the Creative Commons Attribution 4.0 License.
the Creative Commons Attribution 4.0 License.
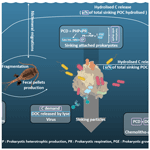
Reconstructing the ocean's mesopelagic zone carbon budget: sensitivity and estimation of parameters associated with prokaryotic remineralization
Robin Fuchs
Marc Garel
Jean-Christophe Poggiale
Laurent Memery
Frédéric A. C. Le Moigne
Christian Tamburini
Through the constant rain of sinking marine particles in the ocean, carbon (C) trapped within is exported into the water column and sequestered when reaching depths below the mesopelagic zone. Atmospheric CO2 levels are thereby strongly related to the magnitude of carbon export fluxes in the mesopelagic zone. Sinking particles represent the main source of carbon and energy for mesopelagic organisms, attenuating the C export flux along the water column. Attempts to quantify the amount of C exported versus C consumed by heterotrophic organisms have increased in recent decades. Yet, most of the conducted estimations have led to estimated C demands several times higher than the measured C export fluxes. The choice of parameters such as growth efficiencies or various conversion factors is known to greatly impact the resulting C budget. In parallel, field or experimental data are sorely lacking to obtain accurate values of these crucial overlooked parameters. In this study, we identify the most influential of these parameters and perform inversion of a mechanistic model. Further, we determine the optimal parameter values as the ones that best explain the observed prokaryotic respiration, prokaryotic production and zooplankton respiration. The consistency of the resulting C budget suggests that such budgets can be adequately balanced when using appropriate parameters.
- Article
(769 KB) - Full-text XML
-
Supplement
(797 KB) - BibTeX
- EndNote
The biological carbon pump (BCP) is the main mechanism by which CO2 is exported and stored in the deep ocean in the long term. This ecosystem service is defined as the sum of the biological processes that lead to carbon export from the euphotic zone into the deep ocean (Eppley and Peterson, 1979). This process exports 5 to 20 Gt C yr−1 in the form of particulate organic carbon (POC) gravitationally sinking from the sunlit ocean to the mesopelagic zone typically located between 200 and 1000 m (Henson et al., 2011). Therefore, atmospheric CO2 levels are strongly related to any change in carbon export into the mesopelagic zone (Kwon et al., 2009). Five downward pathways of organic matter export to the mesopelagic zone are defined: phytoplankton (senescent cells, colonies, spores, cysts), zooplankton (carcasses or fecal pellets), aggregates (marine snow of different compositions including the two latter categories), vertical migration of zooplankton, and mixing, diffusion, and advection (Siegel et al., 2016; Le Moigne, 2019).
Gravitationally sinking POC supply, combining the first three pathways described above, constitutes the main organic carbon input to the mesopelagic zone (Boyd et al., 2019). Consequently, the downward flux of organic carbon is attenuated with increasing depth as it is fragmented, metabolized and remineralized by different biological processes until only the refractory material remains. The majority of POC flux attenuation occurs in the mesopelagic zone (Martin et al., 1987; Marsay et al., 2015; Fuchs et al., 2022). The remineralization of exported carbon is mainly performed by two types of organisms: microorganisms (mostly heterotrophic prokaryotes, i.e., Bacteria and Archaea) and zooplankton. Heterotrophic prokaryotes primarily use dissolved organic carbon (DOC) as a source of carbon. However, some prokaryotes, colonizing particles upon formation, undergo changes in environmental conditions during their descent, such as the increase in the hydrostatic pressure and the variations in temperature (Tamburini et al., 2003, 2021; Baumas et al., 2021). Such particle-attached prokaryotes primarily use POC as a carbon source. Only organic matter of below 600 Da in size diffuses directly through prokaryotic membranes; therefore attached prokaryotes produce ectoenzymes required to solubilize larger molecules (Weiss et al., 1991). Smith et al. (1992) observed that the amount of DOC produced by ectoenzymatic solubilization of POC may be 10 to 100 times greater than the absorption capacity of a cell. DOC is thereby released into the surrounding water (the so-called solubilization). This increases the amount of DOC available for free-living prokaryotes. In addition, several types of zooplankton are involved in marine particles: POC-feeding detritivores (e.g., copepods), prokaryote consumers (e.g., flagellates) and carnivores (e.g., chaetognaths). Furthermore, zooplankton lose POC through excretion (moult, mucilage, urine), fecal pellets (decomposed organic matter) and sloppy feeding. Giering et al. (2014) specify that 30 % of a particle supplied by the downward flux is fragmented by the action of the detritivores and is transformed into suspended matter.
Given their importance regarding the BCP, all the processes described above were extensively studied in the last decades (e.g., Alldredge and Silver, 1988; Smith et al., 1992; Kiørboe et al., 2002, 2003; Kiørboe, 2003; Lampitt et al., 2008; Steinberg et al., 2008; Iversen et al., 2010; Giering et al., 2014; Koski et al., 2020; and references therein). However, the scientific community has struggled to reconcile the mesopelagic carbon budget with measurements and estimates showing a biological carbon demand often greater than the amount of known organic carbon sources (Reinthaler et al., 2006; Steinberg et al., 2008; Burd et al., 2010; Collins et al., 2015; Boyd et al., 2019). In other words, the measured export flux cannot sustain measured metabolic demands of prokaryotes and zooplankton altogether in the mesopelagic zone, leading to a discrepancy in C budgets.
A first explanation may lie in the choices of the boundaries of the mesopelagic zone used to integrate fluxes and to estimate the carbon budget as investigated in Fuchs et al. (2022). Indeed they specifically designed a method to determine from CTD-cast variables (fluorescence, O2 concentration, potential temperature, salinity and density; CTD: conductivity–temperature–depth) accurate boundaries which vary in space and time. With their method, named RUBALIZ, they show that 90 % of the POC flux attenuation occurs within newly determined boundaries, which is not the case of the fixed 200–1000 m often used. Furthermore, integrating prokaryotic C demand within RUBALIZ boundaries helps to reduce the discrepancy. Other sources of discrepancy may be found focusing on the carbon demand of prokaryotes (which are responsible for the final step of the remineralization), whose estimation is usually provided by adding rates of prokaryotic heterotrophic production (PHP) to that of prokaryotic respiration (PR) (Burd et al., 2010). PHP rates are often measured from tritiated leucine (Leu) incorporation rates in incubations, which are then multiplied by a Leu-to-carbon conversion factor (CF) (Kirchman et al., 1985). The PR is more challenging to measure (especially in the dark ocean; Nagata et al., 2010) and, therefore, often estimated from measurements of PHP and a prokaryotic growth efficiency (PGE) taken from the literature (as PR = PHP × (1 − PGE) PGE; del Giorgio and Cole, 1998). Unfortunately, in situ measurements of both CF and PGE are time-consuming and operationally complex to perform (especially for the mesopelagic zone). In addition, such data for particle-attached prokaryotic communities are scarce since the adequate sampling devices (to specifically sample biologically intact sinking particles) were only recently validated (Baumas et al., 2021). Furthermore, PHP and PR data are usually obtained after decompression or carried out from experiments at atmospheric pressure, being a source of misevaluation (Tamburini et al., 2013). As a result, mean values from global literature compilation or theoretical values are often used as references for both CF and PGE (Burd et al., 2010; Giering and Evans, 2022) and may be far from the actual in situ values.
In parallel, model predictions help to estimate unmeasurable processes along with the comparison and validation of data. The biological processes occurring in the mesopelagic zone are not yet well constrained (see sections above). Consequently, only a few models specifically designed to assess the fluxes governing the BCP in the mesopelagic zone exist (e.g., Tian et al., 2000; Anderson and Ryabchenko, 2009; Anderson and Tang, 2010; Fennel et al., 2022). For instance, the model developed by Anderson and Tang (2010) enables the evaluation of the remineralization of different compartments such as prokaryotes attached to sinking and suspended particles, free-living prokaryotes, and up to six trophic levels of zooplankton. This model describes the various known biological processes involved in the BCP system. However, the model is also required to be set up with parameters such as the PGE. For example, the Anderson–Tang model requires 20 parameters, which often present large uncertainties.
Giering et al. (2014) attempted to reconcile carbon input and biological carbon demand in the mesopelagic zone using the Anderson and Tang (2010) model and measurements carried out in the North Atlantic (Porcupine Abyssal Plain site; 49.0∘ N, 16.5∘ W; summer 2009). They found that prokaryotes were responsible for 70 %–92 % of the remineralization of organic carbon. In this study, the model results were consistent with the measurements performed in situ, showing a reconciliation of the carbon budget between 50 and 1000 m depths. Giering et al. (2014) balanced their C budget by using a rather low CF (CF = 0.44 kg C mol−1) compared to the one generally used in the literature (CF = 1.55 kg C mol−1) and a PGE of 8 % for free-living prokaryotes and 24 % for particle-attached prokaryotes. All these values were chosen as medians of literature values compiled from various measurement methods. Wisely choosing these parameter is therefore crucial to determine the reconciliation or the imbalance of carbon budget.
In this respect, we rely on model inversion methods (Tarantola, 2005) to provide meaningful estimations of parameters of interest. For a given phenomenon, inversion methods rely on a model taking as input the parameters to be estimated and whose outputs can be compared with in situ measurements. The inversion procedure thus gives the values of the parameters that best replicate the in situ measurements. This type of procedure has already been used in oceanography modeling. For instance, Saint-Béat et al. (2018) studied the phytoplankton marine food web in the Arctic, and Saint-Béat et al. (2020) examined pelagic ecosystems of two different zones in the Arctic Baffin Bay using the inversion method and sensitivity analyses to identify which biological processes impact the planktonic ecosystem functioning the most.
Here, we investigate the impact of widely but inadequately used parameters associated with the prokaryotic remineralization (e.g., CF, PGEs) on the magnitude of the discrepancy. Our aims are (1) to highlight the most sensitive parameters for which the determination of an accurate value is critical in the context of balancing the mesopelagic carbon budget, (2) to perform a mathematical inversion method to estimate the most plausible in situ values of the most sensitive parameters from a limited field dataset and (3) to discuss our results in the context of mesopelagic carbon budget.
2.1 In situ data
Most of the data used in this study originated from the DY032 (June–July 2015) cruise at the PAP (Porcupine Abyssal Plain) site in the North Atlantic on board the RRS Discovery. Some data unavailable for DY032 were estimated from a previous PAP cruise, D341 (July–August 2009). Most of the in situ data were compiled from already-published cruise data (e.g., Giering et al., 2014; Belcher et al., 2016; Baumas et al., 2021; Fuchs et al., 2022). Their post-treatments to suit our study framework are described below. Additionally, we used data (ectoenzymatic activities along with total hydrolyzable amino acids and carbohydrates and depth profile of heterotrophic prokaryotic production and respiration under in situ pressure versus atmospheric pressure) from the PEACETIME cruise (Guieu et al., 2020) that occurred in May 2017 in the Mediterranean Sea to illustrate some points in our discussions (see Supplement).
2.1.1 Carbon fluxes
(a) Determination of the active-mesopelagic-zone boundaries
Fuchs et al. (2022) introduced the “RUBALIZ” method, using CTD data, which allows the estimation of vertical boundaries targeting the zone of the dark ocean where most of the POC flux attenuation occurs. At the PAP station during cruise DY032, this so-called “active mesopelagic zone” was located between 127 and 751 m.
(b) Carbon inputs
The POC inputs to the active mesopelagic zone mainly involve the gravitational export of POC. Gravitational input was taken from Fuchs et al. (2022), who fitted a power law Martin curve (b of 0.84) to data obtained from 30 to 500 m using a marine snow catcher (Belcher et al., 2016). However, gravitational input is not the only POC input known in the literature. Recently, Boyd et al. (2019) provided an estimation of other particle-injection pumps (PIPs), such as the mixed-layer pump, physical pump or seasonal lipid pump, or the active transport related to metazoan migrations. At the PAP site during summer, only the eddy subduction pump, metazoan migrations and large-scale physical pumps were relevant to take into account. Other PIPs do not correspond to the location and season considered in our study. From the review of Boyd et al. (2019), these three particle-injection pumps seem to represent altogether around 52 % of the gravitational export of POC. We therefore add up this proportion of POC to the purely gravitational inputs. This yields an overall POC flux of 134 mg C m−2 d−1 exported into the active mesopelagic zone. The corresponding net POC input is 117 mg C m−2 d−1 (that is POC fluxes at the end – 751 m – of the active mesopelagic zone subtracted from the one at the start – 127 m – for PAP DY032).
DOC inputs are taken from Giering et al. (2014) and are considered to be the sum of direct DOC export via physical processes (advection–diffusion) and active flux from zooplankton migrations. We estimated from the extended data in their Fig. 2 that the DOC gradient below 100 m is hardly visible, meaning that physical vertical DOC export is insignificant for the active mesopelagic zone studied here. As a result, we set the DOC export at 3 mg C m−2 d−1, which corresponds only to the active flux from zooplankton migrations from Giering et al. (2014).
(c) Carbon demands
As explained above, prokaryotic carbon demand is generally assessed by adding rates of prokaryotic heterotrophic production (PHP) to that of prokaryotic respiration (PR). PHP of non-sinking prokaryotes (that is, free-living and suspended-particle-attached prokaryotes) are derived from leucine incorporation measurements on seawater samples and are taken from Fuchs et al. (2022). These data did not permit the separation of the free-living prokaryotes from those attached to suspended particles (Baumas et al., 2021). Hence, from here on, we no longer make this distinction and group both types under the term “non-sinking prokaryotes”. During DY032, marine snow catchers (MSCs) were deployed to separate slow- and fast-sinking particles from 100 L of samples (Riley et al., 2012; Baumas et al., 2021). PHP rates associated with prokaryotic communities of fast-sinking particles were taken from Baumas et al. (2021), and slow-sinking particles are presented here. Briefly, slow-sinking-particle fractions were sampled in the 7 L base of the MSC. Samples were incubated, and leucine incorporation rates were measured as for fast-sinking particles in Baumas et al. (2021). The formula described in Baumas et al. (2021) was then applied to normalize to 100 L as particles were concentrated in 7 L after 2 h of decantation and to remove the contribution of non-sinking prokaryotes, which were initially in this compartment around slow-sinking particles of interest. Total sinking-prokaryote PHP rates were obtained by adding both fast-sinking- and slow-sinking-prokaryote PHP rates. In addition, we were able to use the respiration rates of fast-sinking particle-attached prokaryote particles obtained at the PAP site during the DY032 cruise by Belcher et al. (2016). For each depth (30–500 m) the mean total O2 consumption per particle in nanomoles per particle per day was converted to milligrams of carbon per cubic meter per day (assuming a respiration quotient RQ (CO2 O2)= 1) by multiplying by the total number of particles (i.e., fecal pellets + phytoplanktonic aggregates) and dividing by 95 L, which is the volume of the MSC used (Riley et al., 2012). It is also important to note that PR for slow-sinking particles is missing. Thus, when we mention the respiration of sinking prokaryotes, only fast-sinking particle-attached prokaryotes are taken into account, which certainly underestimates the respiration used. All prokaryotic carbon demand (PHPs and PRs) estimates were integrated within RUBALIZ boundaries (i.e., 127–751 m). Non-sinking-prokaryote PHP rates were integrated using a piecewise model with a single node on the log data as described in Fuchs et al. (2022). Sinking-prokaryote PHP rates were integrated using a power law. Sinking PRs were integrated using a trapeze because data are only available until 500 m, and without any a priori curve shape, this method is certainly the most conservative.
Zooplankton activities are known to be related to POC concentration (Steinberg et al., 2008). Zooplankton respiration data were available only for the cruise D341, when the net POC input into the active mesopelagic layer was 59 mg C m−2 d−1 (including PIPs) instead of 134 mg C m−2 d−1 for DY032 (see above). For D341, zooplankton respiration integrated within the active mesopelagic zone (135–726 m; Fuchs et al., 2022) was 9 mg C m−2 d−1. Zooplankton respiration was integrated using a power law as in Giering et al. (2014). Zooplankton respiration data are missing for DY032; thus we consider this quantity as a percentage of the POC input that we calculate from the D341 dataset, i.e., 14.67 %. The zooplankton respiration value used here is therefore 17 mg C m−2 d−1.
Table 1Fluxes and their associated values used in this study. The Anderson–Tang model's terms (Anderson and Tang, 2010) corresponding to these fluxes are also shown. Values are integrated between 127 and 751 m, which are boundaries of the active mesopelagic zone defined by Fuchs et al. (2022). POC and DOC refer to particulate and dissolved organic carbon, respectively; PHP to prokaryotic heterotrophic production; and PR to prokaryotic respiration.
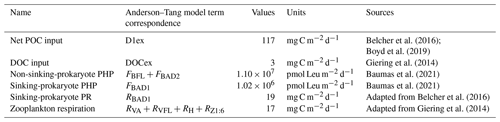
2.2 Mathematical methods
2.2.1 Parameter estimation
The scope of our study is to estimate in situ parameters by inverting the model introduced by Anderson and Tang (2010), adapted by Giering et al. (2014). We do not intend to present the model in detail here. The details of the equations constituting the version of the model used can be found in the original paper (Anderson and Tang, 2010) and in the R code available at https://github.com/RobeeF/InverseCarbonBudgetEstim (last access: 20 August 2023; https://doi.org/10.5281/zenodo.8412947), and the specific terms related to variables used are reported in Table 1. The model is calibrated by choosing the set of input parameters that yields the best fit between the model output and the data. As the model outputs 85 outfluxes, we used a subset of 4 measurable outfluxes to calibrate the model: the PHP of non-sinking prokaryotes, the PHP of sinking prokaryotes, the PR of sinking prokaryotes and the respiration of zooplankton. These fluxes have been chosen because of their near-direct correspondence with outputs of the model linked to the C demand of all groups (sinking prokaryotes, non-sinking prokaryotes, detritivores, bacterivores and carnivores).
Similarly, the model relies on 20 input parameters (Table S1 in the Supplement), which makes the parameter space of significant size and therefore challenging to explore. As such, we first determine the set of parameters that have the largest impact on the output of the model. Then for these parameters, the values that give the best fit between the data and the solution given by the model are determined.
(a) Sensitivity of the model to its inputs
In order to reduce the size of the input parameter space, Sobol indices (Sobol, 1993) were used to determine the most influential parameters. These indices enable quantification of the share of the variation in the output that can be imputed to each input parameter.
In essence, the first-order Sobol indices account for the direct influence of an input variable on the output. However, first-order Sobol indices neglect the interactions existing between this input variable and the other input variables. As such, in addition to the first-order Sobol indices, we used the total Sobol indices introduced by Homma and Saltelli (1996), which encompass both the direct effect of a parameter and also its interactions with the other parameters.
First-order and total Sobol indices were computed to quantify the influence of each parameter over each of the four outfluxes. Only the parameters which had significant Sobol indices (i.e., Sobol indices > 0.20) for at least one outflux were kept.
(b) Estimation of the parameters
The parameters which had no substantial effects on the output of the model were set to the values indicated by Anderson and Tang (2010) and Giering et al. (2014) and given in the Supplement (Table S1). The other parameters were estimated by minimizing the distance between the four outfluxes predicted by the model and their in situ measured counterpart. The distance chosen here is a standardized Euclidean distance:
where outfluxobs,i is the ith measured flux and outfluxmodel,i its modeled counterpart. The optimization method used is the Nelder–Mead algorithm (Nelder and Mead, 1965): if the function to minimize depends on N variables (the number of input parameters here), a simplex composed of N+1 points is defined. The coordinates of the simplex are updated in turn so that the simplex vertices become closer to the local minimum. Even if this method gives few theoretical guarantees of convergence, it has proven to work well in practice (Lagarias et al., 1998) and has the advantage that it does not require computation of the gradient of each outflux with respect to each input parameter.
As the model takes 20 inputs and outputs 85 fluxes, concerns might be raised about the uniqueness of the solution found to minimize the term (1). To make the model identifiable (i.e., sufficiently constrained to estimate the true value of the parameters), the number of input parameters to estimate is limited to the number of output fluxes available, here four. In this respect, the CFs have been fixed to 0.5 kg C mol Leu−1 (estimates without fixing the CFs have however been carried out; see Table S4). This value, contrary to the previously classically used value of 1.55 kg C mol Leu−1 (Simon and Azam, 1989; Nagata et al., 2010), was determined by Giering and Evans (2022) as the median value of 15 studies conducted in the mesopelagic zone. Doing so, we limit the number of free parameters to be estimated to four so that the model remains identifiable. The model is mostly linear, and our experiments have shown the solution to be unique and independent of the initial values taken.
The codes and data to reproduce the results are available at https://github.com/RobeeF/InverseCarbonBudgetEstim (https://doi.org/10.5281/zenodo.8412947).
3.1 Most sensitive parameters
Using Sobol indices, we identified the parameters from the 20 of the Anderson and Tang (2010) model most sensitive to the 4 flux outputs of the model for which we have the measured counterpart (i.e., PHP and PR of sinking prokaryotes, PHP of non-sinking prokaryotes, and respiration of zooplankton). All parameter definitions are given in Table S1. For the outflux “PHP of non-sinking prokaryotes”, only the PGEnon-sinking appears to be sensitive, with a Sobol index of 0.68, meaning that it explains 68 % of the variance (Table 2). Fluxes related to sinking prokaryotes, i.e., their PHP and their PR, appear to be highly influenced by Ψ, α and PGEsinking. For instance, our analysis yields indices of 0.22 and 0.23 for Ψ, 0.24 and 0.24 for α, and 0.27 and 0.25 for PGEsinking, respectively. Surprisingly, zooplankton respiration is more impacted by the PGEnon-sinking (Sobol index of 0.52) than proper zooplankton parameters. All other parameters exhibit Sobol indices below 1 %. Total Sobol indices, indicating the part of the variance of fluxes due to the parameter alone and in interaction with the others, were similar to the first-order indices, suggesting no interactions of parameters regarding the variance of fluxes. This sensitivity analysis enabled the identification of Ψ, α and both PGEs as the most influential parameters, suggesting that their values should be set with particular care, especially for PGEnon-sinking, which can be responsible for more than 50 % of the variance of PHPnon-sinking and zooplankton respiration. PGEs are growth efficiencies defined as the amount of new prokaryotic biomass produced per unit of organic C substrate assimilated and are a way to relate PHP and PR (del Giorgio and Cole, 1998). Ψ corresponds to the percentage of POC consumed by prokaryotes and α to the fraction of hydrolyzed POC which is lost into the surrounding water, i.e., not assimilated by sinking prokaryotes that hydrolyzed it.
Table 2First-order Sobol indices for the parameters of the model by Anderson and Tang (2010). The definition of each parameter can be found in Table S1. Significant Sobol indices (> 0.2) are shown in italics. PHP and PR refer to prokaryotic heterotrophic production and prokaryotic respiration, respectively.

3.2 Model inversion
The optimization method, described in the “Material and methods” section, enabled the determination of the four parameters identified as sensitive above: Ψ, α, PGEsinking and PGEnon-sinking in the case study of PAP DY032. Table 3 reports the combination found by model inversion. By construction of the procedure (e.g., same number of inputs and outputs), the solution is unique, explaining why no confidence intervals are reported. The errors between the four fluxes generated by the model and their measured counterparts were less than 1 %, far lower than potential measurement errors. The zooplankton flux was the best matched, followed by the PR of the sinking prokaryotes, the PHP of the non-sinking prokaryotes and the PHP of the sinking prokaryotes.
Table 3Estimation of the parameters Ψ, α, PGEsinking and PGEnon-sinking obtained by inversion of the model by Anderson and Tang (2010). As the model was made identifiable, the solutions are unique, explaining the absence of confidence intervals. The remaining differences between the model outfluxes deriving from the estimated input values and the actual in situ measurements are referred to as “errors” and are expressed in percentage. PHP, PR and ZR stand for prokaryotic heterotrophic production, prokaryotic respiration and zooplankton respiration, respectively.

3.3 C budget
The two PGEs presented above, along with a CF of 0.5 kg C mol Leu−1, were applied to leucine-incorporation-rate measurements to build the corresponding active mesopelagic C budget. The resulting C budget was compared with two other C budgets calculated with different sets of parameters. The three active-mesopelagic-zone C budgets resulting from DY032 measurements or estimation are represented in Fig. 1, with budget 1 obtained with the classical CF value of 1.55 kg C mol Leu−1 and median literature values for PGEs, i.e., 0.07 for PGEnon-sinking (Arístegui et al., 2005; Reinthaler et al., 2006; Baltar et al., 2010; Collins et al., 2015) and 0.02 for PGEsinking (Collins et al., 2015). Budget 2 was obtained with the parameter values from Giering et al. (2014), who reconcile the C budget, i.e., CF of 0.44 kg C mol Leu−1, PGEnon-sinking of 0.07 and PGEsinking of 0.24. Budget 3 was obtained with a CF, PGEsinking and PGEnon-sinking of 0.5, 0.026 and 0.087, respectively, determined in this study. The combination yielding the largest discrepancy is budget 1 (Fig. 1) (discrepancy of −194 mg C m−2 d−1). The C input seems to support the zooplankton respiration and total C demand of sinking prokaryotes but not the one of non-sinking prokaryotes, especially due to their PR of 218 mg C m−2 d−1. Combination of budgets 2 and 3 presented an excess of C (60 and 40 mg C m−2 d−1, respectively) compared to the biological C demand. These two differ mainly in terms of PR of sinking prokaryotes, which is negligible in combination 2 but which is the second-largest flux in the C demand in our study. In all cases, the C demand of non-sinking prokaryotes accounts for most of the total C demand.
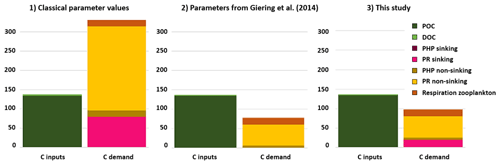
Figure 1Carbon budget for the active-mesopelagic-zone estimation resulting from DY032 measurements or estimation and the combination of CF (1.55, 0.44 and 0.5, respectively, for budget 1, 2 and 3), PGEsinking (0.02, 0.24 and 0.026, respectively, for budget 1, 2 and 3) and PGEnon-sinking (0.07, 0.08 and 0.087, respectively, for budget 1, 2 and 3) applied to leucine incorporation rates of sinking and non-sinking prokaryotes. See Supplement Fig. S1 for value details.
As stated in the Introduction, the scientific community has struggled to reconcile the mesopelagic carbon budget with measurements and estimates showing a carbon demand often greater than the amount of known organic C sources (e.g., Reinthaler et al., 2006; Steinberg et al., 2008; Burd et al., 2010; Collins et al., 2015; Boyd et al., 2019). Building C budget involves a plethora of parameters whose impacts are overlooked and often neglected, mainly because neither their ideal values nor their underlying mechanism in the water column across space and time is clearly understood. The scientific community is concerned about this issue (e.g., Burd et al., 2010; Giering and Evans, 2022), but in the absence of a better option and in an attempt to encourage comparisons, the same parameter values are universally used. A first step towards this direction was conducted thanks to the RUBALIZ method (Fuchs et al., 2022), which precisely determines the vertical location of the “active mesopelagic zone” and thereby estimates the boundaries between which to integrate C fluxes. In the current study, we pursue this investigation and combine measurements with modeling approaches to investigate the role of sensitive parameters related to the remineralization of POC in the mesopelagic zone.
4.1 Optimization method: consistency of parameters estimated
The Anderson and Tang (2010) model takes as inputs the measured C inputs as well as 20 parameters related to the activity of organisms such as sinking prokaryotes, non-sinking prokaryotes, zooplankton detritivores, bacterivores and carnivores. Among the 20 parameters, 4 have been found to be particularly sensitive in assessing the carbon demands of the various groups: Ψ (percentage of particle consumption by prokaryotes), α (percentage of hydrolyzed C released in surrounding water), and PGEnon-sinking and PGEsinking (growth efficiencies of sinking and non-sinking prokaryotes). It is interesting to note that zooplankton respiration (which is the sum of detritivore, bacterivore and carnivore respiration) is mostly sensitive to one parameter (PGEnon-sinking) but not to a parameter specific to zooplankton. This counter-intuitive result suggests a strong synergy between the two model compartments. At this point, it is challenging to establish whether this is the outcome of a complex ecological process or a model artifact.
In the model, the consumption of particles is done by two groups: prokaryotes (Ψ) and detritivores (1−Ψ). It can be estimated by taking the average ratio between PHP and ZR. Anderson and Ryabchenko (2009) estimated Ψ using calculations of POC consumption by prokaryotes and zooplankton between 150 and 1000 m performed by Steinberg et al. (2008) in the Pacific. Following this, they set Ψ at 0.76. The inversion of the Anderson and Tang (2010) model leads to a well-identified solution of Ψ, i.e., 0.67 in the case of the PAP DY032 cruise. This value is in line with the one used by Anderson and Tang (2010). However, data are lacking to compare and explore variations in Ψ values across seasons, locations or depths. In the model, Ψ participates in the repartition of POC input between prokaryotes and detritivores. Whether for modeling purposes to determine Ψ or to build a C budget without a model, PHP and ZR are required. It remains too rare to have both together, and more future efforts should be devoted to get PHP and ZR concomitantly.
Beyond Ψ, according to Sobol indices, α is the second parameter of interest. When prokaryotes consume POC using hydrolytic enzymes, a major fraction of the hydrolyzed C is lost to the surrounding environment as DOC (Smith et al., 1992; Vetter et al., 1998). This loss is represented by α and is very difficult to quantify accurately. Two major experiments, focused on amino acid hydrolysis, aimed to determine such losses: Smith et al. (1992) and Grossart and Ploug (2001). Smith et al. (1992) sampled particles at 25 m and showed that 97 % of particulate combined amino acids are released in the surrounding water. Later, Grossart and Ploug (2001) using aggregates from phytoplankton cultures show a loss of POC of 74 %. Relying on these two studies, Anderson and Tang (2010) followed by Giering et al. (2014) consider that the value should be lower than that of a fresh detritus and choose a conservative value of 0.5. In the case of these two experiments, only the amino acids are considered, and the experiments were conducted under laboratory-controlled settings. However, both amino acids and sugar are major components of POC, constituting between 40 % and 70 % of POC in the mesopelagic zone (Wakeham et al., 1997). Conversely, we used unpublished data from the PEACETIME cruise (see method details in the Supplement) of in situ hydrolysis rates of aminopeptidase and β-glucosidase from sinking prokaryotes (which hydrolyze amino acids and sugar, respectively) that we were able to convert into hydrolyzed carbon fluxes (see measurements and calculation details in the Supplement). Unfortunately, total hydrolyzed C fluxes were below the C demand of the sinking prokaryotes most of the time, which is unrealistic and probably due to the low amount of POC (sinking POC concentration of < 1 mg L−1 in the sinking fraction), resulting in insufficient sinking-prokaryote abundance to detect their activity by volume. However, when total hydrolyzed C fluxes were superior to PHPsinking (indicating that some hydrolyzed C is not assimilated and is released), α was estimated between 0.19 and 0.79 with a mean of 0.41±0.24 and seems to decrease with depth (see calculation details in the Supplement). This could confirm the work of Grossart and Ploug (2001) showing that the older a detritus is, the less enzymatic activity there is and therefore the less amino acid loss. Even if α is not easily measurable, this parameter is identified at 0.78 by the inversion method during a post-bloom period at the PAP site. This value is consistent with Smith et al. (1992) and Grossart and Ploug (2001), evidencing high α for surface aggregates (0.97) with laboratory-made phytoplankton aggregates (0.74), or with our calculations for the Mediterranean Sea (0.41±0.24), an oligotrophic region. This suggests that the optimization method is a relevant alternative to determine α. In addition, α corresponds to a release of C in the surrounding water. Regarding the model, the C demand of free-living prokaryotes matches the hydrolyzed C released, which constitutes their main C sources. The relationship between enzymatic activities and heterotrophic production of free-living prokaryotes is well documented in the deep-sea ocean (Cho and Azam, 1988; Smith et al., 1992; Hoppe and Ullrich, 1999; Tamburini et al., 2002, 2003; Nagata et al., 2010). Total C demand of non-sinking prokaryotes is challenging to measure due to the diversity of existing methods, especially the PR (e.g., Table S2), which leads to an incredibly wide range of estimated values. Subsequently, identifying α via the optimization method could help to avoid these conflicting PR measurements.
The last two sensitive parameters according to Sobol indices were PGEnon-sinking and PGEsinking. A wide range of PGEnon-sinking has been estimated using PHPnon-sinking and PRnon-sinking in the open ocean (e.g., Sherry et al., 1999; Lemée et al., 2002; Carlson et al., 2004; Arístegui et al., 2005; Reinthaler et al., 2006; Baltar et al., 2009, 2010; Collins et al., 2015). Overall it varies from 0.001 to 0.64 (Collins et al., 2015, and Sherry et al., 1999, respectively). However, these values were produced from different protocols for the PHP (changes in biomass, thymidine or leucine incorporation, each with its own conversion factors and/or constants) and for the PR methods (by electron transport system – ETS – measurements, micro-winkler titration, changes in dissolved O2 or using optode sensor spots; see Table S2) and correspond to various locations, seasons and depths. These are all valid reasons that can potentially explain the stark contrast in the values reported. If one focuses only on the mesopelagic zone in the North Atlantic, the median is 0.07 (Arístegui et al., 2005; Reinthaler et al., 2006; Baltar et al., 2010; Collins et al., 2015). The optimization method yielded a value of 0.087 and therefore produces very consistent results for a post-bloom period at the PAP site. Concerning PGEsinking, too few values are available. To our knowledge, only Collins et al. (2015) provided in situ values associated with sinking prokaryotes (from 0.01 to 0.03) at 150 m. This is the only comparison we have, and our value of 0.026 matches this order of magnitude. As a further comparison, the non-integrated data from DY0312 allows us to calculate a PGEsinking using PGEsinking= PHPsinking (PHPsinking + PRsinking) according to del Giorgio and Cole (1998). The result is thus a depth-specific PGE instead of a depth-integrated PGE. This led to a variation of 0.033 at 70 m to 0.0013 at 500 m. Although the lack of data points deeper than 500 m and the low number of points force us to stay cautious about these estimates, it may indicate that PGEsinking is not constant throughout the mesopelagic zone and decreases with depth. Constraining conditions due to the increase in hydrostatic pressure and decrease in temperature experienced by prokaryotes attached to sinking particles could explain this decrease in PGEsinking (Stief et al., 2021; Tamburini et al., 2021). Under highly constrained conditions, Russell and Cook (1995) explained that maintaining respiration at the highest possible rate would allow the supply of active membrane transporters which are vital to the cell. This implies a low but optimal PGE (Westerhoff et al., 1983), which could thus decrease with depth and time as the POC becomes less labile (Grossart and Ploug, 2000). In contrast, the Anderson and Tang (2010) model and the associated model inversion presented here are built so that the mesopelagic zone is considered to be one homogeneous entity. Explicitly, specifying depth-dependent PGEsinking in the mesopelagic zone could lead to more realistic modeling but would entail additional non-negligible model complexity.
It is worth noting that the PGEsinking and PGEnon-sinking estimated here rely on a leucine-to-carbon conversion factor (CF) of 0.5 kg C mol Leu−1. This value comes from the median of 15 values obtained on the free-living prokaryotes of the mesopelagic zone (between 300 and 1000 m), which do not sink and are adapted to their place in the water column (Giering and Evans, 2022). However, to our knowledge, there are no such values measured for the specific case of sinking prokaryotes. The latter are surface prokaryotes that have attached to the particles and will experience changes in conditions (e.g., pressure, temperature) linked to their sink (Baumas et al., 2021; Tamburini et al., 2021). The CF depends, among other things, on the leucine fraction in the proteins and the cellular carbon protein ratio (Kirchman and Ducklow, 1993). It is known that stresses can affect the incorporation of leucine into proteins and general protein production (e.g., Young, 1968; Welch et al., 1993) and that these parameters can vary with prokaryotic diversity, especially between Bacteria and Archaea (Bogatyreva et al., 2006). Stresses occur during the descent throughout the water column, and sinking prokaryotes experienced a drastic decrease in diversity following the sink at PAP DY032 (Baumas et al., 2021; Tamburini et al., 2021). We can therefore easily imagine that the CF for sinking prokaryotes could be impacted. Despite this, without having further data, we applied the same CF to sinking as the 0.5 recommended by Giering and Evans (2022) for non-sinking prokaryotes.
4.2 Influence on mesopelagic C budget
As stated in the introduction, mesopelagic C budgets are constructed by applying a CF and a PGE on leucine incorporation rate data to assess prokaryotic C demand. In Fig. 1, we apply three different combinations of CFs and PGEs to the same data. The combination using a conventional CF of 1.55 kg C mol Leu−1, PGEnon-sinking of 0.07 and PGEsinking of 0.02 led to an aberrant discrepancy such that more than the entire C pool would be remineralized in the active mesopelagic zone and that there would be no source of C to sustain deeper zone life nor sequestration by the BCP. As stated above, this was a recurrent issue in the field (Reinthaler et al., 2006; Steinberg et al., 2008; Burd et al., 2010; Collins et al., 2015; Boyd et al., 2019), with the exception of Giering et al. (2014), who reconcile the C budget of the mesopelagic zone. The results of Giering et al. (2014) were mainly due to the difference in CF applied to their data, i.e., 0.44 kg C mol Leu−1. However, from a model point of view, the main difference between C budgets estimated using the parameters of Giering et al. (2014) and those determined by our optimization method is due to the 10-fold difference between the PGEsinking used. Giering et al. (2014) used 0.24, which is the mean of a 14 d incubation experiment during which PGE varied from 0.45 in the first 3 d to 0.04 at the end for riverine aggregates (Grossart and Ploug, 2000). Despite the fact that PGEsinking data are very scarce, riverine values of 0.24 seem highly unlikely and inappropriate for mesopelagic sinking prokaryotes compared to what is known in marine environments (e.g., Collins et al., 2015). Indeed, if we consider that enzymes account for a large proportion of the proteins produced by cells (see above) the PGEsinking must be low due to the high metabolic cost of their production (Grossart and Ploug, 2000). Finally, the C budget built from a combination of CFs of 0.5 kg C mol Leu−1 and PGEs revealed by our optimization method seems the most reasonable option (from the three budgets built; Fig. 1), with an excess C input of 40 mg C m−2 d−1. In this case, PGEs were determined by the model, which in addition to PHP and PR of sinking and non-sinking prokaryotes and zooplankton respiration also accounts for the production of zooplankton biomass in the calculations. We do not have measurements or estimates for the production of zooplankton biomass, but based on the model, this biomass production is 11 mg C m−2 d−1. Adding this value to the C demand implies a leftover of 29 mg C m−2 d−1 that is not used and is exported below the active mesopelagic zone via gravitationally sinking POC. This value is in accordance with the POC flux estimated from measures at 751 m (thus at the exit of our zone): 17 mg C m−2 d−1. Being aware of the biases that may exist in the fluxes used as well as in the construction of the model itself, our optimization method enables the determination of realistic values of parameters and thus the construction of robust C budgets. As far as we know, the combination of field measurements using consistently defined integration depths, such as RUBALIZ (Fuchs et al., 2022) with the use of optimization method in the Anderson–Tang model, has led to the most complete and realistic mesopelagic carbon budget.
4.3 Model: reliability and potential biases
The Anderson–Tang model (Anderson and Tang, 2010) was originally parameterized with 20 input parameters and 85 output fluxes and is hence by definition an underdetermined model as the number of outputs is higher than the number of inputs. To make the model identifiable, i.e., to obtain unique solutions for each parameter value, the number of parameters allowed to vary, namely Ψ, α, PGEnon-sinking and PGEsinking, was restricted to the number of measurable outputs (here four: PHPsinking, PRsinking, PHPnon-sinking and zooplankton respiration). Measurement errors (e.g., measurement device errors, in situ variabilities, errors due to integration methods) are typically challenging to characterize. Furthermore, even if these four outfluxes describe the prokaryotic and zooplankton compartment fluxes well, one may wonder about the sensitivity of the results to the fact that a given outflux is not available or estimated with error.
As a result, we have tested two settings: a model inversion without the zooplankton respiration flux (using only three fluxes) and a second setting where the PGEs were estimated from the leucine incorporation rate using freely varying CFs, i.e., with CFs no longer fixed at 0.5 as a value. The results are reported in Tables S3 and S4. Not using the zooplankton flux to inverse the model mechanically adds some variability to the estimation results, especially concerning Ψ, α and PGEnon-sinking, in decreasing order of variability (Table S3). The PGEsinking was not affected as its confidence interval length was inferior to 10−7: this underlines the very limited interaction between the zooplankton and sinking-prokaryote compartments in the model (contrary to the zooplankton and non-sinking-prokaryote compartments). Yet, the difference between the four-flux and three-flux parameter estimations was negligible (< 1 % variation for each estimate), highlighting the robustness of the estimates to the potential unavailability of the zooplankton respiration. In contrast, as made visible in Table S4, not fixing the CFs to estimate the PGEs created more variations in the PGE estimations, while the estimations of Ψ and α changed by less than 5 % with respect to Table 2 estimations. The PGEs of the attached and free-living parameters become significantly closer to their fixed boundaries (10 %), while the CFs rise, especially the CF of the attached particles (= 1.87 kg C mol Leu−1). Similarly, if PGEs are no longer bounded, the estimates of PGEs (0.17 for attached prokaryotes and 0.23 for free-living prokaryotes) and CFs (3.93 kg C mol Leu−1 for attached prokaryotes and 1.53 kg C mol Leu−1 for free-living prokaryotes) become unrealistic. This can be explained by the fact that the PGEs and CFs play similar mathematical roles in the current formulation of the model. Hence, without additional fluxes ensuring full model identifiability, one of these two types of quantities needs to be fixed to estimate the other.
In addition to these sensitivity analyses, an uncertainty analysis has been run by simulating errors in the measurements of the POC, DOC and the four output fluxes (see Table S5). Simulating errors from −10 % to 10 % for each flux, the estimation of the four parameters of interest was hardly affected: 1 %, 2 %, 3 % and 1 % on average for the Ψ, PGEsinking, PGEnon-sinking and α, respectively. The PGEnon-sinking was mostly sensitive to measurement errors in POC flux, DOC flux and PHPnon-sinking (generating variations of 6 %, 5 % and 5 %, respectively). Similarly, the PGEsinking was logically mostly sensitive to errors in the PHPsinking and PRsinking (generating variations of 6 % for both). For the measurement errors, the generated variations all remained under 3 %, which is reassuring concerning the stability of the estimation.
Finally, the last potential source of estimation bias results from the assumed stationarity hypothesis of the mesopelagic system. For logistical and technical reasons, measurements and sampling between the upper and lower boundary of the mesopelagic zone are typically performed simultaneously. The stationarity assumption is thus a natural foundation grounded in interpretations and models. However, there is a temporal delay in flux variations between the upper layer and lower measurements (Giering et al., 2017; Stange et al., 2017). This delay depends on the particles' sinking speed, typically ranging from 2 to 1500 m d−1 (Alldredge and Silver, 1988; Armstrong et al., 2002; Trull et al., 2008; Turner, 2015); morphotype; density; porosity; and the timing of their production. Strong meteorological events can also perturbate C fluxes from the water column with an increasing time lag over depth (e.g., Pedrosa-Pàmies et al., 2019). Admittedly, C budgets suffer from lack of time integration into the analysis. Our study regarding the PAP site is also concerned as it undergoes a substantial seasonality (Cole et al., 2012; Giering et al., 2017). Although, we do not have enough understanding of vertical time lag to change the model and to avoid such bias yet. Some long-term observatories such as BATS in the Bermuda Atlantic or HOT in Hawaii provide biogeochemical flux time series, but monthly sampling focuses mostly on the euphotic zone and does not investigate the mesopelagic zone enough. Sampling at discrete times following the sink of a bloom (e.g., Le Moigne et al., 2016) could be a solution, which would nevertheless entail a significant cruise planning effort.
4.4 Grounds for improvements
The Anderson–Tang model allowed us to have a comprehensive vision of the remineralization processes in the mesopelagic zone by including the interactions between various compartments, completing in situ measurements with a comprehensive vision of the mechanisms at stake. The described inversion of the Anderson–Tang model provided meaningful estimations of the parameters of interest. However, as most models represent complex phenomena, some processes are not fully and properly captured by the model. Below, we provide a list of processes that may help in refining mesopelagic C budget estimations.
4.4.1 Other microorganisms
The roles of microbial eukaryotes, viruses and the input of C by chemolithotrophs (Herndl and Reinthaler, 2013; Lara et al., 2017; Kuhlisch et al., 2021; Luo et al., 2022) are not included in the model. For instance, eukaryotes can dominate microbial biomass on bathypelagic particles (Bochdansky et al., 2017) and have the potential to promote the aggregation of particles (Jain et al., 2005; Chang et al., 2014; Hamamoto and Honda, 2019; Xie et al., 2022). Viruses could be the main cause of prokaryotic and phytoplanktonic mortality. Thus, DOC fluxes could be attributed to them, in particular with the cell lyses they provoke (Fuhrman, 2000, and references within; Lara et al., 2017; Kuhlisch et al., 2021). In the North Atlantic, 9 % to 12 % of cells could be infected by viruses, which would cause a DOC production of 0.1 mg C m−3 d−1 (Wilhem and Suttle, 1999). For comparison, PHP results for PAP before integration (with a conversion factor of 0.5 kg C mol−1 Leu) were mostly below this value. In addition, inorganic C fixation by chemoautotrophy would be of the same order of magnitude as PHPnon-sinking rates (Herndl et al., 2005; Reinthaler et al., 2010). It would be important to verify what the contributions of microbial eukaryotes, chemolithotrophs or viruses are, even if the poor understanding of these processes currently prevents their proper integration into models.
4.4.2 Lifestyles
Sinking prokaryotes are poorly considered as they are not sampled with the Niskin bottles classically used in oceanography (Planquette and Sherrell, 2012; Baumas et al., 2021). However, the use of the MSC at PAP DY032 allows us to access fractions of particulate organic carbon that will allow us to evaluate the importance of sinking prokaryotes. We have seen that their C demand is not negligible and represents 18 % of total C demand. The Anderson–Tang model distinguishes sinking particles from neutrally buoyant particles, each with distinct attached communities. Since sampling with MSC only allows us to separate what is sinking from what is not, we merged free-living prokaryotes with those attached to neutrally buoyant particles without distinction. However, unlike free-living prokaryotes, prokaryotes attached to neutrally buoyant particles have access to POC and must produce enzyme activity with different metabolisms than their free-living counterparts. In contrast, prokaryotes attached to neutrally buoyant particles are also different from prokaryotes attached to sinking particles since they do not undergo changes in temperature and pressure related to the sink. They must therefore surely have intrinsically different PGE and associated remineralization rates. It would therefore be valuable to consider them as a third distinct group in laboratory experiments and sampling. Contrary to the sinking or ascending particles, which are naturally split by their sinking and ascending velocity (e.g., respectively Smith et al., 1989; Cowen et al., 2001; McDonnell et al., 2015), no means allow the selective and exclusive sampling of neutrally buoyant particles. The only valid way is to use the MSC to let the sinking particles fall into the lower compartments and to filter the “non-sinking” part to retain the particulate fraction. However, it is known that filtration affects the activities of prokaryotes and generates biases (Edgcomb et al., 2016). This makes investigations of prokaryotes associated with neutrally buoyant particles particularly challenging, and future endeavors should urgently attempt to target them.
4.4.3 OC inputs
Continuing along the same lines, the inputs of C that the model takes into account are only the gravitational POC and the DOC. We chose to artificially increase the gravitational POC flux to add sources of neutrally buoyant particles in the form of PIPs (eddy subduction pump, metazoan migrations and large-scale physical pumps). Indeed, Boyd et al. (2019) clearly showed that these PIPs can be of paramount importance (here we have estimated them at 51.6 % of the gravitational flux). Yet, explicitly describing them in a dedicated compartment of the model could be an improvement for future research, as these neutrally buoyant particles have an effect on the whole system, including the prokaryotes linked to various types of particles and their predators or on particle fragmentation. Given the existence of the neutrally buoyant particle compartment, it is feasible to adapt the model to account for these C inputs. This is even more relevant as new optical instruments have flourished (e.g., Briggs et al., 2013; Giering et al., 2020; Picheral et al., 2022) and would make it easier to better quantify these neutrally buoyant particle fluxes.
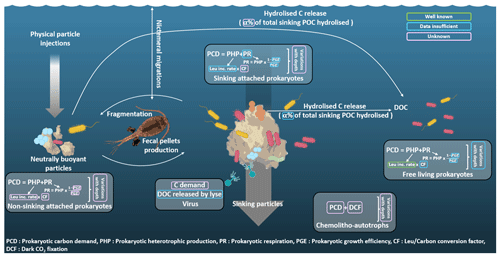
Figure 2Sinking particles export carbon (C) down to the mesopelagic zone through gravitational POC fluxes, where the latter is attenuated to satisfy C demand of different groups of organisms such as prokaryotes living attached to sinking particles, prokaryotes attached to non-sinking particles or free-living prokaryotes. In turn, viruses and chemoautotrophs can increase the amount of usable labile C. Quantifying C demand and role of POC fluxes of these different groups is crucial to truly assess C sequestration in the deeper layer of the water column. However, a multitude of uncertainties remain for each group. The quantities enclosed in green are well known, those in blue lack data, and those in pink are unknown. C demand is the sum of heterotrophic production (PHP) and respiration (PR). The understanding of these two quantities is currently better for the free-living prokaryotes, whereas data are still insufficient for sinking prokaryotes and even absent for prokaryotes attached to non-sinking particles. Moreover, to build C budgets, these variables are integrated over a few hundred meters of water column, and the relationship between in situ pressure and C demand often remains neglected even if this relationship highly depends on the prokaryote type considered (not constant for sinking prokaryotes unadapted to the increased pressure; constant for free-living prokaryotes well adapted to their living depth; and constant for prokaryotes attached to non-sinking particles, which can be adapted or not if the particle was sinking before being stopped in its sink).
4.4.4 In situ pressure effect
Our last major concern deals with the fact that neither Niskin nor MSC avoids disruption introduced through the process of depressurization when samples are collected at depth (Tamburini et al., 2013; Garel et al., 2019). Heterotrophic activities associated with non-sinking prokaryotes are known to decrease with depth but were mostly sampled without taking care of the in situ pressure (e.g., Turley and Mackie 1994; Arístegui et al., 2009). From our knowledge, some devices such as the IODA6000 (Robert, 2012) were specifically designed to measure in situ PR of non-sinking prokaryotes. However, enigmatically high PR values (2–3 orders of magnitude higher than PHP) are measured by IODA6000, making it difficult to have confidence in these in-situ-measured PR rates. During the PEACETIME cruise, we use a pressure-retaining sampler (methods presented in the Supplement), allowing for the first time access to both PHPnon-sinking and PRnon-sinking rates and comparison with classical depressurization procedures (Fig. S1). We observed that activity rates of non-sinking prokaryotes kept under pressure were always higher when kept at in situ hydrostatic pressure than their decompressed counterparts and, surprisingly, seem to increase with depth rather than decrease, as typically depicted and found when the samples are decompressed (Fig. S1). From a C budget point of view, taking in situ pressure into account will increase C demand of free-living prokaryotes well adapted to their living depth.
The effect of pressure acts inversely on sinking prokaryotes, as they are surface prokaryotes (unadapted to high hydrostatic pressure) that undergo a dynamic pressure increase as the particle sinks (Baumas et al., 2021; Tamburini et al., 2021). Furthermore, repeated results (Tamburini et al., 2006, 2009, 2021; Riou et al., 2018) have shown that, while performing a sinking simulation experiment, the activities of sinking prokaryotes are affected during the sink. For instance, they noticed that the aminopeptidase activity was always lower with increasing pressure over time than at atmospheric pressure on diatom aggregates (Tamburini et al., 2006).
Handling high-pressure sampling or experiments requires much more effort and material than usual methods. However, it seems highly worthy when investigating both sinking- and non-sinking-prokaryote activities with regard to C budget purposes.
By combining in situ data from the DY032 cruise at the PAP site with inversion of the Anderson–Tang model, which includes known processes from the biological C pump, we provide robust and ecologically realistic estimates of key parameters to better characterize the patterns at stake.
-
We showed that the most sensitive parameters in the model are the ones related to prokaryotes such as prokaryotic growth efficiencies and C hydrolyzed by sinking prokaryotes released to the surrounding water.
-
By inversion of the Anderson–Tang model, we determined consistent values of the parameters listed above.
-
We showed that using these values instead of the classical mean from the literature or inadequate theoretical values resulted in a more consistent and realistic C budget than previously considered.
-
Additional measurements are needed to better understand both prokaryotic growth efficiencies and leucine-to-carbon conversion factors in the mesopelagic zone. However, we recommend measuring fewer fluxes which we are confident are associated with inversion model procedures in order to access parameter values challenging to measure for other places, cruises or seasons.
Figure 2 summarizes processes involved in mesopelagic C budget estimations and highlights missing knowledge. We attempt to classify the processes according to their degree of understanding (well known, insufficient data or unknown) and point out that the majority of these processes require a better understanding. Among other things, it is crucial to quantify the roles of microbial eukaryotes, viruses and chemoautotrophs in the entire process of C budgets. Suspended particles should have a dedicated well-identified compartment in future studies instead of being neglected and drowned in others. Finally, accounting for in situ hydrostatic pressure when studying prokaryotic C demand is key. This is because (1) it may reduce prokaryotic carbon demand for sinking prokaryotes unadapted to increasing pressure, and (2) it may increase PCD for free-living prokaryotes well adapted to their living depth.
The codes and data to reproduce the results are available at https://github.com/RobeeF/InverseCarbonBudgetEstim (https://doi.org/10.5281/zenodo.8412947, RobF, 2023).
The supplement related to this article is available online at: https://doi.org/10.5194/bg-20-4165-2023-supplement.
The idea was conceived by CB, CT and JCP. Sampling and experiments onboard the PEACETIME cruise were conducted by CT and MG. The processing of PAP DY032 data was conducted by CB with advice from FLM, and the processing of PEACETIME data was conducted by CB and MG. RF designed the inversion detection methodology and performed the estimation with advice from LM. CB and RF led the writing, with significant contributions from all authors.
The contact author has declared that none of the authors has any competing interests.
Publisher’s note: Copernicus Publications remains neutral with regard to jurisdictional claims made in the text, published maps, institutional affiliations, or any other geographical representation in this paper. While Copernicus Publications makes every effort to include appropriate place names, the final responsibility lies with the authors.
We thank the crew and officers of the RRS Discovery (NERC) for their help during the PAP DY032 cruise and of the N/O Pourquoi Pas? during the PEACETIME cruise. This study is a contribution to the PEACETIME project (MISTRALS CNRS INSU; https://doi.org/10.17600/17000300) managed by Cécile Guieu (LOV) and Karine Desboeufs (LISA). We warmly thank France Van Wanbeke, Sophie Guasco and Birthe Zancker for onboard work (enzymatic activities, sugar and amino acid concentrations measurements) during the PEACETIME cruise. We wish to express our gratitude to France Van Wanbeke, Sarah Giering, Tom Anderson and Anna Belcher for stimulating and informative discussions. Chloé Baumas would also like to thank Yves Williatte for sharing his expertise throughout the years. These moments of complicity transformed her overall perception of mathematics, and they continue and will continue to influence her work such as in this paper. Merci Papy.
This paper is a contribution of the APERO project funded by the National Research Agency (grant no. ANR-21-CE01-0027) and by the French LEFE Cyber program.
This paper was edited by Jack Middelburg and reviewed by two anonymous referees.
Alldredge, A. L. and Silver, M. W.: Characteristics, dynamics and significance of marine snow, Prog. Oceanogr., 20, 41–82, https://doi.org/10.1016/0079-6611(88)90053-5, 1988.
Anderson, T. R. and Ryabchenko, V. A.: Carbon cycling in the Mesopelagic Zone of the central Arabian Sea: Results from a simple model, Washington DC American Geophysical Union Geophysical Monograph Series, 185, 281–297, https://doi.org/10.1029/2007GM000686, 2009.
Anderson, T. R. and Tang, K. W.: Carbon cycling and POC turnover in the mesopelagic zone of the ocean: Insights from a simple model, Deep-Sea Res. Pt. II, 57, 1581–1592, https://doi.org/10.1016/j.dsr2.2010.02.024, 2010.
Arístegui, J., Duarte, C. M., Gasol, J. M., and Alonso-Sáez, L.: Active mesopelagic prokaryotes support high respiration in the subtropical northeast Atlantic Ocean, Geophys. Res. Lett., 32, L03608, https://doi.org/10.1029/2004GL021863, 2005.
Arístegui, J., Gasol, J. M., Duarte, C. M., and Herndld, G. J.: Microbial oceanography of the dark ocean's pelagic realm, Limnol. Oceanogr., 54, 1501–1529, https://doi.org/10.4319/lo.2009.54.5.1501, 2009.
Armstrong, R. A., Lee, C., Hedges, J. I., Honjo, S., and Wakeham, S. G.: A new, mechanistic model for organic carbon fluxes in the ocean based on the quantitative association of POC with ballast minerals, Deep-Sea Res. Pt. II, 49, 219–236, https://doi.org/10.1016/S0967-0645(01)00101-1, 2002.
Baltar, F., Arístegui, J., Gasol, J. M., Sintes, E., and Herndl, G. J.: Evidence of prokaryotic metabolism on suspended particulate organic matter in the dark waters of the subtropical North Atlantic, Limnol. Oceanogr., 54, 182–193, https://doi.org/10.4319/lo.2009.54.1.0182, 2009.
Baltar, F., Arístegui, J., Sintes, E., Gasol, J. M., Reinthaler, T., and Herndl, G. J.: Significance of non-sinking particulate organic carbon and dark CO2 fixation to heterotrophic carbon demand in the mesopelagic northeast Atlantic, Geophys. Res. Lett., 37, L09602, https://doi.org/10.1029/2010GL043105, 2010.
Baumas, C. M. J., Le Moigne, F. A. C., Garel, M., Bhairy, N., Guasco, S., Riou, V., Armougom, F., Grossart, H.-P., and Tamburini, C.: Mesopelagic microbial carbon production correlates with diversity across different marine particle fractions, ISME J., 15, 1695–1708, https://doi.org/10.1038/s41396-020-00880-z, 2021.
Belcher, A., Iversen, M., Giering, S., Riou, V., Henson, S. A., Berline, L., Guilloux, L., and Sanders, R.: Depth-resolved particle-associated microbial respiration in the northeast Atlantic, Biogeosciences, 13, 4927–4943, https://doi.org/10.5194/bg-13-4927-2016, 2016.
Bochdansky, A. B., Clouse, M. A., and Herndl, G. J.: Eukaryotic microbes, principally fungi and labyrinthulomycetes, dominate biomass on bathypelagic marine snow, ISME J., 11, 362–373, https://doi.org/10.1038/ismej.2016.113, 2017.
Bogatyreva, N. S., Finkelstein, A. V., and Galzitskaya, O. V.: Trend of amino acid composition of proteins of different taxa, J. Bioinform. Comput. B., 04, 597–608, https://doi.org/10.1142/S0219720006002016, 2006.
Boyd, P. W., Claustre, H., Levy, M., Siegel, D. A., and Weber, T.: Multi-faceted particle pumps drive carbon sequestration in the ocean, Nature, https://doi.org/10.1038/s41586-019-1098-2, 2019.
Briggs, N. T., Slade, W. H., Boss, E., and Perry, M. J.: Method for estimating mean particle size from high-frequency fluctuations in beam attenuation or scattering measurements, Appl. Opt., 52, 6710–6725, https://doi.org/10.1364/AO.52.006710, 2013.
Burd, A. B., Hansell, D. A., Steinberg, D. K., Anderson, T. R., Arístegui, J., Baltar, F., Beaupré, S. R., Buesseler, K. O., DeHairs, F., Jackson, G. A., Kadko, D. C., Koppelmann, R., Lampitt, R. S., Nagata, T., Reinthaler, T., Robinson, C., Robison, B. H., Tamburini, C., and Tanaka, T.: Assessing the apparent imbalance between geochemical and biochemical indicators of meso- and bathypelagic biological activity: What the @$#! is wrong with present calculations of carbon budgets?, Deep-Sea Res. Pt. II, 1557–1571, https://doi.org/10.1016/j.dsr2.2010.02.022, 2010.
Carlson, C. A., Giovannoni, S. J., Hansell, D. A., Goldberg, S. J., Parsons, R., and Vergin, K.: Interactions among dissolved organic carbon, microbial processes, and community structure in the mesopelagic zone of the northwestern Sargasso Sea, Limnol. Oceanogr., 49, 1073–1083, https://doi.org/10.4319/lo.2004.49.4.1073, 2004.
Chang, K. J. L., Nichols, C. M., Blackburn, S. I., Dunstan, G. A., Koutoulis, A., and Nichols, P. D.: Comparison of Thraustochytrids Aurantiochytrium sp., Schizochytrium sp., Thraustochytrium sp., and Ulkenia sp. for Production of Biodiesel, Long-Chain Omega-3 Oils, and Exopolysaccharide, Mar. Biotechnol., 16, 396–411, https://doi.org/10.1007/s10126-014-9560-5, 2014.
Cho, B. C. and Azam, F.: Major role of bacteria in biogeochemical fluxes in the ocean's interior, Nature, 332, 441–443, https://doi.org/10.1038/332441a0, 1988.
Cole, H., Henson, S., Martin, A., and Yool, A.: Mind the gap: The impact of missing data on the calculation of phytoplankton phenology metrics, J. Geophys. Res.-Oceans, 117, C08030, https://doi.org/10.1029/2012JC008249, 2012.
Collins, J. R., Edwards, B. R., Thamatrakoln, K., Ossolinski, J. E., DiTullio, G. R., Bidle, K. D., Doney, S. C., and Van Mooy, B. A. S.: The multiple fates of sinking particles in the North Atlantic Ocean, Global Biogeochem. Cy., 29, 1471–1494, https://doi.org/10.1002/2014GB005037, 2015.
Cowen, J. P., Bertram, M. A., Wakeham, S. G., Thomson, R. E., William Lavelle, J., Baker, E. T., and Feely, R. A.: Ascending and descending particle flux from hydrothermal plumes at Endeavour Segment, Juan de Fuca Ridge, Deep-Sea Res. Pt. I, 48, 1093–1120, https://doi.org/10.1016/S0967-0637(00)00070-4, 2001.
Edgcomb, V. P., Taylor, C., Pachiadaki, M. G., Honjo, S., Engstrom, I., and Yakimov, M.: Comparison of Niskin vs. in situ approaches for analysis of gene expression in deep Mediterranean Sea water samples, Deep-Sea Res. Pt. II, 129, 213–222, https://doi.org/10.1016/j.dsr2.2014.10.020, 2016.
Eppley, R. W. and Peterson, B. J.: Particulate organic matter flux and planktonic new production in the deep ocean, Nature, 282, 677–680, https://doi.org/10.1038/282677a0, 1979.
Fennel, K., Mattern, J. P., Doney, S. C., Bopp, L., Moore, A. M., Wang, B., and Yu, L.: Ocean biogeochemical modelling, Nat. Rev. Methods Primers, 2, 1–21, https://doi.org/10.1038/s43586-022-00154-2, 2022.
Fuchs, R., Baumas, C. M. J., Garel, M., Nerini, D., Le Moigne, F. A. C., and Tamburini, C.: A RUpture-Based detection method for the Active mesopeLagIc Zone (RUBALIZ): A crucial step toward rigorous carbon budget assessments, Limnol. Oceanogr.-Meth., 21, 24–39, https://doi.org/10.1002/lom3.10520, 2022.
Fuhrman, J.: Impact of Viruses on Bacterial Processes, in: Microbial ecology of the oceans, Wiley, 327–350, 542 pp., ISBN 0-471-29992-8.X, 2000.
Garel, M., Bonin, P., Martini, S., Guasco, S., Roumagnac, M., Bhairy, N., Armougom, F., and Tamburini, C.: Pressure-Retaining Sampler and High-Pressure Systems to Study Deep-Sea Microbes Under In Situ Conditions, Front. Microbiol., 10, 453, https://doi.org/10.3389/FMICB.2019.00453, 2019.
Giering, S. L. C. and Evans, C.: Overestimation of prokaryotic production by leucine incorporation – and how to avoid it, Limnol. Oceanogr., 67, 726–738, https://doi.org/10.1002/lno.12032, 2022.
Giering, S. L. C., Sanders, R., Lampitt, R. S., Anderson, T. R., Tamburini, C., Boutrif, M., Zubkov, M. V., Marsay, C. M., Henson, S. A., Saw, K., Cook, K., and Mayor, D. J.: Reconciliation of the carbon budget in the ocean's twilight zone, Nature, 507, 480–483, https://doi.org/10.1038/nature13123, 2014.
Giering, S. L. C., Sanders, R., Martin, A. P., Henson, S. A., Riley, J. S., Marsay, C. M., and Johns, D. G.: Particle flux in the oceans: Challenging the steady state assumption, Global Biogeochem. Cy., 31, 159–171, https://doi.org/10.1002/2016GB005424, 2017.
Giering, S. L. C., Cavan, E. L., Basedow, S. L., Briggs, N., Burd, A. B., Darroch, L. J., Guidi, L., Irisson, J. O., Iversen, M. H., Kiko, R., Lindsay, D., Marcolin, C. R., McDonnell, A. M. P., Möller, K. O., Passow, U., Thomalla, S., Trull, T. W., and Waite, A. M.: Sinking Organic Particles in the Ocean–Flux Estimates From in situ Optical Devices, Front. Mar. Sci., 6, 834, https://doi.org/10.3389/fmars.2019.00834, 2020.
del Giorgio, P. A. and Cole, J. J.: BACTERIAL GROWTH EFFICIENCY IN NATURAL AQUATIC SYSTEMS, Annu. Rev. Ecol. Syst., 29, 503–541, https://doi.org/10.1146/annurev.ecolsys.29.1.503, 1998.
Grossart, H.-P. and Ploug, H.: Bacterial production and growth efficiencies: Direct measurements on riverine aggregates, Limnol. Oceanogr., 45, 436–445, https://doi.org/10.4319/lo.2000.45.2.0436, 2000.
Grossart, H.-P. and Ploug, H.: Microbial degradation of organic carbon and nitrogen on diatom aggregates, Limnol. Oceanogr., 46, 267–277, https://doi.org/10.4319/lo.2001.46.2.0267, 2001.
Guieu, C., Desboeufs, K., Albani, S., Alliouane, S., Aumont, O., Barbieux, M., Barrillon, S., Baudoux, A.-C., Berline, L., Bhairy, N., Bigeard, E., Bloss, M., Bressac, M., Brito, J., Carlotti, F., de, Liege, G., Dinasquet, J., Djaoudi, K., Doglioli, A., D'Ortenzio, F., Doussin, J.-F., Duforet, L., Dulac, F., Dutay, J.-C., Engel, A., Feliu-Brito, G., Ferre, H., Formenti, P., Fu, F., Garcia, D., Garel, M., Gazeau, F., Giorio, C., Gregori, G., Grisoni, J.-M., Guasco, S., Guittonneau, J., Haëntjens, N., Heimburger, L.-E., Helias, S., Jacquet, S., Laurent, B., Leblond, N., Lefevre, D., Mallet, M., Marañón, E., Nabat, P., Nicosia, A., Obernosterer, I., Perez, Lorenzo, M., Petrenko, A., Pulido-Villena, E., Raimbault, P., Ridame, C., Riffault, V., Rougier, G., Rousselet, L., Roy-Barman, M., Saiz-Lopez, A., Schmechtig, C., Sellegri, K., Siour, G., Taillandier, V., Tamburini, C., Thyssen, M., Tovar-Sanchez, A., Triquet, S., Uitz, J., Van, Wambeke, F., Wagener, T., and Zaencker, B.: BIOGEOCHEMICAL dataset collected during the PEACETIME cruise, SEANOE [data set], https://doi.org/10.17882/75747, 2020.
Hamamoto, Y. and Honda, D.: Nutritional intake of Aplanochytrium (Labyrinthulea, Stramenopiles) from living diatoms revealed by culture experiments suggesting the new prey–predator interactions in the grazing food web of the marine ecosystem, PLoS ONE, 14, e0208941, https://doi.org/10.1371/journal.pone.0208941, 2019.
Henson, S. A., Sanders, R., Madsen, E., Morris, P. J., Le Moigne, F., and Quartly, G. D.: A reduced estimate of the strength of the ocean's biological carbon pump, Geophys. Res. Lett., 38, L04606, https://doi.org/10.1029/2011GL046735, 2011.
Herndl, G. and Reinthaler, T.: Microbial control of the dark end of the biological pump, Nat. Geosci., 6, 718–724, https://doi.org/10.1038/ngeo1921, 2013.
Herndl, G. J., Reinthaler, T., Teira, E., van Aken, H., Veth, C., Pernthaler, A., and Pernthaler, J.: Contribution of Archaea to Total Prokaryotic Production in the Deep Atlantic Ocean, Appl. Environ. Microb., 71, 2303–2309, https://doi.org/10.1128/AEM.71.5.2303-2309.2005, 2005.
Homma, T. and Saltelli, A.: Importance measures in global sensitivity analysis of nonlinear models, Reliab. Eng. Syst. Safe., 52, 1–17, https://doi.org/10.1016/0951-8320(96)00002-6, 1996.
Hoppe, H. and Ullrich, S.: Profiles of ectoenzymes in the Indian Ocean: phenomena of phosphatase activity in the mesopelagic zone, Aquat. Microb. Ecol., 19, 139–148, https://doi.org/10.3354/ame019139, 1999.
Iversen, M. H., Nowald, N., Ploug, H., Jackson, G. A., and Fischer, G.: High resolution profiles of vertical particulate organic matter export off Cape Blanc, Mauritania: Degradation processes and ballasting effects, Deep-Sea Res. Pt. I, 57, 771–784, https://doi.org/10.1016/j.dsr.2010.03.007, 2010.
Jain, R., Raghukumar, S., Tharanathan, R., and Bhosle, N. B.: Extracellular Polysaccharide Production by Thraustochytrid Protists, Mar. Biotechnol., 7, 184–192, https://doi.org/10.1007/s10126-004-4025-x, 2005.
Kiørboe, T.: Marine snow microbial communities: scaling of abundances with aggregate size, Aquat. Microb. Ecol., 33, 67–75, https://doi.org/10.3354/ame033067, 2003.
Kiørboe, T., Grossart, H.-P., Ploug, H., and Tang, K.: Mechanisms and Rates of Bacterial Colonization of Sinking Aggregates, Appl. Environ. Microb., 68, 3996–4006, https://doi.org/10.1128/AEM.68.8.3996-4006.2002, 2002.
Kiørboe, T., Tang, K., Grossart, H.-P., and Ploug, H.: Dynamics of Microbial Communities on Marine Snow Aggregates: Colonization, Growth, Detachment, and Grazing Mortality of Attached Bacteria, Appl. Environ. Microb., 69, 3036–3047, https://doi.org/10.1128/AEM.69.6.3036-3047.2003, 2003.
Kirchman, D., K'nees, E., and Hodson, R.: Leucine incorporation and its potential as a measure of protein synthesis by bacteria in natural aquatic systems, Appl. Environ. Microb., 49, 599–607, https://doi.org/10.1128/aem.49.3.599-607.1985, 1985.
Kirchman, D. L. and Ducklow, H. W.: Estimating Conversion Factors for the Thymidine and Leucine Methods for Measuring Bacterial Production, in: Handbook of Methods in Aquatic Microbial Ecology, Lewis publishers, https://doi.org/10.1201/9780203752746, ISBN 9780203752746, 1993.
Koski, M., Valencia, B., Newstead, R., and Thiele, C.: The missing piece of the upper mesopelagic carbon budget? Biomass, vertical distribution and feeding of aggregate-associated copepods at the PAP site, Prog. Oceanogr., 181, 102243, https://doi.org/10.1016/j.pocean.2019.102243, 2020.
Kuhlisch, C., Schleyer, G., Shahaf, N., Vincent, F., Schatz, D., and Vardi, A.: Viral infection of algal blooms leaves a unique metabolic footprint on the dissolved organic matter in the ocean, Science Advances, 7, eabf4680, https://doi.org/10.1126/sciadv.abf4680, 2021.
Kwon, E. Y., Primeau, F., and Sarmiento, J. L.: The impact of remineralization depth on the air–sea carbon balance, Nat. Geosci., 2, 630–635, https://doi.org/10.1038/ngeo612, 2009.
Lagarias, J. C., Reeds, J. A., Wright, M. H., and Wright, P. E.: Convergence properties of the Nelder-Mead simplex method in low dimensions, SIAM J. Optimiz., 9, 112–147, https://doi.org/10.1137/S1052623496303470, 1998.
Lampitt, R. S., Boorman, B., Brown, L., Lucas, M., Salter, I., Sanders, R., Saw, K., Seeyave, S., Thomalla, S. J., and Turnewitsch, R.: Particle export from the euphotic zone: Estimates using a novel drifting sediment trap, 234Th and new production, Deep-Sea Res. Pt. I, 55, 1484–1502, https://doi.org/10.1016/j.dsr.2008.07.002, 2008.
Lara, E., Vaqué, D., Sà, E. L., Boras, J. A., Gomes, A., Borrull, E., Díez-Vives, C., Teira, E., Pernice, M. C., Garcia, F. C., Forn, I., Castillo, Y. M., Peiró, A., Salazar, G., Morán, X. A. G., Massana, R., Catalá, T. S., Luna, G. M., Agustí, S., Estrada, M., Gasol, J. M., and Duarte, C. M.: Unveiling the role and life strategies of viruses from the surface to the dark ocean, Sci. Adv., 3, e1602565, https://doi.org/10.1126/sciadv.1602565, 2017.
Le Moigne, F. A. C.: Pathways of organic carbon downward transport by the oceanic biological carbon pump, Front. Mar. Sci., 6, 1–8, https://doi.org/10.3389/fmars.2019.00634, 2019.
Le Moigne, F. A. C., Henson, S. A., Cavan, E., Georges, C., Pabortsava, K., Achterberg, E. P., Ceballos-Romero, E., Zubkov, M., and Sanders, R. J.: What causes the inverse relationship between primary production and export efficiency in the Southern Ocean?, Geophys. Res. Lett., 43, 4457–4466, https://doi.org/10.1002/2016GL068480, 2016.
Lemée, R., Rochelle-Newall, E., Van Wambeke, F., Pizay, M., Rinaldi, P., and Gattuso, J.: Seasonal variation of bacterial production, respiration and growth efficiency in the open NW Mediterranean Sea, Aquat. Microb. Ecol., 29, 227–237, https://doi.org/10.3354/ame029227, 2002.
Luo, E., Leu, A. O., Eppley, J. M., Karl, D. M., and DeLong, E. F.: Diversity and origins of bacterial and archaeal viruses on sinking particles reaching the abyssal ocean, ISME J., 16, 1627–1635, https://doi.org/10.1038/s41396-022-01202-1, 2022.
Marsay, C. M., Sanders, R. J., Henson, S. A., Pabortsava, K., Achterberg, E. P., and Lampitt, R. S.: Attenuation of sinking particulate organic carbon flux through the mesopelagic ocean, P. Natl. Acad. Sci. USA, 112, 1089–1094, https://doi.org/10.1073/pnas.1415311112, 2015.
Martin, J. H., Knauer, G. A., Karl, D. M., and Broenkow, W. W.: VERTEX: carbon cycling in the northeast Pacific, Deep-Sea Res. Pt. A, 34, 267–285, https://doi.org/10.1016/0198-0149(87)90086-0, 1987.
McDonnell, A. M. P., Lam, P. J., Lamborg, C. H., Buesseler, K. O., Sanders, R., Riley, J. S., Marsay, C., Smith, H. E. K., Sargent, E. C., Lampitt, R. S., and Bishop, J. K. B.: The oceanographic toolbox for the collection of sinking and suspended marine particles, Prog. Oceanogr., 133, 17–31, https://doi.org/10.1016/j.pocean.2015.01.007, 2015.
Nagata, T., Tamburini, C., Arístegui, J., Baltar, F., Bochdansky, A. B., Fonda-Umani, S., Fukuda, H., Gogou, A., Hansell, D. A., Hansman, R. L., Herndl, G. J., Panagiotopoulos, C., Reinthaler, T., Sohrin, R., Verdugo, P., Yamada, N., Yamashita, Y., Yokokawa, T., and Bartlett, D. H.: Emerging concepts on microbial processes in the bathypelagic ocean – ecology, biogeochemistry, and genomics, Deep-Sea Res. Pt II, 57, 1519–1536, https://doi.org/10.1016/j.dsr2.2010.02.019, 2010.
Nelder, J. A. and Mead, R.: A Simplex Method for Function Minimization, Comput. J., 7, 308–313, https://doi.org/10.1093/comjnl/7.4.308, 1965.
Pedrosa-Pàmies, R., Conte, M. H., Weber, J. C., and Johnson, R.: Hurricanes Enhance Labile Carbon Export to the Deep Ocean, Geophys. Res. Lett., 46, 10484–10494, https://doi.org/10.1029/2019GL083719, 2019.
Picheral, M., Catalano, C., Brousseau, D., Claustre, H., Coppola, L., Leymarie, E., Coindat, J., Dias, F., Fevre, S., Guidi, L., Irisson, J. O., Legendre, L., Lombard, F., Mortier, L., Penkerch, C., Rogge, A., Schmechtig, C., Thibault, S., Tixier, T., Waite, A., and Stemmann, L.: The Underwater Vision Profiler 6: an imaging sensor of particle size spectra and plankton, for autonomous and cabled platforms, Limnol. Oceanogr.-Meth., 20, 115–129, https://doi.org/10.1002/lom3.10475, 2022.
Planquette, H. and Sherrell, R. M.: Sampling for particulate trace element determination using water sampling bottles: methodology and comparison to in situ pumps, Limnol. Oceanogr.-Meth., 10, 367–388, https://doi.org/10.4319/lom.2012.10.367, 2012.
Reinthaler, T., van Aken, H., Veth, C., Arístegui, J., Robinson, C., Williams, P. J. L. B., Lebaron, P., and Herndl, G. J.: Prokaryotic respiration and production in the meso- and bathypelagic realm of the eastern and western North Atlantic basin, Limnol. Oceanogr., 51, 1262–1273, https://doi.org/10.4319/lo.2006.51.3.1262, 2006.
Reinthaler, T., van Aken, H. M., and Herndl, G. J.: Major contribution of autotrophy to microbial carbon cycling in the deep North Atlantic's interior, Deep-Sea Res. Pt. II, 57, 1572–1580, https://doi.org/10.1016/j.dsr2.2010.02.023, 2010.
Riley, J. S., Sanders, R., Marsay, C., Le Moigne, F. A. C., Achterberg, E. P., and Poulton, A. J.: The relative contribution of fast and slow sinking particles to ocean carbon export, Global Biogeochem. Cy., 26, 1–10, https://doi.org/10.1029/2011GB004085, 2012.
Riou, V., Para, J., Garel, M., Guigue, C., Al Ali, B., Santinelli, C., Lefèvre, D., Gattuso, J. P., Goutx, M., Jacquet, S., Le Moigne, F. A. C., Tachikawa, K., and Tamburini, C.: Biodegradation of Emiliania huxleyi aggregates by a natural Mediterranean prokaryotic community under increasing hydrostatic pressure, Prog. Oceanogr., 163, 271–281, https://doi.org/10.1016/j.pocean.2017.01.005, 2018.
Robert, A.: Minéralisation in situ de la matière organique le long de la colonne d'eau: application sur une station eulérienne, PhD, Aix-Marseille, https://www.theses.fr/2012AIXM4045 (last access: 15 August 2023), 2012.
RobF: RobeeF/InverseCarbonBudgetEstim: Paper publication (paper_release), Zenodo [data set], https://doi.org/10.5281/zenodo.8412947, 2023.
Russell, J. B. and Cook, G. M.: Energetics of Bacterial Growth: Balance of Anabolic and Catabolic Reactions, Microbiol. Rev., 59, 48–62, https://doi.org/10.1128/mr.59.1.48-62.1995, 1995.
Saint-Béat, B., Maps, F., and Babin, M.: Unraveling the intricate dynamics of planktonic Arctic marine food webs. A sensitivity analysis of a well-documented food web model, Prog. Oceanogr., 160, 167–185, https://doi.org/10.1016/j.pocean.2018.01.003, 2018.
Saint-Béat, B., Fath, B. D., Aubry, C., Colombet, J., Dinasquet, J., Fortier, L., Galindo, V., Grondin, P.-L., Joux, F., Lalande, C., Leblanc, M., Raimbault, P., Sime-Ngando, T., Tremblay, J.-E., Vaulot, D., Maps, F., and Babin, M.: Contrasting pelagic ecosystem functioning in eastern and western Baffin Bay revealed by trophic network modeling, Elementa: Science of the Anthropocene, 8, 1, https://doi.org/10.1525/elementa.397, 2020.
Sherry, N. D., Boyd, P. W., Sugimoto, K., and Harrison, P. J.: Seasonal and spatial patterns of heterotrophic bacterial production, respiration, and biomass in the subarctic NE Pacific, Deep-Sea Res. Pt. II, 46, 2557–2578, https://doi.org/10.1016/S0967-0645(99)00076-4, 1999.
Siegel, D. A., Buesseler, K. O., Behrenfeld, M. J., Benitez-Nelson, C. R., Boss, E., Brzezinski, M. A., Burd, A., Carlson, C. A., D'Asaro, E. A., Doney, S. C., Perry, M. J., Stanley, R. H. R., and Steinberg, D. K.: Prediction of the Export and Fate of Global Ocean Net Primary Production: The EXPORTS Science Plan, Front. Mar. Sci., 3, 22, https://doi.org/10.3389/fmars.2016.00022, 2016.
Simon, M. and Azam, F.: Protein content and protein synthesis rates of planktonic marine bacteria, Mar. Ecol.-Prog. Ser., 51, 201–213, https://doi.org/10.3354/meps051201, 1989.
Smith, D. C., Simon, M., Alldredge, A. L., and Azam, F.: Intense hydrolytic enzyme activity on marine aggregates and implications for rapid particle dissolution, Nature, 359, 139–142, https://doi.org/10.1038/359139a0, 1992.
Smith, K. L., Williams, P. M., and Druffel, E. R. M.: Upward fluxes of particulate organic matter in the deep North Pacific, Nature, 337, 724–726, https://doi.org/10.1038/337724a0, 1989.
Sobol, I. M.: Sensitivity Estimates for Nonlinear Mathematical Models, John Wiley and Sons, Inc., 1, 407–414, 1993.
Stange, P., Bach, L. T., Le Moigne, F. a. C., Taucher, J., Boxhammer, T., and Riebesell, U.: Quantifying the time lag between organic matter production and export in the surface ocean: Implications for estimates of export efficiency, Geophys. Res. Lett., 44, 268–276, https://doi.org/10.1002/2016GL070875, 2017.
Steinberg, D. K., Van Mooy, B. A. S., Buesseler, K. O., Boyd, P. W., Kobari, T., and Karl, D. M.: Bacterial vs. zooplankton control of sinking particle flux in the ocean's twilight zone, Limnol. Oceanogr., 53, 1327–1338, https://doi.org/10.4319/lo.2008.53.4.1327, 2008.
Stief, P., Elvert, M., and Glud, R. N.: Respiration by “marine snow” at high hydrostatic pressure: Insights from continuous oxygen measurements in a rotating pressure tank, Limnol. Oceanogr., 66, 2797–2809, https://doi.org/10.1002/lno.11791, 2021.
Tamburini, C., Garcin, J., Ragot, M., and Bianchi, A.: Biopolymer hydrolysis and bacterial production under ambient hydrostatic pressure through a 2000 m water column in the NW Mediterranean, Deep-Sea Res. Pt. II, 49, 2109–2123, https://doi.org/10.1016/S0967-0645(02)00030-9, 2002.
Tamburini, C., Garcin, J., and Bianchi, A.: Role of deep-sea bacteria in organic matter mineralization and adaptation to hydrostatic pressure conditions in the NW Mediterranean Sea, Aquat. Microb. Ecol., 32, 209–218, https://doi.org/10.3354/ame032209, 2003.
Tamburini, C., Garcin, J., Grégori, G., Leblanc, K., Rimmelin, P., and Kirchman, D. L.: Pressure effects on surface Mediterranean prokaryotes and biogenic silica dissolution during a diatom sinking experiment, Aquat. Microb. Ecol., 43, 267–276, https://doi.org/10.3354/ame043267, 2006.
Tamburini, C., Goutx, M., Guigue, C., Garel, M., Lefèvre, D., Charrière, B., Sempéré, R., Pepa, S., Peterson, M. L., Wakeham, S., and Lee, C.: Effects of hydrostatic pressure on microbial alteration of sinking fecal pellets, Deep-Sea Res. Pt. II, 56, 1533–1546, https://doi.org/10.1016/j.dsr2.2008.12.035, 2009.
Tamburini, C., Boutrif, M., Garel, M., Colwell, R. R., and Deming, J. D.: Prokaryotic responses to hydrostatic pressure in the ocean – a review, Env. Microbiol. Rep., 15, 1262–1274, 2013.
Tamburini, C., Garel, M., Barani, A., Boeuf, D., Bonin, P., Bhairy, N., Guasco, S., Jacquet, S., Le Moigne, F. A. C., Panagiotopoulos, C., Riou, V., Veloso, S., Santinelli, C., and Armougom, F.: Increasing Hydrostatic Pressure Impacts the Prokaryotic Diversity during Emiliania huxleyi Aggregates Degradation, Water, 13, 2616, https://doi.org/10.3390/w13192616, 2021.
Tarantola, A.: Inverse Problem Theory and Methods for Model Parameter Estimation, Society for Industrial and Applied Mathematics, 348 pp., https://doi.org/10.1137/1.9780898717921, 2005.
Tian, R. C., Ve, A. F., Klein, B., Packard, T., Roy, S., Savenko, C., and Silverberg, N.: E!ects of pelagic food-web interactions and nutrient remineralization on the biogeochemical cycling of carbon: a modeling approach, Journal Deep Sea Reasearch II, 47, 637–662, 2000.
Trull, T. W., Bray, S. G., Buesseler, K. O., Lamborg, C. H., Manganini, S., Moy, C., and Valdes, J.: In situ measurement of mesopelagic particle sinking rates and the control of carbon transfer to the ocean interior during the Vertical Flux in the Global Ocean (VERTIGO) voyages in the North Pacific, Deep-Sea Res. Pt. II, 55, 1684–1695, https://doi.org/10.1016/j.dsr2.2008.04.021, 2008.
Turley, C. and Mackie, P.: Biogeochemical significance of attached and free-living bacteria and the flux of particles in the NE Atlantic Ocean, Mar. Ecol.-Prog. Ser., 115, 191–203, https://doi.org/10.3354/meps115191, 1994.
Turner, J. T.: Zooplankton fecal pellets, marine snow, phytodetritus and the ocean's biological pump, Prog. Oceanogr., 130, 205–248, https://doi.org/10.1016/j.pocean.2014.08.005, 2015.
Vetter, Y. A., Deming, J. W., Jumars, P. A., and Krieger-Brockett, B. B.: A Predictive Model of Bacterial Foraging by Means of Freely Released Extracellular Enzymes, Microb. Ecol., 36, 75–92, https://doi.org/10.1007/s002489900095, 1998.
Wakeham, S. G., Lee, C., Hedges, J. I., Hernes, P. J., and Peterson, M. J.: Molecular indicators of diagenetic status in marine organic matter, Geochim. Cosmochim. Ac., 61, 5363–5369, https://doi.org/10.1016/S0016-7037(97)00312-8, 1997.
Weiss, M. S., Abele, U., Weckesser, J., Welte, W., Schiltz, E., and Schulz, G. E.: Molecular architecture and electrostatic properties of a bacterial porin, Science, 254, 1627–1630, https://doi.org/10.1126/science.1721242, 1991.
Welch, W. J., Gething, M. -j., Clarke, A. R., Viitanen, P., Lund, P., Haas, I. G., Georgopoulos, C., Ellis, R. J., Laskey, R. A., and Lorimer, G. H.: Heat shock proteins functioning as molecular chaperones: their roles in normal and stressed cells, Philos. T. R. Soc. B, 339, 327–333, https://doi.org/10.1098/rstb.1993.0031, 1993.
Westerhoff, H. V., Hellingwerf, K. J., and Van Dam, K.: Thermodynamic efficiency of microbial growth is low but optimal for maximal growth rate, P. Natl. Acad. Sci. USA, 80, 305–309, https://doi.org/10.1073/pnas.80.1.305, 1983.
Wilhem, W. and Suttle, C. A.: Viruses and Nutrient Cycles in the Sea, BioScience, 49, 781–788, https://doi.org/10.2307/1313569, 1999.
Xie, N., Bai, M., Liu, L., Li, J., He, Y., Collier, J. L., Hunt, D. E., Johnson, Z. I., Jiao, N., and Wang, G.: Patchy Blooms and Multifarious Ecotypes of Labyrinthulomycetes Protists and Their Implication in Vertical Carbon Export in the Pelagic Eastern Indian Ocean, Microbiol. Spectr., 10, e00144-22, https://doi.org/10.1128/spectrum.00144-22, 2022.
Young, H. L.: Uptake and Incorporation of Exogenous Leucine in Bacterial Cells under High Oxygen Tension, Nature, 219, 1068–1069, https://doi.org/10.1038/2191068a0, 1968.