the Creative Commons Attribution 4.0 License.
the Creative Commons Attribution 4.0 License.
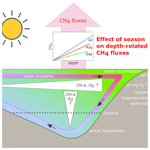
Spatial and temporal variability of methane emissions and environmental conditions in a hyper-eutrophic fishpond
Petr Znachor
Jiří Nedoma
Vojtech Kolar
Estimations of methane (CH4) emissions are often based on point measurements using either flux chambers or a transfer coefficient method, which may lead to strong underestimation of the total CH4 fluxes. In order to demonstrate more precise measurements of the CH4 fluxes from an aquaculture pond, using a higher resolution sampling approach we examined the spatiotemporal variability of CH4 concentrations in the water, related fluxes (diffusive and ebullitive) and relevant environmental conditions (temperature, oxygen, chlorophyll a) during three diurnal campaigns in a hyper-eutrophic fishpond. Our data show remarkable variance spanning several orders of magnitude while diffusive fluxes accounted for only a minor fraction of total CH4 fluxes (4.1 %–18.5 %). Linear mixed-effects models identified water depth as the only significant predictor of CH4 fluxes. Our findings necessitate complex sampling strategies involving temporal and spatial variability for reliable estimates of the role of fishponds in a global methane budget.
- Article
(9829 KB) - Full-text XML
-
Supplement
(2097 KB) - BibTeX
- EndNote
Freshwater aquaculture ponds (fishponds) represent artificial counterparts to natural shallow lakes (Scheffer, 2004) which are mainly used for fish production (mostly of common carp, Cyprinus carpio L.) and water retention in the landscape. Fishponds serve also as secondary biotope for various organisms (Kolar et al., 2021), supporting noteworthy animal and plant diversity (Pokorný and Hauser, 2002). However, most fishponds suffer from high fish stock densities, excessive carbon and nutrient loading from supplemental fish feeding, sewage pollution, and fertiliser runoffs from agricultural catchments or nutrient mobilisation from the anoxic sediment layers (Pechar, 2000). As a result, the trophic structure of plankton communities has shifted towards a reduction of large zooplankton and massive development of phytoplankton, especially cyanobacterial blooms (Potužák et al., 2007), limiting light penetration in the water column. Rapid changes in the intensity of biological processes, such as photosynthesis and respiration, often result in pronounced daily or seasonal fluctuations in dissolved oxygen (Baxa et al., 2021), signalling decreasing ecosystem stability. The extent of anoxia, accumulation of organic biomass, and rapid heating of the shallow water during the summer result in enhanced production of greenhouse gases (Grasset et al., 2018; Zhang et al., 2021; Bartosiewicz et al., 2021).
Most concerning are CH4 emissions as freshwater aquaculture systems release more than 6 Tg CH4 yr−1 (Yuan et al., 2019). Methane can be emitted via several pathways: simple molecular diffusion, ebullition (in the form of bubbles released from oversaturated sediments), plant-mediated flux (Bastviken et al., 2004), but also through so far neglected pathways including aeration, emissions from dry and/or drying sediments, or dredged organic material (Kosten et al., 2020). Among these, ebullition is considered the dominant pathway (van Bergen et al., 2019; Kosten et al., 2020), which can contribute 50 %–96 % (Casper et al., 2000; Xiao et al., 2017; van Bergen et al., 2019; Yang et al., 2020; Zhao et al., 2021) to the total CH4 flux. Along with the second important pathway, molecular diffusion, both exhibit high spatiotemporal variability due to various physical and biological factors acting on very short timescales, for instance, temperature (van Bergen et al., 2019), nutrient loading (Zhang et al., 2021), CH4 production rates (Zhou et al., 2019), CH4 oxidation rates (Sanseverino et al., 2012), dissolved oxygen concentration (Xiao et al., 2017), management regimen (Yang et al., 2019), or the quality of organic matter in the sediment (Schmiedeskamp et al., 2021). Recently, the potential involvement of phytoplankton in CH4 production and emissions has been suggested (Yan et al., 2019; Bižić et al., 2020; Bartosiewicz et al., 2021). The complex interactions between physical and biological factors lead to a dynamic and ever-changing environment, characterised by high spatial and temporal variability of methane fluxes in ponds.
Although fishponds are recognised as powerful model systems for studies in ecology and evolutionary or conservation biology (De Meester et al., 2005; Céréghino et al., 2008), the extent of environmental heterogeneity in fishponds and shallow inland small waterbodies remains poorly understood (Ortiz and Wilkinson, 2021), largely because the driving factors are either system-specific or highly variable on short timescales (Laas et al., 2012). Most of current information on lentic ecosystem structure and function comes from single-site sampling, in which measurements are taken over time at the deepest point in the lake, which does not sufficiently account for within-lake spatial variation (Stanley et al., 2019). The motivation for our study was the growing concern about the role of fishponds as important sources of CH4 fluxes to the atmosphere (Wik et al., 2016). Unfortunately, the majority of global CH4 flux estimates rely on upscaling methods (DelSontro et al., 2018a) based on a limited number of measurements that do not account for diurnal and seasonal variability or ecosystem spatial heterogeneity. Yang et al. (2019) indicated that a larger number of spatial replicates over a number of months is mandatory to improve the accuracy of whole pond CH4 flux estimates. The published research from other aquaculture studies have been performed mainly in tropical and subtropical zones in fish or crab aquacultures (e.g. Hu et al., 2016; Ma et al., 2018; Yang et al., 2019, 2020; Yuan et al., 2019, 2021). To better understand the spatial dynamics of CH4 fluxes and environmental heterogeneity in temperate freshwater shallow lakes, we conducted a spatial sampling of the hyper-eutrophic Dehtář fishpond (Czech Republic, Europe). As the seasonal CH4 production is strongly affected by temperature, we focused on warm summer months where the total CH4 fluxes were expected to be the highest (Jansen et al., 2019). The objectives of our study were (i) to determine the spatial heterogeneity of CH4 diffusive and total fluxes and fundamental limnological variables (oxygen, temperature, chlorophyll a) and their change daily and monthly in the hyper-eutrophic pond, and (ii) to identify the factors that influence CH4 fluxes to improve our understanding of the importance of spatiotemporal variability for global estimates of CH4 efflux to the atmosphere.
2.1 Study site description
The Dehtář fishpond (49∘00′24.4′′ N, 14∘7′39.3′′ E) is a shallow artificial lake (average and maximum depth: 2.4 and 6 m, respectively) constructed in 1479 (Potužák et al., 2016). It is used for polycultural, semi-intensive production of common carp, which account for 90 %–95 % of the fish biomass (Rutegwa et al., 2019). The pond is stocked with 2-year-old carp harvested at the end of a 2-year production cycle. To increase fish production, the original management based mainly on natural processes, has been intensified, and today manuring and supplementary feeding in the form of grain or fish pellets, are common practices (Pechar, 2000).The pond lies in a flat agricultural landscape at 406.4 m (a.s.l. (above sea level) in the upper Vltava River basin in South Bohemia (Czech Republic) which is characteristic with its network of fishponds (Fig. 1b). Due to the orography of the landscape, the Dehtář fishpond, surrounded by narrow belts of littoral vegetation and adjacent to grassland and arable land, is exposed to wind, mainly from the northwest (for aerial photograph, see Fig. S1 in the Supplement). The catchment area is 91.4 km2. The main inflow is the Dehtářský stream in the south, while several smaller tributaries flow in from the west (Fig. 1c). The fishpond has a dam 234 m long and 10 m high, with two outlets and a safety spillway. Covering 2.28 km2, the Dehtář fishpond is among the 10 largest fishponds in the Czech Republic, holding a volume of 4.71×103 m3 and having a water residence time of 146–445 d (Potužák et al., 2016).
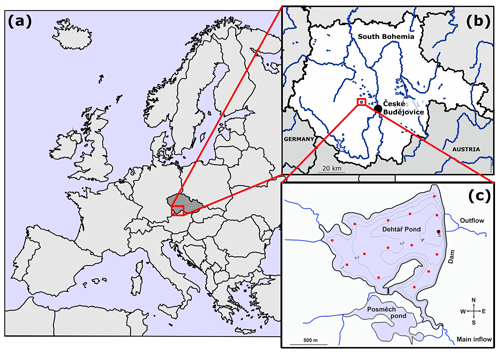
Figure 1Location (a, b; © D-maps.com, 2013a, b; modified) and bathymetric map (c; credited to Jiří Jarošík) of the sampled Dehtář fishpond: blue lines indicate hydrological connections; red dots represent the sampling points. Highlighted sampling point at the dam depicts the deepest site where vertical profiles were measured. Numbers indicate isobath depth.
2.2 Sampling design and measurement
To measure spatial heterogeneity and temporal changes in limnological parameters and methane fluxes, we conducted three surveys each of 36 h in summer 2019 (2–3 July, 13–14 August, 19–20 September). In the morning (between 05:00–06:00 LT), we first measured surface values and vertical profiles of temperature, oxygen, and chlorophyll a concentration at the deepest point (see later for details). We subsequently installed 15 floating polyethylene chambers (as shown in Fig. 1c), serving as fixed sampling sites and at the same time for accumulation of CH4 fluxes (see further), starting in the western part of the fishpond. During installation (and during each sampling), temperature, pH, and oxygen concentrations were measured at 0.3 m depth using the WTW 330i pH meter, and oximeter (WTW, Weilheim, Germany). Vertical chlorophyll a profiles were measured at each sampling site using a submersible fluorescence probe (FluoroProbe, bbe Moldaenke, Kiel, Germany). From each site, the average chlorophyll a concentration in the surface layer (0–1 m depth) was used to assess the phytoplankton spatial heterogeneity.
To minimise the chance that the differences observed among sites were due to the time of day, we conducted repeated measurements at the deepest point at the end of each sampling. This was relevant mainly to the initial measurement, when the installation of all floating chambers took a total of 3 h and 50 min. All other measurements, i.e. the interval between the first and last sampling point, required approximately 2 h each. If there was a change all values were corrected for the sampling time by linear interpolation:
where Pcorr is the corrected value of a parameter, Pt is its value measured at the time t, P0 and Pend are parameter values measured at the deepest point at the start (time t0) and at the end (tend) of the sampling. In the evening and morning of the second day (roughly a 12 h interval), we performed additional measurements of spatial heterogeneity, allowing us to assess diurnal and nocturnal changes. In addition, samples for measuring CH4 concentration in the surface water were collected at each site and analysed as described later. To assess diurnal variations in thermal structure and oxygen concentration in the water column, we made vertical profile measurements at the deepest point (Fig. 1c) at 3–6 h intervals using the YSI EXO 2 multiparametric probe (YSI Inc., Yellow Springs, USA).
2.3 Methane measurements
Water samples for determining CH4 concentrations in the surface water were collected at all 15 sampling sites in triplicate into 20 mL glass bottles. The bottles were capped bubble-free under water with black butyl rubber stoppers (Ochs, Germany) and sealed with aluminium crimps. Immediately after sampling, the water samples were preserved by injecting 100 µL of concentrated sulfuric acid to stop the microbial activity (Bussmann et al., 2015). The samples were processed within one week in the laboratory using a headspace technique according to McAuliffe (1971). The methane concentration in the headspace was measured using an HP 5890 Series II gas chromatograph (Agilent Technologies, USA) and calculated with the solubility coefficient given by Yamamoto et al. (1976).
Methane diffusive fluxes (F) were then calculated for each sampling site indirectly using the two-layer model with the equation:
where Csur is the CH4 concentration in surface water in µmol L−1, Ceq is the CH4 concentration in surface water in equilibrium with the atmosphere in µmol L−1, and k is the CH4 exchange constant (cm h−1). The atmospheric partial pressure of CH4 was set to 1.8 ppm. To compute k values, we first derived k600 estimates using a wind speed-based relationship according to Crusius and Wanninkhof (2003):
where U10 represents the wind speed at a height of 10 m (in m s−1; obtained from the nearby gauging station) approximated by U10=1.22 U, where U is the wind speed at 1.5 m height. We then converted k600 to k using the Eq. (4) according to Crusius and Wanninkhof (2003):
where k600 is the gas transfer velocity for a Schmidt number (Sc) of 600; n is a wind speed-dependent conversion factor, for which we used for U10<3.7 m s−1 (Jähne et al., 1987). The Schmidt number for CH4 was calculated according to Wanninkhof (2014):
where t (∘C) is the water temperature at the time of CH4 extraction. The parameter Ceq in Eq. (1) was determined from the equation:
where β is the solubility coefficient of CH4 as a function of temperature according to Wiesenburg and Guinasso (1979), and pCH4 is the partial pressure of CH4 in the atmosphere.
To estimate total CH4 fluxes from the water column to the atmosphere (i.e. diffusive and ebullitive fluxes), we measured CH4 accumulation in open-bottomed floating polyethylene chambers (volume 3.1 L, area 0.024 m2). Each gas chamber was anchored at 15 individual fixed sampling sites but allowed to float freely on the water surface. Gas was allowed to accumulate for approximately 12 h (each incubation had a starting and end point) during a particular sampling period, i.e. during the day and night periods. Afterwards, 30 mL of gas was carefully taken from each chamber, after mixing the headspace in the chamber, and stored in evacuated Exetainers® (Labco Limited, UK). Chambers were ventilated after each sampling period to reset the incubation conditions. Methane fluxes were calculated as the difference between initial background and final concentrations in the chamber headspace and expressed as the 1 m2 area of the surface level per day according to Bastviken et al. (2004).
2.4 Background limnological parameters
During each campaign water samples for analysis of nutrient concentration and phytoplankton composition were collected from the surface at the deepest point using a Friedinger sampler. Water transparency was measured using a Secchi disc. Total phosphorus (TP) and soluble reactive phosphorus (SRP) were analysed spectrophotometrically according to Kopáček and Hejzlar (1993) and Murphy and Riley (1962), respectively. Concentrations of NH and NO were determined according to the procedure of Kopáček and Procházková (1993) and Procházková (1959), respectively. Phytoplankton samples were preserved with Lugol's solution and examined for species composition with an inverted microscope (Olympus IMT-2). Weather data were obtained from the gauging station at the fishpond dam.
2.5 Statistical analyses
Two-tailed paired Student's t tests and two-way ANOVA with post hoc Tukey's multiple comparison test (Prism 9.3, GraphPad Software Inc., La Jolla, CA, USA) were used to test for differences between diffusive and total CH4 fluxes between day and night and among three sampling campaigns. The percentage of data variability explained by different factors (daytime, month and site) was calculated with the two-way repeated measures (RM) ANOVA. Contour graphs illustrating changes in spatial heterogeneity of measured parameters were constructed in Surfer 10 (Golden Software Inc., CO, USA) using the kriging contouring method. Spatial heterogeneity was quantified for each sampling by calculating the spatial variance (i.e. coefficient of variation of values measured at 15 sampling sites; see, e.g. Fig. 2):
Higher spatial variance indicates increasing ecosystem patchiness. Linear mixed-effects models were used to analyse the effects of O2, pH, temperature, and water depth on the CH4 diffusive fluxes with the random effect of time of day nested within the effect of the sampling date. The most parsimonious model was obtained by a manual backward selection, where we sequentially removed all insignificant predictors (p>0.05) using likelihood ratio tests implemented in the drop1 function (Zuur et al., 2009). We also compared the slopes of the month-specific regression lines produced by the model using analysis of covariance (Zar, 1984). Linear mixed-effects models were implemented in the lme4 package version 1.1-21 (Bates et al., 2015), and Kenward–Roger F tests were computed using the ANOVA Type II function from the pbkrtest package version 0.4-7 (Halekoh and Hojsgaard, 2014). The prediction of the resulting final model was visualised in the package ggeffects version 0.14.1 (Lüdecke, 2018). Package performance version 0.4.4 (Lüdecke et al., 2020) was used to calculate Nakagawa's R2 of the linear model. The statistical analyses were performed using R software (v. 3.5.2, R Core Team, 2018).
3.1 Weather and background fishpond characteristics
Weather parameters varied among sampling campaigns. In July, clear skies prevailed with the daily air temperature above 30 ∘C (Table 1). During the August and September measurements it was very cloudy and daily air temperatures decreased to 22 and 18 ∘C, respectively. The water level was stable during the whole study period with a monthly fluctuation of ∼10 cm. Water transparency was low (15–40 cm), with an increasing trend towards the end of summer (Table 1). Concentrations of total phosphorus and soluble reactive phosphorus were high (Table 1), consistent with a hyper-eutrophic state of the fishpond. In contrast, nitrogen concentrations were relatively low, with ammonium nitrogen being the predominant form of inorganic N in the water (Table 1).
Table 1Basic characteristics of the Dehtář fishpond during the studied period, measured at the surface at the deepest point.
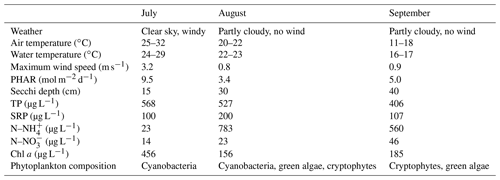
Chlorophyll a concentrations were highest in July due to the dense cyanobacterial bloom accumulated at the surface (Table 1). The phytoplankton consisted of only three cyanobacterial taxa: Dolichospermum flos-aquae, Planktothrix agardhii, and Raphidiopsis mediterranea, and Cuspidothrix issatschenkoi. In August, the phytoplankton was more diverse but also dominated by cyanobacteria: P. agardhii, Aphanizomenon issatschenkoi, and D. flos-aquae. In September, cyanobacteria were absent and instead, cryptophytes (Cryptomonas reflexa), green algae (Pediastrum, Coelastrum and Desmodesmus) and dinoflagellates (Ceratium hirundinella) prevailed.
3.2 Methane concentration and fluxes
The CH4 concentration in surface water was highly supersaturated over the whole study period. The values obtained varied from 0.003 up to 3.75 µmol L−1 (Fig. 2), which corresponded to saturation levels of 108 %–12 834 %. It is obvious that the obtained data showed remarkable variance: the mean (± SD) values were 0.22±0.18 for July, 0.34±0.45 for August, and 1.61±0.61 µmol L−1 for September (Fig. S11).
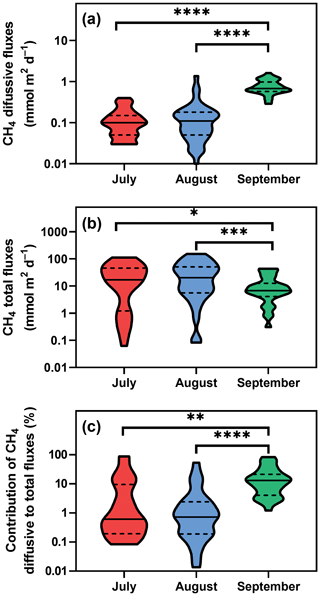
Figure 3Violin plots of CH4 diffusive (a) and total fluxes (b) during the studied period. Panel (c) depicts differences in the percentage contribution of diffusive to total fluxes. Solid lines are medians, and dashed lines denote quartiles. Asterisks indicate significant differences (* p<0.05, p<0.01, p<0.001, p<0.0001) between sampling dates determined by two-way ANOVA with Tukey's multiple comparison test. Note that a log scale is used here for clarity.
Diffusive fluxes (i.e. calculated from CH4 concentration, see Eq. 2) showed the lowest values in July and August (average 0.12 and 0.16 mmol m−2 d−1, respectively) and pronouncedly peaked in September (average 0.78 mmol m−2 d−1, Fig. 3a). By contrast, in July and August, the average total CH4 fluxes (obtained with floating chambers) showed the highest values (average 31.8 mmol m−2 d−1; ranging from 0.08 to 152 mmol m−2 d−1) while in September, total CH4 fluxes were 3 times lower than before (average 11.8 mmol m−2 d−1, range 0.3–43.5 mmol m−2 d−1, Fig. 3b). As a result, diffusive fluxes accounted for only a minor fraction of total CH4 fluxes to the atmosphere (on average, 9.2 % in July, 4.1 % in August and 18.5 % in September, Fig. 3c).
The total CH4 fluxes showed spatial variability within the fishpond that ranged over four orders of magnitude (Figs. 3, 4 and S11; Table S1 in the Supplement). The observed spatial pattern showed high temporal variability on both daily and monthly scales (Figs. 2 and 4, Table S1). Most of the variability in CH4 diffusive fluxes was explained by the sampling date (62.4 %), while for the total CH4 fluxes, spatial heterogeneity accounted for 87.2 % of data variability (Table 2). Using linear mixed-effects models, we identified water depth as the only significant predictor of total CH4 fluxes (df=1, p<0.0001, marginal Nakagawa's R2=0.348; Fig. 5).
Table 2The percentage of data variability explained by different factors (daytime, month = sampling date, and site) calculated with the two-way repeated measures ANOVA. Statistically significant values (p<0.01) are in bold type.
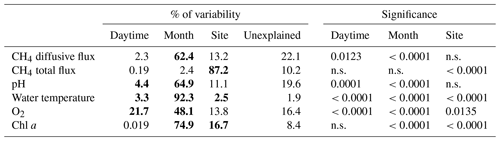
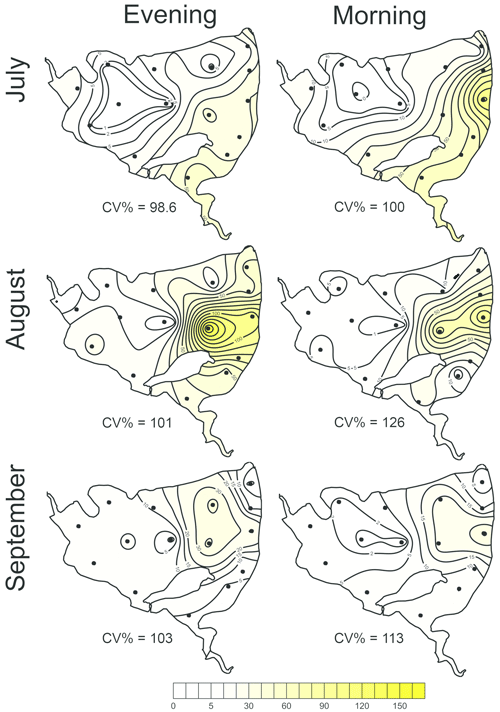
Figure 4Contour graphs of methane total fluxes in the Dehtář fishpond. Isopleths connect sites with the same value of methane fluxes (mmol m−2 d−1). CV % is a measure of spatial heterogeneity. Black dots representing the sampling sites.
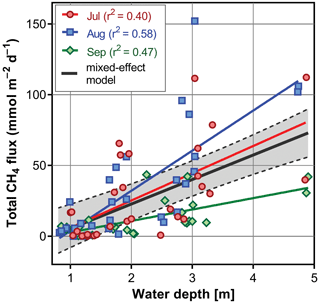
Figure 5The most parsimonious linear mixed-effect model of methane total fluxes showing the water depth as the only significant predictor. Symbols are the measured values, the solid black line is the prediction, and dashed lines are 95% confidence intervals. Colours indicate the month-specific relationship between total methane fluxes and water depth. Differences in slopes were tested using the F test. In September the slope of the regression line was significantly different from those in July and August.
Interestingly, the slopes of the linear regressions differed significantly among individual sampling campaigns (Fig. 5), indicating an additional season-related factor that affects CH4 fluxes in the fishpond. Calculated CH4 diffusive fluxes were not correlated with total fluxes. Linear mixed-effects models did not identify any significant predictor of the fluxes, indicating that factors and processes out of the study's scope are involved. We found no significant difference in either diffusive or total CH4 fluxes between day and night.
3.3 Diurnal changes in vertical profiles of oxygen and temperature
Several contrasting patterns of vertical temperature and oxygen profiles occurred during summer 2019. Diurnal changes were most pronounced in July (Fig. 6). Surface temperatures varied from 25 ∘C in the morning to nearly 30 ∘C in the afternoon. Thermal stratification of the water column was weak in the morning but became strongest at 14:30 LT with a thermocline at 0.5 m depth (Fig. 6). Later in the afternoon, the water column began to be mixed by wind. The morning vertical oxygen profile was characterised by a surface value of 4.3 mg L−1, corresponding to 51 % saturation and anoxia below 3 m.
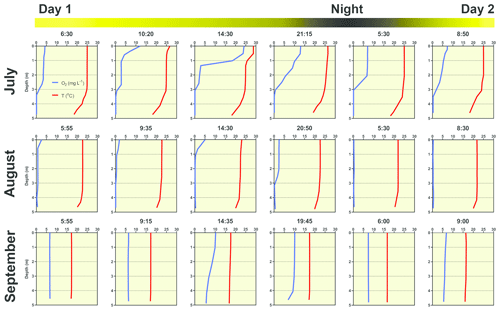
Figure 6Diurnal changes in vertical profiles of temperature and oxygen concentration measured at the deepest point of the fishpond. Numbers above each graph indicate the time of measurement.
Due to the high photosynthetic activity of cyanobacteria, the surface oxygen concentration increased to 24 mg L−1 (320 % saturation, Fig. 6), and a steep oxycline was established at a depth of 0.5–1.5 m with no effect on the anoxic conditions at the deeper layers. Wind action eroded both the oxyclines and thermoclines in the evening, and by the next morning, the vertical profiles were similar to those at the beginning.
In August the water column was almost entirely mixed and low in oxygen in the morning, with only 2.6 mg L−1 (30 % saturation) of oxygen at the surface. Due to cloudy weather, the daily photosynthetic activity of phytoplankton resulted in only a slight increase in oxygen concentration at 0–1.5 m depth (4 mg L−1, 47 % saturation). By the morning of the next day, the entire water column turned very close to anoxic (0.4 mg L−1, 4 % saturation; Fig. 6), which in turn affected the spatial distribution of zooplankton, as evidenced by the formation of dense zooplankton clouds accumulated in the thin layer just at the surface (see Fig. S3). In September, the water column was completely mixed, and we observed only weak daily changes in thermal and oxygen vertical structures (Fig. 6).
3.4 Effect of wind on spatial heterogeneity of temperature, oxygen and chlorophyll a
During the summer all measured parameters showed remarkable within-lake spatial heterogeneity (Figs. S5–S8). In July meteorological conditions enabled demonstration of the effect of wind on fishpond spatial heterogeneity. In the morning, there were no substantial differences in the surface temperature and oxygen concentrations (Fig. 7a and b). The phytoplankton biomass was accumulated mostly in the shallow western part, with the maximum in the centre (Fig. 7c). At 14:00 LT a light breeze started to blow from the northwest, achieving a maximum of 3.2 m s−1 (Fig. S9). This episode lasted until the evening measurement, and the wind ceased by 21:00 LT. The wind was strong enough to substantially change the spatial distribution (Figs. 7d–f and S4). In the evening, the surface water temperature on the windward (south) side of the fishpond was ∼4 ∘C higher than on the north side (Fig. 7d). The wind also induced order of magnitude differences in oxygen concentration along the north–south axis of the fishpond (3 mg L−1 of O2 at the north, 24 mg L−1 of O2 at the south; Fig. 7e) and affected the phytoplankton distribution in the fishpond, resulting in remarkable bloom accumulation in the south (Figs. 7f and S8). During the calm night after the disturbance, the north–south gradient substantially weakened. In August and September the thermal heterogeneity of the pond was relatively low, but the spatial distribution of oxygen and chlorophyll a remained highly variable (Figs. S5–S8, Table S1).
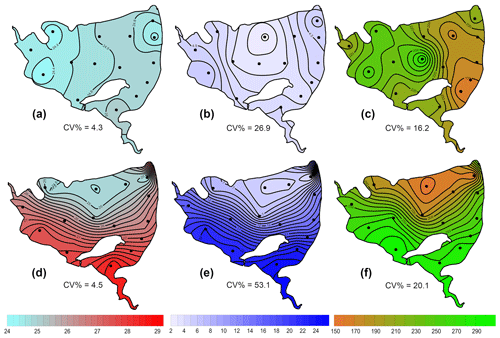
Figure 7Contour graphs of surface temperature (a, d; ∘C), oxygen concentration (b, e; mg L−1) and chlorophyll a concentration (c, f; µg L−1) measured on 2 July at different times of day: (a)–(c) are the morning measurements; (d)–(f) are evening measurements following a wind disturbance. Coefficient of variation (CV %) is a measure of spatial heterogeneity of measured parameters. Black dots representing the sampling sites.
4.1 Methane fluxes
Assessing the spatial heterogeneity of the CH4 fluxes within a fishpond is critical for a reliable estimate of its contribution to the global CH4 budget. In our study, the variability in total CH4 fluxes spanned several orders of magnitude (ranging from 0.06 up to 1121.3 mmol m−2 d−1), which is in agreement with similar studies (Casper et al., 2000; DelSontro et al., 2016; Natchimuthu et al., 2016). However, most system-specific CH4 flux estimates relied on upscaling from a limited number of sites (Bastviken et al., 2004; Rasilo et al., 2015; Wik et al., 2016) because obtaining spatial variability in CH4 emission is methodologically challenging. In general, spatial heterogeneity may reflect differences in water sources, physical mixing, local transformations and biogeochemical processes and rates among lake habitats (Loken et al., 2019). In deep lakes littoral areas can contribute disproportionately to total lake CH4 fluxes (Hofmann et al., 2010; Hofmann, 2013; Natchimuthu et al., 2016; Schilder et al., 2013) and are often missed by traditional sampling approaches (Wik et al., 2016). According to Wik et al. (2016), low temporal and spatial resolutions are unlikely to cause overestimates. On the other hand, DelSontro et al. (2018b) suggested that horizontal transport of CH4 produced in littoral zones and the interaction between physical and biological processes (e.g. air–water gas exchange, water column mixing, the interplay between CH4 production and microbial oxidation) may result in an underestimation of whole-lake CH4 fluxes based on centre samples. Similarly, Natchimuthu et al. (2016) found that up to 78 % underestimation would occur if samples obtained only from the lake centre are used to extrapolate the total CH4 flux. However, extrapolating our data from the deepest point of the Dehtář fishpond would lead to an overestimation of the CH4 fluxes by a factor of 2.9 (Fig. S12).
The bias introduced by the deepest point measurement appears to be highly variable among systems with different morphology, geographical location, mixing regimes or trophic states. For instance, analysis of 22 European lakes during late summer has shown that spatially resolved CH4 diffusive fluxes were highly variable for individual lakes, yielding 55 %–300 % differences in the whole-lake estimates (Schilder et al., 2013). Schmiedeskamp et al. (2021) observed an increase in CH4 fluxes from the shore towards the centre in response to increasing sediment C-content in two shallow German lakes. In line with these findings, our results provide further evidence that spatially resolved data are needed to validate the uncertainties that come from using single-point samples to represent whole-lake processes in hyper-eutrophic systems. As stated by Loken et al. (2019) rather than assuming spatial homogeneity, scaling-up exercises of global carbon budgets should acknowledge the uncertainty that comes from extrapolating from spatially limited data sets.
In the Dehtář fishpond, the total CH4 fluxes increased with water depth, and this relationship was month-specific. The highest CH4 fluxes at the deepest points may seem contradictory to previous studies, in which the highest fluxes were typically observed in littoral areas (e.g. DelSontro et al., 2018b; Hofman et al., 2010; Natchimuthu et al., 2016; Schilder et al., 2013). However, these findings are based on studying mostly large, shallow to medium deep oligotrophic lakes where the morphology, trophic state or oxygen regime sharply contrast with the hyper-eutrophic Dehtář fishpond, where the upper 2 m of the water column were oxygen-saturated while the deepest strata were mostly anoxic, i.e. the extent and duration of bottom anoxia could be the most influential factor contributing to the highest methane fluxes at the deepest point of the pond. In hyper-eutrophic systems high nutrient loading increases autochthonous primary production (Potužák et al., 2007; Rutegwa et al., 2019) and promotes oxygen consumption and anaerobic decomposition in the sediments (Baxa et al., 2021), leading to enhanced CH4 production (Bastviken et al., 2004; Grasset et al., 2018). In aquaculture ponds in southeast China, CH4 fluxes exhibited considerable spatial variations and peaked in the relatively deep feeding zone, where the large loads of sediment organic matter fuelled CH4 production (Yang et al., 2020). Furthermore, sediment temperature was the strongest predictor of CH4 fluxes in shallow ponds with an anoxic hypolimnion (DelSontro et al., 2016; Yang et al., 2020). It is, therefore, reasonable to assume that both temperature and oxygen concentration in the sediment likely contributed to changes in observed CH4 fluxes during the studied period in our study. Although both parameters were not directly measured in the sediment, it can be deduced from their vertical profiles that the probability of sediment anoxia was highest in August and lowest in September, and the sediment temperature was lowest in September (see Fig. 5).
Our results agree with the generally accepted view that processes other than diffusive fluxes, most likely ebullition, represent the major CH4 pathway to the atmosphere in hyper-eutrophic ponds used for intensive fish production (Kosten et al., 2020). Although freshwater lakes with high primary production are more likely to have high CH4 ebullition rates (DelSontro et al., 2016), the dominant role of ebullition was also found across lentic systems differing in size, trophic status and geographical location (Aben et al., 2017). Ebullition accounted on average for 56 % of total CH4 fluxes in northern ponds in Canada (DelSontro et al., 2016), 49 % and 71 % in two different zones of Lake Taihu (Xiao et al., 2017) and 48–83 % in three Swedish lakes (Natchimuthu et al., 2016; Jansen et al., 2019). The highest contribution was found in the small hyper-eutrophic Priest Pot (UK), where ebullition represented 96 % of the total CH4 flux from the pond (Casper et al., 2000). Apparently, the contribution of ebullition can vary among systems and will remain uncertain until measurement designs cover enough spatiotemporal variability to yield representative values for the whole ecosystem.
In shallow water bodies, a semi-stable flux of microbubbles was suggested to account for a significant portion of the total CH4 flux (Prairie and del Giorgio, 2013). When the CH4 concentration in the water column is above a certain threshold of microbubble density, these microbubbles likely aggregate, fuse, and escape to the atmosphere due to buoyancy (Prairie and del Giorgio, 2013). Even a small fluctuation in hydrostatic pressure (e.g. due to changes in atmospheric pressure) or lake water level was shown to trigger enhanced CH4 ebullition (Bastviken et al., 2004; Casper et al., 2000; Varadharajan and Hemond, 2012). As ebullition rates increase exponentially with temperature, CH4 fluxes tend to peak in warm summer months (van Bergen et al., 2019). In our study, the 1 % lower air pressure in July and August than in September, along with bottom anoxia and higher water temperature, could account for the enhanced release of CH4 bubbles from the sediment (31.7 mmol m−2 d−1, >90 % of total CH4 fluxes; Fig. S2). In September, when we observed the lowest water temperatures from the studied period and the oxygen profile was relatively uniform, ebullition accounted for 81 % (11 mmol m−2 d−1) of the total CH4 fluxes. The spatially pooled data of the total CH4 fluxes measured in the Dehtář fishpond varied from 11.8 to 34.5 mmol m−2 d−1, which is comparable with similar systems elsewhere (e.g. Bastviken et al., 2010; van Bergen et al., 2019; Baron et al., 2022). To sum up, both diffusive fluxes and ebullition must be addressed to understand the magnitude of total aquatic CH4 fluxes and how their relative contributions vary across and within aquatic systems (Kosten et al., 2020). Moreover, with an improved determination of CH4 hot spots and the causes, the management of ponds could be changed accordingly and so the overall emissions reduced for example by decreasing P-availability and dredging (Nijman et al., 2022).
4.2 Effect of wind event on ecosystem spatial structure
Sudden changes in ecosystem spatial structure in response to meteorological forcing have rarely been documented (Loken et al., 2019) as they are hard to predict. Research into them using traditional methods requires intensive effort or expensive instrumentation (Ortiz and Wilkinson, 2021), and it remains a matter of luck to obtain a relevant dataset. In the July sampling campaign, we observed a strong impact of the wind on environmental heterogeneity in the fishpond, which was apparent at a sub-daily timescale. Due to the methodological constraints, i.e. lack of initial measurement, we can only speculate about the effect of wind on the total CH4 fluxes. The northwest wind during the day advected warmed surface water with cyanobacterial bloom from the north basin to the south. In the evening, it resulted in bloom accumulation on the upward side and a north–south gradient of more than 4 ∘C and 20 mg L−1 oxygen. After the winds dropped the observed gradients declined during cooling at night. We assume that the wind blowing across the pond surface drove buoyant cyanobacteria and surface water downwind and caused an upwelling of deeper, colder, and hypoxic water on the upwind side. This wind-related circulation pattern has been described as a “conveyer belt” in classical textbooks (Reynolds, 2006), held responsible for a disruption of the thermal structure of the water column and the non-uniform spatial distribution of pH, oxygen, CO2 or CH4 and also plankton assemblages (e.g. Loken et al., 2019; Natchimuthu et al., 2016; Rinke et al., 2009; Ortiz and Wilkinson, 2021).
Similar to our study, mild winds (∼4 m s−1) were strong enough to redistribute heat and induce lake-wide circulation driving upwelling and downwelling in the 24 m deep Lake Pleasant (Czikowsky et al., 2018). As the wind blows harder and lasts longer, the effects on ecosystem functioning may target higher trophic levels and become more complex (Rinke et al., 2009). In Lake Constance, a 3 d storm event with wind velocities of ∼10 m s−1 resulted in a lake-wide displacement of water masses and the formation of the 6–15 ∘C horizontal surface water gradient, which in turn changed the spatial distribution of phytoplankton, zooplankton and juvenile fish (Rinke et al., 2009). After several stormy days (wind velocities of 12–15 m s−1), Čech et al. (2011) observed negative effects of wind-driven changes in water temperature and wave action on perch (Perca fluviatilis) spawning in Lake Milada. Although wind events affect shallow and deep lakes differently, there is growing evidence that they can have far-reaching consequences on the functioning of aquatic ecosystems by disrupting energy flows, nutrient fluxes, productivity and reproduction, and consequently altering community composition and trophic interactions in the short and long terms (Stockwell et al., 2020). As the frequency, intensity, spatial extent and duration of these extreme meteorological events are projected to increase due to ongoing climate change (Comou and Rahmstorf, 2012), there is an urgent need for a better understanding of the mechanisms underlying their impacts on the maintenance of the ecosystem services.
4.3 Summer changes in the oxygen regime
Our data demonstrate that shallow, hyper-eutrophic ponds have disrupted oxygen regimes (Baxa et al., 2021) with anoxic hypolimnion and may experience severe whole-water column hypoxia critical for aquatic biota (Miranda et al., 2001). The hypoxic periods may result, for example, from sudden weather change (Jeppesen et al., 1990) and last several days, during which physical processes and phytoplankton photosynthesis cannot compensate for intense community respiration (Baxa et al., 2021). This became obvious in August when severe oxygen depletion was measured at the surface across the whole pond, mostly far below a critical level of 4.5 mg L−1, when adverse effects came into play (Banerjee et al., 2019). However, oxygen surface concentrations in shallow parts of the pond were substantially higher regardless of the time of day, which contrasts with findings of Miranda et al. (2001), who emphasised shallow waters as the most sensitive parts of lakes, where hypoxic events can occur due to the respiration of sediment biota. The observed spatial gradients of oxygen may create temporal refugia which allow fish to survive harsh conditions that occur in the deepest part of the pond. To minimise economic losses and negative impacts on the ecosystem, future research should identify the interplay between meteorological forcing, trophic status and anthropogenic pressures (e.g. management practices) that affect oxygen fluctuations at various timescales.
4.4 Study limitations
Like in other research, there are some limitations in the current study. As our measurement had only a limited temporal resolution (three samplings during the summer season), it is not appropriate to extrapolate CH4 emissions for annual values. Noticeably, future research must increase the frequency of the sampling and also include innovative techniques to measure CH4 fluxes at multiple fishponds, with different management regimes. In our study, the 12 h deployment time of the floating chambers could have led to extensive gas accumulation, which in turn might have resulted in an underestimation of the total CH4 fluxes due to the dissolution of the CH4 from the chamber into the water once the equilibrium concentration in the chamber is overcome (Bastviken et al., 2010). However, CH4 concentrations in water corresponded to a supersaturation of several orders of magnitude, so the introduced bias appears to be of minor importance. In any case, our daily spatially pooled total CH4 fluxes (11.8–34.5 mmol m−2 d−1) represent a rather conservative estimate for the global methane budget. In our study, we also did not address the important processes that could shed light on the lake CH4 budget, such as CH4 oxidation rates (Bastviken et al., 2008) or biological interaction (e.g. protistan grazing on CH4 oxidising bacteria) in aquatic food webs (Sanseverino et al., 2012) that can affect the overall CH4 fluxes. We also lack information about spatial differences in sediment microbiota and organic carbon content and compositions, which were found to affect CH4 production rates (Berberich et al., 2020; Emerson et al., 2021). Despite the limitation mentioned, our results show that complementary spatial surveys help contextualise the fixed station dynamics and provide additional, management-relevant information about the fishpond.
For improved monitoring strategies, however, a continuous measurement approach, such as eddy covariance would be generally more efficient than traditional sampling at regular intervals. Eddy covariance accounts for temporal variability and provides high temporal resolution data by continuously measuring wind speed, gas concentration, and vertical turbulent fluxes to estimate methane emissions (Erkkilä et al., 2018). More importantly, it also offers spatially integrated measurements, averaging emissions over a larger area and therefore accounts for pond spatial heterogeneity. However, it is worth noting that the choice of monitoring approach depends on various factors, including the specific objectives, available resources, and the characteristics of the emission sources. To accurately capture both short-term variability and lake spatial heterogeneity of methane ebullition and diffusion fluxes, the most efficient approach was found to be a combination of continuous measurements with traditional methods including floating chambers, anchored funnels and boundary model calculations (Schubert et al., 2012; Podgrajsek et al., 2014; Erkkilä et al., 2018). This integrated approach would provide a comprehensive understanding of methane emissions, enabling better estimation and more effective mitigation efforts.
Many fishponds are hundreds of years old (Potužák et al., 2007), and as such, they are an integral part of our cultural heritage. Nowadays, ponds face a variety of conflicting interests often leading to a focus on maximising fish production that comes at the expense of other ecological services. Intensification of fish production has brought a transition from the traditional management based on natural processes to practices involving supplementary feeding, fertilisation, and overstocking (Pechar, 2000). These changes coupled with the impacts of climate change has resulted in frequent anoxic events and cyanobacterial blooms that reduce biodiversity and limit the recreational activities increasingly valued by the public. Our study not only illustrates common water quality problems in fishponds but also provides compelling evidence that methane emissions in these degraded ecosystems further exacerbates negative climate feedbacks and should be considered in discussions to advance the development of sustainable management.
Deciphering the mechanisms that drive spatial and temporal heterogeneity in aquatic ecosystem structure and function not only expands our understanding of pond ecology but also provides insights into improving the management of these ecosystems and the services they provide. Our results suggest that spatial heterogeneity needs to be considered when designing experiments and monitoring programmes. Without the spatially resolved sampling, we introduce bias into our datasets, hampering our limnological understanding of the ecosystem's functioning and impeding our ability to accurately estimate rates such as methane emissions on a global scale (DelSontro et al., 2018a). In agreement with Kosten et al. (2020), we demonstrated that neglecting ebullition leads to a considerable underestimating of the total CH4 fluxes. As there are thousands of these intensively managed fishponds, we argue for changing the management practices toward sustainable use of natural resources to mitigate the overall emissions of greenhouse gases from these ecosystems. Future studies are needed to characterise CH4 fluxes over a greater number and diversity of aquaculture ponds and examine the mechanisms controlling CH4 emissions in aquatic ecosystems.
The dataset associated with the article can be found in Zenodo: https://doi.org/10.5281/zenodo.7524355 (Matousu, 2023).
The supplement related to this article is available online at: https://doi.org/10.5194/bg-20-4273-2023-supplement.
All authors contributed to the study conception and design. PZ planned the campaign; PZ, AM and JN performed the sampling and analysed the data; AM performed the gas measurements; VK performed statistical analyses and modelling; PZ and AM wrote the manuscript. All authors read and approved the final manuscript.
The contact author has declared that none of the authors has any competing interests.
Publisher's note: Copernicus Publications remains neutral with regard to jurisdictional claims made in the text, published maps, institutional affiliations, or any other geographical representation in this paper. While Copernicus Publications makes every effort to include appropriate place names, the final responsibility lies with the authors.
We thank Martin Rulík for providing the gas chambers. We especially thank Miloslav Šimek and Linda Jíšová for enabling gas analyses. We are grateful to Anna Sieczko for consultation on the calculation of CH4 fluxes. The English correction on an earlier version was done by Anton Baer.
This research has been supported by the Grantová Agentura České Republiky (grant nos. 17-09310S, 19-23261S, and P504/19-16554S).
This paper was edited by Andrew Thurber and reviewed by three anonymous referees.
Aben, R. C. H., Barros, N., van Donk, E., Frenken, T., Hilt, S., Kazanjian, G., Lamers, L. P. M., Peeters, E. T. H. M., Roelofs, J. G. M., de Senerpont Domis, L. N., Stephan, S., Velthuis, M., Van de Waal, D. B., Wik, M., Thornton, B. F., Wilkinson, J., DelSontro, T., and Kosten, S.: Cross continental increase in methane ebullition under climate change, Nat. Commun., 8, 1682, https://doi.org/10.1038/s41467-017-01535-y, 2017.
Banerjee, A., Chakrabarty, M., Rakshit, N., Bhowmick, A. R., and Ray, S.: Environmental factors as indicators of dissolved oxygen concentration and zooplankton abundance: deep learning versus traditional regression approach, Ecol. Indic., 100, 99–117, https://doi.org/10.1016/j.ecolind.2018.09.051, 2019.
Baron, A. A. P., Dyck, L. T., Amjad, H., Bragg, J., Kroft, E., Newson, J., Oleson, K., Casson, N. J., North, R. L., Venkiteswaran, J. J., and Whitfield, C. J.: Differences in ebullitive methane release from small, shallow ponds present challenges for scaling, Sci. Total Environ., 802, 149685, https://doi.org/10.1016/j.scitotenv.2021.149685, 2022.
Bartosiewicz, M., Maranger, R., Przytulska, A., and Laurion, I.: Effects of phytoplankton blooms on fluxes and emissions of greenhouse gases in a eutrophic lake, Water Res., 196, 116985, https://doi.org/10.1016/j.watres.2021.116985, 2021.
Bastviken, D., Cole, J., Pace, M., and Tranvik, L.: Methane emissions from lakes: Dependence of lake characteristics, two regional assessments, and a global estimate, Global Biogeochem. Cy., 18, GB4009, https://doi.org/10.1029/2004GB002238, 2004.
Bastviken, D., Cole, J. J., Pace, M. L., and Van de Bogert, M. C.: Fates of methane from different lake habitats: connecting whole-lake budgets and CH4 emissions, J. Geophys. Res.-Biogeo., 113, G02024, https://doi.org/10.1029/2007JG000608, 2008.
Bastviken, D., Santoro, A. L., Marotta, H., Pinho, L. Q., Calheiros, D. F., Crill, P., and Enrich-Prast, A.: Methane Emissions from Pantanal, South America, during the Low Water Season: Toward More Comprehensive Sampling, Environ. Sci. Tech., 44, 5450–5455, https://doi.org/10.1021/es1005048, 2010.
Bates, D., Maechler, M., Bolker, B., and Walker, S.: Fitting Linear Mixed-Effects Models Using lme4, J. Stat. Soft., 67, 1–48, https://doi.org/10.18637/jss.v067.i01, 2015.
Baxa, M., Musil, M., Kummel, M., Hazlík, O., Tesařová, B., and Pechar, L.: Dissolved oxygen deficits in a shallow eutrophic aquatic ecosystem (fishpond) – Sediment oxygen demand and water column respiration alternately drive the oxygen regime, Sci. Total Environ., 766, 142647, https://doi.org/10.1016/j.scitotenv.2020.142647, 2021.
Berberich, M. E., Beaulieu, J. J., Hamilton, T. L., Waldo, S., and Buffam, I.: Spatial variability of sediment methane production and methanogen communities within a eutrophic reservoir: Importance of organic matter source and quantity, Limnol. Oceanogr., 65, 1336–1358, https://doi.org/10.1002/lno.11392, 2020.
Bižić, M., Klintzsch, T., Ionescu, D., Hindiyeh, M. Y., Günthel, M., Muro-Pastor, A. M., Eckert, W., Urich, T., Keppler, F., and Grossart, H. P.: Aquatic and terrestrial cyanobacteria produce methane, Sci. Adv., 6, 1–10, https://doi.org/10.1126/sciadv.aax5343, 2020.
Bussmann, I., Matoušů, A., Osudar, R., and Mau, S.: Assessment of the radio 3H-CH4 tracer technique to measure aerobic methane oxidation in the water column, Limnol. Oceanogr.-Meth., 13, 312–327, https://doi.org/10.1002/lom3.10027, 2015.
Casper, P., Maberly, S. C., Hall, G. H., and Finlay, B. J.: Fluxes of methane and carbon dioxide from a small productive lake to the atmosphere, Biogeochemistry, 49, 1–19, https://doi.org/10.1023/A:1006269900174, 2000.
Čech, M., Peterka, J., Říha, M., Muška, M., Hejzlar, J., and Kubečka, J.: Location and timing of the deposition of eggs strands by perch (Perca fluviatilis L.): the roles of lake hydrology, spawning substrate and female size, Knowl. Manage. Aquat. Ecosyst., 403, 1–12, https://doi.org/10.1051/kmae/2011070, 2011.
Céréghino, R., Biggs, J., Oertli, B., and Declerck, S.: The ecology of European ponds: defining the characteristics of a neglected freshwater habitat, Hydrobiologia, 597, 1–6, https://doi.org/10.1007/s10750-007-9225-8, 2008.
Coumou, D. and Rahmstorf, S.: A decade of weather extreme, Nat. Clim. Change, 2, 491–496, https://doi.org/10.1038/nclimate1452, 2012.
Crusius, J. and Wanninkhof, R.: Gas transfer velocities measured at low wind speed over a lake, Limnol. Oceanogr., 48, 1010–1017, https://doi.org/10.4319/lo.2003.48.3.1010, 2003.
Czikowsky, M. J., MacIntyre, S., Tedford, E. W., Vidal, J., and Miller, S. D.: Effects of wind and buoyancy on carbon dioxide distribution and air-water flux of a stratified temperate lake, J. Geophys. Res.-Biogeo., 123, 2305–2322, https://doi.org/10.1029/2017JG004209, 2018.
DelSontro, T., Boutet, L., St-Pierre, A., del Giorgio, P. A., and Prairie, Y. T.: Methane ebullition and diffusion from northern ponds and lakes regulated by the interaction between temperature and system productivity, Limnol. Oceanogr., 61, 62–77, https://doi.org/10.1002/lno.10335, 2016.
DelSontro, T., Beaulieu, J. J., and Downing, J. J.: Greenhouse gas emissions from lakes and impoundments: upscaling in the face of global change, Limnol. Oceanogr. Lett., 3, 64–75, https://doi.org/10.1002/lol2.10073, 2018a.
DelSontro, T., del Giorgio, P. A., and Prairie, Y. T.: No Longer a Paradox: The Interaction Between Physical Transport and Biological Processes Explains the Spatial Distribution of Surface Water Methane Within and Across Lakes, Ecosystems, 21, 1073–1087, https://doi.org/10.1007/s10021-017-0205-1, 2018b.
De Meester, L., Declerck, S., Stoks, R., Louette, G., Van de Meutter, F., De Bie, T., Michels, E., and Brendonck, L.: Ponds and pools as model systems in conservation biology, ecology and evolutionary biology, Aquat. Cons., 15, 715–725, https://doi.org/10.1002/aqc.748, 2005.
D-maps.com: Map of Europe, https://d-maps.com/carte.php?num_car=2232& 105lang=en (last access: 18 September 2023), 2023a.
D-maps.com: Map of South Bohemian (Czech Republic), https://d-maps.com/carte.php?num_car=265046&lang=en (last access: 18 September 2023), 2023b.
Emerson, J. B., Varner, R. K., Wik, M., Parks, D. H., Neumann, R. B., Johnson, J. E., Singleton, C. M., Woodcroft, B. J., Tollerson II, R., Owusu-Dommey, A., Binder, M., Freitas, N. L., Crill, P. M., Saleska, S. R., Tyson, G. W., and Rich, V. I.: Diverse sediment microbiota shape methane emission temperature sensitivity in Arctic lakes, Nat. Commun., 12, 5815, https://doi.org/10.1038/s41467-021-25983-9, 2021.
Erkkilä, K.-M., Ojala, A., Bastviken, D., Biermann, T., Heiskanen, J. J., Lindroth, A., Peltola, O., Rantakari, M., Vesala, T., and Mammarella, I.: Methane and carbon dioxide fluxes over a lake: comparison between eddy covariance, floating chambers and boundary layer method, Biogeosciences, 15, 429–445, https://doi.org/10.5194/bg-15-429-2018, 2018.
Grasset, Ch., Mendonça, R,., Saucedo, G. V., Bastviken, D., Roland, F., and Sobek, S.: Large but variable methane production in anoxic freshwater sediment upon addition of allochthonous and autochthonous organic matter, Limnol. Oceanogr., 63, 1488–1501, https://doi.org/10.1002/lno.10786, 2018.
Halekoh, H. and Hojsgaard, S.: A Kenward-Roger Approximation and Parametric Bootstrap Methods for Tests in Linear Mixed Models – The R Package pbkrtest, J. Stat. Soft., 59, 1–30, https://doi.org/10.18637/jss.v059.i09, 2014.
Hofmann, H.: Spatiotemporal distribution patterns of dissolved methane in lakes: How accurate are the current estimations of the diffusive flux path?, Geophys. Res. Lett., 40, 2779–2784, https://doi.org/10.1002/grl.50453, 2013.
Hofmann, H., Federwisch, L., and Peeters, F.: Wave-induced release of methane: littoral zones as a source of methane in lakes. Limnol. Oceanogr., 55, 1990–2000, https://doi.org/10.4319/lo.2010.55.5.1990, 2010.
Hu, Z., Wu, S., Ji, Ch., Zou, J., Zhou, Q., and Liu, S.: A comparison of methane emissions following rice paddies conversion to crab-fish farming wetlands in southeast China, Environ. Sci. Pollut. Res., 23, 1505–1515, https://doi.org/10.1007/s11356-015-5383-9, 2016.
Jähne, B., Münnich, K. O., Bösinger, R., Dutzi, A., Huber, W., and Libner, P.: On the parameters influencing air-water gas exchange, J. Geophys. Res., 92, 1937–1949, https://doi.org/10.1029/JC092iC02p01937, 1987.
Jansen, J., Thornton, B. F., Jammet, M. M., Wik, M., Cortés, A., Friborg, T., MacIntyre, S., and Crill, P. M.: Climate-sensitive controls on large spring emissions of CH4 and CO2 from northern lakes, J. Geophys. Res.-Biogeo., 124, 2379–2399, https://doi.org/10.1029/2019JG005094, 2019.
Jeppesen, E., Søndergaard, M., Sortkjaer, O., Mortensen, E., and Kristensen, P.: Interactions between phytoplankton zooplankton and fish in a shallow hypertrophic Lake a study of phytoplankton collapses in Lake Sobygaard, Denmark, Hydrobiologia, 1991, 149–164, https://doi.org/10.1007/BF00026049, 1990.
Kolar, V., Vlašánek, P., and Boukal, D. S.: The influence of successional stage on local odonate communities in man-made standing waters, Ecol. Eng., 173, 106440, https://doi.org/10.1016/j.ecoleng.2021.106440, 2021.
Kopáček, J. and Hejzlar, J.: Semi-micro determination of total phosphorus in fresh waters with perchloric acid digestion, Int. J. Environ. Anal. Chem., 53, 173–183, https://doi.org/10.1080/03067319308045987, 1993.
Kopáček, J. and Procházková, L.: Semi-Micro Determination of Ammonia in Water by the Rubazoic Acid Method, Int. J. Environ. Anal. Chem., 53, 243–248, https://doi.org/10.1080/03067319308045993, 1993.
Kosten, S., Almeida, R. M., Barbosa, I., Mendonça, R., Muzitano, I. S., Oliveira-Junior, E. S., Vroom, R. J. E., Wang, H. J., and Barros, N.: Better assessments of greenhouse gas emissions from global fish ponds needed to adequately evaluate aquaculture footprint, Sci. Total Environ., 748, 141247, https://doi.org/10.1016/j.scitotenv.2020.141247, 2020.
Laas, A., Noges, P., Koiv, T., and Noges, T.: High-frequency metabolism study in a large and shallow temperate lake reveals seasonal switching between net autotrophy and net heterotrophy, Hydrobiologia, 694, 57–74, https://doi.org/10.1007/s10750-012-1131-z, 2012.
Loken, L. C., Crawford, J. T., Schramm, P. J., Stadler, P., Desai, A. R., and Stanley, E. H.: Large spatial and temporal variability of carbon dioxide and methane in a eutrophic lake, J. Geophys. Res.-Biogeo., 124, 2248–2266, https://doi.org/10.1029/2019JG005186, 2019.
Lüdecke, D.: ggeffects: Tidy Data Frames of Marginal Effects from Regression Models, J. Open Source Softw., 3, 772, https://doi.org/10.21105/joss.00772, 2018.
Lüdecke, D., Makowski, D., and Waggoner, P.: performance: Assessment of Regression Models Performance, R package version 0.4.4, CRAN, https://CRAN.R-project.org/package=performance (last access: 12 September 2023), 2020.
Ma, Y., Sun, L., Liu, C., Yang, X., Zhou, W., Yang, B., Schwenke, G., and Liu, D. L.: A comparison of methane and nitrous oxide emissions from inland mixed-fish and crab aquaculture ponds, Sci. Total Environ., 637–638, 517–523, https://doi.org/10.1016/j.scitotenv.2018.05.040, 2018.
Matousu, A.: Matousu/Znachor-at-al.-2023: v1.0.0 (v1.0), Zenodo [data set], https://doi.org/10.5281/zenodo.7524355, 2023.
McAuliffe, C.: Gas Chromatographic determination of solutes by multiple phase equilibrium, Chem. Technol., 1, 46–51, 1971.
Miranda, L. E., Hargreaves, J. A., and Raborn, S. W.: Predicting and managing risk of unsuitable dissolved oxygen in a eutrophic lake, Hydrobiologia, 457, 177–185, https://doi.org/10.1023/A:1012283603339, 2001.
Murphy, J. and Riley, J. P.: A modified single-solution method for the determination of phosphate in natural waters, Anal. Chim. Acta, 27, 31–36, https://doi.org/10.1016/S0003-2670(00)88444-5, 1962.
Natchimuthu, S., Sundgren, I., Gålfalk, M., Klemedtsson, L., Crill, P., Danielsson, Å., and Bastviken, D.: Spatio-temporal variability of lake CH4 fluxes and its influence on annual whole lake emission estimates, Limnol. Oceanogr., 61, 13–26, https://doi.org/10.1002/lno.10222, 2016.
Nijman, T. P. A., Lemmens, M., Lurling, M., Kosten, S., Welte, C., and Veraart, A.J.: Phosphorus control and dredging decrease methane emissions from shallow lakes, Sci. Total Environ., 847, 15758, https://doi.org/10.1016/j.scitotenv.2022.157584, 2022.
Ortiz, D. A. and Wilkinson, G. M.: Capturing the spatial variability of algal bloom development in a shallow temperate lake, Freshwater Biol., 66, 2064–2075, https://doi.org/10.1111/fwb.13814, 2021.
Pechar, L.: Impacts of long-term changes in fishery management on the trophic level and water quality in Czech fishponds, Fish. Manage. Ecol., 7, 23–32, https://doi.org/10.1046/j.1365-2400.2000.00193.x, 2000.
Podgrajsek, E., Sahlée, E., Bastviken, D., Holst, J., Lindroth, A., Tranvik, L., and Rutgersson, A.: Comparison of floating chamber and eddy covariance measurements of lake greenhouse gas fluxes, Biogeosciences, 11, 4225–4233, https://doi.org/10.5194/bg-11-4225-2014, 2014.
Pokorný, J. and Hauser, V.: The restoration of fish ponds in agricultural landscapes, Ecol. Eng., 18, 555–574, https://doi.org/10.1016/S0925-8574(02)00020-4, 2002.
Potužák, J., Hůda, J., and Pechar, L.: Changes in fish production effectivity in eutrophic fishponds – impact of zooplankton structure, Aquacult. Int., 15, 201–210, https://doi.org/10.1007/s10499-007-9085-2, 2007.
Potužák, J., Duras, J., and Drozd, B.: Mass balance of fishponds: are they sources or sinks of phosphorus?, Aquacult. Int., 24, 1725–1745, https://doi.org/10.1007/s10499-016-0071-4, 2016.
Prairie, Y. T. and del Giorgio, P. A.: A new pathway of freshwater methane emissions and the putative importance of microbubbles, Inland Waters, 3, 311–320, https://doi.org/10.5268/IW-3.3.542, 2013.
Procházková, L.: Bestimmung der Nitrate im Wasser, Z. Analyt. Chem., 167, 254–260, 1959.
Rasilo, T., Prairie, Y. T., and del Giorgio, P. A.: Large-scale patterns in summer diffusive CH4 fluxes across boreal lakes, and contribution to diffusive C emissions, Global Change Biol., 21, 1124–1139, https://doi.org/10.1111/gcb.12741, 2015.
R Core Team: A language and environment for statistical computing, R version 4.1.2, R Foundation for Statistical Computing, Vienna, Austria, https://www.R-project.org/ (last access: 1 November 2021), 2018.
Reynolds, C. S.: Ecology of phytoplankton, Cambridge University Press, Cambridge, https://doi.org/10.1017/CBO9780511542145, 2006.
Rinke, K., Huber, A. M. R., Kempke, S., Eder, M., Wolf, T., Probst, W. N., and Rothhaupt, K.: Lake-wide distributions of temperature, phytoplankton, zooplankton, and fish in the pelagic zone of a large lake, Limnol. Oceanogr., 54, 1306–1322, https://doi.org/10.4319/lo.2009.54.4.1306, 2009.
Rutegwa, M., Potužák, J., Hejzlar, J., and Drozd, B.: Carbon metabolism and nutrient balance in a hypereutrophic semi-intensive fishpond, Knowl. Manage. Aquat. Ecosyst., 420, 49, https://doi.org/10.1051/kmae/2019043, 2019.
Sanseverino, A. M., Bastviken, D., Sundh, I., Pickova, J., and Enrich-Prast, A.: Methane carbon supports aquatic food webs to the fish level, PLoS One, 7, e42723, hhttps://doi.org/10.1371/journal.pone.0042723, 2012.
Scheffer, M.: Ecology of shallow lakes, in: Population and Community Biology Series, Springer, p. 357, https://doi.org/10.1007/978-1-4020-3154-0, 2004.
Schilder, J., Bastviken, D., van Hardenbroek, M., Kankaala, P., Rinta, P., Stötter, T., and Heiri, O.: Spatial heterogeneity and lake morphology affect diffusive greenhouse gas emission estimates of lakes, Geophys. Res. Lett., 40, 5752–5756, https://doi.org/10.1002/2013GL057669, 2013.
Schmiedeskamp, M., Praetzel, L. S. E., Bastviken, D., and Knorr, K. H.: Whole-lake methane emissions from two temperate shallow lakes with fluctuating water levels: Relevance of spatiotemporal patterns, Limnol. Oceanogr., 66, 2455–2469, https://doi.org/10.1002/lno.11764, 2021.
Schubert, C. J., Diem, T., and Eugster, W.: Methane emissions from a small wind shielded lake determined by eddy covariance, flux chambers, anchored funnels, and boundary model calculations: a comparison, Environ. Sci. Technol., 46, 4515–4522, https://doi.org/10.1021/es203465x, 2012.
Stanley, E. H., Collins, S. M., Lottig, N. R., Oliver, S. K., Webster, K. E., Cheruvelil, K. S., and Soranno, P. A.: Biases in lake water quality sampling and implications for macroscale research, Limnol. Oceanogr., 64, 1572–1585, https://doi.org/10.1002/lno.11136, 2019.
Stockwell, J. D., Doubek, J. P., Adrian, R., Anneville, O., Carey, C. C., Carvalho, L., Domis, L. N. D. S., Dur, G., Frassl, M. A., Grossart, H.-P., Ibelings, B. W., Lajeunesse, M. J., Lewandowska, A. M., Llames, M. E., Matsuzaki, S.-I. S., Nodine, E. R., Nõges, P., Patil, V. P., Pomati, F., Rinke, K., Rudstam, L. G., Rusak, J. A., Salmaso, N., Seltmann, C. T., Straile, D., Thackeray, S. J., Thiery, W., Urrutia-Cordero, P., Venail, P., Verburg, P., Woolway, R. I., Zohary, T., Andersen, M. R., Bhattacharya, R., Hejzlar, J., Janatian, N., Kpodonu, A. T. N. K., Williamson, T. J., and Wilson, H. L.: Storm impacts on phytoplankton community dynamics in lakes, Global Change Biol., 26, 2756–2784, https://doi.org/10.1111/gcb.15033, 2020.
van Bergen, T. J. H. M., Barros, N., Mendonça, R., Aben, R. C. H., Althuizen, I. H. J., Huszar, V., Lamers, L. P. M., Lürling, M., Roland, F., and Kosten, S.: Seasonal and diel variation in greenhouse gas emissions from an urban pond and its major drivers, Limnol. Oceanogr., 64, 2129–2139, https://doi.org/10.1002/lno.11173, 2019.
Varadharajan, C. and Hemond, H. F.: Time-series analysis of high-resolution ebullition fluxes from a stratified, freshwater lake, J. Geophys. Res., 117, G02004, https://doi.org/10.1029/2011JG001866, 2012.
Wanninkhof, R.: Relationship between wind speed and gas exchange over the ocean revisited, Limnol. Oceanogr.-Meth., 12, 351–362, https://doi.org/10.4319/lom.2014.12.351, 2014.
Wiesenburg, D. A. and Guinasso, N. L.: Equilibrium solubilities of methane, carbon monoxide, and hydrogen in water and sea water, J. Chem. Eng. Data, 24, 356–360, https://doi.org/10.1021/je60083a006, 1979.
Wik, M., Varner, R. K., Anthony, K. W., MacIntyre, S., and Bastviken, D.: Climate-sensitive northern lakes and ponds are critical components of methane release, Nat. Geosci., 9, 99–105, https://doi.org/10.1038/ngeo2578, 2016.
Xiao, Q., Zhang, M., Hu, Z., Gao, Y., Hu, C., Liu, C., Liu, S., Zhang, Z., Zhao, J., Xiao, W., and Lee, X.: Spatial variations of methane emission in a large shallow eutrophic lake in subtropical climate, J. Geophys. Res.-Biogeo., 122, 1597–1614, https://doi.org/10.1002/2017JG003805, 2017.
Yamamoto, S., Alcauskas, J. B., and Crozier, T. E.: Solubility of methane in distilled water and seawater, J. Chem. Eng. Data, 21, 78–80, https://doi.org/10.1021/je60068a029, 1976.
Yan, X., Xu, X., Ji, M., Zhang, Z., Wang, M., Wu, S., Wang, G., Zhang, C., and Liu, H.: Cyanobacteria blooms: A neglected facilitator of CH4 production in eutrophic lakes, Sci. Total Environ., 651, 466–474, https://doi.org/10.1016/j.scitotenv.2018.09.197, 2019.
Yang, P., Zhang, Y., Yang, H., Zhang, Y., Xu, J., Tan, L., Tong, C., and Lai, D. Y.: Large fine-scale spatiotemporal variations of CH4 diffusive fluxes from shrimp aquaculture ponds affected by organic matter supply and aeration in Southeast China, J. Geophys. Res.-Biogeo., 124, 1290–1307, https://doi.org/10.1029/2019JG005025, 2019.
Yang, P., Zhang, Y., Yang, H., Guo, Q., Lai, D. Y. F., Zhao, G., Li, L., and Tong, C.: Ebullition was a major pathway of methane emissions from the aquaculture ponds in Southeast China, Water Res., 184, 116176, https://doi.org/10.1016/j.watres.2020.116176, 2020.
Yuan, J., Xiang, J., Liu, D. Y., Kang, H., He, T. H., Kim, S., Lin, Y. X., Freeman, C., and Ding, W. X.: Rapid growth in greenhouse gas emissions from the adoption of industrial-scale aquaculture, Nat. Clim. Change, 9, 318–322, https://doi.org/10.1038/s41558-019-0425-9, 2019.
Yuan, J., Liu, D., Xiang, J., He, T., Kang, H., and Ding, W.: Methane and nitrous oxide have separated production zones and distinct emission pathways in freshwater aquaculture ponds, Water Res., 190, 116739, https://doi.org/10.1016/j.watres.2020.116739, 2021.
Zar, J. H.: Biostatistical analysis, Prentice Hall, Inc., Englewood Cliffs, New York, p. 663, ISBN 9780130779250, ISBN 0130779253, 1984.
Zhang, L., Liao, Q., Gao, R., Luo, R., Liu, Ch., Zhong, J., and Wang, Z.: Spatial variations in diffusive methane fluxes and the role of eutrophication in a subtropical shallow lake, Sci. Total Environ., 759, 143495, https://doi.org/10.1016/j.scitotenv.2020.143495, 2021.
Zhao, J., Zhang, M., Xiao, W., Jia, L., Zhang, X., Wang, J., Zhang, Z., Xie, Y., Yini, P., Liu, S., Feng, Z., and Lee, X.: Large methane emission from freshwater aquaculture ponds revealed by long-term eddy covariance observation, Agr. Forest Meteorol., 308–309, 108600, https://doi.org/10.1016/j.agrformet.2021.108600, 2021.
Zhou, Y. Q., Zhou, L., Zhang, Y. L., de Souza, J. G., Podgorski, D. C., Spencer, R. G. M., Jeppesen, E., and Davidson, T. A.: Autochthonous dissolved organic matter potentially fuels methane ebullition from experimental lakes, Water Res., 166, 115048, https://doi.org/10.1016/j.watres.2019.115048, 2019.
Zuur, A. F., Ieno, E. N., Walker, N. J., Saveliev, A. A., and Smith, G. M.: Mixed effects models and extensions in ecology with R, Springer, New York, USA, p. 574, https://doi.org/10.1007/978-0-387-87458-6, 2009.