature (SST), (d) sea surface salinity (SSS), (e) multivariate ENSO index (MEI), and (f) Southern Annular Mode (SAM) inter-annual components. White regions indicate no significant correlations, and green regions indicate no analysis was performed due to missing satellite data.
Significant inter-annual correlations for the CO2 flux were also identified by the X-11 analysis (Fig. 4), which generally covered a larger spatial area to the corresponding ΔpCO2 correlations (Fig. 3). Positive correlations between the CO2 flux and SST were observed in the subtropics (Fig. 4c), consistent with the correlations with ΔpCO2 (i.e. by comparing Figs. 4c and 3c). Nevertheless, negative correlations between the CO2 flux and SST were observed at the border between the equatorial region and subtropics, which was not identified in the ΔpCO2 correlations. Negative correlations between NCP and the CO2 flux were also identified over a spatially larger area (Figs. 4a, 3a). Correlations between the MEI and CO2 flux were positive in the subtropics (Fig. 4e) and included a band of negative correlations to the south between 35 and 45∘ S (Fig. 4e).
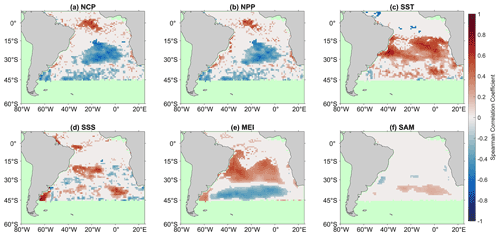
Figure 4Significant Spearman correlations between the air–sea CO2 flux inter-annual component of the X-11 analysis and (a) net community production (NCP), (b) net primary production (NPP), (c) sea surface temperature (SST), (d) sea surface salinity (SSS), (e) multivariate ENSO index (MEI), and (f) Southern Annular Mode (SAM) inter-annual components. White regions indicate no significant correlations, and green regions indicate no analysis was performed due to missing satellite data.
Positive correlations between NCP and CO2 flux were observed in the western equatorial Atlantic, alongside spatially variable negative correlations to SST (Fig. 4a, c). Positive correlations between SSS and CO2 flux were identified in the region of the Amazon plume (Fig. 4d). Weak positive correlations between the SAM and CO2 flux were identified between 30 and 45∘ S (Fig. 4f).
3.3 Trends in inter-annual ΔpCO2 and CO2 flux
Regions of significant trends in the inter-annual component of ΔpCO2 were observed (Fig. 5a). Negative trends occurred in the South Atlantic gyre. Positive trends in ΔpCO2 were identified along the South African coast, which switched to strong negative trends moving offshore into the central South Atlantic gyre. Positive trends were also observed in the equatorial Atlantic consistent with the positions of the Amazon plume and equatorial upwelling.
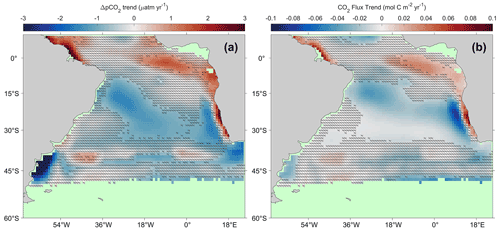
Figure 5Linear trends in (a) ΔpCO2 and (b) the air–sea CO2 flux between 2002 and 2018. Hashed areas indicate non-significant trends when accounting for the uncertainties. Green regions indicate insufficient data to calculate trends.
Regions of significant trends in the CO2 flux were identified (Fig. 5b) but over much larger spatial areas than evident in the ΔpCO2 results (i.e. comparing Fig. 5a with 5b). The trends in CO2 flux are generally in the same direction as trends in ΔpCO2. Strong positive trends in the CO2 flux occurred in the Benguela upwelling region and switched to a negative trend offshore of similar magnitude but occupied a larger spatial area.
4.1 Seasonal drivers of ΔpCO2 and CO2 flux
Previous studies have explored the seasonal drivers of ΔpCO2 and to a lesser extent the air–sea CO2 flux. In this study, we investigated the drivers of ΔpCO2 and CO2 flux at both seasonal and inter-annual timescales in the South Atlantic Ocean. In the North Atlantic, Henson et al. (2018) indicated that the seasonal variability in subtropical ΔpCO2 variability is driven by SST, whereas the variability in ΔpCO2 in subpolar regions is biologically driven, similar to previous studies (Takahashi et al., 2002; Landschützer et al., 2013). The X-11 analysis conducted on spatially complete ΔpCO2 and CO2 flux displayed consistent seasonal results (Figs. 1, 2), though for the CO2 flux significant correlations occupied a larger area. These both indicated a similar pattern in seasonal drivers for the South Atlantic Ocean, with subtropical ΔpCO2 and CO2 flux driven by SST and subpolar correlated with biological controls, although the equatorial region exhibited more complex patterns (Fig. 1).
In the equatorial Atlantic, the correlations between ΔpCO2, SST, and biological production were spatially variable (Fig. 1). Landschützer et al. (2013) suggested that the temperature and non-temperature (i.e. biological and circulation) drivers generally compensated each other. We found positive correlations between the NCP, ΔpCO2, and CO2 flux seasonal components, indicating that biological activity is likely a key driver of seasonal variability in response to the equatorial upwelling. Ford et al. (2022) showed that the SA-FNN improved the seasonal pCO2 (sw) variability in the equatorial Atlantic compared to the current “state of the art” SOM-FNN methodology (Watson et al., 2020). Elevated ΔpCO2 associated with elevated NCP in the eastern equatorial Atlantic was consistent with the seasonal equatorial upwelling (Radenac et al., 2020). Parard et al. (2010) indicated strong negative correlations between SST and ΔpCO2 during the upwelling season ( for June to September), which is also consistent with our results. By contrast, Lefèvre et al. (2016) showed that correlations between pCO2 (sw) and SST were weak across the whole year (), and SSS (R=0.93) was the primary driver at the same station.
In the western equatorial Atlantic, negative correlations between NCP and ΔpCO2 and positive correlations between the SSS and ΔpCO2 seasonal component occurred in the vicinity of the Amazon River mouth. The mixing of the Amazon River and oceanic water decreases SSS (Ibánhez et al., 2016; Lefèvre et al., 2020; Bonou et al., 2016; Lefévre et al., 2010) and increases the nutrient supply to the ocean which can in turn enhance NPP and NCP, leading to a decrease in ΔpCO2 within the Amazon plume (Körtzinger, 2003; Cooley et al., 2007). This coupling produces an extensive area of depressed ΔpCO2 which is a CO2 sink (Ibánhez et al., 2016). Lefèvre et al. (2010) indicated that rainfall from the intertropical convergence zone could reduce SSS, with an associated decrease in ΔpCO2. The eastern Tropical Atlantic is also subject to large river input, especially from the Congo (Hopkins et al., 2013) and Niger rivers, which could produce nutrient-rich plumes that fuel NCP and decrease ΔpCO2 (Lefèvre et al., 2016, 2021).
Between 30 and 45∘ S, dissolved inorganic carbon and SST exert a similar influence on pCO2 (sw), indicating that seasonal changes in dissolved inorganic carbon driven by biological uptake in the summer and upwelling in winter are approximately balanced by seasonal changes in SST and their control on the solubility pump (Henley et al., 2020). This most likely explains the band of positive correlations between NCP, NPP, and ΔpCO2 and sharp transitions in correlations between SST and ΔpCO2 across ∼ 40∘ S.
Deviations from the expected drivers in the subtropics, occurred within the Benguela upwelling system between 20 and 35∘ S. Positive correlations between NCP and the CO2 flux (Fig. 2a) alongside negative correlations between SST, SSS, and the CO2 flux (Fig. 2c, d) are indicative of upwelled waters that have both elevated pCO2 (sw) and nutrients, which cause an increase in NPP (Lamont et al., 2014). These upwelled waters move offshore in filaments (Rubio et al., 2009) where NPP decreases and SST becomes the dominant driver, which is confirmed by the positive correlations between SST and the CO2 flux further offshore. Ford et al. (2021b) indicated a switch in NCP drivers in the Benguela upwelling from wind-driven upwelling on the shelf to filaments that propagate offshore from the upwelling front, which is consistent with the switch in the drivers observed for the CO2 flux as these filaments move offshore.
At between 12 and 17∘ S along the South American coast, there were also deviations from the expected drivers as there were positive correlations between NPP and ΔpCO2 (Fig. 1b) and negative correlations between SSS and ΔpCO2 (Fig. 1d), which are consistent with an upwelling signature that occurs along the coast. Aguiar et al. (2018) also showed intense seasonal upwelling events in this region that are driven by wind and currents. The southern coast of South America is strongly influenced by riverine water input that reduces the total alkalinity and therefore causes an increase in pCO2 (sw) (Liutti et al., 2021). This is associated with an increased supply of nutrients which in turn enhances NPP, though the main drivers of pCO2 (sw) in this region are still total alkalinity and SST (Liutti et al., 2021). This potentially explains the positive correlation between ΔpCO2 and both NCP and NPP (Fig. 1a, b), as well as the negative correlations between ΔpCO2 and SSS. The extension offshore of this negative correlation between SSS and ΔpCO2 (Fig. 1d) could be caused by the advection of water masses due to intense mesoscale eddy activity arising from the Brazil–Malvinas confluence (Mason et al., 2017).
The seasonal correlations between the CO2 flux and the drivers were similar to ΔpCO2, but for CO2 flux these occurred over a larger spatial area. The South Atlantic subtropical anticyclone which controls wind speeds across the region (Reboita et al., 2019) and therefore the gas transfer velocity could enhance the CO2 flux into the subtropical ocean through higher (or lower) wind speeds in winter (or summer; Xiong et al., 2015). Since seasonal variations in ΔpCO2 largely explain the seasonal variability in the CO2 flux, ΔpCO2 can be used as a proxy to understand seasonal variations in the CO2 flux in this region.
4.2 Inter-annual drivers of ΔpCO2 and CO2 flux
The larger geographic region of significant correlations for the air–sea CO2 flux compared to ΔpCO2 and the consistency between the two results (i.e. comparing the smaller regions of ΔpCO2 correlations with their equivalent in the flux results; Figs. 3, 4) suggest that analysing the CO2 flux is the better dataset to investigate drivers of variations on inter-annual and longer timescales. The results become clearer when analysing the CO2 flux, in which the effects of solubility and gas transfer (estimated via wind speed proxy) could reinforce correlations and multi-year trends, which will be retrieved by performing long time series analyses on the CO2 flux. Landschützer et al. (2015) showed that variations in the Southern Ocean carbon sink were primarily driven by changes in ΔpCO2, when integrating across basin scales. At localized scales of 1∘ by 1∘ as performed in our analysis, changes in surface turbulence and solubility are shown to be important in determining inter-annual variability, consistent with Keppler and Landschützer (2019). In the North Atlantic Ocean, Henson et al. (2018) showed that the seasonal and inter-annual drivers of ΔpCO2 are different, which could arise from the necessity to study CO2 fluxes over longer timescales.
The inter-annual component of NCP and the CO2 flux were negatively correlated in the subtropical gyre (Fig. 4a), alongside a positive correlation between SST and CO2 flux (Fig. 4b). El Niño (La Niña) events are known to influence the South Atlantic Ocean, causing an increase (decrease) in SST across the basin (Rodrigues et al., 2015; Colberg et al., 2004) and a decrease (increase) in NPP and NCP (Ford et al., 2021b; Tilstone et al., 2015). Positive correlations between the MEI and CO2 flux (Fig. 4e) indicate that the MEI partially controls the inter-annual variability in CO2 flux in the South Atlantic subtropical gyre through modulations primarily in SST and to a lesser extent NCP. The South Atlantic Subtropical Anticyclone has been observed to strengthen (weaken) and move south (north) during La Niña (El Niño) events. This displacement increases (decreases) wind speeds across the subtropical South Atlantic, which will enhance (weaken) gas exchange and elevate (depress) NCP (Ford et al., 2021b). These results suggest a more significant role of NCP in controlling the inter-annual variability in the CO2 flux than has previously been thought.
The negative correlation between the CO2 flux and the MEI in a band between 30 and 45∘ S (Fig. 4e) indicates that reduced (elevated) wind speeds that occur during La Niña (El Niño) events in this region suppress (enhance) the gas exchange (Colberg et al., 2004) and therefore act as a weaker (stronger) CO2 sink. In the equatorial region, neither ΔpCO2 or the CO2 flux were correlated with the MEI, in sharp contrast with Lefèvre et al. (2013) who showed stronger outgassing of CO2 in the western equatorial Atlantic for the year following the 2009 El Niño. In that respect, it should be noted that our analysis would not identify such lagged correlations.
The SAM has known meteorological connections to the MEI (Fogt et al., 2011), in which El Niño (La Niña) events generally coincide with negative (positive) SAM phases, resulting in northward (southward) displacement of the westerly winds in the Southern Ocean. Our results showed positive correlations between the CO2 flux and the SAM between 30 and 45∘ S (Fig. 4f) indicating stronger (weaker) CO2 drawdown into the oceans during negative (positive) SAM phases. Although no significant correlations were found between ΔpCO2 and the SAM (Fig. 3f), the changes in the gas transfer driven by the displacement of the westerly winds could control the CO2 flux. It should be noted that the effect of the SAM may be more pronounced outside the domain of the present study (i.e. south of 45∘ S; Keppler and Landschützer, 2019). Landschützer et al. (2015) indicated that the SAM is unlikely to be the main driver of changes in the Southern Ocean CO2 flux, but an observed zonally asymmetric atmospheric pattern could induce changes in the CO2 flux (Keppler and Landschützer, 2019; Landschützer et al., 2015). This asymmetric atmospheric pattern, however, may not be captured within the SAM index.
4.3 Multi-year trends in ΔpCO2 and CO2 flux
The trends in ΔpCO2 and CO2 flux over 16 years (Fig. 5) showed some similarities to previous trend assessments in the South Atlantic Ocean (Landschützer et al., 2016). Our results indicated a lower number of significant trends, however, since uncertainties in the trend analysis were accounted for. The uncertainties in both the pCO2 (sw) estimates from extrapolation techniques and the gas transfer velocity are rarely propagated through previous trend analyses. By accounting for these uncertainties, the trend analyses provide a robust depiction of regions that can confidently be determined as changing. As with the seasonal and inter-annual analysis, the CO2 flux-based trend analysis showed a greater spatial area of significant trends when compared to ΔpCO2 (Fig. 5).
The strongest trends in ΔpCO2 and the CO2 flux were observed in the Benguela upwelling system. Arnone et al. (2017) reported positive trends in in situ pCO2 (sw) of 6.1 ± 1.4 µatm yr−1, between 2005 and 2015. Assuming an atmospheric CO2 increase of 1.5 µatm yr−1 (Takahashi et al., 2002; Zeng et al., 2014), these results are consistent with the ΔpCO2 trends observed in this study (1.5 ± 1.1–3.8 ± 1.1 µatm yr−1, Fig. 5a). Arnone et al. (2017) also suggested that the positive trend was due to a stronger influence of upwelling (Rouault et al., 2010), which injects CO2 and nutrients into the area that is then not completely removed by the enhanced NPP/NCP. Varela et al. (2015) indicated an increase in the strength of the Benguela upwelling. By contrast, Lamont et al. (2018) showed no significant change in upwelling in the southern Benguela but increases in the northern Benguela that are consistent with our data, which highlight an increasing efflux of CO2 to the atmosphere (Fig. 5b). The CO2 flux trends in this study (0.03 ± 0.01–0.09 ± 0.02 mol m−2 yr−1, Fig. 5b) were also consistent with but slightly lower than the 0.13 ± 0.03 mol m−2 yr−1 trend in CO2 flux observed by Arnone et al. (2017). An increase in the strength of the upwelling that injects CO2 into the surface layer will be driven by enhanced (upwelling-conducive) winds that also enhance the gas transfer. This highlights the importance of studying multi-year trends using the CO2 flux because the enhancement of these trends by meteorological conditions would not be observed using ΔpCO2 alone.
Offshore from the upwelling region negative ΔpCO2 and CO2 flux trends were observed. Rubio et al. (2009) showed that mesoscale filaments and eddies propagate away from the upwelling front, transporting nutrients offshore into the South Atlantic gyre. Ford et al. (2021b) showed negative correlations between sea level height anomalies (SLHAs) and NPP/NCP anomalies (negative SLHAs; positive NCP/NPP), indicating an influence of mesoscale features on ΔpCO2 and the CO2 flux. Xiu et al. (2018) indicated that an increase in upwelling-conducive winds could increase the number of mesoscale eddies, which would transport nutrients offshore of the Californian upwelling. Although the Benguela and Californian upwelling systems are not identical, these connections could suggest an elevated nutrient export offshore, driving elevated NPP/NCP, which would increase the CO2 sink. Kulk et al. (2020) showed significant increases in NPP of ∼ 2 % yr−1 between 1998 and 2018 in the region of strong negative trends in the CO2 flux observed in this study, which supports the contribution of NCP to multi-year trends in the CO2 flux.
There were also positive trends in ΔpCO2 and CO2 flux in the equatorial Atlantic. In the eastern equatorial Atlantic, Lefèvre et al. (2016) previously suggested a negative trend in in situ ΔpCO2, between 2006 and 2013, but indicated that the trend may be biased by extreme events at either end of the record. From 1995 to 2007, Parard et al. (2010) indicated a greater increase in in situ pCO2 (sw) than pCO2 (atm) (increasing ΔpCO2), but the trend was derived from data from only two research cruises. For the equatorial upwelling, an increase in ΔpCO2 (as shown here and in Landschützer et al., 2016) is counter intuitive because there is evidence that upwelled water has recently been in contact with the atmosphere (∼ 15 years; Reverdin et al., 1993). Dissolved inorganic carbon in these upwelled waters has been shown to increase at a similar rate to the surface waters (e.g. Woosley et al., 2016). Therefore, the trend in ΔpCO2 should be ∼ 0 with increasing pCO2 (atm). This could suggest a missing component within the SA-FNN to estimate pCO2 (sw), such as changes in the biological export efficiency (Kim et al., 2019), which could then suppress upwelling-induced CO2 outgassing.
The western Tropical Atlantic, in the vicinity of the Amazon plume, also showed positive trends in ΔpCO2 and CO2 flux. Previous studies have not investigated the trends in ΔpCO2 or CO2 flux in the Amazon plume; however, the carbon retention in a coloured ocean site (CARIACO), situated to the northwest, displayed positive trends in pCO2 (sw) of 2.95 ± 0.43 µatm yr−1 (Bates et al., 2014). Araujo et al. (2019) identified a positive trend in pCO2 (sw) of 1.20 µatm yr−1 but a trend in pCO2 (atm) of 1.70 µatm yr−1 (i.e. decreasing ΔpCO2) for the northeast Brazilian coast, although the air–sea CO2 flux and ΔpCO2 within the Amazon plume region are spatially and temporally variable (Valerio et al., 2021; Ibánhez et al., 2016; Bruto et al., 2017).
The South Atlantic gyre exhibited negative trends in ΔpCO2 and the CO2 flux indicating an increasing drawdown of atmospheric CO2 into the ocean, which were consistent with Landschützer et al. (2016) over the period from 1982 and 2011 though the trends were at the limits of the uncertainties (Fig. B2). Fay and Mckinley (2013) showed weak negative trends in ΔpCO2 using in situ observations over different time series lengths. Using an ensemble of complete pCO2 (sw) fields, Gregor et al. (2019) indicated negative trends in ΔpCO2; however, there was low confidence in these trends especially in the South Atlantic gyre. By contrast, Kitidis et al. (2017) reported a mean trend in in situ ΔpCO2 between 1995 and 2013 that was not significantly different from zero. These contradictory trends support the conclusion that ΔpCO2 is unlikely to be representative of the CO2 flux over multi-year timescales. Therefore, we recommend that the CO2 flux should be used to assess multi-year variability in the oceanic CO2 sink, as the importance of changes in solubility and gas transfer velocity (estimated via wind speed) increases (Keppler and Landschützer, 2019).
During the United Nations decade of ocean science (2021–2030) , the Integrated Ocean Carbon Research (IOC-R) highlights that the role of biology is a key issue to understanding the global ocean CO2 sink (Aricò et al., 2021). The biological contribution to both inter-annual and multi-year variations in the South Atlantic air–sea CO2 flux shown in this study, and supported by Ford et al. (2022), indicates that the biological activity through NCP cannot be assumed to be in steady state. The biological effect of NCP on ΔpCO2 and CO2 flux should therefore not be overlooked when assessing the inter-annual and multi-year variations in the global ocean carbon sink.
In this paper, we have investigated the seasonal and inter-annual drivers of ΔpCO2 and the air–sea CO2 flux in the South Atlantic Ocean using satellite observations. Seasonally, our results indicated that the subtropics were controlled by SST, and the subpolar regions were correlated with biological processes. Deviations from this trend occurred in the Benguela upwelling where predominately biological processes correlated with variability in the ΔpCO2, as well as upwelling. The equatorial Atlantic showed spatially variable drivers associated with the Amazon plume and equatorial upwelling which induced a biological effect. These regions imply a strong biological control on ΔpCO2 through local physical processes. The CO2 flux had similar seasonal drivers to ΔpCO2 but with significant correlations over a larger spatial area. This highlights that ΔpCO2 can be used to indicate the important drivers of the CO2 flux on seasonal timescales, but it is still possible that ΔpCO2 will miss some of the spatial correlations and will likely overestimate the strength of these correlations.
The inter-annual variability in ΔpCO2 and the CO2 flux was correlated with the MEI through a reduction (increase) of NCP and increase (decrease) in SST during El Niño (La Niña) events, again highlighting the importance of biology to the inter-annual variability. The CO2 flux response extended over a larger geographical region, indicating that the CO2 flux should be used to assess inter-annual trends in the oceanic CO2 sink, as opposed to a proxy such as ΔpCO2, which may overestimate the strength of the correlations and does not include variability in the solubility and the gas transfer velocity (estimated via wind speed). The 16-year trends in ΔpCO2 and the CO2 flux were determined with associated uncertainties which identified negative trends in the CO2 flux in the South Atlantic gyre. Positive trends in the CO2 flux were observed in the Benguela upwelling region, which were associated with an increase in the strength and frequency of upwelling. A transition to negative trends offshore were consistent with elevated nutrient export from the upwelling area and subsequent biological drawdown of CO2. These results highlight that changes in biological activity in the South Atlantic Ocean can control the inter-annual and multi-year trends in the oceanic CO2 flux. This emphasizes the importance of biology and specifically NCP in assessing the global ocean carbon sink.
Henson et al. (2018) performed the X-11 analysis using in situ pCO2 (sw) observations to estimate average ΔpCO2 for the Longhurst provinces (Longhurst, 1998). The in situ pCO2 (sw) observations were obtained from SOCATv2020 (https://www.socat.info/, last access: 16 June 2020; Bakker et al., 2016) and were reanalysed to a temperature dataset representative of a consistent and fixed depth (Reynolds et al., 2002) which is used to represent the base of the mass boundary layer. The reanalysis method used the “fe_reanalyse_socat.py” routine within FluxEngine (Holding et al., 2019; Shutler et al., 2016), which follows the methodology of Goddijn-Murphy et al. (2015) and that used in Woolf et al. (2019) and Watson et al. (2020). ΔpCO2 was calculated using the reanalysed in situ pCO2 (sw) observations and pCO2 (atm). These ΔpCO2 estimates were used within the driver analysis as described by Henson et al. (2018), using the drivers described in Sect. 2.4 for the South Atlantic Longhurst provinces (Longhurst, 1998). The seasonal drivers of in situ ΔpCO2 (Fig. A1) showed a similar spatial distribution as the SA-FNN ΔpCO2 (Fig. 1). The inter-annual drivers (Fig. A2) showed some differences to the SA-FNN (Fig. 3). The averaging required to produce the in situ ΔpCO2 time series may mask inter-annual signals, and Ford et al. (2021b) indicated that averaging over large province areas could mask correlations, especially in dynamic regions, and locally these correlations may be significant.
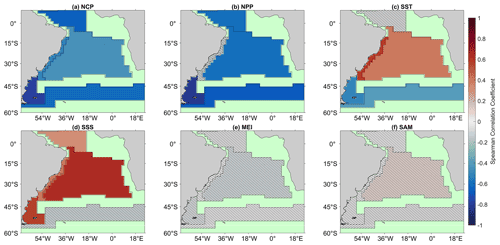
Figure A1Spearman correlations between the in situ ΔpCO2 seasonal component of the X-11 analysis and (a) net community production (NCP), (b) net primary production (NPP), (c) sea surface temperature (SST), (d) sea surface salinity (SSS), (e) multivariate ENSO index (MEI), and (f) Southern Annular Mode (SAM) seasonal components on a per province basis. Hashed areas indicate no significant correlations, and green regions indicate no analysis was performed due to missing data.
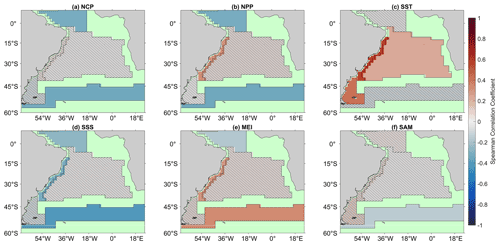
Figure A2Spearman correlations between the in situ ΔpCO2 inter-annual component of the X-11 analysis and (a) net community production (NCP), (b) net primary production (NPP), (c) sea surface temperature (SST), (d) sea surface salinity (SSS), (e) multivariate ENSO index (MEI), and (f) Southern Annular Mode (SAM) inter-annual components on a per province basis. Hashed areas indicate no significant correlations, and green regions indicate no analysis was performed due to missing data.
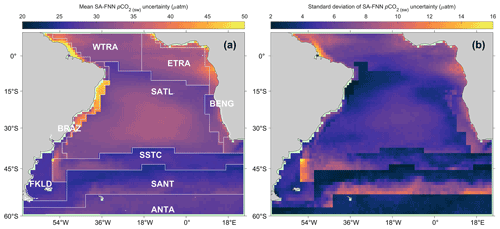
Figure B1(a) Mean SA-FNN pCO2 (sw) uncertainty between July 2002 and December 2018. Longhurst provinces (Longhurst, 1998) used within the SA-FNN training described in Ford et al. (2022; note the WTRA and ETRA are merged into one province). The province area acronyms are listed as follows: WTRA is western tropical Atlantic, ETRA is eastern equatorial Atlantic, SATL is South Atlantic gyre, BRAZ is the coastal Brazilian Current, BENG is Benguela Current coastal upwelling, FKLD is southwest Atlantic shelves, SSTC is South Subtropical Convergence, SANT is sub-Antarctic, and ANTA is Antarctic. (b) Standard deviation of SA-FNN pCO2 (sw) uncertainty.
Moderate Resolution Imaging Spectroradiometer on Aqua (MODIS-A) estimates of chlorophyll a (https://doi.org/10.5067/AQUA/MODIS/L3M/CHL/2018, NASA OBPG, 2017a), photosynthetically active radiation (https://doi.org/10.5067/AQUA/MODIS/L3M/PAR/2018, NASA OBPG, 2017b), and sea surface temperature (https://doi.org/10.5067/MODSA-1D4D4, NASA OBPG, 2015) are available from the National Aeronautics Space Administration (NASA) Ocean Colour website (https://oceancolor.gsfc.nasa.gov/, last access: 10 December 2020). Modelled sea surface salinity from the Copernicus Marine Environment Modelling Service global ocean physics reanalysis product (GLORYS12V1) is available from CMEMS (https://doi.org/10.48670/moi-00021, CMEMS, 2021). ERA5 monthly reanalysis wind speeds are available from the Copernicus Climate Data Store (https://doi.org/10.24381/cds.f17050d7, Hersbach et al., 2019). pCO2 (atm) data are available from v5.5 of the global estimates of pCO2 (sw) dataset (https://doi.org/10.7289/v5z899n6, Landschützer et al., 2017, 2016). pCO2 (sw) estimates generated by the SA-FNN are available from Pangaea (https://doi.org/10.1594/PANGAEA.935936, Ford et al., 2021a). SOCATv2020 in situ pCO2 (sw) observations (Bakker et al., 2016) are available from https://www.socat.info/index.php/version-2020/.
DJF, GHT, JDS, and VK conceived and directed the research. DJF developed the code and prepared the manuscript. GHT, JDS, and VK provided comments that shaped the final manuscript.
The contact author has declared that none of the authors has any competing interests.
Publisher’s note: Copernicus Publications remains neutral with regard to jurisdictional claims in published maps and institutional affiliations.
We thank the Atlantic Meridional Transect funded by the UK Natural Environment Research Council through its National Capability Long-term Single Centre Science Programme, Climate Linked Atlantic Sector Science (grant no. NE/R015953/1). This study contributes to the international Integrated Marine Biosphere Research (IMBeR) project and is contribution number 372 of the AMT programme. We also thank the Natural Environment Research Council Earth Observation Data Acquisition and Analysis Service (NEODAAS) for use of the Linux cluster to process the MODIS-A satellite imagery. We thank two anonymous reviewers for their comments, which have improved the manuscript.
The Surface Ocean CO2 Atlas (SOCAT) is an international effort, endorsed by the International Ocean Carbon Coordination Project (IOCCP), the Surface Ocean Lower Atmosphere Study (SOLAS), and the IMBeR programme, to deliver a uniformly quality-controlled surface ocean CO2 database. The many researchers and funding agencies responsible for the collection of data and quality control are thanked for their contributions to SOCAT.
Daniel J. Ford was supported by a NERC GW4+ Doctoral Training Partnership studentship from the UK Natural Environment Research Council (NERC (grant no. NE/L002434/1)). Gavin H. Tilstone and Vassilis Kitidis were supported by the AMT4CO2Flux (grant no. 4000125730/18/NL/FF/gp) contract from the European Space Agency and by the NERC National Capability funding to Plymouth Marine Laboratory for the Atlantic Meridional Transect (CLASS-AMT (grant no. NE/R015953/1)).
This paper was edited by Koji Suzuki and reviewed by two anonymous referees.
Aguiar, A. L., Cirano, M., Marta-Almeida, M., Lessa, G. C., and Valle-Levinson, A.: Upwelling processes along the South Equatorial Current bifurcation region and the Salvador Canyon (13∘ S), Brazil, Cont. Shelf Res., 171, 77–96, https://doi.org/10.1016/j.csr.2018.10.001, 2018.
Araujo, M., Noriega, C., Medeiros, C., Lefèvre, N., Ibánhez, J. S. P., Flores Montes, M., Silva, A. C. da, and Santos, M. de L.: On the variability in the CO2 system and water productivity in the western tropical Atlantic off North and Northeast Brazil, J. Mar. Syst., 189, 62–77, https://doi.org/10.1016/j.jmarsys.2018.09.008, 2019.
Aricò, S., Arrieta, J. M., Bakker, D. C. E., Boyd, P. W., Cotrim da Cunha, L., Chai, F., Dai, M., Gruber, N., Isensee, K., Ishii, M., Jiao, N., Lauvset, S. K., McKinley, G. A., Monteiro, P., Robinson, C., Sabine, C., Sanders, R., Schoo, K. L., Schuster, U., Shutler, J. D., Thomas, H., Wanninkhof, R., Watson, A. J., Bopp, L., Boss, E., Bracco, A., Cai, W., Fay, A., Feely, R. A., Gregor, L., Hauck, J., Heinze, C., Henson, S., Hwang, J., Post, J., Suntharalingam, P., Telszewski, M., Tilbrook, B., Valsala, V., and Rojas, A.: Integrated Ocean Carbon Research: A Summary of Ocean Carbon Research, and Vision of Coordinated Ocean Carbon Research and Observations for the Next Decade, edited by: Wanninkhof, R., Sabine, C., and Aricò, S., UNESCO, Paris, 46 pp., https://doi.org/10.25607/h0gj-pq41, 2021.
Arnone, V., González-Dávila, M., and Magdalena Santana-Casiano, J.: CO2 fluxes in the South African coastal region, Mar. Chem., 195, 41–49, https://doi.org/10.1016/j.marchem.2017.07.008, 2017.
Bakker, D. C. E., Pfeil, B., Landa, C. S., Metzl, N., O'Brien, K. M., Olsen, A., Smith, K., Cosca, C., Harasawa, S., Jones, S. D., Nakaoka, S., Nojiri, Y., Schuster, U., Steinhoff, T., Sweeney, C., Takahashi, T., Tilbrook, B., Wada, C., Wanninkhof, R., Alin, S. R., Balestrini, C. F., Barbero, L., Bates, N. R., Bianchi, A. A., Bonou, F., Boutin, J., Bozec, Y., Burger, E. F., Cai, W.-J., Castle, R. D., Chen, L., Chierici, M., Currie, K., Evans, W., Featherstone, C., Feely, R. A., Fransson, A., Goyet, C., Greenwood, N., Gregor, L., Hankin, S., Hardman-Mountford, N. J., Harlay, J., Hauck, J., Hoppema, M., Humphreys, M. P., Hunt, C. W., Huss, B., Ibánhez, J. S. P., Johannessen, T., Keeling, R., Kitidis, V., Körtzinger, A., Kozyr, A., Krasakopoulou, E., Kuwata, A., Landschützer, P., Lauvset, S. K., Lefèvre, N., Lo Monaco, C., Manke, A., Mathis, J. T., Merlivat, L., Millero, F. J., Monteiro, P. M. S., Munro, D. R., Murata, A., Newberger, T., Omar, A. M., Ono, T., Paterson, K., Pearce, D., Pierrot, D., Robbins, L. L., Saito, S., Salisbury, J., Schlitzer, R., Schneider, B., Schweitzer, R., Sieger, R., Skjelvan, I., Sullivan, K. F., Sutherland, S. C., Sutton, A. J., Tadokoro, K., Telszewski, M., Tuma, M., van Heuven, S. M. A. C., Vandemark, D., Ward, B., Watson, A. J., and Xu, S.: A multi-decade record of high-quality fCO2 data in version 3 of the Surface Ocean CO2 Atlas (SOCAT), Earth Syst. Sci. Data, 8, 383–413, https://doi.org/10.5194/essd-8-383-2016, 2016 (data available at: https://www.socat.info/index.php/version-2020/, last access: 16 June 2020).
Bates, N. R., Astor, Y. M., Church, M. J., Currie, K., Dore, J. E., González-Dávila, M., Lorenzoni, L., Muller-Karger, F., Olafsson, J., and Santana-Casiano, J. M.: A time-series view of changing surface ocean chemistry due to ocean uptake of anthropogenic CO2 and ocean acidification, Oceanography, 27, 126–141, https://doi.org/10.5670/oceanog.2014.16, 2014.
Bonou, F. K., Noriega, C., Lefèvre, N., and Araujo, M.: Distribution of CO2 parameters in the Western Tropical Atlantic Ocean, Dyn. Atmos. Ocean., 73, 47–60, https://doi.org/10.1016/j.dynatmoce.2015.12.001, 2016.
Bruto, L., Araujo, M., Noriega, C., Veleda, D., and Lefèvre, N.: Variability of CO2 fugacity at the western edge of the tropical Atlantic Ocean from the 8∘ N to 38∘ W PIRATA buoy, Dyn. Atmos. Ocean., 78, 1–13, https://doi.org/10.1016/j.dynatmoce.2017.01.003, 2017.
CMEMS: Copernicus Marine Modelling Service global ocean physics reanalysis product (GLORYS12V1), Copernicus Mar. Model. Serv. [data set], https://doi.org/10.48670/moi-00021, 2021.
Colberg, F., Reason, C. J. C., and Rodgers, K.: South Atlantic response to El Niño-Southern Oscillation induced climate variability in an ocean general circulation model, J. Geophys. Res.-Oceans, 109, 1–14, https://doi.org/10.1029/2004JC002301, 2004.
Cooley, S. R., Coles, V. J., Subramaniam, A., and Yager, P. L.: Seasonal variations in the Amazon plume-related atmospheric carbon sink, Global Biogeochem. Cy., 21, 1–15, https://doi.org/10.1029/2006GB002831, 2007.
Dickson, A. G., Sabine, C. L., and Christian, J. R.: Guide to Best Practices for Ocean CO2 measurements, PICES Special Publication, IOCCP Report No. 8, 191 p., 2007.
Dong, Y., Yang, M., Bakker, D. C. E., Kitidis, V., and Bell, T. G.: Uncertainties in eddy covariance air–sea CO2 flux measurements and implications for gas transfer velocity parameterisations, Atmos. Chem. Phys., 21, 8089–8110, https://doi.org/10.5194/acp-21-8089-2021, 2021.
Donlon, C. J., Nightingale, T. J., Sheasby, T., Turner, J., Robinson, I. S., and Emergy, W. J.: Implications of the oceanic thermal skin temperature deviation at high wind speed, Geophys. Res. Lett., 26, 2505–2508, https://doi.org/10.1029/1999GL900547, 1999.
Fay, A. R. and McKinley, G. A.: Global trends in surface ocean pCO2 from in situ data, Global Biogeochem. Cy., 27, 541–557, https://doi.org/10.1002/gbc.20051, 2013.
Fogt, R. L., Bromwich, D. H., and Hines, K. M.: Understanding the SAM influence on the South Pacific ENSO teleconnection, Clim. Dyn., 36, 1555–1576, https://doi.org/10.1007/s00382-010-0905-0, 2011.
Ford, D. J., Tilstone, G. H., Shutler, J. D., and Kitidis, V.: Interpolated surface ocean carbon dioxide partial pressure for the South Atlantic Ocean (2002–2018) using different biological parameters, Pangaea [data set], https://doi.org/10.1594/PANGAEA.935936, 2021a.
Ford, D., Tilstone, G. H., Shutler, J. D., Kitidis, V., Lobanova, P., Schwarz, J., Poulton, A. J., Serret, P., Lamont, T., Chuqui, M., Barlow, R., Lozano, J., Kampel, M., and Brandini, F.: Wind speed and mesoscale features drive net autotrophy in the South Atlantic Ocean, Remote Sens. Environ., 260, 112435, https://doi.org/10.1016/j.rse.2021.112435, 2021b.
Ford, D. J., Tilstone, G. H., Shutler, J. D., and Kitidis, V.: Derivation of seawater pCO2 from net community production identifies the South Atlantic Ocean as a CO2 source, Biogeosciences, 19, 93–115, https://doi.org/10.5194/bg-19-93-2022, 2022.
Friedlingstein, P., O'Sullivan, M., Jones, M. W., Andrew, R. M., Hauck, J., Olsen, A., Peters, G. P., Peters, W., Pongratz, J., Sitch, S., Le Quéré, C., Canadell, J. G., Ciais, P., Jackson, R. B., Alin, S., Aragão, L. E. O. C., Arneth, A., Arora, V., Bates, N. R., Becker, M., Benoit-Cattin, A., Bittig, H. C., Bopp, L., Bultan, S., Chandra, N., Chevallier, F., Chini, L. P., Evans, W., Florentie, L., Forster, P. M., Gasser, T., Gehlen, M., Gilfillan, D., Gkritzalis, T., Gregor, L., Gruber, N., Harris, I., Hartung, K., Haverd, V., Houghton, R. A., Ilyina, T., Jain, A. K., Joetzjer, E., Kadono, K., Kato, E., Kitidis, V., Korsbakken, J. I., Landschützer, P., Lefèvre, N., Lenton, A., Lienert, S., Liu, Z., Lombardozzi, D., Marland, G., Metzl, N., Munro, D. R., Nabel, J. E. M. S., Nakaoka, S.-I., Niwa, Y., O'Brien, K., Ono, T., Palmer, P. I., Pierrot, D., Poulter, B., Resplandy, L., Robertson, E., R¨denbeck, C., Schwinger, J., Séférian, R., Skjelvan, I., Smith, A. J. P., Sutton, A. J., Tanhua, T., Tans, P. P., Tian, H., Tilbrook, B., van der Werf, G., Vuichard, N., Walker, A. P., Wanninkhof, R., Watson, A. J., Willis, D., Wiltshire, A. J., Yuan, W., Yue, X., and Zaehle, S.: Global Carbon Budget 2020, Earth Syst. Sci. Data, 12, 3269–3340, https://doi.org/10.5194/essd-12-3269-2020, 2020.
Goddijn-Murphy, L. M., Woolf, D. K., Land, P. E., Shutler, J. D., and Donlon, C.: The OceanFlux Greenhouse Gases methodology for deriving a sea surface climatology of CO2 fugacity in support of air–sea gas flux studies, Ocean Sci., 11, 519–541, https://doi.org/10.5194/os-11-519-2015, 2015.
González-Dávila, M., Santana-Casiano, J. M., and Ucha, I. R.: Seasonal variability of fCO2 in the Angola-Benguela region, Prog. Oceanogr., 83, 124–133, https://doi.org/10.1016/j.pocean.2009.07.033, 2009.
Gregor, L., Lebehot, A. D., Kok, S., and Scheel Monteiro, P. M.: A comparative assessment of the uncertainties of global surface ocean CO2 estimates using a machine-learning ensemble (CSIR-ML6 version 2019a) – have we hit the wall?, Geosci. Model Dev., 12, 5113–5136, https://doi.org/10.5194/gmd-12-5113-2019, 2019.
Henley, S. F., Cavan, E. L., Fawcett, S. E., Kerr, R., Monteiro, T., Sherrell, R. M., Bowie, A. R., Boyd, P. W., Barnes, D. K. A., Schloss, I. R., Marshall, T., Flynn, R., and Smith, S.: Changing Biogeochemistry of the Southern Ocean and Its Ecosystem Implications, Front. Mar. Sci., 7, 1–31, https://doi.org/10.3389/fmars.2020.00581, 2020.
Henson, S. A., Humphreys, M. P., Land, P. E., Shutler, J. D., Goddijn-Murphy, L., and Warren, M.: Controls on Open-Ocean North Atlantic ΔpCO2 at Seasonal and Interannual Time Scales Are Different, Geophys. Res. Lett., 45, 9067–9076, https://doi.org/10.1029/2018GL078797, 2018.
Hersbach, H., Bell, B., Berrisford, P., Biavati, G., Horányi, A., Muñoz Sabater, J., Nicolas, J., Peubey, C., Radu, R., Rozum, I., Schepers, D., Simmons, A., Soci, C., Dee, D., and Thépaut, J.-N.: ERA5 monthly averaged data on single levels from 1979 to present, Copernicus Clim. Chang. Serv. Clim. Data Store [data set], https://doi.org/10.24381/cds.f17050d7, 2019.
Ho, D. T., Law, C. S., Smith, M. J., Schlosser, P., Harvey, M., and Hill, P.: Measurements of air-sea gas exchange at high wind speeds in the Southern Ocean: Implications for global parameterizations, Geophys. Res. Lett., 33, L16611, https://doi.org/10.1029/2006GL026817, 2006.
Holding, T., Ashton, I. G., Shutler, J. D., Land, P. E., Nightingale, P. D., Rees, A. P., Brown, I., Piolle, J.-F., Kock, A., Bange, H. W., Woolf, D. K., Goddijn-Murphy, L., Pereira, R., Paul, F., Girard-Ardhuin, F., Chapron, B., Rehder, G., Ardhuin, F., and Donlon, C. J.: The FluxEngine air–sea gas flux toolbox: simplified interface and extensions for in situ analyses and multiple sparingly soluble gases, Ocean Sci., 15, 1707–1728, https://doi.org/10.5194/os-15-1707-2019, 2019.
Hopkins, J., Lucas, M., Dufau, C., Sutton, M., Stum, J., Lauret, O., and Channelliere, C.: Detection and variability of the Congo River plume from satellite derived sea surface temperature, salinity, ocean colour and sea level, Remote Sens. Environ., 139, 365–385, https://doi.org/10.1016/j.rse.2013.08.015, 2013.
Hu, C., Lee, Z., and Franz, B.: Chlorophyll a algorithms for oligotrophic oceans: A novel approach based on three-band reflectance difference, J. Geophys. Res.-Oceans, 117, 1–25, https://doi.org/10.1029/2011JC007395, 2012.
Ibánhez, J. S. P., Araujo, M., and Lefèvre, N.: The overlooked tropical oceanic CO2 sink, Geophys. Res. Lett., 43, 3804–3812, https://doi.org/10.1002/2016GL068020, 2016.
IPCC: Climate Change 2021: The Physical Science Basis. Contribution of Working Group I to the Sixth Assessment Report of the Intergovernmental Panel on Climate Change, edited by: Masson-Delmotte, V., Zhai, P., Pirani, A., Connors, S. L., Péan, C., Berger, S., Caud, N., Chen, Y., Goldfarb, L., Gomis, M. I., Huang, M., Leitzell, K., Lonnoy, E., Matthews, J. B. R., Maycock, T. K., Waterfield, T., Yelekçi, O., Yu, R., and Zhou, B., Cambridge University Press, https://www.ipcc.ch/report/ar6/wg1/downloads/report/IPCC_AR6_WGI_FullReport.pdf (last access: 28 November 2021), 2021.
Jean-Michel, L., Eric, G., Romain, B.-B., Gilles, G., Angélique, M., Marie, D., Clément, B., Mathieu, H., Olivier, L. G., Charly, R., Tony, C., Charles-Emmanuel, T., Florent, G., Giovanni, R., Mounir, B., Yann, D., and Pierre-Yves, L. T.: The Copernicus Global ∘ Oceanic and Sea Ice GLORYS12 Reanalysis, Front. Earth Sci., 9, 1–27, https://doi.org/10.3389/feart.2021.698876, 2021.
Kendall, M. G.: Rank Correlation Methods, 4th edn., Charles Griffin, London, UK, ISBN: 9780852641996, 1975.
Keppler, L. and Landschützer, P.: Regional Wind Variability Modulates the Southern Ocean Carbon Sink, Sci. Rep., 9, 1–10, https://doi.org/10.1038/s41598-019-43826-y, 2019.
Kim, H. J., Kim, T., Hyeong, K., Yeh, S., Park, J., Yoo, C. M., and Hwang, J.: Suppressed CO2 Outgassing by an Enhanced Biological Pump in the Eastern Tropical Pacific, J. Geophys. Res.-Oceans, 124, 7962–7973, https://doi.org/10.1029/2019JC015287, 2019.
Kitidis, V., Brown, I., Hardman-mountford, N., and Lefèvre, N.: Surface ocean carbon dioxide during the Atlantic Meridional Transect (1995–2013); evidence of ocean acidification, Prog. Oceanogr., 158, 65–75, https://doi.org/10.1016/j.pocean.2016.08.005, 2017.
Körtzinger, A.: A significant CO2 sink in the tropical Atlantic Ocean associated with the Amazon River plume, Geophys. Res. Lett., 30, 2–5, https://doi.org/10.1029/2003GL018841, 2003.
Kulk, G., Platt, T., Dingle, J., Jackson, T., Jönsson, B. F., Bouman, H. A., Babin, M., Brewin, R. J. W., Doblin, M., Estrada, M., Figueiras, F. G., Furuya, K., González-Benítez, N., Gudfinnsson, H. G., Gudmundsson, K., Huang, B., Isada, T., Kovač, Ž., Lutz, V. A., Marañón, E., Raman, M., Richardson, K., Rozema, P. D., van de Poll, W. H., Segura, V., Tilstone, G. H., Uitz, J., van Dongen-Vogels, V., Yoshikawa, T., and Sathyendranath, S.: Primary production, an index of climate change in the ocean: Satellite-based estimates over two decades, Remote Sens., 12, 826, https://doi.org/10.3390/rs12050826, 2020.
Lamont, T., Barlow, R. G., and Kyewalyanga, M. S.: Physical drivers of phytoplankton production in the southern Benguela upwelling system, Deep-Res. Pt. I, 90, 1–16, https://doi.org/10.1016/j.dsr.2014.03.003, 2014.
Lamont, T., García-Reyes, M., Bograd, S. J., van der Lingen, C. D., and Sydeman, W. J.: Upwelling indices for comparative ecosystem studies: Variability in the Benguela Upwelling System, J. Mar. Syst., 188, 3–16, https://doi.org/10.1016/j.jmarsys.2017.05.007, 2018.
Landschützer, P., Gruber, N., Bakker, D. C. E., Schuster, U., Nakaoka, S., Payne, M. R., Sasse, T. P., and Zeng, J.: A neural network-based estimate of the seasonal to inter-annual variability of the Atlantic Ocean carbon sink, Biogeosciences, 10, 7793–7815, https://doi.org/10.5194/bg-10-7793-2013, 2013.
Landschützer, P., Gruber, N., Bakker, D. C. E., and Schuster, U.: Recent variability of the global ocean carbon sink, Global Biogeochem. Cy., 28, 927–949, https://doi.org/10.1002/2014GB004853, 2014.
Landschützer, P., Gruber, N., Haumann, F. A., Rödenbeck, C., Bakker, D. C. E., van Heuven, S., Hoppema, M., Metzl, N., Sweeney, C., Takahashi, T., Tilbrook, B., and Wanninkhof, R.: The reinvigoration of the Southern Ocean carbon sink, Science, 349, 1221–1224, https://doi.org/10.1126/science.aab2620, 2015.
Landschützer, P., Gruber, N., and Bakker, D. C. E.: Decadal variations and trends of the global ocean carbon sink, Global Biogeochem. Cy., 30, 1396–1417, https://doi.org/10.1002/2015GB005359, 2016.
Landschützer, P., Gruber, N., and Bakker, D. C. E.: An observation-based global monthly gridded sea surface pCO2 product from 1982 onward and its monthly climatology (NCEI Accession 0160558), NOAA Natl. Centers Environ. Information [data set], https://doi.org/10.7289/v5z899n6, 2017.
Lefèvre, N., Guillot, A., Beaumont, L., and Danguy, T.: Variability of fCO2 in the Eastern Tropical Atlantic from a moored buoy, J. Geophys. Res.-Oceans, 113, C01015, https://doi.org/10.1029/2007JC004146, 2008.
Lefévre, N., Diverrés, D., and Gallois, F.: Origin of CO2 undersaturation in the western tropical Atlantic, Tellus B, 62, 595–607, https://doi.org/10.1111/j.1600-0889.2010.00475.x, 2010.
Lefèvre, N., Caniaux, G., Janicot, S., and Gueye, A. K.: Increased CO2 outgassing in February-May 2010 in the tropical Atlantic following the 2009 Pacific El Niño, J. Geophys. Res.-Oceans, 118, 1645–1657, https://doi.org/10.1002/jgrc.20107, 2013.
Lefèvre, N., Veleda, D., Araujo, M., and Caniaux, G.: Variability and trends of carbon parameters at a time series in the eastern tropical Atlantic, Tellus B, 68, 30305, https://doi.org/10.3402/tellusb.v68.30305, 2016.
Lefèvre, N., Tyaquiçã, P., Veleda, D., Perruche, C., and van Gennip, S. J.: Amazon River propagation evidenced by a CO2 decrease at 8∘ N, 38∘ W in September 2013, J. Mar. Syst., 211, 103419, https://doi.org/10.1016/j.jmarsys.2020.103419, 2020.
Lefèvre, N., Mejia, C., Khvorostyanov, D., Beaumont, L., and Koffi, U.: Ocean Circulation Drives the Variability of the Carbon System in the Eastern Tropical Atlantic, Oceans, 2, 126–148, https://doi.org/10.3390/oceans2010008, 2021.
Liutti, C. C., Kerr, R., Monteiro, T., Orselli, I. B. M., Ito, R. G., and Garcia, C. A. E.: Sea surface CO2 fugacity in the southwestern South Atlantic Ocean: An evaluation based on satellite-derived images, Mar. Chem., 236, 104020, https://doi.org/10.1016/j.marchem.2021.104020, 2021.
Longhurst, A.: Ecological geography of the sea, Academic Press, San Diego, ISBN: 9780124555211, 1998.
Mann, H. B.: Nonparametric Tests Against Trend, Econometrica, 13, 245, https://doi.org/10.2307/1907187, 1945.
Mason, E., Pascual, A., Gaube, P., Ruiz, S., Pelegrí, J. L., and Delepoulle, A.: Subregional characterization of mesoscale eddies across the Brazil-Malvinas Confluence, J. Geophys. Res.-Oceans, 122, 3329–3357, https://doi.org/10.1002/2016JC012611, 2017.
Morel, A.: Light and marine photosynthesis: a spectral model with geochemical and climatological implications, Prog. Oceanogr., 26, 263–306, https://doi.org/10.1016/0079-6611(91)90004-6, 1991.
NASA OBPG: MODIS Aqua Level 3 SST Thermal IR Daily 4 km Daytime v2014.0, NASA Phys. Oceanogr. DAAC [data set], https://doi.org/10.5067/MODSA-1D4D4, 2015.
NASA OBPG: MODIS-Aqua Level 3 Mapped Chlorophyll Data Version R2018.0, NASA Ocean Biol. DAAC [data set], https://doi.org/10.5067/AQUA/MODIS/L3M/CHL/2018, 2017a.
NASA OBPG: MODIS-Aqua Level 3 Mapped Photosynthetically Available Radiation Data Version R2018.0, NASA Ocean Biol. DAAC [data set], https://doi.org/10.5067/AQUA/MODIS/L3M/PAR/2018, 2017b.
Nightingale, P. D., Malin, G., Law, C. S., Watson, A. J., Liss, P. S., Liddicoat, M. I., Boutin, J., and Upstill-Goddard, R. C.: In situ evaluation of air-sea gas exchange parameterizations using novel conservative and volatile tracers, Global Biogeochem. Cy., 14, 373–387, https://doi.org/10.1029/1999GB900091, 2000.
O'Reilly, J. E. and Werdell, P. J.: Chlorophyll algorithms for ocean color sensors – OC4, OC5 & OC6, Remote Sens. Environ., 229, 32–47, https://doi.org/10.1016/j.rse.2019.04.021, 2019.
O'Reilly, J. E., Maritorena, S., Mitchell, B. G., Siegel, D. A., Carder, K. L., Garver, S. A., Kahru, M., and Mcclain, C.: Ocean color chlorophyll algorithms for SeaWiFS encompassing chlorophyll concentrations between, J. Geophys. Res., 103, 24937–24953, 1998.
Parard, G., Lefévre, N., and Boutin, J.: Sea water fugacity of CO2 at the PIRATA mooring at 6∘ S, 10∘ W, Tellus B, 62, 636–648, https://doi.org/10.1111/j.1600-0889.2010.00503.x, 2010.
Pezzulli, S., Stephenson, D. B., and Hannachi, A.: The variability of seasonality, J. Clim., 18, 71–88, https://doi.org/10.1175/JCLI-3256.1, 2005.
Radenac, M.-H., Jouanno, J., Tchamabi, C. C., Awo, M., Bourlès, B., Arnault, S., and Aumont, O.: Physical drivers of the nitrate seasonal variability in the Atlantic cold tongue, Biogeosciences, 17, 529–545, https://doi.org/10.5194/bg-17-529-2020, 2020.
Reboita, M. S., Ambrizzi, T., Silva, B. A., Pinheiro, R. F., and da Rocha, R. P.: The south atlantic subtropical anticyclone: Present and future climate, Front. Earth Sci., 7, 1–15, https://doi.org/10.3389/feart.2019.00008, 2019.
Reverdin, G., Weiss, R. F., and Jenkins, W. J.: Ventilation of the Atlantic Ocean equatorial thermocline, J. Geophys. Res., 98, 16289, https://doi.org/10.1029/93JC00976, 1993.
Reynolds, R. W., Rayner, N. A., Smith, T. M., Stokes, D. C., and Wang, W.: An improved in situ and satellite SST analysis for climate, J. Clim., 15, 1609–1625, https://doi.org/10.1175/1520-0442(2002)015<1609:AIISAS>2.0.CO;2, 2002.
Rodrigues, R. R., Campos, E. J. D., and Haarsma, R.: The impact of ENSO on the south Atlantic subtropical dipole mode, J. Clim., 28, 2691–2705, https://doi.org/10.1175/JCLI-D-14-00483.1, 2015.
Rouault, M., Pohl, B., and Penven, P.: Coastal oceanic climate change and variability from 1982 to 2009 around South Africa, African J. Mar. Sci., 32, 237–246, https://doi.org/10.2989/1814232x.2010.501563, 2010.
Rubio, A., Blanke, B., Speich, S., Grima, N., and Roy, C.: Mesoscale eddy activity in the southern Benguela upwelling system from satellite altimetry and model data, Prog. Oceanogr., 83, 288–295, https://doi.org/10.1016/j.pocean.2009.07.029, 2009.
Sabine, C. L., Hankin, S., Koyuk, H., Bakker, D. C. E., Pfeil, B., Olsen, A., Metzl, N., Kozyr, A., Fassbender, A., Manke, A., Malczyk, J., Akl, J., Alin, S. R., Bellerby, R. G. J., Borges, A., Boutin, J., Brown, P. J., Cai, W.-J., Chavez, F. P., Chen, A., Cosca, C., Feely, R. A., González-Dávila, M., Goyet, C., Hardman-Mountford, N., Heinze, C., Hoppema, M., Hunt, C. W., Hydes, D., Ishii, M., Johannessen, T., Key, R. M., Körtzinger, A., Landschützer, P., Lauvset, S. K., Lefèvre, N., Lenton, A., Lourantou, A., Merlivat, L., Midorikawa, T., Mintrop, L., Miyazaki, C., Murata, A., Nakadate, A., Nakano, Y., Nakaoka, S., Nojiri, Y., Omar, A. M., Padin, X. A., Park, G.-H., Paterson, K., Perez, F. F., Pierrot, D., Poisson, A., Ríos, A. F., Salisbury, J., Santana-Casiano, J. M., Sarma, V. V. S. S., Schlitzer, R., Schneider, B., Schuster, U., Sieger, R., Skjelvan, I., Steinhoff, T., Suzuki, T., Takahashi, T., Tedesco, K., Telszewski, M., Thomas, H., Tilbrook, B., Vandemark, D., Veness, T., Watson, A. J., Weiss, R., Wong, C. S., and Yoshikawa-Inoue, H.: Surface Ocean CO2 Atlas (SOCAT) gridded data products, Earth Syst. Sci. Data, 5, 145–153, https://doi.org/10.5194/essd-5-145-2013, 2013.
Santana-Casiano, J. M., González-Dávila, M., and Ucha, I. R.: Carbon dioxide fluxes in the Benguela upwelling system during winter and spring: A comparison between 2005 and 2006, Deep-Sea Res. Pt. II, 56, 533–541, https://doi.org/10.1016/j.dsr2.2008.12.010, 2009.
Sen, P. K.: Estimates of the Regression Coefficient Based on Kendall's Tau, J. Am. Stat. Assoc., 63, 1379–1389, https://doi.org/10.1080/01621459.1968.10480934, 1968.
Shiskin, J., Young, A. J., and Musgrave, J. C.: The X-11 variant of the Census Method II Seasonal Adjustment Program, US Dept of Commerce, 68 p., 1967.
Shutler, J. D., Land, P. E., Piolle, J. F., Woolf, D. K., Goddijn-Murphy, L., Paul, F., Girard-Ardhuin, F., Chapron, B., and Donlon, C. J.: FluxEngine: A flexible processing system for calculating atmosphere-ocean carbon dioxide gas fluxes and climatologies, J. Atmos. Ocean. Technol., 33, 741–756, https://doi.org/10.1175/JTECH-D-14-00204.1, 2016.
Smyth, T. J., Tilstone, G. H., and Groom, S. B.: Integration of radiative transfer into satellite models of ocean primary production, J. Geophys. Res.-Oceans, 110, 1–11, https://doi.org/10.1029/2004JC002784, 2005.
Takahashi, T., Sutherland, S. C., Sweeney, C., Poisson, A., Metzl, N., Tilbrook, B., Bates, N., Wanninkhof, R., Feely, R. A., Sabine, C., Olafsson, J., and Nojiri, Y.: Global sea–air CO2 flux based on climatological surface ocean pCO2, and seasonal biological and temperature effects, Deep-Sea Res. Pt. II, 49, 1601–1622, https://doi.org/10.1016/S0967-0645(02)00003-6, 2002.
Takahashi, T., Sutherland, S. C., Wanninkhof, R., Sweeney, C., Feely, R. A., Chipman, D. W., Hales, B., Friederich, G., Chavez, F., Sabine, C., Watson, A., Bakker, D. C. E., Schuster, U., Metzl, N., Yoshikawa-Inoue, H., Ishii, M., Midorikawa, T., Nojiri, Y., Körtzinger, A., Steinhoff, T., Hoppema, M., Olafsson, J., Arnarson, T. S., Tilbrook, B., Johannessen, T., Olsen, A., Bellerby, R., Wong, C. S., Delille, B., Bates, N. R., and de Baar, H. J. W.: Climatological mean and decadal change in surface ocean pCO2, and net sea-air CO2 flux over the global oceans, Deep.-Sea Res. Pt. II, 56, 554–577, https://doi.org/10.1016/j.dsr2.2008.12.009, 2009.
Tilstone, G. H., Smyth, T. J., Gowen, R. J., Martinez-Vicente, V., and Groom, S. B.: Inherent optical properties of the Irish Sea and their effect on satellite primary production algorithms, J. Plankton Res., 27, 1127–1148, https://doi.org/10.1093/plankt/fbi075, 2005.
Tilstone, G. H., Smyth, T., Poulton, A., and Hutson, R.: Measured and remotely sensed estimates of primary production in the Atlantic Ocean from 1998 to 2005, Deep-Sea Res. Pt. II, 56, 918–930, https://doi.org/10.1016/j.dsr2.2008.10.034, 2009.
Tilstone, G. H., Xie, Y., Robinson, C., Serret, P., Raitsos, D. E., Powell, T., Aranguren-Gassis, M., Garcia-Martin, E. E., and Kitidis, V.: Satellite estimates of net community production indicate predominance of net autotrophy in the Atlantic Ocean, Remote Sens. Environ., 164, 254–269, https://doi.org/10.1016/j.rse.2015.03.017, 2015.
Valerio, A. M., Kampel, M., Ward, N. D., Sawakuchi, H. O., Cunha, A. C., and Richey, J. E.: CO2 partial pressure and fluxes in the Amazon River plume using in situ and remote sensing data, Cont. Shelf Res., 215, 104348, https://doi.org/10.1016/j.csr.2021.104348, 2021.
Vantrepotte, V. and Mélin, F.: Inter-annual variations in the SeaWiFS global chlorophyll a concentration (1997–2007), Deep.-Res. Pt. I, 58, 429–441, https://doi.org/10.1016/j.dsr.2011.02.003, 2011.
Varela, R., Álvarez, I., Santos, F., DeCastro, M., and Gómez-Gesteira, M.: Has upwelling strengthened along worldwide coasts over 1982–2010?, Sci. Rep., 5, 1–15, https://doi.org/10.1038/srep10016, 2015.
Wanninkhof, R.: Relationship between wind speed and gas exchange over the ocean revisited, Limnol. Oceanogr. Methods, 12, 351–362, https://doi.org/10.4319/lom.2014.12.351, 2014.
Watson, A. J., Schuster, U., Shutler, J. D., Holding, T., Ashton, I. G. C., Landschützer, P., Woolf, D. K., and Goddijn-Murphy, L.: Revised estimates of ocean-atmosphere CO2 flux are consistent with ocean carbon inventory, Nat. Commun., 11, 1–6, https://doi.org/10.1038/s41467-020-18203-3, 2020.
Weiss, R. F.: Carbon dioxide in water and seawater: the solubility of a non-ideal gas, Mar. Chem., 2, 203–215, https://doi.org/10.1016/0304-4203(74)90015-2, 1974.
Wilks, D. S.: On “field significance” and the false discovery rate, J. Appl. Meteorol. Climatol., 45, 1181–1189, https://doi.org/10.1175/JAM2404.1, 2006.
Woolf, D. K., Land, P. E., Shutler, J. D., Goddijn-Murphy, L. M., and Donlon, C. J.: On the calculation of air-sea fluxes of CO2 in the presence of temperature and salinity gradients, J. Geophys. Res.-Oceans, 121, 1229–1248, https://doi.org/10.1002/2015JC011427, 2016.
Woolf, D. K., Shutler, J. D., Goddijn-Murphy, L., Watson, A. J., Chapron, B., Nightingale, P. D., Donlon, C. J., Piskozub, J., Yelland, M. J., Ashton, I., Holding, T., Schuster, U., Girard-Ardhuin, F., Grouazel, A., Piolle, J. F., Warren, M., Wrobel-Niedzwiecka, I., Land, P. E., Torres, R., Prytherch, J., Moat, B., Hanafin, J., Ardhuin, F., and Paul, F.: Key Uncertainties in the Recent Air-Sea Flux of CO2, Global Biogeochem. Cy., 33, 1548–1563, https://doi.org/10.1029/2018GB006041, 2019.
Woosley, R. J., Millero, F. J., and Wanninkhof, R.: Rapid anthropogenic changes in CO2 and pH in the Atlantic Ocean: 2003–2014, Global Biogeochem. Cy., 30, 70–90, https://doi.org/10.1002/2015GB005248, 2016.
Xiong, X., Masuda, Y., Hashioka, T., Ono, T., and Yamanaka, Y.: Effect of seasonal change in gas transfer coefficient on air–sea CO2 flux in the western North Pacific, J. Oceanogr., 71, 685–701, https://doi.org/10.1007/s10872-015-0313-5, 2015.
Xiu, P., Chai, F., Curchitser, E. N., and Castruccio, F. S.: Future changes in coastal upwelling ecosystems with global warming: The case of the California Current System, Sci. Rep., 8, 1–9, https://doi.org/10.1038/s41598-018-21247-7, 2018.
Zeng, J., Nojiri, Y., Landschützer, P., Telszewski, M., and Nakaoka, S.: A global surface ocean fCO2 climatology based on a feed-forward neural network, J. Atmos. Ocean. Technol., 31, 1838–1849, https://doi.org/10.1175/JTECH-D-13-00137.1, 2014.
- Abstract
- Introduction
- Data and methods
- Results
- Discussion
- Conclusions
- Appendix A: Driver analysis using in situ ΔpCO2
- Appendix B: SA-FNN pCO2 (sw) and trend uncertainties
- Data availability
- Author contributions
- Competing interests
- Disclaimer
- Acknowledgements
- Financial support
- Review statement
- References
- Abstract
- Introduction
- Data and methods
- Results
- Discussion
- Conclusions
- Appendix A: Driver analysis using in situ ΔpCO2
- Appendix B: SA-FNN pCO2 (sw) and trend uncertainties
- Data availability
- Author contributions
- Competing interests
- Disclaimer
- Acknowledgements
- Financial support
- Review statement
- References