the Creative Commons Attribution 4.0 License.
the Creative Commons Attribution 4.0 License.
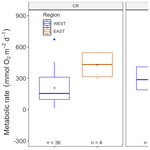
Mediterranean seagrasses as carbon sinks: methodological and regional differences
Anna Escolano-Moltó
Susana Flecha
Raquel Vaquer-Sunyer
Marlene Wesselmann
Núria Marbà
The increasing rates of CO2 due to anthropogenic activities are causing important potential climate threats for the Mediterranean Sea: ocean acidification and warming. In this region, two seagrass species, Posidonia oceanica and Cymodocea nodosa, can play a crucial role in climate change mitigation. Seagrasses can act as carbon sinks, buffer lowering pH values during the day and storing carbon in the sediment underneath their meadows. However, available data documenting these processes are scattered and collected using different methodologies, which makes its interpretation and generalization very challenging. In this study, we analyzed published and unpublished data (collected for this study) on seagrass community metabolism to compare two methodologies, benthic chambers and multiparametric sensors, and evaluate trends through time for these two species. Furthermore, we analyzed seasonal trends of both seagrass species' metabolic rates and their variation between the eastern and western Mediterranean basins. Most evaluated meadows, 80.9 %, were autotrophic. Calculated metabolic rates differ between methodologies, with multiparametric sensors estimating rates almost an order of magnitude higher, 143.22±28.21 (SE) mmol O2 m−2 d−1 for net community production (NCP) compared to an average of 18.75±3.80 (SE) mmol O2 m−2 d−1 for measurements with benthic chambers. However, sensors are not able to differentiate between habitats and only useful to assess seagrass metabolism at a broader community level, whereas benthic chambers are capable of evaluating rates at the species level and confirm that P. oceanica is more productive compared to C. nodosa. We found similar metabolic rates in the eastern and western Mediterranean regions for P. oceanica with the benthic-chamber technique and higher NCP in the west based on sensor measurements.
- Article
(1428 KB) - Full-text XML
-
Supplement
(939 KB) - BibTeX
- EndNote
A fifth of the global carbon sequestration in marine sediments (Duarte et al., 2005; Kennedy et al., 2010) can be attributed to seagrass meadows, despite the fact that they cover only a 0.1 % of the ocean surface. This “blue carbon”, which is defined as organic carbon buried in sediments underneath marine vegetation (Duarte et al., 2005; Kennedy et al., 2010; Mcleod et al., 2011; Greiner et al., 2013), is the result of the combination of intense metabolic activity of the vegetation, high trapping capacity of allochthonous matter and an effective carbon preservation in sediments underneath meadows (Cebrian, 1999). Due to the enhanced deposition rates caused by the physical presence of the canopies in the water column, seagrass meadows capture suspended organic matter, which accumulates as organic matter in the sediment (Romero et al., 1994; Pergent et al., 1997; Mateo et al., 2006; Hendriks et al., 2008; Kennedy et al., 2010). However, also the in situ plant growth, for which productivity can be a proxy, contributes to organic matter accumulation in the sediment (Greiner et al., 2013). Even though carbon dioxide (CO2) uptake through metabolic activity is on much shorter timescales compared to carbon storage, seagrass productivity and growth contribute to the sequestration and storage of a considerable amount of carbon in underlying sediments (Mcleod et al., 2011; Fourqurean et al., 2012).
There are species-specific differences in carbon burial rates and stocks; for Cymodocea nodosa, the annual carbon burial from productivity at a specific site has been estimated as 4.4 g C m−2 yr−1, whereas P. oceanica accumulated at 66.4 g C m−2 yr−1 at the same location (Cebrian et al., 1997). In general, seagrass communities tend to be autotrophic, although primary production varies depending on the evaluated temporal scales: daily, seasonally (Bay, 1984; Alcoverro et al., 1995; Gobert et al., 2006; Hendriks et al., 2014) and annually (Champenois et al., 2012, 2019). Furthermore, primary production and related carbon storage also depend on many other variables such as structural complexity (Trevathan-Tackett et al., 2015), size of the meadow (Ricart et al., 2017), local nutrient dynamics (Armitage and Fourqurean, 2016), hydrodynamics (Samper-Villarreal et al., 2016) and water depth (Serrano et al., 2014). A consistent methodology to estimate seagrass productivity is crucial to estimate its contribution to the global carbon sink capacity and to approximate the economic and ecological consequences of the decline of this ecosystem worldwide (Orth et al., 2006; Waycott et al., 2009).
Despite their importance, little is known about the effect of increasing rates of atmospheric CO2 on these ecosystems, and predictions for the open ocean may not reflect future conditions in coastal zones (Hendriks et al., 2010; Hofmann et al., 2011; Kelly and Hofmann, 2013; Lacoue-Labarthe et al., 2016), the main habitat for seagrasses. Increasing dissolved CO2 in the water column generates pH changes and contributes to the complex biogeochemical dynamics driving coastal ecosystems (Aufdenkampe et al., 2011), which are often also affected by human activities. Dynamics of the carbonate system in coastal regions are frequently influenced by benthic ecosystems that have the capacity to buffer physical and chemical conditions of the environment (Gutiérrez et al., 2011). By capturing CO2 and releasing oxygen (O2), seagrass ecosystems drive fluctuations in pH and dissolved oxygen concentrations in the adjacent water column that follow daily and seasonal patterns modulated by metabolic activity (Duarte et al., 2013; Hendriks et al., 2014). Primary production is hence an important component in the assessment of pH variation in coastal ecosystems. Through their photosynthetic activity, pH modification of the adjacent water mass by seagrasses attenuates ocean acidification, possibly providing a temporary refuge for calcifying organisms while oxygenating the water column through oxygen production (Hendriks et al., 2014).
Seagrass metabolism can be conditioned by abiotic parameters such as temperature and can be assessed through the changes in concentration of dissolved oxygen in water. Water temperature has an effect on the air–water exchange by modifying oxygen solubility and affects ecosystem metabolism (Brown et al., 2004; Vaquer-Sunyer and Duarte, 2013). In addition, anthropogenic pressures such as eutrophication (Paerl, 2006) or climate change are affecting oxygen cycling (Keeling and Garcia, 2002; Conley et al., 2009; Keeling et al., 2010), and dissolved oxygen is one of the environmental parameters that has changed more drastically in a short period of time (Díaz and Rosenberg, 1995; Diaz, 2001), with potential catastrophic consequences for marine life (Vaquer-Sunyer and Duarte, 2008). In coastal ecosystems, increased nutrient inputs contribute to higher organic production and oxygen demand with a consequently greater likelihood of hypoxia (Karim et al., 2003; Zhang et al., 2010). Measuring dissolved oxygen concentrations in seagrass meadows allows for inferring metabolic parameters such as gross primary production (GPP) for the meadow community. Metabolic community status is defined by the net community production (NCP), the difference between GPP and community respiration (CR). When a community exports organic carbon to adjacent systems, it is considered net autotrophic (NCP > 0); on the other hand, if the community gets external organic carbon inputs to sustain its own metabolism, it is considered net heterotrophic (NCP < 0) (Champenois and Borges, 2012).
The dominant seagrass species in the Mediterranean Sea are Cymodocea nodosa and Posidonia oceanica, inhabiting a region that has been defined as a “hotspot for climate change” (Giorgi, 2006) with warming rates of 2- to 4-fold higher than in other regions (Vargas-Yáñez et al., 2008; Burrows et al., 2011). An increase in the seasonal average temperature of 2.2 ∘C in winter and 3.4 ∘C in summer is projected for the end of this century for the western basin of the Mediterranean Sea in a scenario with moderated greenhouse gas emissions (Jordà et al., 2012). Furthermore, extreme thermal events are expected to be more intense and frequent in the Mediterranean region (Ali et al., 2022), while the basin is not subject to the same rates of warming, with indications that the eastern Mediterranean is warming faster than the western Mediterranean (Amitai et al., 2020; Nykjaer, 2009). Climate warming can impact P. oceanica meadows negatively, as higher temperatures stress the species physiologically (Marbà and Duarte, 2010), with shoot mortality increasing during heat waves exceeding 28 ∘C at the end of summer (Diaz-Almela et al., 2007). The total surface area occupied by P. oceanica meadows is estimated to range between 1 % and 2 % of the total surface area of the Mediterranean Sea (Béthoux and Copin-Montégut, 1986; Pasqualini et al., 1998), although this number is uncertain (Bonacorsi et al., 2013). The distribution has been estimated as 510.710 ha in the western and 713.992 ha in the eastern Mediterranean basin (Telesca et al., 2015), which might be conservative due to a lack of data, with many more data available in the western basin compared with the eastern part where the absence of data is common. Contrary to other European seagrasses with decreasing loss rates (de los Santos et al., 2019), the extent of P. oceanica meadows is decreasing considerably (Boudouresque et al., 2009; Telesca et al., 2015) with ranges between 13 % and 50 % areal extent lost since the 1960s (Marbà et al., 2014). C. nodosa meadows have a higher thermal tolerance and are supposed to cope better with increasing temperatures (Egea et al., 2018), even though high temperatures during heat waves over the coming decades might increase the annual mean temperature by 4 ∘C and will probably exceed the limit beyond which C. nodosa losses can be expected in the Mediterranean Sea (Olsen et al., 2012; Chefaoui et al., 2018). Also, all Mediterranean waterbodies are affected by anthropogenic CO2 emissions; however they are more so in the western basin, demonstrated by decreasing values of pH ranging from −0.14 to −0.005 pH unit drops since the beginning of the industrial era to 2001, higher than elsewhere in the open ocean (Touratier and Goyet, 2011). Therefore, ocean acidification is a climate change indicator that has been characterized as one of the most important for the Mediterranean Sea, together with temperature and UV radiation (Micheli et al., 2013). In addition to climate change, the Mediterranean Sea is likely to be more impacted by human disturbances like overfishing, increasing pollution from wastewater outfalls, riverine and agricultural runoff, fish farming, and the introduction of alien species (Lejeusne et al., 2010) from other seas (Giorgi and Lionello, 2008; Richon et al., 2019). With these multiple pressures increasing, it is crucial to evaluate the functions and services provided by key coastal ecosystems such as seagrasses.
Seagrass metabolism has classically been measured using closed benthic chambers (Duarte et al., 2010). However, the spatial heterogeneity of these ecosystems (Gazeau et al., 2005) and their high temporal variability cannot be easily estimated with this approach (Karl et al., 2003). For this purpose, using sensors can be more suitable, as dissolved oxygen concentrations can be evaluated for longer periods of time. Additionally, the aquatic eddy covariance technique can give even more precise values for productivity, although these are spatially limited; nevertheless so far there has only been one study including P. oceanica in the Mediterranean Sea (Koopmans et al., 2020). GPP values obtained with the use of benthic chambers could provide an underestimate as a result of photorespiration, while the use of multiparametric sensors measuring oxygen in the canopy probably provides more realistic GPP values (Champenois and Borges, 2012). The use of multiparametric sensors to measure oxygen also provides the opportunity to measure metabolic rates without damaging roots or rhizomes. In river and lake ecosystems, the measurement of metabolism by oxygen sensors and loggers is a commonly used method (Cole et al., 2000; Coloso et al., 2008), while it is not as widespread in coastal waters (Odum and Hoskin, 1958; Odum and Wilson, 1962; Ziegler and Benner, 1998; Vaquer-Sunyer et al., 2012) due to the higher lateral transport rates of water in these systems.
The aim of this study is to evaluate the potential carbon capture of the dominant Mediterranean Sea seagrass (Posidonia oceanica and Cymodocea nodosa) communities through their metabolic activity, comparing two methodologies (benthic chambers and multiparametric sensors), and evaluate the spatial and temporal differences between Mediterranean regions. We do so by conducting field measurements amended by published data compiled from the literature.
2.1 Data compilation
Data for the metabolic parameters were extracted from the literature, through
a literature search on Scopus and the Web of Science using the keywords
“Posidonia”, OR “Cymodocea”, OR “Seagrass”, AND “Productivity”, OR “Metabolism”
, and the results were manually screened for data on metabolism in the
Mediterranean basin. This database was extended with submitted data and data
from dedicated sampling campaigns in 2016 in Mallorca (western
Mediterranean) and 2017 in the eastern basin (Crete and Cyprus; see Table 1,
Fig. 1). We compiled data from multiparametric sensors, collected during
different periods ranging from 2011 to 2019 (for details see Table 1), and
data using the benthic chambers methodology, which had a higher number of
literature studies, with a total of 12 publications for P. oceanica and/or C. nodosa meadows
(for details see Table 2), and a wider temporal cover with studies carried
out from 1982 to 2019. Importantly, this study adds new data on
Mediterranean seagrasses metabolism in the eastern Mediterranean basin
(Crete, Cyprus; Table 1), where few data have been published before.
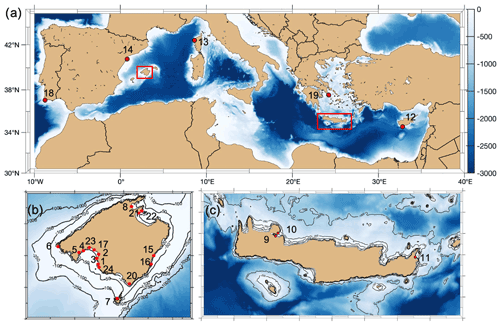
Figure 1Location of sampling sites with the numbered ID as described in Tables 1 and 2 included in this compilation (map generated with the M_Map package of MATLAB, 2012).
Table 1Characteristics of sampling stations with data for multiparametric sensors. Temperature and salinity represent average values during the deployment.
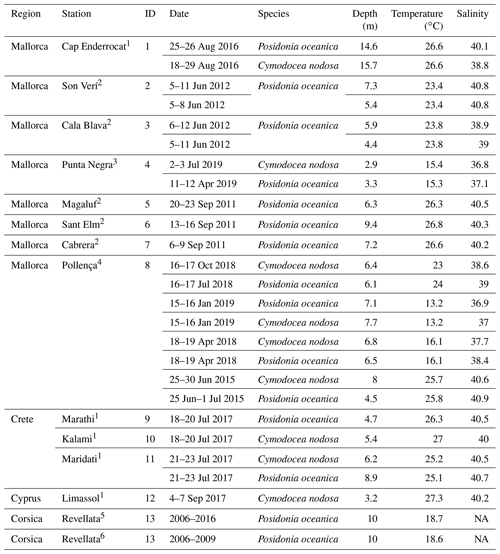
Source: (1) Vaquer-Sunyer et al. (unpublished data), (2) Hendriks et al. (2014), (3) Marx et al. (2021), (4) Hendriks et al. (unpublished data), (5) Champenois and Borges (2019), (6) Champenois and Borges (2012). NA – not available.
Table 2Characteristics of sampling stations with data for benthic-chamber deployments. Temperature and salinity represent average values during the deployment.
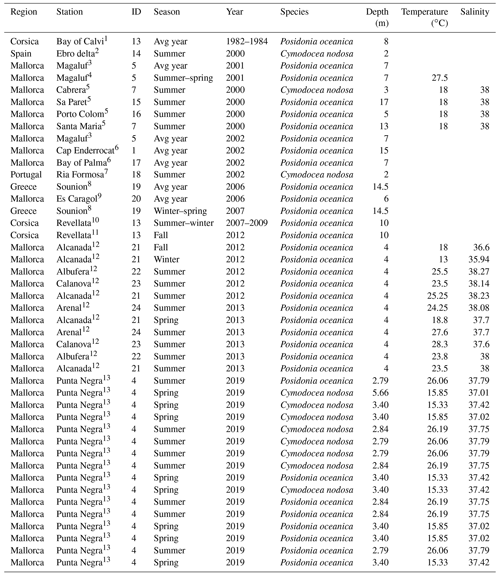
Source: (1) Frankignoulle and Bouquegneau (1987), (2) Barrón et al. (2004), (3) Barrón and Duarte (2009), (4) Barrón et al. (2006), (5) Holmer et al. (2004), (6) Gazeau et al. (2005), (7) Santos et al. (2004), (8) Apostolaki et al. (2010), (9) Gacia et al. (2012), (10) Champenois and Borges (2012), (11) Olivé et al. (2016), (12) Agawin et al. (2017), (13) Marx et al. (2021).
2.2 Site description
Data from multiparametric sensors in either Posidonia oceanica and/or Cymodocea nodosa meadows came from one site in France (Corsica, literature), eight in Spain (Mallorca, of which one was collected for this study), three in Crete (Greece) and one in Cyprus (Republic of Cyprus), with the last four all collected for this study (see Table 1). All were shallow sites, ranging from 2.9 m depth (Punta Negra, Mallorca) to 15.7 m depth (Cap Enderrocat, Mallorca). Extracted data from Corsica came from Cape Revellata, a protected area. The site in Limassol (Cyprus) is adjacent to the second-largest city in Cyprus, and it is considered an impacted area affected by high anthropogenic pressures related to intense tourism and the construction of extensive coastal infrastructures. Marathi and Kalami are located close to each other next (<10 km) to the port of Souda in western Crete; both sampling sites have similar environmental conditions and are impacted by notable sewage discharge, agriculture and industrial/chemical pollution (Simboura et al., 2016). Maridati, in eastern Crete, is a pristine bay but affected by intermittent discharges of an ephemeral stream (Wesselmann et al., 2021). In Mallorca, multiparametric sensors were deployed in sites with a range of environmental impacts, encompassing sites with protection like Cap Enderrocat, which forms part of an SPA (Special Protection Area) under the Birds Directive and is a SIC (Site of Community Importance, Natura 2000), and Son Verí and Cala Blava, which are also protected and host 11.5 % of the total P. oceanica meadows within the marine protected area of Cap Enderrocat–Cap Blanc. Even though the locations are protected, the Bay of Palma is influenced by various outlets of wastewater treatment plants. On the opposite site in the Bay of Palma, Punta Negra is considered a Natural Area of Special Interest (SPA and a natural space protected by law by the government of the Balearic Islands). Magaluf (Mallorca, Bay of Palma) is in front of a touristic beach, but the location of the sensors was sheltered behind an island (Isla de sa Porrassa). Sant Elm (Mallorca) is located in a relatively pristine area but near a sewage plant effluent. Pollença (Mallorca) is in an enclosed bay affected by considerable organic input from the Albufera wetlands, an effluent of the sewage plant, nearby harbor and urban area. The least impacted site would be Santa Maria, a bay located on the coast of the island of Cabrera and the most pristine sampling area. The island of Cabrera is part of the Cabrera Archipelago Maritime–Terrestrial National Park and recognized internationally as a special protection zone with importance for the Mediterranean and SAC (Special Area of Conservation). The sampling sites where sensors were deployed in the Mediterranean therefore include sites with different degrees of human impact and protected areas with very low anthropogenic impact.
2.3 Data analysis
We compare metabolic data obtained by both methods, benthic chambers and multiparametric sensors. For benthic chambers, reported metabolic data as well as accompanying biotic parameters were extracted from the literature. In these articles net community production (NCP) and respiration (R) was generally estimated from changes in dissolved oxygen using the Winkler titration spectrophotometric method (Labasque et al., 2004). Benthic chambers enclose a section of the seagrass meadow, and flexible fitted plastic bags, not permeable for gases, assure the possibility of movement of the shoots inside; see details in the method section of each paper for the exact construction used. The benthic-chamber methodology has been more generally used to assess metabolism of seagrass meadows, and the database of this study contains a total of 100 NCP estimations. For multiparametric sensor data, data available as oxygen concentration over time were processed and analyzed to obtain the metabolic parameters (see Sect. 2.3.1); when this was not available, we used the reported metabolic rates. We compare the data obtained by both methodologies, with calculated metabolism from raw oxygen profiles obtained with the multiparametric sensors where possible, and only used directly reported productivity values for the sensor data obtained from Champenois and Borges (2012, 2019).
2.3.1 Calculations of metabolic rate from multiparametric sensors
Where available, we used time series of dissolved oxygen (DO, in mg L−1), salinity and temperature (∘C) measured in P. oceanica and/or C. nodosa meadows with multiparametric sensors (OTT Hydrolab DS5X and HL4), with an accuracy for dissolved oxygen evaluated as ±0.2 mg L−1. The duration of the data collection was different depending on the site, from 1 full day to 4 consecutive days (see Table 1), while 24 h periods were used for calculations.
Sensors were deployed 0.2 m above the seafloor in seagrass meadows. Data were recorded every 15 min except in Cap Enderrocat where readings were taken every 10 min (Table 1). Biological metadata detailing habitat traits were obtained following the methodology described by Hendriks et al. (2014).
Oxygen sensors (Hach LDOTM) were calibrated using the water saturated air method calibration. For validation of salinity, specific conductance calibrations were performed with 50 000 µS cm−1 buffers. For depth measurements, pressure readings were corrected for specific conductance.
Meteorological data for the deployment period were obtained from the Agencia Estatal de Meteorología (AEMET) for the stations in Mallorca, from the Cyprus Department of Meteorology for Cyprus sampling sites and from the Hellenic National Meteorological Service for the locations in Crete (see Table S1 in the Supplement).
With input parameters of dissolved oxygen (DO), temperature (∘C) and salinity we calculated the metabolic rates of the seagrass habitats using a modification of the model of Coloso et al. (2008) implemented in MATLAB (version 7.5, MathWorks Inc.) explained in detail in Vaquer-Sunyer et al. (2012). For each station, we manually introduced the mixed-layer depth (MLD); the latitude; and year, day and time as the day fraction. For wind speed (m s−1) we used the gas transfer velocity (k660) calculations based on Kihm and Körtzinger (2010). Net community production (NCP) was calculated as gross primary production (GPP) − community respiration (CR), taking into account diffusive exchange with the atmosphere (D), following Eq. (1):
The diffusion with the atmosphere is regulated by the difference in DO concentration linked to atmospheric equilibrium (DOsat) and the air–sea gas velocity transfer for oxygen (k) at a given temperature according to Eq. (2):
where D can be positive (DO addition to the system) or negative (DO removal from the system). Wind speed was estimated at each station for 15 min intervals (10 min for the Cap Enderrocat station) to predict k660 (air–sea gas transfer velocity for oxygen at 20 ∘C and a salinity of 35) based on Kihm and Körtzinger (2010) and Cole and Caraco (1998). Schmidt number equations for seawater according to Wanninkhof (1992) were used for the k calculation from k660. As the cubic model equals the model proposed by Wanninkhof and McGillis (1999) for short-term winds, this parameterization by Kihm and Körtzinger (2010) is used.
The model assumes that the only metabolic activity during the night is respiration (community respiration; CR), as in the absence of sunlight there is no photosynthetic production. CR can be extracted from the change in oxygen concentration during the night (net community production at night = CR), from 1 h past sunset to 1 h before sunrise. During the daylight period net community production (NCP) is considered to be the result of the balance between gross primary production (GPP) and CR. NCP was calculated using the rate of change in DO within the interval of 24 h, while CR is calculated from night values and converted to hourly rates. As is common, we assumed that CR rates during the light period equal those at night and use the equivalent hourly values of CR for the light period to estimate GPP, adding the oxygen consumption (CR) for this period to NCP to get GPP. GPP and CR could be underestimated, since it is likely that CR during daytime exceeds CR at night (Grande et al., 1989; Pace and Prairie, 2005; Pringault et al., 2007), but this underestimation would not affect NCP values (Cole et al., 2000). Individual estimates of CR, NCP and GPP within the measured intervals obtained from the multiparametric sensors were calculated over a 24 h period for each day and station. As we did not dispose of vertical profiles of conductivity, temperature and depth (CTD) for each station to calculate the mixed-layer depth, we used the model of Condie and Webster (2001) to calculate the MLD following Eq. (3):
where S represents the non-dimensional parameter of the ratio of the input of kinetic energy by the wind to the input of potential energy by solar radiation. ρ is the density, calculated from the salinity, temperature and pressure collected by the in situ multiparametric sensor, following the formula of Fofonoff and Millard (1983). Cp represents the specific heat, considered here to be 3850 J kg−1 ∘C−1 as the relative value for seawater. U2 refers to the diurnally averaged wind speed specified here to be measured 2 m above the waterbody (Simpson and Hunter, 1974; Holloway, 1980). In our case, the wind data were measured at 10 m above the upstream edge of the waterbody and were converted, according to the wind profile power law (Eq. 4):
where ϑ is the wind speed (in m s−1) at a determined height z (in m) and ϑr is the speed that is known at a reference height (zr). The exponential α is a coefficient derived empirically which varies upon the stability of the atmosphere. In our case, neutral stability is assumed, and within those conditions α is approximately 0.143 g (Eq. 3), corresponding to the gravitational acceleration (9.8 m s−1). α (Eq. 3) represents the thermal expansion coefficient which was calculated as a function of the absolute salinity, in situ temperature and pressure. This function is included in the Gibbs SeaWater (GSW) Oceanographic Toolbox (McDougall and Barker, 2011) and evaluates the thermal expansion coefficient αt with respect to the in situ temperature (t) from Eq. (2.18.1) of the TEOS-10 manual (Thermodynamic Equation of Seawater – 2010; IOC, 2015), following Eq. (5):
This function uses the full TEOS-10 Gibbs function g () of IOC (2015) as the sum of the IAPWS (2008, 2009) Gibbs functions. Finally, H (Eq. 3) equals the average water depth (in m), and Q (Eq. 3) is the diurnally averaged shortwave radiative heat flux (W m−2). Then, the surface mixed layer (zs) was approximated following Eq. (6) by Condie and Webster (2001):
To be as accurate as possible, we tried different intervals for the wind and radiation data. Taking in account that stratification starts to develop between 09:00 and 10:00 GMT+1 and reaches its maximum around 16:00 (Condie and Webster, 2001), we decided to take the interval from 09:00 to 16:00 into account for the wind data. This interval resulted to be the more accurate (highest R2 obtained for that interval, R2=0.9) for the wind data, based on the linear regressions between obtained with the different wind and radiation intervals.
2.3.2 Statistical analysis
We used mixed linear models with the package “lme4” in the R environment (R Core Team, 2021) to evaluate differences between methods, regions and species. To reflect the variability between study approaches and sampling procedures and therefore variability in the precision of outcome of each study, we used a linear model where the publication was included as a random effect unless specified differently. To obtain the statistical significance for the fixed factors, we ran an ANOVA on the mixed model. We also analyzed abiotic (wind speed, temperature and depth) parameters related to sensor data, as there were more additional data associated with these measurements. In this linear model we also included the publication as a random effect. As the data were not normally distributed according to the Shapiro–Wilk test, we log-transformed data for GPP and CR before analysis. NCP could not be log-transformed due to negative values. Finally, we evaluated if trends in metabolic rates over time were apparent with simple lineal regressions.
We compiled 133 CR, 141 GPP and 168 NCP estimates, most of them (86.4 %) were restricted to seagrass meadows located in the western Mediterranean (Table S2). The studied meadows were situated at water depths between 0.5 and 22 m (Tables 1, 2), where water salinity ranged from 35.94 to 40.98 psu (Tables 1, 2). The majority (50.0 %) of the metabolic rates were assessed in summer, with 19.9 % of data being sampled in spring, 10.6 % in fall and 7.2 % in winter and with seawater temperatures during the measurements varying between 13 and 28.5 ∘C (average 23.2 ∘C ± 4.4 SD) between locations (Tables 1, 2). Estimates of seagrass metabolic rates in Mediterranean seagrass meadows span from 1982 to 2019 (Tables 1, 2), but most measurements were conducted after the year 2007 for benthic chambers and even later, after 2015, for sensors. Benthic-chamber deployments were concentrated in the western basin (72 metabolic measurements) with only six measurements in the eastern basin. While 55 metabolic measurements were made in the western basin with multiparametric sensors, 14 were made in the eastern basin.
The amount of CR estimates assessed with multiparametric sensors (66) and benthic chambers (67) was similar, whereas GPP was more often estimated using multiparametric sensors (74) compared to benthic chambers (67). For net community productivity (NCP), benthic chambers (99) were preferred over sensors (69 measurements). However, we found negative respiration rates (oxygen production) at night for many sensor deployments, an indication of the influence of lateral advection and passing of different water masses. Therefore, we trimmed the dataset to contain only measurements where this influence was not detected (see number of measurements in each figure). Benthic chambers and multiparametric sensors yielded very different CR values with 41.2 ± 4.55 (SE) mmol O2 m−2 d−1 for benthic chambers and 229.9 ± 25.57 mmol O2 m−2 d−1 for sensors (, p<0.0001) in a mixed model, with only a fixed-factor methodology and as a random-effect study. This difference with almost an order of magnitude is found for NCP as well with 18.8 ± 3.80 and 143.2 ± 28.21 mmol O2 m−2 d−1 for benthic chambers and sensors respectively (, p<0.01) as well as for GPP (55.3 ± 6.39 and 329.2 ± 29.91 mmol O2 m−2 d−1 for chambers and sensors; , p<0.0001) (Fig. 2). Therefore we decided to analyze the metabolic rates estimated using benthic chambers and multiparametric sensors separately. For a statistical summary table, see Table S3.
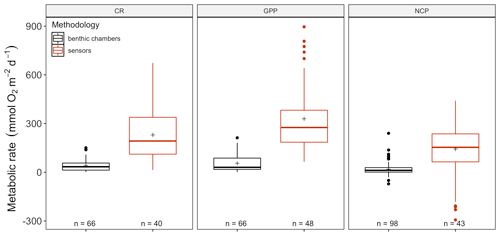
Figure 2Comparison between benthic-chamber (black) and sensor data (red) for CR, GPP and NCP (mmol O2 m−2 d−1) for the full dataset. Upper and lower hinges correspond to the upper and lower quartiles; the line inside the boxes corresponds to the median; the mean is marked by a crosshair; and the error bars are based on minimum and maximum standard deviation for each parameter. We found differences (p>0.01) in metabolic rates between methods for CR, GPP and NCP.
3.1 Multiparametric sensors
Sensor data were collected in the water column, with lateral movement between habitats of water masses, and there were no significant differences in GPP (, p=0.94), CR (, p=0.37) and NCP (, p=0.93) between the two species (P. oceanica and C. nodosa) tested in a mixed model with “site” as a random factor, including depth, region and seasons (as metabolic rate ∼ species + region + depth + season + (1|site)). Therefore, we did not divide the sensor data for the two species. In a reduced model (metabolic rate ∼ region + depth + season + (1|site)), GPP (, p=0.48) and CR (, p=0.23) were similar between the eastern and western Mediterranean basins (Fig. 3, Table 3), but NCP was higher the western basin (, p=0.02). No significant influence of depth was identified for any of the metabolic parameters, nor season for CR or GPP; however NCP was lower in spring (, p<0.01). In spring, we also found the highest GPP rates (mean ± SE) with 382.68 ± 41.28 mmol O2 m−2 d−1; however, the corresponding CR rates for spring were 444.88 ± 76.32 mmol O2 m−2 d−1, leading to a negative NCP of −62.19 ± 47.08 mmol O2 m−2 d−1. For the rest of the seasons productivity was higher than respiration, reflected in positive averaged NCP rates and confirming that seagrass meadows normally tend to be autotrophic ecosystems with a mean production respiration (P R) ratio above 1 (2.1 ± 0.26), confirming the tendency of net autotrophy. In the eastern Mediterranean basin, only data recorded with sensors in summer were available to compare to measurements in the western basin. Average GPP in the western basin in summer was 408.11 ± 52.01 (SE) mmol O2 m−2 d−1, and while the average yearly GPP did not differ between basins, the productivity in summer is significantly different from the eastern basin, with a lower average of 192.90 ± 61.66 mmol O2 m−2 d−1. Average NCP in the eastern basin (summer) was negative with −236.12 ± 19.83 mmol O2 m−2 d−1, based on only four measurements, and in the western basin positive with 182.12 ± 23.20 mmol O2 m−2 d−1 averaged over the year and 228.98 ± 25.05 mmol O2 m−2 d−1 in summer. The negative NCP rates in the eastern basin are the result of the high CR measured there, with 429.01 ± 69.27 mmol O2 m−2 d−1, more than twice the CR measured in summer for the western basin of 187.77 ± 26.82 mmol O2 m−2 d−1. This led to low ratios, with on average 0.41 ± 0.08 in the eastern basin in summer. In the western basin in summer, the seagrass communities tended to be net autotrophic, reflected in an average ratio of 2.31±0.27. In general, for both basins, the threshold GPP where the ecosystem switched between net heterotrophy and net autotrophy (NCP = 0) was 254.03 mmol O2 m−2 d−1.
Table 3Summary of metabolic rates (mmol O2 m−2 d−1), for the different methodologies, per region; season; and when, possible, species. Group averages are in bold, and italics denote standard error (SE) of averages.
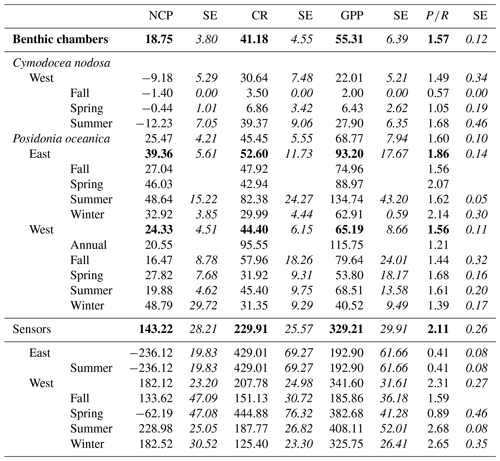
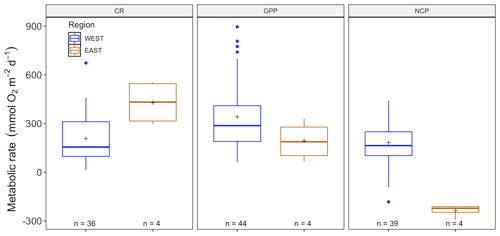
Figure 3Averaged GPP, NCP and CR (mmol O2 m−2 d−1) values for eastern (blue) and western (orange) Mediterranean basins calculated from measurements of multiparametric sensors. Upper and lower hinges correspond to the upper and lower quartiles; the lines inside the boxes correspond to the median; the mean is marked by a crosshair; and the error bars are based on minimum and maximum standard deviation for each parameter. We found differences with p<0.05 for NCP between the regions.
The temperature recorded during the highest GPP measurement in P. oceanica in the western basin was 26.6 ∘C, which is close, even though a bit higher, to the optimal value reported for P. oceanica of 25.8 ∘C (Savva et al., 2018). For the eastern Mediterranean basin, the highest GPP obtained was 329.94 mmol O2 m−2 d−1 at Maridati (Crete) during July, and the in situ temperature registered at that moment was 25.0 ∘C, which was not the highest temperature registered in the eastern basin (28.5 ∘C) and lower than the mean temperature in the eastern basin during the summer sampling campaign (25.9 ± 0.8 ∘C). The lowest GPP values found in the western and eastern regions were similar; we found the lowest value for GPP of 64.72 mmol O2 m−2 d−1 for the western basin in the Bay of Pollença (Mallorca) during fall, whereas the lowest GPP value in the eastern basin was 66.19 mmol O2 m−2 d−1 in Maridati (Crete) in summer. Temperatures during both measurements were different with 2.5 ∘C of difference between them, 25.4 ∘C at Maridati station (Crete) and 22.9 ∘C in Pollença (Mallorca).
We tested with individual regression models for the effect of temperature (metabolic rate ∼ temperature + (1|site)), which did not significantly affect GPP (, p=0.23); however NCP increased (, p=0.02) with increasing temperatures, while, surprisingly, CR decreased (, p=0.03) (see Supplement, Fig. S1). Wind speed (metabolic rate ∼ wind speed + (|site)) did not drive metabolic rates with (p=0.51), (p=0.41) and (p=0.32) respectively for GPP, NCP and CR. Depth was not a driver of NCP or CR with (p=0.64) and (p=0.92) respectively but did influence GPP with (p<0.01), while this positive effect is mainly driven by the high productivity in Cap Enderrocat around 15 m depth. Over the 12 years of data available for sensor measurements (2007–2019), we found an increase in CR (, p<0.05) but significant changes in neither GPP (, p=0.23) nor NCP (, p=0.57; Fig. S3).
3.2 Benthic chambers
We found significant differences for CR (13.88, p<0.001) and GPP (, p<0.001) but not NCP (, p=0.18; Fig. 4) between P. oceanica and C. nodosa productivity, in a model including depth and season, as well as the publication as a random factor. At a seasonal scale, there were no significant differences for NCP or CR for C. nodosa with neither NCP (, p=0.89), CR (, p=0.28) nor GPP (, p=0.08). GPP was lower than CR during all seasons for which measurements were available, reflected in the averaged NCP, with a negative rate (−9.2 ± 5.29 (SE) mmol O2 m−2 d−1), revealing that the C. nodosa community tends to be net heterotrophic. The threshold GPP to switch from a heterotrophic to an autotrophic system (NCP = 0) was 23.12 O2 m−2 d−1 for C. nodosa.
There were no significant differences between NCP (, p=0.41), CR (, p=0.08) and CR (, p=0.28) across seasons (with year as a random factor) for P. oceanica, but there was for GPP (, p<0.01; Fig. S2). As we did not have C. nodosa data for the eastern Mediterranean basin, we only examined P. oceanica to distill patterns between eastern and western Mediterranean regions. There were no significant differences for NCP (, p=0.76), GPP (, p=0.54) or CR (, p=0.50) in Posidonia incubations between eastern and western regions (Fig. 5), due to the high variability between sites, which was incorporated in the model as a random factor. For the western basin, averaged NCP was 24.33 ± 4.51 (SE) mmol O2 m−2 d−1, with the average GPP (65.19 ± 8.66 mmol O2 m−2 d−1) higher than the CR rate (44.4 ± 6.15 mmol O2 m−2 d−1), reflecting the tendency of P. oceanica communities to be net autotrophic. This was also the case for the eastern basin, with average NCP even higher at 39.36 ± 5.61 (SE) mmol O2 m−2 d−1 and average GPP (93.20 ± 17.67 mmol O2 m−2 d−1) higher than the CR rate (52.60 ± 11.73 mmol O2 m−2 d−1). The threshold GPP to switch from a heterotrophic to an autotrophic system (NCP = 0) was 36.64 O2 m−2 d−1 for P. oceanica, with both basins combined. Contrary to the sensor data, temperature was not correlated with any metabolic rate, with NCP (, p=0.84), GPP (, p=0.12) and CR (, p=0.21; Fig. S1). For chamber incubations we found a tendency over time, albeit not significant (see Fig. S3), of decreasing GPP (, p=0.06) and CR (, p=0.05) but not NCP (, p=0.70).
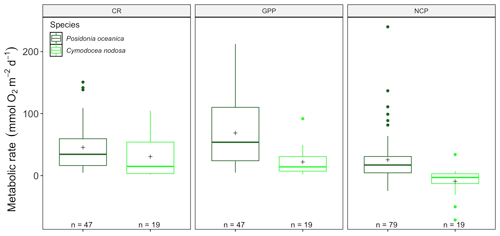
Figure 4Metabolic rates for GPP, CR and NCP (mmol O2 m−2 d−1) for Cymodocea nodosa (light green) and Posidonia oceanica (dark green) for the benthic chambers dataset in the western Mediterranean basin. Upper and lower hinges correspond to the upper and lower quartiles; the line inside the boxes corresponds to the median; the mean is marked by a crosshair; and the error bars are based on minimum and maximum standard deviation for each parameter (rates were different between species with p<0.001 for GPP and p<0.01 for CR).
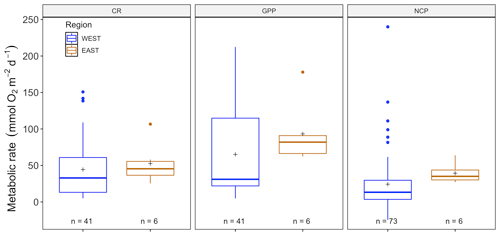
Figure 5Metabolic rates for GPP, NCP and CR (mmol O2 m−2 d−1) for the eastern (blue) and western (orange) Mediterranean as obtained from the benthic-chamber dataset for Posidonia oceanica. Upper and lower hinges correspond to the upper and lower quartiles. The line inside the boxes corresponds to the median; the mean is marked by a crosshair; and the error bars are based on minimum and maximum standard deviation for each parameter. No differences were found for metabolic rates between regions.
By comparing compiled data we found significant differences in metabolic rates between Cymodocea nodosa and Posidonia oceanica in benthic chambers, while no species-specific pattern could be distilled with sensor data. Only significant differences in NCP estimated with sensor data were found between the eastern and western Mediterranean basins, which might be due to the persistence of a much lower number of observations in the eastern basin, with a subsequent loss of statistical power to detect differences. The bias towards a higher number of observations in summer compared to other seasons could have prevented the detection of seasonal patterns, with only lower NCP in spring detected with sensors and a higher GPP in summer with detected benthic chambers and similar metabolic rates for the rest of the seasons. Over half of NCP measurements were done in summer, with 55.1 % for benthic-chamber data and 69.8 % for sensor data. Over three-quarters (80.4 %) of the data for P. oceanica and C. nodosa communities show that these seagrass meadows are net autotrophic in almost all seasons and locations and are capable of acting as carbon sinks and modifying pH diurnally on an annual timescale. When this productivity is buried as biomass, carbon could be fixed over long timescales, highlighting the role of these seagrass meadows in climate change mitigation. The threshold GPP where the ecosystem switched between net heterotrophy and net autotrophy (NCP = 0) was 254.03 mmol O2 m−2 d−1 for measurements from sensors, which is higher than the estimate of 186 mmol O2 m−2 d−1 for different seagrass species and regions pooled by Duarte et al. (2010). For estimates from benthic chambers, this threshold was much lower, 36.64 O2 m−2 d−1 for P. oceanica and 23.12 O2 m−2 d−1 for C. nodosa.
4.1 Multiparametric sensors
Due to the effect of lateral advection and mixing of water masses masking a species-specific signal, it was not possible to demonstrate differences in the metabolic rates between Cymodocea nodosa and Posidonia oceanica meadows, since the sensors are measuring a composed signal in the water column. Due to logistic constraints, sensor deployment in C. nodosa and P. oceanica meadows when both seagrass species were present in a same site was separated by a distance of less than 10 m, which adds to the lack of differentiation of values found between the two studied species. Measurements with multiparametric sensors should therefore be interpreted as measurement at an ecosystem level, as the influence of oxygen dynamics of macrophytes near the measuring site cannot be separated. The influence of phytoplankton and other primary producers may affect sensors as well as benthic-chamber measurements. During a spring bloom a relationship between Chl a in the water column and GPP has been shown for the Bay of Palma (Gazeau et al., 2005). However, a correlation with planktonic Chl a can not always be demonstrated; for instance, during a study of annual patterns in the Bay of Revellata (Italy), the highest GPP values recorded in a P. oceanica meadow where found when planktonic Chl a was particularly low and the highest values of Chl a did not reflect an increase in GPP and NCP values (Champenois and Borges, 2012). Sensor measurements in meadows of both seagrass species allowed us to estimate the metabolic activity of the whole ecosystem and compare between regions, showing similar rates for CR and GPP between the eastern and western Mediterranean basins, while NCP was higher in the western basin, with negative values for the eastern basin (Table 1). This difference is caused by the relatively high CR rates in the eastern basin of on average 429.0 ± 69.27 (SE) mmol O2 m−2 d−1, 2 times higher than the averaged CR rate in the western basin of 207.78 ± 24.98 (SE) mmol O2 m−2 d−1. No significant differences were found for GPP, which has relatively low values in the eastern basin of 192.90 ± 61.66 (SE) mmol O2 m−2 d−1 compared to a yearly average of 341.60 ± 31.61 (SE) mmol O2 m−2 d−1 for the western Mediterranean due to the low sample size (n=4), limited measurements over different seasons and high variability in measurements in the east. When we use GPP of the western basin in summer only, there is a significant difference in GPP between the basins. More measurements are necessary in the eastern basin, during all seasons, in order to obtain a more robust comparison.
The highest GPP was recorded in Cap Enderrocat (Mallorca, Spain) during summer in a C. nodosa meadow with a production of 895.78 mmol O2 m−2 d−1. Even if these values are high, this is lower than the 1338.0 mmol O2 m−2 d−1 measured at the Bay of Revellata (Corsica) by Champenois and Borges (2012). These authors suggest that extreme GPP values in P. oceanica meadows may be rare events that are hardly captured by the classic benthic chambers methodology, and the presence of high values measured with multiparametric sensors in this database might reflect that these events could be less uncommon than previously thought. We find the highest GPP values during the summer months and the highest CR rates in spring (Fig. S2), with a positive relationship with increasing temperatures up to an optimum temperature (Fig. S1), confirming that increasing temperatures enhance metabolic rates (Brown et al., 2004) until a threshold is reached. Abiotic and biotic factors that drive seagrass community metabolism differ between regions. In the eastern basin, GPP was affected by temperature (p<0.05, Fig. S1). No effect of water depth was found on NCP or CR, while depth determines light availability, which in turn determines seagrass distribution, biomass and productivity (Dennison, 1987). This lack of an effect is probably due to the limited depth range of the sites in the database (max 22 m, min 0.6 m depth), with measurements mainly in shallow sites, with an average depth of 7.69 ± 0.36 (SE) m. The counterintuitive relationship between GPP and increasing depth is driven by one study only, at almost twice the average depth (approx. 15 m), encountering very high productivity in a C. nodosa meadow in Enderrocat. There was a relation between temperature and NCP and CR for the sensor data, with increasing net production with rising temperatures but surprisingly lower community respiration rates. There are no data available for intermediate temperatures, leaving two clusters, one between 13 and 16 ∘C and one between 23 and 27 ∘C. The bulk of the data were collected between 23 and 27 ∘C, therefore dominating the regression, while at lower temperatures a clear increase in CR is visible between 13 and 16 ∘C. Differences between sites for summer measurements might obscure a possible relationship of CR and temperature.
4.2 Benthic chambers
Due to the effective enclosure with benthic chambers, it was possible to assess the productivity of Posidonia oceanica and Cymodocea nodosa communities separately, confirming higher CR and GPP in P. oceanica communities (Fig. 4). Estimates are for the whole community and include epiphyte and bacterial communities associated with each seagrass species. The higher gross productivity (GPP) and community respiration (CR), calculated per surface area (m2), is logical as P. oceanica in general has a higher biomass per square meter and therefore is more productive relative to C. nodosa. Caution should be taken in interpreting these results, as no data for C. nodosa from the eastern basin were available and the database contained a higher number of P. oceanica estimates (n=42 for CR, GPP; n=79 for NCP), compared to C. nodosa (n=19).
There was a tendency for GPP and CR to decrease over the progressing years (2001–2019) for incubations of P. oceanica (Fig. S3); however, our analysis with mixed models and each publication as a random factor did not indicate significant differences. With a simpler model (linear model) the decrease in CR would have been significant (, p<0.001), as well as for GPP (, p<0.001) but not for the longer time series (1982–2019) of NCP. The decrease in CR is in contrast with the increase in CR found through time with sensor data (2007–2019); these differences may be due to the fact that sensors also detect trends from other photosynthetic organisms or limitations for seagrass communities in benthic chambers, as water renewal is limited (Champenois and Borges, 2012, 2019). No differences could be demonstrated in NCP and CR for both species through the seasons; however GPP was higher in summer. There were remarkable individual differences between the two species with an average positive NCP for P. oceanica, with only positive values found in benthic incubations and clear net autotrophic communities during the sampling periods in P. oceanica meadows. These meadows appear more productive than C. nodosa meadows, in agreement with previous studies (Duarte et al., 2010; Champenois and Borges, 2012, 2019), which had a higher incidence of heterotrophic communities.
4.3 Sensors vs. benthic chambers
Significantly different GPP, NCP and CR values were obtained for the different methods, with values almost an order of magnitude larger when estimated from sensor data (Table 1) compared to estimations from benthic chambers as previously reported by Champenois and Borges (2012). This difference may be due to a possible underestimation of the metabolic rates assessed by the benthic chambers methodology or an overestimation associated with water renovation at the placement of sensors. There are some limitations linked to the methodology using benthic chambers, as, even if most incubations use flexible material for the bags, which allows for movement and some mixing, there is no real interchange with the water column and nutrient limitation could occur. However the effect of oxygen or nutrient limitation should be limited when incubations are short (24 h) (Barrón and Duarte, 2009). The reduction in water motion could lead to the increase in the width of the diffusive boundary layer (DBL) between a seagrass leaf and the water column and slower exchange of nutrients and CO2 with the water column, since water velocity determines DBL boundary thickness (Enríquez and Rodríguez-Román, 2006; Hendriks et al., 2017). Another possible explanation for the underestimation in benthic chambers of metabolic rates could be the fact that the insertion of the base of the benthic chambers into the sediment may cut the roots and rhizomes, but this should be considered a rare event, as most of the biomass of the belowground tissues of P. oceanica and C. nodosa is located at deeper strata. For the seagrass physiology, rhizomes play an important role, as they translocate resources between shoots (Marbà et al., 2002), therefore affecting seagrass metabolism if they are severed. Another reason that may explain a possible underestimation in metabolic rates is the fact that pH may increase, together with oxygen during the day; those two factors, together with a high irradiance, conduct the ribulose-1,5-biphosphate-carboxylase-oxygenase (RuBisCo) enzyme to change from carboxylase to oxygenase (Heber et al., 1996). Under this reaction there is a higher consumption of oxygen and a carbon dioxide exudation which may be conducive to a lower GPP estimation from the change in oxygen (Champenois and Borges, 2012). Nonetheless, benthic chambers can provide measurements for meadows of a single species when enclosures are properly selected. Multiparametric sensors measure a composed signal affected by all surrounding photosynthetic (and heterotrophic) organisms, and measured metabolic rates reflect an ecosystem estimation. Measurements with the eddy covariance methodology, providing estimates on shorter timescales and therefore limiting the effect of lateral transport of water masses, have led to estimations of NCP for P. oceanica ranging from 85 to 119 mmol O2 m−2 d−1 in nearshore meadows (Koopmans et al., 2020). These estimates are closer to our estimates obtained from sensors, which on average were between 1.7 and 1.2 times higher, while the values obtained from benthic chambers are much lower, with eddy covariance estimates 4.5–6.3 times higher than the NCP obtained from benthic chambers. The used method should therefore be selected depending on the study objectives taking the focus of the study into account – whether it is more important to attribute productivity to a certain species or surface or if an ecosystem estimate is required.
Independent of the method used, two main limitations remain: the lower number of available data within the eastern Mediterranean and the higher sampling frequency during summer compared to other seasons. More than half (55 % for benthic chambers and 70 % for sensor data) of the data were collected in summer, due to the logistic restraints of underwater observations. The lack of data for the eastern basin is specifically urgent to solve, as climate change does not act homogeneously on the two regions and warming is faster for the eastern basin (Amitai et al., 2020; Nykjaer, 2009). Failing to collect actual data on metabolic rates could be detrimental for our knowledge on the rates of change in the Mediterranean in the future. We also highlight the lack of data for C. nodosa compared to P. oceanica. This is probably due to the fact that C. nodosa has not had a marked historical presence in many locations, especially in the western Mediterranean. Its current expanse and apparent lower sensitivity to higher temperatures compared to P. oceanica convert C. nodosa meadows into potential important players governing coastal metabolic signals deserving a closer look regarding productivity and controlling factors.
4.4 Seagrass metabolism and carbon burial
More than three-quarters, 80.9 %, of the NCP values were positive, reflecting the strong capacity of seagrass meadows to act as carbon sinks, which is also exemplified by the high number of ratios above one for both methodologies (Table 1). P. oceanica communities tended to be net autotrophic. The exception for this net autotrophic averaged NCP values was found in the eastern basin, in Maridati (Crete, Greece), where the averaged NCP was −236.1 mmol O2 m−2 d−1 during July 2017. This value is in agreement with the low decadal carbon burial rates and stocks measured by Wesselmann et al. (2021) at the same site. This station is located near a temporary stream and receives its discharges, which implies an extra nutrient input into this area. In the 1980s there was a massive sediment input (Wesselmann et al., 2021) that may have caused mortality and physiological stress to the seagrass meadow, and this may be still reflected in the net heterotrophic metabolism observed 2–3 decades later. We also saw heterotrophic meadows in the Bay of Pollença (Mallorca, Spain) during spring 2018 (on average −137.1 mmol O2 m−2 d−1). This sampling site is located near a nutrient source with a high input of organic matter from the Albufera coastal lagoon. Additionally, the organic matter input from the nearby harbor and the sewage systems from urban areas and hotels may have affected the metabolism of the seagrasses in this site. Sampling for additional parameters like nutrient values in the water column and sedimentation of organic matter and nutrients at the sites of the metabolism measurements could add important information, enabling the explanation of deviant metabolic rates. Seagrass ecosystems are mainly net autotrophic and hence act as carbon sinks but might be threatened and disappear due to high organic inputs and other anthropic threats (e.g., mechanic destruction of the seagrass meadows).
Seagrass metabolic rates (net community production, NCP; gross primary production, GPP; and community respiration, CR) are significantly different depending on the methodology used. The rates obtained with benthic chambers are lower than those obtained with multiparametric sensors. With the benthic-chamber methodology, seagrass metabolism at a species level can be compared, with demonstrated differences between Posidonia oceanica and Cymodocea nodosa for GPP and CR. P. oceanica was the more productive species compared to C. nodosa, but it also has higher respiration rates. Multiparametric sensors can assess metabolism at an ecosystem level and showed NCP was higher in the western Mediterranean basin compared to the eastern basin. Benthic-chamber measurements could not demonstrate a link between temperature and metabolic rates, while sensor data showed an increase in NCP and an unexpected decrease in CR with higher temperatures. When we plot our data according to the year, we see an increase in CR calculated from sensor data, while benthic-chamber rates have been decreasing for CR and GPP over the years. However, care should be taken in interpreting these trends, as this in inconclusive due to the variability in seasonal measurements and measurement sites.
There is a publication bias with a higher number of observations in the western region and a more elevated number of observations in summer compared to other seasons. The possibility of deploying multiparametric sensors for longer periods than benthic chambers allows for longer observational periods and detection of high GPP values that could be underestimated due to possible nutrient limitation or missed due to the shorter incubation time of the benthic-chamber technique. Sampling during different time periods confirmed the switch between negative and positive NCP values, with changes between autotrophy and heterotrophy in a same location during different periods of the year, therefore reinforcing the importance of monitoring during the whole year and not only summer. The high percentage of autotrophic meadows highlights their key role for climate change mitigation, by acting as carbon sinks through growth as well as through accumulation of allochthonous carbon through particle retention. Therefore, it is important to augment the knowledge on seagrass metabolism in regions and seasons where there are few data available to prevent the deterioration of seagrass meadows in the context of climate change where they play an essential role.
Metabolic rates of the seagrass meadows were calculated using a modification of the model of Cole et al. (2000), implemented in MATLAB (version 7.5, MathWorks Inc.) and explained in detail in Vaquer-Sunyer et al. (2012). The current version is available at https://github.com/PAgueda/Code (Agueda et al., 2022).
The full dataset is publicly available at https://digital.csic.es/handle/10261/263004 (Hendriks et al., 2022).
The supplement related to this article is available online at: https://doi.org/10.5194/bg-19-4619-2022-supplement.
IEH and NM conceptualized the project. MW, SF, RVS, IEH and NM collected the data. IEH, AEM and SF performed the analyses. IEH and AEM compiled the relevant literature. All authors contributed to the writing of the article.
The contact author has declared that none of the authors has any competing interests.
Publisher's note: Copernicus Publications remains neutral with regard to jurisdictional claims in published maps and institutional affiliations.
We would like to thank Eugenia Apostolaki and the two anonymous reviewers for their reviews and comments.
This work was funded by the Spanish Ministry of Economy and Competitiveness
(MEDSHIFT, grant no. CGL2015-71809-P) and the Spanish Ministry of Science, Universities and Innovation (project SUMAECO, grant no. RTI2018-095441-B-C21).
Susana Flecha was supported by a Margalida Comas postdoctoral scholarship, funded
by the government of the Balearic Islands.
We acknowledge support of the publication fee by the CSIC Open Access Publication Support Initiative through its Unit of Information Resources for Research (URICI).
This paper was edited by Aninda Mazumdar and reviewed by two anonymous referees.
Agawin, N. S. R., Ferriol, P., Sintes, E., and Moyà, G.: Temporal and spatial variability of in situ nitrogen fixation activities associated with the Mediterranean seagrass Posidonia oceanica meadows, Limnol. Oceanogr., 62, 2575–2592, https://doi.org/10.1002/lno.10591, 2017.
Agueda, P., Hendriks, I. E., Flecha, S., and Jorda, B.: Modified matlab model based on Cole et al. (2000), GitHub [code], https://github.com/PAgueda/Code, last access: 16 June 2022.
Alcoverro, T., Duarte, C. M., and Romero, J.: Annual growth dynamics of Posidonia oceanica: contribution of large-scale versus local factors to seasonality, Mar. Ecol.-Prog. Ser., 120, 203–210, 1995.
Ali, E., Cramer, W., Carnicer, J., Georgopoulou, E., Hilmi, N. J. M., Le Cozannet, G., and Lionello, P.: Cross-Chapter Paper 4: Mediterranean Region, in: Climate Change 2022: Impacts, Adaptation and Vulnerability. Contribution of Working Group II to the Sixth Assessment Report of the Intergovernmental Panel on Climate Change, edited by: Pörtner, H.-O., Roberts, D. C., Tignor, M., Poloczanska, E. S., Mintenbeck, K., Alegría, A., Craig, M., Langsdorf, S., Löschke, S., Möller, V., Okem, A., and Rama, B., Cambridge University Press, Cambridge, UK and New York, NY, USA, 2233–2272, https://www.ipcc.ch/report/ar6/wg2/downloads/report/IPCC_AR6_WGII_CCP4.pdf, last access: 20 September 2022.
Amitai, Y., Yam, R., Montagna, P., Devoti, S., Correa, M. L., and Shemesh, A.: Spatial and temporal variability in Mediterranean climate over the last millennium from vermetid isotope records and CMIP5/PMIP3 models, Global Planet. Change, 189, 103159, https://doi.org/10.1016/j.gloplacha.2020.103159, 2020.
Apostolaki, E. T., Holmer, M., Marbà, N., and Karakassis, I.: Metabolic Imbalance in Coastal Vegetated (Posidonia oceanica) and Unvegetated Benthic Ecosystems, Ecosystems, 13, 459–471, https://doi.org/10.1007/s10021-010-9330-9, 2010.
Armitage, A. R. and Fourqurean, J. W.: Carbon storage in seagrass soils: long-term nutrient history exceeds the effects of near-term nutrient enrichment, Biogeosciences, 13, 313–321, https://doi.org/10.5194/bg-13-313-2016, 2016.
Aufdenkampe, A. K., Mayorga, E., Raymond, P. A., Melack, J. M., Doney, S. C., Alin, S. R., Aalto, R. E., and Yoo, K.: Riverine coupling of biogeochemical cycles between land, oceans, and atmosphere, Front. Ecol. Environ., 9, 53–60, https://doi.org/10.1890/100014, 2011.
Barrón, C. and Duarte, C. M.: Dissolved organic matter release in a Posidonia oceanica meadow, Mar. Ecol.-Prog. Ser., 374, 75–84, 2009.
Barrón, C., Marbà, N., Terrados, J., Kennedy, H., and Duarte, C. M.: Community metabolism and carbon budget along a gradient of seagrass (Cymodocea nodosa) colonization, Limnol. Oceanogr., 49, 1642–1651, https://doi.org/10.4319/lo.2004.49.5.1642, 2004.
Barrón, C., Duarte, C. M., Frankignoulle, M., and Borges, A. V.: Organic carbon metabolism and carbonate dynamics in a Mediterranean seagrass (Posidonia oceanica), meadow, Estuar. Coast., 29, 417–426, https://doi.org/10.1007/BF02784990, 2006.
Bay, D.: A field study of the growth dynamics and productivity of Posidonia oceanica (L.) delile in Calvi Bay, Corsica, Aquat. Bot., 20, 43–64, https://doi.org/10.1016/0304-3770(84)90026-3, 1984.
Béthoux, J. P. and Copin-Montégut, G.: Biological fixation of atmospheric nitrogen in the Mediterranean Sea, Limnol. Oceanogr., 31, 1353–1358, https://doi.org/10.4319/lo.1986.31.6.1353, 1986.
Bonacorsi, M., Pergent-Martini, C., Breand, N., and Pergent, G.: Is Posidonia oceanica regression a general feature in the Mediterranean Sea?, Mediterr. Mar. Sci., 14, 193–203, https://doi.org/10.12681/mms.334, 2013.
Boudouresque, C. F., Bernard, G., Pergent, G., Shili, A., and Verlaque, M.: Regression of Mediterranean seagrasses caused by natural processes and anthropogenic disturbances and stress: a critical review, Bot. Mar., 52, 395–418, https://doi.org/10.1515/BOT.2009.057, 2009.
Brown, J. H., Gillooly, J. F., Allen, A. P., Savage, V. M., and West, G. B.: Toward a metabolic theory of ecology, Ecology, 85, 1771–1789, https://doi.org/10.1890/03-9000, 2004.
Burrows, M. T., Schoeman, D. S., Buckley, L. B., Moore, P., Poloczanska, E. S., Brander, K. M., Brown, C., Bruno, J. F., Duarte, C. M., Halpern, B. S., Holding, J., Kappel, C. V., Kiessling, W., O'Connor, M. I., Pandolfi, J. M., Parmesan, C., Schwing, F. B., Sydeman, W. J., and Richardson, A. J.: The Pace of Shifting Climate in Marine and Terrestrial Ecosystems, Science, 334, 652–655, https://doi.org/10.1126/science.1210288, 2011.
Cebrian, J.: Patterns in the Fate of Production in Plant Communities, Am. Nat., 154, 449–468, https://doi.org/10.1086/303244, 1999.
Cebrian, J., Duarte, C. M., Marba, N., and Enriquez, S.: Magnitude and fate of the production of four co-occurring western Mediterranean seagrass species, Mar. Ecol.-Prog. Ser., 155, 29-44, 1997.
Champenois, W. and Borges, A. V.: Seasonal and interannual variations of community metabolism rates of a Posidonia oceanica seagrass meadow, Limnol. Oceanogr., 57, 347–361, https://doi.org/10.4319/lo.2012.57.1.0347, 2012.
Champenois, W. and Borges, A. V.: Inter-annual variations over a decade of primary production of the seagrass Posidonia oceanica, Limnol. Oceanogr., 64, 32–45, https://doi.org/10.1002/lno.11017, 2019.
Chefaoui, R. M., Duarte, C. M., and Serrão, E. A.: Dramatic loss of seagrass habitat under projected climate change in the Mediterranean Sea, Glob. Change Biol., 24, 4919–4928, https://doi.org/10.1111/gcb.14401, 2018.
Cole, J. J. and Caraco, N. F.: Atmospheric exchange of carbon dioxide in a low-wind oligotrophic lake measured by the addition of SF6, Limnol. Oceanogr., 43, 647–656, https://doi.org/10.4319/lo.1998.43.4.0647, 1998.
Cole, J. J., Pace, M. L., Carpenter, S. R., and Kitchell, J. F.: Persistence of net heterotrophy in lakes during nutrient addition and food web manipulations, Limnol. Oceanogr., 45, 1718–1730, https://doi.org/10.4319/lo.2000.45.8.1718, 2000.
Coloso, J. J., Cole, J. J., Hanson, P. C., and Pace, M. L.: Depth-integrated, continuous estimates of metabolism in a clear-water lake, Can. J. Fish. Aquat. Sci., 65, 712–722, 10.1139/f08-006, 2008.
Condie, S. A. and Webster, I. T.: Estimating Stratification in Shallow Water Bodies from Mean Meteorological Conditions, J. Hydraul. Eng., 127, 286–292, https://doi.org/10.1061/(ASCE)0733-9429(2001)127:4(286), 2001.
Conley, D. J., Carstensen, J., Vaquer-Sunyer, R., and Duarte, C. M.: Ecosystem thresholds with hypoxia, in: Eutrophication in Coastal Ecosystems: Towards better understanding and management strategies. Selected Papers from the Second International Symposium on Research and Management of Eutrophication in Coastal Ecosystems, 20–23 June 2006, Nyborg, Denmark, edited by: Andersen, J. H. and Conley, D. J., Springer Netherlands, Dordrecht, 21–29, https://doi.org/10.1007/978-90-481-3385-7_3, 2009.
de los Santos, C. B., Krause-Jensen, D., Alcoverro, T., Marbà, N., Duarte, C. M., van Katwijk, M. M., Pérez, M., Romero, J., Sánchez-Lizaso, J. L., Roca, G., Jankowska, E., Pérez-Lloréns, J. L., Fournier, J., Montefalcone, M., Pergent, G., Ruiz, J. M., Cabaço, S., Cook, K., Wilkes, R. J., Moy, F. E., Trayter, G. M.-R., Arañó, X. S., de Jong, D. J., Fernández-Torquemada, Y., Auby, I., Vergara, J. J., and Santos, R.: Recent trend reversal for declining European seagrass meadows, Nat. Commun., 10, 3356, https://doi.org/10.1038/s41467-019-11340-4, 2019.
Dennison, W. C.: Effects of light on seagrass photosynthesis, growth and depth distribution, Aquat. Bot., 27, 15–26, https://doi.org/10.1016/0304-3770(87)90083-0, 1987.
Diaz, R. J.: Overview of hypoxia around the world, J. Environ. Qual., 30, 275–281, https://doi.org/10.2134/jeq2001.302275x, 2001.
Díaz, R. J. and Rosenberg, R.: Marine benthic hypoxia: a review of its ecological effects and the behavioural responses of benthic macrofauna, Oceanogr. Mar. Biol., 33, 245–303, 1995.
Diaz-Almena, E., Marbà, N., and Duarte, C. M.: Consequences of Mediterranean warming events in seagrass (Posidonia oceanica) flowering records, Glob. Change Biol., 13, 224–235, https://doi.org/10.1111/j.1365-2486.2006.01260.x, 2007.
Duarte, C. M., Middelburg, J. J., and Caraco, N.: Major role of marine vegetation on the oceanic carbon cycle, Biogeosciences, 2, 1–8, https://doi.org/10.5194/bg-2-1-2005, 2005.
Duarte, C. M., Marbà, N., Gacia, E., Fourqurean, J. W., Beggins, J., Barrón, C., and Apostolaki, E. T.: Seagrass community metabolism: Assessing the carbon sink capacity of seagrass meadows, Global Biogeochem. Cy., 24, GB4032, https://doi.org/10.1029/2010GB003793, 2010.
Duarte, C. M., Hendriks, I. E., Moore, T. S., Olsen, Y. S., Steckbauer, A., Ramajo, L., Carstensen, J., Trotter, J. A., and McCulloch, M.: Is Ocean Acidification an Open-Ocean Syndrome? Understanding Anthropogenic Impacts on Seawater pH, Estuar. Coasts, 36, 221–236, https://doi.org/10.1007/s12237-013-9594-3, 2013.
Egea, L. G., Jiménez-Ramos, R., Vergara, J. J., Hernández, I., and Brun, F. G.: Interactive effect of temperature, acidification and ammonium enrichment on the seagrass Cymodocea nodosa, Mar. Pollut. Bull., 134, 14–26, https://doi.org/10.1016/j.marpolbul.2018.02.029, 2018.
Enríquez, S. and Rodríguez-Román, A.: Effect of water flow on the photosynthesis of three marine macrophytes from a fringing-reef lagoon, Mar. Ecol.-Prog. Ser., 323, 119–132, 2006.
Fofonoff, N. P. and Millard Jr., R. C.: Algorithms for the computation of fundamental properties of seawater, in: UNESCO Technical Papers in Marine Sciences, UNESCO, Paris, France, 53 pp., https://doi.org/10.25607/OBP-1450, 1983.
Fourqurean, J. W., Duarte, C. M., Kennedy, H., Marbà, N., Holmer, M., Mateo, M. A., Apostolaki, E. T., Kendrick, G. A., Krause-Jensen, D., McGlathery, K. J., and Serrano, O.: Seagrass ecosystems as a globally significant carbon stock, Nat. Geosci., 5, 505–509, https://doi.org/10.1038/ngeo1477, 2012.
Frankignoulle, M. and Bouquegneau, J. M.: Seasonal variation of the diel carbon budget of a marine macrophyte ecosystem, Mar. Ecol.-Prog. Ser., 38, 197–199, 1987.
Gacia, E., Marba, N., Cebrian, J., Vaquer-Sunyer, R., Garcias-Bonet, N., and Duarte, C.: Thresholds of irradiance for seagrass (Posidonia oceanica) meadow metabolism: An experimental approach, Mar. Ecol.-Prog. Ser., 466, 69–79, https://doi.org/10.3354/meps09928, 2012.
Gazeau, F., Duarte, C. M., Gattuso, J.-P., Barrón, C., Navarro, N., Ruiz, S., Prairie, Y. T., Calleja, M., Delille, B., Frankignoulle, M., and Borges, A. V.: Whole-system metabolism and CO2 fluxes in a Mediterranean Bay dominated by seagrass beds (Palma Bay, NW Mediterranean), Biogeosciences, 2, 43–60, https://doi.org/10.5194/bg-2-43-2005, 2005.
Giorgi, F.: Climate change hot-spots, Geophys. Res. Lett., 33, L08707, https://doi.org/10.1029/2006GL025734, 2006.
Giorgi, F. and Lionello, P.: Climate change projections for the Mediterranean region, Global Planet. Change, 63, 90–104, https://doi.org/10.1016/j.gloplacha.2007.09.005, 2008.
Gobert, S., Cambridge, M. L., Velimirov, Pergent, G., Lepoint, G., Bouquegneau, J.-M., Dauby, P., Pergent-Martini, C., and Walker, D.: Biology of Posidonia, in: SEAGRASSES: BIOLOGY, ECOLOGYAND CONSERVATION, Springer, 387 pp., https://doi.org/10.1007/978-1-4020-2983-7_17, 2006.
Grande, K. D., Marra, J. F., Langdon, C., Heinemann, K., and Bender, M. L.: Rates of respiration in the light measured in marine phytoplankton using an 18O isotope-labelling technique, J. Exp. Mar. Biol. Ecol., 129, 95–120, 1989.
Greiner, J. T., McGlathery, K. J., Gunnell, J., and McKee, B. A.: Seagrass Restoration Enhances “Blue Carbon” Sequestration in Coastal Waters, PLOS ONE, 8, e72469, https://doi.org/10.1371/journal.pone.0072469, 2013.
Gutiérrez, J. L., Jones, C. G., Byers, J. E., Arkema, K. K., Berkenbusch, K., Commito, J. A., Duarte, C. M., Hacker, S. D., Lambrinos, J. G., Hendriks, I. E., Hogarth, P. J., Palomo, M. G., and Wild, C.: 7.04 – Physical Ecosystem Engineers and the Functioning of Estuaries and Coasts A2 – Wolanski, Eric, in: Treatise on Estuarine and Coastal Science, edited by: McLusky, D., Academic Press, Waltham, 53–81, https://doi.org/10.1016/B978-0-12-374711-2.00705-1, 2011.
Heber, U., Bligny, R., Streb, P., and Douce, R.: Photorespiration is Essential for the Protection of the Photosynthetic Apparatus of C3 Plants Against Photoinactivation Under Sunlight, Bot. Acta, 109, 307–315, https://doi.org/10.1111/j.1438-8677.1996.tb00578.x, 1996.
Hendriks, I. E., Sintes, T., Bouma, T. J., and Duarte, C. M.: Experimental assessment and modeling evaluation of the effects of the seagrass Posidonia oceanica on flow and particle trapping, Mar. Ecol.-Prog. Ser., 356, 163–173, https://doi.org/10.3354/meps07316, 2008.
Hendriks, I. E., Duarte, C. M., and Alvarez, M.: Vulnerability of marine biodiversity to ocean acidification: A meta-analysis, Estuar. Coast. Shelf S., 86, 157–164, https://doi.org/10.1016/j.ecss.2009.11.022, 2010.
Hendriks, I. E., Olsen, Y. S., Ramajo, L., Basso, L., Steckbauer, A., Moore, T. S., Howard, J., and Duarte, C. M.: Photosynthetic activity buffers ocean acidification in seagrass meadows, Biogeosciences, 11, 333–346, https://doi.org/10.5194/bg-11-333-2014, 2014.
Hendriks, I. E., Duarte, C. M., Marbà, N., and Krause-Jensen, D.: pH gradients in the diffusive boundary layer of subarctic macrophytes, Polar Biol., 40, 2343–2348, https://doi.org/10.1007/s00300-017-2143-y, 2017.
Hendriks, I. E., Escolano-Moltó, A., Flecha, S., Vaquer-Sunyer, R., Wesselmann, M., and Marbà, N.: Mediterranean seagrasses as carbon sinks: Methodological and regional differences, Digital CSIC [data set], https://digital.csic.es/handle/10261/263004, last access: 1 June 2022.
Hofmann, G. E., Smith, J. E., Johnson, K. S., Send, U., Levin, L. A., Micheli, F., Paytan, A., Price, N. N., Peterson, B., Takeshita, Y., Matson, P. G., Crook, E. D., Kroeker, K. J., Gambi, M. C., Rivest, E. B., Frieder, C. A., Yu, P. C., and Martz, T. R.: High-Frequency Dynamics of Ocean pH: A Multi-Ecosystem Comparison, PLOS ONE, 6, e28983, https://doi.org/10.1371/journal.pone.0028983, 2011.
Holloway, P. E.: A Criterion for Thermal Stratification in a Wind-Mixed System, J. Phys. Oceanogr., 10, 861–869, 1980.
Holmer, M., Duarte, C. M., Boschker, H. T. S., and Barrón, C.: Carbon cycling and bacterial carbon sources in pristine and impacted Mediterranean seagrass sediments, Aquat. Microb. Ecol., 36, 227–237, 2004.
IAPWS: Release on the IAPWS Formulation 2008 for the Thermodynamic Properties of Seawater, The International Association for the Properties of Water and Steam, Berlin, Germany, September 2008, http://www.iapws.org/relguide/seawater.pdf (last access: 22 October 2020), 2008.
IAPWS: Supplementary Release on a Computationally Efficient Thermodynamic Formulation for Liquid Water for Oceanographic Use. The International Association for the Properties of Water and Steam. Doorwerth, the Netherlands, September 2009, https://www.iapws.org, 2009.
IOC (Intergovernmental Oceanographic Commission, Scientific Committee on Oceanic Research; International Association for the Physical Sciences of the Oceans): The International thermodynamic equation of seawater – 2010: calculation and use of thermodynamic properties [includes corrections up to 31st October 2015], in: Intergovernmental Oceanographic Commission Manuals and Guides, UNESCO, Paris, France, 196 pp., https://doi.org/10.25607/OBP-1338, 2015.
Jordà, G., Marbà, N., and Duarte, C. M.: Mediterranean seagrass vulnerable to regional climate warming, Nat. Clim. Change, 2, 821–824, https://doi.org/10.1038/nclimate1533, 2012.
Karim, M. R., Sekine, M., Higuchi, T., Imai, T., and Ukita, M.: Simulation of fish behavior and mortality in hypoxic water in an enclosed bay, Ecol. Model., 159, 27–42, https://doi.org/10.1016/S0304-3800(02)00282-X, 2003.
Karl, D. M., Laws, E. A., Morris, P., Williams, P. J. L., and Emerson, S.: Metabolic balance of the open sea, Nature, 426, 32–32, https://doi.org/10.1038/426032a, 2003.
Keeling, R. F. and Garcia, H. E.: The change in oceanic O2 inventory associated with recent global warming, P. Natl. Acad. Sci. USA, 99, 7848-7853, https://doi.org/10.1073/pnas.122154899, 2002.
Keeling, R. F., Körtzinger, A., and Gruber, N.: Ocean Deoxygenation in a Warming World, Annu. Rev. Mar. Sci., 2, 199–229, https://doi.org/10.1146/annurev.marine.010908.163855, 2010.
Kelly, M. W. and Hofmann, G. E.: Adaptation and the physiology of ocean acidification, Funct. Ecol., 27, 980–990, https://doi.org/10.1111/j.1365-2435.2012.02061.x, 2013.
Kennedy, H., Beggins, J., Duarte, C. M., Fourqurean, J. W., Holmer, M., Marbà, N., and Middelburg, J. J.: Seagrass sediments as a global carbon sink: Isotopic constraints, Global Biogeochem. Cy., 24, GB4026, https://doi.org/10.1029/2010GB003848, 2010.
Kihm, C. and Körtzinger, A.: Air-sea gas transfer velocity for oxygen derived from float data, J. Geophys. Res.-Oceans, 115, C12003, https://doi.org/10.1029/2009JC006077, 2010.
Koopmans, D., Holtappels, M., Chennu, A., Weber, M., and de Beer, D.: High Net Primary Production of Mediterranean Seagrass (Posidonia oceanica) Meadows Determined With Aquatic Eddy Covariance, Front. Mar. Sci., 7, 118, https://doi.org/10.3389/fmars.2020.00118, 2020.
Labasque, T., Chaumery, C., Aminot, A., and Kergoat, G.: Spectrophotometric Winkler determination of dissolved oxygen: re-examination of critical factors and reliability, Mar. Chem., 88, 53–60, https://doi.org/10.1016/j.marchem.2004.03.004, 2004.
Lacoue-Labarthe, T., Nunes, P. A. L. D., Ziveri, P., Cinar, M., Gazeau, F., Hall-Spencer, J. M., Hilmi, N., Moschella, P., Safa, A., Sauzade, D., and Turley, C.: Impacts of ocean acidification in a warming Mediterranean Sea: An overview, Regional Studies in Marine Science, 5, 1–11, https://doi.org/10.1016/j.rsma.2015.12.005, 2016.
Lejeusne, C., Chevaldonné, P., Pergent-Martini, C., Boudouresque, C. F., and Pérez, T.: Climate change effects on a miniature ocean: the highly diverse, highly impacted Mediterranean Sea, Trends Ecol. Evol., 25, 250–260, https://doi.org/10.1016/j.tree.2009.10.009, 2010.
Marbà, N. and Duarte, C. M.: Mediterranean warming triggers seagrass (Posidonia oceanica) shoot mortality, Glob. Change Biol., 16, 2366–2375, 2010.
Marbà, N., Hemminga, M. A., Mateo, M. A., Duarte, C. M., Mass, Y. E. M., Terrados, J., and Gacia, E.: Carbon and nitrogen translocation between seagrass ramets, Mar. Ecol.-Prog. Ser., 226, 287–300, 2002.
Marbà, N., Díaz-Almela, E., and Duarte, C. M.: Mediterranean seagrass (Posidonia oceanica) loss between 1842 and 2009, Biol. Conserv., 176, 183–190, 2014.
Marx, L., Flecha, S., Wesselmann, M., Morell, C., and Hendriks, I. E.: Marine Macrophytes as Carbon Sinks: Comparison Between Seagrasses and the Non-native Alga Halimeda incrassata in the Western Mediterranean (Mallorca), Front. Mar. Sci., 8, 746379, https://doi.org/10.3389/fmars.2021.746379, 2021.
Mateo, M. A., Cebrián, J., Dunton, K., and Mutchler, T.: Carbon flux in seagrass ecosystems, in: Seagrasses: Biology, Ecology and Conservation, edited by: Larkum, A. W. D., Orth, R. J., and Duarte, C. M., Springer, Dordrecht, the Netherlands, 159–192, https://link.springer.com/chapter/10.1007/978-1-4020-2983-7_7 (last access: 20 September 2022), 2006.
MATLAB: MATLAB and Statistics Toolbox Release, The MathWorks, Inc., Natick, Massachusetts, United States, https://es.mathworks.com/products/statistics.html (last access: 18 August 2021), 2012.
McDougall, T. J. and Barker, P. M.: Getting started with TEOS-10 and the Gibbs Seawater (GSW) oceanographic toolbox, SCOR/IAPSO WG, 127, 1–28, https://www.TEOS-10.org (last access: 19 September 2022), 2011.
Mcleod, E., Chmura, G. L., Bouillon, S., Salm, R., Björk, M., Duarte, C. M., Lovelock, C. E., Schlesinger, W. H., and Silliman, B. R.: A blueprint for blue carbon: toward an improved understanding of the role of vegetated coastal habitats in sequestering CO2, Front. Ecol. Environ., 9, 552–560, https://doi.org/10.1890/110004, 2011.
Micheli, F., Halpern, B. S., Walbridge, S., Ciriaco, S., Ferretti, F., Fraschetti, S., Lewison, R., Nykjaer, L., and Rosenberg, A. A.: Cumulative Human Impacts on Mediterranean and Black Sea Marine Ecosystems: Assessing Current Pressures and Opportunities, PLOS ONE, 8, e79889, https://doi.org/10.1371/journal.pone.0079889, 2013.
Nykjaer, L.: Mediterranean Sea surface warming 1985–2006, Clim. Res., 39, 11–17, 2009.
Odum, H. T. and Hoskin, C. M.: Comparative studies on the metabolism of marine waters, Publications of the Institute of Marine Science, Texas, 5, 16–46, 1958.
Odum, H. T. and Wilson, R. F.: Further studies on reaeration and metabolism of Texas bays, 1958–1960, Publications of the Institute of Marine Science, Texas, 8, 23–55, 1962.
Olivé, I., Silva, J., Costa, M. M., and Santos, R.: Estimating Seagrass Community Metabolism Using Benthic Chambers: The Effect of Incubation Time, Estuaries and Coasts, 39, 138–144, https://doi.org/10.1007/s12237-015-9973-z, 2016.
Olsen, Y. S., Sánchez-Camacho, M., Marbà, N., and Duarte, C. M.: Mediterranean Seagrass Growth and Demography Responses to Experimental Warming, Estuar. Coasts, 35, 1205–1213, https://doi.org/10.1007/s12237-012-9521-z, 2012.
Orth, R. J., Carruthers, T. J. B., Dennison, W. C., Duarte, C. M., Fourqurean, J. W., Heck, K. L., Hughes, A. R., Kendrick, G. A., Kenworthy, W. J., Olyarnik, S., Short, F. T., Waycott, M., and Williams, S. L.: A Global Crisis for Seagrass Ecosystems, Bioscience, 56, 987–996, https://doi.org/10.1641/0006-3568(2006)56[987:Agcfse]2.0.Co;2, 2006.
Pace, M. L. and Prairie, Y. T.: Respiration in lakes, Respiration in aquatic ecosystems, 1, 103–122, 2005.
Paerl, H. W.: Assessing and managing nutrient-enhanced eutrophication in estuarine and coastal waters: Interactive effects of human and climatic perturbations, Ecol. Eng., 26, 40–54, 2006.
Pasqualini, V., Pergent-Martini, C., Clabaut, P., and Pergent, G.: Mapping of Posidonia oceanica using Aerial Photographs and Side Scan Sonar: Application off the Island of Corsica (France), Estuar. Coast. Shelf S., 47, 359–367, 1998.
Pergent, G., Rico-Raimondino, V., and Pergent-Martini, C.: Fate of primary production in Posidonia oceanica meadows of the Mediterranean, Aquat. Bot., 59, 307–321, https://doi.org/10.1016/S0304-3770(97)00052-1, 1997.
Pringault, O., Tassas, V., and Rochelle-Newall, E.: Consequences of respiration in the light on the determination of production in pelagic systems, Biogeosciences, 4, 105–114, https://doi.org/10.5194/bg-4-105-2007, 2007.
R Core Team: R: A language and environment for statistical computing, R Foundation for Statistical Computing, Vienna, Austria, https://www.R-project.org/ (last access: 13 June 2022), 2021.
Ricart, A. M., Pérez, M., and Romero, J.: Landscape configuration modulates carbon storage in seagrass sediments, Estuar. Coast. Shelf S., 185, 69–76, 2017.
Richon, C., Dutay, J.-C., Bopp, L., Le Vu, B., Orr, J. C., Somot, S., and Dulac, F.: Biogeochemical response of the Mediterranean Sea to the transient SRES-A2 climate change scenario, Biogeosciences, 16, 135–165, https://doi.org/10.5194/bg-16-135-2019, 2019.
Romero, J., Pérez, M., Mateo, M. A., and Sala, E.: The belowground organs of the Mediterranean seagrass Posidonia oceanica as a biogeochemical sink, Aquat. Bot., 47, 13–19, https://doi.org/10.1016/0304-3770(94)90044-2, 1994.
Samper-Villarreal, J., Lovelock, C. E., Saunders, M. I., Roelfsema, C., and Mumby, P. J.: Organic carbon in seagrass sediments is influenced by seagrass canopy complexity, turbidity, wave height, and water depth, Limnol. Oceanogr., 61, 938–952, 2016.
Santos, R., Silva, J., Alexandre, A., Navarro, N., Barrón, C., and Duarte, C. M.: Ecosystem metabolism and carbon fluxes of a tidally-dominated coastal lagoon, Estuaries, 27, 977–985, https://doi.org/10.1007/BF02803424, 2004.
Savva, I., Bennett, S., Roca, G., Jordà, G., and Marbà, N.: Thermal tolerance of Mediterranean marine macrophytes: Vulnerability to global warming, Ecol. Evol., 8, 12032–12043, https://doi.org/10.1002/ece3.4663, 2018.
Serrano, O., Lavery, P. S., Rozaimi, M., and Mateo, M. Á.: Influence of water depth on the carbon sequestration capacity of seagrasses, Global Biogeochem. Cy., 28, 950–961, 2014.
Simboura, N., Pavlidou, A., Bald, J., Tsapakis, M., Pagou, K., Zeri, C., Androni, A., and Panayotidis, P.: Response of ecological indices to nutrient and chemical contaminant stress factors in Eastern Mediterranean coastal waters, Ecol. Indic., 70, 89–105, https://doi.org/10.1016/j.ecolind.2016.05.018, 2016.
Simpson, J. and Hunter, J.: Fronts in the Irish sea, Nature, 250, 404–406, 1974.
Telesca, L., Belluscio, A., Criscoli, A., Ardizzone, G., Apostolaki, E. T., Fraschetti, S., Gristina, M., Knittweis, L., Martin, C. S., Pergent, G., Alagna, A., Badalamenti, F., Garofalo, G., Gerakaris, V., Louise Pace, M., Pergent-Martini, C., and Salomidi, M.: Seagrass meadows (Posidonia oceanica) distribution and trajectories of change, Sci. Rep. UK, 5, 12505, https://doi.org/10.1038/srep12505, 2015.
Touratier, F. and Goyet, C.: Impact of the Eastern Mediterranean Transient on the distribution of anthropogenic CO2 and first estimate of acidification for the Mediterranean Sea, Deep-Sea Res. Pt. I, 58, 1–15, https://doi.org/10.1016/j.dsr.2010.10.002, 2011.
Trevathan-Tackett, S. M., Kelleway, J., Macreadie, P. I., Beardall, J., Ralph, P., and Bellgrove, A.: Comparison of marine macrophytes for their contributions to blue carbon sequestration, Ecology, 96, 3043–3057, 2015.
Vaquer-Sunyer, R. and Duarte, C. M.: Thresholds of hypoxia for marine biodiversity, P. Natl. Acad. Sci. USA, 105, 15452–15457, 2008.
Vaquer-Sunyer, R. and Duarte, C. M.: Experimental evaluation of the response of coastal Mediterranean planktonic and benthic metabolism to warming, Estuar. Coasts, 36, 697–707, 2013.
Vaquer-Sunyer, R., Duarte, C. M., Jordà, G., and Ruiz-Halpern, S.: Temperature Dependence of Oxygen Dynamics and Community Metabolism in a Shallow Mediterranean Macroalgal Meadow (Caulerpa prolifera). Estuar. Coasts, 35, 1182–1192, https://doi.org/10.1007/s12237-012-9514-y, 2012.
Vargas-Yáñez, M., Jesús García, M., Salat, J., García-Martínez, M. C., Pascual, J., and Moya, F.: Warming trends and decadal variability in the Western Mediterranean shelf, Global Planet. Change, 63, 177–184, 2008.
Wanninkhof, R.: Relationship between wind speed and gas exchange over the ocean, J. Geophys. Res., 97, 7373–7382, https://doi.org/10.1029/92JC00188, 1992.
Wanninkhof, R. and McGillis, W. R.: A cubic relationship between air-sea CO2 exchange and wind speed, Geophys. Res. Lett., 26, 1889–1892, 1999.
Waycott, M., Duarte, C. M., Carruthers, T. J., Orth, R. J., Dennison, W. C., Olyarnik, S., Calladine, A., Fourqurean, J. W., Heck, K. L., and Hughes, A. R.: Accelerating loss of seagrasses across the globe threatens coastal ecosystems, P. Natl. Acad. Sci. USA, 106, 12377–12381, 2009.
Wesselmann, M., Geraldi, N. R., Duarte, C. M., Garcia-Orellana, J., Díaz-Rúa, R., Arias-Ortiz, A., Hendriks, I. E., Apostolaki, E. T., and Marbà, N.: Seagrass (Halophila stipulacea) invasion enhances carbon sequestration in the Mediterranean Sea, Glob. Change Biol., 27, 2592–2607, https://doi.org/10.1111/gcb.15589, 2021.
Zhang, J., Gilbert, D., Gooday, A. J., Levin, L., Naqvi, S. W. A., Middelburg, J. J., Scranton, M., Ekau, W., Peña, A., Dewitte, B., Oguz, T., Monteiro, P. M. S., Urban, E., Rabalais, N. N., Ittekkot, V., Kemp, W. M., Ulloa, O., Elmgren, R., Escobar-Briones, E., and Van der Plas, A. K.: Natural and human-induced hypoxia and consequences for coastal areas: synthesis and future development, Biogeosciences, 7, 1443–1467, https://doi.org/10.5194/bg-7-1443-2010, 2010.
Ziegler, S. and Benner, R.: Ecosystem metabolism in a subtropical, seagrass-dominated lagoon, Mar. Ecol.-Prog. Ser., 173, 1–12, 1998.