the Creative Commons Attribution 4.0 License.
the Creative Commons Attribution 4.0 License.
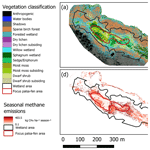
Optical and radar Earth observation data for upscaling methane emissions linked to permafrost degradation in sub-Arctic peatlands in northern Sweden
Sofie Sjögersten
Martha Ledger
Matthias Siewert
Betsabé de la Barreda-Bautista
Andrew Sowter
David Gee
Giles Foody
Doreen S. Boyd
Permafrost thaw in Arctic regions is increasing methane (CH4) emissions into the atmosphere, but quantification of such emissions is difficult given the large and remote areas impacted. Hence, Earth observation (EO) data are critical for assessing permafrost thaw, associated ecosystem change and increased CH4 emissions. Often extrapolation from field measurements using EO is the approach employed. However, there are key challenges to consider. Landscape CH4 emissions result from a complex local-scale mixture of micro-topographies and vegetation types that support widely differing CH4 emissions, and it is difficult to detect the initial stages of permafrost degradation before vegetation transitions have occurred. This study considers the use of a combination of ultra-high-resolution unoccupied aerial vehicle (UAV) data and Sentinel-1 and Sentinel-2 data to extrapolate field measurements of CH4 emissions from a set of vegetation types which capture the local variation in vegetation on degrading palsa wetlands. We show that the ultra-high-resolution UAV data can map spatial variation in vegetation relevant to variation in CH4 emissions and extrapolate these across the wider landscape. We further show how this can be integrated with Sentinel-1 and Sentinel-2 data. By way of a soft classification and simple correction of misclassification bias of a hard classification, the output vegetation mapping and subsequent extrapolation of CH4 emissions closely matched the results generated using the UAV data. Interferometric synthetic-aperture radar (InSAR) assessment of subsidence together with the vegetation classification suggested that high subsidence rates of palsa wetland can be used to quantify areas at risk of increased CH4 emissions. The transition of a 50 ha area currently experiencing subsidence to fen vegetation is estimated to increase emissions from 116 kg CH4 per season to emissions as high as 6500 to 13 000 kg CH4 per season. The key outcome from this study is that a combination of high- and low-resolution EO data of different types provides the ability to estimate CH4 emissions from large geographies covered by a fine mixture of vegetation types which are vulnerable to transitioning to CH4 emitters in the near future. This points to an opportunity to measure and monitor CH4 emissions from the Arctic over space and time with confidence.
Please read the corrigendum first before continuing.
-
Notice on corrigendum
The requested paper has a corresponding corrigendum published. Please read the corrigendum first before downloading the article.
-
Article
(3323 KB)
- Corrigendum
-
Supplement
(5270 KB)
-
The requested paper has a corresponding corrigendum published. Please read the corrigendum first before downloading the article.
- Article
(3323 KB) - Full-text XML
- Corrigendum
-
Supplement
(5270 KB) - BibTeX
- EndNote
The severe impact of climate heating in the Arctic has raised grave concerns regarding positive feedback from ecosystems that store substantial amounts of soil organic carbon in soils and peat deposits (Biskaborn et al., 2019; Chadburn et al., 2017; Grosse et al., 2016; Hugelius et al., 2020; Mishra et al., 2021; Schneider von Deimling et al., 2012; Sjöberg et al., 2020). Although the overall carbon balance in permafrost and non-permafrost peatlands is similar (Olefeldt et al., 2012), permafrost thaw increases methane (CH4) emissions across a range of Arctic landscapes (Olefeldt et al., 2013). Any increase in emissions of CH4 is of particular concern, as it is a powerful greenhouse gas with a global warming potential 28 times that of CO2 on a 100-year time frame, with Arctic ecosystems acting as strong emitters of CH4 (Euskirchen et al., 2014; Glagolev et al., 2011; IPCC, 2021; Maksyutov et al., 2010; McGuire et al., 2009; Turetsky et al., 2020).
Palsas are elevated peat mounds with a frozen core formed in peatlands. They are a common landform in the sporadic and discontinuous zone of the permafrost region, where palsa peatlands cover substantial areas of the total permafrost region (Ballantyne, 2018). From a climate feedback perspective, these are critical systems as they are carbon (C) rich, with an estimated storage of 100 Gt C in the Arctic region (based on a combination of field and modelled data in Hugelius et al., 2020; Schuur and Abbott, 2011; and Tarnocai et al., 2009). Palsa peatlands are dynamic systems that comprise of raised peatland plateaus, which can vary in size from tens of metres to kilometres in size; these plateaus are often surrounded by lower-lying waterlogged areas or have borders with thermokarst lakes (Ballantyne, 2018; Zuidhoff and Kolstrup, 2000). As a result of the raised nature of palsas their surface tends to be relatively dry. However, this changes as the permafrost in these peatlands degrade and the peat surface subsides which, over time, results in waterlogging (Olvmo et al., 2020; Sjöberg et al., 2015). Such degradation is already being observed in many areas of the Arctic in response to the greater-than-average warming occurring in this region (Åkerman and Johansson, 2008; Borge et al., 2017; de la Barreda-Bautista et al., 2022; Luoto and Seppälä, 2003; Olvmo et al., 2020; Sannel et al., 2016; Sannel and Kuhry, 2011). The loss of permafrost and switch to waterlogged conditions represents a profound change in how this ecosystem functions, driving increased CH4 emissions which have been observed across many Arctic landscapes (Glagolev et al., 2011; Miglovets et al., 2021; Varner et al., 2022; Walter Anthony et al., 2016; Walter et al., 2006). To understand fully the magnitude of this feedback and their impacts on the global climate, it is imperative to both quantify current emissions and predict new areas that may become CH4 emitters across Arctic landscapes undergoing permafrost degradation.
Given the size of the areas under threat from warming and their remoteness, remote sensing techniques are necessary to detect when and where permafrost degradation and subsidence are occurring. Coupled with measures of CH4 flux, this may offer an approach for understanding, estimating and predicting CH4 feedback at landscape scales across permafrost peatlands of the circum-Arctic region. Detecting year-to-year degradation of permafrost manifested as subsidence is possible using the Advanced Pixel System using the Intermittent Baseline Subset (ASPIS InSAR; interferometric synthetic-aperture radar) and other InSAR techniques (Alshammari et al., 2018; de la Barreda-Bautista et al., 2022; van Huissteden et al., 2021). Measures of surface subsidence of permafrost-affected areas and the rate of ground motion can give an indication of permafrost degradation extents and rates. Furthermore, the ASPIS InSAR data are unaffected by clouds, are based on publicly available Sentinel-1 data and can be used to analyse impact at a continental scale. This means that ASPIS InSAR can be used to identify areas that are at risk of increased CH4 emissions and help to assess the radiative forcing ecosystem feedback resulting from permafrost thaw.
Complementary vegetation maps derived from remote sensing can be used to extrapolate plot-based CH4 emission data to the wider landscape (Varner et al., 2022). However, the scale dependency of ecosystem properties that are non-linearly distributed in space (Siewert, 2018; Siewert and Olofsson, 2020) means that the spatial resolution of the remotely sensed data are important. Coarse-spatial-resolution remote sensing data, such as those from openly available and high-temporal-resolution optical-satellite-borne sensors, run the risk of missing small areas of those vegetation types with high CH4 emissions, which may lead to an underestimate of total emissions (Bernhardt et al., 2017). Further, the relatively slow rate of the response of vegetation to ground condition changes resulting from permafrost degradation means mapping vegetation may not fully explain patterns of CH4 emissions (de la Barreda-Bautista et al., 2022). After subsidence starts to occur, the original vegetation type can persist for many years (>10 years) until the site conditions have changed to a point that the original vegetation is outcompeted (Johansson et al., 2013). This makes it difficult to use vegetation change in isolation as a year-on-year proxy for permafrost degradation and linked potential regulation of CH4 emissions. Furthermore, permafrost degradation and linked increases in CH4 production are driven by several processes across scales. Variation in root inputs of labile carbon or oxygen in the peat profile occur over millimetres and can vary seasonally in response to water levels (Ström and Christensen, 2007; Ström et al., 2003). Lateral erosion and subsidence linked to permafrost degradation occur on metre scales when measured over several years, while changes in the vegetation community from raised-palsa vegetation to fen type vegetation occur over tens of metres at decadal timescales (de la Barreda-Bautista et al., 2022; Varner et al., 2022). Together the different processes associated with permafrost degradation result in a spatially varied landscape with a mixture of functionally distinct geomorphological units with regard to soil moisture content and vegetation productivity, creating a palimpsest of processes and properties determining the effective greenhouse gas feedback of these regions (Siewert et al., 2021). Therefore, remote sensing methodologies which capture fine-scale spatial variation in vegetation composition, in addition to permafrost degradation over seasonal to yearly timescales, are needed to provide appropriate observations of degrading peatlands in the Arctic in order to measure their CH4 emissions accurately.
With a view to estimating CH4 emissions resulting from permafrost degradation in peatlands, this paper explores two remote sensing technologies to provide data for extrapolating plot-based methane measurements over sub-Arctic peatlands of northern Sweden. Specific objectives were to (i) carry out detailed field surveys to capture small-sized or local-scale variation in CH4 emissions linked to vegetation type and permafrost subsidence; (ii) conduct vegetation mapping from Sentinel-2 satellite data and, in particular, demonstrate the value of rigorous map validation on the quantification of CH4 emissions; and (iii) quantify CH4 emissions from areas experiencing permafrost degradation and those at high risk of subsidence as determined from Sentinel-1 InSAR (processed using ASPIS) data.
2.1 Field site description
Three peatland locations affected by permafrost conditions – the Tourist Station, Storflaket, and Stordalen wetlands (Fig. 1) – situated to the south of Torneträsk Lake near Abisko, northern Sweden (68∘12′ N, 19∘03′ E; 351 m a.s.l.), were the focus of study. The study sites are in the sub-Arctic climatic zone; the mean annual temperature (MAT) was 0.3 ∘C, and the mean annual precipitation (MAP) was 347.2 mm between 1990–2020 (http://www.smhi.se, last access: 31 January 2023). The study sites comprise areas of raised palsas (i.e. relatively dry peat plateaus) and waterlogged areas with fen or birch forest vegetation. All three sites show signs of active permafrost thaw indicated by a lowering of the ground surface (i.e. ground subsidence), high soil moisture levels and areas of palsa collapse (i.e. complete permafrost degradation and transition from palsas to wetland) (Åkerman and Johansson, 2008). The raised-palsa parts of the sites tend to get snow free in April–May, but snow can remain in hollows into June.
Previous mapping of the vegetation at these sites using unoccupied aerial vehicles (UAVs) and subsidence estimated using ASPIS InSAR (de la Barreda-Bautista et al., 2022) show the vegetation on the palsas to be composed of bryophytes (e.g. Sphagnum fuscum), lichens and dwarf shrubs (Empetrum nigrum, Andromeda polifolia and Betula nana; (Sjögersten et al., 2016)). The most common herbaceous species was Rubus chamaemorus. Recently collapsed areas adjacent to and within the plateau areas tended to be vegetated by Sphagnum sp. and Eriophorum vaginatum and E. angustifolium. Extensively collapsed and subsequently flooded areas tended to be vegetated by graminoids, mainly Carex and Eriophorum species; herbaceous plants, mainly Menyanthes trifoliata and Comarum palustre; and deciduous shrubs, such as Salix laponica, Salix spp. and Betula nana. Forested-wetland areas tended to be located adjacent to streams and at the perimeters of the wetland areas. The mean peat depth was ca. 60, 94, 50 and 40 cm on palsas, Sphagnum sp., sedge and forested-wetland areas, respectively; the mean soil C storage in the top 100 cm was 52, 34, 33 and 40 kg SOC m−2 for palsas (soil organic carbon), Sphagnum sp., sedge and forested wetland, respectively (Siewert, 2018). The depth of the active layer (the seasonally thawed upper part of the soil) varies between drier areas, which have a shallower (ca. 30–60 cm) active layer, and wetter areas, where the active layer is deeper or where there is no permafrost at all in the upper 1.5 m. In the Torneträsk area, the active-layer depths have increased, between 0.2 and 2.0 cm yr−1 over 1978–2006, with a higher rate in more recent years (Åkerman and Johansson, 2008).
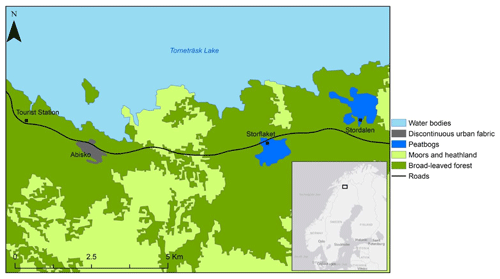
Figure 1Map showing the location of the three permafrost peatland study sites: Tourist Station, Storflaket and Stordalen. The inset is the location of Abisko in northern Sweden. The land cover classes were based on the CORINE (Coordination of information on the environment) land cover classification.
2.2 Experimental design
The fieldwork to measure CH4 emissions at the three study sites was carried out across the dates of 8–15 June, 18–29 July and 8–12 September 2021. At each site, we targeted six common vegetation types. The vegetation types dry lichen, dwarf shrub, moist moss, Sphagnum wetland, sedge wetland and willow wetland along with the dry lichen, dwarf shrub and moist moss occurred on the drier parts on the palsa. The vegetation types Sphagnum wetland, sedge wetland and willow wetland occurred in waterlogged fen or thermokarst areas. We also measured CH4 emissions from subsiding areas with the vegetation types dry lichen, dwarf shrub and moist moss subsiding. Areas were identified visually based on changes in topography, subsidence and waterlogging. Measurements in each vegetation type were carried out in blocks of ca. 10 m diameter with three to five sampling points in each block. Sampling blocks were distributed across the sites, and for the majority of vegetation type data from at least two spatially separate (i.e. >100 m apart) blocks were collected. As the vegetation type dry lichen subsiding was not common, this vegetation was sampled less than the other classes. To facilitate the collection of spatially distributed data from the common vegetation types, we selected sites with relatively easy access (e.g. sampling of waterlogged areas was completed in parts of the sites accessible by boardwalks). This approach allowed us to collect CH4 flux data from a large number of sampling points with the aim of generating scalable data from each vegetation type. A total of 502 measurements were collected across the three sites and the three dates, with all vegetation types measured in several locations at each site. Specifically, on the raised palsas and subsiding-palsa areas, the total number of measurements were 47 and 9 for dry lichen (DL) and dry lichen subsiding (DLS), respectively; 42 and 68 for dwarf shrub (DS) and dwarf shrub subsiding (DSS), respectively; and 50 and 56 for moist moss (MM) and moist moss subsiding (MMS), respectively. In the flooded fen vegetation areas, 102, 78 and 50 measurements were made in Sphagnum wetland (SW), sedge wetland (SE) and willow wetland (WW), respectively. A detailed description of the vegetation types are in de la Barreda-Bautista et al. (2022). Additional measurements of soil temperature and moisture using a Theta probe connected to a Theta-Meter (Delta-T Devices, Cambridge, UK) were carried out at each point where methane emissions were measured. The active-layer depths were determined using a graded metal pole in a subset of plots (ca. 10 of each vegetation type in each of the three mires in June, July and September 2021; de la Barreda-Bautista et al., 2022).
2.3 CH4 emission measurements
Methane flux measurements were conducted using a dynamic closed chamber connected to a Los Gatos ultraportable greenhouse gas (GHG) analyser (San Jose, California, USA). The Los Gatos analyser uses a cavity ring laser spectroscopy system for detecting CH4 and allows for the detection of CH4 in the field. It also has the advantage of being stable over time and not requiring regular calibration. Two types of chambers were used, one 15 and one 40 cm tall; the diameter was 40 cm. The chambers were equipped with two push-fit connectors that acted as an inlet and outlet allowing for the gas to flow from the chamber to the analyser and then to flow back into the chamber. After the chamber was placed on the measuring point, it was gently pushed on a 5 cm deep groove in the peat to create an airtight headspace between the ground surface and the chamber walls. To reduce problems with bubbles being released into the chamber, the chamber was first placed on the ground and then lifted and allowed to vent. The chambers were then placed back on the ground, and the sampling tubes were connected. The soil CH4 concentration (in ppm) increment inside the chamber was measured every 4 to 6 min, with data automatically recorded every 20 s. If the change in CH4 concentrations was not linear, the measurement was stopped, chambers were lifted from the soil and a new measurement was taken after CH4 readings had stabilized.
Trace gas concentrations, determined by the Los Gatos instrument, were converted to mass units using the ideal gas law (Eq. 1). Thereafter, using the slope of the linear change in gas concentration over time and Eq. (2), CH4 fluxes were calculated. Positive fluxes indicated GHG emissions from the soil into the atmosphere, and negative fluxes indicated uptake of atmospheric GHG by the soil.
where n is the number moles of trace gas (mol L−1), P is the atmospheric pressure (Pa), V is the volume of trace gas per litre of air (L L−1), R is the gas law constant (8314.46 L Pa K−1 mol−1) and T is the temperature (K). F is the calculated flux for either CH4 (mol m−2 h−1), slope is the slope of the linear regression (mol L−1 h−1), volumechamber is the chamber headspace volume (L) and areachamber is the area of the chamber (m2). Fluxes were converted to µg m−2 h−1 using the molecular weight of CH4 (i.e. 16.04 g mol−1, respectively). To avoid excluding very low CH4 fluxes from the seasonal calculations, fluxes with low r2 values were used in the calculation of cumulative emissions. By contrast, high CH4 fluxes with r2<0.7 were discarded as these fluxes were considered to be either affected by CH4 ebullition or gas leakage. Seasonal fluxes were calculated to the thaw period (i.e. spring–autumn) by scaling the CH4 flux measurements made in June, July and September to the length of the spring season, summer season and autumn season, respectively, and then summing these. The length of the three seasons follows a rapid transition due to the high latitude. A typical range was estimated using a combination of the seasonal NDVI (normalized difference vegetation index) and air temperature data. The NDVI data from the Torneträsk valley are available from a 6-year UAV time series (Siewert and Olofsson, 2020; Matthias Siewert, unpublished data), indicating the typical trajectory of rising and falling values during spring and autumn of the snow-free season and an NDVI peak plateau (summer) (see Siewert and Olofsson, 2020). Long-term air temperature data from Abisko are monitored by the Swedish meteorological and hydrological service (http://www.smhi.se, last access: 31 January 2023). We defined the spring period as starting from when the ground became snow free, air temperature rose above 5 ∘C and soil temperatures at 5 cm depth rose to above zero while the NDVI remained low (<0.25); this coincided with the month of June. We defined the summer growing season as the periods from the start of July when the NDVI increased from the NDVI of the non-growing season of <0.25 to >0.5, soil temperatures were consistently over 5 ∘C and daily mean air temperatures were mostly 10 ∘C or above. The NDVI peaked in mid-August with values around 0.7. The summer period included July and August. We identified the onset of autumn by a drop in the NDVI at the end of August that occurred in parallel with a drop in the daily mean air temperature which fell below 10 ∘C at this time. By the end of September, the vegetation had senesced and the mean daily air temperatures started reaching freezing conditions.
2.4 UAV-captured data for digital elevation models (DEMs) and vegetation mapping
Multispectral and true-colour RGB ultra-high-resolution (UHR) data were captured across Storflaket and Stordalen in 2020 and from the Tourist Station site in 2021 from ca. 106 and 100 m height using a fixed-wing UAV – Sensefly eBee fitted with a Parrot Sequoia multispectral sensor. Imagery was collected during the peak vegetation season in the last week of July and first week of August approximately at noon. The Parrot Sequoia obtained spectral measurements across four bands: green (550 nm ± 40 nm), red (660 nm ± 40 nm), red edge (735 nm ± 10 nm) and near infrared (790 nm ± 40 nm). The images, ranging between ∼ 1300–2000 per flight, were processed using Pix4D version 4.6.4 (Berlin, Germany) to produce an orthomosaic for each spectral band. Radiometric calibration was performed using spectral reflectance targets (MosaicMill). This resulted in a ground resolution of ∼ 11–13 cm for the multispectral and 2–3 cm for the RGB data. To ensure the orthomosaic and DEM generated from the UAV data were accurately geolocated, we collected ca. 50 ground control points using a differential GPS (dGPS; Trimble R8s) across all three sites. Further details on the UAV data collection are in de la Barreda-Bautista et al. (2022).
The multispectral UAV data were resampled to 0.5 m spatial resolution and then classified to produce a vegetation type map for the three study sites using the same nine classes (i.e. the dominant vegetation types found on raised-palsa plateaus, dry lichen, dwarf shrub and moist moss, and vegetation and areas covered by those vegetation types impacted by subsidence plus three vegetation types which dominate the flooded areas, Sphagnum moss, sedge and willow wetland) as those for which in situ CH4 flux data were measured. A support vector machine classification approach (Foody and Mathur, 2004) was used in ArcMap 10.4 (Esri) using the Train Support Vector Machine Classifier in the Spatial Analyst package, with simple random sampling of ground data in June 2021 for the Storflaket (n=258) and Stordalen (n=395) sites and August 2021 for the Tourist Station (n=657) site. The ground points from each site were randomly split into training and validation datasets of 70 % and 30 % proportions. A cutoff of 70 % was used to determine a sufficient level of accuracy for the vegetation mapping. We decided on this threshold because it is high enough to ensure good overall accuracy, whilst it is not so high as to account for the difficulty of mapping classes with very similar spectral signatures, e.g. non-subsiding vegetation classes and their subsiding counterparts. Furthermore, it is also important to note that ground data are never perfect. Indeed, the level of error can be quite large even when arising from authoritative sources. For example, trained aerial-photograph interpreters are known to differ in their labelling of forest classes by up to 30 % in some studies (Powell et al., 2004).
2.5 Sentinel-2 remotely sensed data for vegetation mapping
We used a neural network classification approach (Xie et al., 2008) within R using the “neuralnet” package (https://github.com/bips-hb/neuralnet, last access: 15 May 2023) to predict vegetation cover over the three sites of interest using Sentinel-2 data (wavebands B2, B3, B4, B5, B6, B7, B8a, B11 and B12) and elevation, slope and roughness data derived from the ArcticDEM, an optical stereo imagery model at 2 m spatial resolution (Morin et al., 2016). Cloud-free Sentinel-2 reflectance data from 27 July 2019 were used to produce vegetation maps for the sites of interest. Reflectance data were atmospherically corrected using Sen2Cor in the ESA Sentinel Application Platform (SNAP). Only one image was required to cover the wider study domain. To match the 20 m × 20 m resolution of the Sentinel-2 pixels, average elevation was computed for each pixel. Sentinel-2 wavebands 2, 3 and 4 were resampled from 10 to 20 m resolution using nearest-neighbour interpolation to match the resolution of the other wavebands (Ledger et al., 2023). A Sentinel-2 waveband specification is presented in Table S1 in Supplement S1. The UAV-derived vegetation maps were used to train the Sentinel-2 scenes covering the three study sites Tourist Station, Storflaket and Stordalen. For this the UAV data were upscaled to 20 m × 20 m using majority classification; this yielded a dataset of 2376 pixels. Training and test datasets were created from this. The testing set used was generated as a random stratified sample comprising 50 pixels per vegetation class, unless there was less than that (e.g. the willow wetland class had only 20 test pixels; Ledger et al., 2023).
The neural network approach was used to classify the Sentinel-2 data into vegetation classes from which the total areal extents of each vegetation class across the three sites were calculated. We chose a neural network approach for this purpose because of its ability to build both a hard and soft classification and it is easily implemented in R with rapid processing. Specifically, a multi-layer perceptron network with backpropagation and weight backtracking was used; therefore the “learning rate” and “momentum” parameters were not required (Xie et al., 2008). Two neural networks were generated: one that produced a standard hard classification (i.e. a single class label is produced for each pixel) and one that produced a soft classification (i.e. grades of class membership are produced for each pixel). The approaches of optimal model structure for the majority and the soft classification consisted of 12 principal components, normalized between 0–1; 1 hidden layer with 3 neurons (hard classification) or 10 neurons (soft classification); and an output layer of 15 units corresponding to the land cover types identified within the three study sites. Both models were developed through trial and error and multiple iterations to arrive at the maximum overall accuracy. The soft classification was undertaken as it enables the estimation of the proportion of different vegetation types within each pixel, offering greater understanding of the area covered by each class, in particular to capture and map those smaller patches of vegetation that have a larger contribution to CH4 flux.
Misclassification errors in the maps may substantially bias estimates of class areal extent generated from the maps. An adjustment for misclassification bias in a map can be made if its accuracy has been rigorously evaluated. Here, good-practice methods for accuracy and area estimation (Olofsson et al., 2014, 2013) were followed to produce misclassification bias-corrected estimates of class area; a bias correction for the soft classification would ideally require more detailed ground reference data than were available. Thus in total, three vegetation type outputs (maps and class areal extents) from the Sentinel-2 data were produced (soft classification, hard classification and misclassification bias-corrected hard classification). The same 70 % accuracy cutoff as described under the UAV mapping section was applied also to the Sentinel-2 datasets. The areal estimates of the vegetation classes were subsequently multiplied by the mean CH4 fluxes measured for each vegetation class to produce CH4 budgets for the entire mapped area.
2.6 Surface motion from Sentinel-1 InSAR
We used C-band SAR data from the Sentinel-1 satellites (European Union's Copernicus programme) with a wavelength and frequency of 5.55 cm and 5.41 GHz, respectively, to map multi-annual average velocity, subsequently denoted as the surface motion of the study areas. Details of this approach are in de la Barreda-Bautista et al. (2022). Briefly we gathered images from 2017 to 2020, focusing on the thaw season between April and October for 3 separate years. A total of 125 images were acquired from the descending mode, and surface motion was obtained using the APSIS method (formerly known as the Intermittent Small Baseline Subset, ISBAS), which relaxes the requirement for consistent phase stability for all observations using a coherence threshold of 0.45. Following InSAR processing the ground resolution was 20 m × 20 m. Using a stable reference point located in the centre of the village of Abisko, first the multi-annual average velocity (or average surface displacement) between 2017 and 2020 (with negative values representing subsidence) was calculated and second, for each season, a time series of surface motion was produced, showing the change in relative height of the surface. Surface motion ranges for the 2017 and 2020 thaw periods were calculated by subtracting the minimum surface motion value from the maximum surface motion value within respective time series between June and October. The InSAR data were thereafter compared to the CH4 flux data as described in the section on statistical analysis below.
2.7 Data analysis
We used residual maximum likelihood (REML) mixed models to test for significant differences in CH4 fluxes among sites and vegetation and their interactions. For this analysis we used the vegetation type as the fixed effect and sampling location (i.e. block), with the month and site as random effects. The residuals were inspected to ensure the normality assumption of the analysis was met. The standard error in the difference was used to evaluate which vegetation types differed from each other. We tested for the relationships between the UAV-predicted CH4 budgets per vegetation type and the three different Sentinel-2-based classifications using linear regression analysis. Relationships between ASPIS InSAR linear motion between the 2017 and 2020 thaw periods and seasonal CH4 fluxes were explored using REML, in which the ground motion categorized into 2.5 mm ranges was used as the fixed effect and the site was used as the random effect. For this analysis comparison, the CH4 fluxes extrapolated for each target vegetation type to the three sites using the UHR UAV data, which are able to capture the local-scale variation in the vegetation types, were aggregated to the 20 m × 20 m pixel size of the InSAR data.
We also calculated the net emissions of CH4 across the subsiding parts of the three wetlands (as detected by InSAR). This was done by summing the CH4 emissions attributed to each subsiding InSAR pixel for all three sites based on the dominant vegetation type (using the UAV vegetation classification) in that pixel. To explore how CH4 emissions may change as subsidence progresses and results in palsa collapse, waterlogging and vegetation change, we multiplied the currently subsiding wetland areas by the seasonal CH4 emissions that were measured in the lower- and higher-emitting fen vegetation types, respectively, to generate two exemplar future emissions scenarios. This CH4 emission scenario “after permafrost loss and palsa collapse” used the assumption that all of the subsiding area will be covered with a particular fen vegetation type. We want to highlight that this is not a realistic scenario as the fen vegetation that will replace the current vegetation on the raised palsas will be a mixture of vegetation communities suited to permanently waterlogged conditions. Hence the calculations of future CH4 emissions should be viewed as illustrations of the ranges of emissions that could occur from the sites following a full transition to a fen vegetation type based on their current-day emissions.
3.1 Variation in CH4 fluxes linked to vegetation type and permafrost degradation
The intact vegetation types of raised palsas (DL, DS and MM) generally had low CH4 fluxes; the vegetation type dry lichen even acted as a weak CH4 sink in spring (Fig. 2a). The areas identified as showing signs of permafrost degradation/subsidence had higher soil moisture content (, p<0.001; standard error of the difference of SED = 3.6 %; Fig. 3a) and deeper active-layer depths (, p<0.001 and , p<0.001, for July and September, respectively; Fig. 4) than areas that were less affected by degradation. Degrading areas had elevated CH4 fluxes compared to the corresponding vegetation types in areas that did not display visual signs of degradation (, p<0.001; SED = 2.85 mg CH4 m−2 h−1), with the largest increase in emissions being for subsiding areas of dwarf shrub (Fig. 2). The emissions from the subsiding-palsa areas were highest in summer, corresponding to the period of the highest air and soil temperatures (Figs. 2b, 3). The highest emissions were from the vegetation types of flooded fen, where emissions peaked in autumn (Fig. 2c). Soil temperature peaked in July at the non-flooded sites, while at flooded sites temperatures remained relatively constant after the initial thaw period. The coldest soil temperatures were measured in DS and DSS areas in September (, p<0.001; SED = 0.7 ∘C).
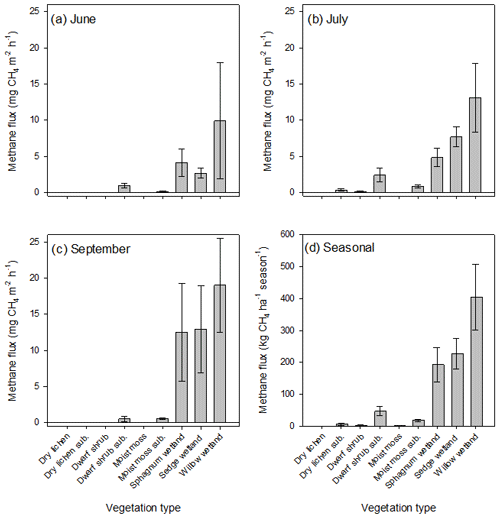
Figure 2CH4 fluxes measured in different vegetation types in (a) June, (b) July and (c) September and (d) cumulative seasonal CH4 emissions; sub. denotes measurements made in subsiding areas of vegetation types found on the raised-palsa plateaus. For September, no data are available from the class of dry lichen sub. Means and SE values are shown.
Integrated CH4 fluxes per vegetation type showed the highest seasonal fluxes of ca. 400 kg CH4 ha−1 per season from willow wetland areas with emissions from Sphagnum and sedge wetland vegetation types being ca. half those of the willow areas at ca. 200 kg CH4 ha−1 per season. The seasonal emissions from the areas of dwarf shrub subsiding, moist moss and dry lichen were 50 and 25 kg CH4 ha−1 per season, respectively, which were higher than in un-degraded areas where emissions from vegetation types of raised palsas were low at less than and/or equal to 2 kg CH4 ha−1 per season.
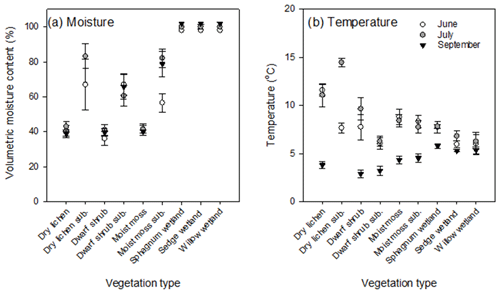
Figure 3Variation in (a) moisture and (b) temperature among vegetation types measured in June, July and September; sub. denotes measurements made in subsiding areas of vegetation types found on the raised-palsa plateaus. For September no data are available from the class of dry lichen sub. Means and SE values are shown.
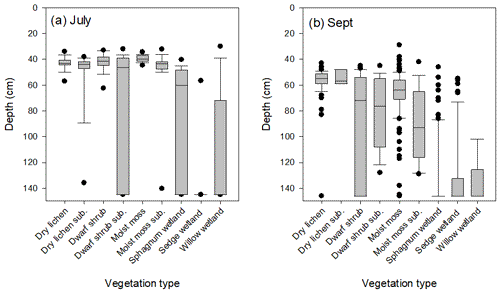
Figure 4Variation in active-layer depth among vegetation types in (a) July and (b) September; sub. denotes measurements made in subsiding areas of vegetation types found on the raised-palsa plateaus. Medians and 25th and 75th percentiles are shown. The whiskers show minimum and maximum values, excluding outliers; the black dots represent outliers.
3.2 Scaling CH4 fluxes via vegetation mapping and calculation of class areal extent
The mapping of the nine vegetation classes found on degrading palsa mires using the UHR UAV data gave an overall classification accuracy of 71 % (confusion matrices were generated in base R; Supplement S2). The area covered by the target wetland vegetation classes (i.e. excluding rock outcrops, birch forest areas, water bodies and anthropogenic surfaces) across the three sites was ca. 50 ha, while the total surveyed area was 83.7 ha (Table 1). Subsequently percentage estimates of areas are made relative to the target vegetation area. Areas where the visual inspection of the surface topography in the field clearly indicated advanced subsidence equated to 10.0 % of the area covered by the vegetation types. The three subsiding vegetation classes covered 1.5, 2.4 and 1.1 ha – or 3.0 %, 4.8 % and 2.2 % of the target vegetation type area – for dry lichen subsiding, dwarf shrub subsiding and moist moss subsiding, respectively (Table 1). The key here was that the classification was of a high enough accuracy for this output to be used as the “ground” data for the Sentinel-2 classifications. We considered 70 % overall accuracy as the threshold for suitable classification accuracy for vegetation classifications. This threshold was decided upon because it is high enough to ensure good overall accuracy, whilst it is not so high as to account for the difficulty of mapping classes with very similar spectral signatures, for example, vegetation classes compared with their subsiding counterparts.
Table 1Area of different wetland vegetation types at each of the three study sites and their proportional (Prop) contribution to the area covered by the target vegetation types. (Please note that areas of anthropogenic surfaces, exposed rock, sparse birch forest on mineral soil, forested wetland and water, as well as pixels impacted by shadows, are not in the CH4 flux study and hence are not shown in the table.)
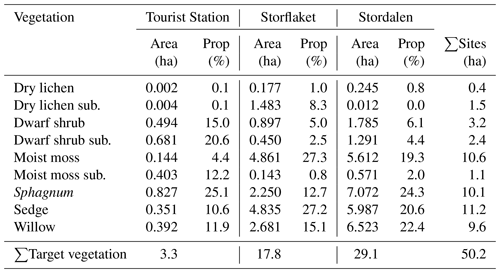
The resultant classification vegetation maps from the Sentinel-2 hard and soft classifications had overall accuracies of 50.2 % and 83.2 %, respectively. The class areal extents from these classification outputs, along with class areal extents obtained when output of the hard classification was corrected for misclassification bias, are presented in Table 2 (which depicts those classes shown in Table 1). The hard classification of Sentinel-2 data did not capture the fine-scale vegetation mosaic on the raised part of the palsa mire (i.e. dry lichen and dwarf shrub prior to subsidence); instead it mapped the vegetation types in those areas as vegetation classes for either moist moss or dwarf shrub subsiding, leading to a lower overall classification accuracy than that obtained using the approach of soft classification. Once corrected for misclassification bias, the areal extents of the hard classification for each vegetation class improved considerably in that the estimates are comparable to that mapped using the UAV data and the soft classification of the Sentinel-2 data. The soft classification of the Sentinel-2 data was able to map all nine target vegetation types and followed the same distribution of more or less abundant vegetation types, predicting total class areal extents similar to that of the UAV-derived maps. In both cases (bias-corrected hard classification and soft classification), those vegetation types on the raised-palsa area missed by the hard classification have a calculated areal extent.
The soft classification of the Sentinel-2 data produced a higher estimate of the areal extent of the fen vegetation types at 35.4 ha than the UAV classification but succeeded in mapping all the vegetation classes present across the study site. The mapping from the hard classification of Sentinel-2 underestimated the vegetation types of fen slightly at 28.64 ha and missed some of the other vegetation classes (i.e. dry lichen, dry lichen subsiding and dwarf shrub). After correcting for classification bias, the areal extents of vegetation classes from the hard classification closely matched those of the UAV-mapped areas, with 32.21 ha of the vegetation types of fen estimated to occupy the study site.
Based on the UAV classification vegetation mapping (class areal extents), 30.9 ha (or 61 %) of the area covered by the target vegetation types was comprised of the three vegetation classes (Sphagnum, sedge and willow wetland – known as vegetation types of fen) associated with the highest CH4 emissions. Using the mapping of areal extents from the UAV data to model CH4 fluxes shows marked differences in CH4 fluxes between raised palsas and the wetland types surrounding these (Fig. 5; Table 3). Areas of the raised palsas, which do not show visual signs of subsidence, generally have very low emissions, while areas with degrading permafrost and subsidence, as well as those areas with no permafrost, emit CH4. Among the vegetation types that are associated with high CH4 emissions, the maps highlight the importance of the vegetation type willow wetland as a source of methane from these wetlands. This is because it was both high emissions per area and substantial covered areas in particular at the Stordalen mire site. Although the highest CH4 emissions are from the areas which have lost their permafrost that are surrounding the remaining palsas, there are indications of increased emissions also within the palsas.
Table 2Comparing class areal estimates for the target vegetation types using Sentinel-2 (S-2) hard and soft classification and bias-corrected hard classification. For comparison the estimates are provided for the UAV-derived classification which served as the ground data.
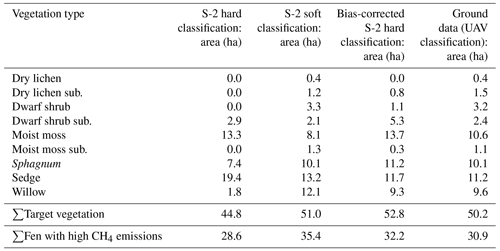
Methane emissions from within the palsa areas are commonly associated with vegetation of moist moss subsiding and dwarf shrub subsiding, as well as development of Sphagnum wetland vegetation in subsiding areas that have become waterlogged either at the edges of the palsas or in their interior following degradation. The vegetation of dwarf shrub subsiding is mainly found along the edges of the palsas that are experiencing lateral erosion, while the vegetation type moist moss subsiding occurs in the interior parts of the palsas in areas with deeper active layers and the development of a hummocky surface.
When CH4 emissions are integrated across the three wetland areas using the UAV-derived vegetation mapping, net emissions are 8519 kg CH4 per season (Table 3). The total emissions are composed of 16.6, 142.6 and 8359.5 kg CH4 per season from the vegetation types of raised palsas (DL, DS, MM), subsiding palsa (DLS, DSS, MMS) and waterlogged (SW, SE, WW), respectively. This shows that currently emissions from intact areas in the early stages of degradation are low compared to the already degraded areas. However, as the areas of raised-palsa vegetation and subsiding-palsa vegetation are large (ca. 20 ha – or 40 % of the area covered by target vegetation types – when combined), there are substantial risks of higher CH4 emissions from these sites associated with continued degradation of the currently subsiding areas and onset of degradation of the remaining palsa areas.
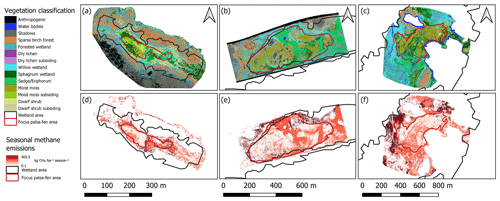
Figure 5(a–c) Detailed vegetation maps separating out wetland vegetation types including vegetation types associated with raised- and subsiding-palsa areas at the Tourist Station, Storflaket and Stordalen mires. The vegetation maps were generated from UAV-captured data. (d–f) Maps of seasonal CH4 emissions based on the seasonal CH4 emissions measured in the nine target vegetation types across the three mire sites. The palsa–fen wetland area outlined in purple is the area where ground data for CH4 fluxes as well as for the vegetation mapping were collected. The full wetland area at each site is indicated by the black boundary line.
Table 3Seasonal methane emissions for 2021 for the total area of each target vegetation class within the area covered by the UAV flights. Methane emissions are estimated for three different models using Sentinel-2 data. The CH4 flux calculated from the UAV data is derived from the area of each vegetation type multiplied by the seasonal CH4 flux for each vegetation type. Means and SE values are shown.
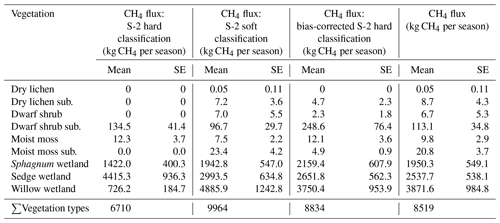
Taking the Sentinel-2 sets of estimates of areal extent of vegetation types to estimate the CH4 emissions of the vegetation types showed both over- and underestimation of emissions (compared to the UAV-derived CH4 estimates) – the hard classification underestimated CH4 emissions across the site by 21 %, while the soft classification overestimated emissions by 17 % (Table 3). The mismatch between the Sentinel-2 hard classification and the UAV estimates was linked to a misclassification of willow wetland as sedge wetland (Table 2, Supplement S2). Correcting the output of the hard classification for misclassification bias improved the match of CH4 estimates to that derived from the UAV data, and the key was that target vegetation classes were included in the mapping following this post-classification process. For the soft classification, it was the misclassification of sedge and willow wetland area. Correlation analysis between the UAV- and Sentinel-2 classification outputs showed that the bias-corrected hard classification was more closely correlated to the UAV predictions, followed closely by the soft classification with R2=0.99 and R2=0.99 (p<0.001 and p<0.001), respectively. The hard classification was not significantly related to the UAV-predicted CH4 fluxes (p>0.05; Fig. 6).
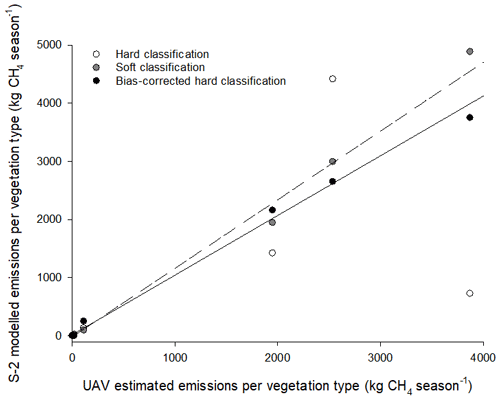
Figure 6Relationship between UAV-estimated methane emissions per vegetation types and the three Sentinel-2 (S-2) models. The dashed and solid lines show the relationships between the soft and bias-corrected hard classification and the UAV-estimated CH4 emissions, respectively. Note that no regression line was plotted for the hard classification as there was no significant relationship.
3.3 Linking InSAR-determined ground motion to CH4 emissions
InSAR detected subsidence across 90 % of the study area, indicating that the majority of the surface of these sites was impacted by millimetre level rates of subsidence. However, rates of ground motion varied considerably across the study area and among vegetation types. Importantly, across the three sites, areas with relatively low levels of ground motion were associated with the highest mean CH4 emissions (, p<0.001, SED = 961.5; Fig. 7), while both uplifting (5 to 7.5 mm) and the most subsiding (−10 to −12.5 mm) areas tended to have lower fluxes. High cumulative CH4 emissions (summed over all pixels for a given range of ground motions) were found for areas that were either stable or subsiding at an intermediate rate (Fig. 8), while the most subsiding areas had low cumulative CH4 emissions.
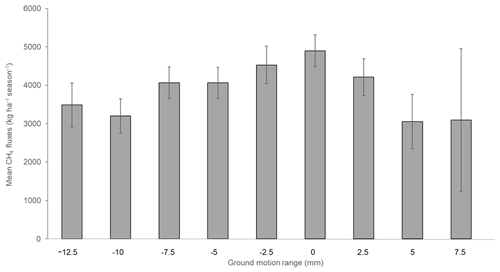
Figure 7Average seasonal CH4 emissions for different ground motion ranges (categories in 2.5 mm ranges) across the three study sites. Negative values indicate subsidence, and positive values indicate uplift. Means and SE values are shown.
This pattern of areas of high subsidence being linked to areas of low CH4 emissions was also apparent in the subsidence and CH4 emission maps (Fig. 9). For example, at the Tourist Station site mire areas of high subsidence (comprising mainly of vegetation of moist moss subsiding; Fig. 5a) corresponded to areas with relatively low CH4 emissions, while areas that have already transitioned to Sphagnum or willow wetland are associated with bands of high CH4 emissions along the degrading edge of the palsa plateaus (Fig. 5a and d). Similar patterns were evident at the Storflaket mire, where degrading areas within the palsa plateaus did not display high CH4 emissions, while areas that have transitioned to Sphagnum or sedge wetland have high CH4 emissions (Fig. 9b and e). The Stordalen site showed the largest areas of high subsidence that traversed the boundary between the palsa plateau and the fen areas (Fig. 9c and f). In this part of Stordalen the vegetation type Sphagnum wetland was present both in the hollows in the degrading interior of the palsa plateaus and in adjacent areas where the palsas had already collapsed, explaining the high CH4 emissions (together with the presence of the vegetation type willow wetland) from this area.
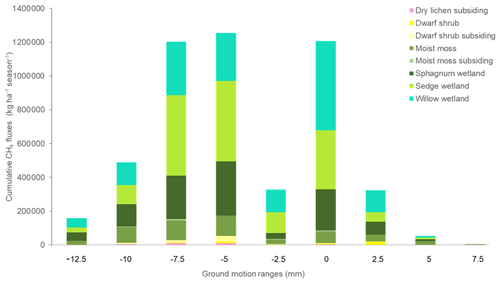
Figure 8Cumulative CH4 emissions (± SE) for different ground motions across the thaw seasons of 2017 and 2020 for pixels (i.e. a sum of the CH4 emissions from all pixels of a given ground motion; categories in 2.5 mm ranges) of the target vegetation classes. The vegetation types of dry lichen did not cover large enough areas to be evaluated on the 20 m × 20 m pixel scale of the ground motion data and are therefore not included in this comparison. Negative values indicate subsidence, and positive values indicate uplift.
The net emissions of CH4 across subsiding parts of the three wetlands (as detected by InSAR) were modest at 116 kg CH4 per season. However, when these areas have degraded to such a point that they are fully waterlogged and have transitioned to either Sphagnum or sedge vegetation, we estimate emissions from these, currently low-emitting, areas to be around 6480 kg per season or, if we use the highest-emitting vegetation type as a predictor of future emissions, 12 960 kg per season.
Methane emissions varied strongly across the palsa peatlands with consistent differences among vegetation types over space and time. The low emissions from the intact dry palsa areas and trends of increased emissions following degradation and flooding were comparable with those reported from other parts of Scandinavia and Russia, e.g. −1.7 to 1.2 kg CH4 ha−1 per season from dry lichen and raised palsas, respectively (Glagolev et al., 2011; Liebner et al., 2015; Miglovets et al., 2021; Nykänen et al., 2003; Olefeldt et al., 2013; Varner et al., 2022). However, there was strong variation in the magnitude of that response depending on the degradation stage, level of flooding and vegetation community present. For example, as long as sites were mainly impacted by increased waterlogging but not vegetation change (i.e. the raised-palsa vegetation persisted despite experiencing subsidence), CH4 emissions only increased modestly (Fig. 2). This suggests that waterlogging per se was not sufficient to drive high emissions as these were only recorded following flooding and a transition of the vegetation community to Sphagnum mosses, sedges and other wetland-adapted species.
An important finding in our study is the high CH4 emissions reported from fen and in particular the vegetation type willow. These emissions were consistent across the three study sites and were at the upper range of emissions reported for permafrost fens in the literature, e.g. maximum fluxes of ca. 130 to 360 kg CH4 ha−1 per season (Miglovets et al., 2021; Olefeldt et al., 2013), and in the range of emissions from ponds and thermokarst lakes in degrading permafrost in the literature (Dzyuban, 2022; Glagolev et al., 2011; Walter et al., 2006). Possible explanations for this variation among vegetation types and very high CH4 emissions are the impact of the vegetation on CH4 emissions both via plant-mediated transport, root exudate quality and quantity, and the level of rhizosphere oxidation. For example, both M. trifoliate and in particular C. rupestris, which occur in the fen vegetation communities, are known to transport CH4 through their vascular tissues, while other species common in our system, e.g. S. laponica, do not share this trait (Ge et al., 2022). An additional way in which plants may contribute to high CH4 production and emissions is by releasing labile carbon in the form of root exudates into the rhizosphere. This carbon flow can represent a substantial part of net primary production (NPP) and can have a large impact on CH4 emissions (Girkin, 2018; Ström et al., 2003). We speculate that some of the species of the vegetation type willow found at the study sites, S. laponica, Salix spp. and Comarum palustre, which were abundant within the willow wetland vegetation type, may drive high emissions via root exudation or that those species may reflect specific edaphic conditions that are favourable for CH4 production. Further, root exudation may be an explanation for high emissions also from the vegetation type tall sedge. However, further studies would be required to establish if the high emissions from the vegetation community of willow wetland in our study is consistent across greater spatial ranges as well as the specific drivers of the high emissions. Given the variation in flux rates among waterlogged areas with different vegetation types, it is likely that the species composition within the wide vegetation classes often used for describing permafrost peatlands (raised palsa and fen) directly impact the magnitude of the CH4 emissions as these peatlands degrade. Hence improved understanding of the plant-species-specific mechanisms that control CH4 emissions beyond abiotic drivers (such as waterlogging, redox, soil and air temperature) (Olefeldt et al., 2013) are important for estimations of landscape level CH4 emissions and how these will be altered as these palsa peatlands continue to degrade and their vegetation composition change.
The fact that small extents and local-scale variation in ground or vegetation conditions strongly impact net emissions (Dias et al., 2010; Ström et al., 2005) is important when it comes to developing spatial models for predicting CH4 emissions over large areas. Our three models using Sentinel-2 data differed in their ability to detect the local-scale variation in the vegetation. The hard classification did not succeed in capturing this variability and underestimated the total wetland area. The soft and bias-corrected hard classifications were both successful in estimating the overall area of the target vegetation types compared to the UAV-estimated areas. When the areal estimates from the three models were used for extrapolating the CH4 emissions, only the bias-corrected hard classification estimated the emissions within a error margin of 5 % compared to the UAV-estimated fluxes. The hard classification underestimated fluxes by 21 %, while the soft classification overestimated emissions by 17 %. This means that Sentinel-2 (or other freely available satellite data products, e.g. Landsat) has sufficient spatial resolution to be an effective tool for scaling up CH4 emissions linked to particular vegetation types in the local-scale vegetation mosaic that composes degrading peatland. However, among the three Sentinel-2 models we used, the bias-corrected hard classification clearly outperformed the other two models. Both the uncorrected hard and soft classification would have included considerable error when used to extrapolate the emissions to the regional scale due to scale dependency leading to inaccuracy in the relative distribution of individual land cover classes at coarser resolutions (Hugelius, 2012; Siewert, 2018). This highlights both the risks of substantially increasing the error in the modelled estimates and the opportunity offered by the approach of the bias-corrected hard classification for scaling CH4 emissions using remote sensing data (Olofsson et al., 2014, 2013). The bias-correction methods used in this study are simple, effective and transferable; however, there is a need for ground data if these approaches are applied to new areas. Spatially distributed high-resolution studies and data collection in remote areas such as the Canadian Arctic and Siberia are in the context of permafrost degradation and CH4 feedback of high value and necessary to calibrate regional to Arctic estimates due to high spatial variability in tundra terrain (Siewert et al., 2021; Siewert and Olofsson, 2020).
The implication of this is that current estimates of changes in GHG emissions following permafrost degradations that often only use the distinction between palsas and fen (e.g. Miglovets et al., 2021; Nykänen et al., 2003; Olefeldt et al., 2013; Varner et al., 2022) may result in considerable uncertainty and substantial underestimation of CH4 emissions linked to degradation. This is because, although this simple classification will reflect the major shift from dry to wet conditions and the large increase in CH4 emissions that are associated with this, hot spots of high emissions will be missed as well as variation in CH4 emissions among different vegetation types of fen. The role of different vegetation types is evident from our CH4 data from a range of wetland vegetation communities found at the study sites. These show that variation in CH4 emissions among common vegetation types within the vegetation class “fen” can result in emissions nearly twice as high on an areal basis. Here the detailed vegetation-mapping capabilities of drones provide an important way forward together with higher-resolution aerial photography and satellite data (e.g. WorldView, QuickBird and Planet Lab's “SuperDove” mission when upscaling plot data to the landscape scale) (Siewert et al., 2015; Siewert and Olofsson, 2020).
The relatively low CH4 emissions from areas with high subsidence (Figs. 7–9) reflect both the fact that (i) they represent the permafrost degradation front, which is spatially less extensive than already degraded or stable areas, and (ii) although the subsiding areas of a given vegetation type (Fig. 2) release more CH4 than their intact counterparts, emissions are low compared to areas which are fully degraded and flooded. Further, although CH4 emissions increased as specific vegetation types showed signs of permafrost degradation and subsidence (Fig. 2), the initial increase in CH4 emissions at most of the subsiding sites is modest compared to the high CH4 emissions in areas where degradation has progressed to a point where permafrost has thawed all together and subsidence has slowed down (although some consolidation of the peat material is still ongoing as suggested by subsidence rates at a lower rate in areas which have lost their permafrost).
Our work suggests that subsidence measured using the novel ASPIS InSAR method can be used for assessing the risk of future elevated CH4 emissions linked to areas that are subsiding and will turn into CH4-emitting areas in the near future. The InSAR method also offers a highly sensitive early warning system of the initial stages of degradation. This is valuable because early stages of permafrost degradation are difficult to capture using optical data or ground surveys, which tend to rely on changes in the vegetation or increased surface heterogeneity which only becomes apparent after the degradation has progressed substantially. This is evident in the different areal estimates of the subsiding area using the two different vegetation-mapping approaches with UAV and Sentinel 2 data. Mapping for subsiding areas using the ground truth of UHR UAV optical data estimated the subsiding area of the study sites to be ca. 10 %, while the InSAR data detected subsidence in 90 % of the wetland area. The implication of the InSAR data is that the majority of the palsa peatland area at our study sites is already experiencing permafrost degradation. Indeed, ongoing large-scale permafrost degradation at the sites is further supported by analysis of historical orthophotos and long-term satellite data analysis as well as model predictions that suggest that permafrost in wetlands will no longer be supported in northern Scandinavia by 2050 (de la Barreda-Bautista et al., 2022; Fewster et al., 2022; Varner et al., 2022). This raises concerns about the fate of the ca. 5500 ha of palsa peatlands in Sweden over the next decades (Backe, 2014). The evidence of large-scale degradation at our study sites and the potential for very high CH4 emissions from areas that currently have low emissions present a serious risk linked to climate heating in northern Scandinavia, highlighting the need for regional estimates of the potential for increased CH4 emissions associated with degradation of palsa peatlands.
The code is available at https://doi.org/10.6084/m9.figshare.24248545 (Ledger et al., 2023).
The Sentinel 1 and 2 data are available to download at https://scihub.copernicus.eu/dhus/#/home (Copernicus, ESA, 2023). The orthophoto and methane flux data are available on request.
Supplement Sect. S1 contains Table S1 which presents the Sentinel-2 data specification and wavebands listed with a spatial resolution of 10 m; these were resampled to 20 m to match other wavebands. Supplement Sect. S2 contains an Excel file which presents confusion matrixes for the hard and soft classifications. Supplement Sect. S3 contains TIFF files for the vegetation and methane maps. The supplement related to this article is available online at: https://doi.org/10.5194/bg-20-4221-2023-supplement.
SS conceived and directed the study, carried out fieldwork to assess CH4 fluxes, made a significant contribution to the data analysis and interpretation, and formulated and finalized the text. ML carried out the majority of the data analysis and made a significant contribution to the data interpretation and writing. MS contributed to the conception of the study; advised on the data analysis and interpretation; and contributed to the structuring, writing and refining of the text. DG carried out the initial InSAR data processing. BdlBB contributed to the conception of the study and refining of the text. AS contributed to the conception of the study and advised on the InSAR data processing. GF advised on the data analysis. DSB contributed to the conception of the study, carried out and advised on the data analysis, and contributed to refining the text and finalizing the paper.
The contact author has declared that none of the authors has any competing interests.
Publisher’s note: Copernicus Publications remains neutral with regard to jurisdictional claims in published maps and institutional affiliations.
We are grateful for the fieldwork support from Mattias Dalkvist, Veronica Escubar Ruiz and staff at the Abisko Scientific Research Station. We also acknowledge that the climate data evaluation has been made by data provided by the Abisko Scientific Research Station and the Swedish Infrastructure for Ecosystem Science (SITES). We are grateful to Samuel Valman for advice on data processing.
This paper was edited by Ji-Hyung Park and reviewed by two anonymous referees.
Åkerman, H. J. and Johansson, M.: Thawing permafrost and thicker active layers in sub-arctic Sweden, Permafrost Periglac., 19, 279–292, https://doi.org/10.1002/ppp.626, 2008.
Alshammari, L., Large, D. J., Boyd, D. S., Sowter, A., Anderson, R., Andersen, R., and Marsh, S.: Long-term peatland condition assessment via surface motion monitoring using the ISBAS DInSAR technique over the Flow Country, Scotland, Remote Sens., 10, 1103, https://doi.org/10.3390/rs10071103, 2018.
Backe, S.: Kartering av Sveriges palsmyrar, Länsstyrelsen i Norrbottens län., 2014.
Ballantyne, C. K.: Periglacial Geomorphology, John Wiley and Sons, https://doi.org/10.1111/nzg.12203, 2018.
Bernhardt, E. S., Blaszczak, J. R., Ficken, C. D., Fork, M. L., Kaiser, K. E., and Seybold, E. C.: Control points in ecosystems: Moving beyond the hot spot hot moment concept, Ecosystems, 20, 665–682, https://doi.org/10.1007/s10021-016-0103-y, 2017.
Biskaborn, B. K., Smith, S. L., Noetzli, J., Matthes, H., Vieira, G., Streletskiy, D. A., Schoeneich, P., Romanovsky, V. E., Lewkowicz, A. G., Abramov, A., Allard, M., Boike, J., Cable, W. L., Christiansen, H. H., Delaloye, R., Diekmann, B., Drozdov, D., Etzelmüller, B., Grosse, G., Guglielmin, M., Ingeman-Nielsen, T., Isaksen, K., Ishikawa, M., Johansson, M., Johannsson, H., Joo, A., Kaverin, D., Kholodov, A., Konstantinov, P., Kröger, T., Lambiel, C., Lanckman, J. P., Luo, D., Malkova, G., Meiklejohn I., Moskalenko, N., Oliva, M., Phillips M., Ramos, M., Sannel, A. B. K., Sergeev, D., Seybold, C., Skryabin, P., Vasiliev, A., Wu, Q., Yoshikawa, K., Zheleznyak, M., and Lantuit, H.: Permafrost is warming at a global scale, Nat. Commun., 10, 264, https://doi.org/10.1038/s41467-018-08240-4, 2019.
Borge, A. F., Westermann, S., Solheim, I., and Etzelmüller, B.: Strong degradation of palsas and peat plateaus in northern Norway during the last 60 years, The Cryosphere, 11, 1–16, https://doi.org/10.5194/tc-11-1-2017, 2017.
Chadburn, S. E., Burke, E. J., Cox, P. M., Friedlingstein, P., Hugelius, G., and Westermann, S.: An observation-based constraint on permafrost loss as a function of global warming, Nat. Clim. Change, 7, 340–344, https://doi.org/10.1038/nclimate3262, 2017.
Copernicus, ESA: Copernicus Open Access Hub, https://scihub.copernicus.eu/dhus/#/home, last access: 5 October 2023.
de la Barreda-Bautista, B., Boyd, D. S., Ledger, M., Siewert, M. B., Chandler, C., Bradley, A. V., Gee, D., Large, D. J., Olofsson, J., Sowter, A., and Sjögersten, S.: Towards a monitoring approach for understanding permafrost degradation and linked subsidence in Arctic peatlands, Remote Sens., 14, 444, https://doi.org/10.3390/rs14030444, 2022.
Dias, A. T. C., Hoorens, B., Van Logtestijn, R. S. P., Vermaat, J. E., and Aerts, R.: Plant species composition can be used as a proxy to predict methane missions in peatland ecosystems after land-use changes, Ecosystems, 13, 526–538, https://doi.org/10.1007/s10021-010-9338-1, 2010.
Dzyuban, A.: Intensity of the microbiological processes of the methane cycle in different types of Baltic lakes, Microbiology, 71, 98–104, 2022.
Euskirchen, E. S., Edgar, C. W., Turetsky, M. R., Waldrop, M. P., and Harden, J. W.: Differential response of carbon fluxes to climate in three peatland ecosystems that vary in the presence and stability of permafrost, J. Geophys. Res.-Biogeo., 119, 1576–1595, https://doi.org/10.1002/2014JG002683, 2014.
Fewster, R. E., Morris, P. J., Ivanovic, R. F., Swindles, G. T., Peregon, A. M., and Smith, C. J.: Imminent loss of climate space for permafrost peatlands in Europe and Western Siberia, Nat. Clim. Change, 12, 373–379, https://doi.org/10.1038/s41558-022-01296-7, 2022.
Foody, G. M. and Mathur, A.: A relative evaluation of multiclass image classification by support vector machines, IEEE T. Geosci. Remote, 42, 1335–1343, https://doi.org/10.1109/TGRS.2004.827257, 2004.
Ge, M., Koskinen, M., Korrensalo, A., Mäkiranta, P., Lohila, A., and Pihlatie, M.: Species-specific effects of vascular plants on methane transport in northern peatlands, EGU General Assembly 2022, Vienna, Austria, 23–27 May 2022, EGU22-2574, https://doi.org/10.5194/egusphere-egu22-2574, 2022.
Girkin, N. T.: Tropical Forest Greenhouse Gas Emissions: Root Regulation of Soil Processes and Fluxes, University of Nottingham, https://eprints.nottingham.ac.uk/id/eprint/52949 (last access: 6 October 2023), 2018.
Glagolev, M., Kleptsova, I., Filippov, I., Maksyutov, S., and Machida, T.: Regional methane emission from West Siberia mire landscapes, Environ. Res. Lett., 6, 045214, https://doi.org/10.1088/1748-9326/6/4/045214, 2011.
Grosse, G., Goetz, S., McGuire, A. D., Romanovsky, V. E., and Schuur, E. A. G.: Changing permafrost in a warming world and feedbacks to the Earth system, Environ. Res. Lett., 11, 040201, https://doi.org/10.1088/1748-9326/11/4/040201, 2016.
Hugelius, G.: Spatial upscaling using thematic maps: An analysis of uncertainties in permafrost soil carbon estimates, Global Biogeochem. Cy., 26, GB2026, https://doi.org/10.1029/2011GB004154, 2012.
Hugelius, G. A.-O., Loisel, J. A.-O., Chadburn, S. A.-O. X., Jackson, R. A.-O., Jones, M. A.-O., MacDonald, G., Marushchak, M., Olefeldt, D. A.-O., Packalen, M., Siewert, M. A.-O., Treat, C. A.-O., Turetsky, M., Voigt, C. A.-O., and Yu, Z. A.-O.: Large stocks of peatland carbon and nitrogen are vulnerable to permafrost thaw, P. Natl. Acad. Sci. USA, 117, 20438–20446, https://doi.org/10.1073/pnas, 2020.
IPCC: Climate Change 2021: The Physical Science Basis. Contribution of Working Group I to the Sixth Assessment Report of the Intergovernmental Panel on Climate Change, edited by: Masson-Delmotte, V., Zhai, P., Pirani, A., Connors, S. L., Péan, C., Berger, S., Caud, N., Chen, Y., Goldfarb, L., Gomis, M. I., Huang, M., Leitzell, K., Lonnoy, E., Matthews, J. B. R., Maycock, T. K., Waterfield, T., Yelekçi, O., Yu, R., and Zhou, B., Cambridge University Press, Cambridge, United Kingdom and New York, NY, USA, 2391 pp., https://doi.org/10.1017/9781009157896, 2021.
Johansson, M., Callaghan, T. V., Bosiö, J., Åkerman, H. J., Jackowicz-Korczynski, M., and Christensen, T. R.: Rapid responses of permafrost and vegetation to experimentally increased snow cover in sub-arctic Sweden, Environ. Res. Lett., 8, 035025, https://doi.org/10.1088/1748-9326/8/3/035025, 2013.
Ledger, M., Sjögersten, S., Siewert, M., de la Barreda-Bautista, B., Sowter, A., Gee, D., Foody, G., and Boyd, D.: Generating vegetation and methane maps for sub-Arctic peatlands with degrading permafrost, Figshare [code], https://doi.org/10.6084/m9.figshare.24248545, 2023.
Liebner, S., Ganzert, L., Kiss, A., Yang, S., Wagner, D., and Svenning, M. M.: Shifts in methanogenic community composition and methane fluxes along the degradation of discontinuous permafrost, Front. Microbiol., 6, 356, https://doi.org/10.3389/fmicb.2015.00356, 2015.
Luoto, M. and Seppälä, M.: Thermokarst ponds as indicators of the former distribution of palsas in Finnish Lapland, Permafrost Periglac., 14, 19–27, https://doi.org/10.1002/ppp.441, 2003.
Maksyutov, S., Glagolev, M., Kleptsova, I., Sabrekov, A., Peregon, A., and Machida, T.: Methane emissions from the West Siberian wetlands, American Geophysical Union, Fall Meeting 2010, abstract id. GC41D-02, 2010.
McGuire, A. D., Anderson, L. G., Christensen, T. R., Dallimore, S., Guo, L., Hayes, D. J., Heimann, M., Lorenson, T. D., Macdonald, R. W., and Roulet, N.: Sensitivity of the carbon cycle in the Arctic to climate change, Ecol. Monogr., 79, 523–555, https://doi.org/10.1890/08-2025.1, 2009.
Miglovets, M., Zagirova, S., Goncharova, N., and Mikhailov, O.: Methane emission from palsa mires in northeastern European Russia, Russ. Meteorol. Hydrol., 46, 52–59, 2021.
Mishra, U., Hugelius, G., Shelef, E., Yang, Y., Strauss, J., Lupachev, A., Harden, J. W., Jastrow, J. D., Ping, C. L., Riley, W. J., Schuur, E. A. G., Matamala, R., Siewert, M., Nave, L. E., Koven, C. D., Fuchs, M., Palmtag, J., Kuhry, P., Treat, C. C., Zubrzycki, S., Hoffman, F. M., Elberling, B., Camill, P., Veremeeva, A., and Orr, A.: Spatial heterogeneity and environmental predictors of permafrost region soil organic carbon stocks, Sci. Adv., 7, eaaz5236, https://doi.org/10.1126/sciadv.aaz5236, 2021.
Morin, P., Porter, C., Cloutier, M., Howat, I., Noh, M.-J., Willis, M., Bates, B., Willamson, C., and Peterman, K.: ArcticDEM; a publically available, high resolution elevation model of the Arctic, EGU General Assembly Conference Abstracts, EPSC2016-8396, 2016.
Nykänen, H., Heikkinen, J. E., Pirinen, L., Tiilikainen, K., and Martikainen, P. J.: Annual CO2 exchange and CH4 fluxes on a subarctic palsa mire during climatically different years, Global Biogeochem. Cy., 17, 1018, https://doi.org/10.1029/2002GB001861, 2013.
Olefeldt, D., Roulet, N. T., Bergeron, O., Crill, P., Bäckstrand, K., and Christensen, T. R.: Net carbon accumulation of a high-latitude permafrost palsa mire similar to permafrost-free peatlands, Geophys. Res. Lett., 39, L03501, https://doi.org/10.1029/2011GL050355, 2012.
Olefeldt, D., Turetsky, M. R., Crill, P. M., and McGuire, A. D.: Environmental and physical controls on northern terrestrial methane emissions across permafrost zones, Glob. Change Biol., 19, 589–603, 2013.
Olofsson, P., Foody, G. M., Stehman, S. V., and Woodcock, C. E.: Making better use of accuracy data in land change studies: Estimating accuracy and area and quantifying uncertainty using stratified estimation, Remote Sens. Environ., 129, 122–131, https://doi.org/10.1016/j.rse.2012.10.031, 2013.
Olofsson, P., Foody, G. M., Herold, M., Stehman, S. V., Woodcock, C. E., and Wulder, M. A.: Good practices for estimating area and assessing accuracy of land change, Remote Sens. Environ., 148, 42–57, https://doi.org/10.1016/j.rse.2014.02.015, 2014.
Olvmo, M., Holmer, B., Thorsson, S., Reese, H., and Lindberg, F.: Sub-arctic palsa degradation and the role of climatic drivers in the largest coherent palsa mire complex in Sweden (Vissátvuopmi), 1955–2016, Sci. Rep.-UK, 10, 8937, https://doi.org/10.1038/s41598-020-65719-1, 2020.
Powell, R. L., Matzke, N., Souza, C., Clark, M., Numata, I., Hess, L., and Roberts, D.: Sources of error in accuracy assessment of thematic land-cover maps in the Brazilian Amazon, Remote Sens. Environ., 90, 221–234, https://doi.org/10.1016/j.rse.2003.12.007, 2004.
Sannel, A. B. K. and Kuhry, P.: Warming-induced destabilization of peat plateau/thermokarst lake complexes, J. Geophys. Res.-Biogeo., 116, G03035, https://doi.org/10.1029/2010JG001635, 2011.
Sannel, A. B. K., Hugelius, G., Jansson, P., and Kuhry, P.: Permafrost warming in a subarctic peatland – which meteorological controls are most important?, Permafrost Periglac., 27, 177–188, https://doi.org/10.1002/ppp.1862, 2016.
Schneider von Deimling, T., Meinshausen, M., Levermann, A., Huber, V., Frieler, K., Lawrence, D. M., and Brovkin, V.: Estimating the near-surface permafrost-carbon feedback on global warming, Biogeosciences, 9, 649–665, https://doi.org/10.5194/bg-9-649-2012, 2012.
Schuur, E. A. G. and Abbott, B.: High risk of permafrost thaw, Nature, 480, 32–33, https://doi.org/10.1038/480032a, 2011.
Siewert, M. B.: High-resolution digital mapping of soil organic carbon in permafrost terrain using machine learning: a case study in a sub-Arctic peatland environment, Biogeosciences, 15, 1663–1682, https://doi.org/10.5194/bg-15-1663-2018, 2018.
Siewert, M. B. and Olofsson, J.: Scale-dependency of Arctic ecosystem properties revealed by UAV, Environ. Res. Lett., 15, 094030, https://doi.org/10.1088/1748-9326/aba20b, 2020.
Siewert, M. B., Hanisch, J., Weiss, N., Kuhry, P., Maximov, T. C., and Hugelius, G.: Comparing carbon storage of Siberian tundra and taiga permafrost ecosystems at very high spatial resolution, J. Geophys. Res.-Biogeo., 120, 1973–1994, https://doi.org/10.1002/2015JG002999, 2015.
Siewert, M. B., Lantuit, H., Richter, A., and Hugelius, G.: Permafrost causes unique fine-scale spatial variability across tundra soils, Global Biogeochem. Cy., 35, e2020GB006659, https://doi.org/10.1029/2020GB006659, 2021.
Sjöberg, Y., Marklund, P., Pettersson, R., and Lyon, S. W.: Geophysical mapping of palsa peatland permafrost, The Cryosphere, 9, 465–478, https://doi.org/10.5194/tc-9-465-2015, 2015.
Sjöberg, Y., Siewert, M. B., Rudy, A. C. A., Paquette, M., Bouchard, F., Malenfant-Lepage, J., and Fritz, M.: Hot trends and impact in permafrost science, Permafrost Periglac., 31, 461–471, https://doi.org/10.1002/ppp.2047, 2020.
Sjögersten, S., Caul, S., Daniell, T. J., Jurd, A. P. S., O'Sullivan, O. S., Stapleton, C. S., and Titman, J. J.: Organic matter chemistry controls greenhouse gas emissions from permafrost peatlands, Soil Biol. Biochem., 98, 42–53, https://doi.org/10.1016/j.soilbio.2016.03.016, 2016.
Ström, L. and Christensen, T. R.: Below ground carbon turnover and greenhouse gas exchanges in a sub-arctic wetland, Soil Biol. Biochem., 39, 1689–1698, https://doi.org/10.1016/j.soilbio.2007.01.019, 2007.
Ström, L., Ekberg, A., Mastepanov, M., and Røjle Christensen, T.: The effect of vascular plants on carbon turnover and methane emissions from a tundra wetland, Glob. Change Biol., 9, 1185–1192, https://doi.org/10.1046/j.1365-2486.2003.00655.x, 2003.
Ström, L., Mastepanov, M., and Christensen, T. R.: Species-specific effects of vascular plants on carbon turnover and methane emissions from wetlands, Biogeochemistry, 75, 65–82, https://doi.org/10.1007/s10533-004-6124-1, 2005.
Tarnocai, C., Canadell, J. G., Schuur, E. A. G., Kuhry, P., Mazhitova, G., and Zimov, S.: Soil organic carbon pools in the northern circumpolar permafrost region, Global Biogeochem Cy., 23, GB2023, https://doi.org/10.1029/2008GB003327, 2009.
Turetsky, M. R., Abbott, B. W., Jones, M. C., Anthony, K. W., Olefeldt, D., Schuur, E. A. G., Grosse, G., Kuhry, P., Hugelius, G., Koven, C., Lawrence, D. M., Gibson, C., Sannel, A. B. K., and McGuire, A. D.: Carbon release through abrupt permafrost thaw, Nat. Geosci., 13, 138–143, https://doi.org/10.1038/s41561-019-0526-0, 2020.
van Huissteden, J., Teshebaeva, K., Cheung, Y., Magnússon, R. Í., Noorbergen, H., Karsanaev, S. V., Maximov, T. C., and Dolman, A. J.: Geomorphology and InSAR-tracked surface displacements in an ice-rich Yedoma landscape, Front. Earth Sci., 9, 680565, https://doi.org/10.3389/feart.2021.680565, 2021.
Varner, R. K., Crill, P. M., Frolking, S., McCalley, C. K., Burke, S. A., Chanton, J. P., Holmes, M. E., Saleska, S., and Palace, M. W.: Permafrost thaw driven changes in hydrology and vegetation cover increase trace gas emissions and climate forcing in Stordalen Mire from 1970 to 2014, Philos. T. R. Soc. A, 380, 20210022, https://doi.org/10.1098/rsta.2021.0022, 2022.
Walter, K. M., Zimov, S., Chanton, J. P., Verbyla, D., and Chapin, F. S.: Methane bubbling from Siberian thaw lakes as a positive feedback to climate warming, Nature, 443, 71–75, 2006.
Walter Anthony, K., Daanen, R., Anthony, P., Schneider von Deimling, T., Ping, C.-L., Chanton, J. P., and Grosse, G.: Methane emissions proportional to permafrost carbon thawed in Arctic lakes since the 1950s, Nat. Geosci., 9, 679–682, https://doi.org/10.1038/ngeo2795, 2016.
Xie, Y., Sha, Z., and Yu, M.: Remote sensing imagery in vegetation mapping: a review, J. Plant Ecol., 1, 9–23, https://doi.org/10.1093/jpe/rtm005, 2008.
Zuidhoff, F. S. and Kolstrup, E.: Changes in palsa distribution in relation to climate change in Laivadalen, northern Sweden, especially 1960–1997, Permafrost Periglac., 11, 55–69, https://doi.org/10.1002/(SICI)1099-1530(200001/03)11:1<55::AID-PPP338>3.0.CO;2-T, 2000.
The requested paper has a corresponding corrigendum published. Please read the corrigendum first before downloading the article.
- Article
(3323 KB) - Full-text XML
- Corrigendum
-
Supplement
(5270 KB) - BibTeX
- EndNote