the Creative Commons Attribution 4.0 License.
the Creative Commons Attribution 4.0 License.
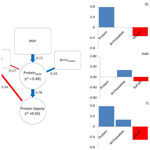
Climate and geology overwrite land use effects on soil organic nitrogen cycling on a continental scale
Lisa Noll
Shasha Zhang
Qing Zheng
Yuntao Hu
Florian Hofhansl
Wolfgang Wanek
Soil fertility and plant productivity are globally constrained by N availability. Proteins are the largest N reservoir in soils, and the cleavage of proteins into small peptides and amino acids has been shown to be the rate-limiting step in the terrestrial N cycle. However, we are still lacking a profound understanding of the environmental controls of this process. Here we show that integrated effects of climate and soil geochemistry drive protein cleavage across large scales. We measured gross protein depolymerization rates in mineral and organic soils sampled across a 4000 km long European transect covering a wide range of climates, geologies and land uses. Based on structural equation models we identified that soil organic N cycling was strongly controlled by substrate availability, e.g., by soil protein content. Soil geochemistry was a secondary predictor, by controlling protein stabilization mechanisms and protein availability. Precipitation was identified as the main climatic control on protein depolymerization, by affecting soil weathering and soil organic matter accumulation. In contrast, land use was a poor predictor of protein depolymerization. Our results highlight the need to consider geology and precipitation effects on soil geochemistry when estimating and predicting soil N cycling at large scales.
- Article
(2521 KB) - Full-text XML
-
Supplement
(1283 KB) - BibTeX
- EndNote
Microbial decomposition of soil organic matter is a fundamental driver of soil ecosystem functions and services. For example, nutrient regeneration through decomposition maintains soil fertility and plant productivity. For example, the extracellular cleavage of plant- and microbial-derived soil proteins, chitin or peptidoglycan to small organic compounds such as peptides, amino acids and amino sugars regulates organic N uptake by soil microbes, contributes to plant N nutrition and further drives terrestrial inorganic N cycling (Hu et al., 2018; Noll et al., 2019b). Proteins account for up to 90 % of soil N (Martens and Loeffelmann, 2003; Schulten and Schnitzer, 1997). Protein depolymerization is mediated by extracellular enzymes and facilitates microbes and plants to utilize the by far single largest N reservoir in soils. However, the large-scale controls of gross protein depolymerization are largely unknown. Since protein depolymerization is mediated by extracellular enzymes, this process is expected to be either enzyme-limited or substrate-limited. Thereby it is expected to be tied to soil geochemistry and vegetation, which affect substrate availability, and to microbial community composition and microbial N demand, which drive enzyme production (Sinsabaugh et al., 2008).
Microbial community structure may influence protein depolymerization through several pathways. Across biogeographic regions peptidase activity increases strongly with soil pH, since the pH optima of most proteolytic enzymes are about 7–8 (Sinsabaugh et al., 2008; Hendriksen et al., 2016). However, soil pH is a major control on bacterial community composition, and cross-continental studies have shown that this pattern is consistent across soil types and biomes (Lauber et al., 2009; Rousk et al., 2010; Fierer and Jackson, 2006). Given the large difference in the excreted enzyme complement between microbial taxa, soil nutrient status and edaphic properties (e.g., soil pH, texture and cation exchange capacity) have been shown to shape the set of excreted proteolytic enzymes (Lauber et al., 2009, 2008; Jangid et al., 2008; Fuka et al., 2008) by their effects on microbial community composition. Effects of climate on peptidase activity are mainly indirect, indicated by shifts in vegetation type and in soil nutrient stoichiometry from low to high latitudes (Hendriksen et al., 2016; Sinsabaugh et al., 2008; Peng and Wang, 2016). Soil C : N ratios are typically higher in forest soils than in agricultural soils and affect in particular the fungi : bacteria ratios (De Vries et al., 2006; Lauber et al., 2008). Land use can consequently affect the production of soil extracellular enzymes through its effect on microbial community composition, but it also reflects the external inputs of fertilizer and lime and soil management (e.g., plowing), which deplete organic N reservoirs in soils and down-regulate extracellular N-mining enzyme activities (Jangid et al., 2008; Xiao et al., 2018; Chen et al., 2022; Padbhushan et al., 2022).
Substrate availability is likely the most striking control on organic N depolymerization rates and has been shown to be driven by land use and soil properties at the regional scale (Noll et al., 2019b). Soil N stocks (as a proxy for soil protein contents) typically increase with mean annual precipitation and decrease with the level of aridity (Delgado-Baquerizo et al., 2013; Marty et al., 2017; Callesen et al., 2007). Changes in temperature and precipitation patterns are associated with changes in the potential natural vegetation, where N becomes progressively limiting with vegetation changes from deciduous to coniferous shrubs and trees, as well as from low to high latitudes (Kang et al., 2010; Reich and Oleksyn, 2004). Moreover, soil N stocks decrease with intensification of land management, from forests to grasslands and croplands (Six and Jastrow, 2002). Decomposition experiments of plant litter and organic soils have shown an inverse relationship of gross protein depolymerization rates and resource C : N ratios and a positive relation with resource N content, though none with potential peptidase activities, suggesting that protein depolymerization is rather controlled by substrate availability than by the pool size of extracellular enzymes (Mooshammer et al., 2012). However, in mineral soils the former relationship was less pronounced, indicating that protein stabilization on mineral surfaces may restrict soil protein cleavage (Wild et al., 2013; Noll et al., 2019b).
In mineral soils, organic nitrogen availability is constrained by interactions of organic compounds with the soil matrix, e.g., by the formation of organo-mineral associations, and restricted accessibility in small pores and soil aggregates render soil organic matter to become protected from enzymatic attack (Kögel-Knabner et al., 2008; Quiquampoix, 2000). Stabilization mechanisms are controlled by soil texture and soil mineral assemblage, and particularly by the amounts of Fe- and Al-(oxyhydr)oxides, which are major sorption sites of soil organic matter in soils (Kaiser and Guggenberger, 2000). Their amount and composition are shaped by soil parent material (primary minerals) and environmental conditions during pedogenesis, which control bedrock weathering and the formation of secondary minerals. Both protein availability and proteolytic activity are further constrained by substrates/exoenzymes being inactivated by formation of metal–organic complexes or by the complexation with tannins (Nierop et al., 2002; Hernes et al., 2001; Adamczyk et al., 2009).
Land use, bedrock and biogeographic region are therefore key controls on soil nutrient status and edaphic properties and affect microbial community structure, substrate availability, and microbial N and C demands (Lauber et al., 2008, 2009; Xu et al., 2013; Angst et al., 2018; Elrys et al., 2021). Changes in environmental conditions might thereby be translated into altered organic N process rates (Fig. 1). To investigate the major controls on organic N cycling, we sampled a large-scale transect across Europe, from the Mediterranean to the subarctic, covering three different land use types (forest/shrubland, grassland and cropland) as well as a wide range of climates and geologies, and determined gross protein depolymerization rates using an isotope pool dilution approach targeting soil amino acid production (protein depolymerization).
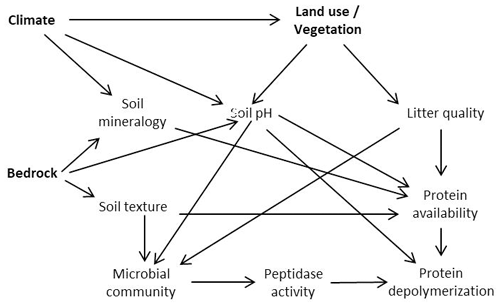
Figure 1Proposed model relating climate, bedrock and land use effects to protein depolymerization rates.
We hypothesized that (I) protein depolymerization is restricted by lower soil organic matter content and microbial activity in cropland soils compared to grassland and forest soils. (II) We further expected that the availability of proteins and thereby gross protein depolymerization rates are controlled by soil geochemical properties (e.g., soil pH), mineral assemblage and texture. (III) We further hypothesized that climate is a rather indirect control on organic N cycling by its effects on vegetation and soil geochemistry as well as on soil N stocks.
2.1 Sampling
Soil samples were collected during summer 2017 (May to August) at the peak of the growing season across a European continental transect from the warm Mediterranean to the cold subarctic and from the humid Atlantic western climate to the dry continental steppes in Romania (Fig. 2). The sampled soils were distinct in soil parent material, soil type, land use and vegetation. Sampling sites were selected to represent the natural vegetation as defined in the “Map of the natural vegetation of Europe” (Bohn and Katenina, 2000). For each sampling site climate data scaled to 100 m were extracted from the WorldClim database v. 1.4 (Fick and Hijmans, 2017). Bedrock was obtained from the international geological map of Europe (IGME5000, 1 : 5 000 000; Asch, 2005), and dominant soil types were obtained from the “Soil regions of European Union and adjacent areas” map (EUSR5000, 1 : 5 000 000; BGR, Bundesanstalt Für Geowissenschaften Und Rohstoffe, 2005).
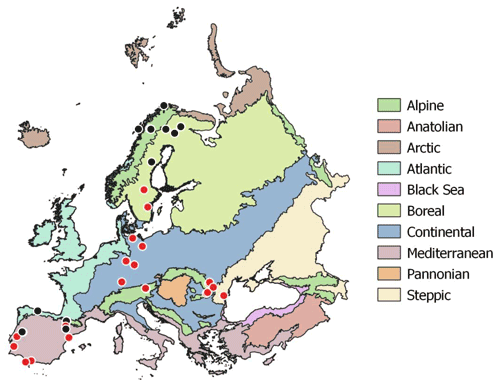
Figure 2Sampling sites across European biogeographical regions. Red circles symbolize sampling sites including three land use types (woodland, grassland, cropland). Map of European biogeographical regions was obtained from biogeographical regions data set of the European Environment Agency.
For statistical analyses bedrock types were binned into three groups: limestone, sediment and silicate. Sediment geologies included flysch, molasse, till and fluvial sand; silicate bedrock included plutonic, igneous and metamorphic formations; and carbonate bedrock ranged from dolomite to limestone and marl. Mean annual temperature (MAT) of the sampling sites ranged from −3.5 to 17.8 ∘C, and mean annual precipitation ranged from 415 to 1396 mm yr−1. Where possible, all three management types (woodland/forest, grassland and cropland) as well as mineral and organic soils were sampled in close vicinity. In the following we only use “woodland” for subarctic tundra, open woodlands and forests. At each site bulk samples of mineral top soil (0–15 cm) were taken with a soil corer (5 cm). Each bulk soil sample consisted of five replicates with about 5 m distance from each other. In total we sampled 96 mineral top soils from 43 sites; 23 sites included woodland, grassland and cropland soils (Table S1 in the Supplement). Organic layers were sampled at 13 sites using a 20×20 cm frame to cut out the organic horizon down to the mineral soil surface. The depth of the individual organic horizons varied from 2 to 30 cm. Representative leaf litter samples were collected at each site and represent the dominating vegetation. Roots and stones were removed from the soil samples manually immediately after sampling. Soil samples, roots, stones and litter samples were cooled (4–8 ∘C) and shipped within 3 to 7 d to the University of Vienna for further analyses. Soil samples were homogenized by sieving to 2 mm, and separate aliquots were air dried or stored moist at 4 ∘C. Litter and root samples were washed and dried in a drying oven at 60 ∘C.
2.2 Basic soil parameters
Soil texture, CaCO3 content, cation exchange capacity (CEC), base saturation (BS), and exchangeable Ca2+, Mg2+, K+, Na+, Al3+, Fe3+ and H+ were determined by the Austrian Agency for Health and Food Safety (AGES) according to European and international standards (ÖNORM). Iron and aluminum oxyhydroxides were determined in acid ammonium oxalate and in Na-dithionite extracts (Loeppert, 1996) at the Institute of Soil Research (IBF, University of Natural Resources and Life Sciences, Vienna, Austria). Oxalate-extractable Fe (Feoxalate) and Al (Aloxalate) refer to amorphous iron and aluminum oxyhydroxides and Fe bound in organo-metal complexes. Dithionite-extractable Fe minus oxalate-extractable Fe represents Fe bound in crystalline oxyhydroxides (Fed-o). The ratio of oxalate-extractable Fe over dithionite-extractable Fe presents a measure of the activity of the Fe-mineral phase (Feo/d). To determine the soil water content, sieved soils were dried at 85 ∘C for 48 h. Water-holding capacity (WHC) was measured by repeatedly saturating 10 g field-moist soil with deionized water and draining in between for 2.5 h in a funnel with an ash-free cellulose filter paper. Field-moist soils were either adjusted to 60 % WHC by gentle drying at room temperature or by addition of deionized water. Before further analyses all soils were pre-incubated for 2 weeks at 20 ∘C and 60 % water-holding capacity (WHC) in PE-Ziploc bags. Soil pH was measured in water and 10 mM CaCl2 (1 : 5 (w : v)) using an ISFET pH sensor (Sentron, Leek, the Netherlands). To determine total C and total N in root and litter as well as soil organic C (SOC) and soil total N (TN) oven-dried root, litter and soil samples were ground with a ball mill (MM 200, Retsch, Germany) and analyzed by an elemental analyzer (Carlo Erba 1110, CE Instruments) coupled to a DeltaPlus Isotope Ratio Mass Spectrometer (Finnigan MAT, Germany) via a ConFlo III interface (Thermo Fisher, Austria). If necessary, carbonates were removed from soil samples with 2 M HCl prior to SOC and TN measurements. Soil total P (TP) and soil total inorganic P (TIP) were determined in 0.5 M H2SO4 extracts of ignited (450, 4 ∘C; Lajtha et al., 1999) and control soil aliquots followed by malachite green measurements of reactive phosphate (Kuo, 1996). Total soil organic P (TOP) was calculated as the difference of TP–TIP. Soils were extracted with 1 M KCl (1 : 5 (w : v)) for 1 h and filtered through ash-free cellulose filters (Whatman). Dissolved organic C (DOC) and total N (TDN) were measured in the extracts by a TOC/TN analyzer (TOC-VCPH/TNM-1, Shimadzu, Austria). NH and NO were measured colorimetrically in the same extracts (Hood-Nowotny et al., 2010). Dissolved organic N (DON) was calculated as TDN minus NO and NH. Free amino acids (FAA) were determined fluorimetrically in 1 M KCl extracts by the OPAME fluorescence method (Jones et al., 2002) as modified by Prommer et al. (2014). Dissolved inorganic P (DIP, Olsen P) was extracted with 0.5 M NaHCO3 (1 : 7.5 (w : v), pH 8.5) for 1 h, filtered through ash-free cellulose filters and measured by malachite green. Total dissolved P (TDP) was measured following acid persulfate digestion, and dissolved organic P (DOP) was calculated as the difference of P concentration between digested and non-digested samples (Lajtha et al., 1999). Soil microbial community composition was analyzed by phospholipid fatty acid (PLFA) analyses according to Kaiser et al. (2010) and Hu et al. (2018). Microbial C, N and P were determined by chloroform fumigation extraction (Brookes et al., 1985). Sample aliquots were fumigated for 48 h and subsequently extracted as described above with 1 M KCl or 0.5 M NaHCO3. Potential activities of leucine aminopeptidase (EC 3.4.11.1) were determined in buffered (Na-acetate, pH 5.5) and unbuffered (ultra-pure water) soil slurries using L-leucine-7-amido-4-methyl coumarin (AMC-leucine) as a substrate (Kaiser et al., 2010). Triplicates of each sample were incubated for 2 h at 25 ∘C and measured every 30 min. Fluorescence was measured with a TECAN InfiniteR M200 (Austria) spectrophotometer at an excitation wavelength of 365 nm and an emission wavelength of 450 nm, and it was corrected for sample blank fluorescence and quenching prior to calculations of AMC concentration.
2.3 NaOH-extractable protein
A total of 2 g of fresh soil was extracted with 0.5 M NaOH (1 : 10 (w : v)) for 2 h in an ultra-sonic bath (160 W, Sonorex RK510, Germany) and subsequently for a further 16 h on a rotary shaker. NaOH extracts free and loosely bound proteins e.g from organo-mineral associations but not proteins stabilized in metal-organo complexes (Wattel-Koekkoek et al., 2001). Extracts were centrifuged for 15 min at 1600×g. As high salt concentrations interfere with the consequent measurement of hydrolyzed amino acids, 2.5 mL of supernatant was desalted using Sephadex™ G-25 columns (PD10 GE Healthcare, Uppsala, Sweden). For determination of total amino acids we adopted a method published by Martens and Loeffelmann (2003) and Hu et al. (2018). The purified extracts were freeze-dried and re-dissolved in 1.5 mL methanesulfonic acid (4 M MSA); 1 mL of samples, bovine serum albumin (BSA) standards, and blanks were hydrolyzed in an autoclave for 1 h at 135 ∘C. Hydrolyzed extracts were neutralized with 4 M KOH, and measurements were performed on an HPLC system (Dionex ICS-3000, Thermo Fisher Scientific, Bremen, Germany) coupled to an electrochemical detector. Amino acids were separated using a PA-10 IC column (Thermo Fisher Scientific, Bremen, Germany). NaOH-extractable protein (proteinNaOH) was calculated as the sum of the 20 measured amino acids.
2.4 Gross organic N processes
One day before starting the pool dilution experiment, FAA concentrations were determined in an aliquot of pre-incubated soil. The isotope pool dilution experiment and sample analyses were conducted as described previously by Noll et al. (2019a). In brief, 4 g of soil was weighed into transparent HDPE vials in duplicates and 400 µL of a 15N tracer solution was added dropwise. Samples were shaken vigorously to guarantee good mixing of the tracer. The tracer solution was prepared from a highly 15N-enriched amino acid mixture (U-15N-98 at. % 15N amino acid mixture from crude algal protein, Cambridge Isotope Laboratories, Radeberg, Germany). The total amount of added 15N was adjusted to about 20 % of the native FAA pool. The incubation was terminated after 15 and 45 min by addition of cold KCl (4 ∘C), and samples were extracted for 1 h on a rotary shaker and filtered at 4 ∘C. Prior to measuring the isotopic composition of FAA, NH was removed by microdiffusion (Lachouani et al., 2010; Noll et al., 2019a). Extracts were microdiffused for 48 h. To measure the concentration and atom %15N of FAA, 2 mL of pre-treated extracts was transferred into 12 mL glass exetainers and the α-amino group was cleaved/oxidized by NaClO and KBr as a catalyst under alkaline conditions as described by Zhang and Altabet (2008) and modified by Noll et al. (2019a). Subsequently, the produced NO was converted to N2O by buffered NaN3 (NaN3 in 100 % acetic acid 1 : 1). The produced N2O was measured with a purge-and-trap isotope ratio mass spectrometer (PT-IRMS) consisting of a Finnigan Delta V Advantage IRMS (Thermo Fisher, Germany) and a GasBench II headspace analyzer (Thermo Fisher, Germany) with a cryo-focusing unit. Calibration was done according to Lachouani et al. (2010) and Noll et al. (2019a).
2.5 Data analyses and statistics
Gross rates of protein depolymerization (GP) and microbial amino acid uptake (GU) were calculated according to Kirkham and Bartolomew (1954) and Wanek et al. (2010):
where and are the concentrations of FAA-N at the time points t1 (15 min) and t2 (45 min). 15N content in amino acids at the time points of termination are expressed as at. %15N and at. %15N, while at. %15Nb is the background 15N abundance (0.366 at. %15N) in non-labeled samples. Mean residence times of FAA were estimated as free amino acid pool size divided by microbial amino acid uptake rate. Microbial C : N and N : P imbalances were calculated as the ratio of resource C : N or N : P over microbial C : N or N : P.
For statistical analyses of single variables, mineral soils were grouped by bedrock (limestone, sediments, silicates) or by land use (cropland, grassland, woodlands). Prior to statistical analyses data were checked for normality and transformed if necessary. Land use effects on process rates and soil properties were analyzed for the 22 sites where cropland, grassland and woodland soils could be sampled in close vicinity (66 data points). Since only one composite sample was analyzed per land use at each site and therefore single observations were not independent, “site” was included as a factor in a two-way ANOVA to account for differences between sites (climate, bedrock, soil type). Given the low (non-significant) land use effects across sites the effects of bedrock were analyzed by one-way analysis of variance (ANOVA) followed by Tukey HSD tests. Not accounting for land use here allowed the analysis of the whole data set (n=91) instead of restricting this to the 22-site data set (n=66). Differences in process rates and soil properties between organic and underlying mineral soil horizons were analyzed by paired t tests for the 13 sites where organic and mineral horizons were sampled. Linear mixed models were used to explore the effect of soil properties and climate on protein depolymerization rates with land use as a random factor. The most parsimonious model was selected by Akaike's information criterion (AIC). Multicollinearity was assessed by variance inflation factors (VIFs). Variables with VIFs larger than 2.5 were excluded from the model. Partial correlations were used to control for the effect of soil geochemical properties on the relationship between climate and the response variables (i.e., protein depolymerization rates, leucine aminopeptidase activity and NaOH-extractable protein; Doetterl et al., 2015; Luo et al., 2017). Significant changes of the correlation coefficient were assumed when the 95 % confidence interval of the zero-order correlation and the partial correlation did not overlap. Partial correlations were analyzed using “ppcor” in R environment (Kim, 2015). Effects of climate parameters and their interactions on process rates were assessed by linear mixed-effect models with soil parent material or land use as random effects. We used structural equation modeling (SEM) to explore direct and indirect effects of climate, geology and soil properties on protein depolymerization rates. We used parameters which correlated significantly with protein depolymerization to construct a base model for gross protein depolymerization rates. Input variables were tested for multivariate normality and linearity. If necessary, variables were log transformed to mitigate departure from model assumptions. The model was then analyzed using the “lavaan” package (Rosseel, 2018) in R. Model fit was evaluated using Chi-square statistics (p>0.05). The most parsimonious model was identified by step-wise deletion of non-significant paths. Akaike's information criterion (AIC) was used to compare competing model fits. We followed the two-index strategy proposed by Hu and Bentler (1999) to describe the specified model and the data covariance matrix and reported root mean square error of approximation (RMSEA) and standardized root mean square residual (SRMR). Good model–data fit is indicated by RMSEA ≤ 0.06 and SRMR ≤ 0.08. All statistics were performed in R 3.1.3 (R Development Core Team, 2008). Direct and indirect effect sizes in path analysis were assessed by “lavaan”, indirect effects being calculated by multiplying the (direct) path effects that constitute the effect.
3.1 Effects of bedrock, land use, soil horizon and climate
Protein depolymerization rates were strongly related to soil physicochemical properties like soil pH, amorphous Fe and Al minerals (Feoxalate, Aloxalate) as well as to soil organic matter (Corg, total N), NaOH-extractable protein and microbial biomass (Cmic, PLFA) (Fig. 3, Table S3). NaOH-extractable protein content increased with SOC, soil TN, root biomass, and amorphous Fe- and Al-(hydr)oxides (Table S3, Fig. 3). Soil pH was negatively correlated with gross depolymerization and NaOH-extractable protein but positively with peptidase activity (Fig. 3). However, across all sites as well as within subgroups we found no significant (putatively positive) correlation between aminopeptidase activity, a wide spread soil proteolytic enzyme, and protein depolymerization rates (Fig. S5 in the Supplement). In order to further examine the potential edaphic controls on gross protein depolymerization in mineral soils as well as interaction effects with land use, we used multiple linear regression analyses. In the most parsimonious model NaOH-extractable protein explained 37 % of the variance, emphasizing the prominent role of substrate availability controlling depolymerization rates (Fig. 2). Land use did not interact with specific edaphic properties, and linear mixed-effect models with land use as a random factor confirmed the suggested main controls on depolymerization rates, i.e., protein availability and soil pH (Table S4).
Climate effects on depolymerization rates were analyzed by linear regression analyses including climate parameters, land use and interaction effects. We found significant effects of mean annual temperature (MAT) and mean annual precipitation (MAP) and of their interaction (MAP : MAT) (Table S5). Land use had no significant effect on the climate response of protein depolymerization, as shown by similar negative correlations between depolymerization and MAT in all three land use types (Fig. 4). The model explained about 42 % of the variance. Although the climatic humidity index (MAP : PET), expressed as MAP over potential evapotranspiration (PET), was not included in the most parsimonious model, the strong logarithmic increase of depolymerization rates with climatic humidity (r2=0.632, p<0.001) across all sites and land use types was striking (Fig. 4). The most parsimonious linear mixed-effect model included land use as a random factor and showed a strong negative effect of MAT and a positive effect of MAP. The model explained about 47 % of the variance in protein depolymerization
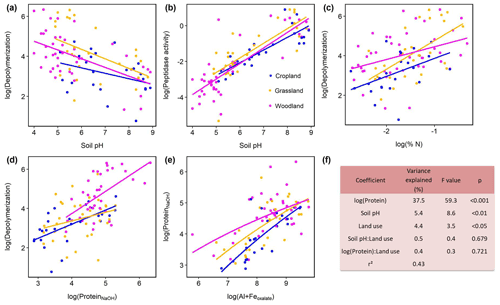
Figure 3Effects of soil properties on gross protein depolymerization rates in mineral soils. Relationship of pH and (a) log(protein depolymerization) and (b) log(leucine aminopeptidase activity). (c) Relationship of soil total N and protein depolymerization rate. (d) Relationship of NaOH-extractable protein and protein depolymerization rate. Color codes indicate land use type. (e) Relationship of oxalate-extractable Al and Fe and NaOH-extractable protein. (f) Analyses of variance of the most parsimonious linear regression model of log(gross protein depolymerization rate) explained by soil properties, land use and their interaction effects (n=95). Total model fit is given as adjusted r2.
3.2 Integrated effects of edaphic properties and climate
Since soil parent material, which is a main driver of soil geochemical properties, is not uniformly distributed across the sampled transect, climate effects (MAT and MAP) on gross protein depolymerization rates, leucine aminopeptidase activity and NaOH-extractable protein were analyzed by partial regression analyses controlling for geochemical parameters (Fig. 4). For instance we found a negative zero-order correlation between protein depolymerization and MAT (, p<0.01), the correlation coefficient decreasing significantly when removing correlations with Al, Fe or the sum of oxalate-extractable Fe and Al (Fig. 4). NaOH-extractable protein was negatively correlated to MAT (, p<0.01), the correlation coefficient decreasing significantly by removing the correlations with Al and the sum of oxalate-extractable Al and Fe. All zero-order correlations with MAT decreased significantly after removing the effects of soil geochemical parameters. Mean annual precipitation was weakly positively correlated with protein depolymerization (r=0.29, p<0.05) and NaOH-extractable protein (r=0.46, p<0.01); however, the removal of correlations with geochemical parameters had no significant effect.
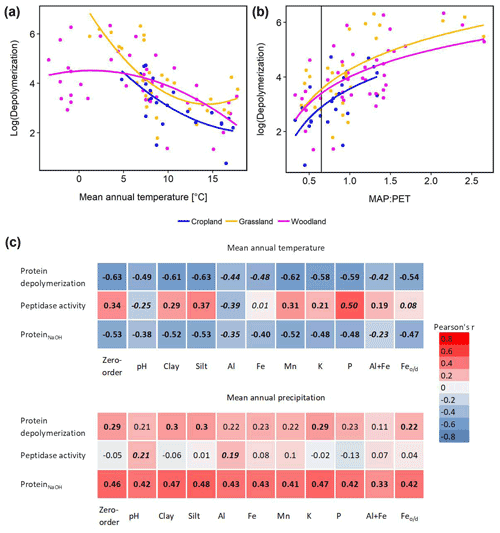
Figure 4Climate effects on gross protein depolymerization. (a) Relationship between the natural logarithm of gross protein depolymerization and second polynomial regression fit for cropland (adjusted r2=0.455, p<0.001, n=24), grassland (r2=0.480, p<0.001, n=28) and woodland (r2=0.219, p<0.01, n=48) soils. (b) Relationship between the natural logarithm of gross protein depolymerization rates and the ratio of mean annual precipitation over potential evapotranspiration (MAP : PET) and regression fit (y=log (x)) for cropland (adjusted r2=0.330, p<0.01, n=24), grassland (adjusted r2=0.371, p<0.001, n=28) and woodland (adjusted r2=0.318, p<0.001, n=48) soils. The vertical line indicates the transition from arid to humid climate conditions (MAP : PET = 0.65). (c) Zero-order and partial correlations (Pearson's r) between climate variables (MAT and MAP) and organic N cycling (protein depolymerization rate, leucine aminopeptidase activity and ProteinNaOH) controlled for geochemical variables). Significant correlations are indicated by bold numbers. Significant changes of the correlation coefficients compared to the zero-order correlation are indicated by italic numbers.
3.3 Path analyses
The a priori model was constructed according to the hypothesis illustrated in Fig. 1. After removing insignificant paths the model contained NaOH-extractable protein, soil pH, amorphous Fe and Al, and MAP (X2=2.49, p=0.288; RMSEA = 0.048, SRMR = 0.023). The revised model explained 43 % of the variance in gross protein depolymerization and 49 % of the variance in NaOH-extractable protein. Protein depolymerization in mineral soils was highly dependent on NaOH-extractable protein. Soil pH had direct and indirect (via NaOH-extractable protein) negative effects on depolymerization rates (Fig. 5). MAP and amorphous Fe and Al had positive effects on NaOH-extractable protein and thereby positive indirect effects on protein depolymerization. The total effects (direct effects + indirect effects) of the model parameters on protein depolymerization increased in the order amorphous Fe and Al < soil pH < MAP < NaOH-extractable protein.
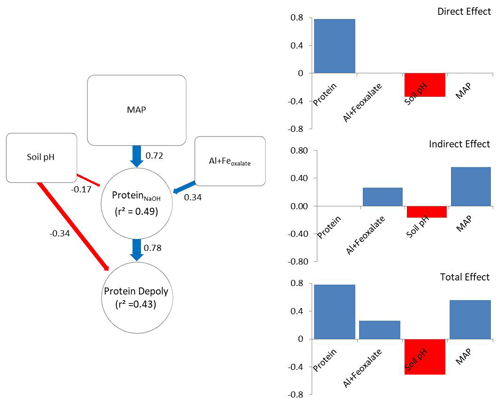
Figure 5Direct and indirect effects in gross protein depolymerization rates. Controls of path analyses for gross protein depolymerization rates in mineral soils and coefficients for direct, indirect and total effects (n=91). Significant effects (p<0.05) are indicated by red (negative) and blue (positive) arrows. Effect sizes are indicated by line width. Numbers beside arrows indicate the standardized parameter estimates. Numbers within boxes indicate the variance explained by the model.
4.1 Land use and soil horizon effects on protein depolymerization
Our results revealed that land use, which is an important driver of soil organic matter (SOM) contents and soil microbial community composition (Lauber et al., 2008; Jangid et al., 2008) and consequently of the set of excreted proteolytic enzymes, might only exert a minor control on soil organic N cycling at large spatial scales. Though effects were significant for individual sampling sites (Table S2), land use had no significant effect on the response of protein depolymerization rates to soil properties, explaining less than 5 % of the total variation in multiple linear regression models (Fig. 3, Table S5). This demonstrates that the same drivers operated on protein depolymerization in croplands, grasslands and woodlands and triggered the same directional and strength of response across land uses. Effects of land use were therefore likely strongly overprinted by large-scale changes in climate and geology, since in the applied sampling scheme the factor land use was nested in large-scale climatic and geological controls across a continental transect. Effects of land use might be more prominent at a smaller regional to local scale (Noll et al., 2019b), which was, however, not accessible with this data set.
At the continental level, gross protein depolymerization rates increased with SOM, from Mediterranean to temperate and boreal ecosystems. Though vegetation N limitation increases with latitude (Kang et al., 2010; Du et al., 2020; Augusto et al., 2017), rising depolymerization rates with latitude indicate increasing labile organic N provisioning to microbes and plants at higher latitudes under lab conditions. This positive effect of substrate availability on depolymerization rates was further confirmed by high gross protein depolymerization rates observed in organic horizons in boreal and alpine biomes, which significantly exceeded those in the underlying mineral soils (Table S2). However, in contrast to findings of Mooshammer et al. (2012) for decomposing litter, our data revealed no indication that resource C : N or microbial C : N imbalances affected protein depolymerization rates in organic soils and thereby highlights the differential element, viz. nutrient limitation of plants and soil microbes across large spatial scales as proposed by Capek et al. (2018).
4.2 Substrate limitation of protein depolymerization is controlled by organo-mineral interactions
Across all land use types NaOH-extractable protein and soil pH were the main predictors for gross protein depolymerization in mineral soils, indicating that soil properties that determine protein availability such as texture, mineral assemblage or soil pH need to be considered when addressing large-scale controls of soil organic N cycling. Gross protein depolymerization was lower in soils developed on limestone than in soils developed on sediments or silicates, which is emphasized by the inverse relationship between depolymerization rates and soil pH (Fig. 3). Moreover, depolymerization rates decreased with increasing clay content. Proteins can be adsorbed to clay surfaces by electrostatic interactions between positively charged amino acid side chains and siloxane surfaces of clay minerals (Staunton and Quiquampoix, 1994; Quiquampoix and Ratcliffe, 1992). Sorption experiments in artificial soils showed that at neutral soil pH (>7) clay minerals are the main sorption sites for organic N (Pronk et al., 2013). This can be further enhanced by polyvalent cations as Ca2+ or Mg2+, which can bridge the negative charges of clay mineral surfaces and proteins (Cao et al., 2011; Lützow et al., 2006). Aside from the stabilization on mineral surfaces, high clay contents, as found in limestone soils, promote soil aggregation and thereby the occlusion of organic matter and proteins rendering them inaccessible for enzymatic attack (Lützow et al., 2006). In contrast iron and aluminum oxyhydroxides, the main sorption sites for SOM at acidic pH, were positively correlated to gross depolymerization rates. SOM accumulation is usually higher in acidic soils due to ligand exchange between protonated hydroxyl groups of Fe- and Al-minerals and carboxyl groups of organic molecules (Gu et al., 1994; Kleber et al., 2005; Kaiser and Guggenberger, 2000). Therefore the overall organic N pool size is expected to be larger in fine textured soils and in soils high in iron and aluminum oxyhydroxides. Moreover, the strength of the binding interaction between iron and aluminum oxyhydroxides and SOM, and more specifically with organic N including proteins, is higher by 50 % than with typical clay minerals (Newcomb et al., 2017). Consequently soils rich in iron and aluminum oxyhydroxides contain larger pools of proteolytic substrates (organic N and proteins), but these substrates can be more strongly bound and therefore be less accessible for microbial utilization. However, column experiments with embedded goethite in acidic soils revealed that sufficiently large amounts of stabilized OM can be re-dissolved by progressing percolation of dissolved OM and the subsequent exchange with adsorbed compounds such as peptides (Leinemann et al., 2018), which thereby become available for enzymatic attack and/or microbial utilization. The bioavailability of oxide-bound organic N is further supported by the strong positive correlation between NaOH-extractable protein and amorphous iron and aluminum oxyhydroxides (Table S3), since NaOH mainly extracts loosely bound proteins (Wattel-Koekkoek et al., 2001). Overall, iron and aluminum oxyhydroxides remained as a significant parameter in linear models and path analyses and should therefore be considered as important predictors for the potential of a soil to retain and accumulate SOM (Moni et al., 2007; Fang et al., 2019), which promotes microbial biomass and activity (Xu et al., 2013; Hartman and Richardson, 2013). The positive effect of the potential to accumulate SOM can be attributed to the continuous exchange of adsorbed compounds and the consequent steady release of organic N. The net effect of these adverse interactions is currently unknown; therefore this study is among the first to show a net positive effect of iron and aluminum oxyhydroxides on the in situ rates of depolymerization of high-molecular-weight ON substrates.
Though the total N pool size was not significantly different between soils developed on the three bedrock types, NaOH-extractable protein increased in the order limestone < sediment < silicate. NaOH-extractable protein accounted for 4.4±1.7 % of total N in sediment soils and for 6.4±3 % in silicate soils, compared to 2.9±2 % in limestone soils. This could be either attributed to a lower extraction efficiency of proteins with 0.5 M NaOH from clay minerals at high soil pH or to an increase of non-hydrolyzable organic N. The studied limestone soils were characterized by higher amounts of crystalline iron (Fed-o), namely hematite, which forms almost irreversible interactions with SOM (Gu et al., 1995), even at high soil pH, due to formation of coordination complexes between carboxyl groups and Fe atoms (Koutsoukos et al., 1983; Quiquampoix, 2000). The formation of strong peptide complexes with crystalline Fe minerals is also supported by findings of Mikutta et al. (2010), who showed an increase of non-hydrolyzable peptide-N with the proportion of crystalline Fe minerals across a soil chronosequence.
From linear regression and path analyses soil pH was revealed as the second most important predictor of gross protein depolymerization rates. Soil pH mirrors the strength of Ca2+ bridging of negatively charged ligands (as protein-carboxylates) to negatively charged soil particles (clays) as well as the weathering status of soils, which comes with the formation of secondary clays and iron and aluminum oxyhydroxides. However, soil pH also directly affects electrostatic interactions between mineral surfaces and proteins. Sorption of proteins on clay and Fe-mineral surfaces is usually highest close to the isoelectric point of a specific protein. Due to the complex nature of proteins including different functional groups and tertiary structures isoelectric points range from pH 1 for pepsin to pH 11 for lysozyme, making predictions for soil proteins at large impossible. Sorption of bovine and human serum albumin on montmorillonite peaked at pH ∼ 5, whereas adsorption of cytochrome c or ribonuclease on hematite peaked at pH 8 to 10, all being close to their isoelectric points (Khare et al., 2006; Koutsoukos et al., 1983; Quiquampoix and Ratcliffe, 1992).
However, the negative effect of soil pH on gross depolymerization is in sharp contrast to the increase of peptidase activity with soil pH. To allow comparisons between enzyme activities and depolymerization rates, enzyme activities were measured (i) in unbuffered soil slurries at natural soil pH and (ii) compared to enzyme activities measured at the same pH in an acetate buffer (pH 5.2). Hence, unbuffered peptidase activities were highest in limestone soils close to the pH optima of proteolytic enzymes at about 8 (Sinsabaugh et al., 2008) (Fig. S5). The lack of correlation between gross depolymerization and peptidase activity, but rather the maximum of protein depolymerization coinciding with the minima of potential protease activity, implies that gross protein depolymerization rates are rather substrate limited compared to enzyme limited. It further highlights that differences in protein depolymerization between alkaline, neutral and acidic soils are due to changes in substrate (protein) availability rather than due to changes in microbial community structure and enzymatic activity. Even when peptidase was measured at the same pH, potential peptidase activity was higher in limestone soils compared to sediment and silicate soils (Table S2, Fig. S6), which implies enhanced microbial enzyme excretion in limestone soils in response to lower protein availability.
The generally low protein depolymerization rates in limestone soils are in accordance with our previous findings from soils developed on limestone and silicate bedrock in Austria (Noll et al., 2019b), demonstrating that soil parent material pre-determines depolymerization rates on regional and continental scales. We assume that in limestone soils proteins are strongly stabilized on phyllosilicates and crystalline Fe oxides or occluded within soil aggregates rendering them inaccessible for proteolytic attack. Soil microorganisms respond to this N limitation by greater investments into the production and excretion of extracellular enzymes mining for these soil organic N forms (Chen et al., 2014), as shown by the enhanced potential activities of aminopeptidase in limestone soils.
4.3 Climate drives protein depolymerization by affecting mineral weathering and plant productivity
Climate is a major control on mineral weathering and net primary productivity (Norton et al., 2014; Doetterl et al., 2015) and thereby affects protein stabilization and input of fresh OM by plants. Across the studied climate transect gross protein depolymerization rates decreased with MAT and increased with MAP and therefore increased with the climatic humidity index (MAP : PET). As demonstrated by the partial correlations, part of the negative effect of MAT on depolymerization rates can be explained by concomitant changes in amorphous iron and aluminum oxyhydroxides and soil pH, which affect protein availability (Fig. 4). The correlation coefficient between MAT and depolymerization significantly decreased by removing the effects of soil iron and aluminum oxyhydroxides, while the decrease by removing effects of soil pH was not significant. The important role of soil geochemical properties on protein stabilization is underpinned by the even stronger effect of soil properties on the relation between MAT and NaOH-extractable protein (Fig. 4). In the Mediterranean region limestone-derived red soils are predominant. The so-called “terra rossa” soils are characterized by high soil pH, high clay contents and relatively high amounts of crystalline Fe as well as a low Feoxalate : Fedithionite ratio, caused by the preferential formation of the Fe-oxide hematite over the Fe-hydroxide goethite during the summer dry period (Yaalon, 1997). As described above, these specific soil properties might foster stabilization of proteins and thereby constrain gross protein depolymerization. Under more humid conditions soil pH drops due to leaching of base cations (e.g., Ca2+) and more intensive chemical weathering promotes the formation of higher amounts of charged mineral surfaces as amorphous iron and aluminum oxyhydroxides (Doetterl et al., 2015). This increase in soil acidification at higher latitude is further facilitated by the predominance of silicate bedrock in northern Europe. Although MAP is an important driver of soil weathering and thereby affects soil pH and the formation of charged mineral surfaces, the positive effect of MAP on depolymerization rates and proteins was not significantly biased by soil properties in the partial correlations (Fig. 4). However, the weak effects of iron and aluminum oxyhydroxides on the relation between protein depolymerization and MAP, or between NaOH-extractable protein and MAP, might indicate the role of MAP in soil mineral formation during pedogenesis. Particularly in arid and sub-arid biomes precipitation determines plant net primary production (Yang et al., 2008; Del Grosso et al., 2008) and thereby the input of fresh organic matter into the soil. This might further explain the strong relationship between NaOH-extractable protein and MAP, as indicated by linear models and path analyses and further supported by the proximate increase in depolymerization with the climatic humidity index (Fig. 4). The logarithmic response implies that the limiting effect of MAP is stronger under sub-arid conditions, which is in accordance with findings showing that in water-limited regions net primary production is strongly controlled by MAP (Yang et al., 2008). Therefore, we conclude that, in sub-arid regions in southern Europe, precipitation constrains plant biomass production and consequently OM input into soils. In contrast, our results reveal that the increase of gross depolymerization with MAT is biased by “concurrent” changes in soil parent material across the studied transect, while MAP likely controls net primary productivity and mineral weathering (Gislason et al., 2009; La Pierre et al., 2016). Both partial correlations and path analyses support our hypothesis that climate is a rather indirect driver of soil organic N cycling by its effects on soil chemical weathering and more specifically the formation of specific minerals and consequently on soil organic matter accumulation.
Path analysis emphasized the important role of climate and bedrock as pre-determinants of OM stabilization and protein availability, and it suggested that MAP, soil pH, and iron and aluminum oxyhydroxides are indirect controls on gross protein depolymerization, which is mediated by protein availability, while soil pH and NaOH-extractable protein are direct controls on gross protein depolymerization. The indirect effect of MAP exceeded the direct effects of soil mineralogy and pH. However, NaOH-extractable protein overall was the main predictor of protein depolymerization rates. The negative direct effect of soil pH on depolymerization rates is explained by the low solubility of proteins at high soil pH (Franco and Pessôa Filho, 2011), which restricts diffusion throughout the soil matrix and limits the accessibility of protein substrates to enzymatic attack. In contrast, the negative pH effect on NaOH-extractable protein is attributed to the accumulation of SOM at acidic soil pH and the increased interactions with iron and aluminum oxyhydroxides (Kaiser and Guggenberger, 2003; Gu et al., 1994). With increasing soil pH amino groups of proteins become de-protonated and thereby proteins become negatively charged, which increases the repulsion from negatively charged mineral surfaces and decreases the adsorption to Fe oxides and phyllosilicates (Cao et al., 2011). Furthermore, soil pH, texture and mineral assemblage are drivers of microbial community composition and affect the availability of other nutrients like P or K (Fierer and Jackson, 2006; Lauber et al., 2008). Neither Ca2+ nor clay was included in the final model, despite their important role in stabilizing soil organic matter (Lützow et al., 2006). We assume that the effects of Ca2+ and clay are outweighed by effects of soil pH and MAP. Soil pH decreased from clay-rich limestone soils to sediment soils and to more sandy silicate soils, and thereby co-varied with Ca2+ and clay content, while MAP regulates mineral dissolution and leaching of Ca2+ (Gislason et al., 2009). Land use was non-significant and therefore was removed from the revised path model, which is in accordance with results from general linear models, showing that soil properties and climate variables explained the greatest percentage of the variance in gross protein depolymerization. Although path analyses provided an integrative model of controls driving gross protein depolymerization, it offered an incomplete picture. In this study we focused on the large-scale patterns, which explained more than 40 % of the variation in organic N cycling. However, regional or local effects, such as by topography, land use history, land use intensity, and plant community composition, were not accessible with this data set, but they are likely important controls on organic N cycling at regional spatial scales.
Our results highlight the important role of soil geochemistry when estimating microbial nutrient cycling on continental to global scales, and they demonstrate that at this scale soil parent material and climate override the effects of land use on soil organic N transformations. The amount of NaOH-extractable protein was here identified as the most important direct predictor of protein depolymerization rates, while peptidase activity negatively related to protein depolymerization, and therefore rather reflects a proxy of microbial N limitation according to enzyme allocation theory (Allison et al., 2010). Since protein availability and thereby protein depolymerization is strongly constrained by soil organic matter–mineral interactions, shifts in climate (precipitation regime) and associated alterations in soil weathering should be considered as drivers of ecosystem N availability with strong repercussions on ecosystem C cycle processes. This also needs to be validated in large-scale coupled climate–biogeochemistry and in Earth system models to help predict and mitigate global change effects.
Data are freely available on Zenodo at https://doi.org/10.5281/zenodo.7395605 (Noll et al., 2022).
The supplement related to this article is available online at: https://doi.org/10.5194/bg-19-5419-2022-supplement.
LN wrote the paper, conducted fieldwork and laboratory work, and analyzed and interpreted the data. SZ, QZ and YH conducted laboratory work, analyzed the data and edited the paper. FH analyzed the data and edited the paper. WW designed the study, interpreted the data and edited the paper.
The contact author has declared that none of the authors has any competing interests.
Publisher's note: Copernicus Publications remains neutral with regard to jurisdictional claims in published maps and institutional affiliations.
We thank Theresa Böckle, Daniel Wasner, Vsevolods Girsovics and Rebecca Lieske for soil sampling and assistance in the lab. We would like to thank Jukka Pumpanen for providing soil samples from the Värriö District Nature Reserve.
This research has been supported by the Austrian Science Fund (grant no. P-28037-B22).
This paper was edited by Luo Yu and reviewed by Richard Marinos and one anonymous referee.
Adamczyk, B., Kitunen, V., and Smolander, A.: Polyphenol oxidase, tannase and proteolytic activity in relation to tannin concentration in the soil organic horizon under silver birch and Norway spruce, Soil Biol. Biochem., 41, 2085–2093, https://doi.org/10.1016/j.soilbio.2009.07.018, 2009.
Allison, S. D., Weintraub, M. N., Gartner, T. B., and Waldrop, M. P.: Evolutionary-economic principles as regulators of soil enzyme production and ecosystem function, in: Soil enzymology, Springer, Berlin, Heidelberg, Germany, 229–243, ISBN 978-3-642-14225-3, 2010.
Angst, G., Messinger, J., Greiner, M., Häusler, W., Hertel, D., Kirfel, K., Kögel-Knabner, I., Leuschner, C., Rethemeyer, J., and Mueller, C. W.: Soil organic carbon stocks in topsoil and subsoil controlled by parent material, carbon input in the rhizosphere, and microbial-derived compounds, Soil Biol. Biochem., 122, 19–30, https://doi.org/10.1016/j.soilbio.2018.03.026, 2018.
Asch, K.: IGME 5000: 1 : 5 Million international geological map of Europe and Adjacent Areas–final version for the internet, BGR, Hannover, 2005.
Augusto, L., Achat, D. L., Jonard, M., Vidal, D., and Ringeval, B.: Soil parent material – A major driver of plant nutrient limitations in terrestrial ecosystems, Glob. Change Biol., 23, 3808–3824, 2017.
BGR [Bundesanstalt für Geowissenschaften und Rohstoffe]: Soil Regions Map of the European Union and Adjacent Countries 1 : 5 000 000 (Version 2.0), Special Publication Ispra, EU catalogue number S.P.I.05.134., https://services.bgr.de/boden/eusr5000 (last access: 29 November 2022), 2005.
Bohn, U. and Katenina, G.: Map of the natural vegetation of Europe: scale 1 : 2,500,000, Part 2: Legend, Bundesamt für Naturschutz (German Federal Agency for Nature conservation), Bonn, ISBN 3784338372, 2000.
Brookes, P., Landman, A., Pruden, G., and Jenkinson, D.: Chloroform fumigation and the release of soil nitrogen: a rapid direct extraction method to measure microbial biomass nitrogen in soil, Soil Biol. Biochem., 17, 837–842, 1985.
Callesen, I., Raulund-Rasmussen, K., Westman, C. J., and Tau-Strand, L.: Nitrogen pools and C : N ratios in well-drained Nordic forest soils related to climate and soil texture, Boreal Environ. Res., 12, 681–692, 2007.
Cao, Y., Wei, X., Cai, P., Huang, Q., Rong, X., and Liang, W.: Preferential adsorption of extracellular polymeric substances from bacteria on clay minerals and iron oxide, Colloids Surface., 83, 122–127, https://doi.org/10.1016/j.colsurfb.2010.11.018, 2011.
Capek, P. T., Manzoni, S., Kastovska, E., Wild, B., Diakova, K., Barta, J., Schnecker, J., Blasi, C., Martikainen, P. J., Alves, R. J. E., Guggenberger, G., Gentsch, N., Hugelius, G., Palmtag, J., Mikutta, R., Shibistova, O., Urich, T., Schleper, C., Richter, A., and Santruckova, H.: A plant-microbe interaction framework explaining nutrient effects on primary production, Nat. Ecol. Evol., 2, 1588–1596, https://doi.org/10.1038/s41559-018-0662-8, 2018.
Chen, Q., Yang, F., and Cheng, X.: Effects of land use change type on soil microbial attributes and their controls: Data synthesis, Ecol. Indic., 138, 108852, https://doi.org/10.1016/j.ecolind.2022.108852, 2022.
Chen, R., Senbayram, M., Blagodatsky, S., Myachina, O., Dittert, K., Lin, X., Blagodatskaya, E., and Kuzyakov, Y.: Soil C and N availability determine the priming effect: microbial N mining and stoichiometric decomposition theories, Glob. Change Biol., 20, 2356–2367, 2014.
De Vries, F. T., Hoffland, E., van Eekeren, N., Brussaard, L., and Bloem, J.: Fungal/bacterial ratios in grasslands with contrasting nitrogen management, Soil Biol. Biochem., 38, 2092–2103, 2006.
Del Grosso, S., Parton, W., Stohlgren, T., Zheng, D., Bachelet, D., Prince, S., Hibbard, K., and Olson, R.: Global potential net primary production predicted from vegetation class, precipitation, and temperature, Ecology, 89, 2117–2126, 2008.
Delgado-Baquerizo, M., Maestre, F. T., Gallardo, A., Bowker, M. A., Wallenstein, M. D., Quero, J. L., Ochoa, V., Gozalo, B., García-Gómez, M., and Soliveres, S.: Decoupling of soil nutrient cycles as a function of aridity in global drylands, Nature, 502, 672–676, 2013.
Doetterl, S., Stevens, A., Six, J., Merckx, R., Van Oost, K., Pinto, M., Casanova-Katny, A., Munoz, C., Boudin, M., Venegas, E., and Boeckx, P.: Soil carbon storage controlled by interactions between geochemistry and climate, Nat. Geosci., 8, 780–783, https://doi.org/10.1038/NGEO2516, 2015.
Du, E., Terrer, C., Pellegrini, A. F., Ahlström, A., van Lissa, C. J., Zhao, X., Xia, N., Wu., X. and Jackson, R. B.: Global patterns of terrestrial nitrogen and phosphorus limitation, Nat. Geosci., 13, 221–226, 2020.
Elrys, A. S., Ali, A., Zhang, H., Cheng, Y., Zhang, J., Cai, Z. C., Mueller, C., and Chang, S. X.: Patterns and drivers of global gross nitrogen mineralization in soils, Glob. Change Biol., 27, 5950–5962, 2021.
Fang, K., Qin, S., Chen, L., Zhang, Q., and Yang, Y.: Al/Fe mineral controls on soil organic carbon stock across Tibetan alpine grasslands, J. Geophys. Res.-Biogeo., 124, 247–259, 2019.
Fick, S. E. and Hijmans, R. J.: Worldclim 2: New 1-km spatial resolution climate surfaces for global land areas, Int. J. Climatol., 37, 4302–4315, https://doi.org/10.1002/joc.5086, 2017.
Fierer, N. and Jackson, R. B.: The diversity and biogeography of soil bacterial communities, P. Natl. Acad. Sci. USA, 103, 626–631, 2006.
Franco, L. F. M. and Pessôa Filho, P. d. A.: On the solubility of proteins as a function of pH: Mathematical development and application, Fluid Phase Equilibr., 306, 242–250, https://doi.org/10.1016/j.fluid.2011.04.015, 2011.
Fuka, M. M., Engel, M., Gattinger, A., Bausenwein, U., Sommer, M., Munch, J. C., and Schloter, M.: Factors influencing variability of proteolytic genes and activities in arable soils, Soil Biol. Biochem., 40, 1646–1653, 2008.
Gislason, S. R., Oelkers, E. H., Eiriksdottir, E. S., Kardjilov, M. I., Gisladottir, G., Sigfusson, B., Snorrason, A., Elefsen, S., Hardardottir, J., Torssander, P., and Oskarsson, N.: Direct evidence of the feedback between climate and weathering, Earth Planet. Sc. Lett., 277, 213–222, https://doi.org/10.1016/j.epsl.2008.10.018, 2009.
Gu, B., Schmitt, J., Chen, Z., Liang, L., and McCarthy, J. F.: Adsorption and desorption of natural organic matter on iron oxide: mechanisms and models, Environ. Sci. Technol., 28, 38–46, 1994.
Gu, B., Schmitt, J., Chen, Z., Liang, L., and McCarthy, J. F.: Adsorption and desorption of different organic matter fractions on iron oxide, Geochim. Cosmochim. Ac., 59, 219–229, https://doi.org/10.1016/0016-7037(94)00282-Q, 1995.
Hartman, W. H. and Richardson, C. J.: Differential nutrient limitation of soil microbial biomass and metabolic quotients (q CO2): is there a biological stoichiometry of soil microbes?, PloS one, 8, e57127, https://doi.org/10.1371/journal.pone.0057127, 2013.
Hendriksen, N. B., Creamer, R. E., Stone, D., and Winding, A.: Soil exo-enzyme activities across Europe – The influence of climate, land-use and soil properties, Appl. Soil Ecol., 97, 44–48, https://doi.org/10.1016/j.apsoil.2015.08.012, 2016.
Hernes, P. J., Benner, R., Cowie, G. L., Goñi, M. A., Bergamaschi, B. A., and Hedges, J. I.: Tannin diagenesis in mangrove leaves from a tropical estuary: a novel molecular approach, Geochim. Cosmochim. Ac., 65, 3109–3122, 2001.
Hood-Nowotny, R., Umana, N. H.-N., Inselbacher, E., Oswald-Lachouani, P., and Wanek, W.: Alternative methods for measuring inorganic, organic, and total dissolved nitrogen in soil, Soil Sci. Soc. Am. J., 74, 1018, https://doi.org/10.2136/sssaj2009.0389, 2010.
Hu, L. T. and Bentler, P. M.: Cutoff Criteria for Fit Indexes in Covariance Structure Analysis: Conventional Criteria Versus New Alternatives, Struct. Equ. Modeling, 6, 1–55, https://doi.org/10.1080/10705519909540118, 1999.
Hu, Y., Zheng, Q., Zhang, S., Noll, L., and Wanek, W.: Significant release and microbial utilization of amino sugars and d-amino acid enantiomers from microbial cell wall decomposition in soils, Soil Biol. Biochem., 123, 115–125, 2018.
Jangid, K., Williams, M. A., Franzluebbers, A. J., Sanderlin, J. S., Reeves, J. H., Jenkins, M. B., Endale, D. M., Coleman, D. C., and Whitman, W. B.: Relative impacts of land-use, management intensity and fertilization upon soil microbial community structure in agricultural systems, Soil Biol. Biochem., 40, 2843–2853, 2008.
Jones, D. L., Owen, A. G., and Farrar, J. F.: Simple method to enable the high resolution determination of total free amino acids in soil solutions and soil extracts, Soil Biol. Biochem., 34, 1893–1902, 2002.
Kaiser, C., Koranda, M., Kitzler, B., Fuchslueger, L., Schnecker, J., Schweiger, P., Rasche, F., Zechmeister-Boltenstern, S., Sessitsch, A., and Richter, A.: Belowground carbon allocation by trees drives seasonal patterns of extracellular enzyme activities by altering microbial community composition in a beech forest soil, New Phytol., 187, 843–858, 2010.
Kaiser, K. and Guggenberger, G.: The role of DOM sorption to mineral surfaces in the preservation of organic matter in soils, Org. Geochem., 31, 711–725, https://doi.org/10.1016/S0146-6380(00)00046-2, 2000.
Kaiser, K. and Guggenberger, G.: Mineral surfaces and soil organic matter, Eur. J. Soil Sci., 54, 219–236, https://doi.org/10.1046/j.1365-2389.2003.00544.x, 2003.
Kang, H. Z., Xin, Z. J., Berg, B., Burgess, P. J., Liu, Q. L., Liu, Z. C., Li, Z. H., and Liu, C. J.: Global pattern of leaf litter nitrogen and phosphorus in woody plants, Ann. For. Sci., 67, 8, https://doi.org/10.1051/forest/2010047, 2010.
Khare, N., Eggleston, C. M., Lovelace, D. M., and Boese, S. W.: Structural and redox properties of mitochondrial cytochrome c co-sorbed with phosphate on hematite (α-Fe2O3) surfaces, J. Colloid Interf. Sci., 303, 404–414, 2006.
Kim, S.: ppcor: Partial and Semi-Partial (Part) Correlation [code], https://CRAN.R-project.org/package=ppcor (last access: 14 October 2022), 2015.
Kirkham, D. and Bartholomew, W.: Equations for following nutrient transformations in soil, utilizing tracer data, Soil Sci. Soc. Am. J., 18, 33–34, 1954.
Kleber, M., Mikutta, R., Torn, M., and Jahn, R.: Poorly crystalline mineral phases protect organic matter in acid subsoil horizons, Eur. J. Soil Sci., 56, 717–725, 2005.
Kögel-Knabner, I., Guggenberger, G., Kleber, M., Kandeler, E., Kalbitz, K., Scheu, S., Eusterhues, K., and Leinweber, P.: Organo-mineral associations in temperate soils: Integrating biology, mineralogy, and organic matter chemistry, J. Plant Nutr. Soil Sc., 171, 61–82, https://doi.org/10.1002/jpln.200700048, 2008.
Koutsoukos, P. G., Norde, W., and Lyklema, J.: Protein adsorption on hematite (α-Fe2O3) surfaces, J. Colloid Interf. Sci., 95, 385–397, https://doi.org/10.1016/0021-9797(83)90198-4, 1983.
Kuo, S.: Phosphorus, Part 3, in: Methods of Soil Analysis Part 3, edited by: Sparks, D. L., SSSA Book Series, Soil Science Society of America, Inc. & American society of Agronomy, Inc., Madison, WI, 869–919, 1996.
Lachouani, P., Frank, A. H., and Wanek, W.: A suite of sensitive chemical methods to determine the δ15N of ammonium, nitrate and total dissolved N in soil extracts, Rapid Commun. Mass Sp., 24, 3615–3623, https://doi.org/10.1002/rcm.4798, 2010.
Lajtha, K., Driscoll, C., Jarrell, W., and Elliott, E.: Soil phosphorus: characterization and total element analysis, Standard soil methods for long-term ecological research, Oxford University Press, New York, 115–142, 1999.
La Pierre, K. J., Blumenthal, D. M., Brown, C. S., Klein, J. A., and Smith, M. D.: Drivers of Variation in Aboveground Net Primary Productivity and Plant Community Composition Differ Across a Broad Precipitation Gradient, Ecosystems, 19, 521–533, https://doi.org/10.1007/s10021-015-9949-7, 2016.
Lauber, C. L., Strickland, M. S., Bradford, M. A., and Fierer, N.: The influence of soil properties on the structure of bacterial and fungal communities across land-use types, Soil Biol. Biochem., 40, 2407–2415, https://doi.org/10.1016/j.soilbio.2008.05.021, 2008.
Lauber, C. L., Hamady, M., Knight, R., and Fierer, N.: Pyrosequencing-Based Assessment of Soil pH as a Predictor of Soil Bacterial Community Structure at the Continental Scale, Appl. Environ. Microb., 75, 5111–5120, https://doi.org/10.1128/AEM.00335-09, 2009.
Leinemann, T., Preusser, S., Mikutta, R., Kalbitz, K., Cerli, C., Höschen, C., Mueller, C. W., Kandeler, E., and Guggenberger, G.: Multiple exchange processes on mineral surfaces control the transport of dissolved organic matter through soil profiles, Soil Biol. Biochem., 118, 79–90, https://doi.org/10.1016/j.soilbio.2017.12.006, 2018.
Loeppert, R. H.: Iron Methods of Soil Analysis. Part 3, in: Methods of Soil Analysis Part 3, edited by: Sparks, D. L., SSSA Book Series, Soil Science Society of America, Inc. & American society of Agronomy, Inc., Madison, WI, 639–664, 1996.
Luo, Z., Feng, W., Luo, Y., Baldock, J., and Wang, E.: Soil organic carbon dynamics jointly controlled by climate, carbon inputs, soil properties and soil carbon fractions, Glob. Change Biol., 23, 4430–4439, https://doi.org/10.1111/gcb.13767, 2017.
Lützow, M. V., Kögel-Knabner, I., Ekschmitt, K., Matzner, E., Guggenberger, G., Marschner, B., and Flessa, H.: Stabilization of organic matter in temperate soils: mechanisms and their relevance under different soil conditions – a review, Eur. J. Soil Sci., 57, 426–445, 2006.
Martens, D. A. and Loeffelmann, K. L.: Soil amino acid composition quantified by acid hydrolysis and anion chromatography-pulsed amperometry, J. Agr. Food Chem., 51, 6521–6529, 2003.
Marty, C., Houle, D., Gagnon, C., and Courchesne, F.: The relationships of soil total nitrogen concentrations, pools and C : N ratios with climate, vegetation types and nitrate deposition in temperate and boreal forests of eastern Canada, Catena, 152, 163–172, https://doi.org/10.1016/j.catena.2017.01.014, 2017.
Mikutta, R., Kaiser, K., Dörr, N., Vollmer, A., Chadwick, O. A., Chorover, J., Kramer, M. G., and Guggenberger, G.: Mineralogical impact on organic nitrogen across a long-term soil chronosequence (0.3–4100 kyr), Geochim. Cosmochim. Ac., 74, 2142–2164, 2010.
Mooshammer, M., Wanek, W., Schnecker, J., Wild, B., Leitner, S., Hofhansl, F., Blöchl, A., Hämmerle, I., Frank, A. H., and Fuchslueger, L.: Stoichiometric controls of nitrogen and phosphorus cycling in decomposing beech leaf litter, Ecology, 93, 770–782, 2012.
Moni, C., Chabbi, A., Nunan, N., Rumpel, C., and Chenu, C.: Do iron and aluminium oxides stabilise organic matter in soil? A multi-scale statistical analysis, from field to horizon, in: AGU Fall Meeting Abstracts, B11G-04, 2007.
Newcomb, C. J., Qafoku, N. P., Grate, J. W., Bailey, V. L., and De Yoreo, J. J.: Developing a molecular picture of soil organic matter–mineral interactions by quantifying organo–mineral binding, Nat. Commun., 8, 396, https://doi.org/10.1038/s41467-017-00407-9, 2017.
Nierop, K. G., Jansen, B., and Verstraten, J. M.: Dissolved organic matter, aluminium and iron interactions: precipitation induced by metal/carbon ratio, pH and competition, Sci. Total Environ., 300, 201–211, 2002.
Noll, L., Zhang, S., and Wanek, W.: Novel high-throughput approach to determine key processes of soil organic nitrogen cycling: Gross protein depolymerization and microbial amino acid uptake, Soil Biol. Biochem., 130, 73–81, https://doi.org/10.1016/j.soilbio.2018.12.005, 2019a.
Noll, L., Zhang, S., Zheng, Q., Hu, Y., and Wanek, W.: Wide-spread limitation of soil organic nitrogen transformations by substrate availability and not by extracellular enzyme content, Soil Biol. Biochem., 133, 37–49, https://doi.org/10.1016/j.soilbio.2019.02.016, 2019b.
Noll, L., Zhang, S., Zheng, Q., Hu, Y., Hofhansl, F., and Wanek, W.: Climate and geology overwrite land use effects on soil organic nitrogen cycling on a continental scale, Zenodo [data set], https://doi.org/10.5281/zenodo.7395605, 2022.
Norton, K. P., Molnar, P., and Schlunegger, F., The role of climate-driven chemical weathering on soil production, Geomorphology, 204, 510–517, 2014.
Padbhushan, R., Kumar, U., Sharma, S., Rana, D. S., Kumar, R., Kohli, A., Kumari, P., Parmar, B., Kaviraj, M, Sinha, A. K., Annapura, K., and Gupta, V. V.: Impact of Land-Use Changes on Soil Properties and Carbon Pools in India: A Meta-analysis, Front. Environ. Sci., 9, 794866, https://doi.org/10.3389/fenvs.2021.794866, 2022.
Peng, X. and Wang, W.: Stoichiometry of soil extracellular enzyme activity along a climatic transect in temperate grasslands of northern China, Soil Biol. Biochem., 98, 74–84, https://doi.org/10.1016/j.soilbio.2016.04.008, 2016.
Prommer, J., Wanek, W., Hofhansl, F., Trojan, D., Offre, P., Urich, T., Schleper, C., Sassmann, S., Kitzler, B., Soja, G., and Hood-Nowotny, R. C.: Biochar decelerates soil organic nitrogen cycling but stimulates soil nitrification in a temperate arable field trial, PLoS One, 9, e86388, https://doi.org/10.1371/journal.pone.0086388, 2014.
Pronk, G. J., Heister, K., and Kögel-Knabner, I.: Is turnover and development of organic matter controlled by mineral composition?, Soil Biol. Biochem., 67, 235–244, 2013.
Quiquampoix, H.: Mechanisms of protein adsorption on surfaces and consequences for extracellular enzyme activity in soil, in: Soil biochemistry, edited by: Stotzky, G., 1st Edn., CRC Press, 171–206, ISBN 9780429182372, 2000.
Quiquampoix, H. and Ratcliffe, R. G.: A 31P NMR study of the adsorption of bovine serum albumin on montmorillonite using phosphate and the paramagnetic cation Mn2+: modification of conformation with pH, J. Colloid Interf. Sc., 148, 343–352, https://doi.org/10.1016/0021-9797(92)90173-J, 1992.
R Development Core Team: R: A language and environment for statistical computing, R Foundation for Statistical Computing [code], https://www.r-project.org/ (last access: 22 June 2022), 2008.
Reich, P. B. and Oleksyn, J.: Global patterns of plant leaf N and P in relation to temperature and latitude, P. Natl. Acad. Sci. USA, 101, 11001–11006, https://doi.org/10.1073/pnas.0403588101, 2004.
Rosseel, Y.: The lavaan tutorial, https://lavaan.ugent.be/tutorial/ and https://github.com/yrosseel/lavaan/ (last access: 28 November 2022), 2018.
Rousk, J., Bååth, E., Brookes, P. C., Lauber, C. L., Lozupone, C., Caporaso, J. G., Knight, R., and Fierer, N.: Soil bacterial and fungal communities across a pH gradient in an arable soil, ISME J., 4, 1340–1351, 2010.
Schulten, H.-R. and Schnitzer, M.: The chemistry of soil organic nitrogen: a review, Biol. Fert. Soils, 26, 1–15, 1997.
Sinsabaugh, R. L., Lauber, C. L., Weintraub, M. N., Ahmed, B., Allison, S. D., Crenshaw, C., Contosta, A. R., Cusack, D., Frey, S., Gallo, M. E., Gartner, T. B., Hobbie, S. E., Holland, K., Keeler, B. L., Powers, J. S., Stursova, M., Takacs-Vesbac, C., Waldrop, M. P., Wallenstein, M. D., Zak, D. R., and Zeglin, L. H.: Stoichiometry of soil enzyme activity at global scale, Ecol. Lett., 11, 1252–1264, https://doi.org/10.1111/j.1461-0248.2008.01245.x, 2008.
Six, J. and Jastrow, J. D.: Organic matter turnover, Encyclopedia of soil science, edited by: Chesworth, W., Springer Verlag, 936–942, ISBN 978-1-4020-3994-2, 2002.
Staunton, S. and Quiquampoix, H.: Adsorption and conformation of bovine serum albumin on montmorillonite: Modification of the balance between hydrophobic and electrostatic interactions by protein methylation and pH variation, J. Colloid Interf. Sci., 166, 89–94, https://doi.org/10.1006/jcis.1994.1274, 1994.
Wanek, W., Mooshammer, M., Blöchl, A., Hanreich, A., and Richter, A.: Determination of gross rates of amino acid production and immobilization in decomposing leaf litter by a novel 15N isotope pool dilution technique, Soil Biol. Biochem., 42, 1293–1302, https://doi.org/10.1016/j.soilbio.2010.04.001, 2010.
Wattel-Koekkoek, E. J. W., van Genuchten, P. P. L., Buurman, P., and van Lagen, B.: Amount and composition of clay-associated soil organic matter in a range of kaolinitic and smectitic soils, Geoderma, 99, 27–49, https://doi.org/10.1016/S0016-7061(00)00062-8, 2001.
Wild, B., Schnecker, J., Barta, J., Capek, P., Guggenberger, G., Hofhansl, F., Kaiser, C., Lashchinsky, N., Mikutta, R., Mooshammer, M., Santruckova, H., Shibistova, O., Urich, T., Zimov, S. A., and Richter, A.: Nitrogen dynamics in Turbic Cryosols from Siberia and Greenland, Soil Biol. Biochem., 67, 85–93, https://doi.org/10.1016/j.soilbio.2013.08.004, 2013.
Xiao, W., Chen, X., Jing, X., and Zhu, B.: A meta-analysis of soil extracellular enzyme activities in response to global change, Soil Biol. Biochem., 123, 21–32, 2018.
Xu, X., Thornton, P. E., and Post, W. M.: A global analysis of soil microbial biomass carbon, nitrogen and phosphorus in terrestrial ecosystems, Global Ecol. Biogeogr., 22, 737–749, 2013.
Yaalon, D. H.: Soils in the Mediterranean region: what makes them different?, CATENA, 28, 157–169, https://doi.org/10.1016/S0341-8162(96)00035-5, 1997.
Yang, Y., Fang, J., Ma, W., and Wang, W.: Relationship between variability in aboveground net primary production and precipitation in global grasslands, Geophys. Res. Lett., 35, L23710, https://doi.org/10.1029/2008GL035408, 2008.
Zhang, L. and Altabet, M. A.: Amino-group-specific natural abundance nitrogen isotope ratio analysis in amino acids, Rapid Commun. Mass Sp., 22, 559–566, https://doi.org/10.1002/rcm.3393, 2008.