the Creative Commons Attribution 4.0 License.
the Creative Commons Attribution 4.0 License.
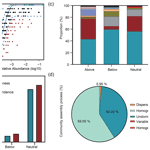
Diversity and assembly processes of microbial eukaryotic communities in Fildes Peninsula Lakes (West Antarctica)
Chunmei Zhang
Huirong Li
Yinxin Zeng
Haitao Ding
Bin Wang
Yangjie Li
Zhongqiang Ji
Yonghong Bi
Wei Luo
The diversity, co-occurrence patterns, and assembly processes of microbial eukaryotes (0.2–20 µm) in Antarctic freshwater lakes are not well understood, despite their wide distribution and ecological importance. This study used Illumina high-throughput sequencing to investigate the microbial eukaryotic communities of five freshwater lakes on the Fildes Peninsula over three summer seasons. A total of 28 phyla were detected, with phytoplankton occupying the highest percentage of sequences (accounting for up to 98 %). The dominant taxa consisted of Chrysophyta, Chlorophyta, and Cryptophyta. The species richness (113–268) and Shannon index (1.70–3.50) varied among the lakes, with higher values recorded in Lake Chang Hu and Lake Kitec and the lowest value obtained for Lake Yue Ya. There were significant differences between the microbial eukaryotic communities of the lakes, with spatial and temporal heterogeneity in the relative abundance of the dominant taxa (P<0.05). Environmental variables explained about 39 % of the variation in community structures, with water temperature and phosphate identified as the driving factors (P<0.05). Network analysis revealed comprehensive co-occurrence relationships (positive correlation 82 % vs. negative correlation 18 %). The neutral community model revealed that neutral processes explained more than 55 % of the community variation. Stochastic processes (e.g. homogenizing dispersal and undominated processes) predominated in community assembly over the deterministic processes. These findings demonstrate the diversity of the microbial eukaryotic communities in the freshwater lakes of the Fildes Peninsula and have important implications for understanding the community assembly in these ecosystems.
- Article
(5687 KB) -
Supplement
(529 KB) - BibTeX
- EndNote
The Fildes Peninsula, which makes up the southwestern end of King George Island, South Shetland Islands, Antarctica, is home to a relatively high number of scientific research stations. The peninsula is commonly ice-free throughout the austral summer, making it the largest ice-free area (40 km2) on King George Island. Located within the maritime Antarctic, the peninsula experiences 400–600 mm of precipitation each year and has an average annual temperature of −3 ∘C (Holdgate, 1977). Nevertheless, permafrost and periglacial processes also occur in the region (Barsch and Caine, 1984). Lakes on the Fildes Peninsula, along with those found in other ice-free areas of Antarctica, represent the year-round liquid water reservoirs on the continent (Priscu, 2010; Lyons et al., 2007). The availability and quality of water are impacted by sea conditions, macro-fauna usage, and anthropogenic influences, such as solid, volatile, and fluid waste production and disposal (Kawecka et al., 1998). Antarctic lake systems are sentinels for climate change and host globally relevant microbes and biogeochemical cycles (Marsh et al., 2020; Wilkins et al., 2013), thus making a more complete understanding of the processes shaping microbial communities there a priority. Moreover, the physical stability observed in these lakes makes them a good model system for interrogating biogeochemical processes within water columns (Comeau et al., 2012).
Microbial eukaryotes (0.2–20 µm, pico- and nanoeukaryotes are important components in microbial food webs and play a significant role in the biogeochemical cycles (Massana et al., 2015; Unrein et al., 2014; Grob et al., 2007), as well as contributing to plankton biomass and carbon export (Hernandez-Ruiz et al., 2018; Leblanc et al., 2018). However, microbial eukaryotes are generally difficult to study due to their small size and a common lack of distinguishing morphological features, especially among pico- and nanoeukaryotes. A molecular approach can be used to compensate for the lack of traditional microscopic methods, providing us with a convenient way to study these small-sized eukaryotes. The application of 18S rRNA gene-based molecular tools has revealed a high taxonomic diversity of microbial eukaryotes in some oligotrophic and extreme regions (Richards et al., 2005; Zhao et al., 2011; Marquardt et al., 2016). Nevertheless, previous research exploring the molecular diversity and the population fluctuations in these far cold and oligotrophic lakes of Antarctica is limited.
The small size, short generation time, quick reflection of environmental conditions, and genetic plasticity of microbial eukaryotes make them excellent bioindicators reflecting environmental disturbances and the quality of ecosystems (Karimi et al., 2017; Bouchez et al., 2016). A previous study revealed the presence of alveolates and dinoflagellates among small deep-sea Antarctic plankton eukaryotes (0.2–5 µm) and demonstrated that their diversity was affected by temperature and salinity (Lopez-Garcia et al., 2001). Microscopic observations have also been used to show that diatoms in the lakes of Fildes Peninsula region accounted for 59.8 % of the total number of phytoplankton species, and that water temperature and nutrients resulted in the variations in nano- and microphytoplankton abundance and composition (Zhu et al., 2010). A relatively low number of taxa, such as Nitzschia, Achnanthes, and Navicula, etc., have also been investigated from periodically brackish water ponds near the Polish Antarctic station on King George Island, whose abundance were influenced by the nutrients in the water (Kawecka et al., 1998). Indeed, the microphytoplankton communities of the shallow lakes of the Antarctic Peninsula are influenced by nutrients and surrounding inputs (Mataloni et al., 2000). Although several studies on other regions have explored the fluctuations of microbial eukaryotes and their relationship with environmental factors, the spatio-temporal variation, co-occurrence pattern, and community assembly of microbial eukaryotes in Antarctic (Fildes Peninsula) freshwater lakes have rarely been investigated.
Deterministic and stochastic processes are considered the two main ecological processes in community assembly (Ofiteru et al., 2010). Deterministic processes are based on the ecological niche theory; some deterministic factors (environmental conditions and species interactions) influence and determine community assembly (Powell et al., 2015). Stochastic processes are based on the neutral theory, which states that random birth or death, drift, and dispersal events also play an essential role in community assembly (Bahram et al., 2016). Deterministic processes are prevalent in building whole ecosystem communities (Liu et al., 2020) where selection leads to species classification, and applying similar habitats thus results in similar community assemblages. However, other studies have supported an important role of stochastic processes (e.g. drift and dispersal) in community assembly, where dispersal is the movement of species over spatial locations and drift is associated with the relative abundance of the species (Massana and Logares, 2013; Wu et al., 2019). Stochastic processes have been shown to account for up to 95 % of the microbial eukaryotic community assembly in a set of lakes in Eastern Antarctica (Logares et al., 2018). Stochastic processes have also been found to prevail in micro- and nanoplankton eukaryotic communities in the intertidal zones of southeast Fujian, China (Kong et al., 2019). Nonetheless, the study of microbial eukaryotic community assembly processes in Antarctica still requires further investigations for a more comprehensive view.
Few studies have been conducted on the microbial eukaryotic diversity and community assembly processes of the freshwater lakes on the Fildes Peninsula, Antarctica. Our study attempted to analyse the microbial eukaryotic samples of five freshwater lakes collected over three summers using high-throughput 18S rRNA sequencing. We aimed to (I) understand the diversity and co-occurrence of microbial eukaryotes and (II) explore the influencing factors and their community assembly processes.
2.1 Study area, sample collection, and measurement of environmental variables
Field samples were collected on the 34th (2017/2018), 35th (2018/2019), and 36th (2018/2019) Chinese Antarctic Research Expedition (CHINARE) in January 2018 (34th), December 2018 (35th), and December 2019 (36th). The investigations were conducted in the Chinese Great Wall Station area on King George Island, the largest of the South Shetland Islands. Surface water samples were collected from five lakes, namely Chang Hu (CH), Kitec (KT), Xi Hu (XH), Yan Ou (YO), and Yue Ya (YY) (Fig. 1). Moreover, the physio-chemical parameters of water samples were measured synchronously.
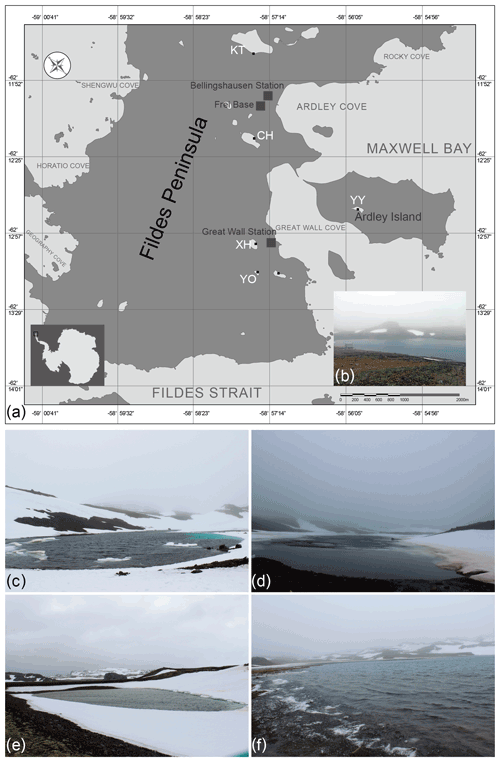
Figure 1(a) Location of the five studied lakes on the Fildes Peninsula, King George Island, Antarctica, (b) Lake Xi Hu (XH), (c) Lake Yan Ou (YO), (d) Lake Chang Hu (CH), (e) Lake Yue Ya (YY), and (f) Lake Kitec (KT).
CH has a narrow strip shape, surrounded by bulges, with major inputs from surrounding glacial melting water. KT is the closest to the Corinthian ice cap and is the source of drinking water for the Chilean station, located close to the airport for access to the Fildes Peninsula. KT is the largest lake in the investigated area. XH is the drinking water source area for the Chinese Great Wall Station. YO is surrounded by mountains and is snow-covered, with moss and lichen growing in the soil; it is the smallest lake in the area of investigation and is relatively sensitive to the effects of scientific expeditions. YY, situated on Ardley Island, is situated far from human activities, but the chemistry of its waters is influenced by the excrements of penguins dwelling on the island.
Water temperature (WT), pH, and salinity (Sal) were measured using an RBRconcerto C.T.D (Canada). In addition to the in-situ measurements, a water sample obtained 0.5 m below the surface was collected at each monitoring site using a 5 L plexiglass sampler. For measurements of chlorophyll a (Chl a) contents, 1 L water samples were filtered using GF/F filters (0.70 µm, Whatman), and Chl a was then extracted with 90 % acetone over 24 h and measured spectrophotometrically. Nutrients, including ammonia (NH), nitrite (NO), silicate (SiO), and phosphate (PO) were measured spectrophotometrically with a San continuous flow auto-analyser (Skalar, the Netherlands) after filtering following the procedure described by Hansen and Koroleff (1999).
2.2 Polymerase chain reaction (PCR) and Illumina MiSeq
1 L surface water was collected and prefiltered through a 20 µm mesh sieve to remove most of the mesozooplankton and large particles; it was then directly filtered through a 0.2 µm pore size nucleopore membrane filter (Whatman). The filters were frozen at −80 ∘C in CTAB buffer prior to the laboratory experiments. The DNA extraction was performed as described by Luo et al. (2015).
PCR was performed using primers by barcode flanking the hypervariable V4 region of the 18S rRNA gene: 3NDf (Charvet et al., 2012) with the reverse primer V4_euk_R2 (Brate et al., 2010). The PCRs were conducted in 20 µL reactions with 0.2 µM of each primer, 10 ng of template DNA, 1× PCR buffer, and 2.5 U of Pfu DNA Polymerase (Promega, USA). The amplification programme consisted of an initial denaturation step at 95 ∘C for 2 min, followed by 30 cycles of 95 ∘C for 30 s, 55 ∘C for 30 s, and 72 ∘C for 30 s, and a final extension of 72 ∘C for 5 min. The PCR product was extracted from 2 % agarose gel and purified using the AxyPrep DNA Gel Extraction Kit (Axygen Biosciences, Union City, CA, USA) according to the manufacturer's instructions and quantified using a Quantus™ Fluorometer (Promega, USA). Purified amplicons were pooled in equimolar and paired-end sequenced (2×300) on an Illumina MiSeq platform (Illumina, San Diego, USA) according to the standard protocols described by Wefind Biotechnology Co., Ltd. (Wuhan, China).
The raw 18S rRNA gene sequencing reads were demultiplexed, quality-filtered by fastp version 0.20.0 (Chen et al., 2018) and merged by FLASH version 1.2.7 (Magoc and Salzberg, 2011) with the following criteria: (i) the 300 bp reads were truncated at any site receiving an average quality score of <20 over a 50 bp sliding window, the truncated reads shorter than 50 bp were discarded, and reads containing ambiguous characters were also discarded; (ii) only overlapping sequences longer than 10 bp were assembled according to their overlapped sequence. The maximum mismatch ratio of overlap region is 0.2. Reads that could not be assembled were discarded; (iii) samples were distinguished according to the barcode and primers, and the sequence direction was adjusted, exact barcode matching, and maximum 2-nucleotide mismatch in primer matching.
Operational taxonomic units (OTUs) with a 97 % similarity cutoff were clustered using UPARSE version 7.1 (Edgar, 2013), and chimeric sequences were identified and removed. The taxonomy of each representative sequence was analysed by RDP Classifier version 2.2 (Wang et al., 2007) against the 18S rRNA database (Silva v138) (Quast et al., 2013) using a confidence threshold of 0.7.
The analysis of the OTUs obtained using the UPARSE clustering method have been widely used for high-throughput sequencing analysis (Gad et al., 2022; Xu et al., 2022; Zhang et al., 2022; Reboul et al., 2021; Sun et al., 2022). Some of the diversity indices in our study were more comparable to previous research using 97 % sequence similarity OTUs (Chen et al., 2022; Wang et al., 2021; Y. Wang et al., 2020), as comparisons of these statistics using the same bioinformatics tool seem to remain persuasive (Li et al., 2019; Glassman and Martiny, 2018).
A total of 726 700 valid sequences of the 18S rRNA gene in all samples were obtained, and the average length of the acquired reads was 443 base pairs. The OTUs, classified as metazoa, unassigned and unclassified sequences, were filtered based on taxonomic metadata. Finally, the sequences were normalized at the lowest sequences depth and rarefied at 16 717 reads, yielding a total of 520 OTUs, distributed among 28 phyla. Good's coverage values were above 99.9 %, confirming that the libraries could represent most species in these lakes, with rarefaction curves reaching saturation (Fig. S1 in the Supplement).
2.3 Community composition and diversity
The richness and Shannon index (H) were measured using the “vegan” R package based on the OTUs table, respectively. The nearest-taxon index (NTI) was used to measure the degree of phylogenetic clustering of the taxa on a within-community scale for communities. High or positive values indicated clustering taxa across the overall phylogeny, while lower negative values indicated an overdispersion of taxa across the phylogeny (Horner-Devine and Bohannan, 2006). The NTI quantifies the number of standard deviations that the observed mean nearest taxon distance (MNTD) is from the mean of the null distribution with 999 randomizations determined in the “picante” R package.
The non-metric multidimensional scaling (NMDS) of the microbial eukaryotic communities was performed using the relative abundance of OTUs (Roberts, 2013). An analysis of similarity (ANOSIM) investigated differences in the microbial eukaryotic communities between the groups. The unweighted pair–group method with arithmetic means (UPGMA) was used to determine the similarity between the samples using clustering analysis according to community composition similarity. These analyses were performed in the “vegan” and “phangorn” R packages. All calculations were based on similarity matrices (1-dissimilarity of the Bray–Curtis distance metric).
2.4 Influencing factors of the community structure
The Canoco 4.5 software (ter Braak and Smilauer, 2002) was used to rank species and environmental factor data, and the ranking model was determined by the de-trending correspondence analysis (DCA) of OTUs data. All environmental factors, except pH, were log (x+1) transformed prior to analysis to improve normality and homoscedasticity. To reduce multicollinearity among the environmental factors, all variance inflation factors (VIFs) were kept below 10. The environmental factors influencing the composition of the microbial eukaryotic community were selected by 999 Monte Carlo permutation tests at the significant level (P<0.05). In addition, the relative importance of water temperature, physicochemical factors, and nutrients was assessed using the variation partitioning analysis (VPA).
2.5 Co-occurrence network analysis
We constructed one co-occurrence network based on the samples acquired over the whole study period. To reduce the complexity of the data sets, OTUs occurring in at least five samples were retained to construct the network. Only robust () and statistically significant (P<0.05) correlations were incorporated into the network analyses. Finally, the network was visualized by Gephi software (Bastian et al., 2009).
2.6 Ecological community assembly analysis
The neutral community model (NCM) was used to measure the potential role of stochastic processes in the assembly of microbial eukaryotic communities based on the relationship between the OTUs frequency and their relative abundance (Chen et al., 2019), which was derived from neutral theory (Zhou et al., 2014). The parameter Nm represents the metacommunity size, and R2 represents the degree of fit to a neutral community model.
The Stegen null model was used to further evaluate the contributions of deterministic and stochastic processes to community assembly (Stegen et al., 2012). The β-nearest taxon index (βNTI) was calculated using phylogenetic distance and OTU abundance (Stegen et al., 2013; Webb et al., 2002). The relative contributions of variable selection and homogeneous selection were estimated from the percentage of pairwise comparisons whose βNTI were >2 and , respectively. We further calculated the Bray-Curtis-based Raup-Crick index (RCbray) to investigate the pairwise comparisons that deviated from the selection (Evans et al., 2017; Stegen et al., 2013). When integrated with the value of the underlying community assembly processes could represent a homogenizing dispersal ( and RC), dispersal limitation ( and RC), and undominated processes (i.e. weak selection, weak dispersal, diversification, and drift processes) with and . The null community of all the samples was randomized 999 times to obtain the average null expectations.
3.1 Physicochemical properties
The water temperature ranged from 0.90 to 7.14 ∘C, and the water temperature in YO was significantly higher than in the other lakes (Table S1 in the Supplement, P<0.05). Nutrients in the lakes sampled were in general quite low in concentration, with values of 0.00–0.15 µM (NO), 0.05–0.74 µM (NH), and 0.02–2.29 µM (PO). Relatively higher and lower levels of nutrients were identified in YY and XH, respectively. The lowest value of SiO was recorded in YY (1.43 µM) and the highest in CH (51.5 µM). The highest and lowest Chl a were reached in YY and CH (2.11 and 0.25 µg L−1, respectively). The pH showed minimum and maximum values in YY (7.65) and CH (8.27). Salinity values ranged from 0.00–0.14 PSU and were significantly lower in YO than in the other lakes (P<0.05).
3.2 Diversity and composition of microbial eukaryotic communities
A total of nine dominant phyla were identified, accounting for over 98 % of the sequences. These dominant phyla mainly consisted of microbial eukaryotic phytoplankton, with various relative abundances between different lakes (Fig. 2a). Chrysophyta (34.91 % in CH–78.88 % in XH), Chlorophyta (15.17 % in KT–35.88 % in YY), and Cryptophyta (0.01 % in YO–23.71 % in CH) were the most abundant in the lakes studied. In KT, Cryptophyta was significantly more abundant than in XH and YO, and in XH, Pyrrophyta was significantly more abundant than in YY (P<0.05) (Fig. 2b). Haptophyta represented 0.09 % of the sequence in KT and up to 4.93 % in YO; Alveolates represented 0.02 % of the sequence in XH and up to 1.00 % in CH, while unclassified Stramenopiles-Alveolates-Rhizaria (SAR) represented 1.12 % in XH and 8.05 % in YO.
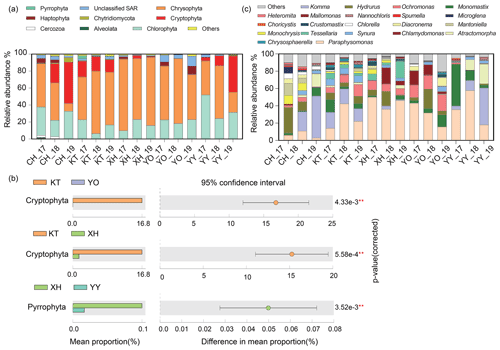
Figure 2Comparison of microbial eukaryotic community composition. (a) Temporal and spatial dynamics of relative abundance on Phylum level in five lakes from 2017 to 2019. The 17, 18, and 19 represent the expedition seasons 2017/2018, 2018/2019, and 2019/2020, respectively. (b) Differential analysis of microbial eukaryotes in different lakes. The lakes that showed no significant differences are not shown (P>0.05). (c) Temporal and spatial dynamics of relative abundance on the genus level in the five lakes studied. (Note: P<0.01, * P<0.05.) (Chlorophyta: Atractomorpha, Chlamydomonas, Mantoniella, Chlorella, Choricystis, Crustomastix, Microglena, Monomastix, Nannochloris; Chrysophyta: Chrysosphaerell, Hydrurus, Mallomonas, Monochrysis, Ochromonas, Paraphysomonas, Spumella, Synura, Tessellaria; Cryptophyta: Komma; Haptophyta: Diacronema; Cercozoa: Heteromita. Any lake where the relative abundance was less than 1 % is defined as others.)
The relative abundances of the dominant taxa in the same lake exhibited some interannual variations. The relative abundances of Chrysophyta in the CH_19, YO_19, and YY_19 samples were lower than in the other samples, while the relative abundances of Cryptophyta in CH_19 and YY_19 were lower than in the other samples of CH and YY, respectively (Fig. 2a).
A total of 21 dominant genera were identified (Fig. 2c), accounting for over 87 % of the sequences. The dominant genera included Hydrurus, Paraphysomonas, Ochromonas, Synura, and Monochrysis belonging to Chrysophyta, Komma in Cryptophyta, Monomastix, Chlamydomonas, and Mantoniella belonging to Chlorophyta.
As shown in Fig. 2c, the abundance of the dominant genera differed interannually among the investigated lakes. The relative abundance of Komma varied from 0 % to 48.52 %, which showed an increasing trend over the years in CH and YY. The ranges of Paraphysomonas and Ochromonas were 3.06 %–56.88 % and 0.06 %–18.86 %, showing an increase followed by a decrease in XH over the years. The highest relative abundances of Hydrurus were in samples XH_18 and YO_18 compared to other samples. Ochromonas in CH was significantly lower relative abundance than in YO and XH (P<0.05, Table S2).
The richness, Shannon index, and NTI exhibited interannual variations but showed no significance (P>0.05) (Fig. 3a, c, e). The order of NTI and Shannon index values all showed 2018 > 2017 > 2019; species richness was highest in 2017 and lowest in 2018. The ranges of richness and Shannon index were 113–268 and 1.70–3.50, respectively, with YY having the lowest values, which were significantly lower than in KT (Fig. 3b, d, P<0.05). The range of NTI was 0.62–1.56, with the lowest value recorded in YO, which was significantly lower than in KT (Fig. 3f, P<0.05). KT had the highest Shannon index, richness, and NTI, and CH also exhibited a relatively higher richness value (Fig. 3b, d, f).
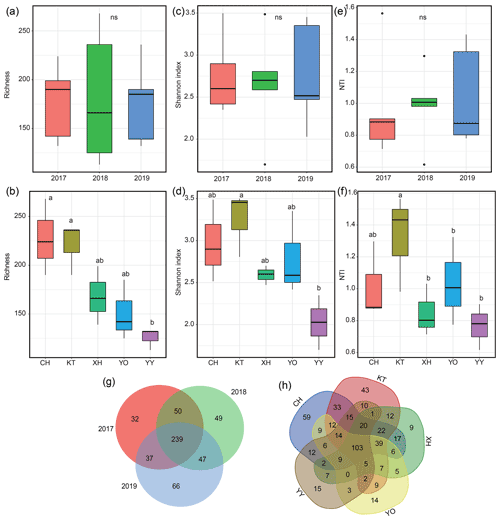
Figure 3Microbial diversity and Venn diagram for different years and lakes. (a, b) Variations in the number of microbial operational taxonomic units (OTUs); (c, d) variations in the microbial Shannon index; (e, f) variations in the within-community nearest-taxon index (NTI); (g, h) Venn diagram showing the unique and shared OTUs. Homogeneity and one-way ANOVA analyses of variance were used to test the significance of the indices; “ns” represents no significant differences (P>0.05); significant differences (P<0.05) are indicated by different letters between lakes, and lakes containing the same letters showed no significant difference (P>0.05).
The total number of OTUs shared between 2017–2019 was 239, and 32, 49, and 66 unique OTUs were identified for 2017, 2018, and 2019, respectively (Fig. 3g). The total number of OTUs shared by the five lakes was 103, and 59 (CH), 43 (KT), 9 (XH), 14 (YO), and 15 (YY) unique OTUs were distinguished among the lakes (Fig. 3h).
The NMDS results divided the samples into five clusters according to their similarity of microbial eukaryotic community (stress value = 0.12) (Fig. 4a). In addition, the ANOSIM based on Bray-Curtis distance indicated that the differences between the lakes were significant (Global R=0.70, P<0.01). Meanwhile, no significant differences were detected by ANOSIM among the interannual variation (R=0.013, P=0.393).
The UPGMA clustering analysis (Fig. 4b) showed the same lakes in a different year, such as CH_17 and CH_18, YY_17 and YY_18, YO_17 and YO_18 clustered together. YO lake was distant from the other lakes and clustered into a separate group.
3.3 Driving factors and co-occurrence patterns
Canonical correspondence analysis (CCA) demonstrated that the first two sequencing axes explained 21.84 % and 17.11 % of the community variation, respectively (Fig. 5a). The samples from the same lake were closer, with a more similar community structure. More importantly, Monte Carlo analysis confirmed that the water temperature and PO significantly affected the microbial eukaryotic communities (P<0.05). The VPA indicated that the monitored environmental factors explained 9.34 % of the microbial eukaryotic community variability among lakes, but there was still a large amount of unexplained variation (90.66 %, Fig. 5b).
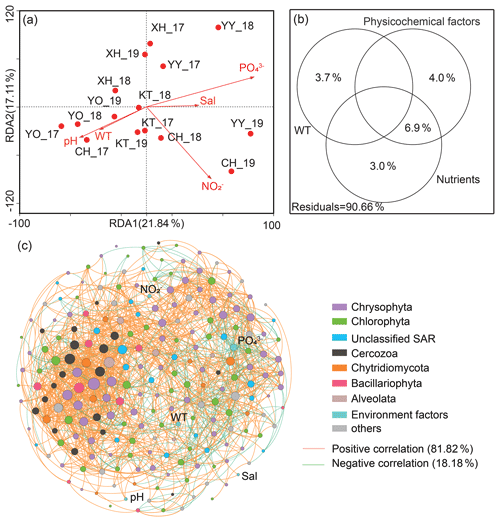
Figure 5Effect of environmental variables on microbial eukaryotic communities and co-occurrence pattern. (a) Canonical correlation analysis plots and (b) variance partitioning analysis. Sal: salinity; WT: water temperature; NO: nitrite nitrogen; PO: phosphate. (c) Network analysis revealing the interspecies associations between microbial eukaryotic operational taxonomic units (OTUs) and the correlation between environmental factors and OTUs in the lakes' integrated networks. The size of each OTUs or environmental factor (node) is proportional to the degree centrality. Others: other phyla and unclassified taxa.
A total of 220 nodes linked by 1521 edges comprised the microbial eukaryotic community network. The majority of nodes in the network had many connections. Notably, the positive associations among species were predominant in the network (Fig. 5c), comprising 81.82 % of the associations, whereas the portion of negative association was only 18.18 %. In addition, the positive interactions were mainly found within the same taxonomic affiliations, such as Chrysophyta, or between a few different taxonomic affiliations, such as Chrysophyta and Chlorophyta. The negative correlations, on the other hand, were mainly reflected between Chrysophyta and Chlorophyta. Only around 8 % of OTUs correlated directly with environmental factors (P<0.05). Meanwhile, only two of the top 20 OTUs with the highest degree of centrality were directly associated with environmental factors (water temperature and PO); one belonged to Chrysophyta and the other to Chytridiomycota.
3.4 Community assembly processes
The Sloan neutral community model (NCM) showed the importance of neutral processes for microbial eukaryotic communities (Fig. 6a), with the neutral processes explaining 55.5 % of the community variation. In addition, the NCM classified microbial eukaryotic taxa into three groups (above prediction, below prediction, and neutral prediction). In addition, compared to the above and below prediction groups, we observed a higher contribution of neutral prediction group to community abundance (86.4 %) and richness (80 %), which was dominated by Chrysophyta, Chlorophyta, and Cryptophyta (Fig. 6b, c). The above prediction group accounted for 10.58 % of the microbial eukaryotic richness but corresponded to only 0.96 % of the abundance and was dominated by Chrysophyta, Chlorophyta, and Cercozoa. Cryptophyta accounted for 16.58 % of the abundance in the neutral prediction group but was almost absent in the above prediction group. In contrast, Cercozoa was present with a 9.38 % abundance in the above prediction group but only comprised 0.32 % of the neutral prediction group.
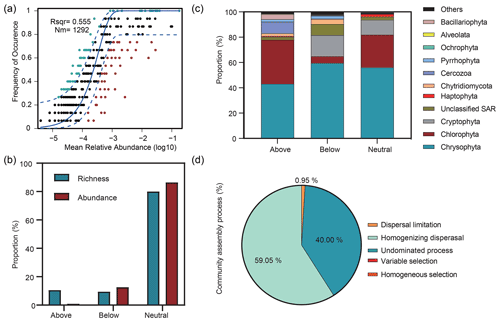
Figure 6Relative influences of deterministic and stochastic processes on microbial eukaryotic community assembly based on the neutral community model (NCM) and the null model. (a) Fit of the NCM of community assembly. Nm indicates the metacommunity size, and R2 indicates the fit to the NCM. Neutral prediction was within a 95 % confidence interval (black), while non-neutral processes included the above and below prediction (dark green and red). (b) Proportions of the richness and abundance of the three groups (above prediction, below prediction, and neutral prediction) based on the NCM. (c) Abundance composition of the three groups in the microbial eukaryotic community. (d) Null model analysis revealing the fraction of ecological processes. The percent of community assembly is governed primarily by various deterministic processes, such as homogeneous and variable selections, and stochastic processes, including dispersal limitations, homogenizing dispersal, and undominated processes (i.e. weak selection, weak dispersal, diversification, and drift processes).
The βNTI was mainly distributed in the region of stochastic processes with a mean value of −0.48 and supported the results of the neutral model. The analysis of community assembly processes showed that stochastic rather than deterministic processes controlled the community assembly. Among them, homogenizing dispersal dominated, with a proportion of 59.05 %, followed by undominated processes and dispersal limitation (Fig. 6d).
4.1 Dominant taxa and diversity
The environmental conditions (e.g. low light and low nutrient contents, etc.) in Antarctic freshwater lakes differ from those of temperate lakes. These special features and their relative isolation has resulted in the development of unique communities and survival strategies of the species adapted to such conditions (Pearce and Galand, 2008). In our study, the survival of taxa (Chrysophyta, Chlorophyta, and Cryptophyta) might have depended on their survival strategies and adaptation to harsh conditions, which allowed them to become the predominant species.
Chrysophyta dominated in the five lakes examined in our study, including Hydrurus, Paraphysomonas, Ochromonas, and Monochrysis. First, their dominance might have been due to their adaptation to a low nutrient availability. Chrysophyta have been widely distributed in oligo- and mesotrophic lakes from both the maritime and continental regions of Antarctica (Allende, 2009; Allende and Izaguirre, 2003; Izaguirre et al., 2020; Richards et al., 2005). Secondly, Chrysophyta can still retained a high cell density under low light conditions, since they can adapt to changing light conditions (Yubuki et al., 2008). Furthermore, Chrysophyta is mixotrophic and can even swim, allowing them to obtain available nutrients from other microorganisms and, thus, reducing the need for dissolved nutrients in the water (Pick and Lean, 1984; Katechakis and Stibor, 2006). In addition, when environmental conditions change dramatically, such as through freezing and nutrient changes, Chrysophyta can form cysts (Nicholls, 1995), protecting their cells from resisting an unsuitable environment. All these characteristics allow Chrysophyta to be the predominant species in the five Antarctic lakes.
Chlorophyta was the second most dominant taxon in our study (15.17 %–35.88 %), mainly consisting of the Monomastix, Chlamydomonas, and Mantoniella. Chlorophyta was typically represented by flagellated species such as Chlamydomonas spp., which dominate phytoplankton communities in different trophic statuses and respond to adverse environmental conditions by forming temporary, non-swimming cell populations encased in a gelatinous mother cell membrane (Izaguirre et al., 2003; Allende and Mataloni, 2013; Toro et al., 2007). Several unicellular algae can undergo mix acid fermentation, and some obligate photoautotrophic species can increase their light capture efficiency through photoadaptation processes involving chlorophyll accumulation (Morgan-Kiss et al., 2016; Atteia et al., 2013). These characteristics might partially explain how Chlorophyta survived and occupied a specific advantage in the lakes studied here.
Cryptophyta was the third most abundant taxon observed. Indeed, their dominance has been interpreted as evidence of heterotrophic growth in winter and mixotrophic growth throughout the year (Unrein et al., 2014). Cryptophyta dominated under perennially ice-covered and coastal saline lakes in continental Antarctica (Izaguirre et al., 2020). The ingestion of bacteria by mixotrophic Cryptophyta has previously been observed in two perennially ice-covered lakes (Fryxell and Hoare) in the McMurdo Dry Valleys (Roberts and Laybourn-Parry, 1999).
Compared to other aquatic ecosystems (Wang et al., 2021; Y. Wang et al., 2020; Hernandez-Ruiz et al., 2018), the diversity of microbial eukaryotes in the Antarctic lakes studied here was significantly lower (Shannon index 1.70–3.50, richness 113–268). The isolation and harsh conditions, especially the lower temperatures and nutrient availability, prevailing in Antarctic lakes accounted for a low microbial eukaryotic diversity. In addition, the species–area relationships model (SAR) states that there should be an increased species number as habitat area increases within a specific area (Ma, 2018). An increase in ice-free area may drastically modify the biodiversity of an area (Pertierra et al., 2017; Duffy et al., 2017; Lee et al., 2017). Our results support the SAR model since a greater alpha diversity was observed in the CH and KT, where the habitat areas were much larger than the YY and YO.
4.2 Influence of environmental factors on the community
Previous studies have demonstrated that abiotic factors affect microbial diversity and community composition (Quiroga et al., 2013; Sun et al., 2017). In this study, we found that water temperature and PO were significant factors driving community changes among the abiotic factors analysed. Some microorganisms have evolved to grow under defined temperatures, allowing differences in the temperature adaptation of different species (Wilkins et al., 2013). Water temperature has become a major driving factor for microbial eukaryotic community variation since it can regulate cellular activity and metabolic rates (Margesin and Miteva, 2011). The retreat of glaciers due to global warming has the risk of reducing the abundance and diversity of microorganisms, and more attention should be paid to the impact of temperature changes on the community structure (Garcia-Rodriguez et al., 2021). The water temperature in the YO was significantly higher than in the other lakes, and the community of YO clustered into a separate group (Fig. 5b). Microorganisms are highly sensitive to nutrients, and indeed, nutrients have been shown to represent important factors of community differences in microbial eukaryote communities in different areas (Zouari et al., 2018). In addition, PO has been demonstrated to be one of the environmental variables that “best” explained the picoeukaryotic distributions in the Western Pacific Ocean (Liu et al., 2021).
Nevertheless, a small amount of community variation could be explained by the environmental variables measured in our analysis. Our results indicate that these environmental factors played a minor role in shaping the microbial eukaryotic community structures. A substantial amount of variation remained unaccounted for (Fig. 5), which could be due to several reasons. First, it is impossible to measure all the environmental factors that could influence microbial communities, and, thus, some significant driving factors may not have been included in our study (W. Wang et al., 2020). Potentially vital abiotic factors in Antarctic lakes may include the solar cycle, light availability, ice cover (thickness and duration), physical mixing of snow melt, and other hydrological processes (Allende and Izaguirre, 2003; Lizotte, 2008). Secondly, the relationships between the microorganisms considered here could not be quantified, and these relationships could represent potentially essential factors shaping the community structures in the lakes studied. For example, predation pressure can manifest as a top-down control of microbial eukaryotes (Blomqvist, 1997). Finally, stochastic processes such as ecological drift (birth, death) might also cause unexplained community variation (Zhang et al., 2018).
4.3 Co-occurrence patterns
Network analysis can help us understand complex biological interactions and ecological rules for community assembly within a specific ecological niche (Li and Hu, 2021; Lupatini et al., 2014). Microorganisms can form various ecological relationships, ranging from mutualism to competition, ultimately shaping the microbial abundances (Faust and Raes, 2012). Positive associations in a network often indicate common preferred environmental conditions or niche-overlapping, whereas negative associations imply competition or niche division (Faust and Raes, 2012). Our network analysis revealed that positive correlations were much more common (82 %) than negative ones (18 %). These results suggested that positive relationships (e.g. due to cross-feeding, niche overlap, mutualism, or commensalism) might play a more important role in the lake ecosystems of Antarctica than negative relationships (e.g. predator–prey, host–parasite, or competition) (Chen and Wen, 2021). Similar results have been obtained through the study of small planktonic eukaryotes (0.2–20 µm) inhabiting the surface waters of a coastal upwelling system (Hernandez-Ruiz et al., 2018). Notwithstanding, further studies are needed to corroborate the biological interactions and other nonrandom processes (for example, cross-feeding versus niche overlap) between the species pairs detected by network analyses.
4.4 Community assembly processes
In general, deterministic and stochastic processes exist simultaneously in a community assembly (He et al., 2021; Chase, 2010). Several factors, such as habitat connectivity and size (Orrock and Watling, 2010), productivity (Chase, 2010), disturbance (Liang et al., 2020), predation (Chase et al., 2009), and resource availability (Kardol et al., 2013), can influence the relative importance of stochastic and deterministic processes in a community assembly. The importance of stochastic processes has been illustrated for other microbial eukaryotic communities in aquatic ecosystems (Chen et al., 2019; W. Wang et al., 2020). For example, picoeukaryotic communities in the lower oxygen layer of the Bengal Bay were shown to be dominated by stochastic processes (Chen et al., 2022). Results from our study supported a more prominent role of stochastic processes over deterministic ones in shaping the assembly of microbial eukaryotic communities. Hence, environmental variables explained only a small amount of variation in the microbial eukaryotic community studied here, and a small number of taxa were significantly correlated with environmental factors.
In our study, the microbial eukaryotic community showed a good fit with the neutral model (Fig. 6a), suggesting that community variation could be explained by stochastic processes such as birth, death, and migration to a large extent. The NCM can also attribute the patterns observed of community assembly to different population behaviour (Zhang et al., 2021). Here, the NCM separated the studied taxa into three groups that had different community structures (Fig. 6), indicating that these taxa might differ in their adaptability to the environment or their dispersal rates (Chen et al., 2019). In addition, in our study, the neutral prediction group contributed a high proportion to both the abundance (86.4 %) and the richness (80.0 %) of the species. Previous studies have shown that the neutral prediction group also dominated the microbial eukaryotic community in a subtropical river in terms of richness and abundance (Chen et al., 2019). Similar results in this study suggest that microbial eukaryotes studied here were more susceptible to stochastic processes.
The importance of stochastic processes has been illustrated by the microbial eukaryotic communities of lakes in East Antarctica (Logares et al., 2018). Antarctic freshwater lakes can receive external microbial colonies from the input of microorganisms from the surrounding ice melt, atmospheric transport, human activities, or bird migration (Unrein et al., 2005). Zeng et al. (2019) showed that homogenizing dispersal occupied a high proportion of community assembly processes in freshwater lakes, and similar results were obtained in our study. Most microorganisms detected in the sea have also been found in lakes in East Antarctica, suggesting that some marine taxa in the lakes could be the product of homogenizing dispersal from the sea to the lakes (Logares et al., 2018). In addition, the five lakes studied were covered in ice for most of the year, and there were long geographical distances between the lakes, resulting in the limited dispersal of microorganisms (0.95 %). Undominant processes accounted for 40 % of the community assembly in our study, including ecological drift and other complex processes that have not been fully quantified, such as weak selection and diffusion (Mo et al., 2018). This suggests that microbial eukaryotic communities might be formed by some highly complex assembly mechanisms in Antarctic freshwater lakes.
In conclusion, a unique microbial eukaryotic community structure and low alpha diversity (richness and Shannon index) have been demonstrated in five freshwater lakes of the Fildes Peninsula, Antarctica. Water temperature and PO were identified as important factors driving the variation of community structures (P<0.05). Stochastic processes and biotic co-occurrence patterns were shown to be important in shaping microbial eukaryotic communities in the area. Our study provides a better understanding of the dynamic patterns and ecological assembly processes of microbial eukaryotic communities in Antarctic oligotrophic lakes (Fildes Peninsula).
The raw 18S reads have been deposited into the NCBI Sequence Read Archive database with the accession numbers PRJNA805287.
The supplement related to this article is available online at: https://doi.org/10.5194/bg-19-4639-2022-supplement.
CZ, YB, and WL designed and conceptualized the study. CZ analysed data and wrote the manuscript. YB and WL reviewed and edited this manuscript. HL performed molecular biology analysis. YZ and HD collected the samples, and the physicochemical variables of the samples measured by BW, YL, and ZJ.
The contact author has declared that none of the authors has any competing interests.
Publisher's note: Copernicus Publications remains neutral with regard to jurisdictional claims in published maps and institutional affiliations.
We gratefully acknowledge to the 34th, 35th, and 36th Chinese Antarctic Research Expedition (CHINARE) and the Antarctic Great Wall Ecology National Observation and Research Station (PRIC) for their strong logistic support of this field survey in the summer season of 2017/2018, 2018/2019, and 2019/2020. The field samples were approved by the Chinese Arctic and Antarctic Administration (CAA).
This research has been supported by the National Natural Science Foundation of China (grant nos. 91851201 and 31971477) and the Open Fund from Hubei Key Laboratory of Three Gorges Project for Conservation of Fishes (SXSN/4194).
This paper was edited by Andrew Thurber and reviewed by Shoubing Wang and one anonymous referee.
Allende, L.: Combined effects of nutrients and grazers on bacterioplankton and phytoplankton abundance in an Antarctic lake with even food-chain links, Polar Biol., 32, 493–501, https://doi.org/10.1007/s00300-008-0545-6, 2009.
Allende, L. and Izaguirre, I.: The role of physical stability on the establishment of steady states in the phytoplankton community of two Maritime Antarctic lakes, Hydrobiologia, 502, 211–224, https://doi.org/10.1023/B:HYDR.0000004283.11230.4a, 2003.
Allende, L. and Mataloni, G.: Short-term analysis of the phytoplankton structure and dynamics in two ponds with distinct trophic states from Cierva Point (maritime Antarctica), Polar Biol., 36, 629–644, https://doi.org/10.1007/s00300-013-1290-z, 2013.
Atteia, A., van Lis, R., Tielens, A. G. M., and Martin, W. F.: Anaerobic energy metabolism in unicellular photosynthetic eukaryotes, BBA-Bioenergetics, 1827, 210–223, https://doi.org/10.1016/j.bbabio.2012.08.002, 2013.
Bahram, M., Kohout, P., Anslan, S., Harend, H., Abarenkov, K., and Tedersoo, L.: Stochastic distribution of small soil eukaryotes resulting from high dispersal and drift in a local environment, ISME J., 10, 885–896, https://doi.org/10.1038/ismej.2015.164, 2016.
Barsch, D. and Caine, N.: The nature of mountain geomorphology, Mt. Res. Dev., 4, 287–298, https://doi.org/10.2307/3673231, 1984.
Bastian, M., Heymann, S., and Jacomy, M.: Gephi: an open source software for exploring and manipulating networks, Int. Conf. Weblogs Soc. media., 3, 361–362, https://ojs.aaai.org/index.php/ICWSM/article/view/13937 (last access: 26 September 2022), 2009.
Blomqvist, P.: Early summer phytoplankton responses to experimental manipulations of grazing and nutrients in unlimed and limed Lake Njupfatet, central Sweden, Arch. Hydrobiol., 140, 321–346, https://doi.org/10.1127/archiv-hydrobiol/137/1996/425, 1997.
Bouchez, T., Blieux, A. L., Dequiedt, S., Domaizon, I., Dufresne, A., Ferreira, S., Godon, J. J., Hellal, J., Joulian, C., Quaiser, A., Martin-Laurent, F., Mauffret, A., Monier, J. M., Peyret, P., Schmitt-Koplin, P., Sibourg, O., D'oiron, E., Bispo, A., Deportes, I., Grand, C., Cuny, P., Maron, P. A., and Ranjard, L.: Molecular microbiology methods for environmental diagnosis, Environ. Chem. Lett., 14, 423–441, https://doi.org/10.1007/s10311-016-0581-3, 2016.
Brate, J., Logares, R., Berney, C., Ree, D. K., Klaveness, D., Jakobsen, K. S., and Shalchian-Tabrizi, K.: Freshwater Perkinsea and marine-freshwater colonizations revealed by pyrosequencing and phylogeny of environmental rDNA, ISME J., 4, 1144–1153, https://doi.org/10.1038/ismej.2010.39, 2010.
Charvet, S., Vincent, W. F., and Lovejoy, C.: Chrysophytes and other protists in High Arctic lakes: molecular gene surveys, pigment signatures and microscopy, Polar Biol., 35, 733–748, https://doi.org/10.1007/s00300-011-1118-7, 2012.
Chase, J. M.: Stochastic community assembly causes higher biodiversity in more productive environments, Science, 328, 1388–1391, https://doi.org/10.1126/science.1187820, 2010.
Chase, J. M., Biro, E. G., Ryberg, W. A., and Smith, K. G.: Predators temper the relative importance of stochastic processes in the assembly of prey metacommunities, Ecol. Lett., 12, 1210–1218, https://doi.org/10.1111/j.1461-0248.2009.01362.x, 2009.
Chen, S. F., Zhou, Y. Q., Chen, Y. R., and Gu, J.: fastp: an ultra-fast all-in-one FASTQ preprocessor, Bioinformatics, 34, 884–890, https://doi.org/10.1093/bioinformatics/bty560, 2018.
Chen, W., Ren, K., Isabwe, A., Chen, H., Liu, M., and Yang, J.: Stochastic processes shape microeukaryotic community assembly in a subtropical river across wet and dry seasons, Microbiome, 7, 138, https://doi.org/10.1186/s40168-019-0749-8, 2019.
Chen, W. D. and Wen, D. H.: Archaeal and bacterial communities assembly and co-occurrence networks in subtropical mangrove sediments under Spartina alterniflora invasion, Environ. Microbiol., 16, 10, https://doi.org/10.1186/s40793-021-00377-y, 2021.
Chen, Z., Gu, T., Wang, X., Wu, X., and Sun, J.: Oxygen gradients shape the unique structure of picoeukaryotic communities in the Bay of Bengal, Sci. Total. Environ., 814, 152862, https://doi.org/10.1016/j.scitotenv.2021.152862, 2022.
Comeau, A. M., Harding, T., Galand, P. E., Vincent, W. F., and Lovejoy, C.: Vertical distribution of microbial communities in a perennially stratified Arctic lake with saline, anoxic bottom waters, Sci. Rep.-UK, 2, 604, https://doi.org/10.1038/srep00604, 2012.
Duffy, G. A., Coetzee, B. W. T., Latombe, G., Akerman, A. H., McGeoch, M. A., and Chown, S. L.: Barriers to globally invasive species are weakening across the Antarctic, Divers. Distrib., 23, 982–996, https://doi.org/10.1111/ddi.12593, 2017.
Edgar, R. C.: UPARSE: highly accurate OTU sequences from microbial amplicon reads, Nat. Methods., 10, 996–998, https://doi.org/10.1038/Nmeth.2604, 2013.
Evans, S., Martiny, J. B., and Allison, S. D.: Effects of dispersal and selection on stochastic assembly in microbial communities, ISME J., 11, 176–185, https://doi.org/10.1038/ismej.2016.96, 2017.
Faust, K. and Raes, J.: Microbial interactions: from networks to models, Nat. Rev. Microbiol., 10, 538–550, https://doi.org/10.1038/nrmicro2832, 2012.
Gad, M., Hou, L., Cao, M., Adyari, B., Zhang, L., Qin, D., Yu, C. P., Sun, Q., and Hu, A.: Tracking microeukaryotic footprint in a peri-urban watershed, China through machine-learning approaches, Sci. Total Environ., 806, 150401, https://doi.org/10.1016/j.scitotenv.2021.150401, 2022.
Garcia-Rodriguez, F., Piccini, C., Carrizo, D., Sanchez-Garcia, L., Perez, L., Crisci, C., Oaquim, A. B. J., Evangelista, H., Soutullo, A., Azcune, G., and Luning, S.: Centennial glacier retreat increases sedimentation and eutrophication in Subantarctic periglacial lakes: A study case of Lake Uruguay, Sci. Total Environ., 754, 142066, https://doi.org/10.1016/j.scitotenv.2020.142066, 2021.
Glassman, S. I. and Martiny, J. B. H.: Broadscale ecological patterns are robust to use of exact sequence variants versus operational taxonomic units, mSphere, 3, e00148-00118, https://doi.org/10.1128/mSphere.00148-18, 2018.
Grob, C., Ulloa, O., Li, W. K. W., Alarcon, G., Fukasawa, M., and Watanabe, S.: Picoplankton abundance and biomass across the eastern South Pacific Ocean along latitude 32.5∘ S, Mar. Ecol.-Prog. Ser., 332, 53–62, https://doi.org/10.3354/meps332053, 2007.
Hansen, H. P. and Koroleff, F.: Determination of nutrients, Methods Seawater Anal., 23, 159–228, https://doi.org/10.1002/9783527613984.ch10, 1999.
He, Q., Wang, S., Hou, W., Feng, K., Li, F., Hai, W., Zhang, Y., Sun, Y., and Deng, Y.: Temperature and microbial interactions drive the deterministic assembly processes in sediments of hot springs, Sci. Total Environ., 772, 145465, https://doi.org/10.1016/j.scitotenv.2021.145465, 2021.
Hernandez-Ruiz, M., Barber-Lluch, E., Prieto, A., Alvarez-Salgado, X. A., Logares, R., and Teira, E.: Seasonal succession of small planktonic eukaryotes inhabiting surface waters of a coastal upwelling system, Environ. Microbiol., 20, 2955–2973, https://doi.org/10.1111/1462-2920.14313, 2018.
Holdgate, M. W.: Terrestrial ecosystems in Antarctic, Philos. T. Roy. Soc. B, 279, 5–25, https://doi.org/10.1098/rstb.1977.0068, 1977.
Horner-Devine, M. C. and Bohannan, B. J. M.: Phylogenetic clustering and overdispersion in bacterial communities, Ecology, 87, S100–S108, https://doi.org/10.1890/0012-9658(2006)87[100:Pcaoib]2.0.Co;2, 2006.
Izaguirre, I., Allende, L., and Marinone, M. C.: Comparative study of the planktonic communities from lakes of contrasting trophic status at Hope Bay (Antarctic Peninsula), J. Plankton Res., 25, 1079–1097, https://doi.org/10.1093/plankt/25.9.1079, 2003.
Izaguirre, I., Allende, L., and Romina Schiaffino, M.: Phytoplankton in Antarctic lakes: biodiversity and main ecological features, Hydrobiologia, 848, 177–207, https://doi.org/10.1007/s10750-020-04306-x, 2020.
Kardol, P., Souza, L., and Classen, A. T.: Resource availability mediates the importance of priority effects in plant community assembly and ecosystem function, Oikos, 122, 84–94, https://doi.org/10.1111/j.1600-0706.2012.20546.x, 2013.
Karimi, B., Maron, P. A., Chemidlin-Prevost Boure, N., Bernard, N., Gilbert, D., and Ranjard, L.: Microbial diversity and ecological networks as indicators of environmental quality, Environ. Chem. Lett., 15, 265–281, https://doi.org/10.1007/s10311-017-0614-6, 2017.
Katechakis, A. and Stibor, H.: The mixotroph Ochromonas tuberculata may invade and suppress specialist phago- and phototroph plankton communities depending on nutrient conditions, Oecologia, 148, 692–701, https://doi.org/10.1007/s00442-006-0413-4, 2006.
Kawecka, B., Olech, M., Nowogrodzka-Zagorska, M., and Wojtun, B.: Diatom communities in small water bodies at H. Arctowski Polish Antarctic Station (King George Island, South Shetland Islands, Antarctica), Polar Biol., 19, 183–192, https://doi.org/10.1007/s003000050233, 1998.
Kong, J., Wang, Y., Warren, A., Huang, B., and Sun, P.: Diversity distribution and assembly mechanisms of planktonic and benthic microeukaryote communities in intertidal zones of southeast Fujian, China, Front. Microbiol., 10, 2640, https://doi.org/10.3389/fmicb.2019.02640, 2019.
Leblanc, K., Queguiner, B., Diaz, F., Cornet, V., Michel-Rodriguez, M., Durrieu de Madron, X., Bowler, C., Malviya, S., Thyssen, M., Gregori, G., Rembauville, M., Grosso, O., Poulain, J., de Vargas, C., Pujo-Pay, M., and Conan, P.: Nanoplanktonic diatoms are globally overlooked but play a role in spring blooms and carbon export, Nat. Commun., 9, 953, https://doi.org/10.1038/s41467-018-03376-9, 2018.
Lee, J. R., Raymond, B., Bracegirdle, T. J., Chades, I., Fuller, R. A., Shaw, J. D., and Terauds, A.: Climate change drives expansion of Antarctic ice-free habitat, Nature, 547, 49–54, https://doi.org/10.1038/nature22996, 2017.
Li, Y. and Hu, C.: Biogeographical patterns and mechanisms of microbial community assembly that underlie successional biocrusts across northern China, NPJ Biofilms Microbiomes, 7, 15, https://doi.org/10.1038/s41522-021-00188-6, 2021.
Li, Y., Gao, Y., Zhang, W., Wang, C., Wang, P., Niu, L., and Wu, H.: Homogeneous selection dominates the microbial community assembly in the sediment of the Three Gorges Reservoir, Sci. Total Environ., 690, 50–60, https://doi.org/10.1016/j.scitotenv.2019.07.014, 2019.
Liang, Y., Ning, D., Lu, Z., Zhang, N., Hale, L., Wu, L., Clark, I. M., McGrath, S. P., Storkey, J., Hirsch, P. R., Sun, B., and Zhou, J.: Century long fertilization reduces stochasticity controlling grassland microbial community succession, Soil Biol. Biochem., 151, 108023, https://doi.org/10.1016/j.soilbio.2020.108023, 2020.
Liu, K., Liu, Y., Hu, A., Wang, F., Chen, Y., Gu, Z., Anslan, S., and Hou, J.: Different community assembly mechanisms underlie similar biogeography of bacteria and microeukaryotes in Tibetan lakes, FEMS Microbiol. Ecol., 96, fiaa071, https://doi.org/10.1093/femsec/fiaa071, 2020.
Liu, Q., Zhao, Q., Jiang, Y., Li, Y., Zhang, C., Li, X., Yu, X., Huang, L., Wang, M., Yang, G., Chen, H., and Tian, J.: Diversity and co-occurrence networks of picoeukaryotes as a tool for indicating underlying environmental heterogeneity in the Western Pacific Ocean, Mar. Environ. Res., 170, 105376, https://doi.org/10.1016/j.marenvres.2021.105376, 2021.
Lizotte, M. P.: Phytoplankton and primary production, in: Polar Lakes and Rivers: Limnology of Arctic and Antarctic Aquatic Ecosystems, edited by: Vincent, W. F. and Laybourn-Parry, J., Oxford, 9, 157–178, https://doi.org/10.1093/acprof:oso/9780199213887.003.0009, 2008.
Logares, R., Tesson, S. V. M., Canback, B., Pontarp, M., Hedlund, K., and Rengefors, K.: Contrasting prevalence of selection and drift in the community structuring of bacteria and microbial eukaryotes, Environ. Microbiol., 20, 2231–2240, https://doi.org/10.1111/1462-2920.14265, 2018.
Lopez-Garcia, P., Rodriguez-Valera, F., Pedros-Alio, C., and Moreira, D.: Unexpected diversity of small eukaryotes in deep-sea Antarctic plankton, Nature, 409, 603–607, https://doi.org/10.1038/35054537, 2001.
Luo, W., Li, H., Gao, S., Yu, Y., Lin, L., and Zeng, Y.: Molecular diversity of microbial eukaryotes in sea water from Fildes Peninsula, King George Island, Antarctica, Polar Biol., 39, 605–616, https://doi.org/10.1007/s00300-015-1815-8, 2015.
Lupatini, M., Suleiman, A. K. A., Jacques, R. J. S., Antoniolli, Z. I., de Siqueira Ferreira, A. o., Kuramae, E. E., and Roesch, L. F. W.: Network topology reveals high connectance levels and few key microbial genera within soils, Front. Environ. Sci., 2, 10, https://doi.org/10.3389/fenvs.2014.00010, 2014.
Lyons, W. B., Laybourn-Parry, J., Welch, K. A., and Priscu, J. C.: Antarctic lake systems and climate change, in: Trends in Antarctic Terrestrial and Limnetic Ecosystems, edited by: Bergstrom, D. M., Convey, P., and Huiskes, A. H. L., Springer, Dordrecht, 273–295, https://doi.org/10.1007/1-4020-5277-4_13, 2007.
Ma, Z. S.: DAR (diversity-area relationship): Extending classic SAR (species-area relationship) for biodiversity and biogeography analyses, Ecol. Evol., 8, 10023–10038, https://doi.org/10.1002/ece3.4425, 2018.
Magoc, T. and Salzberg, S. L.: FLASH: fast length adjustment of short reads to improve genome assemblies, Bioinformatics, 27, 2957–2963, https://doi.org/10.1093/bioinformatics/btr507, 2011.
Margesin, R. and Miteva, V.: Diversity and ecology of psychrophilic microorganisms, Res. Microbiol., 162, 346–361, https://doi.org/10.1016/j.resmic.2010.12.004, 2011.
Marquardt, M., Vader, A., Stubner, E. I., Reigstad, M., and Gabrielsen, T. M.: Strong Seasonality of Marine Microbial Eukaryotes in a High-Arctic Fjord (Isfjorden, in West Spitsbergen, Norway), Appl. Environ. Microb., 82, 1868–1880, https://doi.org/10.1128/AEM.03208-15, 2016.
Marsh, N. B., Lacelle, D., Faucher, B., Cotroneo, S., Jasperse, L., Clark, I. D., and Andersen, D. T.: Sources of solutes and carbon cycling in perennially ice-covered Lake Untersee, Antarctica, Sci. Rep.-UK, 10, 12290, https://doi.org/10.1038/s41598-020-69116-6, 2020.
Massana, R. and Logares, R.: Eukaryotic versus prokaryotic marine picoplankton ecology, Environ. Microbiol., 15, 1254–1261, https://doi.org/10.1111/1462-2920.12043, 2013.
Massana, R., Gobet, A., Audic, S., Bass, D., Bittner, L., Boutte, C., Chambouvet, A., Christen, R., Claverie, J. M., Decelle, J., Dolan, J. R., Dunthorn, M., Edvardsen, B., Forn, I., Forster, D., Guillou, L., Jaillon, O., Kooistra, W. H. C. F., Logares, R., Mahe, F., Not, F., Ogata, H., Pawlowski, J., Pernice, M. C., Probert, I., Romac, S., Richards, T., Santini, S., Shalchian-Tabrizi, K., Siano, R., Simon, N., Stoeck, T., Vaulot, D., Zingone, A., and de Vargas, C.: Marine protist diversity in European coastal waters and sediments as revealed by high-throughput sequencing, Environ. Microbiol., 17, 4035–4049, https://doi.org/10.1111/1462-2920.12955, 2015.
Mataloni, G., Tesolin, G., Sacullo, F., and Tell, G.: Factors regulating summer phytoplankton in a highly eutrophic Antarctic lake, Hydrobiologia, 432, 65–72, https://doi.org/10.1023/A:1004045219437, 2000.
Mo, Y., Zhang, W., Yang, J., Lin, Y., Yu, Z., and Lin, S.: Biogeographic patterns of abundant and rare bacterioplankton in three subtropical bays resulting from selective and neutral processes, ISME J., 12, 2198–2210, https://doi.org/10.1038/s41396-018-0153-6, 2018.
Morgan-Kiss, R. M., Lizotte, M. P., Kong, W., and Priscu, J. C.: Photoadaptation to the polar night by phytoplankton in a permanently ice-covered Antarctic lake, Limnol. Oceanogr., 61, 3–13, https://doi.org/10.1002/lno.10107, 2016.
Nicholls, K. H.: Chrysophyte blooms in the plankton and neuston of marine and freshwater systems, in: Chrysophyte Algae: Ecology, Phylogeny and Development, edited by: Sandgren, C., Smol, J., and Kristiansen, J., Cambridge University Press, Cambridge, 181–213, https://doi.org/10.1017/CBO9780511752292.010, 1995.
Ofiteru, I. D., Lunn, M., Curtis, T. P., Wells, G. F., Criddle, C. S., Francis, C. A., and Sloan, W. T.: Combined niche and neutral effects in a microbial wastewater treatment community, P. Natl. Acad. Sci. USA, 107, 15345–15350, https://doi.org/10.1073/pnas.1000604107, 2010.
Orrock, J. L. and Watling, J. I.: Local community size mediates ecological drift and competition in metacommunities, P. Roy. Soc. B-Biol. Sci., 277, 2185–2191, https://doi.org/10.1098/rspb.2009.2344, 2010.
Pearce, D. A. and Galand, P. E.: Microbial biodiversity and biogeography, in: Polar lakes and rivers: Limnology of Arctic and Antarctic Aquatic Ecosystems, edited by: Vincent, W. F. and Laybourn-Parry, J., Oxford University Press, Oxford, 213–230, https://doi.org/10.1093/acprof:oso/9780199213887.003.0012, 2008.
Pertierra, L. R., Aragón, P., Shaw, J. D., Bergstrom, D. M., Terauds, A., and Olalla-Tárraga, M. Á.: Global therma niche models of two European grasses show high invasion risks in Antarctica, Glob. Change Biol, 23, 2863–2873, https://doi.org/10.1111/gcb.13596, 2017.
Pick, F. R. and Lean, D.: Diurnal movements of metalimnetic phytoplankton, J. Phycol., 20, 430–436, https://doi.org/10.1111/j.0022-3646.1984.00430.x, 1984.
Powell, J. R., Karunaratne, S., Campbell, C. D., Yao, H., Robinson, L., and Singh, B. K.: Deterministic processes vary during community assembly for ecologically dissimilar taxa, Nat. Commun., 6, 8444, https://doi.org/10.1038/ncomms9444, 2015.
Priscu, J. C.: The biogeochemistry of nitrous oxide in permanently ice-covered lakes of the McMurdo Dry Valleys, Antarctica, Glob. Change Biol., 3, 301–315, https://doi.org/10.1046/j.1365-2486.1997.00147.x, 2010.
Quast, C., Pruesse, E., Yilmaz, P., Gerken, J., Schweer, T., Yarza, P., Peplies, J., and Glöckner, F.: The SILVA ribosomal RNA gene database project: improved data processing and web-based tools, Nucleic Acids Res., 41, 590–596, https://doi.org/10.1093/nar/gks1219, 2013.
Quiroga, M. V., Unrein, F., González Garraza, G., Küppers, G., Lombardo, R., Marinone, M. C., Menu Marque, S., Vinocur, A., and Mataloni, G.: The plankton communities from peat bog pools: structure, temporal variation and environmental factors, J. Plankton Res., 35, 1234–1253, https://doi.org/10.1093/plankt/fbt082, 2013.
Reboul, G., Moreira, D., Annenkova, N. V., Bertolino, P., Vershinin, K. E., and Lopez-Garcia, P.: Marine signature taxa and core microbial community stability along latitudinal and vertical gradients in sediments of the deepest freshwater lake, ISME J., 15, 3412–3417, https://doi.org/10.1038/s41396-021-01011-y, 2021.
Richards, T. A., Vepritskiy, A. A., Gouliamova, D. E., and Nierzwicki-Bauer, S. A.: The molecular diversity of freshwater picoeukaryotes from an oligotrophic lake reveals diverse, distinctive and globally dispersed lineages, Environ. Microbiol., 7, 1413–1425, https://doi.org/10.1111/j.1462-2920.2005.00828.x, 2005.
Roberts, D.: labdsv: Ordination and Multivariate Analysis for Ecology, CRAN [code], https://CRAN.R-project.org/package=labdsv (last access: 26 September 2022), 2013.
Roberts, E. C. and Laybourn-Parry, J.: Mixotrophic cryptophytes and their predators in the Dry Valley lakes of Antarctica, Freshwater Biol., 41, 737–746, https://doi.org/10.1046/j.1365-2427.1999.00401.x, 1999.
Stegen, J. C., Lin, X., Konopka, A. E., and Fredrickson, J. K.: Stochastic and deterministic assembly processes in subsurface microbial communities, ISME J., 6, 1653–1664, https://doi.org/10.1038/ismej.2012.22, 2012.
Stegen, J. C., Lin, X., Fredrickson, J. K., Chen, X., Kennedy, D. W., Murray, C. J., Rockhold, M. L., and Konopka, A.: Quantifying community assembly processes and identifying features that impose them, ISME J., 7, 2069–2079, https://doi.org/10.1038/ismej.2013.93, 2013.
Sun, D., Bi, Q., Li, K., Dai, P., Yu, Y., Zhou, W., Lv, T., Liu, X., Zhu, J., Zhang, Q., Jin, C., Lu, L., and Lin, X.: Significance of temperature and water availability for soil phosphorus transformation and microbial community composition as affected by fertilizer sources, Biol. Fert. Soils, 54, 229–241, https://doi.org/10.1007/s00374-017-1252-7, 2017.
Sun, P., Wang, Y., Huang, X., Huang, B., and Wang, L.: Water masses and their associated temperature and cross-domain biotic factors co-shape upwelling microbial communities, Water Res., 215, 118274, https://doi.org/10.1016/j.watres.2022.118274, 2022.
ter Braak, C. and Smilauer, P.: CANOCO Reference Manual and CanoDraw for Windows User's Guide: Software for Canonical Community Ordination (version 4.5), Microcomputer Power, Ithaca, NY, USA, https://www.canoco.com, https://edepot.wur.nl/405659 (last access: 26 September 2022), 2002.
Toro, M., Camacho, A., Rochera, C., Rico, E., Banon, M., Fernandez-Valiente, E., Marco, E., Justel, A., Avendano, M. C., Ariosa, Y., Vincent, W. F., and Quesada, A.: Limnological characteristics of the freshwater ecosystems of Byers Peninsula, Livingston Island, in maritime Antarctica, Polar Biol., 30, 635–649, https://doi.org/10.1007/s00300-006-0223-5, 2007.
Unrein, F., Izaguirre, I., Massana, R., Balague, V., and Gasol, J. M.: Nanoplankton assemblages in maritime Antarctic lakes: characterisation and molecular fingerprinting comparison, Aquat. Microb. Ecol., 40, 269–282, https://doi.org/10.3354/ame040269, 2005.
Unrein, F., Gasol, J. M., Not, F., Forn, I., and Massana, R.: Mixotrophic haptophytes are key bacterial grazers in oligotrophic coastal waters, ISME J., 8, 164–176, https://doi.org/10.1038/ismej.2013.132, 2014.
Wang, F., Huang, B., Xie, Y., Cai, S., Wang, X., and Mu, J.: Diversity, Composition, and Activities of Nano- and Pico-Eukaryotes in the Northern South China Sea With Influences of Kuroshio Intrusion, Front. Mar. Sci., 8, 658233, https://doi.org/10.3389/fmars.2021.658233, 2021.
Wang, Q., Garrity, G. M., Tiedje, J. M., and Cole, J. R.: Naive Bayesian classifier for rapid assignment of rRNA sequences into the new bacterial taxonomy, Appl. Environ. Microb., 73, 5261–5267, https://doi.org/10.1128/Aem.00062-07, 2007.
Wang, W., Ren, K., Chen, H., Gao, X., Ronn, R., and Yang, J.: Seven-year dynamics of testate amoeba communities driven more by stochastic than deterministic processes in two subtropical reservoirs, Water Res., 185, 116232, https://doi.org/10.1016/j.watres.2020.116232, 2020.
Wang, Y., Li, G., Shi, F., Dong, J., Gentekaki, E., Zou, S., Zhu, P., Zhang, X., and Gong, J.: Taxonomic diversity of pico-/nanoeukaryotes is related to dissolved oxygen and productivity, but functional composition is shaped by limiting nutrients in eutrophic coastal oceans, Front Microbiol., 11, 601037, https://doi.org/10.3389/fmicb.2020.601037, 2020.
Webb, C. O., Ackerly, D. D., McPeek, M. A., and Donoghue, M. J.: Phylogenies and Community Ecology, Annu. Rev. Ecol. Syst., 33, 475–505, https://doi.org/10.1146/annurev.ecolsys.33.010802.150448, 2002.
Wilkins, D., Yau, S., Williams, T. J., Allen, M. A., Brown, M. V., DeMaere, M. Z., Lauro, F. M., and Cavicchioli, R.: Key microbial drivers in Antarctic aquatic environments, FEMS Microbiol. Rev., 37, 303–335, https://doi.org/10.1111/1574-6976.12007, 2013.
Wu, L. W., Ning, D. L., Zhang, B., Li, Y., Zhang, P., Shan, X. Y., Zhang, Q. T., Brown, M. R., Li, Z. X., Van Nostrand, J. D., Ling, F. Q., Xiao, N. J., Zhang, Y., Vierheilig, J., Wells, G. F., Yang, Y. F., Deng, Y., Tu, Q. C., Wang, A. J., Zhang, T., He, Z. L., Keller, J., Nielsen, P. H., Alvarez, P. J. J., Criddle, C. S., Wagner, M., Tiedje, J. M., He, Q., Curtis, T. P., Stahl, D. A., Alvarez-Cohen, L., Rittmann, B. E., Wen, X. H., and Zhou, J. Z.: Global diversity and biogeography of bacterial communities in wastewater treatment plants, Nat. Microbiol., 4, 2579–2579, https://doi.org/10.1038/s41564-019-0617-0, 2019.
Xu, D., Kong, H., Yang, E. J., Wang, Y., Li, X., Sun, P., Jiao, N., Lee, Y., Jung, J., and Cho, K. H.: Spatial dynamics of active microeukaryotes along a latitudinal gradient: Diversity, assembly process, and co-occurrence relationships, Environ. Res., 212, 113234, https://doi.org/10.1016/j.envres.2022.113234, 2022.
Yubuki, N., Nakayama, T., and Inouye, I.: A unique life cycle and perennation in a colorless chrysophyte Spumella sp., J. Phycol., 44, 164–172, https://doi.org/10.1111/j.1529-8817.2007.00441.x, 2008.
Zeng, J., Jiao, C., Zhao, D., Xu, H., Huang, R., Cao, X., Yu, Z., and Wu, Q. L.: Patterns and assembly processes of planktonic and sedimentary bacterial community differ along a trophic gradient in freshwater lakes, Ecol. Indic., 106, 105491, https://doi.org/10.1016/j.ecolind.2019.105491, 2019.
Zhang, L., Yin, W., Wang, C., Zhang, A., Zhang, H., Zhang, T., and Ju, F.: Untangling microbiota diversity and assembly patterns in the world's largest water diversion canal, Water Res., 204, 117617, https://doi.org/10.1016/j.watres.2021.117617, 2021.
Zhang, W., Wan, W., Lin, H., Pan, X., Lin, L., and Yang, Y.: Nitrogen rather than phosphorus driving the biogeographic patterns of abundant bacterial taxa in a eutrophic plateau lake, Sci. Total Environ., 806, 150947, https://doi.org/10.1016/j.scitotenv.2021.150947, 2022.
Zhang, W. J., Pan, Y. B., Yang, J., Chen, H. H., Holohan, B., Vaudrey, J., Lin, S. J., and McManus, G. B.: The diversity and biogeography of abundant and rare intertidal marine microeukaryotes explained by environment and dispersal limitation, Environ. Microbiol., 20, 462–476, https://doi.org/10.1111/1462-2920.13916, 2018.
Zhao, B., Chen, M., Sun, Y., Yang, J., and Chen, F.: Genetic diversity of picoeukaryotes in eight lakes differing in trophic status, Can. J. Microbiol., 57, 115–126, https://doi.org/10.1139/w10-107, 2011.
Zhou, J., Deng, Y., Zhang, P., Xue, K., Liang, Y., Van Nostrand, J. D., Yang, Y., He, Z., Wu, L., Stahl, D. A., Hazen, T. C., Tiedje, J. M., and Arkin, A. P.: Stochasticity, succession, and environmental perturbations in a fluidic ecosystem, P. Natl. Acad. Sci. USA, 111, 836–845, https://doi.org/10.1073/pnas.1324044111, 2014.
Zhu, G. H., Shuji, O., Hu, C. Y., He, J. F., Jin, M., Yu, P. S., and Pan, J. M.: Impact of global climate change on antarctic freshwater algae, China Environ. Sci., 30, 400–404, 2010.
Zouari, A. B., Hassen, M. B., Balague, V., Sahli, E., Ben Kacem, M. Y., Akrout, F., Hamza, A., and Massana, R.: Picoeukaryotic diversity in the Gulf of Gabes: variability patterns and relationships to nutrients and water masses, Aquat. Microb. Ecol., 81, 37–53, https://doi.org/10.3354/ame01857, 2018.